CINXE.COM
<!DOCTYPE html><html lang="en"><head><meta charSet="utf-8" data-next-head=""/><meta name="viewport" content="width=device-width" data-next-head=""/><title data-next-head="">How to get started in AI without excessive cost, or emissions!<!-- --> <!-- --> | Scaleway Blog</title><meta name="description" content="How can startups take their first steps with Large Language Models (LLMs)? Leveraging AI needn't cost the earth, explains MindMatch's Zofia Smoleń" data-next-head=""/><meta property="og:url" content="https://www.scaleway.com/en/blog/get-started-ai-cost-emissions-mindmatch/" data-next-head=""/><meta property="og:type" content="article" data-next-head=""/><meta property="og:title" content="How to get started in AI without excessive cost, or emissions!" data-next-head=""/><meta property="og:description" content="How can startups take their first steps with Large Language Models (LLMs)? Leveraging AI needn't cost the earth, explains MindMatch's Zofia Smoleń" data-next-head=""/><meta property="article:author" content="https://www.scaleway.com/en/blog/author/zofia-smolen" data-next-head=""/><meta property="og:image" content="https://www-uploads.scaleway.com/Automatic_Speech_Recognition_AI_Illustration_Blog_e8870a4451.webp" data-next-head=""/><meta content="https://www-uploads.scaleway.com/Automatic_Speech_Recognition_AI_Illustration_Blog_e8870a4451.webp" name="twitter:image" data-next-head=""/><meta content="summary" name="twitter:card" data-next-head=""/><meta content="@Scaleway" name="twitter:creator" data-next-head=""/><meta content="How to get started in AI without excessive cost, or emissions!" name="twitter:title" data-next-head=""/><meta content="How can startups take their first steps with Large Language Models (LLMs)? Leveraging AI needn't cost the earth, explains MindMatch's Zofia Smoleń" name="twitter:description" data-next-head=""/><link href="/favicon/blog/favicon.svg" type="image/svg+xml" rel="icon" data-next-head=""/><link href="/favicon/blog/favicon.ico" rel="icon" data-next-head=""/><link href="/favicon/blog/apple-touch-icon-180x180.png" rel="apple-touch-icon" sizes="180x180" data-next-head=""/><link href="/favicon/blog/apple-touch-icon-180x180.png" type="image/png" rel="shortcut icon" sizes="180x180" data-next-head=""/><link href="https://www.scaleway.com/en/blog/get-started-ai-cost-emissions-mindmatch/" rel="canonical" data-next-head=""/><link rel="preload" href="/_next/static/media/a34f9d1faa5f3315-s.p.woff2" as="font" type="font/woff2" crossorigin="anonymous" data-next-font="size-adjust"/><link rel="preload" href="/_next/static/media/2d141e1a38819612-s.p.woff2" as="font" type="font/woff2" crossorigin="anonymous" data-next-font="size-adjust"/><link rel="preload" href="/_next/static/css/167c96f3591d2921.css" as="style"/><link rel="stylesheet" href="/_next/static/css/167c96f3591d2921.css" data-n-g=""/><link rel="preload" href="/_next/static/css/0540dd5abe2c353c.css" as="style"/><link rel="stylesheet" href="/_next/static/css/0540dd5abe2c353c.css" data-n-p=""/><link rel="preload" href="/_next/static/css/92ffb8ebc71df939.css" as="style"/><link rel="stylesheet" href="/_next/static/css/92ffb8ebc71df939.css" data-n-p=""/><link rel="preload" href="/_next/static/css/8c86baaf62d4e650.css" as="style"/><link rel="stylesheet" href="/_next/static/css/8c86baaf62d4e650.css" data-n-p=""/><noscript data-n-css=""></noscript><script defer="" nomodule="" src="/_next/static/chunks/polyfills-42372ed130431b0a.js"></script><script src="/_next/static/chunks/webpack-6e462b92e6c8d3a0.js" defer=""></script><script src="/_next/static/chunks/framework-53ea874194e1abc4.js" defer=""></script><script src="/_next/static/chunks/main-89a27af27eefdb26.js" defer=""></script><script src="/_next/static/chunks/pages/_app-df5edb74c54ac48f.js" defer=""></script><script src="/_next/static/chunks/725-4cb47eb93e1704d7.js" defer=""></script><script src="/_next/static/chunks/192-12b8575b735ac4f4.js" defer=""></script><script src="/_next/static/chunks/943-f28b13fb2cb3c8c1.js" defer=""></script><script src="/_next/static/chunks/341-5be8fc4bc325b722.js" defer=""></script><script src="/_next/static/chunks/655-9ac0451386b6be56.js" defer=""></script><script src="/_next/static/chunks/564-9502a1580dab5ad9.js" defer=""></script><script src="/_next/static/chunks/pages/blog/%5Bslug%5D-2f4890f58654917f.js" defer=""></script><script src="/_next/static/4xZKwUKlhtIRe3nXE5xXw/_buildManifest.js" defer=""></script><script src="/_next/static/4xZKwUKlhtIRe3nXE5xXw/_ssgManifest.js" defer=""></script></head><body><div id="__next"><style data-emotion="css-global 0"></style><div class="__variable_375d66 __variable_f77ac8 container"><div class="blog"><header class="HeaderBlog_headerContainer__n3f6s full-width"><div class="container"><div class="HeaderBlog_header__CTV5V"><div class="HeaderBlog_logo__kbnMY"><a href="/en/blog/"><img alt="Scaleway Blog" loading="lazy" width="240" height="40" decoding="async" data-nimg="1" style="color:transparent" srcSet="/_next/static/media/logo-blog.49246fc4.svg 1x, /_next/static/media/logo-blog.49246fc4.svg 2x" src="/_next/static/media/logo-blog.49246fc4.svg"/></a><a href="#main" class="SkipLink_link__wUma3">Skip to main content</a><a href="#footer" class="SkipLink_link__wUma3">Skip to footer section</a><button class="HeaderBlog_menuButton__PP1O7" type="button"><style data-emotion="css 3sqif5">.css-3sqif5{vertical-align:middle;fill:currentColor;height:1em;width:1em;min-width:1em;min-height:1em;}.css-3sqif5 .fillStroke{stroke:currentColor;fill:none;}</style><svg viewBox="0 0 20 20" class="css-3sqif5 elxvigq0"><path fill-rule="evenodd" d="M2 4.75A.75.75 0 0 1 2.75 4h14.5a.75.75 0 0 1 0 1.5H2.75A.75.75 0 0 1 2 4.75M2 10a.75.75 0 0 1 .75-.75h14.5a.75.75 0 0 1 0 1.5H2.75A.75.75 0 0 1 2 10m0 5.25a.75.75 0 0 1 .75-.75h14.5a.75.75 0 0 1 0 1.5H2.75a.75.75 0 0 1-.75-.75" clip-rule="evenodd"></path></svg></button></div><nav class="HeaderBlog_topNav__cNrI_ font-body-small-regular"><ul class="HeaderBlog_links__1jfH4"><li><a href="/en/blog/incidents/">Incidents</a></li><li><a href="https://www.scaleway.com/en/docs/" class="cta-inline cta-size-big">Docs</a></li><li><a href="https://www.scaleway.com/en/contact/" class="cta-inline cta-size-big">Contact</a></li></ul><ul class="HeaderBlog_language__IixQV"><li><span class="sr-only">English</span><span>en</span></li></ul></nav><nav class="HeaderBlog_bottomNav__wIZob"><a class="cta-primary cta-size-small" href="/en/">Discover Scaleway</a><div class="HeaderBlog_socials__eZU_7"><a href="https://x.com/Scaleway/"><style data-emotion="css x3mert">.css-x3mert{vertical-align:middle;fill:currentColor;height:1.25rem;width:1.25rem;min-width:1.25rem;min-height:1.25rem;}.css-x3mert .fillStroke{stroke:currentColor;fill:none;}</style><svg viewBox="0 0 20 20" class="css-x3mert elxvigq0"><path d="M15.203 1.875h2.757l-6.023 6.883 7.085 9.367h-5.547l-4.345-5.68-4.972 5.68H1.4l6.442-7.363-6.797-8.887h5.688l3.928 5.193zm-.967 14.6h1.527L5.903 3.438H4.264z"></path></svg><span class="sr-only">X</span></a><a href="https://slack.scaleway.com/"><style data-emotion="css x3mert">.css-x3mert{vertical-align:middle;fill:currentColor;height:1.25rem;width:1.25rem;min-width:1.25rem;min-height:1.25rem;}.css-x3mert .fillStroke{stroke:currentColor;fill:none;}</style><svg viewBox="0 0 20 20" class="css-x3mert elxvigq0"><path fill-rule="evenodd" d="M6.056 3.419a1.75 1.75 0 0 0 1.75 1.751H9.39a.167.167 0 0 0 .167-.166V3.419a1.75 1.75 0 1 0-3.501 0m3.5 4.392a1.75 1.75 0 0 0-1.75-1.751H3.417a1.75 1.75 0 0 0-1.75 1.751 1.75 1.75 0 0 0 1.75 1.752h4.39a1.75 1.75 0 0 0 1.75-1.752m-6.123 6.142a1.75 1.75 0 0 0 1.75-1.752v-1.585a.167.167 0 0 0-.167-.166H3.433a1.75 1.75 0 0 0-1.75 1.751 1.75 1.75 0 0 0 1.75 1.752m4.376-3.503a1.75 1.75 0 0 0-1.75 1.751v4.38a1.75 1.75 0 1 0 3.5 0V12.2a1.75 1.75 0 0 0-1.75-1.751m7.01-2.639a1.75 1.75 0 1 1 3.501 0 1.75 1.75 0 0 1-1.75 1.752h-1.584a.167.167 0 0 1-.167-.167zm-.875 0a1.75 1.75 0 1 1-3.5 0V3.42a1.75 1.75 0 1 1 3.5 0zm0 8.77a1.75 1.75 0 0 0-1.75-1.752H10.61a.167.167 0 0 0-.167.167v1.585a1.75 1.75 0 1 0 3.501 0m-3.5-4.38a1.75 1.75 0 0 0 1.75 1.752h4.39a1.75 1.75 0 0 0 1.75-1.752 1.75 1.75 0 0 0-1.75-1.751h-4.39a1.75 1.75 0 0 0-1.75 1.751" clip-rule="evenodd"></path></svg><span class="sr-only">Slack</span></a><a href="/en/blog/rss.xml"><style data-emotion="css x3mert">.css-x3mert{vertical-align:middle;fill:currentColor;height:1.25rem;width:1.25rem;min-width:1.25rem;min-height:1.25rem;}.css-x3mert .fillStroke{stroke:currentColor;fill:none;}</style><svg viewBox="0 0 20 20" class="css-x3mert elxvigq0"><path d="M3.75 3a.75.75 0 0 0-.75.75v.5c0 .414.336.75.75.75H4c6.075 0 11 4.925 11 11v.25c0 .414.336.75.75.75h.5a.75.75 0 0 0 .75-.75V16C17 8.82 11.18 3 4 3z"></path><path d="M3 8.75A.75.75 0 0 1 3.75 8H4a8 8 0 0 1 8 8v.25a.75.75 0 0 1-.75.75h-.5a.75.75 0 0 1-.75-.75V16a6 6 0 0 0-6-6h-.25A.75.75 0 0 1 3 9.25zM7 15a2 2 0 1 1-4 0 2 2 0 0 1 4 0"></path></svg><span class="sr-only">RSS</span></a></div></nav></div></div></header><main class="main" id="main"><nav class="TopBar_navBar__jEc9M"><a class="TopBar_link__c_MXa" href="/en/blog/"><style data-emotion="css 3sqif5">.css-3sqif5{vertical-align:middle;fill:currentColor;height:1em;width:1em;min-width:1em;min-height:1em;}.css-3sqif5 .fillStroke{stroke:currentColor;fill:none;}</style><svg viewBox="0 0 20 20" class="css-3sqif5 elxvigq0"><path fill-rule="evenodd" d="M2 4.727A2.727 2.727 0 0 1 4.727 2h1.978a2.727 2.727 0 0 1 2.727 2.727v1.978a2.727 2.727 0 0 1-2.727 2.727H4.727A2.727 2.727 0 0 1 2 6.705zM4.727 3.5C4.05 3.5 3.5 4.05 3.5 4.727v1.978c0 .677.55 1.227 1.227 1.227h1.978c.677 0 1.227-.55 1.227-1.227V4.727c0-.678-.55-1.227-1.227-1.227zm5.841 1.227A2.727 2.727 0 0 1 13.296 2h1.977A2.727 2.727 0 0 1 18 4.727v1.978a2.727 2.727 0 0 1-2.727 2.727h-1.977a2.727 2.727 0 0 1-2.728-2.727zM13.296 3.5c-.678 0-1.228.55-1.228 1.227v1.978c0 .677.55 1.227 1.228 1.227h1.977c.678 0 1.227-.55 1.227-1.227V4.727c0-.678-.55-1.227-1.227-1.227zM2 13.296a2.727 2.727 0 0 1 2.727-2.728h1.978a2.727 2.727 0 0 1 2.727 2.728v1.977A2.727 2.727 0 0 1 6.705 18H4.727A2.727 2.727 0 0 1 2 15.273zm2.727-1.228c-.678 0-1.227.55-1.227 1.228v1.977c0 .678.55 1.227 1.227 1.227h1.978c.677 0 1.227-.55 1.227-1.227v-1.977c0-.678-.55-1.228-1.227-1.228zm5.841 1.228a2.727 2.727 0 0 1 2.728-2.728h1.977A2.727 2.727 0 0 1 18 13.296v1.977A2.727 2.727 0 0 1 15.273 18h-1.977a2.727 2.727 0 0 1-2.728-2.727zm2.728-1.228c-.678 0-1.228.55-1.228 1.228v1.977c0 .678.55 1.227 1.228 1.227h1.977c.678 0 1.227-.55 1.227-1.227v-1.977c0-.678-.55-1.228-1.227-1.228z" clip-rule="evenodd"></path></svg>all</a><a class="TopBar_link__c_MXa TopBar_isActive__bqGIp" href="/en/blog/build/">build</a><a class="TopBar_link__c_MXa" href="/en/blog/deploy/">deploy</a><a class="TopBar_link__c_MXa" href="/en/blog/scale/">scale</a></nav><section class="Hero_wrapper__l0O5u"><div class="Hero_content__WhyjP"><h1 class="font-heading-secondary-title Hero_title__64Z8x">How to get started in AI without excessive cost, or emissions! - MindMatch guest post</h1><div class="Hero_footer__KFZYB"><div class="blogCategory"><a class="cta-inline cta-size-big" href="/en/blog/build/">Build</a></div><span class="blogDot Hero_dot__OjyBJ" aria-hidden="true">•</span><address class="blogAuthor"><a class="cta-inline cta-size-big" href="/en/blog/author/zofia-smolen/">Zofia Smoleń</a></address><span class="blogDot Hero_dot__OjyBJ" aria-hidden="true">•</span><div><time dateTime="2024-02-26">26/02/24</time><span class="blogDot" aria-hidden="true">•</span><span>7 min read</span></div></div></div><div class="Hero_imageWrapper__tMCgD"><img alt="" loading="lazy" width="512" height="320" decoding="async" data-nimg="1" style="color:transparent" srcSet="https://scaleway.com/cdn-cgi/image/width=640/https://www-uploads.scaleway.com/Automatic_Speech_Recognition_AI_Illustration_Blog_e8870a4451.webp 1x, https://scaleway.com/cdn-cgi/image/width=1080/https://www-uploads.scaleway.com/Automatic_Speech_Recognition_AI_Illustration_Blog_e8870a4451.webp 2x" src="https://scaleway.com/cdn-cgi/image/width=1080/https://www-uploads.scaleway.com/Automatic_Speech_Recognition_AI_Illustration_Blog_e8870a4451.webp"/></div></section><div class="PostPage_post__sMook"><div class="TableOfContent_tableOfContent__e65l6"><nav aria-describedby="tableofcontent-main"><h2 class="font-body-small-bold">Table of contents</h2><ul><li class="TableOfContent_selected__VR351"><a href="#main">Introduction</a></li><li class=""><a href="#1-define-your-needs">1. Define your needs</a></li><li class=""><a href="#2-set-up-your-directory-so-that-you-can-easily-switch-between-different-models-and-architectures">2. Set up your directory so that you can easily switch between different models and architectures</a></li><li class=""><a href="#3-choose-the-right-deployment-model">3. Choose the right deployment model</a></li><li class=""><a href="#4-fit-the-model-to-your-purpose">4. Fit the model to your purpose</a></li><li class=""><a href="#5-run-it-on-the-right-machine">5. Run it on the right machine</a></li><li class=""><a href="#6-optimize-it-even-further-for-readers-with-technical-backgrounds">6. Optimize it even further (for readers with technical backgrounds)</a></li><li class=""><a href="#conclusion">Conclusion</a></li></ul></nav></div><div class="BlogRichText_blogRichText__zXeTD"><p><em>This a guest post by Zofia Smoleń, Founder of Polish startup <a href="https://mindmatch.pl/">MindMatch</a>, a member of Scaleway's <a href="https://www.scaleway.com/en/startup-program/">Startup Program</a> 🚀</em></p> <p>One of the greatest developments of recent years was making computers speak our language. Scientists have been working on language models (which are basically models predicting next sequence of letters) for some time already, but only recently they came up with models that actually work - Large Language Models (LLMs). The biggest issue with them is that they are… Large.</p> <p>LLMs have billions of parameters. In order to run them, you have to own quite a lot of computer power and use a significant amount of energy. For instance, OpenAI spends $700 000 daily on ChatGPT, and their model is highly optimized. For the rest of us, this kind of spending is neither good for your wallet, nor for the climate.</p> <p>So in order to limit your spending and carbon footprint, you cannot just use whatever OpenAI or even Hugging Face provides. You have to dedicate some time and thought to come up with more frugal methods of getting the job done. That is exactly what [Scaleway Startup Program member] MindMatch has been doing lately.</p> <p>MindMatch is providing a place where Polish patients can seek mental help from specialists. Using an open-source LLM from Hugging Face, MindMatch recognizes their patients’ precise needs based on a description of their feelings. With that knowledge, MindMatch can find the right therapy for their patients. It is a Polish-only website, but you can type in English (or any other language) and the chatbot (<a href="https://mindmatch.pl/chat">here</a>) will understand you and give you its recommendation. In this article, we wrap their thoughts on dealing with speed and memory problems in production.</p> <h2 id="1-define-your-needs">1. Define your needs</h2> <p>What do you need to do exactly? Do you need to reply to messages in a human-like manner? Or do you just need to classify your text? Is it only topic extraction?</p> <p>Read your bibliography. Check how people approached your task. Obviously, start from the latest papers, because in AI (and especially Natural Language Processing), all the work becomes obsolete and outdated very quickly. But… taking a quick look at what people did before Transformers (the state-of-the-art model architecture behind ChatGPT) can do no harm. Moreover, you may find solutions that resolve your task almost as well as any modern model would (if your task is comparatively easy) and are simpler, faster and lighter.</p> <p>You could start by simply looking at articles on Towards data science, but we also encourage you to browse through Google Scholar. A lot of work in data science is documented only in research papers so it actually makes sense to read them (as opposed to papers in social science).</p> <p>Why does this matter? You don’t need a costly ChatGPT-like solution just to tell you whether your patient is talking about depression or anxiety. Defining your needs and scouring the internet in search of all solutions applied so far might give you a better view on your options, and help select those that make sense in terms of performance and model size.</p> <h2 id="2-set-up-your-directory-so-that-you-can-easily-switch-between-different-models-and-architectures">2. Set up your directory so that you can easily switch between different models and architectures</h2> <p>This is probably the most obvious step for all developers, but make sure that you store all the models, classes and functions (and obviously constants - for example labels that you want to classify) in a way that allows you to quickly iterate, without needing to dig deep into code. This will make it easier for you, but also for all non-technical people that will want to understand and work on the model.</p> <p>What worked well for MindMatch was even storing all the dictionaries in an external database that was modifiable via Content Management Systems. One of those dictionaries was a list of classes used by the model. This way non-technical people were able to test the model. Obviously, to reduce the database costs, MindMatch had to make sure that they only pull those classes when necessary.</p> <p>Also, the right documentation will make it easier for you to use MLOps tools such as Mlflow. Even if it is just a prototype yet, it is better for you to prepare for the bright future of your product and further iterations.</p> <p>There is a lot of information and guidance about how to set the directory so that it is neat and tidy. Browse Medium and other portals until you find enough inspiration for your purpose.</p> <h2 id="3-choose-the-right-deployment-model">3. Choose the right deployment model</h2> <p>Now you’ve defined your needs, it’s time to choose the right solution. Since you want to use LLMs, you will most likely not even think about training your own model from scratch (unless you are a multi-billion company or a unicorn startup with high aspirations). So your options are limited to pre-trained models.</p> <p>For the pre-trained models, there are basically two options. You can either call them through an API and get results generated on an external computer instance (what OpenAI offers), or you can install the model on your computer and run it there as well (that is what Hugging Face offers, for example).</p> <p>The first option is usually more expensive, but that makes sense - you are using the computer power of another company, and it should come with a price. This way, you don’t have to worry about scalability. Usually, proprietary models like OpenAI’s work like that, so on top of that you also pay a fee for just using the model. But some companies producing open source models, like Mistral, also provide APIs.</p> <p>The second option (installing the model on your computer) comes only with open source models. So you don’t pay for the model itself, but you have to run it on your computer. This option is often chosen by companies who don’t want to be dependent on proprietary models and prefer to have more control over their solution. It comes with a cost: that of storage and computing power. It is pretty rare for organizations to own physical instances with memory sufficient for running LLM models, so most companies (like MindMatch) choose to use cloud services for that purpose.</p> <p>The choice between proprietary and open-source models depends on various factors, including the specific needs of the project, budget constraints, desired level of control and customization, and the importance of transparency and community support. In many cases it also depends on the level of domain knowledge within the organization. Proprietary models are usually easier to deploy.</p> <h2 id="4-fit-the-model-to-your-purpose">4. Fit the model to your purpose</h2> <p>The simpler the better. You should look for models that exactly match your needs. Assuming that you defined your needs already and did your research on Google Scholar, you should already know what solutions you are looking for. What now, then? Chances are, there are already at least a dozen of models that can solve your problem.</p> <p>We strongly advise you to have a look at Hugging Face’s “Models” section. Choose the model type; and then, starting from the most popular (it usually makes the most sense), try those models on your data. Pay particular attention to the accuracy and size of the model. The smaller the model is, the cheaper it is. As for accuracy, remember that your data is different from what the model was trained on. So if you want to use your solution for medical applications, you might want to try models that were trained on medical data.</p> <p>Also, remember that the pre-trained models are just language models. They don’t have any specialist knowledge. In fact, they rarely see any domain-specific words in training data. So don’t expect the model to talk easily about Euphyllophytes plants without any additional fine-tuning, Retrieval Augmented Generation (RAG) or at least prompt engineering. Any of those augmentations come with higher computing power cost.</p> <p>So you need to be smart about what exactly you make your model do. For example, when MindMatch tried to use zero-shot classification to recognize ADHD (a phrase rarely seen in training datasets), they decided to make it recognize Hyperactivity instead. Hyperactivity being a more frequent keyword that could easily act as a proxy for ADHD, allowed MindMatch to improve accuracy without deteriorating speed.</p> <h2 id="5-run-it-on-the-right-machine">5. Run it on the right machine</h2> <p>GPU or CPU? Many would assume that the answer lies simply between the speed and the price, as GPUs are generally more expensive and faster. That is usually true, but not always. Here are a few things to consider.</p> <h3 id="model-size-complexity-and-parallelisation">Model Size, Complexity and Parallelisation</h3> <p>Large and complex models, like GPT-4, benefit significantly from the processing power of GPUs, especially for tasks like training or running multiple instances simultaneously. GPUs have many more computing cores than CPUs, making them adept at parallel processing. This is particularly useful for the matrix and vector computations common in deep learning.<br/> But in order to start up GPU processing data must be transferred from RAM to GPU memory (GRAM), which can be costly. If the data is large and amenable to parallel processing, this overhead is offset by faster processing on the GPU.</p> <p>GPUs may not perform as well with tasks that require sequential processing, such as those involving Recurrent Neural Networks (RNNs) or Long Short-Term Memory (LSTM) networks (this applies to some implementations of Natural Language Processing). The sequential computation in LSTM layers, for instance, doesn't align well with the GPU's parallel processing capabilities, leading to underutilization (10% - 20% GPU load).</p> <p>Despite their limitations in sequential computation, GPUs can be highly effective during the backpropagation phase of LSTM, where derivative computations can be parallelized, leading to higher GPU utilization (around 80%).</p> <h3 id="inference-vs-training">Inference vs. Training</h3> <p>For training large models, GPUs are almost essential due to their speed and efficiency (not in all cases, as mentioned above). However, for inference (especially with smaller models or less frequent requests), <a href="https://www.scaleway.com/en/blog/why-cpus-also-make-sense-for-ai-inference/">CPUs can be sufficient and more cost-effective</a>. If you are using a pre-trained model (you most probably are), you only care about inference, so don’t assume that GPU will be better - compare it with CPUs.</p> <h3 id="scalability-budget-and-resources">Scalability, Budget and Resources</h3> <p>If you need to scale up your operations (e.g., serving a large number of requests simultaneously), GPUs offer better scalability options compared to CPUs.<br/> GPUs are more expensive and consume more power. If budget and resources are limited, starting with CPUs and then scaling up to GPUs as needed can be a practical approach.</p> <h2 id="6-optimize-it-even-further-for-readers-with-technical-backgrounds">6. Optimize it even further (for readers with technical backgrounds)</h2> <p>Are all of the above obvious to you? Here are other techniques (that often require you to dig a little deeper) that allow for optimized runtime and memory.</p> <h3 id="quantization">Quantization</h3> <p>Quantization is a technique used to optimize Large Language Models (LLMs) by reducing the precision of the model’s weights and activations. Typically, LLMs use 32 or 16 bits for each parameter, consuming significant memory. Quantization aims to represent these values with fewer bits, often as low as eight bits, without greatly sacrificing performance.</p> <p>The process involves two key steps: rounding and clipping. Rounding adjusts the values to fit into the lower bit format, while clipping manages the range of values to prevent extremes. This reduction in precision and range enables the model to operate in a more compact format, saving memory space.</p> <p>By quantizing a model, several benefits arise:</p> <ul> <li>Reduced Memory Footprint: The model occupies less space, allowing larger models to fit into the same hardware</li> <li>Enhanced Transfer Efficiency: It speeds up the model, especially in scenarios where bandwidth limits performance.</li> </ul> <p>However, quantizing LLMs comes with challenges:</p> <ul> <li>Quantizing weights is straightforward as they are fixed post-training. But quantizing activations (input of transformer blocks) is more complex due to their varying range and outliers</li> <li>In many GPUs, quantized weights (INT8) need to be converted back to higher precision (like FP16) for calculations, affecting efficiency</li> <li>Managing the dynamic range of activations is crucial, as they often contain outliers. Techniques like selective precision (using higher precision for certain activations) or borrowing the dynamic range from weights are used.</li> </ul> <h3 id="pruning">Pruning</h3> <p>Pruning involves identifying and removing parameters in a model that are either negligible or redundant. One common method of pruning is sparsity, where values close to zero are set to zero, leading to a more condensed matrix representation that only includes non-zero values and their indices. This approach reduces the overall space occupied by the matrix compared to a fully populated, dense matrix.</p> <p>Pruning can be categorized into two types:</p> <ul> <li>Structured Pruning: This method reduces the model's size by eliminating entire structural elements like neurons, channels, or layers. Structured pruning effectively decreases the model size while preserving the general structure of the Large Language Model (LLM). It is more scalable and manageable for larger models compared to unstructured pruning</li> <li>Unstructured Pruning: In this approach, individual weights or neurons are targeted independently, often by setting a threshold and zeroing out parameters that fall below it. It results in a sparser, irregular model structure that may require specialized handling. Unstructured pruning typically needs further fine-tuning or retraining to restore model accuracy. In large models with billions of parameters, this can become a complex and time-consuming process. To address this, techniques such as iterative fine-tuning, combining parameter-efficient tuning with pruning, and the implementation of SparseGPT are employed.</li> </ul> <p>SparseGPT, specifically, adopts a one-shot pruning strategy that bypasses the need for retraining. It approaches pruning as a sparse regression task, using an approximate solver that seeks a sufficiently good solution rather than an exact one. This approach significantly enhances the efficiency of SparseGPT.</p> <p>In practice, SparseGPT has been successful in achieving high levels of unstructured sparsity in large GPT models, such as OPT-175B and BLOOM-176B. It can attain over 60% sparsity - a higher rate than what is typically achieved with structured pruning - with only a minimal increase in perplexity, which measures the model's predictive accuracy.</p> <h3 id="distillation">Distillation</h3> <p>Distillation is a method of transferring knowledge from a larger model (teacher) to a smaller one (student). This is done by training the student model to mimic the teacher’s behavior, focusing on matching either the final layer outputs (logits) or intermediate layer activations. An example of this is DistilBERT, which retains most of BERT's capabilities but at a reduced size and increased speed. Distillation is especially useful when training data is scarce.<br/> However, be careful if you want to distill a model! Many state-of-the-art LLMs have restrictive licenses that prohibit using their outputs to train other LLMs. It is usually ok though, to use open-source models to train other LLMs.</p> <h3 id="model-serving-techniques">Model serving techniques</h3> <p>Model serving techniques aim to maximize the use of memory bandwidth during model execution. Key strategies include:</p> <ul> <li>In-flight Batching: Processing multiple requests simultaneously, continuously replacing finished sequences with new requests to optimize GPU utilization.</li> <li>Speculative Inference: Generating multiple future tokens based on a draft model, and then verifying or rejecting these predictions in parallel. This approach allows for faster text generation compared to the traditional token-by-token method.</li> </ul> <h2 id="conclusion">Conclusion</h2> <p>There are many ways to optimize model performance, leading not only to lower costs but also to less waste and lower carbon footprint. Start from a high-level definition of your needs, test different solutions and then dig into details, reducing the cost even further. MindMatch still is testing different options of reaching satisfying accuracy with lower computational costs - it is a never ending process.</p></div></div><section class="ExtraPosts_container__0fO7Q"><h2 class="font-heading-highlighted ExtraPosts_title__hqJSu">Recommended articles</h2><div class="ExtraPosts_articles__4oTri"><article class="RecommendedArticleCard_articleCard__L95dV"><div class="blogImage RecommendedArticleCard_img__lFn5u"><img alt="" loading="lazy" decoding="async" data-nimg="fill" style="position:absolute;height:100%;width:100%;left:0;top:0;right:0;bottom:0;color:transparent" sizes="100vw" srcSet="https://scaleway.com/cdn-cgi/image/width=640/https://www-uploads.scaleway.com/GPU_Instances_Card_fe6402712e.webp 640w, https://scaleway.com/cdn-cgi/image/width=750/https://www-uploads.scaleway.com/GPU_Instances_Card_fe6402712e.webp 750w, https://scaleway.com/cdn-cgi/image/width=828/https://www-uploads.scaleway.com/GPU_Instances_Card_fe6402712e.webp 828w, https://scaleway.com/cdn-cgi/image/width=1080/https://www-uploads.scaleway.com/GPU_Instances_Card_fe6402712e.webp 1080w, https://scaleway.com/cdn-cgi/image/width=1200/https://www-uploads.scaleway.com/GPU_Instances_Card_fe6402712e.webp 1200w, https://scaleway.com/cdn-cgi/image/width=1920/https://www-uploads.scaleway.com/GPU_Instances_Card_fe6402712e.webp 1920w, https://scaleway.com/cdn-cgi/image/width=2048/https://www-uploads.scaleway.com/GPU_Instances_Card_fe6402712e.webp 2048w, https://scaleway.com/cdn-cgi/image/width=3840/https://www-uploads.scaleway.com/GPU_Instances_Card_fe6402712e.webp 3840w" src="https://scaleway.com/cdn-cgi/image/width=3840/https://www-uploads.scaleway.com/GPU_Instances_Card_fe6402712e.webp"/></div><div class="RecommendedArticleCard_contentContainer__83Lgz"><h2 class="font-heading-title blogArticleTitle RecommendedArticleCard_heading___OIAO"><a class="breakout-link" href="/en/blog/ai-in-practice-generating-video-subtitles/">AI in practice: Generating video subtitles</a></h2><div class="RecommendedArticleCard_excerpt__Gsphk" role="doc-subtitle"><div class="RichText_scwRichtextStyle__xoOiq"><p class="font-body-regular">In this practical example, we roll up our sleeves and put Scaleway's H100 Instances to use by leveraging a couple of open source ML models to optimize our internal communication workflows.</p></div></div><div class="RecommendedArticleCard_footer__avFIY"><div class="blogCategory"><a href="/en/blog/build/">Build</a></div><span class="blogDot RecommendedArticleCard_dot__4FuRq" aria-hidden="true">•</span><address class="blogAuthor"><a href="/en/blog/author/diego-coy/">Diego Coy</a></address><span class="blogDot RecommendedArticleCard_dot__4FuRq" aria-hidden="true">•</span><div><time dateTime="2023-12-01">01/12/23</time><span class="blogDot" aria-hidden="true">•</span><span>5 min read</span></div></div><div aria-label="Tags list. Click to choose as filter." class="Tags_tags__UDbwl"><span class="Tag_tag__JS3kY">ai</span><span class="Tag_tag__JS3kY">H100</span></div></div></article></div><div class="ExtraPosts_articles__4oTri"><article class="RecommendedArticleCard_articleCard__L95dV"><div class="blogImage RecommendedArticleCard_img__lFn5u"><img alt="" loading="lazy" decoding="async" data-nimg="fill" style="position:absolute;height:100%;width:100%;left:0;top:0;right:0;bottom:0;color:transparent" sizes="100vw" srcSet="https://scaleway.com/cdn-cgi/image/width=640/https://www-uploads.scaleway.com/Computer_Vision_AI_Illustration_Blog_7f08d9c374.webp 640w, https://scaleway.com/cdn-cgi/image/width=750/https://www-uploads.scaleway.com/Computer_Vision_AI_Illustration_Blog_7f08d9c374.webp 750w, https://scaleway.com/cdn-cgi/image/width=828/https://www-uploads.scaleway.com/Computer_Vision_AI_Illustration_Blog_7f08d9c374.webp 828w, https://scaleway.com/cdn-cgi/image/width=1080/https://www-uploads.scaleway.com/Computer_Vision_AI_Illustration_Blog_7f08d9c374.webp 1080w, https://scaleway.com/cdn-cgi/image/width=1200/https://www-uploads.scaleway.com/Computer_Vision_AI_Illustration_Blog_7f08d9c374.webp 1200w, https://scaleway.com/cdn-cgi/image/width=1920/https://www-uploads.scaleway.com/Computer_Vision_AI_Illustration_Blog_7f08d9c374.webp 1920w, https://scaleway.com/cdn-cgi/image/width=2048/https://www-uploads.scaleway.com/Computer_Vision_AI_Illustration_Blog_7f08d9c374.webp 2048w, https://scaleway.com/cdn-cgi/image/width=3840/https://www-uploads.scaleway.com/Computer_Vision_AI_Illustration_Blog_7f08d9c374.webp 3840w" src="https://scaleway.com/cdn-cgi/image/width=3840/https://www-uploads.scaleway.com/Computer_Vision_AI_Illustration_Blog_7f08d9c374.webp"/></div><div class="RecommendedArticleCard_contentContainer__83Lgz"><h2 class="font-heading-title blogArticleTitle RecommendedArticleCard_heading___OIAO"><a class="breakout-link" href="/en/blog/how-sustainable-is-ai/">How Sustainable is AI?</a></h2><div class="RecommendedArticleCard_excerpt__Gsphk" role="doc-subtitle"><div class="RichText_scwRichtextStyle__xoOiq"><p class="font-body-regular">Do generative AI's benefits for the planet outweigh its impacts? Let's try to find out...</p></div></div><div class="RecommendedArticleCard_footer__avFIY"><div class="blogCategory"><a href="/en/blog/build/">Build</a></div><span class="blogDot RecommendedArticleCard_dot__4FuRq" aria-hidden="true">•</span><address class="blogAuthor"><a href="/en/blog/author/james-martin/">James Martin</a></address><span class="blogDot RecommendedArticleCard_dot__4FuRq" aria-hidden="true">•</span><div><time dateTime="2024-02-15">15/02/24</time><span class="blogDot" aria-hidden="true">•</span><span>6 min read</span></div></div><div aria-label="Tags list. Click to choose as filter." class="Tags_tags__UDbwl"><span class="Tag_tag__JS3kY">AI</span><span class="Tag_tag__JS3kY">Sustainability</span><span class="Tag_tag__JS3kY">Green IT</span></div></div></article></div><div class="ExtraPosts_articles__4oTri"><article class="RecommendedArticleCard_articleCard__L95dV"><div class="blogImage RecommendedArticleCard_img__lFn5u"><img alt="" loading="lazy" decoding="async" data-nimg="fill" style="position:absolute;height:100%;width:100%;left:0;top:0;right:0;bottom:0;color:transparent" sizes="100vw" srcSet="https://scaleway.com/cdn-cgi/image/width=640/https://www-uploads.scaleway.com/Nabu_Card_827fe79a9e.webp 640w, https://scaleway.com/cdn-cgi/image/width=750/https://www-uploads.scaleway.com/Nabu_Card_827fe79a9e.webp 750w, https://scaleway.com/cdn-cgi/image/width=828/https://www-uploads.scaleway.com/Nabu_Card_827fe79a9e.webp 828w, https://scaleway.com/cdn-cgi/image/width=1080/https://www-uploads.scaleway.com/Nabu_Card_827fe79a9e.webp 1080w, https://scaleway.com/cdn-cgi/image/width=1200/https://www-uploads.scaleway.com/Nabu_Card_827fe79a9e.webp 1200w, https://scaleway.com/cdn-cgi/image/width=1920/https://www-uploads.scaleway.com/Nabu_Card_827fe79a9e.webp 1920w, https://scaleway.com/cdn-cgi/image/width=2048/https://www-uploads.scaleway.com/Nabu_Card_827fe79a9e.webp 2048w, https://scaleway.com/cdn-cgi/image/width=3840/https://www-uploads.scaleway.com/Nabu_Card_827fe79a9e.webp 3840w" src="https://scaleway.com/cdn-cgi/image/width=3840/https://www-uploads.scaleway.com/Nabu_Card_827fe79a9e.webp"/></div><div class="RecommendedArticleCard_contentContainer__83Lgz"><h2 class="font-heading-title blogArticleTitle RecommendedArticleCard_heading___OIAO"><a class="breakout-link" href="/en/blog/infrastructures-for-llms-in-the-cloud/">Infrastructures for LLMs in the cloud</a></h2><div class="RecommendedArticleCard_excerpt__Gsphk" role="doc-subtitle"><div class="RichText_scwRichtextStyle__xoOiq"><p class="font-body-regular">What do you need to know before getting started with state-of-the-art AI hardware like NVIDIA's H100 PCIe 5, or even Scaleway's Jeroboam or Nabuchodonosor supercomputers? Look no further...</p></div></div><div class="RecommendedArticleCard_footer__avFIY"><div class="blogCategory"><a href="/en/blog/build/">Build</a></div><span class="blogDot RecommendedArticleCard_dot__4FuRq" aria-hidden="true">•</span><address class="blogAuthor"><a href="/en/blog/author/fabien-da-silva/">Fabien da Silva</a></address><span class="blogDot RecommendedArticleCard_dot__4FuRq" aria-hidden="true">•</span><div><time dateTime="2024-02-21">21/02/24</time><span class="blogDot" aria-hidden="true">•</span><span>6 min read</span></div></div><div aria-label="Tags list. Click to choose as filter." class="Tags_tags__UDbwl"><span class="Tag_tag__JS3kY">AI</span></div></div></article></div></section></main><footer id="footer" class="Footer_footer__dXXGl full-width"><div class="container"><div class="Footer_categories__GKzcP"><div><div class="Footer_title__SsUPi">Products</div><ul><li><a class="cta-inline cta-size-big" href="/en/all-products/">All Products</a></li><li><a class="cta-inline cta-size-big" href="/en/betas/">Betas</a></li><li><a class="cta-inline cta-size-big" href="/en/bare-metal/">Bare Metal</a></li><li><a class="cta-inline cta-size-big" href="/en/dedibox/">Dedibox</a></li><li><a class="cta-inline cta-size-big" href="/en/elastic-metal/">Elastic Metal</a></li><li><a class="cta-inline cta-size-big" href="/en/virtual-instances/">Compute Instances</a></li><li><a class="cta-inline cta-size-big" href="/en/gpu-instances/">GPU</a></li><li><a class="cta-inline cta-size-big" href="/en/containers/">Containers</a></li><li><a class="cta-inline cta-size-big" href="/en/object-storage/">Object Storage</a></li><li><a class="cta-inline cta-size-big" href="/en/block-storage/">Block Storage</a></li></ul></div><div><div class="Footer_title__SsUPi">Resources</div><ul><li><a href="https://www.scaleway.com/en/docs/" class="cta-inline cta-size-big">Documentation</a></li><li><a href="https://www.scaleway.com/en/docs/changelog/" class="cta-inline cta-size-big">Changelog</a></li><li><a class="cta-inline cta-size-big" href="https://www.scaleway.com/en/blog/">Blog</a></li><li><a href="https://feature-request.scaleway.com/" class="cta-inline cta-size-big">Feature Requests</a></li><li><a href="https://slack.scaleway.com/" class="cta-inline cta-size-big">Slack Community</a></li></ul></div><div><div class="Footer_title__SsUPi">Contact</div><ul><li><a href="https://console.scaleway.com/support/create/" class="cta-inline cta-size-big">Create a ticket</a></li><li><a href="https://console.scaleway.com/support/abuses/create/" class="cta-inline cta-size-big">Report Abuse</a></li><li><a href="https://status.scaleway.com/" class="cta-inline cta-size-big">Status</a></li><li><a href="https://console.online.net/fr/login" class="cta-inline cta-size-big">Dedibox Console online.net</a></li><li><a class="cta-inline cta-size-big" href="/en/assistance/">Support plans</a></li><li><a href="https://ultraviolet.scaleway.com/6dd9b5c45/p/62b4e2-ultraviolet" class="cta-inline cta-size-big">Brand resources</a></li></ul></div><div><div class="Footer_title__SsUPi">Company</div><ul><li><a class="cta-inline cta-size-big" href="/en/about-us/">About us</a></li><li><a class="cta-inline cta-size-big" href="/en/events/">Events</a></li><li><a href="https://www.scaleway.com/en/marketplace/" class="cta-inline cta-size-big">Marketplace</a></li><li><a class="cta-inline cta-size-big" href="/en/environmental-leadership/">Environment </a></li><li><a class="cta-inline cta-size-big" href="/en/social-responsibility/">Social Responsibility</a></li><li><a class="cta-inline cta-size-big" href="/en/security-and-resilience/">Security</a></li><li><a class="cta-inline cta-size-big" href="/en/shared-responsibility-model/">Shared Responsibility Model</a></li><li><a class="cta-inline cta-size-big" href="/en/news/">News</a></li><li><a class="cta-inline cta-size-big" href="/en/careers/">Careers</a></li><li><a class="cta-inline cta-size-big" href="/en/scaleway-learning/">Scaleway Learning</a></li><li><a class="cta-inline cta-size-big" href="/en/customer-testimonials/">Client Success Stories</a></li><li><style data-emotion="css je8g23">.css-je8g23{pointer-events:none;}</style><style data-emotion="css s7jpo2">.css-s7jpo2{background-color:transparent;border:none;padding:0;color:#34a8ff;-webkit-text-decoration:underline;text-decoration:underline;text-decoration-thickness:1px;text-underline-offset:2px;text-decoration-color:transparent;-webkit-transition:text-decoration-color 250ms ease-out;transition:text-decoration-color 250ms ease-out;gap:0.5rem;position:relative;cursor:pointer;width:-webkit-fit-content;width:-moz-fit-content;width:fit-content;font-size:1rem;font-family:Inter,Asap,sans-serif;font-weight:500;letter-spacing:0;line-height:1.5rem;paragraph-spacing:0;text-case:none;}.css-s7jpo2 .e1afnb7a2{-webkit-transition:-webkit-transform 250ms ease-out;transition:transform 250ms ease-out;}.css-s7jpo2 >*{pointer-events:none;}.css-s7jpo2:hover,.css-s7jpo2:focus{outline:none;-webkit-text-decoration:underline;text-decoration:underline;text-decoration-thickness:1px;color:#6fc2ff;text-decoration-color:#6fc2ff;}.css-s7jpo2:hover .e1afnb7a2,.css-s7jpo2:focus .e1afnb7a2{-webkit-transform:translate(-0.25rem, 0);-moz-transform:translate(-0.25rem, 0);-ms-transform:translate(-0.25rem, 0);transform:translate(-0.25rem, 0);}.css-s7jpo2[data-variant='inline']{-webkit-text-decoration:underline;text-decoration:underline;text-decoration-thickness:1px;}.css-s7jpo2:hover::after,.css-s7jpo2:focus::after{background-color:#34a8ff;}.css-s7jpo2:active{text-decoration-thickness:2px;}</style><a href="https://labs.scaleway.com/en/" target="_blank" rel="noopener noreferrer" class="css-s7jpo2 e1afnb7a0" variant="bodyStrong" data-variant="standalone">Labs<style data-emotion="css zpkqdi">.css-zpkqdi{display:-webkit-inline-box;display:-webkit-inline-flex;display:-ms-inline-flexbox;display:inline-flex;padding-bottom:0.25rem;}</style><span class="css-zpkqdi e1afnb7a1"><style data-emotion="css 1f2k2gl">.css-1f2k2gl{margin-left:0.5rem;}</style><style data-emotion="css 1jx3y9">.css-1jx3y9{vertical-align:middle;fill:currentColor;height:14px;width:14px;min-width:14px;min-height:14px;margin-left:0.5rem;}.css-1jx3y9 .fillStroke{stroke:currentColor;fill:none;}</style><svg xmlns="http://www.w3.org/2000/svg" viewBox="0 0 20 20" class="e1afnb7a2 css-1jx3y9 euz0z380"><path d="M6.22 8.72a.75.75 0 0 0 1.06 1.06l5.22-5.22v1.69a.75.75 0 0 0 1.5 0v-3.5a.75.75 0 0 0-.75-.75h-3.5a.75.75 0 0 0 0 1.5h1.69z"></path><path d="M3.5 6.75c0-.69.56-1.25 1.25-1.25H7A.75.75 0 0 0 7 4H4.75A2.75 2.75 0 0 0 2 6.75v4.5A2.75 2.75 0 0 0 4.75 14h4.5A2.75 2.75 0 0 0 12 11.25V9a.75.75 0 0 0-1.5 0v2.25c0 .69-.56 1.25-1.25 1.25h-4.5c-.69 0-1.25-.56-1.25-1.25z"></path></svg></span></a></li></ul></div></div><div class="Footer_socialsContainer__FuhFv"><a href="/en/"><img alt="Scaleway" loading="lazy" width="166" height="32" decoding="async" data-nimg="1" style="color:transparent" srcSet="/_next/static/media/logo.7e2996cb.svg 1x, /_next/static/media/logo.7e2996cb.svg 2x" src="/_next/static/media/logo.7e2996cb.svg"/></a><div><p>Follow us</p><a class="Footer_socialLink__9UK2B" href="https://x.com/Scaleway/"><style data-emotion="css x3mert">.css-x3mert{vertical-align:middle;fill:currentColor;height:1.25rem;width:1.25rem;min-width:1.25rem;min-height:1.25rem;}.css-x3mert .fillStroke{stroke:currentColor;fill:none;}</style><svg viewBox="0 0 20 20" class="css-x3mert elxvigq0"><path d="M15.203 1.875h2.757l-6.023 6.883 7.085 9.367h-5.547l-4.345-5.68-4.972 5.68H1.4l6.442-7.363-6.797-8.887h5.688l3.928 5.193zm-.967 14.6h1.527L5.903 3.438H4.264z"></path></svg><span class="sr-only">x</span></a><a class="Footer_socialLink__9UK2B" href="https://slack.scaleway.com/"><style data-emotion="css x3mert">.css-x3mert{vertical-align:middle;fill:currentColor;height:1.25rem;width:1.25rem;min-width:1.25rem;min-height:1.25rem;}.css-x3mert .fillStroke{stroke:currentColor;fill:none;}</style><svg viewBox="0 0 20 20" class="css-x3mert elxvigq0"><path fill-rule="evenodd" d="M6.056 3.419a1.75 1.75 0 0 0 1.75 1.751H9.39a.167.167 0 0 0 .167-.166V3.419a1.75 1.75 0 1 0-3.501 0m3.5 4.392a1.75 1.75 0 0 0-1.75-1.751H3.417a1.75 1.75 0 0 0-1.75 1.751 1.75 1.75 0 0 0 1.75 1.752h4.39a1.75 1.75 0 0 0 1.75-1.752m-6.123 6.142a1.75 1.75 0 0 0 1.75-1.752v-1.585a.167.167 0 0 0-.167-.166H3.433a1.75 1.75 0 0 0-1.75 1.751 1.75 1.75 0 0 0 1.75 1.752m4.376-3.503a1.75 1.75 0 0 0-1.75 1.751v4.38a1.75 1.75 0 1 0 3.5 0V12.2a1.75 1.75 0 0 0-1.75-1.751m7.01-2.639a1.75 1.75 0 1 1 3.501 0 1.75 1.75 0 0 1-1.75 1.752h-1.584a.167.167 0 0 1-.167-.167zm-.875 0a1.75 1.75 0 1 1-3.5 0V3.42a1.75 1.75 0 1 1 3.5 0zm0 8.77a1.75 1.75 0 0 0-1.75-1.752H10.61a.167.167 0 0 0-.167.167v1.585a1.75 1.75 0 1 0 3.501 0m-3.5-4.38a1.75 1.75 0 0 0 1.75 1.752h4.39a1.75 1.75 0 0 0 1.75-1.752 1.75 1.75 0 0 0-1.75-1.751h-4.39a1.75 1.75 0 0 0-1.75 1.751" clip-rule="evenodd"></path></svg><span class="sr-only">slack</span></a><a class="Footer_socialLink__9UK2B" href="https://www.instagram.com/scaleway/"><style data-emotion="css x3mert">.css-x3mert{vertical-align:middle;fill:currentColor;height:1.25rem;width:1.25rem;min-width:1.25rem;min-height:1.25rem;}.css-x3mert .fillStroke{stroke:currentColor;fill:none;}</style><svg viewBox="0 0 20 20" class="css-x3mert elxvigq0"><path fill-rule="evenodd" d="M1.667 9.719c0-2.848 0-4.272.563-5.356A5 5 0 0 1 4.362 2.23c1.084-.563 2.507-.563 5.355-.563h.566c2.848 0 4.272 0 5.355.563a5 5 0 0 1 2.132 2.133c.563 1.084.563 2.508.563 5.356v.566c0 2.848 0 4.272-.562 5.356a5 5 0 0 1-2.133 2.133c-1.083.563-2.507.563-5.355.563h-.566c-2.848 0-4.271 0-5.355-.563a5 5 0 0 1-2.132-2.133c-.563-1.084-.563-2.508-.563-5.356zm3.67.284a4.668 4.668 0 1 0 9.336 0 4.668 4.668 0 0 0-9.336 0m7.697 0a3.03 3.03 0 1 1-6.06 0 3.03 3.03 0 1 1 6.06 0m2.912-4.854a1.09 1.09 0 1 1-2.18 0 1.09 1.09 0 0 1 2.18 0" clip-rule="evenodd"></path></svg><span class="sr-only">instagram</span></a><a class="Footer_socialLink__9UK2B" href="https://www.linkedin.com/company/scaleway/"><style data-emotion="css x3mert">.css-x3mert{vertical-align:middle;fill:currentColor;height:1.25rem;width:1.25rem;min-width:1.25rem;min-height:1.25rem;}.css-x3mert .fillStroke{stroke:currentColor;fill:none;}</style><svg viewBox="0 0 20 20" class="css-x3mert elxvigq0"><path fill-rule="evenodd" d="M18.332 18.166a.167.167 0 0 1-.167.167h-3.09a.167.167 0 0 1-.167-.167V12.5c0-1.599-.608-2.492-1.874-2.492-1.377 0-2.096.93-2.096 2.492v5.666a.167.167 0 0 1-.167.167H7.804a.167.167 0 0 1-.166-.167V7.39c0-.092.074-.167.166-.167h2.967c.092 0 .167.075.167.167v.67c0 .174.275.26.39.131a3.88 3.88 0 0 1 2.96-1.307c2.357 0 4.044 1.439 4.044 4.415zM3.7 5.767a2.043 2.043 0 0 1-2.035-2.05c0-1.132.91-2.05 2.035-2.05s2.034.918 2.034 2.05-.91 2.05-2.034 2.05m-1.704 12.4c0 .091.074.166.166.166H5.27a.167.167 0 0 0 .167-.167V7.39a.167.167 0 0 0-.167-.167H2.163a.167.167 0 0 0-.166.167z" clip-rule="evenodd"></path></svg><span class="sr-only">linkedIn</span></a></div></div><ul class="Footer_sublinks__Mjpw0"><li><a href="/en/contracts/">Contracts</a></li><li><a href="/en/legal-notice/">Legal Notice</a></li><li><a href="/en/privacy-policy/">Privacy Policy</a></li><li><a href="/en/cookie/">Cookie</a></li><li><a href="https://security.scaleway.com">Security Measures</a></li><li><a hrefLang="fr" href="/fr/accessibility/">Accessibility statement</a></li></ul><span class="Footer_brand__qv1gM">© 1999-<!-- -->2025<!-- --> - Scaleway SAS</span></div></footer></div><div id="portal"></div></div></div><script id="__NEXT_DATA__" type="application/json">{"props":{"pageProps":{"post":{"id":430,"attributes":{"title":"get-started-AI-cost-emissions-mindmatch","path":"get-started-ai-cost-emissions-mindmatch/","description":"_This a guest post by Zofia Smoleń, Founder of Polish startup [MindMatch](https://mindmatch.pl/), a member of Scaleway's [Startup Program](https://www.scaleway.com/en/startup-program/) 🚀_\n\nOne of the greatest developments of recent years was making computers speak our language. Scientists have been working on language models (which are basically models predicting next sequence of letters) for some time already, but only recently they came up with models that actually work - Large Language Models (LLMs). The biggest issue with them is that they are… Large.\n\nLLMs have billions of parameters. In order to run them, you have to own quite a lot of computer power and use a significant amount of energy. For instance, OpenAI spends $700 000 daily on ChatGPT, and their model is highly optimized. For the rest of us, this kind of spending is neither good for your wallet, nor for the climate.\n\nSo in order to limit your spending and carbon footprint, you cannot just use whatever OpenAI or even Hugging Face provides. You have to dedicate some time and thought to come up with more frugal methods of getting the job done. That is exactly what [Scaleway Startup Program member] MindMatch has been doing lately.\n\nMindMatch is providing a place where Polish patients can seek mental help from specialists. Using an open-source LLM from Hugging Face, MindMatch recognizes their patients’ precise needs based on a description of their feelings. With that knowledge, MindMatch can find the right therapy for their patients. It is a Polish-only website, but you can type in English (or any other language) and the chatbot ([here](https://mindmatch.pl/chat)) will understand you and give you its recommendation. In this article, we wrap their thoughts on dealing with speed and memory problems in production.\n\n\n## 1. Define your needs\n\nWhat do you need to do exactly? Do you need to reply to messages in a human-like manner? Or do you just need to classify your text? Is it only topic extraction? \n\nRead your bibliography. Check how people approached your task. Obviously, start from the latest papers, because in AI (and especially Natural Language Processing), all the work becomes obsolete and outdated very quickly. But… taking a quick look at what people did before Transformers (the state-of-the-art model architecture behind ChatGPT) can do no harm. Moreover, you may find solutions that resolve your task almost as well as any modern model would (if your task is comparatively easy) and are simpler, faster and lighter.\n\nYou could start by simply looking at articles on Towards data science, but we also encourage you to browse through Google Scholar. A lot of work in data science is documented only in research papers so it actually makes sense to read them (as opposed to papers in social science).\n\nWhy does this matter? You don’t need a costly ChatGPT-like solution just to tell you whether your patient is talking about depression or anxiety. Defining your needs and scouring the internet in search of all solutions applied so far might give you a better view on your options, and help select those that make sense in terms of performance and model size.\n\n\n## 2. Set up your directory so that you can easily switch between different models and architectures\n\nThis is probably the most obvious step for all developers, but make sure that you store all the models, classes and functions (and obviously constants - for example labels that you want to classify) in a way that allows you to quickly iterate, without needing to dig deep into code. This will make it easier for you, but also for all non-technical people that will want to understand and work on the model. \n\nWhat worked well for MindMatch was even storing all the dictionaries in an external database that was modifiable via Content Management Systems. One of those dictionaries was a list of classes used by the model. This way non-technical people were able to test the model. Obviously, to reduce the database costs, MindMatch had to make sure that they only pull those classes when necessary.\n\nAlso, the right documentation will make it easier for you to use MLOps tools such as Mlflow. Even if it is just a prototype yet, it is better for you to prepare for the bright future of your product and further iterations.\n\nThere is a lot of information and guidance about how to set the directory so that it is neat and tidy. Browse Medium and other portals until you find enough inspiration for your purpose.\n\n\n## 3. Choose the right deployment model\n\nNow you’ve defined your needs, it’s time to choose the right solution. Since you want to use LLMs, you will most likely not even think about training your own model from scratch (unless you are a multi-billion company or a unicorn startup with high aspirations). So your options are limited to pre-trained models.\n\nFor the pre-trained models, there are basically two options. You can either call them through an API and get results generated on an external computer instance (what OpenAI offers), or you can install the model on your computer and run it there as well (that is what Hugging Face offers, for example).\n\nThe first option is usually more expensive, but that makes sense - you are using the computer power of another company, and it should come with a price. This way, you don’t have to worry about scalability. Usually, proprietary models like OpenAI’s work like that, so on top of that you also pay a fee for just using the model. But some companies producing open source models, like Mistral, also provide APIs. \n\nThe second option (installing the model on your computer) comes only with open source models. So you don’t pay for the model itself, but you have to run it on your computer. This option is often chosen by companies who don’t want to be dependent on proprietary models and prefer to have more control over their solution. It comes with a cost: that of storage and computing power. It is pretty rare for organizations to own physical instances with memory sufficient for running LLM models, so most companies (like MindMatch) choose to use cloud services for that purpose.\n\nThe choice between proprietary and open-source models depends on various factors, including the specific needs of the project, budget constraints, desired level of control and customization, and the importance of transparency and community support. In many cases it also depends on the level of domain knowledge within the organization. Proprietary models are usually easier to deploy.\n\n\n## 4. Fit the model to your purpose\n\nThe simpler the better. You should look for models that exactly match your needs. Assuming that you defined your needs already and did your research on Google Scholar, you should already know what solutions you are looking for. What now, then? Chances are, there are already at least a dozen of models that can solve your problem.\n\nWe strongly advise you to have a look at Hugging Face’s “Models” section. Choose the model type; and then, starting from the most popular (it usually makes the most sense), try those models on your data. Pay particular attention to the accuracy and size of the model. The smaller the model is, the cheaper it is. As for accuracy, remember that your data is different from what the model was trained on. So if you want to use your solution for medical applications, you might want to try models that were trained on medical data.\n\nAlso, remember that the pre-trained models are just language models. They don’t have any specialist knowledge. In fact, they rarely see any domain-specific words in training data. So don’t expect the model to talk easily about Euphyllophytes plants without any additional fine-tuning, Retrieval Augmented Generation (RAG) or at least prompt engineering. Any of those augmentations come with higher computing power cost.\n\nSo you need to be smart about what exactly you make your model do. For example, when MindMatch tried to use zero-shot classification to recognize ADHD (a phrase rarely seen in training datasets), they decided to make it recognize Hyperactivity instead. Hyperactivity being a more frequent keyword that could easily act as a proxy for ADHD, allowed MindMatch to improve accuracy without deteriorating speed.\n\n\n## 5. Run it on the right machine\n\nGPU or CPU? Many would assume that the answer lies simply between the speed and the price, as GPUs are generally more expensive and faster. That is usually true, but not always. Here are a few things to consider.\n\n\n### Model Size, Complexity and Parallelisation\n\nLarge and complex models, like GPT-4, benefit significantly from the processing power of GPUs, especially for tasks like training or running multiple instances simultaneously. GPUs have many more computing cores than CPUs, making them adept at parallel processing. This is particularly useful for the matrix and vector computations common in deep learning.\nBut in order to start up GPU processing data must be transferred from RAM to GPU memory (GRAM), which can be costly. If the data is large and amenable to parallel processing, this overhead is offset by faster processing on the GPU.\n\nGPUs may not perform as well with tasks that require sequential processing, such as those involving Recurrent Neural Networks (RNNs) or Long Short-Term Memory (LSTM) networks (this applies to some implementations of Natural Language Processing). The sequential computation in LSTM layers, for instance, doesn't align well with the GPU's parallel processing capabilities, leading to underutilization (10% - 20% GPU load).\n\nDespite their limitations in sequential computation, GPUs can be highly effective during the backpropagation phase of LSTM, where derivative computations can be parallelized, leading to higher GPU utilization (around 80%). \n\n\n### Inference vs. Training\n\nFor training large models, GPUs are almost essential due to their speed and efficiency (not in all cases, as mentioned above). However, for inference (especially with smaller models or less frequent requests), [CPUs can be sufficient and more cost-effective](https://www.scaleway.com/en/blog/why-cpus-also-make-sense-for-ai-inference/). If you are using a pre-trained model (you most probably are), you only care about inference, so don’t assume that GPU will be better - compare it with CPUs.\n\n\n### Scalability, Budget and Resources\n\nIf you need to scale up your operations (e.g., serving a large number of requests simultaneously), GPUs offer better scalability options compared to CPUs. \nGPUs are more expensive and consume more power. If budget and resources are limited, starting with CPUs and then scaling up to GPUs as needed can be a practical approach.\n\n\n## 6. Optimize it even further (for readers with technical backgrounds)\n\nAre all of the above obvious to you? Here are other techniques (that often require you to dig a little deeper) that allow for optimized runtime and memory.\n\n\n### Quantization\n\nQuantization is a technique used to optimize Large Language Models (LLMs) by reducing the precision of the model’s weights and activations. Typically, LLMs use 32 or 16 bits for each parameter, consuming significant memory. Quantization aims to represent these values with fewer bits, often as low as eight bits, without greatly sacrificing performance.\n\nThe process involves two key steps: rounding and clipping. Rounding adjusts the values to fit into the lower bit format, while clipping manages the range of values to prevent extremes. This reduction in precision and range enables the model to operate in a more compact format, saving memory space.\n\nBy quantizing a model, several benefits arise:\n- Reduced Memory Footprint: The model occupies less space, allowing larger models to fit into the same hardware\n- Enhanced Transfer Efficiency: It speeds up the model, especially in scenarios where bandwidth limits performance.\n\nHowever, quantizing LLMs comes with challenges:\n- Quantizing weights is straightforward as they are fixed post-training. But quantizing activations (input of transformer blocks) is more complex due to their varying range and outliers\n- In many GPUs, quantized weights (INT8) need to be converted back to higher precision (like FP16) for calculations, affecting efficiency\n- Managing the dynamic range of activations is crucial, as they often contain outliers. Techniques like selective precision (using higher precision for certain activations) or borrowing the dynamic range from weights are used.\n\n\n### Pruning\n\nPruning involves identifying and removing parameters in a model that are either negligible or redundant. One common method of pruning is sparsity, where values close to zero are set to zero, leading to a more condensed matrix representation that only includes non-zero values and their indices. This approach reduces the overall space occupied by the matrix compared to a fully populated, dense matrix.\n\nPruning can be categorized into two types:\n\n- Structured Pruning: This method reduces the model's size by eliminating entire structural elements like neurons, channels, or layers. Structured pruning effectively decreases the model size while preserving the general structure of the Large Language Model (LLM). It is more scalable and manageable for larger models compared to unstructured pruning\n- Unstructured Pruning: In this approach, individual weights or neurons are targeted independently, often by setting a threshold and zeroing out parameters that fall below it. It results in a sparser, irregular model structure that may require specialized handling. Unstructured pruning typically needs further fine-tuning or retraining to restore model accuracy. In large models with billions of parameters, this can become a complex and time-consuming process. To address this, techniques such as iterative fine-tuning, combining parameter-efficient tuning with pruning, and the implementation of SparseGPT are employed.\n\nSparseGPT, specifically, adopts a one-shot pruning strategy that bypasses the need for retraining. It approaches pruning as a sparse regression task, using an approximate solver that seeks a sufficiently good solution rather than an exact one. This approach significantly enhances the efficiency of SparseGPT.\n\nIn practice, SparseGPT has been successful in achieving high levels of unstructured sparsity in large GPT models, such as OPT-175B and BLOOM-176B. It can attain over 60% sparsity - a higher rate than what is typically achieved with structured pruning - with only a minimal increase in perplexity, which measures the model's predictive accuracy.\n\n\n### Distillation\n\nDistillation is a method of transferring knowledge from a larger model (teacher) to a smaller one (student). This is done by training the student model to mimic the teacher’s behavior, focusing on matching either the final layer outputs (logits) or intermediate layer activations. An example of this is DistilBERT, which retains most of BERT's capabilities but at a reduced size and increased speed. Distillation is especially useful when training data is scarce.\nHowever, be careful if you want to distill a model! Many state-of-the-art LLMs have restrictive licenses that prohibit using their outputs to train other LLMs. It is usually ok though, to use open-source models to train other LLMs.\n\n\n### Model serving techniques\n\nModel serving techniques aim to maximize the use of memory bandwidth during model execution. Key strategies include:\n- In-flight Batching: Processing multiple requests simultaneously, continuously replacing finished sequences with new requests to optimize GPU utilization.\n- Speculative Inference: Generating multiple future tokens based on a draft model, and then verifying or rejecting these predictions in parallel. This approach allows for faster text generation compared to the traditional token-by-token method.\n\n\n## Conclusion\n\nThere are many ways to optimize model performance, leading not only to lower costs but also to less waste and lower carbon footprint. Start from a high-level definition of your needs, test different solutions and then dig into details, reducing the cost even further. MindMatch still is testing different options of reaching satisfying accuracy with lower computational costs - it is a never ending process.\n","createdAt":"2024-02-26T14:20:53.327Z","updatedAt":"2024-02-26T14:25:12.462Z","publishedAt":"2024-02-26T14:25:12.395Z","locale":"en","tags":"AI\nStartups\nSustainability","popular":false,"articleOfTheMonth":false,"category":"Build","timeToRead":7,"excerpt":"How can startups take their first steps with Large Language Models (LLMs)? Leveraging AI needn't cost the earth, explains MindMatch's Zofia Smoleń","author":"Zofia Smoleń","h1":"How to get started in AI without excessive cost, or emissions! - MindMatch guest post","createdOn":"2024-02-26","image":{"data":{"id":3240,"attributes":{"name":"Automatic-Speech-Recognition-AI-Illustration-Blog.webp","alternativeText":null,"caption":null,"width":1216,"height":752,"formats":{"large":{"ext":".webp","url":"https://www-uploads.scaleway.com/large_Automatic_Speech_Recognition_AI_Illustration_Blog_e8870a4451.webp","hash":"large_Automatic_Speech_Recognition_AI_Illustration_Blog_e8870a4451","mime":"image/webp","name":"large_Automatic-Speech-Recognition-AI-Illustration-Blog.webp","path":null,"size":75.83,"width":1000,"height":618},"small":{"ext":".webp","url":"https://www-uploads.scaleway.com/small_Automatic_Speech_Recognition_AI_Illustration_Blog_e8870a4451.webp","hash":"small_Automatic_Speech_Recognition_AI_Illustration_Blog_e8870a4451","mime":"image/webp","name":"small_Automatic-Speech-Recognition-AI-Illustration-Blog.webp","path":null,"size":28.21,"width":500,"height":309},"medium":{"ext":".webp","url":"https://www-uploads.scaleway.com/medium_Automatic_Speech_Recognition_AI_Illustration_Blog_e8870a4451.webp","hash":"medium_Automatic_Speech_Recognition_AI_Illustration_Blog_e8870a4451","mime":"image/webp","name":"medium_Automatic-Speech-Recognition-AI-Illustration-Blog.webp","path":null,"size":51,"width":750,"height":464},"thumbnail":{"ext":".webp","url":"https://www-uploads.scaleway.com/thumbnail_Automatic_Speech_Recognition_AI_Illustration_Blog_e8870a4451.webp","hash":"thumbnail_Automatic_Speech_Recognition_AI_Illustration_Blog_e8870a4451","mime":"image/webp","name":"thumbnail_Automatic-Speech-Recognition-AI-Illustration-Blog.jpg","path":null,"size":8.66,"width":245,"height":152}},"hash":"Automatic_Speech_Recognition_AI_Illustration_Blog_e8870a4451","ext":".webp","mime":"image/webp","size":528.03,"url":"https://www-uploads.scaleway.com/Automatic_Speech_Recognition_AI_Illustration_Blog_e8870a4451.webp","previewUrl":null,"provider":"@website/strapi-provider-upload-scaleway-bucket","provider_metadata":null,"createdAt":"2024-02-15T13:43:21.303Z","updatedAt":"2024-02-26T14:23:17.313Z"}}},"recommendedArticles":{"data":[{"id":403,"attributes":{"title":"ai-in-practice-generating-video-subtitles","path":"ai-in-practice-generating-video-subtitles/","description":"Scaleway is a French company with an international vision, so it is imperative that we provide information to our 550+ employees in both English and French, to ensure clear understanding and information flow. We create a diverse set of training videos for internal usage, with some being originally voiced in English, and others in French. In all cases they should include subtitles for both languages.\n\nCreating subtitles is a time-consuming process that we quickly realized would not scale. Fortunately, we were able to harness the power of AI for this exact task. With the help of [OpenAI’s Whisper](https://github.com/openai/whisper), the University of [Helsinki’s Opus-MT](https://github.com/Helsinki-NLP/Opus-MT) and a bit of code, we were able to not only transcribe, and when required, translate our internal videos; but we could also generate subtitles in [the srt format](https://en.wikipedia.org/wiki/SubRip#:~:text=by%20that%20program.-,SubRip%20file%20format,-%5Bedit%5D), that we can simply import into a video editing software or feed to a video player.\n\n\n## OpenAI’s Whisper\n\nWhisper is an Open Source model created by OpenAI. It is a general-purpose speech recognition model that is able to identify and transcribe a wide variety of spoken languages. It is one of the most popular models around today and is released under MIT license.\n\nOpenAI provides a Python SDK that will interact with the model, which has a wide variety of “flavors” based on the accuracy of their results: tiny, base, small, medium, and large. Larger models have been trained with a greater amount of parameters or examples, which makes them larger in size, and more resource-hungry — the _tiny_ version of the model requires 1GB of VRAM (Video RAM) and the _large_ version requires around 10GB.\n\n\n## Helsinki-NLP’s Opus-MT\n\nThe University of Helsinki made its own Open Source text translation models available based on the Marian-MT framework used by Microsoft Translator. Opus-MT models are provided as language pairs: translation source, and translation target, meaning that the model Helsinki-NLP/opus-mt-fr-en will translate text in French (fr) to English (en), and the other way around with Helsinki-NLP/opus-mt-en-fr.\n\nOpus-MT can be used via the [Transformers Python library](https://huggingface.co/docs/transformers/index) from Hugging Face or using Docker. It is an Open Source project released under the MIT License and requires you to cite the OPUS-MT paper on your implementations:\n\n```\n@InProceedings{TiedemannThottingal:EAMT2020,\n author = {J{\\\"o}rg Tiedemann and Santhosh Thottingal},\n title = {{OPUS-MT} — {B}uilding open translation services for the {W}orld},\n booktitle = {Proceedings of the 22nd Annual Conferenec of the European Association for Machine Translation (EAMT)},\n year = {2020},\n address = {Lisbon, Portugal}\n }\n```\n\n## Generating subtitles\n\nCombining these two models into a subtitle-generating service is only a matter of adding some code to “glue” them together. But before diving into the code, let’s review our requirements:\n\nFirst, we need to create a Virtual Machine capable of running AI models without a hitch, and the [NVIDIA H100-1-80G GPU instance](https://www.scaleway.com/en/h100-pcie-try-it-now/) is a great choice.\n\nWith the type of instance clear, we can now focus on the functional requirements. We want to pass in a video file as input to Whisper to get a transcript. The second step will be to translate that transcript using OPUS-MT from a specific source language to a target language. Finally, we want to create a subtitle file in the target language that is in sync with the audio.\n\n\n### Setting up Whisper\n\nYou will find the latest information about setting it up on [their GitHub repository](https://github.com/openai/whisper), but in general, you can install the Python library using pip:\n\n```\npip install -U openai-whisper\n```\n\nWhisper relies heavily on the FFmpeg project for manipulating multimedia files. FFmpeg can be installed via APT:\n\n ```\n sudo apt install ffmpeg -y\n ```\n\n### The code\n\n\n#### 1. A simple text transcription\n\nThis basic example is the most straightforward way to transcribe audio into text. After importing the Whisper library, you load a _flavor_ of the model by passing [a string with its name](https://github.com/openai/whisper/#available-models-and-languages) to the load_model method. In this case, the _base_ model is accurate enough, but some use cases may require larger or smaller model flavors.\n\nAfter loading the model, you load the audio source by passing the file path. Notice that you can use both audio and video files, and in general, any file type with audio that is [supported by ](https://ffmpeg.org/ffmpeg-formats.html)FFmpeg.\n\nFinally, you make use of the transcribe method of the model by passing it the loaded audio. As a result, you get a dictionary that amongst other items, contains the whole transcription text.\n\n```python\n#main.py\n\nimport whisper\n\nmodel = whisper.load_model(\"base\")\naudio = whisper.load_audio(\"input_file.mp4\")\nresult = model.transcribe(audio)\n\nprint(result[\"text\"])\n```\n\nThis basic example gives you the main tools needed for the rest of the project: loading a model, loading an input audio file, and transcribing the audio using the model. This is already a big step forward and puts us closer to our goal of generating a subtitle file, however, you may have noticed that the resulting text doesn’t include any time references, it’s only text. Syncing this transcribed text with the audio would be a task that would require large amounts of manual work, but fortunately, Whisper’s transcription process also outputs _segments_ that are time-coded.\n\n\n#### 2. Segments\n\nHaving time-coded segments means you can pinpoint them to their specific start and end times during the clip. For instance, if the first speech segment in the clip is “We're no strangers” and it starts at 00:17:50 and ends at 00:18:30, you will get that information in the segment dictionary, giving you all you need to create an srt subtitle file, now all you have to do is to properly format it to conform with the appropriate syntax.\n\n```python\n#Getting the transcription segments\nfrom datetime import timedelta #For when getting the segment time\nimport os #For creating the srt file in the filesystem\nimport whisper\n\nmodel = whisper.load_model(\"base\")\naudio = whisper.load_audio(\"input_file.mp4\")\nresult = model.transcribe(audio)\n\nsegments = result[\"segments\"] #A list of segments\n\nfor segment in segments:\n\t#...\n```\n\n#### 3. An srt subtile file\n\nSubtitle files in the srt format are divided into sequences that include the start and end timecodes — separated by the “ --\u003e \" string — followed by the caption text ending in a line break. Here’s an example:\n\n```\n1\n00:01:26,612 --\u003e 00:01:29,376\nTook you long enough!\nDid you find it? where is it?.\n\n2\n00:01:39,101 --\u003e 00:01:42,609\nI did. But I wish I didn't.\n\n3\n00:02:16,339 --\u003e 00:02:18,169\nWhat are you talking about?\n```\n\nEach segment contains an ID field that can be used as the sequence number. The start and end times — the moments during which the subtitle is supposed to be on screen — can be obtained by padding the `timedelta` of each of the corresponding fields with zeroes (we’re keeping things simple here, but note that a more accurate subtitle syncing result have been achieved by projects such as [stable-ts](https://github.com/jianfch/stable-ts)). And the caption is the segment’s text. Here is the code that will generate each formatted subtitle sequence: \n\n```python\n#Getting segments transcription and formatting it as an srt subtitle\n\n#...\n\nfor segment in segments:\n\tstartTime = str(0)+str(timedelta(seconds=int(segment['start'])))+',000'\n\tendTime = str(0)+str(timedelta(seconds=int(segment['end'])))+',000'\n\ttext = segment['text']\n\n\tsubtitle_segment = f\"{segment['id'] + 1}\\n{startTime} --\u003e {endTime}\\n{ text }\\n\\n\"\n```\n\nAll that is left is to write each `subtitle_segment` to a new file:\n\n```python\n#Writting to the output subtitle file\n\twith open(\"subtitle.srt\", 'a', encoding='utf-8') as srtFile:\n \tsrtFile.write(subtitle_segment)\n```\n\nThe complete example code should look like this:\n\n```python\n#main.py\n\nfrom datetime import timedelta\nimport os\nimport whisper\n\nmodel = whisper.load_model(\"base\")\naudio = whisper.load_audio(\"input_file.mp4\")\nresult = model.transcribe(audio)\n\nsegments = result[\"segments\"]\n\nfor segment in segments:\n startTime = str(0)+str(timedelta(seconds=int(segment['start'])))+',000'\n endTime = str(0)+str(timedelta(seconds=int(segment['end'])))+',000'\n text = segment['text']\n\n subtitle_segment = f\"{segment['id'] + 1}\\n{startTime} --\u003e {endTime}\\n{ text }\\n\\n\"\n #Writting to the output subtitle file\n with open(\"subtitle.srt\", 'a', encoding='utf-8') as srtFile:\n \t srtFile.write(subtitle_segment)\n```\n\nNow to try it out you can download _this example file _— Or bring your own! —_ _with wget for instance:\n\n```sh\nwget https://scaleway.com/ai-book/examples/1/example.mp4 -O input_file.mp4\n```\n\nAnd then simply run the script:\n\n```sh\npython3 main.py\n```\n\nAfter only a few seconds — because you’re using [one of the fastest GPU instances on the planet](https://www.scaleway.com/en/h100-pcie-try-it-now/) —, the script will complete running and you will have a new `subtitle.srt` file that you can use during your video editing process or to load while playing the video file, great! But… the subtitle file is in the same language as the video. It is indeed useful as it is, but you probably want to reach a wider audience by translating it into different languages. We’ll explore that next.\n\n\n#### 4. Translating a segment’s text\n\nTranslating each segment’s text comes down to importing `MarianMTModel` and` MarianTokenizer` from Hugging Face’s Transformers library, passing the desired model name, and generating the translation. Install the dependencies by running the following command:\n\n```sh\npip install transformers SentencePiece\n```\n\nIn this example \"Helsinki-NLP/opus-mt-fr-en\" is used to translate from French to English. The `translate` abstracts the translation process by requiring a source string and returning a translated version of it.\n\n```python\nfrom transformers import MarianMTModel, MarianTokenizer\n# ...\n\nopus_mt_model_name = \"Helsinki-NLP/opus-mt-fr-en\"\ntokenizer = MarianTokenizer.from_pretrained(opus_mt_model_name)\nopus_mt_model = MarianMTModel.from_pretrained(opus_mt_model_name)\n\ndef translate(str):\n\ttranslated = opus_mt_model.generate(**tokenizer(str, return_tensors=\"pt\", padding=True))\n\tres = [tokenizer.decode(t, skip_special_tokens=True) for t in translated]\n\treturn res[0]\n```\n\nThere’s no need to worry about the `**tokenizer` function for now, just know that it receives the source string and some additional parameters that we can leave untouched.\n\nThe complete code example looks like this:\n\n```python\nfrom datetime import timedelta\nimport os\nimport whisper\nfrom transformers import MarianMTModel, MarianTokenizer\n\nmodel = whisper.load_model(\"base\")\naudio = whisper.load_audio(\"input_file.mp4\")\nresult = model.transcribe(audio)\n\nsegments = result[\"segments\"]\n\nopus_mt_model_name = \"Helsinki-NLP/opus-mt-fr-en\"\ntokenizer = MarianTokenizer.from_pretrained(opus_mt_model_name)\nopus_mt_model = MarianMTModel.from_pretrained(opus_mt_model_name)\n\ndef translate(str):\n\ttranslated = opus_mt_model.generate(**tokenizer(str, return_tensors=\"pt\", padding=True))\n\tres = [tokenizer.decode(t, skip_special_tokens=True) for t in translated]\n\treturn res[0]\n\nfor segment in segments:\n startTime = str(0)+str(timedelta(seconds=int(segment['start'])))+',000'\n endTime = str(0)+str(timedelta(seconds=int(segment['end'])))+',000'\n text = translate(segment['text'])\n\n\n subtitle_segment = f\"{segment['id'] + 1}\\n{startTime} --\u003e {endTime}\\n{ text }\\n\\n\"\n #Writting to the output subtitle file\n with open(\"subtitle.srt\", 'a', encoding='utf-8') as srtFile:\n \t srtFile.write(subtitle_segment)\n```\n\nThat’s it! Even though the results are not perfect, and you may need to make a few manual adjustments here and there, considering the rate at which AI is advancing, things can only get better in the time to come.\n\nYou can now extend and adapt this code to your own needs, how about making it dynamically accept a file path as an input parameter? Or what if you made it into a web service others can easily take advantage of? The choice is yours! just don’t forget to cite the OPUS-MT paper on your implementations if you’re using the translation feature.","createdAt":"2023-11-28T18:00:37.698Z","updatedAt":"2024-02-07T15:14:18.482Z","publishedAt":"2023-11-30T08:26:58.333Z","locale":"en","tags":"ai\nH100","popular":false,"articleOfTheMonth":false,"category":"Build","timeToRead":5,"excerpt":"In this practical example, we roll up our sleeves and put Scaleway's H100 Instances to use by leveraging a couple of open source ML models to optimize our internal communication workflows.","author":"Diego Coy","h1":"AI in practice: Generating video subtitles","createdOn":"2023-12-01","image":{"data":{"id":2944,"attributes":{"name":"GPU-Instances-Card.webp","alternativeText":null,"caption":null,"width":1216,"height":752,"formats":{"large":{"ext":".webp","url":"https://www-uploads.scaleway.com/large_GPU_Instances_Card_fe6402712e.webp","hash":"large_GPU_Instances_Card_fe6402712e","mime":"image/webp","name":"large_GPU-Instances-Card.webp","path":null,"size":"602.03","width":1000,"height":618},"small":{"ext":".webp","url":"https://www-uploads.scaleway.com/small_GPU_Instances_Card_fe6402712e.webp","hash":"small_GPU_Instances_Card_fe6402712e","mime":"image/webp","name":"small_GPU-Instances-Card.webp","path":null,"size":"145.28","width":500,"height":309},"medium":{"ext":".webp","url":"https://www-uploads.scaleway.com/medium_GPU_Instances_Card_fe6402712e.webp","hash":"medium_GPU_Instances_Card_fe6402712e","mime":"image/webp","name":"medium_GPU-Instances-Card.webp","path":null,"size":"335.71","width":750,"height":464},"thumbnail":{"ext":".webp","url":"https://www-uploads.scaleway.com/thumbnail_GPU_Instances_Card_fe6402712e.webp","hash":"thumbnail_GPU_Instances_Card_fe6402712e","mime":"image/webp","name":"thumbnail_GPU-Instances-Card.webp","path":null,"size":"35.69","width":245,"height":152}},"hash":"GPU_Instances_Card_fe6402712e","ext":".webp","mime":"image/webp","size":328.65,"url":"https://www-uploads.scaleway.com/GPU_Instances_Card_fe6402712e.webp","previewUrl":null,"provider":"@website/strapi-provider-upload-scaleway-bucket","provider_metadata":null,"createdAt":"2023-11-15T06:03:58.331Z","updatedAt":"2024-02-07T15:13:27.015Z"}}},"recommendedArticles":{"data":[{"id":401,"attributes":{"title":"best-quotes-ai-pulse-2023","path":"best-quotes-ai-pulse-2023/","description":"_The first edition AI conference ai-PULSE, at Station F, November 17, was one to be remembered. Artificial intelligence experts joined politicians and investors to shape Europe’s first concerted response to US and Chinese AI dominance. Here’s a first sweep of the most headline-worthy quotes, before we take a deeper dive into these subjects later on. Enjoy!_\n\n\n## Sovereignty, the key to tech’s latest global battle\n\n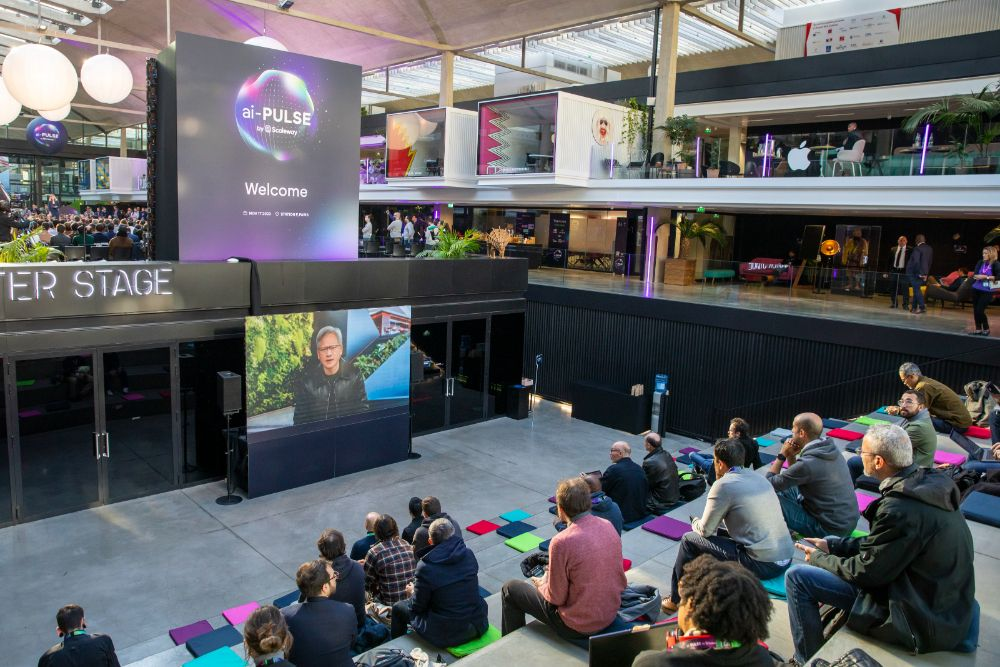\n\nIf speakers and visitors of ai-PULSE agreed on one thing, it was the need for French and European AI. Even **NVIDIA CEO Jensen Huang**, whose GPUs power the world’s leading AI systems, said “**Every country needs to build their sovereign AI that reflects their own language and culture**. Europe has some of the world’s biggest manufacturing companies. The second wave of AI is the expansion of generative AI all around the world.”\n\n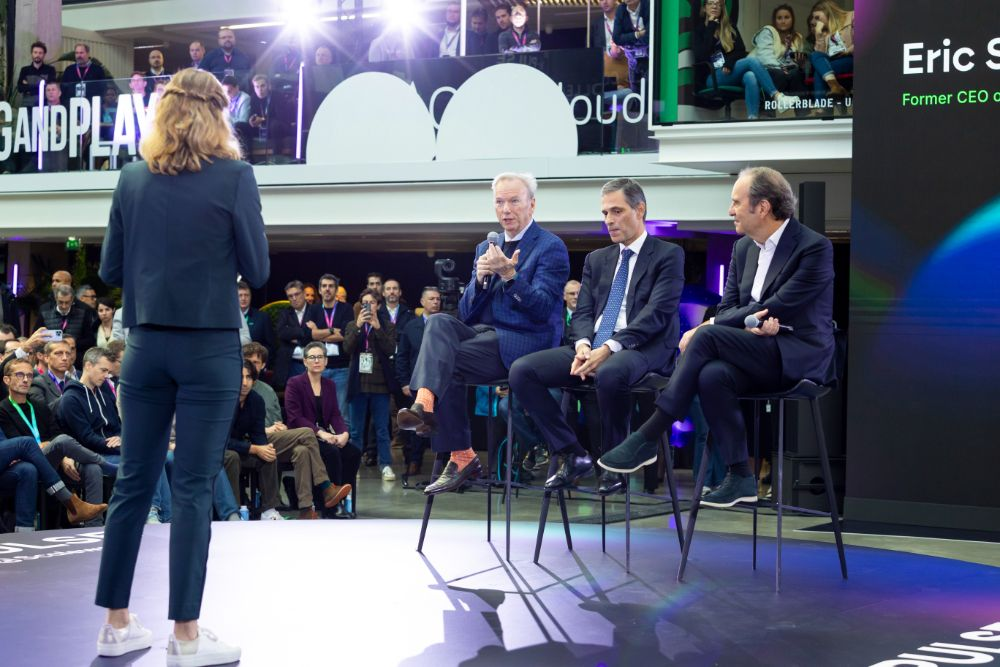\n\n**Eric Schmidt**, who has an equally global view as **former CEO of Google**, agreed: “**It’s obvious that France should be a leader in this domain**. These people have got the tech right. We don’t fully understand how to make profound learning happen. For that you need lots of smart people, and a lot of hardware, which somehow Xavier [Niel, Group iliad Founder] has managed to arrange. **So this is where AI is happening today.**”\n\n**Niel** himself went further when unveiling **Kyutai**, a new France-based AI research lab, a non-profit in which he, Schmidt and **CMA CGM CEO Rodolphe Saadé** have invested nearly €300 million. “**I’d like us to talk about French AI imperialism!**” enthused Niel in a mid-day press conference. “We want to create an ecosystem, like we did when we created 42, or Station F. **The idea is for the whole world to advance positively. We can change the game; and lead it**.”\n\n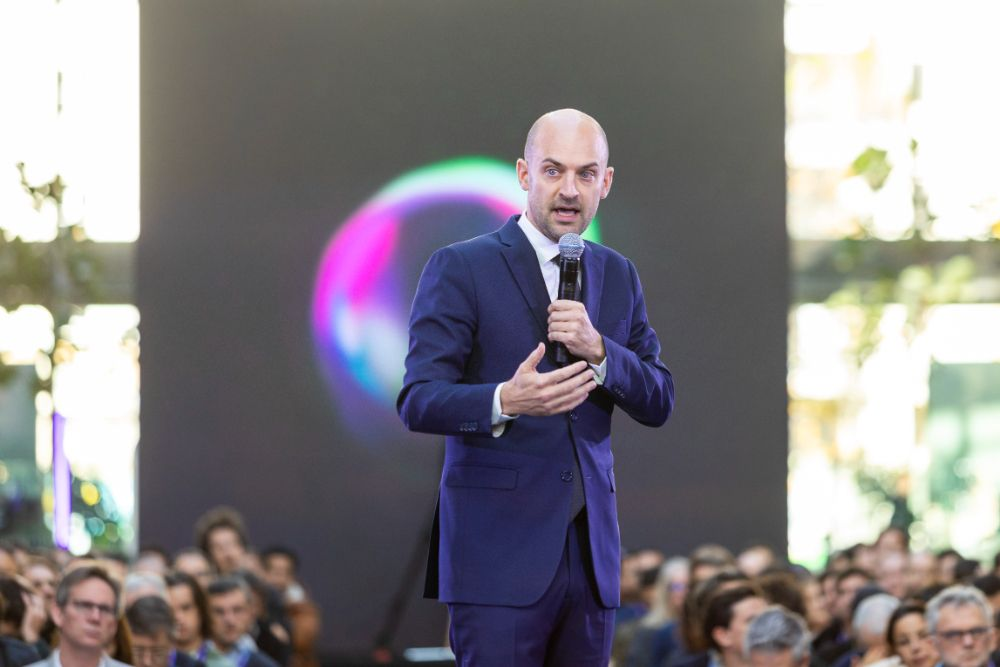\n\n**French digital minister Jean-Noël Barrot** was equally bullish about France’s AI prospects. “We may have lost some battles in the digital war, but we haven’t lost this one”, he said. “And **we have quite an army, as I can see here today: Mistral, and many more, will help France lead and win this war**.”\n\n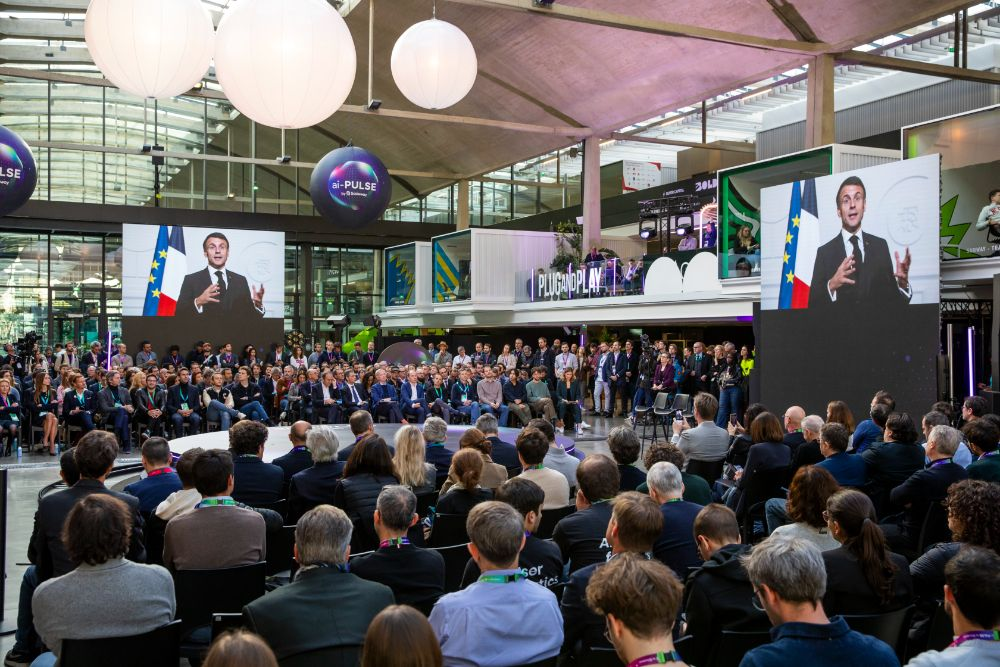\n\nThis enthusiasm was echoed by **French President Emmanuel Macron**, whose video address of ai-PULSE congratulated the decision to form Kyutai. “The commitment of the private sector alongside the public sector is absolutely key”, he said. “The fact you decided to invest at least €300 million in AI here, in order to educate, to keep, to train talents, to help, to increase our capacities, to increase our infrastructures, to be part of this game and to help France to be one of the key leaders in Europe is **a very important moment**. This initiative, and your conference, is not just to speak and to exchange views; it’s the **start of a conference where people put [invest] money and people start deciding**. And what I want us to do in months to come is precisely to follow up, and then to decide.”\n\n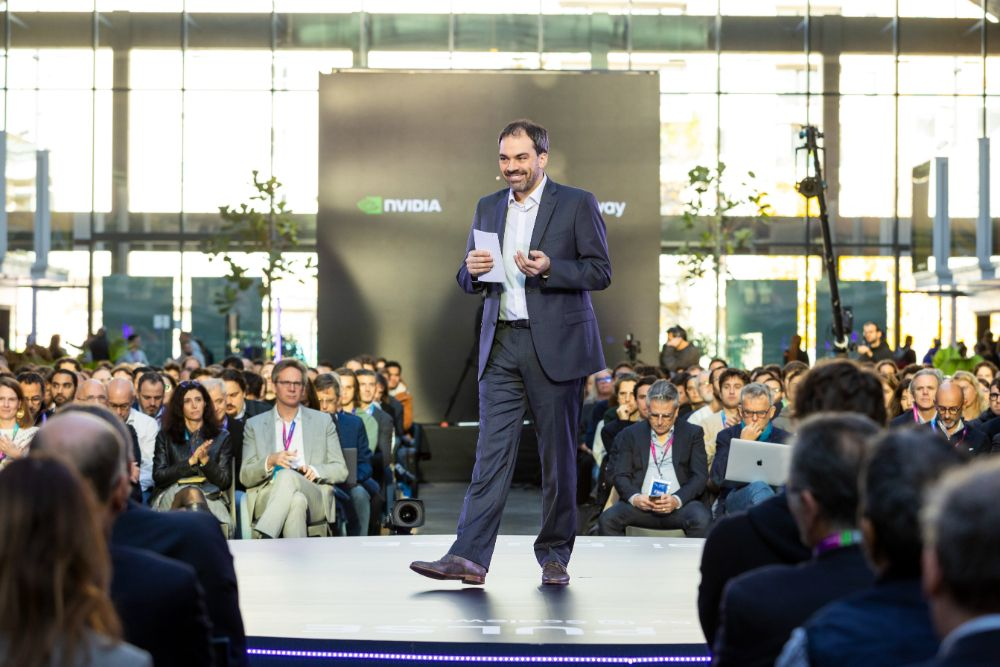\n\n**Sovereignty** has long been a key priority for _Scaleway_, so offering AI solutions within its data centers makes perfect sense, said the company’s **CEO Damien Lucas**, who earlier in the day announced his [company’s new range of AI products](https://www.scaleway.com/en/ai-pulse-new-products/) (above). “When I joined Scaleway, I heard lots of clients complain about only having access to American solutions\", he said in a press conference with Niel. \"**Why would people join Thales [S3NS] when it’s essentially rebranded Google?** It’s our responsibility to offer services of the same quality. Let’s work towards being a plausible alternative in the most possible cases.”\n\n\n## Open source - and science - or nothing\n\nWhat will be the _exception française_ of this new hexagonal revolution? Open source, _bien sûr_! Whilst AI leaders such as OpenAI, and some GAFAMs, are famously opaque about how their models are created, and refuse to explain their systems’ decisions, the European models in the spotlight at ai-PULSE were by definition open to all; i.e. free, and ready to copy and retrain at will, for the greater good of the sector.\n\n**Niel** was also emphatic on this point. “**These [Kyutai's] models will be available to everyone, even AWS!**” said the owner of cloud providers like Scaleway or Free Pro. All of Kyutai’s findings will be **published “in open science, which means the models’ source code will be made public. It’s something GAFAM is less and less tolerant of. Whereas we know that scientists need to publish**. There is no business objective or roadmap. Do we want our children using things that weren’t created in Europe? No. So how do we obtain things that suit us better? ChatGPT’s initial budget was €100m per year. But we’re going to benefit from open source on top of that.”\n\n**Huang** was very much aligned: “**I’m a big fan of open source**,” said NVIDIA’s leader. **Without it, how would AI have made the advances it has in recent years?** Open source’s ability to pull in the engagement of all types of companies keeps the ecosystem innovative… and safe, and responsible. It allows 100,000s of researchers to engage with (AI) innovation.” \n\nThis is precisely why **President Macron wants open source “to be a French force”**... and Barrot expressed his support for “models that are open, and so open source, as that stimulates innovation.”\n\n“**Open source is the way society moves fast**,” affirmed **Schmidt**. “Most of the platforms we use today are basically open source. My guess is the majority of companies here will build closed systems on top of open ones.\n\n\n## Resource optimisation: how to win the AI war\n\nThe majority of experts in ai-PULSE’s afternoon sessions are indeed working on open source solutions. One of their key recurring themes was **how to optimize AI models’ resource usage**. Because the winners in this race will be those that can do more with less. In other words, you don’t necessarily need a sports car to win this race. \n\n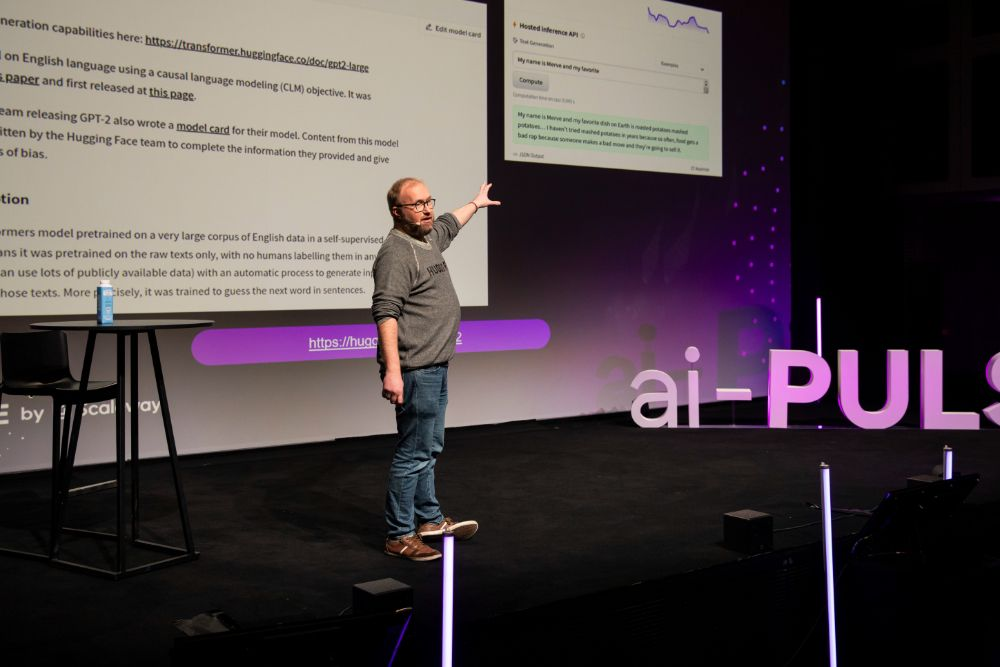\n\n“**You thought you were using a supercluster in a Ferrari, but in the end, you also divided its capacity to move several subjects forward at Dacia speed**. Proving that you learn by doing!\" said **Guillaume Salou**, ML Infra Lead at **Hugging Face**, one of the world’s most resource-aware AI companies, prior to his session on the importance of benchmarking large AI clusters (above).\n\n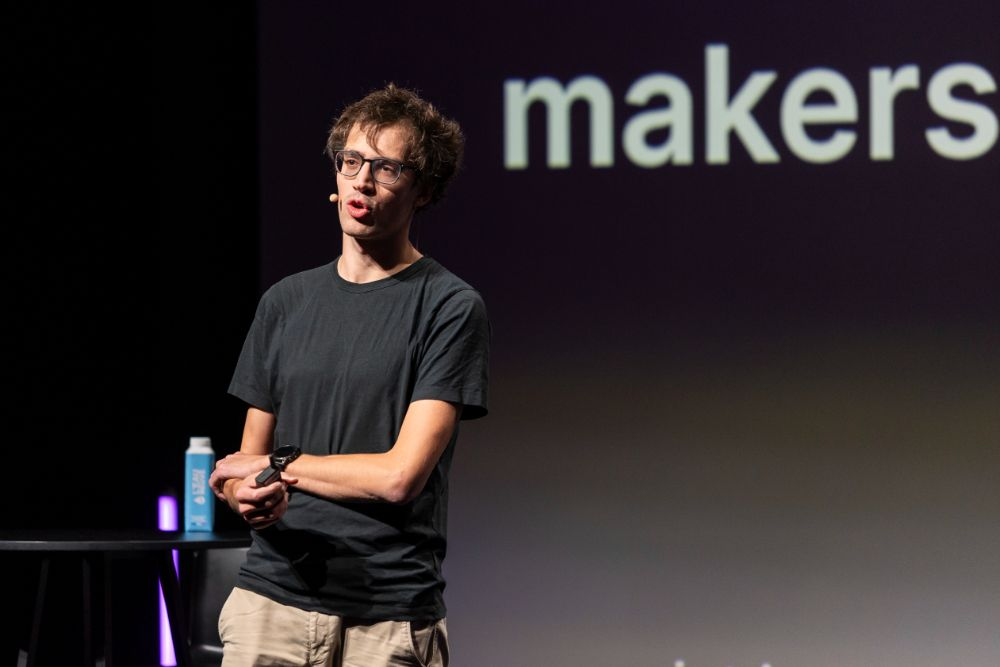\n\nFellow French AI star **Arthur Mensch**, CEO and Co-Founder of **Mistral** also insisted on the importance of resource optimization. “In the ‘Vanilla Attention’ version of Transformers, you need to keep the tokens in memory”, he explained. “**With Mistral AI’s ‘Sliding Window Attention’ model, there are four times less tokens in memory, reducing memory pressure and therefore saving money**. Currently, too much memory is used by generative AI”. This is notably why the company’s latest model, **Mistral-7B**, can run locally on a (recent) smartphone, proving massive resources aren’t always necessary for AI.\n\n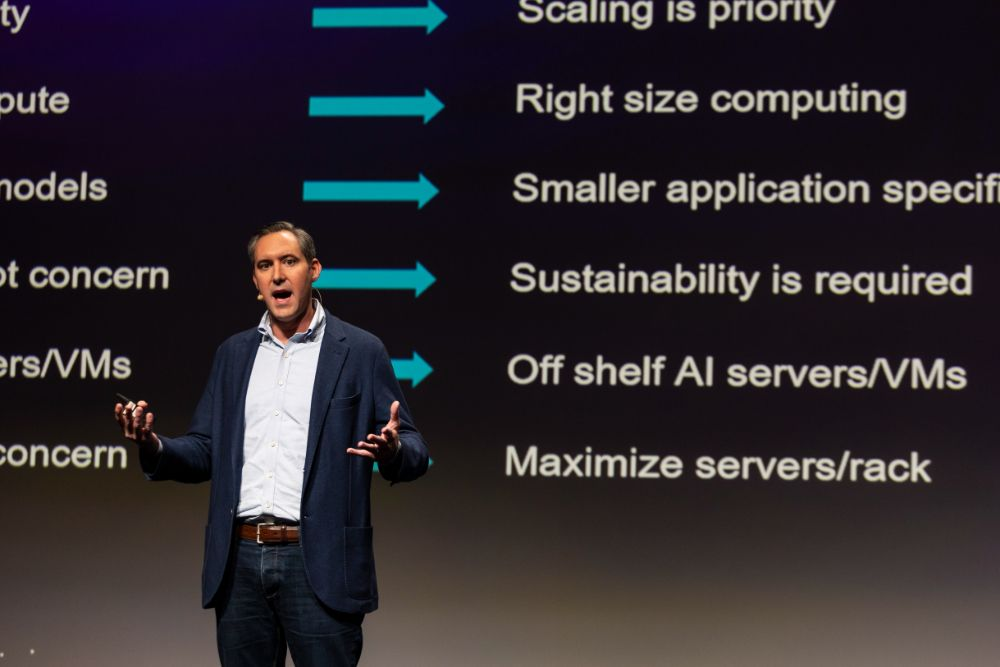\n\n**Jeff Wittich**, CPO of chipmaker **Ampere**, also shared that you don’t always need Ferrari-level GPUs for all AI work. Especially considering, as he pointed out, just **one NVIDIA DGX cluster uses 1% of France’s total renewable energy**… \n\n“Globally, 85% of AI computing is inference, versus 15% for training”, said Wittich. “So you need to right-size the AI compute to maximize cost effectiveness. **For smaller models or computer vision, a CPU-only server is often the right choice**. We’ve even seen amazing results, up into 7 to 10 billion-parameter models running on CPUs.” Major cloud energy and cost savings ensue, promised Wittich: Ampere client **Lampi**, for example, gained **10x speed performance results for one tenth of the cost of using a x86 processor**. More in our [interview with Wittich, here](https://www.scaleway.com/en/blog/why-cpus-also-make-sense-for-ai-inference/).\n\n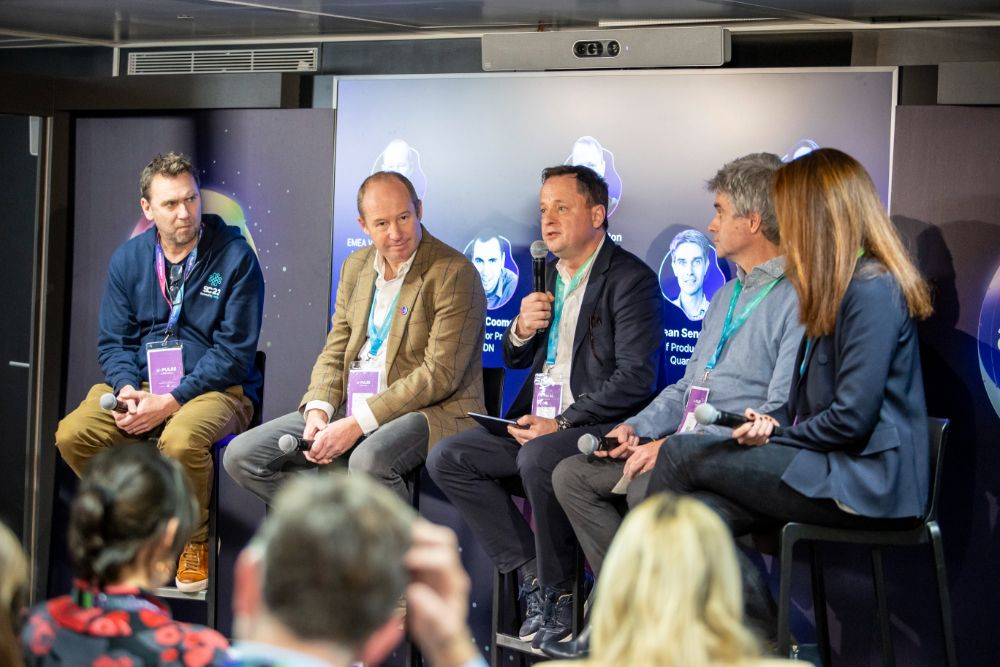\n\nBut what if the entire sector shifted to power-hungry models like GPT-3 or -4? This could lead to **AI consuming as much energy as Holland as early as 2027**, Scaleway COO **Albane Bruyas** pointed out on the “Next-Gen AI Hardware” panel (above), citing research by [Alex de Vries](https://www.sciencedirect.com/science/article/pii/S2542435123003653). “The worst thing you can do is have machines wasting power by being always on,” said **James Coomer**, Senior VP for Products, **DDN**. “[NVIDIA’s] Jensen Huang has the right idea. **We have to do accelerated computing, which means integrating across the whole stack so that the application is talking to the storage, the storage to the network**…”, i.e. the whole system constantly regulates itself to optimize its energy consumption. \n\nFood for thought…\n\n\n\n## A Taste of what’s to come\n\nWhich is all well and good: but what is this amazing technology really capable of today, and in the near future? Whilst **Kyutai**’s **Neil Zeghidour** said his organization’s objective was to “**create the next Transformers**” (the AI model now omnipresent today), **Poolside** gave a particularly enticing glimpse of a future where code could effectively write itself.\n\n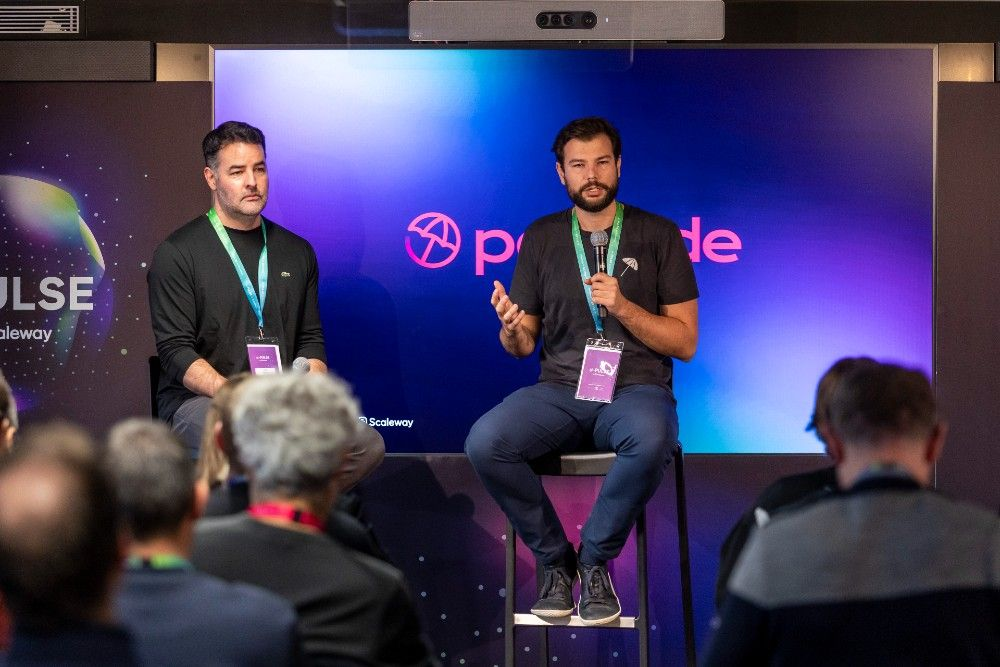\n\nAccording to **Eiso Kant**, Co-Founder \u0026 CTO of this fascinating US company which recently relocated to Paris, “when you have a LLM (large language model) you’re training, you’re teaching it about code, by showing it lots of code. But **when you show it how problems are solved (via our sandbox of 10k codebases) you’re teaching it how to code**. In the next 5 years, all AI models will come from synthetic data - i.e. data made by another AI - so **you’ll end up with code that’s entirely not made by humans**.”\n\n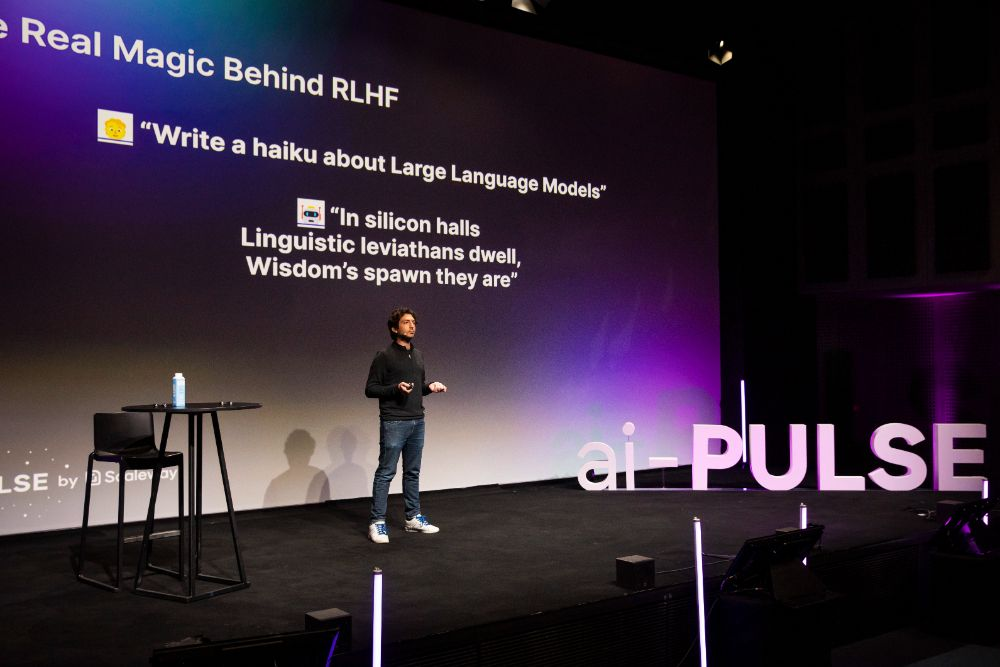\n\nThis was precisely one of the predictions of **Meta** Research Scientist **Thomas Scialom**: “Soon, **you can expect LLMs to make their own tools, because they(‘ll) have some ability to code**. That’s a whole new universe for research. If, for example, I want some code to, say, lower-case all my text, the model generates a code to do that. But now, it can execute the code, see what an input gives and [compare it with] the output from the real world, grounded in code execution. Then **the LLM can reflect [on] its own expectations**”... and effectively learn to code.\n\nSo further off in the future, will these models be “just [stochastic parrots](https://dl.acm.org/doi/10.1145/3442188.3445922) generating text, or are they truly understanding what’s beneath the data?” asked Scialom. It’s hard to say, he concluded, but one thing’s for sure: “we can put more compute in the smaller models, in the bigger models, and **we will have better models with the same recipe in five years’ time.**”\n\n\n_To find out more about Scaleway’s AI solutions, [click here](https://www.scaleway.com/en/ai-solutions/); or to talk to an expert, [click here](https://www.scaleway.com/en/contact-ai-solution/)._\n\n_Watch all of the day's sessions on our [YouTube channel](https://www.youtube.com/channel/UC-Le3MdpQ79hWz8_t2_pGEw)_\n\n_\u0026 stay tuned for more ai-PULSE content soon!_\n","createdAt":"2023-11-22T18:03:23.408Z","updatedAt":"2024-11-18T16:08:52.182Z","publishedAt":"2023-11-22T18:16:44.218Z","locale":"en","tags":"AI\nai-PULSE","popular":false,"articleOfTheMonth":false,"category":"Build","timeToRead":7,"excerpt":"The first edition of AI conference ai-PULSE was one to be remembered. Here’s a first sweep of the most headline-worthy quotes!","author":"James Martin","h1":"The Best quotes from ai-PULSE 2023","createdOn":"2023-11-22"}},{"id":400,"attributes":{"title":"why-cpus-also-make-sense-for-ai-inference","path":"why-cpus-also-make-sense-for-ai-inference/","description":"_As CPO of US-based chipmaker [Ampere Computing](https://amperecomputing.com/), Jeff Wittich has an important message for IT executives: artificial intelligence inference doesn’t necessarily need supercomputers, or GPUs. In many cases, he claims, CPUs are not only good enough, they’re even ideal. Why? Because they can offer right-sized compute power with minimal energy consumption, thereby limiting AI’s impact on the planet and on cloud budgets. We spoke to Wittich ahead of his keynote at [ai-PULSE](https://www.ai-pulse.eu/) on November 17…_\n\n## How does Ampere want to be considered by cloud providers today when it comes to AI? \n**Jeff Wittich**: Ampere’s mission from day one has been to deliver sustainable computing for modern performance environments like the cloud. That extends to AI too. Cloud service providers (CSPs) should consider Ampere for all needs in the cloud, including when looking to build AI workload capabilities. \n\nWe know one of CSPs’ biggest challenges is power consumption. Using more power is costly, plus power is scarce, and you can’t expand your data center infinitely. This means **we need to deliver more efficient systems over time, to provide more compute capacity without consuming more power**.\n\nAI inference has really brought this into the forefront, as demand for it has increased rapidly, making that power challenge even more difficult to solve. We have a solution that tackles that.\n\nOften when we talk about AI, we forget that AI training and inference are two different tasks. \n\n**Training** [or teaching the AI model with large quantities of data] is a one-off, gigantic task that takes a long time; and for that one time, you might be OK to use the considerable amounts of power required by GPUs and supercomputers.\n\n**Inference** [or using the trained AI model on a regular basis] is different, as it can be millions of tasks running every second. Inference is your “scale” model, that you’re running all the time, so efficiency is more important here.\n\nSo whereas accelerators can make a lot of sense for training, **building inference workload doesn’t need to be done on supercomputing hardware**.\n\nIn fact, general-purpose CPUs are good at inference, and they always have been. Our CPUs are especially well-suited to the task because they are high-performance and balanced. Plus you need predictable latency in these cases, and to keep processing close to the core, not have it bouncing around all over the place. Having a lot of cores is useful too, as is flexibility. It may be that AI inference isn’t 100% of what you’re asking a CPU to do. If it can do other things at the same time, you get higher overall utilization.\n\n## How can CPUs be enough for inference, when the current trend is “throwing more expensive, power-hungry, and narrowly specialized hardware at AI”*?\n**JW**: AI needs today cover a whole spectrum. What are your project’s compute requirements? Do you need to be inferencing all the time? What about memory bandwidth? **For the vast majority of that spectrum, CPUs will be the right-sized solution**. Some inference needs may have a particularly high memory footprint, and therefore need a GPU.\n\nBut I think we’ll see a shift in time to smaller, more versatile solutions. It’s like I could have come to work in a Ferrari today, when what I actually need is a more economical electric vehicle that’ll get me here in the same time.\n\nWe’re still in the **hype and research phase for AI**, due to the euphoria around these massive large language models (LLMs), where the instinct is to throw the most possible power at a problem and see what happens. But at some point, **these use cases will mature, and efficiency and sustainability will be the victor**.\n\nNot everyone will be able to pay for a solution like ChatGPT, which features all of human knowledge. We’ll see more specialization of models, as well as refinement of existing models. Overall, models will become smaller, and more focused on specific tasks.\n\n_*A quote from Ampere's recent [white paper](https://info.amperecomputing.com/AI-Whitepaper)._\n\n## What are the most interesting inference use cases for Ampere chips today?\n**JW**: We’re already seeing some great examples, from real-time voice-to-text translation in any language, which makes things easier for meetings with colleagues in other countries, or increasing accessibility for hearing-deficient people; or generative AI use cases, like artwork, videos, or simplifying everyday routine tasks. These cases all work well with our CPUs.\n\nMore specifically, [Matoha](https://matoha.com) uses Ampere CPUs to power its near-infra-red spectroscopy. This allows them to scan a 30-year-old landfill for waste noone back then thought of recycling. They can scan a bottle, figure out what type of plastic it is, and send it to the right recycling location. And it works with other materials too, like fabrics.\n\nWe also have [Red Bull Racing](https://www.redbullracing.com/int-en), the highly successful Formula One team, which uses our processors for pre- and in-race day analysis, to optimize their racing strategies. They have a limited amount of time to run these analyses, using complex models based on past race data. Our CPUs allow them to process a lot of data in a very short time, so they can change strategies in real-time, for example, if the weather changes.\n\n## How exactly do Ampere CPUs transfer training data from Nvidia GPUs, for inference?\n**JW**: **It’s a common misperception that you need to run training and inference on the same models**. It’s actually very easy to take one framework and run it on another piece of hardware. It’s particularly easy when you use [AI frameworks like] **PyTorch** and **Tensorflow**; the models are extremely portable. \n\nWe have a whole AI team at Ampere, which has developed software called **AI-O**, that allows us to have compatibility across all AI frameworks. So **there’s no need to adapt data models at all**. Just take a model trained with any GPU, put it on an Ampere CPU and it’ll run great. AI-O does some optimization on the data and processing sides, but you don’t need to use it unless you really want to improve performance. Otherwise, no need for quantization or anything like that. People think (transferring from training GPUs to inference CPUs) is incredibly complicated, but it’s not!\n\n## Can data models be adapted to get maximum performance from Ampere CPUs?\n**JW**: Yes, just use the software library we have (AI-O): it’s sophisticated, it gets better results, and it makes sure the way the code is compiled is well-suited to our processors. You’ll get several times higher performance for some models, should you choose that option (but you don’t have to).\n\nSometimes, there’s an advantage to running at lower precision. So instead of running an FP32 [data model], run the model in something like int8. Our processors support FP32, FP16, Bfloat16, int8… any numerical format you’ll want to run in. In the case of int8, you’re essentially getting four times more performance capacity than FP32, and in many cases you’re not losing any accuracy as a result if doing so. And that’s just as easy to do on our processors as it would be on an Nvidia GPU, or Intel or AMD CPU. \n\nTo make things even easier, we ensure you get full support from our AI engineers. That doesn’t exist with all the manufacturers today: they’ll have hardware support, but not software. Better still: we haven’t had many help requests yet, so we like to think that means our solution just works. We do know **a lot of people are using AI-O: we’ve seen a sevenfold usage increase in the past six months**, so that’s fantastic.\n\n## AI consumes considerable amounts of energy and (indirectly) water. Can you quantify the energy savings of Ampere CPUs vs other GPUs for AI inference? \n**JW**: If you run [OpenAI’s generative speech recognition model] **Whisper** on our **128-core Altra CPU versus Nvidia’s A10 card, we consume 3.6 times less power per inference**. Or for something lower-power, like Nvidia Tesla T4 cards, we consume 5.6 times less.\n\nYou also have the cooling aspect: the power you’re drawing turns into dissipated heat. So **doing this with 3.6 less times power means it’s that much easier to cool**. So our hardware doesn’t require super-exotic cooling systems, just standard fans.\n\nWater requirements are harder to calculate because there are so many different ways of cooling data centers. But it’s a fact that the easier a CPU is to cool, the less water you need to cool it. \n\n## How can Ampere help cloud providers to become sustainability leaders?\n**JW**: That’s absolutely our mission, as sustainability is one of CSPs’ main pillars. Most people only see the cost, so if we can provide a more efficient processor, great. But we’re seeing more and more CSPs stepping up and providing sustainability messages too, with energy figures, and carbon consumption of Ampere versus Intel and other chipmakers, and so on. **We encourage CSPs to be vocal about that**. \n\nWe’re tackling how to reduce the amount of energy consumed without asking people to use less compute power. So we should be at the forefront of finding ways to create as little impact as possible. Especially **with AI: you hear about some AI usages causing data centers to double their energy consumption**. We need to pick the right solutions to make sure that doesn’t happen.\n\n## What can we expect from Ampere in terms of future developments in CPU technology, particularly in the context of AI and emerging technologies?\n**JW**: Over the next few years, we’ll continue to release CPUs that are more efficient, and deliver ever-higher core counts, as that gives you more and more throughputs for things like AI inferencing. So you’ll see us looking to increase output compute without requiring more incremental power, by adding more cores and increasing memory bandwidth and I/O bandwidth, so that’s perfect for AI inferencing too.\n\nIn AI, as we have a team of dedicated engineers, you’ll see us put more new features into our CPUs: **we’ve got some interesting ideas in the pipeline to increase inference performance disproportionately**. The pace of innovation in the AI space is extraordinarily fast. We’re releasing new products extremely quickly for that reason. We’re also learning from how our clients are using our CPUs in AI today, to anticipate innovations we’ll work into products we’ll release very soon. **If you take five years to make this tech, you’re already obsolete**. So this is why we’ve adapted our development cycle.\n\n## What are you most excited about today?\n**JW**: Sustainability has to be one. Doing something that has a huge impact globally is really exciting. The cloud has a big emissions footprint, globally speaking, so it’s important we take the lead here, including with regards other industries.\n\nMore broadly speaking, I’m excited that we're building a new type of general-purpose compute for the world, which isn’t constrained by the limits of data centers to date. By thinking “What does the cloud need?” we’ve done some really cool things, and that’s why we can deliver such great performance across all CSPs. We have limitless capacity to innovate within our CPUs. **It’s a new generation for the cloud era!**\n\n\u003cbr\u003e\n_Jeff Wittich presents \"The Key to AI's Power Efficiency Revolution\" (17:25) at ai-PULSE November 17, followed by a panel with Gladia and Powder, \"How to make Inference as cost-efficient, sustainable and performant as possible?\", from 17:45. [More info here](https://www.ai-pulse.eu/agenda)..._","createdAt":"2023-11-13T09:10:53.437Z","updatedAt":"2023-12-18T08:50:47.962Z","publishedAt":"2023-11-13T15:11:54.579Z","locale":"en","tags":"AI\nai-PULSE","popular":false,"articleOfTheMonth":false,"category":"Build","timeToRead":4,"excerpt":"Artificial intelligence inference doesn’t necessarily need supercomputers, or GPUs, says Ampere CPO Jeff Wittich. CPUs are not only good enough, they can even be ideal, he says. Find out why...","author":"James Martin","h1":"Why CPUs also make sense for AI inference - interview with Ampere Computing's Jeff Wittich","createdOn":"2023-11-13"}},{"id":397,"attributes":{"title":"optimize-llm-performance-nvidia-H100-golem-ai","path":"optimize-llm-performance-nvidia-h100-golem-ai/","description":"_(Article originally published on Golem.ai's blog, [here](https://golem.ai/en/blog/optimisation-llm-scaleway?hss_channel=lcp-10942745). Reproduced with permission. Thanks, guys!)_\n\nWhy did [Golem.ai](https://golem.ai) decide to experiment with LLMs ? It’s because we believe in the complementary nature of Symbolic \u0026 Generative AI approaches, as explained in our [previous blogpost](https://golem.ai/en/blog/ia-generative-analytique-neurosymbolique).\n\n\n## Why choose LlaMA-2 ?\n\nFacebook parent company Meta caused a stir in the artificial intelligence (AI) industry last July with the launch of LLaMA 2, an open-source large-scale language model (LLM) designed to challenge the restrictive practices of its major technological competitors.\n\nUnlike AI systems launched by Google, OpenAI and others (such as Apple with Apple GPT?), which are tightly guarded in proprietary models, Meta is releasing LLaMA 2's code and data free of charge to enable researchers worldwide to build and improve the technology!\n\nHere are the five key features of Llama 2:\n- Llama 2 outperforms other open-source LLMs in benchmarks for reasoning, coding proficiency, and knowledge tests.\n- The model was trained on almost twice the data of version 1, totaling 2 trillion tokens. Additionally, the training included over 1 million new human annotations and fine-tuning for chat completions.\n- The model comes in three sizes, each trained with 7, 13, and 70 billion parameters.\n- Llama 2 supports longer context lengths, up to 4096 tokens.\n- Version 2 has a more permissive license than version 1, allowing for commercial use.\n\n\n## First tests in “practicing \u0026 learning mode” with Replicate.com\n\nTo Test Llama-2, we first opted for [Replicate.com](https://replicate.com). This allows you to pay as you go, with no need to install on existing hardware. A perfect first approach for experimenting !\n\nHowever, for reasons of privacy and economic intelligence, we’ve opted for a second approach, as explained below.\n\n\n## Why Llama-2 on in-house GPUs after Replicate.com?\n\nAt Golem.ai, trusted artificial intelligence, data sovereignty, security and control of the entire value chain is the most important thing.\nFor this reason, we decided to carry out our own benchmark using the material resources of our French cloud provider, Scaleway.\n\nAlthough the LLaMA-2 model is free to download and use, it should be noted that self-hosting of this model requires GPU power for timely processing.\n\nLLaMA 2 is available in three sizes: 7 billion, 13 billion and 70 billion parameters, depending on the model you choose.\n\nFor the purposes of this demonstration, we will use model 70b to obtain the best relevance !\n\n\n## Setting up the in-house GPUs solution\n\nLet’s get to the heart of the matter 😈\n\n**Integration overview**\n\nThe user provides one input: a prompt input (i.e. ask a question).\n\nAn API call is made to the LLAMA.CPP server, where the prompt input is submitted and the response generated by Llama-2 is obtained and displayed to the user.\n\nWe running Llama-2 70B model using llama.cpp, with NVIDIA CUDA 12.2 on Ubuntu 22.04\n[Llama.cpp](https://github.com/ggerganov/llama.cpp) is a C/C++ library for the inference of [LlaMA/LlaMA-2 models](https://ai.meta.com/llama).\n\nFor this scenario, we will use the [H100-1-80G](https://www.scaleway.com/en/h100-pcie-try-it-now/), the most powerful hardware in the GPUs range from our French Cloud provider Scaleway.\n\nThe method for implementing the solution is specified in the next few lines.\n\nWe estimate that it will take around 30mn to set up, provided you meet our OS, software, hardware requirements and you don’t encounter any errors 🙂\n\n**A. Installation**\n\nTwo possible paths :\n\n1/ The official way to run LLaMA-2 is via their examples repository and in their recipes repository.\n- Benefit: Official method\n- Disadvantages: Developed in python (Slow to run \u0026 Excessive RAM consumption); GPU H100 acceleration may not work.\n\n2/ Run LLaMA-2 via the llama.cpp interface\n- Benefits : This pure C/C++ implementation is faster and more efficient than its official Python counterpart, and supports GPU acceleration via CUDA and Apple's Metal. This considerably speeds up inference on the CPU and makes GPU inference more efficient.\n- Disadvantages: Community-based method (unofficial)\n\nWe've opted to use llama.cpp for this implementation.\n\n\n**B. Model available**\n\nCheck model type :\n[https://www.hardware-corner.net/llm-database/Llama-2/](https://www.hardware-corner.net/llm-database/Llama-2/)\n/!\\ /!\\ llama.cpp no longer supports the GGML models\n[https://huggingface.co/TheBloke/Llama-2-70B-Chat-GGML](https://huggingface.co/TheBloke/Llama-2-70B-Chat-GGML)\n⇒ Replace with GGUF models\n[https://huggingface.co/TheBloke/Llama-2-70B-chat-GGUF](https://huggingface.co/TheBloke/Llama-2-70B-chat-GGUF) (based on Llama-2-70b-chat-hf)\n\n\n**C. Installation process**\n\n**1/ Install NVIDIA CUDA DRIVER (if not installed on your GPU Machine)**\n\nTo start, let's install NVIDIA CUDA on Ubuntu 22.04. The guide presented here is the same as the [CUDA Toolkit download page](https://developer.nvidia.com/cuda-downloads) provided by NVIDIA.\n```js\n$ wget \u003chttps://developer.download.nvidia.com/compute/cuda/repos/ubuntu2204/x86_64/cuda-keyring_1.1-1_all.deb\u003e\n$ sudo dpkg -i cuda-keyring_1.1-1_all.deb\n$ sudo apt-get update\n$ sudo apt-get -y install cuda-toolkit-12-3`\n```\n\nAfter installing, the system should be restarted. This is to ensure that NVIDIA driver kernel modules are properly loaded with dkms. Then, you should be able to see your GPUs by using nvidia-smi.\n\n```js\n$ sudo shutdown -r now\n\nllm@h100-ftw:~$ nvidia-smi\nWed Oct 4 08:44:54 2023\n```\n\n```js\n+---------------------------------------------------------------------------------------+\n| NVIDIA-SMI 535.104.12 Driver Version: 535.104.12 CUDA Version: 12.2 |\n|-----------------------------------------+----------------------+----------------------+\n| GPU Name Persistence-M | Bus-Id Disp.A | Volatile Uncorr. ECC |\n| Fan Temp Perf Pwr:Usage/Cap | Memory-Usage | GPU-Util Compute M. |\n| | | MIG M. |\n|=========================================+======================+======================|\n| 0 NVIDIA H100 PCIe On | 00000000:01:00.0 Off | 0 |\n| N/A 42C P0 51W / 350W | 4MiB / 81559MiB | 0% Default |\n| | | Disabled |\n+-----------------------------------------+----------------------+----------------------+\n \n+---------------------------------------------------------------------------------------+\n| Processes: |\n| GPU GI CI PID Type Process name GPU Memory |\n| ID ID Usage |\n|=======================================================================================|\n| No running processes found |\n+---------------------------------------------------------------------------------------+\n```\n\n\n**2/ Make sure you have the nvcc binary in your path**\n\n```js\nllm@h100-ftw:~$ nvcc --version\nnvcc: NVIDIA (R) Cuda compiler driver\nCopyright (c) 2005-2023 NVIDIA Corporation\nBuilt on Tue_Aug_15_22:02:13_PDT_2023\nCuda compilation tools, release 12.2, V12.2.140\nBuild cuda_12.2.r12.2/compiler.33191640_0`\n\n`*if the command can’t be found : ln -s /usr/local/cuda/bin/ /bin/\n```\n\n\n**3/ Clone and Compile llama.cpp**\n\nAfter installing NVIDIA CUDA, all of the prerequisites to compile llama.cpp are already satisfied. We simply need to clone llama.cpp and compile.\n\n```js\n$ git clone \u003chttps://github.com/ggerganov/llama.cpp\u003e\n$ cd llama.cpp\n```\n\nFor matching CUDA arch and CUDA gencode for various NVIDIA architectures: Modify Makefile before compilation with `NVCCFLAGS += -arch=all-major` instead of `NVCCFLAGS += -arch=native`\n\n```js\n$ make\n$ make clean \u0026\u0026 LLAMA_CUBLAS=1 make -j\n```\n\n\n**4/ Download and Run LLaMA-2 70B**\n\nWe use the converted and quantized model by the awesome HuggingFace community user, [TheBloke](https://huggingface.co/TheBloke). The pre-quantized models are available via [this link](https://huggingface.co/TheBloke/Llama-2-70B-chat-GGUF). In the model repository name, GGUF refers to a new model file format introduced in August 2023 for llama.cpp.\n\nTo download the model files, first we install and initialize git-lfs.\n```js\n$ sudo apt install git-lfs\n$ git lfs install\n```\n\nYou should see \"Git LFS initialized.\" printed in the terminal after the last command. Then, we can clone the repository, only with links to the files instead of downloading all of them.\n\n```js\ncd models\nGIT_LFS_SKIP_SMUDGE=1 git clone \u003chttps://huggingface.co/TheBloke/Llama-2-70B-chat-GGUF\u003e`\n`$ cd Llama-2-70B-GGUF\n$ git lfs pull --include llama-2-70b-chat.Q6_K.gguf-split-a\n$ git lfs pull --include llama-2-70b-chat.Q6_K.gguf-split-b\n$ cat llama-2-70b-chat.Q6_K.gguf-split-* \u003e llama-2-70b-chat.Q6_K.gguf \u0026\u0026 rm llama-2-70b-chat.Q6_K.gguf-split-*\n```\n\nThe one file we actually need is `llama-2-70b-chat.Q6_K.gguf`, which is the Llama 2 70B model processed using one of the 6-bit quantization method.\n\nThis model requires an average of 60GB of memory. On the H100, we’ve 80GB (HBM2e) of VRAM. Processing will be carried out entirely on the H100 GPU!\n\n```js\n$ ./main -ngl 100 -t 1 -m llama-2-70b-chat.Q6_K.gguf --color -c 4096 --temp 0.7 --repeat_penalty 1.1 -n -1 -p \"[INST] \u003c\u003cSYS\u003e\u003e\\\\nYou are a helpful, respectful and honest assistant. Always answer as helpfully as possible, while being safe. Your answers should not include any harmful, unethical, racist, sexist, toxic, dangerous, or illegal content. Please ensure that your responses are socially unbiased and positive in nature. If a question does not make any sense, or is not factually coherent, explain why instead of answering something not correct. If you don't know the answer to a question, please don't share false information.\\\\n\u003c\u003c/SYS\u003e\u003e\\\\n{prompt}[/INST]\"\n```\n\n**5/ Serving Llama-2 70B**\n\nMany useful programs are built when we execute the make command for llama.cpp. \n\nmain is the one to use for generating text in the terminal. \n\nperplexity can be used to compute the perplexity against a given dataset for benchmarking purposes.\n\nIn this part we look at the server program, which can be executed to provide a simple HTTP API server for models that are compatible with llama.cpp. [https://github.com/ggerganov/llama.cpp/blob/master/examples/server/README.md](https://github.com/ggerganov/llama.cpp/blob/master/examples/server/README.md)\n\n```js\n$ ./server -m models/Llama-2-70B-chat-GGUF/llama-2-70b-chat.Q6_K.gguf \\\\\n -c 4096 -ngl 100 -t 1 --host 0.0.0.0 --port 8080\n```\n \nReplace `-t 32` with the number of physical processor cores. For example, if the system has 32 cores / 64 threads, use -t 32. If you're completely offloading the model to the GPU, use -t 1 (as on the H100).\n\nReplace `-ngl 80` with the number of GPU layers for which you have VRAM (such as H100). Use `-ngl 100` to unload all layers onto VRAM - if you have enough VRAM. Otherwise, you can partially offload as many layers as you have VRAM for, onto one or more GPUs.\nparams : [https://huggingface.co/TheBloke/Llama-2-70B-Chat-GGML#how-to-run-in-llamacpp](https://huggingface.co/TheBloke/Llama-2-70B-Chat-GGML#how-to-run-in-llamacpp)\n\n```js\nllm_load_tensors: ggml ctx size = 0.23 MB\nllm_load_tensors: using CUDA for GPU acceleration\nllm_load_tensors: mem required = 205.31 MB\nllm_load_tensors: offloading 80 repeating layers to GPU\nllm_load_tensors: offloading non-repeating layers to GPU\nllm_load_tensors: offloaded 83/83 layers to GPU\nllm_load_tensors: VRAM used: 53760.11 MB\n...................................................................................................\nllama_new_context_with_model: n_ctx = 4096\nllama_new_context_with_model: freq_base = 10000.0\nllama_new_context_with_model: freq_scale = 1\nllama_kv_cache_init: offloading v cache to GPU\nllama_kv_cache_init: offloading k cache to GPU\nllama_kv_cache_init: VRAM kv self = 1280.00 MB\nllama_new_context_with_model: kv self size = 1280.00 MB\nllama_new_context_with_model: compute buffer total size = 573.88 MB\nllama_new_context_with_model: VRAM scratch buffer: 568.00 MB\nllama_new_context_with_model: total VRAM used: 55608.11 MB (model: 53760.11 MB, context: 1848.00 MB)\n```\n\n\nExplanation of Llama.cpp metrics :\n\nWhen you execute your input, various metrics are communicated to you to measure its performance.\n\n```js\nllama_print_timings: load time = 59250.72 ms\nllama_print_timings: sample time = 611.28 ms / 180 runs ( 3.40 ms per token, 294.47 tokens per second)\nllama_print_timings: prompt eval time = 1597.63 ms / 508 tokens ( 3.14 ms per token, 317.97 tokens per second)\nllama_print_timings: eval time = 11703.38 ms / 179 runs ( 65.38 ms per token, 15.29 tokens per second)\nllama_print_timings: total time = 13958.06 ms\n\n- load time: loading model file\n- sample time: generating tokens from the prompt/file choosing the next likely token.\n- prompt eval time: how long it took to process the prompt/file by LLaMa before generating new text.\n- eval time: how long it took to generate the output (until [end of text] or the user set limit).\n- total: all together\n```\n\n\n## Benchmark between Replicate.com and NVIDIA H100 GPUs hosted by Scaleway\n\nAfter running a hundred tests in total between Replicate.com and the NVIDIA H100 hosted by Scaleway, we conclude that the execution difference is 40% in favor of using GPU H100-1-80G processors provided by Scaleway.\n\nThe Hallucination Score on a scale of 0 to 3 that we assign at Golem.ai, which represents the relevance of the response to each test, is not sufficiently representative of any notable difference between Replicate.com and Scaleway.\n\nTo find out more, we invite you to read the article on [Golem.ai's LLM test protocol](https://www.notion.so/b25c874f8c6a45caa0520d4fabc654f9?pvs=21)\n\n\n## Conclusion \u0026 Opening\n\nThe use cases go far beyond this first experiment. At Golem.ai, we believe there are many other ways to use LLMs with our technology, including tooling and support for our users.\n\nThis is just the beginning of a long and exciting adventure.\n\nThere are several Frameworks for Serving LLMs. Each has its own features.\n\nIn this article, we experimented with Llama.cpp running LLaMa 70b model.\n\nTo learn more about this topic, please read the [following article](https://betterprogramming.pub/frameworks-for-serving-llms-60b7f7b23407), which deals specifically with this subject.\n\n\n\n_Scaleway hosts Europe's premier AI conference, ai-PULSE, November 17 at Station F! [Register now to secure your seat, onsite or online!](https://www.eventbrite.co.uk/e/billets-europes-premier-ai-conference-by-scaleway-692505571807?aff=blog)._\n","createdAt":"2023-11-03T10:53:49.064Z","updatedAt":"2023-11-30T10:56:03.330Z","publishedAt":"2023-11-03T15:59:47.445Z","locale":"en","tags":"AI\nGuest Post","popular":false,"articleOfTheMonth":false,"category":"Build","timeToRead":8,"excerpt":"Why did Scaleway partner Golem.ai decide to experiment with LLMs? Because Symbolic \u0026 Generative AI approaches can be complementary. So here's how to optimize the latter!","author":"Kevin Baude","h1":"How to Optimize LLM Performance with NVIDIA H100 GPUs from Scaleway, by Golem.ai","createdOn":"2023-11-03"}}]},"meta":{"id":1251,"title":"AI in practice: Generating subtitles for a video","description":"In this practical example, we roll up our sleeves and put Scaleway's H100 instances to use by leveraging a couple of Open Source ML Models to optimize our internal communication workflows.","ogtype":null,"ogtitle":null,"ogdescription":"In this practical example, we roll up our sleeves and put Scaleway's H100 instances to use by leveraging a couple of Open Source ML Models to optimize our internal communication workflows.","noindex":false},"localizations":{"data":[]}}},{"id":425,"attributes":{"title":"how-sustainable-is-ai","path":"how-sustainable-is-ai/","description":"Just over a year after the ChatGPT-fuelled generative AI explosion, it’s hard to remember a time without these groundbreaking tools. However, it remains to be seen if the breakneck speed of change has given us enough time to fully assess generative AI’s true impact on the planet. So let’s take a look.\n\n\n## The impact, in figures\n\nFirst and foremost, it’s now well established that generative AI requires considerably more computing power than standard calculations. A key reason for this is that **generative AI model training calls for GPUs rather than CPUs. The former generally requires around four times more energy than the latter** (case in point: Ampere’s CPUs for AI consume [3-5 times less energy than the equivalent NVIDIA machines](https://www.scaleway.com/en/blog/why-cpus-also-make-sense-for-ai-inference/)).\n\nFurthermore, as **AI GPUs tend to generate 2.5x more heat than CPUs** (standard CPUs used in cloud computing are in the range of 250-350W TDP, whereas GPUs are in the 750-800W range, cf. [Intel](https://www.intel.com/content/www/us/en/secure/care/products/237263/intel-xeon-gold-6554s-processor-180m-cache-2-2-ghz.html), [AMD](https://www.amd.com/fr/products/cpu/amd-epyc-9534) [x2](https://www.amd.com/fr/products/accelerators/instinct/mi300/mi300a.html), \u0026 [NVIDIA](https://www.nvidia.com/fr-fr/data-center/h100/)), they require that much extra cooling power. So the processors needed for generative AI training and inference are considerably more power-hungry than pre-generative AI models.\n\nThen there’s the difference between training and inference. Looking at the former, or the process required to ‘educate’ a generative AI model by feeding it as much data as possible, the emissions generated by training vary hugely depending on the model:\n- **552 tCO2e** - GPT3.5, 1.3, 6 \u0026 175bn parameters ([source](https://dataforgood.fr/iagenerative/))\n- **284 tCO2e** - a medium-size LLM, 213m parameters ([source](https://arxiv.org/abs/1906.02243))\n- **30 tCO2e** - BLOOM, a frugal LLM, 175bn parameters ([source](https://arxiv.org/abs/1906.02243))\n\n_(tCO2e = tons of CO2 equivalent, namely CO2 + the 3 other most potent greenhouse gasses)_\n\n\nThis means that training a generative AI model can generate anything from the equivalent of three French people’s annual emissions (10 tCO2e), to 50.\n\nBut of course, training is a one-off occurrence. **Inference, or the everyday usage of a model, has its own impact, which has been estimated at 200 times higher than that of training**. According to French tech association [Data for Good](https://dataforgood.fr/iagenerative/), considering ChatGPT has [100m weekly users](https://techcrunch.com/2023/11/06/openais-chatgpt-now-has-100-million-weekly-active-users/), that’s 100,000 tCO2e/year for GPT-3.5.\n\nTo put it another way, **generating one image with generative AI can use as much energy as that required to fully recharge a smartphone**, according to the latest [white paper](https://arxiv.org/pdf/2311.16863.pdf) co-authored by Sasha Luccioni, Climate Lead and AI Researcher at Hugging Face. \"Can\" is the operative word here, however, as [The Verge](https://www.theverge.com/24066646/ai-electricity-energy-watts-generative-consumption) points out, given the huge variety of GenAI models already available. \n\nThen there’s **water**. Also linked to inference, it’s been established that [one conversation with ChatGPT uses half a liter of water](https://arxiv.org/pdf/2304.03271.pdf) in terms of the data center cooling resources required (cf. the considerable heat generated by GPUs, above). Not to mention GPT-3’s training, which required 5.4 million liters of water ([same source](https://arxiv.org/pdf/2304.03271.pdf)). That’s a bit more than one liter per training hour (training GPT-3 took 4.6 million GPU hours, according to… [ChatGPT](https://www.wholegraindigital.com/blog/social-environmental-impacts-of-ai/?utm_source=pocket_saves)!)\n\nGiven these elements, it’s not surprising that AI energy demand is set to outpace supply. \n\nIf Google were to use AI for its around 9 billion daily searches - which it [most likely will](https://www.theverge.com/2023/5/10/23717120/google-search-ai-results-generated-experience-io) - it would need 29.2 terawatt hours (TWh) of power each year, according to researcher Alex de Vries. As such, as de Vries told [Euronews last year](https://www.euronews.com/next/2023/10/10/demand-for-ai-could-mean-technology-consumes-same-energy-as-a-country-analysis-shows), **by 2027, AI could consume as much electricity as a medium-sized country like the Netherlands**.\n\nThe IEA (International Energy Association) recently issued [a similar warning](https://iea.blob.core.windows.net/assets/6b2fd954-2017-408e-bf08-952fdd62118a/Electricity2024-Analysisandforecastto2026.pdf): **data centers’ energy consumption could more than double by 2026, to 1,000TWh, driven by AI** and cryptocurrency.\n\nOne of AI’s most influential leaders naturally saw this coming: at Davos in January 2024, **OpenAI CEO Sam Altman said AI will definitely need much more energy than initially thought**. “There’s no way to get there without a[n energy] breakthrough [like nuclear fusion]”, [Reuters](https://www.usnews.com/news/technology/articles/2024-01-16/openai-ceo-altman-says-at-davos-future-ai-depends-on-energy-breakthrough) reported him saying on a panel. This could well be why OpenAI’s most famous investor, Microsoft, just hired a new Director of Nuclear Development Acceleration: to “help power its own AI revolution”, according to [TechRadar Pro](https://www.techradar.com/pro/microsoft-goes-atomic-worlds-most-valuable-company-just-hired-a-director-of-nuclear-development-acceleration-to-help-power-its-very-own-ai-revolution). \n\nWhilst we’re a [long way off nuclear fusion](https://www.newsweek.com/nuclear-fusion-when-ready-electricity-technology-1773349) - versus current fission methods - a trend of nuclear-powered data centers is definitely bubbling up. \n\nAccording to [AMD CEO Lisa Su](https://www.theregister.com/2023/02/23/amd_zettaflop_systems_nuclear/), in around ten years’ time we may see zettaflop-class supercomputers, whose requirement for 500MW facilities will far outstrip todays’ 20-50MW facilities. Such needs can only be powered by local, dedicated sources like nuclear SMRs (small modular reactors).\n\nThis is why [The Register](https://www.theregister.com/2023/09/27/datacenters_nuclear_power/) reports that last year, [Cumulus Data](https://cumulusinfra.com/) opened a 65MW nuclear data center, which it claims will ultimately reach a capacity of 950MW. In addition, SMR-powered facilities are currently being investigated by Green Energy Partners/IP3 (Virginia, USA) and Bahnhof (Sweden). \n\nGiven our current reliance on fossil fuels (e.g. with the US still dependent on them for 80% of its energy), could nuclear-powered emission-free data centers be a better option for the planet than current solutions? Time will tell, especially for future generations…\n\n\n## How to reduce that impact\n\nThe first rule of any sustainability strategy, especially in tech, should be to ask “do I really need this?”\n\nIndeed, generative AI is neither inevitable, nor adapted to all use cases. As we’ve [already explained here](https://www.scaleway.com/en/blog/symbolic-ai-is-dead-long-live-symbolic-ai/), **symbolic, or “good old-fashioned” AI, can do a lot more than what many of us expect, and with considerably less impact**. French startup Golem.ai has notably established that one of their [email-sorting symbolic AI models emits 1000 less CO2eq than GPT-3](https://golem.ai/fr/ia-frugalite-sobriete).\n\nThat said, if you do decide you absolutely must use generative AI, does it have to be on the scale of ChatGPT? Must it hoover up all of the world’s data, or can it just focus on a specialized dataset, like legal documents, for example?\n\nDo you have to use a supercomputer for training, or would a smaller, single [H100 GPU](https://www.scaleway.com/en/h100-pcie-try-it-now/) do the trick? Could you simultaneously prolong the life of old hardware and save money by using older generation GPUs?\n\n**For inference, could a less energy-hungry CPU, like Ampere’s, meet your needs** (cf. above)? \n\nNext, it can be inspiring to look into **the many ways generative AI is being used today to actively further sustainability; potentially, to an extent that may far outweigh its impact.**\n\nIndeed, a [McKinsey report](https://www.mckinsey.com/capabilities/quantumblack/our-insights/how-artificial-intelligence-can-deliver-real-value-to-companies) once estimated AI-based technologies could help companies to reduce their emissions by up to 10%, and their energy costs by 10-20%.\n\nOne clear example in tech is Google’s AI subsidiary DeepMind, which [declared](https://deepmind.google/discover/blog/deepmind-ai-reduces-google-data-centre-cooling-bill-by-40) as early as 2016 that its application of machine learning in GCP data centers has enabled said facilities to consume 40% less energy. How? By improving anticipation of key internal factors, like how different types of machinery interact with each other, and external ones like the weather, thanks to training data such as past temperatures, power, pump speeds and setpoints.\n\nAI’s impact in data centers can also be reduced by using alternative cooling systems. This is the case of the **DC5 data center, where Scaleway’s AI machines are housed, which consumes 30-40% less energy than standard facilities, because it uses no air conditioning**. Instead, it relies on free cooling most of the year, and, in warmer summer months, adiabatic cooling, a process which cools outside air by passing it through a moist membrane. This, plus French energy’s low carbon intensity, makes **DC5 one of the world’s least impactful AI installations**.\n\n\n## AI for good: We’re just getting started\n\nMachine learning can also help in broader contexts, although many of today's LLM-based solutions are based more on predictive than generative AI. For example, using past data to predict future demand for electricity, thereby optimizing smart grids; anticipating road traffic, which can make travel, deliveries way more efficient, thereby reducing pollution (Google claims its [Green Light initiative with Google Maps](https://blog.google/outreach-initiatives/sustainability/google-ai-reduce-greenhouse-emissions-project-greenlight/) can reduce emissions at intersections by 10%); fine-tuning energy consumption in buildings via temperature prediction; and the forecasting of extreme weather events or incidents, like [Pyronear](https://pyronear.org/en/), which uses AI-equipped towers to detect forest fires.\n\nAll of these examples and more - also covering societal impacts, public policy analysis, education and finance - are already happening thanks to generative AI. This [white paper](https://dl.acm.org/doi/10.1145/3485128) by leading academics and Google thought-leaders demonstrates how these diverse activities are accelerating sustainability as a whole.\n\nGenerative AI can also facilitate access to key information about sustainability. French national ecological agency (and Scaleway client) [ADEME](https://www.linkedin.com/posts/lydia-passet-787a43159_ademe-iagaeznaezrative-genai-activity-7155594529746669570-xm_F/) is currently experimenting with a text-based model trained on the agency's extensive documentation database, with the objective of extracting key data more quickly and understandably. If the experiment is successful, the model could be opened up to the general public. Spearhearded by **Ekimetrics**' \"AI for Sustainability\" team, the project is similar to \"[ClimateQ\u0026A](https://huggingface.co/spaces/Ekimetrics/climate-question-answering)\", a model trained on the IPCC reports, which is essentially a **ChatGPT for sustainability** (and not the only one, cf. this ChatGPT plugin, \"[IPCC Explainer](https://chat.openai.com/g/g-CXYs3qu1D-ipcc-explainer)\"). \n\nThen there are flag-waving applications, which warn us of potential sustainability emergencies. Data for Good notably enabled ocean protection ONG [Bloom](https://www.bloomassociation.org/en/) to detect illegal fishing using AI, and used AI to power its [Carbonbombs.org](http://Carbonbombs.org) website, which flags the world’s most-polluting projects, such as coal mines, and is now influencing global policy. Finally, [Climatetrace.org](http://Climatetrace.org) uses AI to highlight those countries that aren’t decarbonizing as quickly as they say they are.\n\nNot forgetting the AI models themselves: the smaller they are, the less energy they consume, which makes them better for everyone, including the planet. As **Mistral AI CEO and co-founder Arthur Mensch** [told ai-PULSE](https://www.scaleway.com/en/blog/best-quotes-ai-pulse-2023/) last November, “in the ‘Vanilla Attention’ version of Transformers, you need to keep the tokens in memory. “With Mistral AI’s ‘Sliding Window Attention’ model, there are four times less tokens in memory, reducing memory pressure and therefore saving money. Currently, **too much memory is used by generative AI**”. This is notably why the company’s latest model, Mistral-7B, can run locally on a (recent) smartphone.\n\nLooking ahead, this efficiency-first approach will apply to AI solution offerings too. **Scaleway aims to beta release by mid-2024 an inference service which will serve LLMs connected to clients’ private or sensitive data**. Based on LLMs like Llama or those of Mistral AI, such a service is cost- and energy-efficient because:\n- Compute resources can be sized up and down by users according to usage peaks\n- Using existing pre-trained and open source models avoids the emissions generated by training new models from scratch.\n\n\n## The conclusion: it’s too soon to say\n\nIf we consider that there are as many AI solutions as impacts, the jury is out at best. Most experts agree it’s too early in the generative AI revolution to measure its true impact on the planet.\n\nBut there is good news! Firstly, it is totally possible to assess impact before choosing the right AI model for your needs, namely:\n- What its emissions impact is, using tools like [Machine Learning Emissions Calculator](https://mlco2.github.io/impact/#compute)\n- Whether you can re-use, or fine-tune an existing model - nearly 500,000 different ones are available in repositories like [Hugging Face](https://huggingface.co/models) - as this will consume way less energy than creating a new one from scratch\n- Whether it’s hosted by a cloud provider that works to reduce its energy consumption, and whose data centers are in a low-carbon intensity country.\n\n\nFurthermore, the principles of [green IT](https://www.scaleway.com/en/why-shift-to-green-it/) apply just as much to AI as they do to ‘traditional’ computing:\n\n- **Data centers** should use renewable energy - and as little of it as possible - whilst radically limiting water usage. They should also use alternatives to air conditioning, to considerably reduce AI’s environmental impact (see above)\n- **Hardware** should be optimized to use as little energy as possible, and to last for as long as possible\n- **Software** solutions - think AI models in this case, or options like Inference as a Service - should be engineered to consume as few computational resources, and therefore energy, as possible.\n\n\nLast but not least, AI datasets, models and machines should only be as big or powerful as they need to be. Otherwise, tech’s eternal risk of falling into the rebound effect, or [Jevon’s paradox](https://en.wikipedia.org/wiki/Jevons_paradox) - using a service more, rather than less, as it gets more efficient - could have dire consequences. \n\nAs Ekimetrics’ Head of AI for Sustainability Theo Alves Da Costa [puts it](https://vert.eco/articles/lintelligence-artificielle-va-t-elle-donner-le-coup-de-grace-au-climat?utm_source=pocket_saves), “if we use the bulldozer of AI to knock a nail into a wall, the nail will go in, but we also run the risk of knocking the whole wall down. In cases like this, it’s better to just use a hammer”.\n\n\n_Special thanks to Ekimetrics’ [Theo Alves Da Costa](https://www.linkedin.com/in/th%C3%A9o-alves-da-costa-09397a82/) for many of the sources in this article. And to Hugging Face’s [Sasha Luccioni](https://www.sashaluccioni.com/) for the inspiration!_\n","createdAt":"2024-02-15T10:19:55.417Z","updatedAt":"2024-03-11T09:35:03.784Z","publishedAt":"2024-02-15T10:25:58.667Z","locale":"en","tags":"AI\nSustainability\nGreen IT","popular":false,"articleOfTheMonth":false,"category":"Build","timeToRead":6,"excerpt":"Do generative AI's benefits for the planet outweigh its impacts? Let's try to find out...","author":"James Martin","h1":"How Sustainable is AI?","createdOn":"2024-02-15","image":{"data":{"id":3239,"attributes":{"name":"Computer-Vision-AI-Illustration-Blog.webp","alternativeText":null,"caption":null,"width":1216,"height":752,"formats":{"large":{"ext":".webp","url":"https://www-uploads.scaleway.com/large_Computer_Vision_AI_Illustration_Blog_7f08d9c374.webp","hash":"large_Computer_Vision_AI_Illustration_Blog_7f08d9c374","mime":"image/webp","name":"large_Computer-Vision-AI-Illustration-Blog.webp","path":null,"size":"372.39","width":1000,"height":618},"small":{"ext":".webp","url":"https://www-uploads.scaleway.com/small_Computer_Vision_AI_Illustration_Blog_7f08d9c374.webp","hash":"small_Computer_Vision_AI_Illustration_Blog_7f08d9c374","mime":"image/webp","name":"small_Computer-Vision-AI-Illustration-Blog.webp","path":null,"size":"128.76","width":500,"height":309},"medium":{"ext":".webp","url":"https://www-uploads.scaleway.com/medium_Computer_Vision_AI_Illustration_Blog_7f08d9c374.webp","hash":"medium_Computer_Vision_AI_Illustration_Blog_7f08d9c374","mime":"image/webp","name":"medium_Computer-Vision-AI-Illustration-Blog.webp","path":null,"size":"243.71","width":750,"height":464},"thumbnail":{"ext":".webp","url":"https://www-uploads.scaleway.com/thumbnail_Computer_Vision_AI_Illustration_Blog_7f08d9c374.webp","hash":"thumbnail_Computer_Vision_AI_Illustration_Blog_7f08d9c374","mime":"image/webp","name":"thumbnail_Computer-Vision-AI-Illustration-Blog.webp","path":null,"size":"41.65","width":245,"height":152}},"hash":"Computer_Vision_AI_Illustration_Blog_7f08d9c374","ext":".webp","mime":"image/webp","size":507.61,"url":"https://www-uploads.scaleway.com/Computer_Vision_AI_Illustration_Blog_7f08d9c374.webp","previewUrl":null,"provider":"@website/strapi-provider-upload-scaleway-bucket","provider_metadata":null,"createdAt":"2024-02-15T13:43:20.787Z","updatedAt":"2024-02-15T13:43:20.787Z"}}},"recommendedArticles":{"data":[{"id":381,"attributes":{"title":"how-can-engineers-make-it-more-sustainable-part-4","path":"how-can-engineers-make-it-more-sustainable-part-4/","description":"The **digital sector generates 4% of global greenhouse gas emissions**. Data centers and hardware are the main contributors to that impact. However, those machines — not to mention all software, websites, apps and more — run code written by developers. This means developers still have a considerable role to play. So how can engineers, and indeed anyone shaping tech today, reduce their projects’ environmental impact?\n\nIn [part 3](how-can-engineers-make-it-more-sustainable-part-3) of this series, we explored the concrete green IT solutions generating demonstrable results today. In this fourth and final chapter, we take a look at the future. How can green computing help companies achieve their carbon neutrality goals by 2050? What difference will regulatory changes make? And what about AI? Look no further…\n\n\n## 2050: The final countdown\n\nAccording to a [recent IBM study](https://www.ibm.com/thought-leadership/institute-business-value/c-suite-study/ceo) of 3000 CEOs across 24 industries, **42% of these business leaders singled out sustainability as their number one challenge over the next three years**. Most likely because most major companies have committed to reaching carbon neutrality by 2050.\n\nScaleway, for example, is aligned with the objectives of its parent company, Iliad Group, to **reach carbon neutrality for scopes 1 and 2 by 2035, and for scope 3 by 2050**. The **hardware** aspect is particularly important in this case, as it accounts for **three quarters of the digital sector’s emissions**. The environmental impact of producing and disposing of hardware means that developers need to find ways to extend that hardware’s life. By writing widely compatible applications and operating systems, developers can reduce device obsolescence, and as such e-waste.\n\nHowever, the responsibility for green IT does not lie solely with developers. Simply deactivating or deleting unneeded data and resources can make a big difference, according to Google research cited by the [Green Software Foundation](https://stateof.greensoftware.foundation/insights/green-software-vital-for-net-zero/). Google Cloud Platform (GCP) calculated that dormant data, or “unattended projects”, accounted for over [600 gross metric tons of CO2 emissions](https://cloud.google.com/blog/topics/sustainability/new-tools-to-measure-and-reduce-your-environmental-impact?hl=en) (60 times more than what one French person currently emits per year). This is why GCP developed [Unattended Project Recommender](https://cloud.google.com/blog/products/identity-security/google-cloud-launches-unattended-project-recommender), a service that notifies users of their own sleeping data and its CO2 emissions, and then prompts them to remove unnecessary data.\n\n\n## Regulation: are you compliant? \n\nIn Europe alone, companies should prepare for an incoming wave of sustainability regulations. First and foremost, the **Corporate Sustainability Reporting Directive** (CSRD), which is already in place, and which will require companies of a certain size (with over 250 employees, or €40m in turnover, or €20m in assets) to submit annual non-financial reports aligned with CSRD requirements. The deadline is January 1st 2025, covering the 2024 financial year, as [Plan A explains](https://plana.earth/academy/csrd-corporate-sustainability-reporting-directive). This means **impact reporting will soon be mandatory for the majority of European companies**. Furthermore, regulations currently under discussion in the European parliament include laws against unprovable ecological claims (i.e. fines for companies found guilty of greenwashing). \n\nSuch constraints will inevitably further the growth of green IT practices, as demonstrating efforts to reduce computing’s environmental impact will shift from being a nice-to-have to a must-have.\n\nAnd that’s just for starters. As explained in [part 3](https://www.scaleway.com/en/blog/how-can-engineers-make-it-more-sustainable-part-3/), France’s strict **RGESN** guidelines for eco-designed websites are set to become EU regulation; and indeed, many companies and organizations are already expected to follow its requirements. \n\nThen there’s the notion of **PCR**, or **Product Category Rules**, which in France are managed by national ecological transition agency **ADEME**. These rules outline how to measure hardware’s environmental impact, involving a **full lifecycle analysis that covers the manufacture, running, and disposal of all the underlying device components**. Adhering to these rules is a significant challenge; most organizations lack the necessary data to quantify the impact of their supply chains, real estate, and electricity supply. Boavizta, an independent volunteer-led organization working to make such data publicly available, can help here (cf. examples of their work [here](https://dataviz.boavizta.org/manufacturerdata) and [here](https://dataviz.boavizta.org/cloudimpact)).\n\nSpecific PCRs are currently being developed for the cloud and data centers, which will have far-reaching consequences for CTOs, engineers and developers of all kinds. While these rules are currently only proposals being adopted by a few forward-thinking companies, they are likely to become French law by around 2025. This means **cloud providers will come under increased pressure to provide true, accurate and comparable data on the emissions generated by their activities**. Exposing this data will allow clients to choose the cloud provider with the lowest impact, thereby helping them to meet their own CSRD reporting requirements.\n\nIf this all seems somewhat abstract for now, it won’t be for long. AWS recently [came under scrutiny from UK regulators](https://www.computerweekly.com/news/365535550/AWS-under-fire-for-delays-in-delivering-Scope-3-GHG-emissions-data-to-enterprises-and-governments) for not providing timely scope 3 emissions data. The cloud leader quickly responded that such data would be available by “[early 2024](https://www.computerweekly.com/news/366536493/AWS-confirms-Scope-3-GHG-emissions-data-will-be-made-freely-available-to-customers-in-early-2024)”, and then [explained in detail](https://aws.amazon.com/fr/blogs/architecture/managing-data-confidentiality-for-scope-3-emissions-using-aws-clean-rooms/) how said data could already be shared directly with reporting companies (again, think CSRD), using **AWS Clean Rooms**.\n\n\n## How to get ready\n\nGreen IT implies all kinds of new ways of thinking about computing. However, as we’ve seen in [part 2](https://www.scaleway.com/en/blog/how-can-engineers-make-it-more-sustainable-part-2/), **green coding isn’t complicated or costly to put in place**. It merely requires clean, simple programming, which is both cheaper and more efficient. As a result, green IT may be more of an evolution than a revolution, but the question remains: how can your teams get ready for it?\n\nThe [Green Software for Practitioners (LFC131)](https://training.linuxfoundation.org/training/green-software-for-practitioners-lfc131/) course is the first of its kind, in that it **teaches developers and engineers about principles such as carbon awareness, energy efficiency, and making the most of hardware**, allowing them to apply green software principles to their own application development. Conceived by the Green Software Foundation (GSF), this free online course has already been followed by over 50,000 engineers. It’s a great first step in getting development teams to think more deeply about lowering the impact of their work.\n\nBesides training, there are countless ways to measure your activity’s impact, be it [Cloud Carbon Footprint](https://www.cloudcarbonfootprint.org/) or [Scaphandre](https://github.com/hubblo-org/scaphandre) for the cloud, Boavizta’s [Manufacturer Data Repository](https://dataviz.boavizta.org/manufacturerdata) for hardware, or the GSF’s [Software Carbon Intensity](https://greensoftware.foundation/articles/software-carbon-intensity-crafting-a-standard) (SCI) score for software.\n\nFurthermore, new tools emerge on a regular basis. IBM’s new [Cloud Carbon Calculator](https://newsroom.ibm.com/2023-07-26-IBM-Cloud-Carbon-Calculator-Helps-Organizations-Advance-Sustainability-Objectives-and-Address-Greenhouse-Gas-Emissions?utm_id=Cloud23-2021-26-07-Carbon-Cloud-Calculator-Social-Post\u0026sf180422067=1), for example, draws on AI-powered insights… notably to help clients manage the (considerable) impact of AI computing. \n\n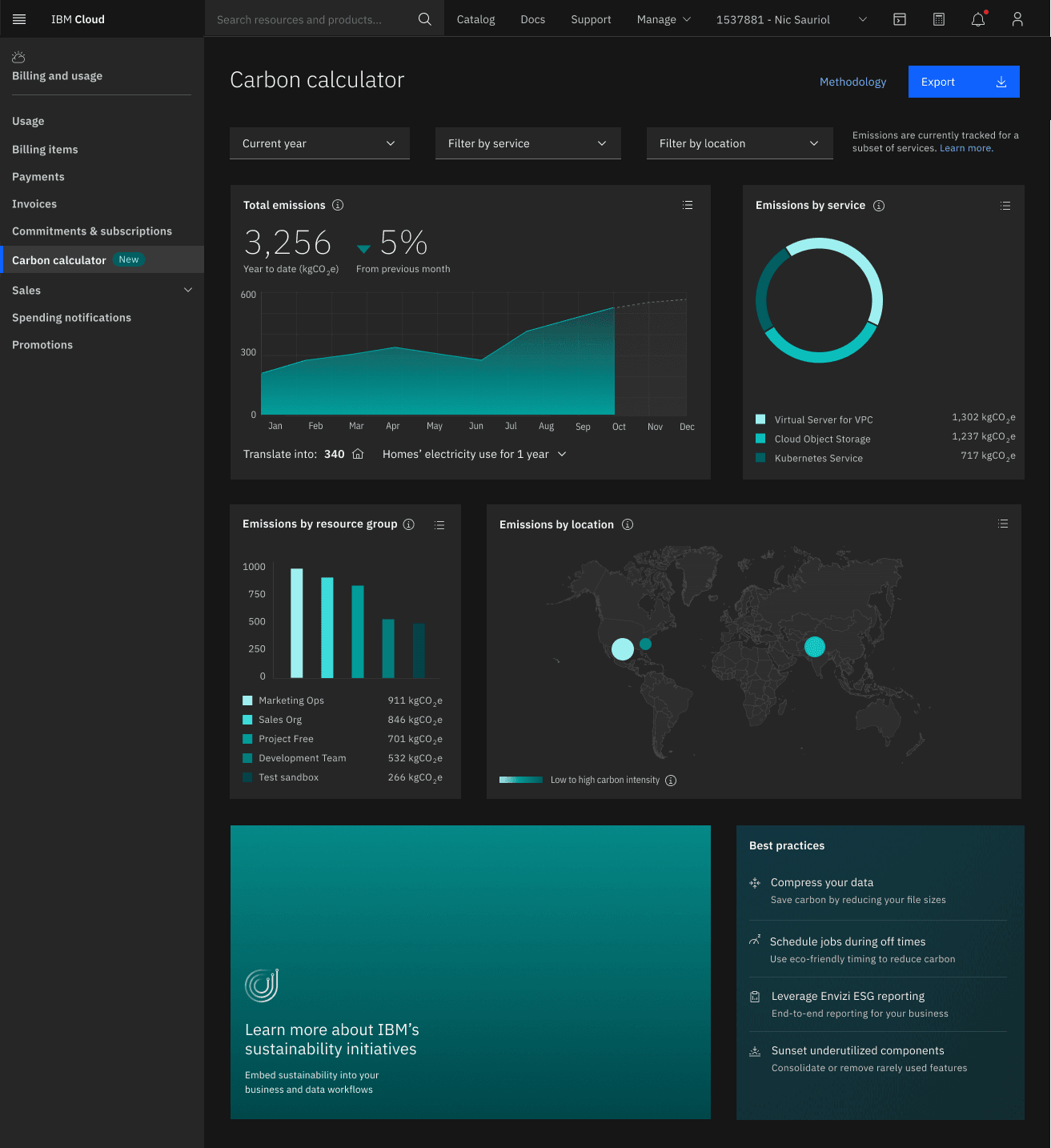\n\nThe tool is “designed to quickly spot patterns, anomalies and outliers in data that are potentially associated with higher GHG (greenhouse gas) emissions,” says the product’s press release. “Based on technology from IBM Research and through a collaboration with Intel, **the tool uses machine learning and advanced algorithms to help organizations uncover emissions hot spots in their IT workload**”. Furthermore, like AWS’ example above, IBM’s Cloud Carbon Calculator can provide tailor-made data for impact reporting.\n\nIt joins a number of SaaS and open-source tools already available to measure cloud impact, with the addition of AI suggesting that competition between makers of such tools will intensify in the near future, to end users’ certain benefit.\n\n\n## Making AI more sustainable\n\nAs IBM’s survey points out, just as many CEOs are investing in generative AI today (43%) as are concerned about sustainability (42%). A potential contradiction in terms when we consider the impact of this fast-growing new technology. \n\nWhilst estimates vary widely, [researchers have calculated that](https://arxiv.org/abs/1906.02243) **training one type of medium-sized generative AI model consumed energy equivalent to 284 tons of CO2 emissions**, or the same as 32 French people in one year. And whilst the training stage is the most energy-intensive, usage, or inference of models like GPT-3 or 4 also has impact: [other researchers estimate](https://www.euronews.com/green/2023/04/20/chatgpt-drinks-a-bottle-of-fresh-water-for-every-20-to-50-questions-we-ask-study-warns) that **having a 20 to 50-question conversation with ChatGPT is like emptying a half-liter bottle of water on the floor** (this being the amount of water used to cool the data centers in which large language models run).\n\nAgain, not all models are as resource-hungry… and, as in other cases, there are ways to measure this impact, in addition to choosing more planet-friendly models. **Using Stable Diffusion for 150,000 hours, for example, produces ‘just’ 11 tons of CO2 equivalent**, according to research by [Hugging Face] (which happens to be one of the AI companies most actively advocating to limit its sector's environmental impact).\n\nThis figure was obtained thanks to the [Machine Learning Emissions Calculator](https://mlco2.github.io/impact/#compute), an independent tool made by ML experts concerned about AI’s environmental impact. It calculates across a range of different hardware types, usage time, cloud provider and region, to give an estimate of the carbon (kg CO2 equivalent) emitted per workload. \n\n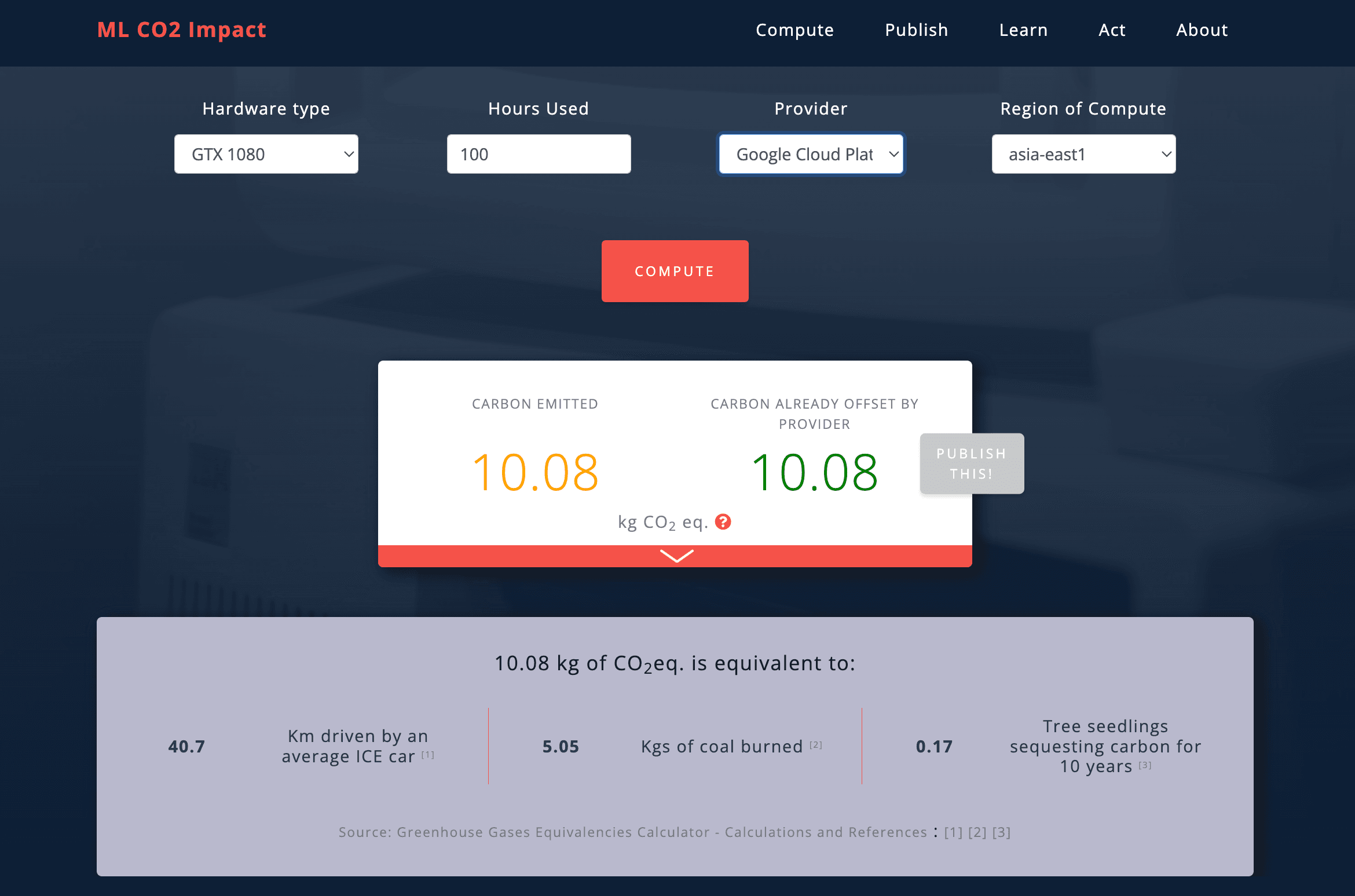\n\nNo tool is perfect, however: this one doesn’t have cloud providers’ PUE data, which means the total carbon emissions it provides have to be multiplied by that number. But still, it’s a start!\n\nHow can AI’s impact be reduced? Advice given by the makers of the ML calculator, applicable by most IT professionals, is essentially common sense:\n- **Choose the right cloud provider**, that clearly states its environmental commitments, and ideally only uses renewable energy\n- **Choose the right region**: the carbon intensity of electricity in France, for example, is over ten times lower than in the US (we’d add that time of day is also important here, as a grid’s carbon mix can vary enormously in the course of 24 hours)\n- Buy carbon offsets \u0026 **push for more transparency** in terms of impact reporting (we’d argue the latter is more effective than [the former](https://www.theguardian.com/environment/2023/jan/18/revealed-forest-carbon-offsets-biggest-provider-worthless-verra-aoe)).\n\n\n\nAjay Kumar, of EMYLON Business School, and Tom Davenport, of Babson College, authors of the aforementioned [Harvard Business Review](https://hbr.org/2023/07/how-to-make-generative-ai-greener) article, share some broader tips:\n- **Use existing large generative models rather than generating your own**, as the latter will provoke unnecessary emissions; existing models can also be fine-tuned to meet specific needs, rather than reinventing the wheel\n- **Use and train LLMs only when they generate extra added value**. For example, “if the usage of a 3x more power-hungry system increases the accuracy of a model by just 1–3%, then it is not worth the extra energy consumption”, say Kumar and Davenport\n- Be discerning about when you use generative AI (GAI). Current hype means GAI is often touted as a magic wand answer to everything, but it’s not. “**Tools just for generating blog posts or creating amusing stories may not be the best use for these computation-heavy tools**”, say the authors. “They may be depleting the earth’s health more than they are helping its people”, unlike applications in health or disaster avoidance, for example.\n\n\nFurthermore, alternatives exist. France’s [Golem.ai](https://golem.ai/en/)’s systems, for example, **use 1000 times less energy than GPT-3. Principally because they are based on symbolic AI**, which uses high-level representations of problems, rather than the massive amounts of internet-scraped data that is used to train generative AI. Additionally, **symbolic AI requires no training**, and therefore consumes no energy before it can be used, in contrast to generative AI’s energy-intensive training stage.\n\n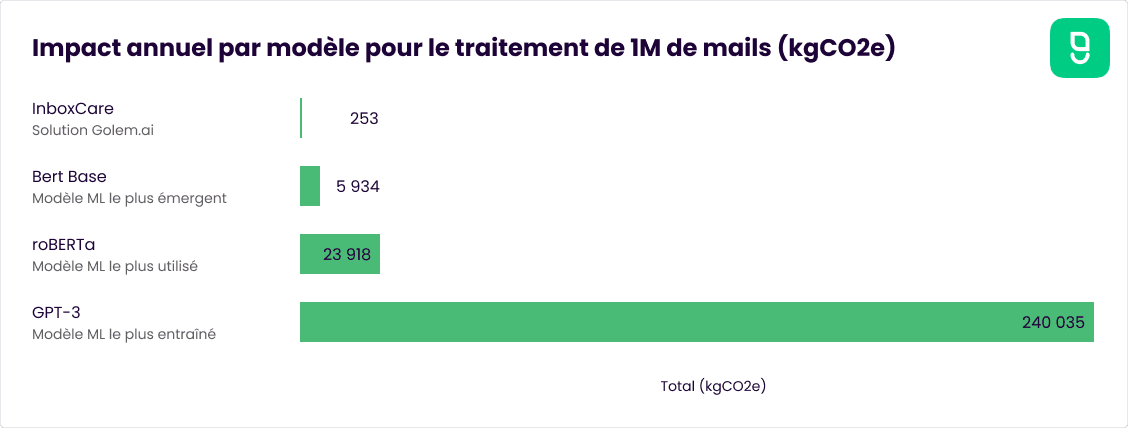\n\nThe “1000 times less” figure was obtained after an independent assessment by sustainability accountants Greenly, which studied the emissions generated by several different AI models to process 1 million emails. Whilst Golem.ai’s InboxCare generated 253 kg of CO2 equivalent, roBERTa generated roughly 100 times more, and GPT-3, almost 1000 times more, when that impact is amortized over one year.\n\n\n## Takeaways\n\nTo conclude, in green IT there’s no single ‘right’ path to take, but a combination of:\n- **Sensible, simple coding** that only uses necessary resources at all times\n- **Avoiding heavyweight applications**, websites and OSs that become obsolete every two years’ time\n- **Favoring hardware that does the required job for as long as possible**, rather than throwing out perfectly functional existing tech whenever fancy new tech is available\n- **Choosing a cloud provider that allows for sustainable computing** via renewable energy, concentrating workloads in places and times with the cleanest possible energy, and deleting unneeded data (cf. GCP, above)…\n\n\n…are bound to deliver concrete results when looking to reduce the impact of your organization’s computing activities. \n\nGreen IT may just be getting off the ground, but its principles are accessible to all, at no extra cost. Indeed, the savings can be considerable, both for your budget, and for the planet. So now’s the time to act!\n\n\n_That’s a wrap! Let’s keep the Green IT conversation going! Watch this space…_\n\n_This blogpost is extracted from the Scaleway white paper \"How can engineers make IT more sustainable?\", which you can [download in full for free here](https://www.scaleway.com/en/why-shift-to-green-it?utm_campaign=greenitwplaunchen0923\u0026utm_medium=socialmedia\u0026utm_source=blog)!_\n\n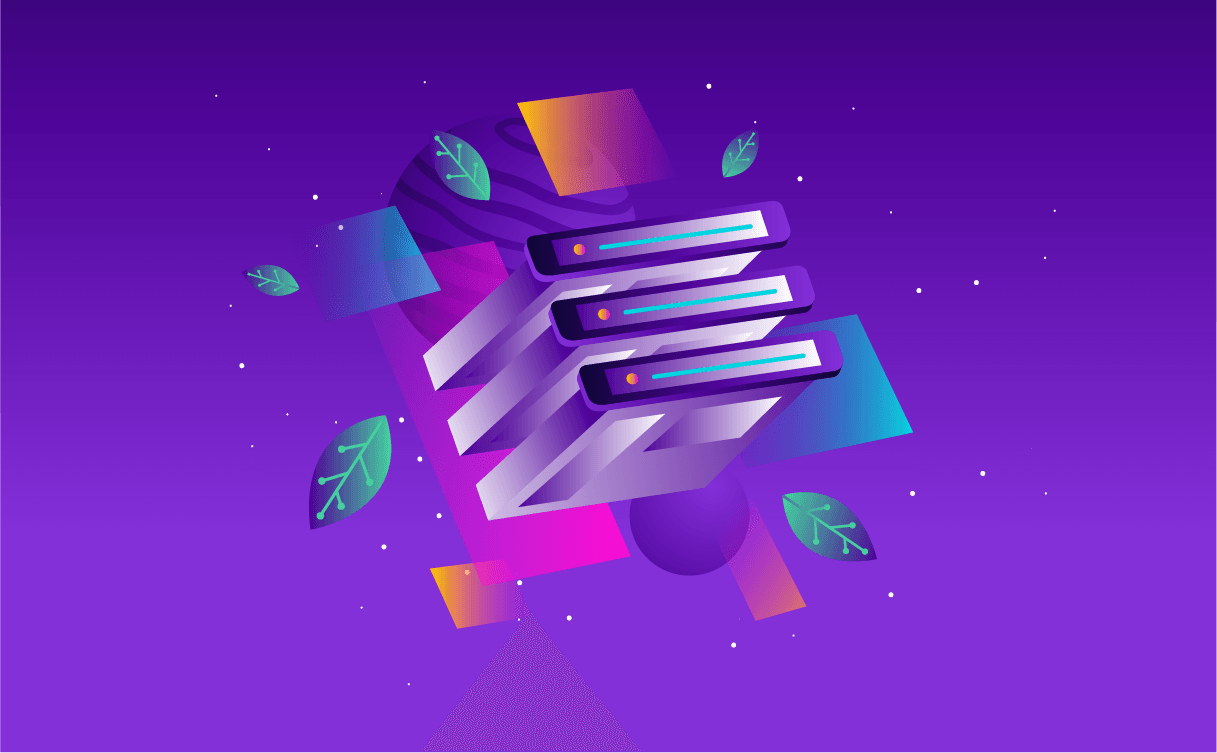","createdAt":"2023-08-10T08:28:31.133Z","updatedAt":"2023-11-08T12:54:48.510Z","publishedAt":"2023-08-10T08:39:57.173Z","locale":"en","tags":"Sustainability\nGreen IT\nDiscover","popular":false,"articleOfTheMonth":false,"category":"Build","timeToRead":7,"excerpt":"What is next for Green IT? Regulation, and 2050 carbon neutrality targets, will drive a shift from nice-to-have to must-have. And then there's AI...","author":"James Martin","h1":"How can engineers make IT more sustainable? Part 4: What to do next","createdOn":"2023-08-10"}}]},"meta":{"id":1343,"title":"How sustainable is AI?","description":"Do generative AI's benefits for the planet outweigh its impacts? Let's try to find out...","ogtype":null,"ogtitle":null,"ogdescription":"Do generative AI's benefits for the planet outweigh its impacts? Let's try to find out...","noindex":false},"localizations":{"data":[{"id":426,"attributes":{"title":"ia-est-elle-durable","path":"ia-est-elle-durable/","description":"Un peu plus d'un an après l'explosion de l'IA générative suite au lancement de ChatGPT, il est difficile de se souvenir d'une époque sans ces outils révolutionnaires. Toutefois, il reste à voir si la vitesse fulgurante du changement nous a laissé suffisamment de recul pour évaluer pleinement l'impact réel de l'IA générative sur la planète. Voyons donc ce qu'il en est.\n\n\n## L'impact, en chiffres\n\nIl est désormais bien établi que l'IA générative nécessite une puissance de calcul considérablement plus importante que les standards actuels. En grande partie parce que **l'apprentissage des modèles d'IA générative nécessite généralement des GPU plutôt que des CPU. Les premiers requièrent environ quatre fois plus d'énergie que les seconds** (par exemple, les CPU d'Ampere conçus pour l'IA consomment [3 à 5 fois moins d'énergie que les GPUs NVIDIA équivalentes](https://www.scaleway.com/en/blog/why-cpus-also-make-sense-for-ai-inference/)).\n\nEn outre, comme **les GPU d'IA ont tendance à générer 2,5 fois plus de chaleur que les CPU** (les CPU standard utilisés dans le cloud computing ont un TDP de 250 à 350 W, alors que les GPU ont un TDP de 750 à 800 W, cf. [Intel](https://www.intel.com/content/www/us/en/secure/care/products/237263/intel-xeon-gold-6554s-processor-180m-cache-2-2-ghz.html), AMD [x2](https://www.amd.com/fr/products/accelerators/instinct/mi300/mi300a.html) et [NVIDIA](https://www.nvidia.com/fr-fr/data-center/h100/)), ils ont besoin d'autant de puissance de refroidissement en plus. Ainsi, il est clair que les processeurs nécessaires à l'apprentissage et à l'inférence de l'IA générative sont considérablement plus gourmands en énergie que les modèles d'IA pré-générative.\n\nEnsuite, il y a la différence entre la formation et l'inférence. En ce qui concerne la première - le processus requis pour \"éduquer\" un modèle d'IA génératif en lui fournissant autant de données que possible - les émissions générées par la formation varient énormément en fonction du modèle :\n- **552 tCO2e** - GPT3.5, 1.3, 6 \u0026 175 milliards de paramètres ([source](https://dataforgood.fr/iagenerative/))\n- **284 tCO2e** - un LLM de taille moyenne, 213 millions de paramètres ([source](https://arxiv.org/abs/1906.02243))\n- **30 tCO2e** - BLOOM, un LLM frugal, 175 milliards de paramètres ([source](https://arxiv.org/pdf/2211.02001.pdf))\n\n_(tCO2e = tonnes d'équivalent CO2, à savoir le CO2 + les 3 autres gaz à effet de serre les plus puissants)_\n\n\nCela signifie que l'entraînement d'un modèle génératif d'IA peut générer l'équivalent des émissions annuelles de trois Français (10 tCO2e), jusqu'à 50.\n\nMais bien sûr, la formation n'est qu'un événement ponctuel, limité dans le temps. **L'inférence, ou l'utilisation quotidienne d'un modèle, a son propre impact, qui a été estimé à 200 fois plus élevé que celui de la formation**. Selon l'association technologique française [Data for Good](https://dataforgood.fr/iagenerative/), si l'on considère que ChatGPT compte [100 millions d'utilisateurs hebdomadaires](https://techcrunch.com/2023/11/06/openais-chatgpt-now-has-100-million-weekly-active-users/), cela représente 100 000 tCO2e/an pour GPT-3.5.\n\nPour donner un autre exemple, **créer une image avec de l'IA générative pourrait utiliser autant d'énergie que de recharger pleinement un smartphone**, d'après le dernier [livre blanc](https://arxiv.org/pdf/2311.16863.pdf) co-signé par Sasha Luccioni, Climate Lead and AI Researcher chez Hugging Face. Il faut cependant bien insister sur le conditionnel dans ce cas, comme le souligne [The Verge](https://www.theverge.com/24066646/ai-electricity-energy-watts-generative-consumption), étant donné la très grande variété de modèles GenAI déjà disponibles.\n\nEnsuite, il y a l'eau. Toujours en lien avec l'inférence, il a été établi qu'[une conversation avec ChatGPT consomme un demi-litre d'eau](https://arxiv.org/pdf/2304.03271.pdf) en termes de ressources de refroidissement du centre de données (cf. la chaleur considérable générée par les GPU, cf. ci-dessus). Sans parler de l'entraînement de GPT-3, qui a nécessité 5,4 millions de litres d'eau ([même source](https://arxiv.org/pdf/2304.03271.pdf)). Cela représente un peu plus d'un litre par heure d'entraînement (l'entraînement de GPT-3 a nécessité 4,6 millions d'heures de GPU, selon... [ChatGPT](https://www.wholegraindigital.com/blog/social-environmental-impacts-of-ai/) !)\n\nCompte tenu de ces éléments, on peut s’attendre à ce que la demande d'énergie pour l'IA dépasse à terme l'offre. \n\nSi Google devait utiliser l'IA pour ses quelque 9 milliards de recherches quotidiennes - ce qui arrivera [très probablement](https://www.theverge.com/2023/5/10/23717120/google-search-ai-results-generated-experience-io) - il lui faudrait 29,2 térawattheures (TWh) d'électricité chaque année, selon le chercheur Alex de Vries. Ainsi, comme l'a déclaré M. de Vries [à Euronews l'année dernière](https://www.euronews.com/next/2023/10/10/demand-for-ai-could-mean-technology-consumes-same-energy-as-a-country-analysis-shows), **d'ici 2027, l'IA pourrait consommer autant d'électricité qu'un pays de taille moyenne comme les Pays-Bas**.\n\nL'IAE (Association internationale de l'énergie) a récemment lancé [un avertissement similaire](https://iea.blob.core.windows.net/assets/6b2fd954-2017-408e-bf08-952fdd62118a/Electricity2024-Analysisandforecastto2026.pdf) : **la consommation d'énergie des centres de données pourrait plus que doubler d'ici 2026, pour atteindre 1 000TWh, sous l'effet de l'IA** et des crypto-monnaies.\n\nL'un des leaders les plus influents de l'IA l'a naturellement vu venir : à Davos en janvier 2024, le **PDG d'OpenAI, Sam Altman, a déclaré que l'IA aura certainement besoin de beaucoup plus d'énergie qu'on ne le pensait au départ**. \"Il n'y a aucun moyen d'y parvenir sans une percée énergétique [comme la fusion nucléaire]\", a-t-il déclaré lors d'une table ronde, selon [Reuters](https://www.usnews.com/news/technology/articles/2024-01-16/openai-ceo-altman-says-at-davos-future-ai-depends-on-energy-breakthrough). Cela pourrait bien être la raison pour laquelle l'investisseur le plus célèbre d'OpenAI, Microsoft, vient d'embaucher un nouveau directeur de l'accélération du développement nucléaire : pour \"aider à alimenter sa propre révolution de l'IA\", selon [TechRadar Pro](https://www.techradar.com/pro/microsoft-goes-atomic-worlds-most-valuable-company-just-hired-a-director-of-nuclear-development-acceleration-to-help-power-its-very-own-ai-revolution). \n\nBien que nous soyons encore loin [de la fusion nucléaire](https://www.newsweek.com/nuclear-fusion-when-ready-electricity-technology-1773349) - par rapport aux méthodes actuelles de fission - la piste des datacenters alimentés par énergie nucléaire se creuse de plus en plus en ce moment. \n\nSelon [Lisa Su, PDG d'AMD](https://www.theregister.com/2023/02/23/amd_zettaflop_systems_nuclear/), dans une dizaine d'années, nous pourrions voir apparaître **des superordinateurs de classe zettaflop, qui auront besoin de datacenters à 500 MW, soit 10 à 20 fois plus puissants que certaines des plus grandes installations actuelles**. De tels besoins ne peuvent être satisfaits que par des sources locales et spécialisées telles que les SMR (petits réacteurs modulaires) nucléaires.\n\nC'est pour cette raison que [The Register](https://www.theregister.com/2023/09/27/datacenters_nuclear_power/) rapporte que [Cumulus Data](https://cumulusinfra.com/) a ouvert l'année dernière un centre de données nucléaire de 65 MW qui, selon lui, atteindra à terme une capacité de 950 MW. En outre, des installations alimentées par des SMR sont actuellement étudiées par Green Energy Partners/IP3 (Virginie, États-Unis) et Bahnhof (Suède). \n\nCompte tenu de notre dépendance actuelle à l'égard des énergies fossiles (les États-Unis en dépendent encore pour 80 % de leur énergie), les datacenters nucléaires et sans émissions pourraient-ils être une meilleure option pour la planète que les solutions actuelles ? L'avenir nous le dira, surtout pour les générations futures…\n\n\n## Comment réduire cet impact\n\nLa première règle de toute stratégie de durabilité, en particulier dans le domaine de la technologie, devrait être de se demander \"**ai-je vraiment besoin de cela ?**\".\n\nEn effet, l'IA générative n'est ni inévitable, ni adaptée à tous les cas d'utilisation. Comme nous l'avons [déjà expliqué ici](https://www.scaleway.com/en/blog/symbolic-ai-is-dead-long-live-symbolic-ai/), l'IA symbolique peut être bien plus utile qu’on ne le pense, et bien moins impactant. La startup française Golem.ai a notamment établi que l'un de [ses modèles d'IA symbolique de tri d'emails émet 1000 moins de CO2eq que le GPT-3](https://golem.ai/fr/ia-frugalite-sobriete).\n\nCela dit, si l'IA générative s’avère indispensable pour vous, devez-vous absolument créer un modèle aussi imposant que ChatGPT ? Doit-elle aspirer toutes les données du monde, ou peut-elle se concentrer sur un ensemble de données spécialisées, comme les documents juridiques, par exemple ?\n\nDevez-vous absolument utiliser un supercomputer pour la formation, ou un simple [GPU H100](https://www.scaleway.com/en/h100-pcie-try-it-now/) plus petit ferait-il l'affaire ? Peut-être même que des GPU d'ancienne génération pourraient répondre à vos besoins ? \n\n**Pour l’inférence, un CPU moins gourmand en énergie qu’un GPU, comme ceux d'Ampere, pourrait-il faire l’affaire** (cf. ci-dessus) ? \n\nEnsuite, pourquoi ne pas s’inspirer des nombreuses façons dont l'IA générative est utilisée aujourd'hui pour faire avancer le développement durable ? \n\nEn effet, un [rapport de McKinsey](https://www.mckinsey.com/capabilities/quantumblack/our-insights/how-artificial-intelligence-can-deliver-real-value-to-companies) indique que les technologies basées sur l'IA pourraient aider les entreprises à réduire leurs émissions jusqu'à 10 % et leurs coûts énergétiques de 10 à 20 %.\n\nPar exemple, DeepMind, la filiale de Google spécialisée dans l'IA, qui a [déclaré](https://deepmind.google/discover/blog/deepmind-ai-reduces-google-data-centre-cooling-bill-by-40) **dès 2016 que son application de machine learning dans les centres de données GCP a permis à ces installations de consommer 40 % d'énergie en moins**. Comment ? En améliorant l'anticipation des facteurs internes clés, comme la façon dont les différents types de machines interagissent entre elles, et externes, comme la météo, grâce à des données d'entraînement telles que les températures, la puissance, la vitesse des pompes et les points de consigne antérieurs.\n\nL'impact de l'IA dans les centres de données peut également être réduit en utilisant des systèmes de refroidissement alternatifs. C'est le cas du **datacenter DC5 - où sont hébergées les machines d'IA de Scaleway - qui consomme 30 à 40 % d'énergie en moins que les installations standard, car il n'utilise pas de climatisation**. Il utilise le free cooling la plupart du temps et, pendant les mois d'été plus chauds, le refroidissement adiabatique, un processus qui refroidit l'air extérieur en le faisant passer à travers une membrane humide. Ceci, ajouté à la faible intensité carbone de l'énergie française, fait de DC5 l'une des installations d'IA les moins impactantes au monde.\n\n\n## L'IA ‘for good’ : Nous n'en sommes qu'au début\n\nLe machine learning peut également être utile dans des contextes plus larges, comme l'utilisation de données antérieures pour prédire la demande future d'électricité, optimisant ainsi les réseaux intelligents ; l'anticipation du trafic routier, qui peut rendre les déplacements et les livraisons plus efficaces, réduisant ainsi la pollution (Google affirme que son [initiative Green Light avec Google Maps](https://blog.google/outreach-initiatives/sustainability/google-ai-reduce-greenhouse-emissions-project-greenlight/) peut réduire les émissions aux intersections de 10 %) ; l'ajustement de la consommation d'énergie dans les bâtiments grâce à la prédiction de la température ; et la prévision d'événements ou d'incidents météorologiques extrêmes, comme [Pyronear](https://pyronear.org/en/), qui utilise des tours équipées d'IA pour détecter les incendies de forêt.\n\nTous ces exemples et bien d'autres encore - couvrant également les impacts sociétaux, l'analyse des politiques publiques, l'éducation et la finance - sont aujourd’hui une réalité grâce à l'IA générative. Ce [livre blanc](https://dl.acm.org/doi/10.1145/3485128) rédigé par des universitaires de renom et des leaders d'opinion de Google montre comment ces diverses activités accélèrent le développement durable dans son ensemble.\n\nIl y a ensuite les applications qui nous avertissent des urgences potentielles en matière de développement durable. Data for Good a notamment permis à l'ONG de protection des océans [Bloom](https://www.bloomassociation.org/en/) de détecter la pêche illégale à l'aide de l'IA, et a utilisé l'IA pour alimenter son site web [Carbonbombs.org](http://carbonbombs.org), qui signale les projets les plus polluants au monde, tels que les mines de charbon. Enfin, [Climatetrace.org](http://climatetrace.org) utilise l'IA pour mettre en évidence les pays qui ne décarbonisent pas aussi rapidement qu'ils le prétendent.\n\nL'IA générative peut également faciliter l'accès aux informations clés à propos de la durabilité. L'agence écologique nationale française (et client de Scaleway) [ADEME](https://www.linkedin.com/posts/lydia-passet-787a43159_ademe-iagaeznaezrative-genai-activity-7155594529746669570-xm_F/) teste actuellement un modèle textuel formé sur la conséquente base de documentation de l'agence, dans le but d'en extraire les données les plus importantes rapidement et facilement. Si l'expérience est concluante, le modèle pourrait à terme être ouvert au grand public. Mené par l'équipe \"AI for Sustainability\" d'**Ekimetrics**, ce projet est similaire à \"[ClimateQ\u0026A](https://huggingface.co/spaces/Ekimetrics/climate-question-answering)\", un modèle formé sur les rapports du GIEC, qui est sensiblement un **ChatGPT de la durabilité** (et pas le seul, cf. ce plugin ChatGPT plugin, \"[IPCC Explainer](https://chat.openai.com/g/g-CXYs3qu1D-ipcc-explainer)\"). \n\nSans oublier les modèles d'IA eux-mêmes : plus ils sont petits, moins ils consomment d'énergie, ce qui les rend meilleurs pour tout le monde, y compris pour la planète. Comme l'a [expliqué](https://www.scaleway.com/en/blog/best-quotes-ai-pulse-2023/) Arthur Mensch, PDG et cofondateur de Mistral AI, à ai-PULSE en novembre dernier, \"dans la version ‘Vanilla Attention’ de Transformers, il faut garder les jetons en mémoire. **Avec le modèle \"Sliding Window Attention\" de Mistral AI, il y a quatre fois moins de jetons en mémoire, ce qui réduit la pression sur la mémoire et permet donc d'économiser de l'argent. Actuellement, l'IA générative utilise trop de mémoire**\". C'est notamment pour cette raison que le dernier modèle de l'entreprise, Mistral-7B, peut fonctionner localement sur un smartphone (récent).\n\nÀ l'avenir, cette approche axée sur l'efficacité s'appliquera également aux offres de solutions d'IA. Scaleway vise à lancer en version bêta d'ici à la mi-2024 un service d'inférence qui servira les LLM connectés aux données privées ou sensibles des clients. Basé sur des LLM tels que Llama ou ceux de Mistral AI, un tel service est rentable en termes de coûts et d'énergie :\n- Les ressources informatiques peuvent être augmentées ou réduites par les utilisateurs en fonction des pics d'utilisation.\n- L'utilisation de modèles pré-entraînés existants et de sources ouvertes permet d'éviter les émissions générées par l'entraînement de nouveaux modèles à partir de zéro.\n\n\n## Conclusion : il est trop tôt pour se prononcer\n\nSi l'on considère qu'il y a autant de solutions d'IA que d'impacts, il est trop tôt pour établir un verdict dans un sens comme dans un autre. La plupart des experts s'accordent à dire qu'il est trop tôt dans la révolution de l'IA générative pour mesurer son véritable impact sur la planète.\n\nMais il y a des bonnes nouvelles ! Tout d'abord, il est tout à fait possible d'évaluer et de minimiser l'impact avant de choisir le modèle d'IA adapté à vos besoins, à savoir :\n- Mesurer l'impact de ses émissions, à l'aide d'outils tels que le [Machine Learning Emissions Calculator](https://mlco2.github.io/impact/%23compute)\n- Si vous pouvez réutiliser ou affiner un modèle existant - près de 500 000 modèles différents sont disponibles dans des référentiels tels que [Hugging Face](https://huggingface.co/models) - cela consommera beaucoup moins d'énergie que d'en créer un nouveau à partir de zéro.\n- Préférer un fournisseur de cloud qui s'efforce de réduire sa consommation d'énergie - et qui le prouve avec des [données chiffrées](https://www.scaleway.com/fr/leadership-environnemental/) - et dont les centres de données se trouvent dans un pays à faible intensité de carbone.\n\nDe plus, sachez que les principes de [Green IT](https://www.scaleway.com/en/why-shift-to-green-it/) s'appliquent tout autant à l'IA qu'à l'informatique \"traditionnelle\" :\n- Les **datacenters** devraient utiliser des énergies renouvelables - et le moins d’énergie possible - tout en limitant radicalement l'utilisation de l'eau. Ils peuvent aussi utiliser des systèmes de refroidissement autres que la climatisation, ce qui réduit considérablement l'impact environnemental de l'IA (voir ci-dessus).\n- Le **hardware** doit être optimisé pour consommer le moins d'énergie possible et durer le plus longtemps possible.\n- Le **software** - en l'occurrence les modèles d'IA, ou des options telles que l'inférence en tant que service - doivent être conçues pour consommer le moins possible de ressources informatiques, et donc d'énergie.\n\n\nEnfin et surtout, les ensembles de données, les modèles et les machines d'IA ne devraient avoir que la taille et la puissance nécessaires. Sinon, l'éternel risque de tomber dans l'effet rebond, ou [paradoxe de Jevon](https://en.wikipedia.org/wiki/Jevons_paradox) - utiliser un service plus, plutôt que moins, au fur et à mesure qu'il devient plus efficace - pourrait avoir des conséquences désastreuses. \n\nComme le [dit](https://vert.eco/articles/lintelligence-artificielle-va-t-elle-donner-le-coup-de-grace-au-climat?utm_source=pocket_saves) Theo Alves da Costa, responsable de l'IA pour le développement durable chez Ekimetrics, \"si nous utilisons le bulldozer de l'IA pour enfoncer un clou dans un mur, le clou entrera, mais nous courons aussi le risque de faire tomber tout le mur. Dans ce cas, il vaut mieux utiliser un marteau\".\n\n\n_Un grand merci à [Theo Alves Da Costa](https://www.linkedin.com/in/th%C3%A9o-alves-da-costa-09397a82/) d’Ekimetrics, dont beaucoup de sources ont inspiré cet article. Et à [Sasha Luccioni](https://www.sashaluccioni.com/) de Hugging Face pour la motivation initiale !_","createdAt":"2024-02-15T10:29:58.151Z","updatedAt":"2024-03-11T09:35:03.907Z","publishedAt":"2024-02-15T10:35:51.350Z","locale":"fr","tags":"AI\nSustainability\nGreen IT","popular":false,"articleOfTheMonth":false,"category":"Build","timeToRead":6,"excerpt":"Les bénéfices de l'IA générative pour la planète dépassent-ils ses impacts ? Voyons voir...","author":"James Martin","h1":"L'IA est-elle durable?","createdOn":"2024-02-15"}}]}}},{"id":428,"attributes":{"title":"infrastructures-for-llms-in-the-cloud","path":"infrastructures-for-llms-in-the-cloud/","description":"Open source makes LLMs (large language models) available to everyone. There are plenty of options available, especially for inference. You’ve probably heard of [Hugging Face’s inference library](https://huggingface.co/docs/huggingface_hub/package_reference/inference_client), but there’s also [OpenLLM](https://github.com/bentoml/OpenLLM), [vLLM](https://docs.vllm.ai/en/latest/), and many others. \n\nThe main challenge, especially if you’re a company like Mistral AI building new LLMs, is that the architecture of your LLM has to be supported by all these solutions. They need to be able to talk to Hugging Face, to NVIDIA, to OpenLLM and so on.\n\nThe second challenge is the cost, especially that of the infrastructures you’ll need to scale your LLM deployment. For that, you have different solutions: \n\n1. Choosing the right GPUs (your LLM has to fit with them)\n2. Choosing the right techniques:\n- Quantization, which involves reducing the number of bytes used by the variables, so you can fit larger models into smaller memory constraints. That’s a give and take between the two, as that can have impacts on the accuracy of your model and its performance results\n- Fine-tuning methods, like parameter-efficient fine-tuning ([PEFT](https://github.com/huggingface/peft)). With PEFT methods, you can significantly decrease computational and memory cost by only fine-tuning a small number of (extra) model parameters instead of all the model's parameters. And you can combine PEFT methods with quantization too.\n\n\nThen you have to decide whether you host it yourself; you use a PaaS solution; or ready-to-use API endpoints, like what OpenAI does.\n\n\n## Choosing the right GPU\n\n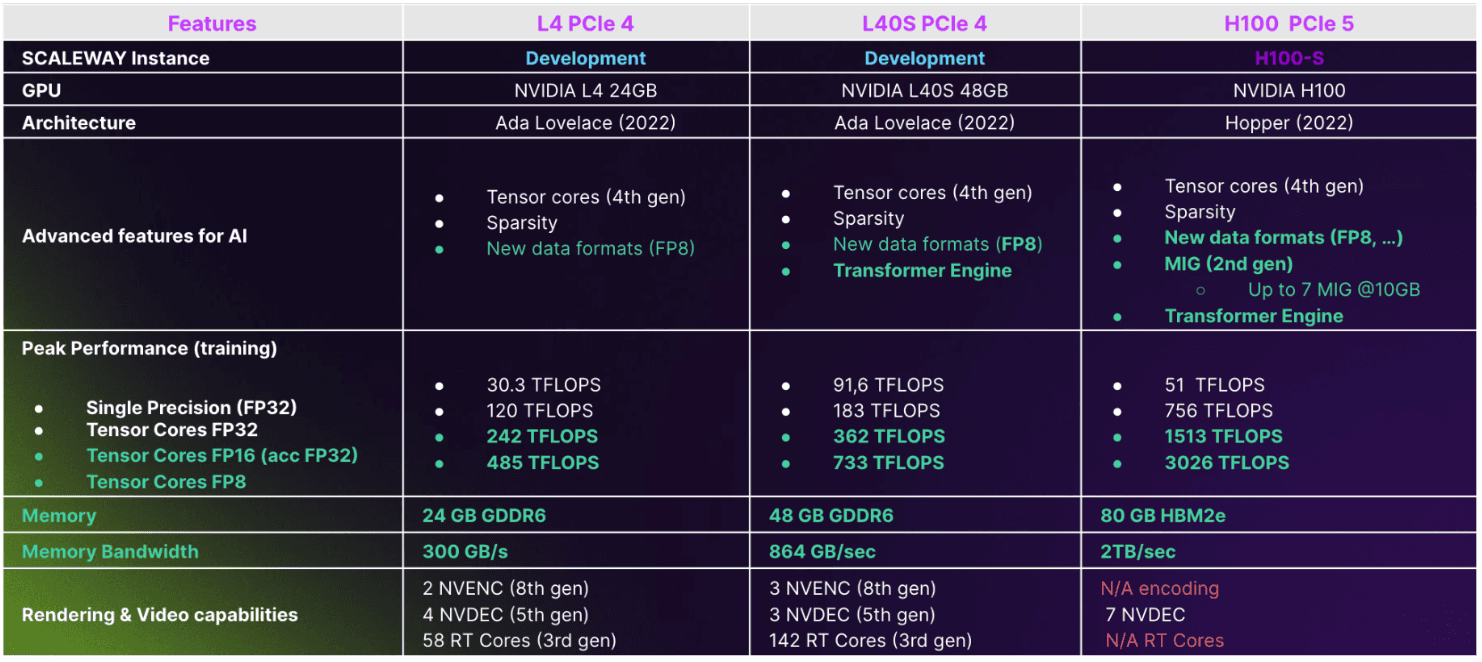\n\nThe above is Scaleway’s offering, but similar offerings are currently being installed with most major cloud providers. \n\n- **H100 PCIe 5** is the flagship, NVIDIA’s most powerful GPU. It has interesting features like the Transformer Engine, a library for accelerating Transformer models on NVIDIA GPUs, including using 8-bit floating point (FP8) precision on Hopper and Ada Lovelace GPUs, to provide better performance with lower memory utilization in both training and inference. It speeds up training of Transformer models, meaning you can put twice the amount of variables in memory, in 8 bits instead of 16. Furthermore, NVIDIA’s Library helps make these changes simpler; plus a large amount of memory and memory bandwidth are key, as the faster you can load your memory, the faster your GPU will be\n- **L4 PCIe 4** can be seen as the modern successor to the NVIDIA T4, intended for inference, but perfectly capable of training smaller LLM models. Like H100, it can manage new data formats like FP8. It has less memory bandwidth than H100, but that may create some bottlenecks for certain use cases, like handling large batches of images for training computer vision models. In these cases, you may not see a significant performance boost compared with previous Ampere architecture for example. And unlike H100, this one has video and 3D rendering capabilities, so if you want to generate a synthetic dataset for computer vision with Blender, you can use this GPU\n- **L40S PCIe 4** is what NVIDIA considers as the new A100. It has twice the amount of memory as the L4, but with a larger memory bandwidth, and stronger compute performance too. For generative AI, according to NVIDIA, when you optimize your code with FP8 and so on, DGX with 8x A100 with 40 Gb NVlink can perform as well as 8 L40S PCIe 4 without NVLink, so that’s a powerful and interesting GPU.\n\n\n## Using GPU Instances tip 1: Docker images\n\n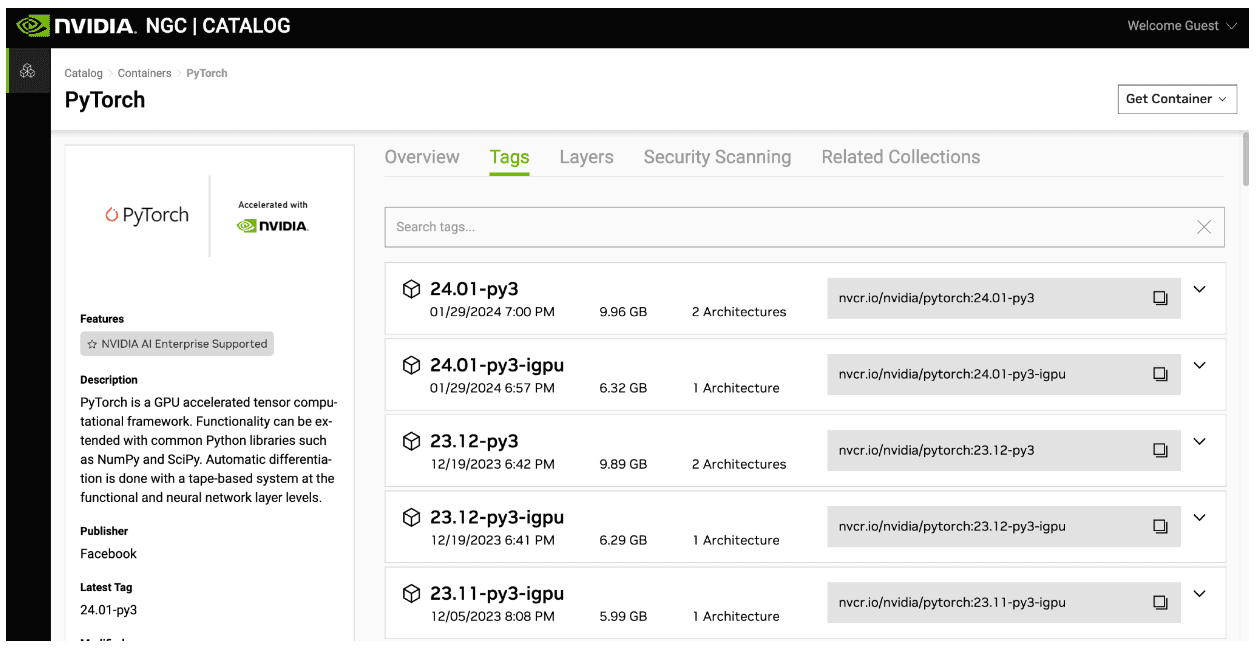\n\nWhen using GPUs, use Docker images, and start with those offered by NVIDIA, which are free. This way, the code is portable, so it can run on your laptop, on a workstation, on a GPU Instance (whatever the cloud provider, so without lock-in), or on a powerful cluster (either with SLURM as the orchestrator if you’re in the HPC/AI world, or Kubernetes if you’re more in the AI/MLOps world).\n\nNVIDIA updates these images regularly, so you can benefit from performance improvements and bug/security fixes. A100 performance is significantly better now than it was at launch, and the same will apply to H100, L4 and so on. Also, there are a lot of time-saving features, which will allow you to make POCs more quickly, like framework and tools like NeMo, Riva and so on, which are available through the NGC catalog (above). \n\nThis also opens up the possibility to use an AI Enterprise license on supported hardware configurations, which is something typically only seen in cloud provider offers), which will give you support in case you meet bugs or performance issues, and even offers help from NVIDIA data scientists, to help you debug your code, and to get the best performance out of all of these softwares. And of course, you can choose your favorite platform, from PyTorch, TensorFlow, Jupyter Lab and so on.\n\n\n### Using Scaleway GPU Instances\n\nIn Scaleway’s GPU OS 12, we’ve already pre-installed Docker, so you can use it right out of the box. I’m often asked why there’s no CUDA or Anaconda preinstalled. The reason is these softwares should be executed inside the containers, because not all users have the same requirements. They may not be using the same versions of CUDA, cuDNN or Pytorch, for example, so it really depends on the user requirements. And it’s easier to use a container built by NVIDIA than installing and maintaining a Python AI environment. Furthermore, doing so makes it easier to reproduce results within your trainings or experiments.\n\nSo basically, you do this:\n\n```js\n## Connect to a GPU instance like H100-1-80G\n\nssh root@\u003creplace_with_instance_public_ip\u003e\n\n## Pull the Nvidia Pytorch docker image (or other image, with the software versions you need)\n\ndocker pull nvcr.io/nvidia/pytorch:24.01-py3\n[...]\n\n## Launch the Pytorch container\n\ndocker run --rm -it --runtime=nvidia \\\n-p 8888:8888 \\\n-p 6006:6006 \\\n-v /root/my-data/:/workspace \\\n-v /scratch/:/workspace/scratch \\\nnvcr.io/nvidia/pytorch:24.01-py3\n\n## You can work with Jupyter Lab, Pytorch etc…\n```\n\nIt’s much easier than trying to install your environment locally.\n\n\n\n## Using GPU Instances tip 2: MIG\n\n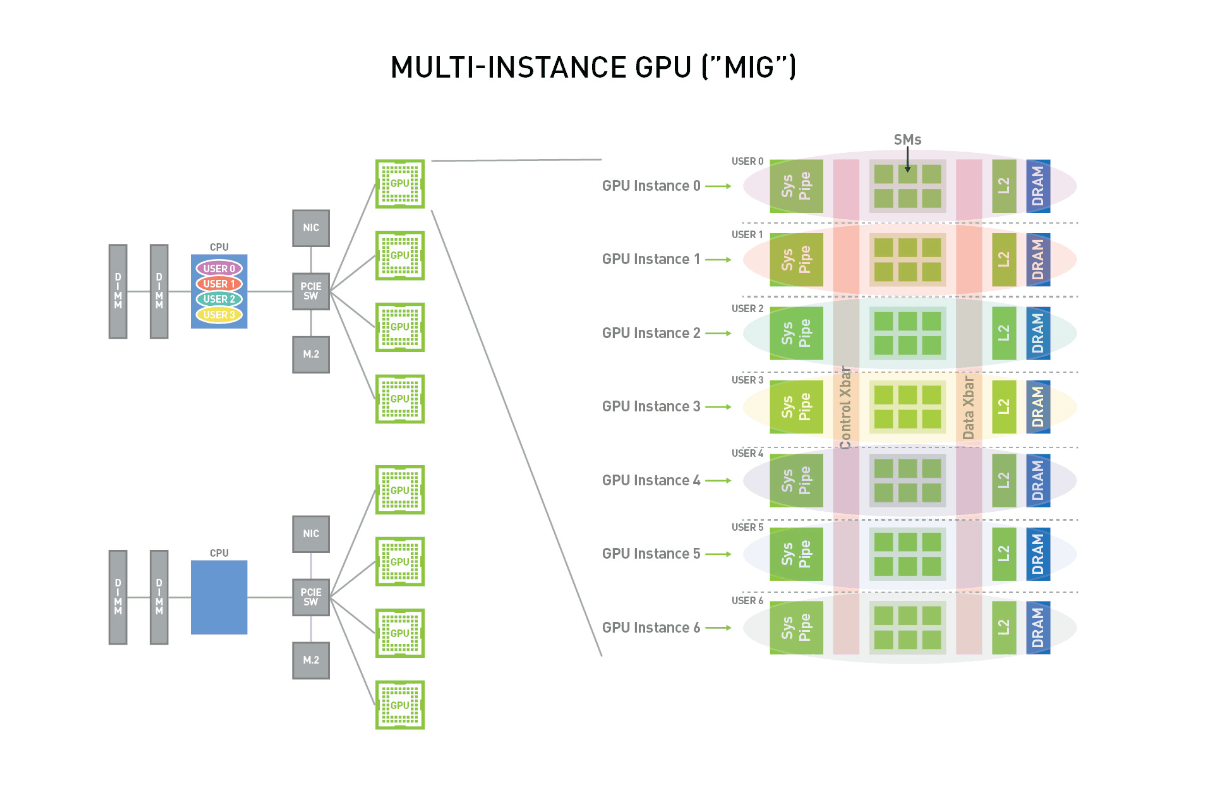\n\nOne unique feature of the H100 is [MIG, or multi-instance GPU](https://docs.nvidia.com/datacenter/tesla/mig-user-guide/index.html), which allows you to split your GPU into up to seven pieces. This is really useful when you want to optimize your workload. If you have workloads that don’t fully saturate GPUs, this is a nice way to have multiple workloads and maximize GPU utilization. It works with standalone VMs, and works really easily in Kubernetes. You request one GPU reference corresponding to the split you want to use for one GPU resource. \n\nIn Kubernetes, it’s is as easy as replacing in your deployment file the classic resource limits\n**nvidia.com/gpu: '1'**. by the desired MIG partition name, for **example, nvidia.com/mig-3g.40gb: 1**\n\n[Here’s the link](https://docs.nvidia.com/datacenter/tesla/mig-user-guide/index.html) if you want to look into that.\n\n\n\n## Using GPU Instances tip 3: NVIDIA Transformer Engine \u0026 FP8\n\n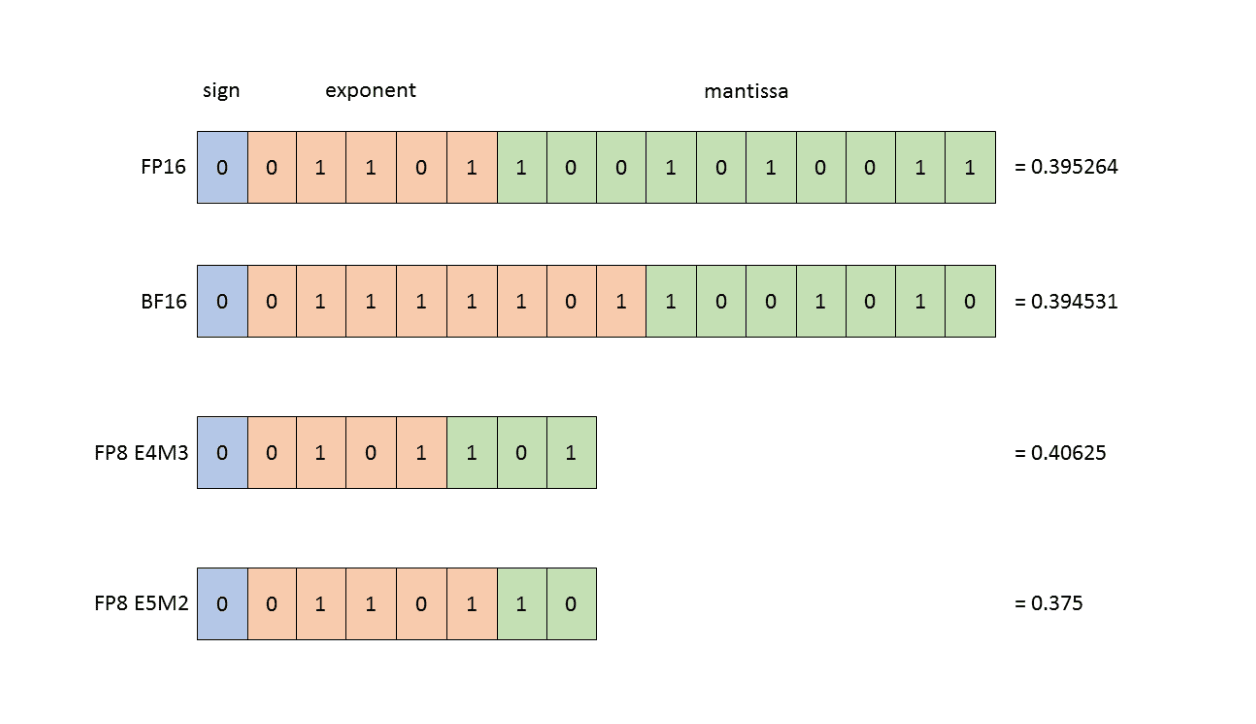\n\nAll the latest generation of GPUs (available in the latest Nvidia GPU architecture, namely Hopper and Ada Lovelace) use the NVIDIA Transformer Engine, a library for accelerating Transformer models on NVIDIA GPUs, including using 8-bit floating point (FP8) precision on Hopper and Ada GPUs, to provide better performance with lower memory utilization in both training and inference.\n\nAs for their use of the FP8 data format, there are actually two kinds of FP8, which offer a tradeoff between the precision and the dynamic range of the numbers you can manipulate (cf. diagram). When training neural networks, both of these types may be utilized. Typically forward activations and weights require more precision, so the E4M3 datatype is best used during forward pass. In the backward pass, however, gradients flowing through the network typically are less susceptible to the loss of precision, but require higher dynamic range. Therefore they are best stored using E5M2 data format. This can even be managed automatically with the 'HYBRID' format ([more information here](https://docs.nvidia.com/deeplearning/transformer-engine/user-guide/api/common.html#transformer_engine.common.recipe.Format)). \n\nThe Transformer Engine is not just for Transformers. As it can also optimize Linear operations, it can benefit other model architectures, like computer vision (cf. the [MNIST example](https://github.com/NVIDIA/TransformerEngine/tree/main/examples/pytorch/mnist)) So basically, you install the Transformer engine package with ‘pip’, load the package, and just test or replace certain operant modules (from your favorite deep learning frameworks) by the one provided in the Transformer engine package (cf. the MNIST example above). If you want to invest a bit of time in optimizing your code by using the Transformer Engine and the FP8 format in your code, you can. It’s good here to optimize, because you’ll use less memory, fit more variables, and speed up your inference and your training. So be sure to optimize your code!\n\n\n## Using LLMs in production: Creating an AI chatbot with RAG\n\n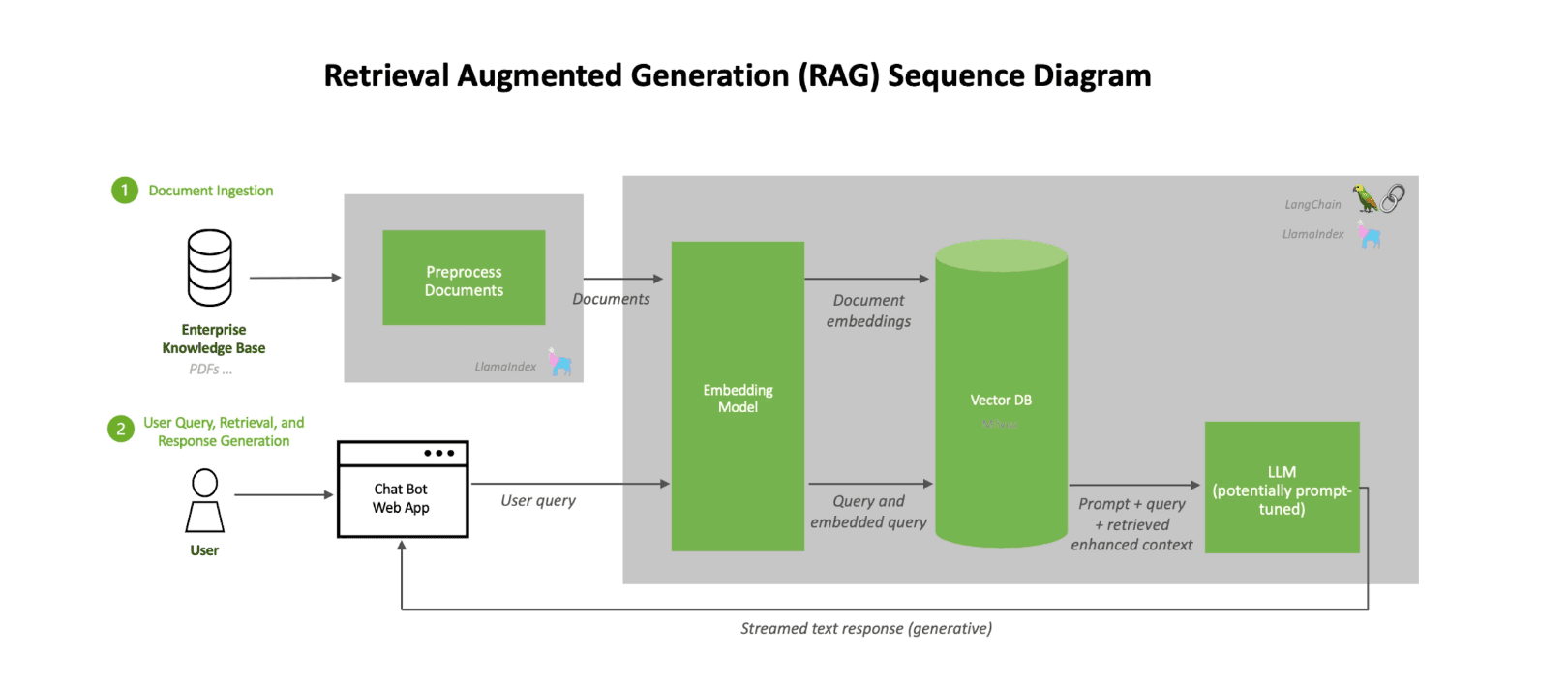\n\nIf you want to do LLMs in production, you might want to create a chatbot, and to do that, you’ll probably want to fine-tune a model on your data for your specific use case. It’s easy with Hugging Face’s Transformers library in terms of code; but it can be hard to improve your results, as this takes lots of trial and error. \n\nAnother technique is to look at RAG, or [Retrieval Augmented Generation](https://blogs.nvidia.com/blog/what-is-retrieval-augmented-generation/), which you can do before fine-tuning, or instead of it. This way there’s less risk of breaking the model, as is a risk with fine-tuning. Plus with RAG, there’s no fine-tuning cost, as you don’t pay for the GPU usage of the multiple tries that fine-tuning requires; and you can keep your data private by hosting it locally. Furthermore, you reduce the risks of hallucinations, which are always a bad thing when you’re trying to [build an AI chatbot for your business](https://www.theguardian.com/world/2024/feb/16/air-canada-chatbot-lawsuit). So I’ve included the [documentation](https://docs.nvidia.com/ai-enterprise/workflows-generative-ai/0.1.0/index.html) that explains this system. NVIDIA even has a [GitHub project](https://github.com/NVIDIA/GenerativeAIExamples/blob/main/examples/README.md) to allow you to build your first AI chatbot with RAG in just five minutes. \n\n\n## What you need to train a foundational LLM\n\nFirstly, a lot of money! LLaMA’s [white paper](https://arxiv.org/abs/2302.13971) says it took 21 days to train LLaMa using 2048 A100 80GB GPUs. We can't possibly speculate on what that costs, but [someone else has here](https://www.reddit.com/r/LocalLLaMA/comments/15ggfjl/cost_of_training_llama_2_by_meta/?rdt=58095) (hint: it's a lot!)\n\nYou’ll also need a team of experts… but not necessarily hundreds! Mistral AI’s Mixture beat GPT3.5 (according to [Mistral AI’s benchmark](https://mistral.ai/news/mixtral-of-experts/)) with a team of less than 20 people. \n\nLots of data will also be required: you may have to scrape the internet for that, or rely on a partnership to help you. Then the data will need to be prepared, i.e. cleaned and deduplicated.\n\nFinally, you’ll need lots of compute power! If we look at this NVIDIA graphic:\n\n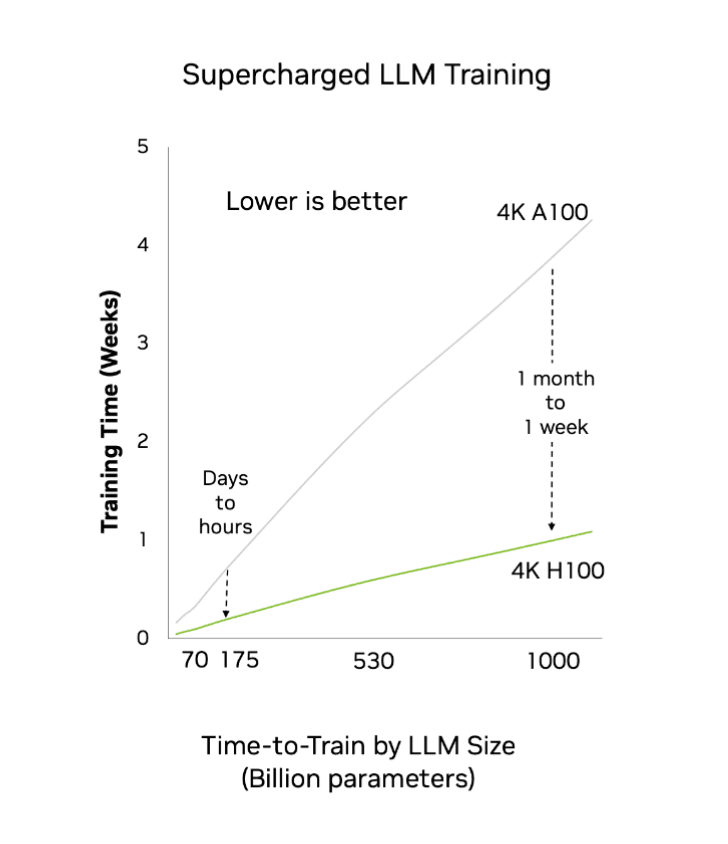\n\n…we see there’s a big leap between A100 and H100 (from one month to one week’s training time for the biggest models).\n\n\n## How to handle lots of data\n\nOur Superpod customers use Spark for the data preparation, which uses CPUs (in the range of 10 000 vCPUs), and around 100 TB of block storage, before the dataset is stored in Object Storage. Scaleway is currently working on a Spark managed cluster offer, by the way: watch this space!\n\nNVIDIA also provides tools like [NeMo data Curator](https://www.nvidia.com/en-us/ai-data-science/products/nemo/get-started/) (through NGC/Nvidia AI Enterprise, so we’re talking about containers), which has functions like data download and text extraction, text re-formatting and cleaning, quality filtering, document-level deduplication, multilingual downstream-task decontamination and more.\n\nEven with these tools, data preparation can take a long time, but it has to be done before you start the training.\n\n\n## How to start training\n\nTo start training, you’ll need more than one GPU, so the building blocks will be **NVIDIA DGX H100**, which are ready-to-use computers with a set maximal server configuration, so you’ve got the best of the best:\n\n- **8x NVIDIA H100 80GB GPUs With 640 Gigabytes of Total GPU Memory**\n- 18x NVIDIA® NVLink® connections per GPU\n- 900 gigabytes per second of bidirectional GPU-to-GPU bandwidth, thanks to NVLink\n- **4x NVIDIA NVSwitches™**\n- 7.2 terabytes per second of bidirectional GPU-to-GPU bandwidth\n- 1.5X more than previous generation\n- **10x NVIDIA ConnectX®-7 400 Gigabits-Per-Second Network Interface**\n- 1 terabyte per second of peak bidirectional network bandwidth\n- Dual Intel Xeon Platinum 8480C processors, 112 cores total, and 2 TB System Memory\n- 30 Terabytes NVMe SSD - High speed storage for maximum performance.\n\n\nTo build a Superpod, you take that server, then put 32 of them together, no more, no less. That's what NVIDIA calls a Scaleable Unit. If you scale up four scalable units, you have 128 nodes, and that’s the SuperPOD H100 system. Each of the four units is 1 ExaFLOPS of FP8 format for a total of up to 4 ExaFLOPS in FP8, and the cluster is orchestrated by NVIDIA Base Command Manager, so NVIDIA software, with a SLURM orchestrator, which can launch jobs across multiple computers to do the training.\n\nSo at Scaleway, we’ve got two [supercomputers](https://www.scaleway.com/en/ai-supercomputers/):\n\n**Jeroboam**, the smaller version of the cluster, which was intended to learn to write code that’s multi-GPU and multi-nodes:\n- **2 NVIDIA DGX H100 nodes (16 Nvidia H100 GPU)** \t\n- Up to 63,2 PFLOPS (FP8 Tensor Core)\n- 8 Nvidia H100 80GB SXM GPUs with NVlink up to 900 GB/s per node\n- Dual CPU Intel Xeon Platinum 8480C (112 cores total at 2GHz)\n- 2TB of RAM \n- 2x 1.92TB NVMe for OS\n- 30,72 TB NVMe for Scratch Storage\n\n- Throughput (for 2 DGX) : Up to 40 GB/s Read and 30 GB/s Write\n- Nvidia Infiniband GPU interconnect network up to 400 Gb/s (at cluster level)\n- 60TB of DDN high-performance, low latency storage.\n\n\n**Nabuchodonosor**, the ‘real thing’ for training, which is also built for people who’ll want to train LLMs with videos, not just text, thanks to the large amount of high-performance storage…\n- **127 NVIDIA DGX H100 nodes (1016 Nvidia H100 GPU)** \n- Up to 4 EFLOPS (FP8 Tensor Core)\n- 8 Nvidia H100 80GB SXM GPUs with NVlink up to 900 GB/s per node\n- Dual CPU Intel Xeon Platinum 8480C (112 cores total at 2GHz)\n- 2TB of RAM \n- 2x 1.92TB NVMe for OS\n- 30,72 TB NVMe for Scratch Storage\n\n- Nvidia Infiniband GPU interconnect network up to 400 Gb/s (at cluster level)\n- 1,8PB of DDN high-performance, low latency storage \n- Throughput (for 127 DGX) : Up to 2,7 TB/s Read and 1,95 TB/s Write\n\n\n## Training LLMs\n\n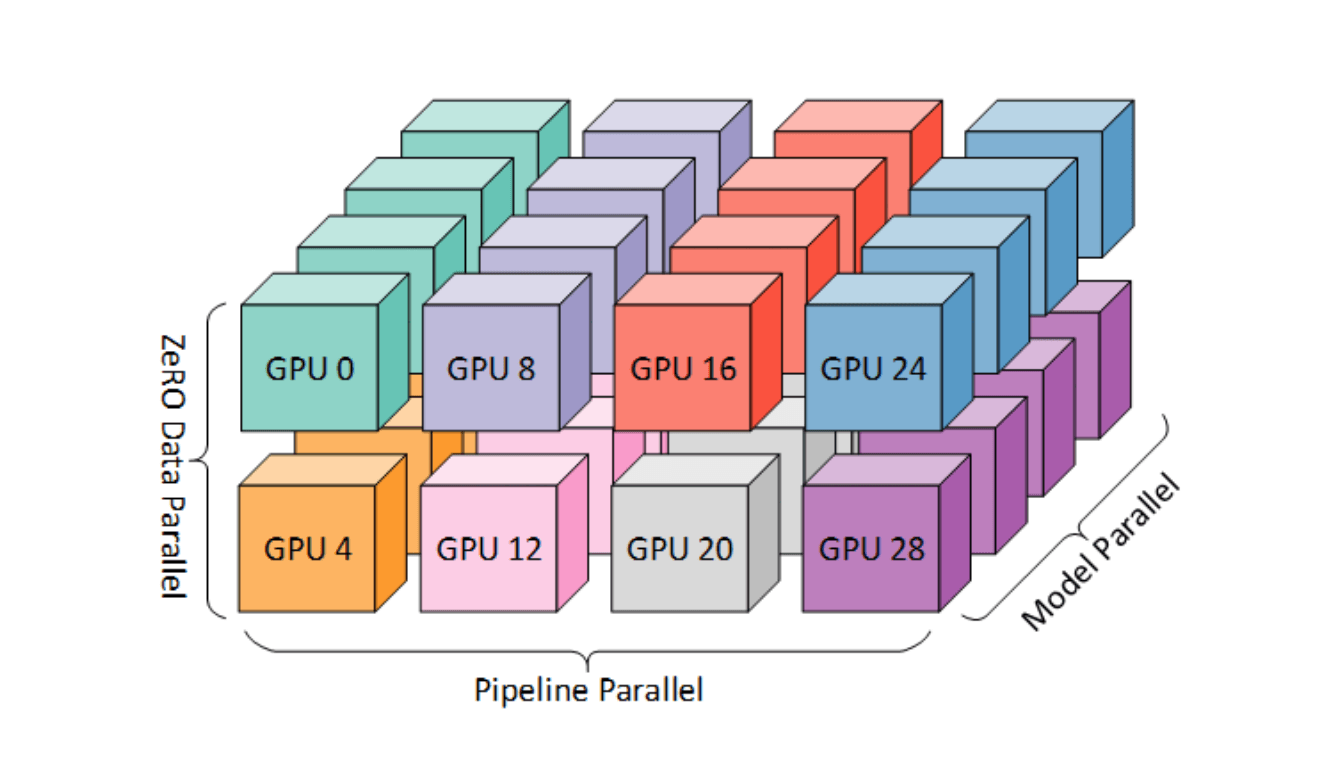\n\nThe challenge of training LLMs on Nabuchodonosor is that it’s an HPC user experience, which means SLURM jobs, not Kubernetes. It’s still containers, though, which you build on top of NVIDIA NGC container images (Pytorch, Tensorflow, Jax…). That’s why when you write your code with these NGC images, even with a single small GPU, your code will be able to scale more easily. One best practice is if you have, say, 100 nodes, don’t launch your jobs on all of them. Keep a few spare in case one or two GPUs fail (it happens!) That way, if you have any issues, you can relaunch your jobs by replacing the faulty nodes.\n\nYou’ll need to write your code in special ways, to maximize performance by using data parallelism and model parallelism (computing across multiple GPUs at the same time); you can use resources like [Deepspeed](https://www.deepspeed.ai/training/) for this.\n\nThen there’s the End-to-End framework [Nvidia NeMo](https://github.com/NVIDIA/NeMo), which will also help you build, finetune and deploy generative AI models.\n\n\n## Superpod challenges\n\n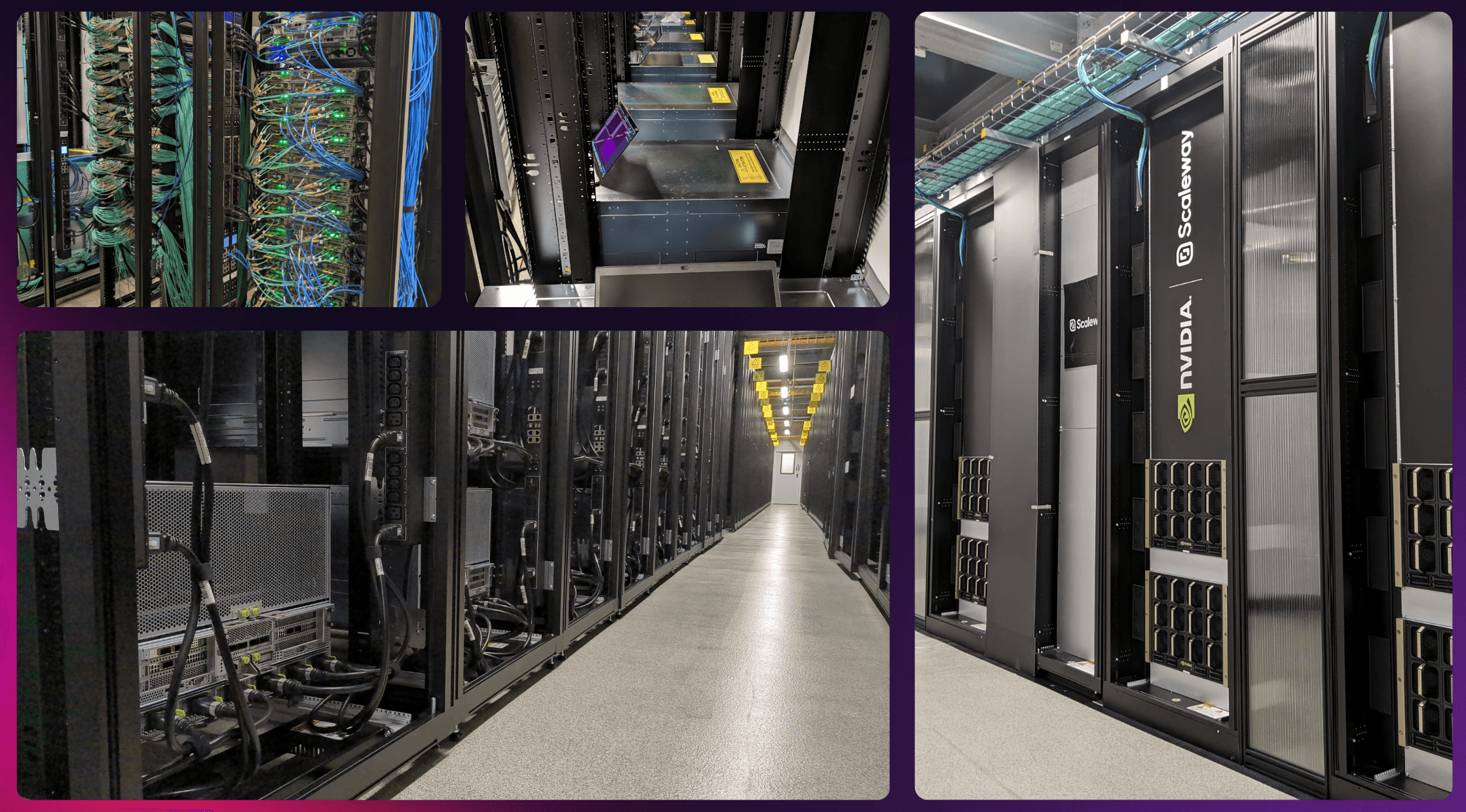\n\nScaleway’s supercomputers were built in just three to seven months, so it was quite a logistical challenge to make sure all the parts were received in time, and connected the right way… with more than 5000 cables! \n\nProviding power is also quite a challenge: the Nabuchodonosor Superpod system’s power usage is 1.2 MW, which means we can only put two DGX units in each rack, so it’s not a great usage of data center surface space. Then there’s the cost of electricity, which is five times more in France than in the USA, for example. But as French electricity’s carbon intensity is very low, it generates around seven times less emissions than in Germany, for example. Furthermore, as all of Scaleway’s AI machines are hosted in DC5, which has no air conditioning and therefore uses 30-40% less energy than standard data centers, we can say this is one of the world’s most sustainable AI installations. [More on AI and sustainability here](https://www.scaleway.com/en/blog/how-sustainable-is-ai/).\n\n\n## What’s next?\n\n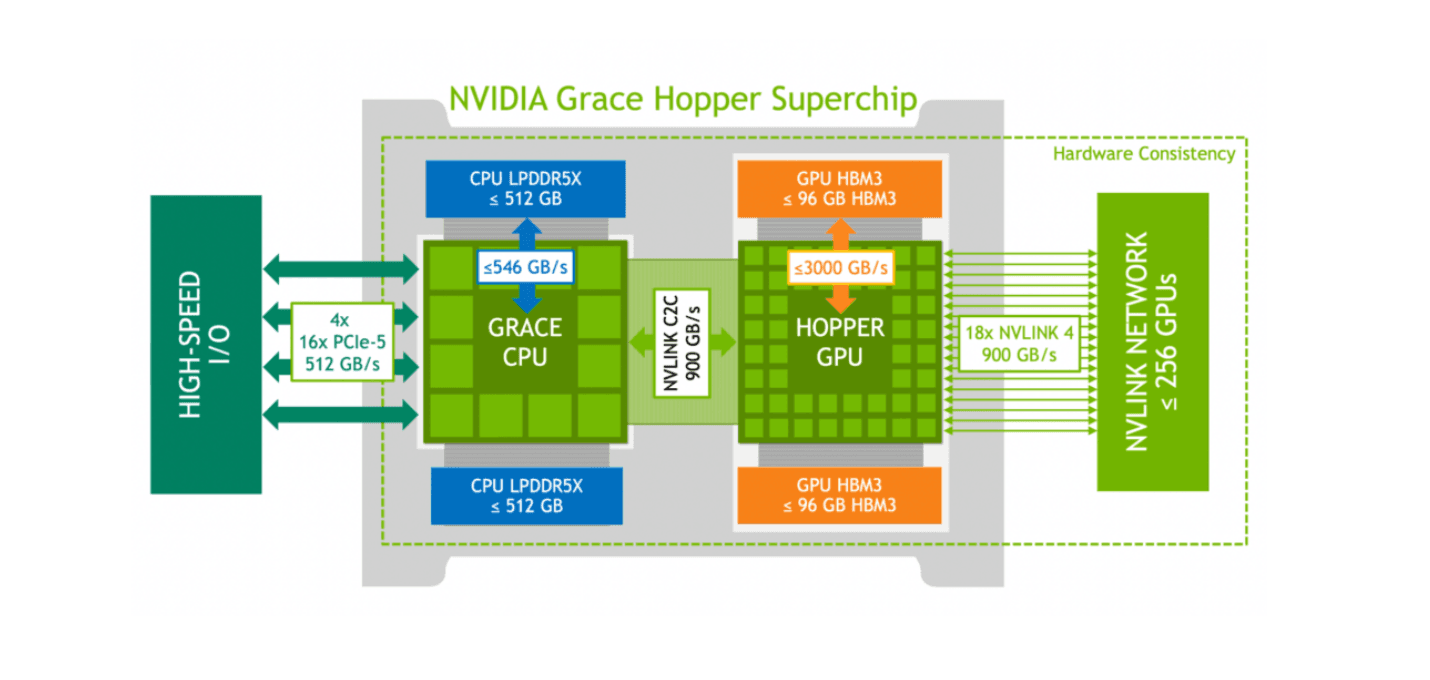\n\nScaleway will launch this year the [NVIDIA GH200 Grace Hopper Superchip](https://resources.nvidia.com/en-us-grace-cpu/nvidia-grace-hopper-2), which combines Grace ARM CPUs with Hopper GPUs in the same device, which are linked at 900 GB/s. You can connect 256 of these devices together, which is much larger than what you can connect in the DGX configuration described above (the 8 GPUs connected at 900 GB/s with NVlink in a single DGX H100 server node). And if you need more you can even connect several mesh of 256 GH200 via Infiniband at 400Gb/s. So it’s really for use cases where the memory is the bottleneck, so it’s really for HPC, and for inference of LLMs. When they’re all put together, it’s like a giant GPU, designed for the most demanding use cases, like healthcare and life sciences, for example. \n","createdAt":"2024-02-21T14:45:08.671Z","updatedAt":"2024-02-22T13:48:55.171Z","publishedAt":"2024-02-22T13:48:55.160Z","locale":"en","tags":"AI","popular":false,"articleOfTheMonth":false,"category":"Build","timeToRead":6,"excerpt":"What do you need to know before getting started with state-of-the-art AI hardware like NVIDIA's H100 PCIe 5, or even Scaleway's Jeroboam or Nabuchodonosor supercomputers? Look no further...","author":"Fabien da Silva","h1":"Infrastructures for LLMs in the cloud","createdOn":"2024-02-21","image":{"data":{"id":2960,"attributes":{"name":"Nabu-SuperPod-Card.webp","alternativeText":null,"caption":null,"width":1216,"height":752,"formats":{"large":{"ext":".webp","url":"https://www-uploads.scaleway.com/large_Nabu_Card_827fe79a9e.webp","hash":"large_Nabu_Card_827fe79a9e","mime":"image/webp","name":"large_Nabu-Card.png","path":null,"size":1061.39,"width":1000,"height":618},"small":{"ext":".webp","url":"https://www-uploads.scaleway.com/small_Nabu_Card_827fe79a9e.webp","hash":"small_Nabu_Card_827fe79a9e","mime":"image/webp","name":"small_Nabu-Card.png","path":null,"size":267.23,"width":500,"height":309},"medium":{"ext":".webp","url":"https://www-uploads.scaleway.com/medium_Nabu_Card_827fe79a9e.webp","hash":"medium_Nabu_Card_827fe79a9e","mime":"image/webp","name":"medium_Nabu-Card.png","path":null,"size":603.54,"width":750,"height":464},"thumbnail":{"ext":".webp","url":"https://www-uploads.scaleway.com/thumbnail_Nabu_Card_827fe79a9e.webp","hash":"thumbnail_Nabu_Card_827fe79a9e","mime":"image/webp","name":"thumbnail_Nabu-Card.webp","path":null,"size":"41.24","width":245,"height":152}},"hash":"Nabu_Card_827fe79a9e","ext":".webp","mime":"image/webp","size":287.74,"url":"https://www-uploads.scaleway.com/Nabu_Card_827fe79a9e.webp","previewUrl":null,"provider":"@website/strapi-provider-upload-scaleway-bucket","provider_metadata":null,"createdAt":"2023-11-15T06:04:36.097Z","updatedAt":"2023-11-15T10:18:01.608Z"}}},"recommendedArticles":{"data":[{"id":403,"attributes":{"title":"ai-in-practice-generating-video-subtitles","path":"ai-in-practice-generating-video-subtitles/","description":"Scaleway is a French company with an international vision, so it is imperative that we provide information to our 550+ employees in both English and French, to ensure clear understanding and information flow. We create a diverse set of training videos for internal usage, with some being originally voiced in English, and others in French. In all cases they should include subtitles for both languages.\n\nCreating subtitles is a time-consuming process that we quickly realized would not scale. Fortunately, we were able to harness the power of AI for this exact task. With the help of [OpenAI’s Whisper](https://github.com/openai/whisper), the University of [Helsinki’s Opus-MT](https://github.com/Helsinki-NLP/Opus-MT) and a bit of code, we were able to not only transcribe, and when required, translate our internal videos; but we could also generate subtitles in [the srt format](https://en.wikipedia.org/wiki/SubRip#:~:text=by%20that%20program.-,SubRip%20file%20format,-%5Bedit%5D), that we can simply import into a video editing software or feed to a video player.\n\n\n## OpenAI’s Whisper\n\nWhisper is an Open Source model created by OpenAI. It is a general-purpose speech recognition model that is able to identify and transcribe a wide variety of spoken languages. It is one of the most popular models around today and is released under MIT license.\n\nOpenAI provides a Python SDK that will interact with the model, which has a wide variety of “flavors” based on the accuracy of their results: tiny, base, small, medium, and large. Larger models have been trained with a greater amount of parameters or examples, which makes them larger in size, and more resource-hungry — the _tiny_ version of the model requires 1GB of VRAM (Video RAM) and the _large_ version requires around 10GB.\n\n\n## Helsinki-NLP’s Opus-MT\n\nThe University of Helsinki made its own Open Source text translation models available based on the Marian-MT framework used by Microsoft Translator. Opus-MT models are provided as language pairs: translation source, and translation target, meaning that the model Helsinki-NLP/opus-mt-fr-en will translate text in French (fr) to English (en), and the other way around with Helsinki-NLP/opus-mt-en-fr.\n\nOpus-MT can be used via the [Transformers Python library](https://huggingface.co/docs/transformers/index) from Hugging Face or using Docker. It is an Open Source project released under the MIT License and requires you to cite the OPUS-MT paper on your implementations:\n\n```\n@InProceedings{TiedemannThottingal:EAMT2020,\n author = {J{\\\"o}rg Tiedemann and Santhosh Thottingal},\n title = {{OPUS-MT} — {B}uilding open translation services for the {W}orld},\n booktitle = {Proceedings of the 22nd Annual Conferenec of the European Association for Machine Translation (EAMT)},\n year = {2020},\n address = {Lisbon, Portugal}\n }\n```\n\n## Generating subtitles\n\nCombining these two models into a subtitle-generating service is only a matter of adding some code to “glue” them together. But before diving into the code, let’s review our requirements:\n\nFirst, we need to create a Virtual Machine capable of running AI models without a hitch, and the [NVIDIA H100-1-80G GPU instance](https://www.scaleway.com/en/h100-pcie-try-it-now/) is a great choice.\n\nWith the type of instance clear, we can now focus on the functional requirements. We want to pass in a video file as input to Whisper to get a transcript. The second step will be to translate that transcript using OPUS-MT from a specific source language to a target language. Finally, we want to create a subtitle file in the target language that is in sync with the audio.\n\n\n### Setting up Whisper\n\nYou will find the latest information about setting it up on [their GitHub repository](https://github.com/openai/whisper), but in general, you can install the Python library using pip:\n\n```\npip install -U openai-whisper\n```\n\nWhisper relies heavily on the FFmpeg project for manipulating multimedia files. FFmpeg can be installed via APT:\n\n ```\n sudo apt install ffmpeg -y\n ```\n\n### The code\n\n\n#### 1. A simple text transcription\n\nThis basic example is the most straightforward way to transcribe audio into text. After importing the Whisper library, you load a _flavor_ of the model by passing [a string with its name](https://github.com/openai/whisper/#available-models-and-languages) to the load_model method. In this case, the _base_ model is accurate enough, but some use cases may require larger or smaller model flavors.\n\nAfter loading the model, you load the audio source by passing the file path. Notice that you can use both audio and video files, and in general, any file type with audio that is [supported by ](https://ffmpeg.org/ffmpeg-formats.html)FFmpeg.\n\nFinally, you make use of the transcribe method of the model by passing it the loaded audio. As a result, you get a dictionary that amongst other items, contains the whole transcription text.\n\n```python\n#main.py\n\nimport whisper\n\nmodel = whisper.load_model(\"base\")\naudio = whisper.load_audio(\"input_file.mp4\")\nresult = model.transcribe(audio)\n\nprint(result[\"text\"])\n```\n\nThis basic example gives you the main tools needed for the rest of the project: loading a model, loading an input audio file, and transcribing the audio using the model. This is already a big step forward and puts us closer to our goal of generating a subtitle file, however, you may have noticed that the resulting text doesn’t include any time references, it’s only text. Syncing this transcribed text with the audio would be a task that would require large amounts of manual work, but fortunately, Whisper’s transcription process also outputs _segments_ that are time-coded.\n\n\n#### 2. Segments\n\nHaving time-coded segments means you can pinpoint them to their specific start and end times during the clip. For instance, if the first speech segment in the clip is “We're no strangers” and it starts at 00:17:50 and ends at 00:18:30, you will get that information in the segment dictionary, giving you all you need to create an srt subtitle file, now all you have to do is to properly format it to conform with the appropriate syntax.\n\n```python\n#Getting the transcription segments\nfrom datetime import timedelta #For when getting the segment time\nimport os #For creating the srt file in the filesystem\nimport whisper\n\nmodel = whisper.load_model(\"base\")\naudio = whisper.load_audio(\"input_file.mp4\")\nresult = model.transcribe(audio)\n\nsegments = result[\"segments\"] #A list of segments\n\nfor segment in segments:\n\t#...\n```\n\n#### 3. An srt subtile file\n\nSubtitle files in the srt format are divided into sequences that include the start and end timecodes — separated by the “ --\u003e \" string — followed by the caption text ending in a line break. Here’s an example:\n\n```\n1\n00:01:26,612 --\u003e 00:01:29,376\nTook you long enough!\nDid you find it? where is it?.\n\n2\n00:01:39,101 --\u003e 00:01:42,609\nI did. But I wish I didn't.\n\n3\n00:02:16,339 --\u003e 00:02:18,169\nWhat are you talking about?\n```\n\nEach segment contains an ID field that can be used as the sequence number. The start and end times — the moments during which the subtitle is supposed to be on screen — can be obtained by padding the `timedelta` of each of the corresponding fields with zeroes (we’re keeping things simple here, but note that a more accurate subtitle syncing result have been achieved by projects such as [stable-ts](https://github.com/jianfch/stable-ts)). And the caption is the segment’s text. Here is the code that will generate each formatted subtitle sequence: \n\n```python\n#Getting segments transcription and formatting it as an srt subtitle\n\n#...\n\nfor segment in segments:\n\tstartTime = str(0)+str(timedelta(seconds=int(segment['start'])))+',000'\n\tendTime = str(0)+str(timedelta(seconds=int(segment['end'])))+',000'\n\ttext = segment['text']\n\n\tsubtitle_segment = f\"{segment['id'] + 1}\\n{startTime} --\u003e {endTime}\\n{ text }\\n\\n\"\n```\n\nAll that is left is to write each `subtitle_segment` to a new file:\n\n```python\n#Writting to the output subtitle file\n\twith open(\"subtitle.srt\", 'a', encoding='utf-8') as srtFile:\n \tsrtFile.write(subtitle_segment)\n```\n\nThe complete example code should look like this:\n\n```python\n#main.py\n\nfrom datetime import timedelta\nimport os\nimport whisper\n\nmodel = whisper.load_model(\"base\")\naudio = whisper.load_audio(\"input_file.mp4\")\nresult = model.transcribe(audio)\n\nsegments = result[\"segments\"]\n\nfor segment in segments:\n startTime = str(0)+str(timedelta(seconds=int(segment['start'])))+',000'\n endTime = str(0)+str(timedelta(seconds=int(segment['end'])))+',000'\n text = segment['text']\n\n subtitle_segment = f\"{segment['id'] + 1}\\n{startTime} --\u003e {endTime}\\n{ text }\\n\\n\"\n #Writting to the output subtitle file\n with open(\"subtitle.srt\", 'a', encoding='utf-8') as srtFile:\n \t srtFile.write(subtitle_segment)\n```\n\nNow to try it out you can download _this example file _— Or bring your own! —_ _with wget for instance:\n\n```sh\nwget https://scaleway.com/ai-book/examples/1/example.mp4 -O input_file.mp4\n```\n\nAnd then simply run the script:\n\n```sh\npython3 main.py\n```\n\nAfter only a few seconds — because you’re using [one of the fastest GPU instances on the planet](https://www.scaleway.com/en/h100-pcie-try-it-now/) —, the script will complete running and you will have a new `subtitle.srt` file that you can use during your video editing process or to load while playing the video file, great! But… the subtitle file is in the same language as the video. It is indeed useful as it is, but you probably want to reach a wider audience by translating it into different languages. We’ll explore that next.\n\n\n#### 4. Translating a segment’s text\n\nTranslating each segment’s text comes down to importing `MarianMTModel` and` MarianTokenizer` from Hugging Face’s Transformers library, passing the desired model name, and generating the translation. Install the dependencies by running the following command:\n\n```sh\npip install transformers SentencePiece\n```\n\nIn this example \"Helsinki-NLP/opus-mt-fr-en\" is used to translate from French to English. The `translate` abstracts the translation process by requiring a source string and returning a translated version of it.\n\n```python\nfrom transformers import MarianMTModel, MarianTokenizer\n# ...\n\nopus_mt_model_name = \"Helsinki-NLP/opus-mt-fr-en\"\ntokenizer = MarianTokenizer.from_pretrained(opus_mt_model_name)\nopus_mt_model = MarianMTModel.from_pretrained(opus_mt_model_name)\n\ndef translate(str):\n\ttranslated = opus_mt_model.generate(**tokenizer(str, return_tensors=\"pt\", padding=True))\n\tres = [tokenizer.decode(t, skip_special_tokens=True) for t in translated]\n\treturn res[0]\n```\n\nThere’s no need to worry about the `**tokenizer` function for now, just know that it receives the source string and some additional parameters that we can leave untouched.\n\nThe complete code example looks like this:\n\n```python\nfrom datetime import timedelta\nimport os\nimport whisper\nfrom transformers import MarianMTModel, MarianTokenizer\n\nmodel = whisper.load_model(\"base\")\naudio = whisper.load_audio(\"input_file.mp4\")\nresult = model.transcribe(audio)\n\nsegments = result[\"segments\"]\n\nopus_mt_model_name = \"Helsinki-NLP/opus-mt-fr-en\"\ntokenizer = MarianTokenizer.from_pretrained(opus_mt_model_name)\nopus_mt_model = MarianMTModel.from_pretrained(opus_mt_model_name)\n\ndef translate(str):\n\ttranslated = opus_mt_model.generate(**tokenizer(str, return_tensors=\"pt\", padding=True))\n\tres = [tokenizer.decode(t, skip_special_tokens=True) for t in translated]\n\treturn res[0]\n\nfor segment in segments:\n startTime = str(0)+str(timedelta(seconds=int(segment['start'])))+',000'\n endTime = str(0)+str(timedelta(seconds=int(segment['end'])))+',000'\n text = translate(segment['text'])\n\n\n subtitle_segment = f\"{segment['id'] + 1}\\n{startTime} --\u003e {endTime}\\n{ text }\\n\\n\"\n #Writting to the output subtitle file\n with open(\"subtitle.srt\", 'a', encoding='utf-8') as srtFile:\n \t srtFile.write(subtitle_segment)\n```\n\nThat’s it! Even though the results are not perfect, and you may need to make a few manual adjustments here and there, considering the rate at which AI is advancing, things can only get better in the time to come.\n\nYou can now extend and adapt this code to your own needs, how about making it dynamically accept a file path as an input parameter? Or what if you made it into a web service others can easily take advantage of? The choice is yours! just don’t forget to cite the OPUS-MT paper on your implementations if you’re using the translation feature.","createdAt":"2023-11-28T18:00:37.698Z","updatedAt":"2024-02-07T15:14:18.482Z","publishedAt":"2023-11-30T08:26:58.333Z","locale":"en","tags":"ai\nH100","popular":false,"articleOfTheMonth":false,"category":"Build","timeToRead":5,"excerpt":"In this practical example, we roll up our sleeves and put Scaleway's H100 Instances to use by leveraging a couple of open source ML models to optimize our internal communication workflows.","author":"Diego Coy","h1":"AI in practice: Generating video subtitles","createdOn":"2023-12-01"}},{"id":407,"attributes":{"title":"ai-and-quality-giskard-combessie","path":"ai-and-quality-giskard-combessie/","description":"In a world where AI has become more and more a common presence in our lives, the quest for quality AI solutions has taken center stage. We sat down with Alexandre Combessie, co-founder and CEO of [Giskard.AI](https://www.giskard.ai/) - which notably exhibited at ai-PULSE last month - to delve into the challenges around ethics and quality faced by AI solutions and their users. \n\nWith a background steeped in AI expertise, Alexandre brings a wealth of experience to the table. Before creating Giskard.AI, he spent five years at Dataiku, focusing on building models for various industries, particularly in NLP (natural language processing) and time series. His experience in crafting models for large-scale enterprises, including in healthcare and financial services, laid the foundations for Giskard.AI’s later innovative work. \n\nToday, Giskard is a French firm that specializes in AI quality, which Combessie co-founded in 2021. It ensures AI professionals maximize Machine Learning (ML) algorithm quality, minimizing errors, biases and vulnerabilities. Giskard is as such notably establishing itself as the leading software platform for aiding compliance with upcoming AI regulations and standards.\n\n\n## Quality: The multifaceted essence of AI\n\nNow that conversing with AI has become commonplace, the distinction between a run-of-the-mill AI and a quality-driven one has never been more important. Combessie emphasizes that quality in results spans multiple dimensions, with two key factors standing out:\n\n1. **Generative AI's hallucinations**\nAt the heart of generative AI lies the ability to create and construct, often leading to intriguing \"hallucinations,\" whereby the AI conjures up information that is false, leading to a range of significant issues. Such fabrications could contribute to the spread of fake news, error of diagnosis, and heighten the risk of poor human decision-making. Moreover, the possibility of errors in critical areas like medical diagnoses due to AI-generated inaccuracies is a particularly concerning aspect. Alexandre encourages us to explore hallucinations even further than has been established to date, to understand both their potential and limitations.\n\n2. **The ethical challenge**\nThe ethical dimension of AI looms large: the algorithms that fuel AI models are derived from existing datasets, potentially perpetuating biases and prejudices. The crucial question arises: could an algorithm be toxic, or offensive? This challenge of ethics and bias calls for profound scrutiny. Even before generative AI’s recent exponential growth, quality concerns were evident, spanning ethical biases in scoring algorithms, lack of transparency regarding AI models’ decisions, and performance issues in production. \n \nEthical biases in consumer applications like facial recognition have already been unearthed, and in the industrial sphere, predictive maintenance or fraud detection could prove particularly sensitive to AI’s potential mistakes. To investigate such cases, drawing on two years of dedicated quality work pre-ChatGPT, Giskard.AI was able to formulate and test diverse solutions that extend beyond chatbots to various business applications of AI, such as tabular data.\n\n\n## Stepping into a maturing market: ethics, risk, and performance\n\nA key hurdle in AI's journey toward quality and ethics is the market's maturity. Concepts like risk, ethics, and performance are relatively new to the AI world, demanding both internal team education and external regulation. The importance of evangelization is at the center of those changes, and not just within a company, but also in terms of regulatory compliance. The objective is clear: minimize errors, offense, and legal concerns for high-risk AI models, which are starting to impact all we see and read.\n\nCombessie's engineering background parallels his dedication to ensuring quality in AI. Drawing a captivating analogy to civil engineering, he emphasizes the high standards that underlie his work. He envisions building a bridge between data scientists and those who grasp the significance of AI's ethical and quality dimensions. \n\nBeyond the accuracy of a result lies the model metrics. Which metrics truly matter for a model to be seen as a great one? Combessie rejects the notion of relying on one single KPI. Such an approach may provide limited accuracy and overlook important aspects of model performance.\n\nThe concept of \"robustness metrics\" emerges as a vital topic, especially for models deployed in production environments. Combessie shares a compelling example from the real estate sector, where AI-driven decisions led to catastrophic financial losses. Zillow deployed an AI algorithm to predict the prices of the homes they would sell. After having put the model in autopilot mode, they lost over $500 million in six months. They stopped trading, and fired their entire data science team. Ensuring AI models do not lead to such disastrous outcomes is a critical aspect of maintaining robustness.\n\n\n## Shaping Ethical AI \n\nThe responsibility for ethical AI lies with the companies that develop and deploy it. If a company's AI lacks ethics or perpetuates discrimination, that company is legally accountable. In high-risk AI scenarios, failure to adhere to ethical standards could result in fines of up to 6% of a company's revenue, according to the upcoming EU AI Act. \n\nAs stated on [Giskard.AI’s blog](https://www.giskard.ai/knowledge/the-eu-ai-act-what-can-you-expect), under the AI Act (which was passed early December), generative foundation models like ChatGPT will be subject to strict controls. These include transparency, or public disclosure of which content was created by AI; declaring what copyrighted data was used in training; and barriers to stop such models generating illegal content.\n\nWith these constraints in mind, Giskard.AI empowers companies to adhere to legislation by simplifying and measuring their compliance efficiently. As such, Giskard.AI has taken the lead in advancing ethical AI. It provides the tools to assess discriminatory biases in models, particularly concerning attributes like age, gender, and ethnicity. Collaborating with organizations like AFNOR, Giskard.AI contributes to setting standards that safeguard against biases.\n\n\n## Conclusion\n\nFor Combessie and Giskard, moving forwards, the key will be finding the ideal balance between innovation and regulation. \"Having testing systems for ML models which are easy to integrate by data scientists are key to making the compliance process to the regulation as easy as possible,\" says Combessie, \"so that regulation is possible without slowing down innovation, but also respecting the rights of citizens.\"\n\nFurthermore, as Giskard does AI \"in an open-source way\", he adds, \"our methods are transparent and auditable\".\n\n\n### About Giskard\n\n_Giskard is a French software publisher specializing in Artificial Intelligence (AI) quality. Founded in 2021 by three AI experts, including two former engineers from Dataiku and a former data scientist from Thales, Giskard's mission is to help AI professionals ensure the quality of their algorithms. It assists in avoiding the risks of errors, biases, and vulnerabilities in AI algorithms._\n\n_Giskard is backed by renowned investors in the AI field, including Elaia and the CTO of Hugging Face. In August 2023, Giskard received a strategic investment from the European Commission to establish itself as the leading software platform for facilitating compliance with the European AI regulation._\n\n_Learn more: [Giskard.AI](https://www.giskard.ai/)_\n\n","createdAt":"2023-12-18T09:19:03.924Z","updatedAt":"2023-12-18T09:20:36.590Z","publishedAt":"2023-12-18T09:20:36.576Z","locale":"en","tags":"AI\nai-PULSE","popular":false,"articleOfTheMonth":false,"category":"Build","timeToRead":4,"excerpt":"How can AI remain innovative whilst complying with regulations and standards? French startup and ai-PULSE exhibitor Giskard.AI has the answer...","author":"James Martin","h1":"AI and the quality conundrum, with Giskard.AI’s Alexandre Combessie","createdOn":"2023-12-18"}}]},"meta":{"id":1351,"title":"Infrastructures for LLMs in the cloud","description":"What do you need to know before getting started with state-of-the-art AI hardware like NVIDIA's H100 PCIe 5, or even Scaleway's Jeroboam or Nabuchodonosor supercomputers? Look no further...","ogtype":null,"ogtitle":"Infrastructures for LLMs in the cloud","ogdescription":"What do you need to know before getting started with state-of-the-art AI hardware like NVIDIA's H100 PCIe 5, or even Scaleway's Jeroboam or Nabuchodonosor supercomputers? Look no further...","noindex":false},"localizations":{"data":[]}}}]},"meta":{"id":1354,"title":"How to get started in AI without excessive cost, or emissions!","description":"How can startups take their first steps with Large Language Models (LLMs)? Leveraging AI needn't cost the earth, explains MindMatch's Zofia Smoleń","ogtype":null,"ogtitle":"How to get started in AI without excessive cost, or emissions!","ogdescription":"How can startups take their first steps with Large Language Models (LLMs)? Leveraging AI needn't cost the earth, explains MindMatch's Zofia Smoleń","noindex":false,"ogimage":{"data":[{"id":3240,"attributes":{"name":"Automatic-Speech-Recognition-AI-Illustration-Blog.webp","alternativeText":null,"caption":null,"width":1216,"height":752,"formats":{"large":{"ext":".webp","url":"https://www-uploads.scaleway.com/large_Automatic_Speech_Recognition_AI_Illustration_Blog_e8870a4451.webp","hash":"large_Automatic_Speech_Recognition_AI_Illustration_Blog_e8870a4451","mime":"image/webp","name":"large_Automatic-Speech-Recognition-AI-Illustration-Blog.webp","path":null,"size":75.83,"width":1000,"height":618},"small":{"ext":".webp","url":"https://www-uploads.scaleway.com/small_Automatic_Speech_Recognition_AI_Illustration_Blog_e8870a4451.webp","hash":"small_Automatic_Speech_Recognition_AI_Illustration_Blog_e8870a4451","mime":"image/webp","name":"small_Automatic-Speech-Recognition-AI-Illustration-Blog.webp","path":null,"size":28.21,"width":500,"height":309},"medium":{"ext":".webp","url":"https://www-uploads.scaleway.com/medium_Automatic_Speech_Recognition_AI_Illustration_Blog_e8870a4451.webp","hash":"medium_Automatic_Speech_Recognition_AI_Illustration_Blog_e8870a4451","mime":"image/webp","name":"medium_Automatic-Speech-Recognition-AI-Illustration-Blog.webp","path":null,"size":51,"width":750,"height":464},"thumbnail":{"ext":".webp","url":"https://www-uploads.scaleway.com/thumbnail_Automatic_Speech_Recognition_AI_Illustration_Blog_e8870a4451.webp","hash":"thumbnail_Automatic_Speech_Recognition_AI_Illustration_Blog_e8870a4451","mime":"image/webp","name":"thumbnail_Automatic-Speech-Recognition-AI-Illustration-Blog.jpg","path":null,"size":8.66,"width":245,"height":152}},"hash":"Automatic_Speech_Recognition_AI_Illustration_Blog_e8870a4451","ext":".webp","mime":"image/webp","size":528.03,"url":"https://www-uploads.scaleway.com/Automatic_Speech_Recognition_AI_Illustration_Blog_e8870a4451.webp","previewUrl":null,"provider":"@website/strapi-provider-upload-scaleway-bucket","provider_metadata":null,"createdAt":"2024-02-15T13:43:21.303Z","updatedAt":"2024-02-26T14:23:17.313Z"}}]}},"localizations":{"data":[]}}},"_nextI18Next":{"initialI18nStore":{"en":{"common":{"open":"Open","close":"Close","backTo":"Back to {{page}}","seeMore":"See more","skip":"Skip {{to}}","toLogin":"to login","toMain":"to main content","toFooter":"to footer section","results":"Number of results: {{resultsLength}}","yourEmail":"Your Email","submit":"Submit","header":{"motdTitle":"Top highlight! "},"footer":{"followUs":"Follow us","subLinks":{"contracts":{"href":"/en/contracts/","title":"Contracts"},"legalNotice":{"href":"/en/legal-notice/","title":"Legal Notice"},"privacyPolicy":{"href":"/en/privacy-policy/","title":"Privacy Policy"},"cookie":{"href":"/en/cookie/","title":"Cookie"},"securityMeasures":{"href":"https://security.scaleway.com","title":"Security Measures"},"accessibility":{"href":"/fr/accessibility/","title":"Accessibility statement","hrefLang":"fr"}}},"breadcrumb":{"homepageLink":{"home":{"href":"/","title":"Home"}}},"cookies":{"acceptAll":"Accept all","rejectAll":"Reject all","save":"Save settings","panelManagementTitle":"Manage cookies settings","panelConsent":{"title":"Cookie time!","description":"We use cookies in order to improve our website and to offer you a better experience. You can also consult our ","linkLabel":"Cookie policy","link":"/en/privacy-policy/","settings":"Manage your preferences"},"categories":{"functional":{"title":"Functional","subtitle":"Always active","description":"These cookies are required for the website to function properly and to allow you to use its services and features. Without these cookies, we would be unable to provide certain requested services or features."},"analytics":{"title":"Analytics","description":"These cookies are used to monitor the performance of our site and to enhance your browsing experience."},"marketing":{"title":"Marketing","description":"These cookies are used to understand user behavior in order to provide you with a more relevant browsing experience or personalize the content on our site."}}}},"blog":{"tagsAriaLabel":"Tags list. Click to choose as filter.","timeToRead":"{{min}} min read","recommendedArticles":"Recommended articles","pagination":{"next":"Forward to Next","previous":"Back to Previous","goToPage":"Go to page ","currentPage":"Current page: "},"copyButton":{"copied":"Copied!","defaultValue":"Copy","code":"Copy code"},"home":{"title":"Scaleway Blog - All posts","description":"Scaleway’s blog helps developers and startups to build, deploy and scale applications.","heading":"Scaleway Blog","articleOfMonth":"Must read","latestArticles":"Latest articles","popularArticles":"Most popular articles"},"categoryPage":{"build":{"title":"Build Projects with Scaleway","description":"Learn how to easily build and develop projects using Scaleway products."},"deploy":{"title":"Deploy Applications with Scaleway","description":"Discover how to deploy your applications smoothly with Scaleway."},"scale":{"title":"Scale Your Applications with Scaleway","description":"Find out how to efficiently scale your applications on Scaleway."},"incidents":{"title":"Incident Reports","description":"All the latest updates on Scaleway Cloud ecosystem incidents, and how they were resolved."}},"authorPage":{"title_one":"A {{author}}'s post","title_other":"All {{author}}'s posts","description_one":"Discover a blog post written by {{author}}.","description_other":"Discover all the blog posts written by {{author}}."}},"pages":{"available_zones":"Available zones:","city":"{{code}}:","AMS":"Amsterdam","PAR":"Paris","WAW":"Warsaw","yes":"Yes","no":"No","daily":"Daily","weekly":"Weekly","monthly":"Monthly","yearly":"Yearly","published":"Published on","seeMore":"See more","blocks":{"calculator":{"choose":"Choose your plan","availabilityZone":"Availability Zone","instanceType":"Instance Type","quantity":"Quantity","selectPlaceholder":"Select...","volumeSize":"Volume Size","volumeSizeHelper":"Min. 10 GB","volumeType":"Volume Type","sizeUnit":"GB","flexibleIp":"Flexible IPv4","ipHelper":"You need a Flexible IP if you want to get an Instance with a public IPv4.\n Uncheck this box if you already have one available on your account, or if you don’t need an IPv4.","noOtherType":"No other type available with this Instance"},"pricingDedibox":{"segmentedControlLabel":"Benefit from our commitment plans :"},"productFaq":{"title":"Frequently asked questions"},"productTutorials":{"title":"Get started with tutorials"},"customerStories":{"defaultTitle":"Customer success stories"}},"templates":{"beta":{"discovery":{"title":"Discovery","description":"Discovery products are prototypical versions of a product. This phase aims to validate an idea and to prove there is interest in the product. During the Discovery phase, customers can be contacted by the Product team to ask them to share their thoughts on the product and to help with the development of the new solution.\nProducts in the Discovery phase are not guaranteed to be released. The duration of the Discovery phase may vary depending on the product."},"early-access":{"title":"Early Access","description":""},"private":{"title":"Private Beta","description":"Private Beta products are early versions of future products or features. This phase allows users to test, validate a product in development, and give feedback to the Product team.\nPrivate Beta is limited to users selected by the Product Development team. Users can request access to the product via a form and the development team will grant access rights. The Private Beta stage usually lasts three to six months."},"public":{"title":"Public Beta","description":"Public Beta products are ready to be tested by all customers. Public Beta products may not include all of the final product’s features.\nPublic Beta is the last stage of testing before a product is released in General Availability.\nThese products are intended to be used in test environments unless specified otherwise. The Public Beta phase lasts six months on average."}},"domains":{"register":"Register","registerInfo":"Price before tax\nFirst year registration's price.","transfer":"Transfer","transferInfo":"Price before tax\nTransfer price for domain during first year.","renewing":"Renewing","renewingInfo":"Price before tax\nSecond year registration's price.","restoration":"Restoration","restorationInfo":"Price before tax\nPrice for renewing after expiration and/or redemption period."},"testimonials":{"title":"Customer Success Story","readMore":"Read more"},"pricingPage":{"backButton":"Back to pricing page","title":"All Range","legalLabel":"Legal notice","legalDescription":"An installation fee equivalent to one month of server rental is charged for each Dedibox server order.\n\nPrices before tax","openAll":"Open all","closeAll":"Close all","close":"Close","open":"Open","regions":"Regions","subtableToggleButtons":"Subtable open buttons","viewPricing":"View pricing","order":"Order","subtableOrderButton":"Subtable order button"},"partnerProgram":{"showingPartners_one":"Showing {{count}} partner","showingPartners_other":"Showing {{count}} partners","type_one":"Partner type","type_other":"Partner types","expertise_one":"Expertise","expertise_other":"Expertises","industry_one":"Industry","industry_other":"Industries","location_one":"Location","location_other":"Locations","filtersTitle":"Filters","noResults":{"info":"No results found for your search criteria.","tip":"Try removing your filters to see more partners!","label":"Clear filters"}},"partnerPage":{"partners":"Partners","details":"Details","activity":"Activity","contact":"Contact","viewWebsite":"View Website","contactEmail":"Contact email"},"eventPage":{"nextEvents":"Next Events","pastEvents":"Past Events","noResults":{"info":"No upcoming events.","tip":"We’ll be back soon! Stay tuned and subscribe to our newsletter at the bottom of the page."},"noFilteredResults":{"info":"No events to show","tip":"Try removing your filters!","label":"Clear filters"},"countdown":{"label":"The event starts in","labelA11y":"Event time :","days":"Days","hours":"Hours","minutes":"Minutes","seconds":"Seconds"},"panelButton":"Filtres","closeButton":"Close filters","searchForEvents":"Search for Events","filters":"Filters"}},"notFound":{"title":"Page not found","text":"It seems that the page you want to access does not exist. Please check your URL or renew your request later.","link":"Return to homepage"}}}},"initialLocale":"en","ns":["common","blog","pages"],"userConfig":{"i18n":{"locales":["default","en","fr"],"defaultLocale":"default","localeDetection":false},"default":{"i18n":{"locales":["default","en","fr"],"defaultLocale":"default","localeDetection":false}}}},"header":{"mainNavigationItems":[{"id":542,"title":"Dedibox and Bare Metal","menuAttached":false,"order":1,"path":"/DediboxBareMetal","type":"WRAPPER","uiRouterKey":"dedibox-and-bare-metal-1","slug":"dedibox-bare-metal","external":false,"items":[{"id":543,"title":"Dedibox - dedicated servers","menuAttached":false,"order":1,"path":"/DediboxBareMetal/Dedibox","type":"INTERNAL","uiRouterKey":"dedibox-dedicated-servers","slug":"dedibox-bare-metal-dedibox","external":false,"related":{"id":29,"title":"Dedibox","path":"/dedibox/","scheduledAt":null,"createdAt":"2022-04-19T15:29:02.488Z","updatedAt":"2024-12-02T21:42:14.962Z","publishedAt":"2022-04-28T17:05:07.122Z","locale":"en","__contentType":"api::page.page","navigationItemId":543,"__templateName":"Generic"},"items":[{"id":544,"title":"Start","menuAttached":false,"order":1,"path":"/DediboxBareMetal/Dedibox/Start","type":"INTERNAL","uiRouterKey":"start-2","slug":"dedibox-bare-metal-dedibox-start","external":false,"related":{"id":53,"title":"Start","path":"/dedibox/start/","scheduledAt":null,"createdAt":"2022-04-21T16:44:17.577Z","updatedAt":"2024-12-02T21:47:26.792Z","publishedAt":"2022-04-28T17:12:40.426Z","locale":"en","__contentType":"api::page.page","navigationItemId":544,"__templateName":"Generic"},"items":[],"description":"Affordable servers with the best price-performance ratio on the market"},{"id":545,"title":"Pro","menuAttached":false,"order":2,"path":"/DediboxBareMetal/Dedibox/Pro","type":"INTERNAL","uiRouterKey":"pro-4","slug":"dedibox-bare-metal-dedibox-pro","external":false,"related":{"id":9,"title":"Pro","path":"/dedibox/pro/","scheduledAt":null,"createdAt":"2022-04-07T13:51:48.537Z","updatedAt":"2025-02-20T10:47:35.455Z","publishedAt":"2022-04-28T17:04:00.983Z","locale":"en","__contentType":"api::page.page","navigationItemId":545,"__templateName":"Generic"},"items":[],"description":"Perfect balance of processing power, memory and storage"},{"id":546,"title":"Core","menuAttached":false,"order":3,"path":"/DediboxBareMetal/Dedibox/Core","type":"INTERNAL","uiRouterKey":"core-1","slug":"dedibox-bare-metal-dedibox-core","external":false,"related":{"id":14,"title":"Core","path":"/dedibox/core/","scheduledAt":null,"createdAt":"2022-04-11T09:05:58.588Z","updatedAt":"2025-02-06T16:24:41.969Z","publishedAt":"2022-04-28T17:04:22.560Z","locale":"en","__contentType":"api::page.page","navigationItemId":546,"__templateName":"Generic"},"items":[],"description":"The high performance backbone of your mission-critical infrastructure"},{"id":547,"title":"Store","menuAttached":false,"order":4,"path":"/DediboxBareMetal/Dedibox/Store","type":"INTERNAL","uiRouterKey":"store-2","slug":"dedibox-bare-metal-dedibox-store","external":false,"related":{"id":5,"title":"Store","path":"/dedibox/store/","scheduledAt":null,"createdAt":"2022-04-01T15:14:47.812Z","updatedAt":"2025-02-10T17:06:12.772Z","publishedAt":"2022-04-28T17:03:51.376Z","locale":"en","__contentType":"api::page.page","navigationItemId":547,"__templateName":"Generic"},"items":[],"description":"For mission-critical data, fast storage, backup and streaming"},{"id":832,"title":"GPU","menuAttached":false,"order":5,"path":"/DediboxBareMetal/Dedibox/GPU_ddx","type":"INTERNAL","uiRouterKey":"gpu-9","slug":"dedibox-bare-metal-dedibox-gpu-ddx","external":false,"related":{"id":1454,"title":"GPU","path":"/dedibox/gpu/","scheduledAt":null,"createdAt":"2024-10-31T10:01:24.876Z","updatedAt":"2025-02-06T16:21:10.102Z","publishedAt":"2024-11-07T07:38:37.573Z","locale":"en","__contentType":"api::page.page","navigationItemId":832,"__templateName":"Generic"},"items":[],"description":"Dedicated GPU power with reliable performance and stability"},{"id":548,"title":"Dedirack","menuAttached":false,"order":6,"path":"/DediboxBareMetal/Dedibox/Dedirack","type":"INTERNAL","uiRouterKey":"dedirack-1","slug":"dedibox-bare-metal-dedibox-dedirack","external":false,"related":{"id":155,"title":"Dedirack","path":"/dedibox/dedirack/","scheduledAt":null,"createdAt":"2022-05-02T10:08:21.002Z","updatedAt":"2024-12-02T21:42:15.571Z","publishedAt":"2022-05-02T10:46:06.212Z","locale":"en","__contentType":"api::page.page","navigationItemId":548,"__templateName":"Generic"},"items":[],"description":"Host your Hardware in our secured French datacenters"},{"id":742,"title":"Dedibox VPS","menuAttached":false,"order":7,"path":"/DediboxBareMetal/Dedibox/VPS","type":"INTERNAL","uiRouterKey":"dedibox-vps","slug":"dedibox-bare-metal-dedibox-vps","external":false,"related":{"id":1234,"title":"Dedibox VPS","path":"/dedibox-vps/","scheduledAt":null,"createdAt":"2024-05-08T16:42:21.258Z","updatedAt":"2024-12-02T22:03:11.926Z","publishedAt":"2024-05-14T16:28:25.184Z","locale":"en","__contentType":"api::page.page","navigationItemId":742,"__templateName":"Generic"},"items":[],"description":"60 locations worldwide, starting at €4,99/month"}],"description":""},{"id":553,"title":"Elastic Metal - bare metal cloud","menuAttached":false,"order":2,"path":"/DediboxBareMetal/elasticmetal","type":"INTERNAL","uiRouterKey":"elastic-metal-bare-metal-cloud-1","slug":"dedibox-bare-metal-elasticmetal","external":false,"related":{"id":87,"title":"Elastic Metal","path":"/elastic-metal/","scheduledAt":null,"createdAt":"2022-04-28T12:45:28.696Z","updatedAt":"2025-01-24T13:35:03.496Z","publishedAt":"2022-04-28T13:22:46.501Z","locale":"en","__contentType":"api::page.page","navigationItemId":553,"__templateName":"Generic"},"items":[{"id":554,"title":"Aluminium","menuAttached":false,"order":1,"path":"/DediboxBareMetal/elasticmetal/Aluminium","type":"INTERNAL","uiRouterKey":"aluminium-1","slug":"dedibox-bare-metal-elasticmetal-aluminium","external":false,"related":{"id":8,"title":"Aluminium","path":"/elastic-metal/aluminium/","scheduledAt":null,"createdAt":"2022-04-06T13:13:04.829Z","updatedAt":"2025-02-14T15:26:58.704Z","publishedAt":"2022-04-28T17:04:04.448Z","locale":"en","__contentType":"api::page.page","navigationItemId":554,"__templateName":"Generic"},"items":[],"description":"Fully dedicated bare metal servers with native cloud integration, at the best price"},{"id":557,"title":"Beryllium","menuAttached":false,"order":2,"path":"/DediboxBareMetal/elasticmetal/Beryllium","type":"INTERNAL","uiRouterKey":"beryllium-1","slug":"dedibox-bare-metal-elasticmetal-beryllium","external":false,"related":{"id":15,"title":"Beryllium","path":"/elastic-metal/beryllium/","scheduledAt":null,"createdAt":"2022-04-11T10:57:25.297Z","updatedAt":"2025-02-14T15:23:03.346Z","publishedAt":"2022-04-28T17:13:35.576Z","locale":"en","__contentType":"api::page.page","navigationItemId":557,"__templateName":"Generic"},"items":[],"description":"Powerful, balanced and reliable servers for production-grade applications"},{"id":556,"title":"Iridium","menuAttached":false,"order":3,"path":"/DediboxBareMetal/elasticmetal/Iridium","type":"INTERNAL","uiRouterKey":"iridium-1","slug":"dedibox-bare-metal-elasticmetal-iridium","external":false,"related":{"id":810,"title":"Iridium","path":"/elastic-metal/iridium/","scheduledAt":null,"createdAt":"2023-04-27T13:53:48.244Z","updatedAt":"2025-02-14T15:28:12.476Z","publishedAt":"2023-05-29T08:52:19.666Z","locale":"en","__contentType":"api::page.page","navigationItemId":556,"__templateName":"Generic"},"items":[],"description":"Powerful dedicated server designed to handle high-workload applications"},{"id":555,"title":"Lithium","menuAttached":false,"order":4,"path":"/DediboxBareMetal/elasticmetal/Lithium","type":"INTERNAL","uiRouterKey":"lithium-1","slug":"dedibox-bare-metal-elasticmetal-lithium","external":false,"related":{"id":16,"title":"Lithium","path":"/elastic-metal/lithium/","scheduledAt":null,"createdAt":"2022-04-11T11:15:36.538Z","updatedAt":"2025-02-20T10:52:48.984Z","publishedAt":"2022-04-28T17:13:30.074Z","locale":"en","__contentType":"api::page.page","navigationItemId":555,"__templateName":"Generic"},"items":[],"description":"Designed with huge local storage to keep, back up, and protect your data"},{"id":833,"title":"Titanium","menuAttached":false,"order":5,"path":"/DediboxBareMetal/elasticmetal/Titanium","type":"INTERNAL","uiRouterKey":"titanium","slug":"dedibox-bare-metal-elasticmetal-titanium","external":false,"related":{"id":1457,"title":"Titanium","path":"/elastic-metal/titanium/","scheduledAt":null,"createdAt":"2024-10-31T15:08:59.416Z","updatedAt":"2025-02-14T15:56:07.147Z","publishedAt":"2024-11-07T06:52:37.648Z","locale":"en","__contentType":"api::page.page","navigationItemId":833,"__templateName":"Generic"},"items":[],"description":"Power and stability of dedicated GPU hardware integrated into the Scaleway ecosystem"}],"description":""},{"id":558,"title":"Apple","menuAttached":false,"order":3,"path":"/DediboxBareMetal/Apple","type":"INTERNAL","uiRouterKey":"apple-2","slug":"dedibox-bare-metal-apple","external":false,"related":{"id":1088,"title":"Apple Mac mini","path":"/apple-mac-mini/","scheduledAt":null,"createdAt":"2024-01-31T15:28:49.276Z","updatedAt":"2025-02-07T13:57:44.141Z","publishedAt":"2024-08-02T07:56:22.454Z","locale":"en","__contentType":"api::page.page","navigationItemId":558,"__templateName":"Generic"},"items":[{"id":561,"title":"Mac mini M1","menuAttached":false,"order":1,"path":"/DediboxBareMetal/Apple/M1","type":"INTERNAL","uiRouterKey":"mac-mini-m1-1","slug":"dedibox-bare-metal-apple-m1","external":false,"related":{"id":91,"title":"Hello m1","path":"/hello-m1/","scheduledAt":null,"createdAt":"2022-04-28T15:24:50.963Z","updatedAt":"2025-02-07T14:01:43.056Z","publishedAt":"2023-10-16T14:15:59.310Z","locale":"en","__contentType":"api::page.page","navigationItemId":561,"__templateName":"Generic"},"items":[],"description":"Enjoy the Mac mini experience with great simplicity"},{"id":560,"title":"Mac mini M2","menuAttached":false,"order":2,"path":"/DediboxBareMetal/Apple/m2","type":"INTERNAL","uiRouterKey":"mac-mini-m2-2","slug":"dedibox-bare-metal-apple-m2","external":false,"related":{"id":1086,"title":"mac mini M2","path":"/mac-mini-m2/","scheduledAt":null,"createdAt":"2024-01-31T09:30:46.938Z","updatedAt":"2025-02-07T14:02:55.309Z","publishedAt":"2024-02-05T15:21:02.196Z","locale":"en","__contentType":"api::page.page","navigationItemId":560,"__templateName":"Generic"},"items":[],"description":"Perform your daily tasks with speed and efficiency"},{"id":559,"title":"Mac mini M2 Pro","menuAttached":false,"order":3,"path":"/DediboxBareMetal/Apple/M2pro","type":"INTERNAL","uiRouterKey":"mac-mini-m2-pro-1","slug":"dedibox-bare-metal-apple-m2pro","external":false,"related":{"id":991,"title":"mac mini M2 pro","path":"/mac-mini-m2-pro/","scheduledAt":null,"createdAt":"2023-10-25T08:56:21.435Z","updatedAt":"2025-02-07T14:03:47.499Z","publishedAt":"2023-11-16T12:11:33.094Z","locale":"en","__contentType":"api::page.page","navigationItemId":559,"__templateName":"Generic"},"items":[],"description":"Realize your most ambitious projects thanks to a new level of power"},{"id":886,"title":"Mac mini M4","menuAttached":false,"order":4,"path":"/DediboxBareMetal/Apple/M4","type":"INTERNAL","uiRouterKey":"mac-mini-m4","slug":"dedibox-bare-metal-apple-m4","external":false,"related":{"id":1606,"title":"Mac mini M4","path":"/mac-mini-m4/","scheduledAt":null,"createdAt":"2025-01-21T15:05:39.847Z","updatedAt":"2025-02-07T14:05:10.096Z","publishedAt":"2025-01-24T08:17:07.190Z","locale":"en","__contentType":"api::page.page","navigationItemId":886,"__templateName":"Generic"},"items":[],"description":"Latest Apple silicon chip for intensive use cases."}],"description":""}],"description":""},{"id":562,"title":"Compute","menuAttached":false,"order":2,"path":"/Compute","type":"WRAPPER","uiRouterKey":"compute-3","slug":"compute-4","external":false,"items":[{"id":563,"title":"Virtual Instances","menuAttached":false,"order":1,"path":"/Compute/VirtualInstances","type":"INTERNAL","uiRouterKey":"virtual-instances-1","slug":"compute-virtual-instances","external":false,"related":{"id":655,"title":"Virtual Instances","path":"/virtual-instances/","scheduledAt":null,"createdAt":"2023-02-20T10:48:52.279Z","updatedAt":"2025-02-11T13:16:39.501Z","publishedAt":"2023-02-28T08:32:03.960Z","locale":"en","__contentType":"api::page.page","navigationItemId":563,"__templateName":"Generic"},"items":[{"id":567,"title":"Production-Optimized","menuAttached":false,"order":1,"path":"/Compute/VirtualInstances/Prod","type":"INTERNAL","uiRouterKey":"production-optimized-2","slug":"compute-virtual-instances-prod","external":false,"related":{"id":657,"title":"Production-Optimized Instances","path":"/production-optimized-instances/","scheduledAt":null,"createdAt":"2023-02-20T15:13:14.415Z","updatedAt":"2025-02-11T14:06:00.080Z","publishedAt":"2023-02-28T08:34:34.739Z","locale":"en","__contentType":"api::page.page","navigationItemId":567,"__templateName":"Generic"},"items":[],"description":"Dedicated vCPU for the most demanding workloads (x86)"},{"id":566,"title":"Workload-Optimized","menuAttached":false,"order":2,"path":"/Compute/VirtualInstances/Workload-Optimized","type":"INTERNAL","uiRouterKey":"workload-optimized-1","slug":"compute-virtual-instances-workload-optimized","external":false,"related":{"id":802,"title":"Workload-Optimized Instances","path":"/workload-optimized-instances/","scheduledAt":null,"createdAt":"2023-04-25T12:38:13.577Z","updatedAt":"2025-02-11T14:01:28.392Z","publishedAt":"2023-05-26T13:36:52.797Z","locale":"en","__contentType":"api::page.page","navigationItemId":566,"__templateName":"Generic"},"items":[],"description":"Secure, scalable VMs, equipped for high memory and compute demands (x86)"},{"id":565,"title":"Cost-Optimized","menuAttached":false,"order":3,"path":"/Compute/VirtualInstances/Cost-Optimized","type":"INTERNAL","uiRouterKey":"cost-optimized-1","slug":"compute-virtual-instances-cost-optimized","external":false,"related":{"id":656,"title":"Cost-Optimized Instances","path":"/cost-optimized-instances/","scheduledAt":null,"createdAt":"2023-02-20T12:55:45.865Z","updatedAt":"2025-02-11T14:09:33.243Z","publishedAt":"2023-02-28T08:34:47.421Z","locale":"en","__contentType":"api::page.page","navigationItemId":565,"__templateName":"Generic"},"items":[],"description":"Highly reliable and priced affordably Instances with shared vCPUs (x86 and ARM)"},{"id":564,"title":"Learning","menuAttached":false,"order":4,"path":"/Compute/VirtualInstances/Learning","type":"INTERNAL","uiRouterKey":"learning-1","slug":"compute-virtual-instances-learning","external":false,"related":{"id":13,"title":"Stardust Instances","path":"/stardust-instances/","scheduledAt":null,"createdAt":"2022-04-11T09:03:33.397Z","updatedAt":"2025-01-10T14:18:17.519Z","publishedAt":"2022-04-28T17:04:10.708Z","locale":"en","__contentType":"api::page.page","navigationItemId":564,"__templateName":"Generic"},"items":[],"description":"A tiny instance to test and host your personal projects (x86)"}],"description":""},{"id":568,"title":"GPU","menuAttached":false,"order":2,"path":"/Compute/gpu","type":"INTERNAL","uiRouterKey":"gpu-8","slug":"compute-gpu","external":false,"related":{"id":1025,"title":"GPU Instances","path":"/gpu-instances/","scheduledAt":null,"createdAt":"2023-11-30T13:15:51.769Z","updatedAt":"2024-11-19T16:38:15.121Z","publishedAt":"2023-12-12T12:52:20.083Z","locale":"en","__contentType":"api::page.page","navigationItemId":568,"__templateName":"Generic"},"items":[{"id":571,"title":"L4 GPU Instance","menuAttached":false,"order":1,"path":"/Compute/gpu/L4","type":"INTERNAL","uiRouterKey":"l4-gpu-instance","slug":"compute-gpu-l4","external":false,"related":{"id":1108,"title":"L4 GPU Instance","path":"/l4-gpu-instance/","scheduledAt":null,"createdAt":"2024-02-28T16:20:43.240Z","updatedAt":"2024-11-20T14:49:27.542Z","publishedAt":"2024-03-04T13:37:45.809Z","locale":"en","__contentType":"api::page.page","navigationItemId":571,"__templateName":"Generic"},"items":[],"description":"Maximize your AI infrastructures with a versatile Instance"},{"id":572,"title":"L40S GPU Instance","menuAttached":false,"order":2,"path":"/Compute/gpu/L40s","type":"INTERNAL","uiRouterKey":"l40-s-gpu-instance","slug":"compute-gpu-l40s","external":false,"related":{"id":1221,"title":"L40S GPU Instance","path":"/l40s-gpu-instance/","scheduledAt":null,"createdAt":"2024-04-26T13:37:31.531Z","updatedAt":"2025-01-14T09:22:19.084Z","publishedAt":"2024-04-29T12:12:07.466Z","locale":"en","__contentType":"api::page.page","navigationItemId":572,"__templateName":"Generic"},"items":[],"description":"Universal Instance, faster than L4 and cheaper than H100 PCIe"},{"id":569,"title":"H100 PCIe GPU Instance","menuAttached":false,"order":3,"path":"https://www.scaleway.com/en/h100-pcie-try-it-now/","type":"EXTERNAL","uiRouterKey":"h100-pc-ie-gpu-instance-4","slug":{},"external":true,"description":"Accelerate your model training with the most high-end AI chip"},{"id":570,"title":"GPU 3070 Instances","menuAttached":false,"order":4,"path":"/Compute/gpu/3070","type":"INTERNAL","uiRouterKey":"gpu-3070-instances-1","slug":"compute-gpu-3070","external":false,"related":{"id":397,"title":"GPU 3070 Instances","path":"/gpu-3070-instances/","scheduledAt":null,"createdAt":"2022-05-30T11:52:26.506Z","updatedAt":"2023-11-16T16:38:12.184Z","publishedAt":"2022-05-30T12:33:10.212Z","locale":"en","__contentType":"api::page.page","navigationItemId":570,"__templateName":"Generic"},"items":[],"description":"Dedicated NVIDIA® RTX 3070 with the best price/performance ratio"},{"id":573,"title":"Render GPU Instances","menuAttached":false,"order":5,"path":"/Compute/gpu/render","type":"INTERNAL","uiRouterKey":"render-gpu-instances","slug":"compute-gpu-render","external":false,"related":{"id":52,"title":"GPU Render Instances","path":"/gpu-render-instances/","scheduledAt":null,"createdAt":"2022-04-21T16:00:29.592Z","updatedAt":"2024-09-25T09:40:12.404Z","publishedAt":"2022-04-28T17:12:46.136Z","locale":"en","__contentType":"api::page.page","navigationItemId":573,"__templateName":"Generic"},"items":[],"description":"Dedicated Tesla P100s for all your Machine Learning \u0026 Artificial Intelligence needs."}],"description":""},{"id":574,"title":"Serverless","menuAttached":false,"order":3,"path":"/Compute/Serverless","type":"INTERNAL","uiRouterKey":"serverless-12","slug":"compute-serverless","external":false,"related":{"id":1582,"title":"Serverless","path":"/serverless/","scheduledAt":null,"createdAt":"2025-01-08T14:22:22.570Z","updatedAt":"2025-01-08T14:39:59.326Z","publishedAt":"2025-01-08T14:39:59.247Z","locale":"en","__contentType":"api::page.page","navigationItemId":574,"__templateName":"Generic"},"items":[{"id":576,"title":"Serverless Functions","menuAttached":false,"order":1,"path":"/Compute/Serverless/Functions","type":"INTERNAL","uiRouterKey":"serverless-functions-1","slug":"compute-serverless-functions","external":false,"related":{"id":50,"title":"Serverless Functions","path":"/serverless-functions/","scheduledAt":null,"createdAt":"2022-04-21T15:28:10.687Z","updatedAt":"2025-01-24T13:36:21.096Z","publishedAt":"2022-04-28T17:12:49.569Z","locale":"en","__contentType":"api::page.page","navigationItemId":576,"__templateName":"Generic"},"items":[],"description":"Experience an easy way to run your code on the cloud"},{"id":575,"title":"Serverless Containers","menuAttached":false,"order":2,"path":"/Compute/Serverless/Containers","type":"INTERNAL","uiRouterKey":"serverless-containers-2","slug":"compute-serverless-containers","external":false,"related":{"id":7,"title":"Serverless Containers","path":"/serverless-containers/","scheduledAt":null,"createdAt":"2022-04-04T07:02:24.178Z","updatedAt":"2025-01-24T13:37:08.972Z","publishedAt":"2022-04-28T17:03:54.693Z","locale":"en","__contentType":"api::page.page","navigationItemId":575,"__templateName":"Generic"},"items":[],"description":"Easily run containers on the cloud with a single command"},{"id":579,"title":"Serverless Jobs","menuAttached":false,"order":3,"path":"/Compute/Serverless/Jobs","type":"INTERNAL","uiRouterKey":"serverless-jobs-1","slug":"compute-serverless-jobs","external":false,"related":{"id":980,"title":"Serverless Jobs","path":"/serverless-jobs/","scheduledAt":null,"createdAt":"2023-10-13T16:05:31.205Z","updatedAt":"2024-08-20T12:28:03.639Z","publishedAt":"2023-12-07T15:55:35.668Z","locale":"en","__contentType":"api::page.page","navigationItemId":579,"__templateName":"Generic"},"items":[],"description":"Run batches of tasks in the cloud"}],"description":""},{"id":580,"title":"Containers","menuAttached":false,"order":4,"path":"/Compute/Containers","type":"INTERNAL","uiRouterKey":"containers-4","slug":"compute-containers","external":false,"related":{"id":465,"title":"Containers","path":"/containers/","scheduledAt":null,"createdAt":"2022-07-29T15:09:20.535Z","updatedAt":"2024-08-28T07:05:23.005Z","publishedAt":"2023-02-27T13:53:48.270Z","locale":"en","__contentType":"api::page.page","navigationItemId":580,"__templateName":"Generic"},"items":[{"id":581,"title":"Kubernetes Kapsule","menuAttached":false,"order":1,"path":"/Compute/Containers/Kapsule","type":"INTERNAL","uiRouterKey":"kubernetes-kapsule-1","slug":"compute-containers-kapsule","external":false,"related":{"id":6,"title":"Kubernetes Kapsule","path":"/kubernetes-kapsule/","scheduledAt":null,"createdAt":"2022-04-01T15:40:18.523Z","updatedAt":"2025-02-20T10:18:20.644Z","publishedAt":"2022-11-02T17:14:27.738Z","locale":"en","__contentType":"api::page.page","navigationItemId":581,"__templateName":"Generic"},"items":[],"description":"Kubernetes exclusively for Scaleway products and resources"},{"id":582,"title":"Kubernetes Kosmos","menuAttached":false,"order":2,"path":"/Compute/Containers/Kosmos","type":"INTERNAL","uiRouterKey":"kubernetes-kosmos-1","slug":"compute-containers-kosmos","external":false,"related":{"id":43,"title":"Kubernetes Kosmos","path":"/kubernetes-kosmos/","scheduledAt":null,"createdAt":"2022-04-20T17:18:27.347Z","updatedAt":"2024-07-12T09:35:39.810Z","publishedAt":"2022-04-28T17:13:15.597Z","locale":"en","__contentType":"api::page.page","navigationItemId":582,"__templateName":"Generic"},"items":[],"description":"Multi-cloud Kubernetes for Scaleway and external providers resources"},{"id":583,"title":"Container Registry","menuAttached":false,"order":3,"path":"/Compute/Containers/containerregisrt","type":"INTERNAL","uiRouterKey":"container-registry-1","slug":"compute-containers-containerregisrt","external":false,"related":{"id":39,"title":"Container Registry","path":"/container-registry/","scheduledAt":null,"createdAt":"2022-04-20T14:07:31.417Z","updatedAt":"2023-11-15T08:49:34.191Z","publishedAt":"2022-04-28T17:06:10.179Z","locale":"en","__contentType":"api::page.page","navigationItemId":583,"__templateName":"Generic"},"items":[],"description":"An easy-to-use Docker repository"}],"description":""}],"description":""},{"id":584,"title":"AI","menuAttached":false,"order":3,"path":"/AI","type":"WRAPPER","uiRouterKey":"ai","slug":"ai-1","external":false,"items":[{"id":585,"title":"Clusters","menuAttached":false,"order":1,"path":"/AI/Clusters","type":"WRAPPER","uiRouterKey":"clusters-1","slug":"ai-clusters","external":false,"items":[{"id":588,"title":"Custom-built Clusters","menuAttached":false,"order":1,"path":"/AI/Clusters/AIsuper","type":"INTERNAL","uiRouterKey":"custom-built-clusters","slug":"ai-clusters-a-isuper","external":false,"related":{"id":953,"title":"Custom-built Clusters","path":"/custom-built-clusters/","scheduledAt":null,"createdAt":"2023-09-22T14:14:40.961Z","updatedAt":"2024-10-29T12:48:55.663Z","publishedAt":"2023-10-04T14:49:01.987Z","locale":"en","__contentType":"api::page.page","navigationItemId":588,"__templateName":"Generic"},"items":[],"description":"Build the next Foundation Model with one of the fastest and most energy-efficient supercomputers in the world"},{"id":776,"title":"On Demand Cluster","menuAttached":false,"order":2,"path":"/AI/Clusters/Clusterondemand","type":"INTERNAL","uiRouterKey":"on-demand-cluster","slug":"ai-clusters-clusterondemand","external":false,"related":{"id":1266,"title":"Cluster On Demand ","path":"/cluster-on-demand/","scheduledAt":null,"createdAt":"2024-05-16T15:00:19.723Z","updatedAt":"2024-11-08T08:52:40.598Z","publishedAt":"2024-05-21T14:10:00.511Z","locale":"en","__contentType":"api::page.page","navigationItemId":776,"__templateName":"Generic"},"items":[],"description":"Rent a GPU-cluster from 32 to more than a thousand GPUs to speed up distributed training"}],"description":""},{"id":592,"title":"Model-as-a-service","menuAttached":false,"order":2,"path":"/AI/ManagedServices","type":"WRAPPER","uiRouterKey":"model-as-a-service-1","slug":"ai-managed-services","external":false,"items":[{"id":593,"title":"Managed Inference","menuAttached":false,"order":1,"path":"/AI/ManagedServices/llm","type":"INTERNAL","uiRouterKey":"managed-inference-2","slug":"ai-managed-services-llm","external":false,"related":{"id":1303,"title":"Inference","path":"/inference/","scheduledAt":null,"createdAt":"2024-06-13T13:16:26.427Z","updatedAt":"2025-02-10T10:29:33.032Z","publishedAt":"2024-06-28T12:43:39.677Z","locale":"en","__contentType":"api::page.page","navigationItemId":593,"__templateName":"Generic"},"items":[],"description":"Deploy AI models in a dedicated inference infrastructure. Get tailored security and predictable throughput"},{"id":824,"title":"Generative APIs","menuAttached":false,"order":2,"path":"/AI/ManagedServices/GenerativeAPIs","type":"INTERNAL","uiRouterKey":"generative-ap-is-2","slug":"ai-managed-services-generative-ap-is","external":false,"related":{"id":1418,"title":"Generative APIs","path":"/generative-apis/","scheduledAt":null,"createdAt":"2024-10-10T16:23:00.732Z","updatedAt":"2025-02-13T16:06:23.818Z","publishedAt":"2024-10-11T12:17:56.286Z","locale":"en","__contentType":"api::page.page","navigationItemId":824,"__templateName":"Generic"},"items":[],"description":"Consume AI models instantly via a simple API call. All hosted in Europe"}],"description":""},{"id":586,"title":"GPU Instances","menuAttached":false,"order":3,"path":"/AI/gpu","type":"WRAPPER","uiRouterKey":"gpu-instances","slug":"ai-gpu","external":false,"items":[{"id":589,"title":"L40S GPU Instance","menuAttached":false,"order":1,"path":"https://www.scaleway.com/en/l40s-gpu-instance/","type":"EXTERNAL","uiRouterKey":"l40-s-gpu-instance-1","slug":{},"external":true,"description":"Accelerate the next generation of AI-enabled applications with the universal L40S GPU Instance, faster than L4 and cheaper than H100 PCIe"},{"id":590,"title":"L4 GPU Instance","menuAttached":false,"order":2,"path":"https://www.scaleway.com/en/l4-gpu-instance/","type":"EXTERNAL","uiRouterKey":"l4-gpu-instance-1","slug":{},"external":true,"description":"Maximize your AI infrastructure's potential with a versatile and cost-effective GPU Instance"},{"id":587,"title":"H100 PCIe GPU Instance","menuAttached":false,"order":3,"path":"https://www.scaleway.com/en/h100-pcie-try-it-now/","type":"EXTERNAL","uiRouterKey":"h100-pc-ie-gpu-instance-2","slug":{},"external":true,"description":"Accelerate your model training with the most high-end AI chip"},{"id":591,"title":"Render GPU Instance","menuAttached":false,"order":4,"path":"https://www.scaleway.com/en/gpu-render-instances/","type":"EXTERNAL","uiRouterKey":"render-gpu-instance-1","slug":{},"external":true,"description":"Dedicated Tesla P100s for all your Machine Learning \u0026 Artificial Intelligence needs"}],"description":""}],"description":""},{"id":594,"title":"Storage","menuAttached":false,"order":4,"path":"/Storage","type":"WRAPPER","uiRouterKey":"storage-3","slug":"storage-2","external":false,"items":[{"id":602,"title":"Storage","menuAttached":false,"order":1,"path":"/Storage/storage","type":"WRAPPER","uiRouterKey":"storage-4","slug":"storage-storage","external":false,"items":[{"id":604,"title":"Object Storage","menuAttached":false,"order":1,"path":"/Storage/storage/ObjectStorage","type":"INTERNAL","uiRouterKey":"object-storage-4","slug":"storage-storage-object-storage","external":false,"related":{"id":652,"title":"Object Storage","path":"/object-storage/","scheduledAt":null,"createdAt":"2023-02-16T09:44:56.414Z","updatedAt":"2024-12-02T14:09:58.690Z","publishedAt":"2023-03-07T18:05:15.061Z","locale":"en","__contentType":"api::page.page","navigationItemId":604,"__templateName":"Generic"},"items":[],"description":"Amazon S3-compatible and Multi-AZ resilient object storage service. Ensuring high availability for your data"},{"id":605,"title":"Scaleway Glacier","menuAttached":false,"order":2,"path":"/Storage/storage/glacier","type":"INTERNAL","uiRouterKey":"scaleway-glacier-1","slug":"storage-storage-glacier","external":false,"related":{"id":17,"title":"Glacier Cold storage","path":"/glacier-cold-storage/","scheduledAt":null,"createdAt":"2022-04-11T11:58:13.079Z","updatedAt":"2024-10-25T13:13:55.154Z","publishedAt":"2022-04-28T17:13:24.608Z","locale":"en","__contentType":"api::page.page","navigationItemId":605,"__templateName":"Generic"},"items":[],"description":"Cold Storage class to secure long-term object storage. Ideal for deep archived data."},{"id":606,"title":"Block Storage","menuAttached":false,"order":3,"path":"/Storage/storage/BlockStorage","type":"INTERNAL","uiRouterKey":"block-storage-3","slug":"storage-storage-block-storage","external":false,"related":{"id":141,"title":"Block Storage","path":"/block-storage/","scheduledAt":null,"createdAt":"2022-05-02T08:20:39.280Z","updatedAt":"2025-02-05T14:21:18.667Z","publishedAt":"2022-05-02T08:28:12.783Z","locale":"en","__contentType":"api::page.page","navigationItemId":606,"__templateName":"Generic"},"items":[],"description":"Flexible and reliable storage for demanding workloads"}],"description":""}],"description":""},{"id":595,"title":"Network","menuAttached":false,"order":5,"path":"/Network","type":"WRAPPER","uiRouterKey":"network-3","slug":"network-4","external":false,"items":[{"id":603,"title":"Network","menuAttached":false,"order":1,"path":"/Network/Network","type":"WRAPPER","uiRouterKey":"network-4","slug":"network-network","external":false,"items":[{"id":607,"title":"Virtual Private Cloud","menuAttached":false,"order":1,"path":"/Network/Network/VPC","type":"INTERNAL","uiRouterKey":"virtual-private-cloud-1","slug":"network-network-vpc","external":false,"related":{"id":885,"title":"VPC","path":"/vpc/","scheduledAt":null,"createdAt":"2023-07-11T14:38:07.412Z","updatedAt":"2025-01-03T17:06:24.192Z","publishedAt":"2023-07-11T14:38:10.387Z","locale":"en","__contentType":"api::page.page","navigationItemId":607,"__templateName":"Generic"},"items":[],"description":"Secure your cloud resources with ease on a resilient regional network"},{"id":609,"title":"Public Gateway","menuAttached":false,"order":2,"path":"/Network/Network/public","type":"INTERNAL","uiRouterKey":"public-gateway-1","slug":"network-network-public","external":false,"related":{"id":54,"title":"Public Gateway","path":"/public-gateway/","scheduledAt":null,"createdAt":"2022-04-22T09:34:12.578Z","updatedAt":"2024-09-11T14:24:49.432Z","publishedAt":"2022-04-28T17:13:01.025Z","locale":"en","__contentType":"api::page.page","navigationItemId":609,"__templateName":"Generic"},"items":[],"description":" A single and secure entrance to your infrastructure"},{"id":608,"title":"Load Balancer","menuAttached":false,"order":3,"path":"/Network/Network/load","type":"INTERNAL","uiRouterKey":"load-balancer-1","slug":"network-network-load","external":false,"related":{"id":45,"title":"Load Balancer","path":"/load-balancer/","scheduledAt":null,"createdAt":"2022-04-21T07:46:46.140Z","updatedAt":"2024-07-24T14:48:37.806Z","publishedAt":"2022-11-18T08:58:30.309Z","locale":"en","__contentType":"api::page.page","navigationItemId":608,"__templateName":"Generic"},"items":[],"description":"Improve the performance of your services as you grow"},{"id":610,"title":"Domains and DNS","menuAttached":false,"order":4,"path":"/Network/Network/DomainsandDNS","type":"INTERNAL","uiRouterKey":"domains-and-dns-1","slug":"network-network-domainsand-dns","external":false,"related":{"id":44,"title":"Domains and DNS","path":"/domains-and-dns/","scheduledAt":null,"createdAt":"2022-04-21T07:26:18.059Z","updatedAt":"2024-03-05T17:01:32.782Z","publishedAt":"2022-04-28T17:13:12.082Z","locale":"en","__contentType":"api::page.page","navigationItemId":610,"__templateName":"Generic"},"items":[],"description":"Buy domain names and manage DNS. Find your favourite extensions at a fair price"},{"id":792,"title":"IPAM (IP Address Manager)","menuAttached":false,"order":5,"path":"/Network/Network/IPAM","type":"INTERNAL","uiRouterKey":"ipam-ip-address-manager","slug":"network-network-ipam","external":false,"related":{"id":1300,"title":"IPAM","path":"/ipam/","scheduledAt":null,"createdAt":"2024-06-07T13:07:18.728Z","updatedAt":"2024-11-29T16:49:38.669Z","publishedAt":"2024-07-10T07:39:07.627Z","locale":"en","__contentType":"api::page.page","navigationItemId":792,"__templateName":"Generic"},"items":[],"description":"Centralize and simplify your Scaleway IP address management"},{"id":820,"title":"Edge Services","menuAttached":false,"order":6,"path":"/Network/Network/EdgeServices","type":"INTERNAL","uiRouterKey":"edge-services-2","slug":"network-network-edge-services","external":false,"related":{"id":1614,"title":"Edge Services","path":"/edge-services/","scheduledAt":null,"createdAt":"2025-01-31T15:54:24.871Z","updatedAt":"2025-01-31T16:01:57.242Z","publishedAt":"2025-01-31T15:54:28.318Z","locale":"en","__contentType":"api::page.page","navigationItemId":820,"__templateName":"Generic"},"items":[],"description":"Expose your HTTP services to the internet with security, reliability, and efficiency by design."},{"id":858,"title":"InterLink","menuAttached":false,"order":7,"path":"/Network/Network/InterLink","type":"INTERNAL","uiRouterKey":"inter-link","slug":"network-network-inter-link","external":false,"related":{"id":900,"title":"Scaleway InterLink","path":"/scaleway-interlink/","scheduledAt":null,"createdAt":"2023-08-03T14:39:22.643Z","updatedAt":"2025-02-06T14:54:50.915Z","publishedAt":"2023-08-04T09:53:13.589Z","locale":"en","__contentType":"api::page.page","navigationItemId":858,"__templateName":"Generic"},"items":[],"description":"Establish a hosted connection from your infrastructure to your VPC via a partner's network"}],"description":""}],"description":""},{"id":836,"title":"Data","menuAttached":false,"order":6,"path":"/data","type":"WRAPPER","uiRouterKey":"data-2","slug":"data-3","external":false,"items":[{"id":837,"title":"Databases","menuAttached":false,"order":1,"path":"/data/Databases","type":"WRAPPER","uiRouterKey":"databases","slug":"data-databases","external":false,"items":[{"id":838,"title":"Managed Database for PostgreSQL and MySQL","menuAttached":false,"order":1,"path":"/data/Databases/PostgreSQL_MySQL","type":"INTERNAL","uiRouterKey":"managed-database-for-postgre-sql-and-my-sql","slug":"data-databases-postgre-sql-my-sql","external":false,"related":{"id":48,"title":"Database","path":"/database/","scheduledAt":null,"createdAt":"2022-04-21T14:06:34.262Z","updatedAt":"2024-07-02T15:50:10.807Z","publishedAt":"2022-04-28T17:12:57.201Z","locale":"en","__contentType":"api::page.page","navigationItemId":838,"__templateName":"Generic"},"items":[],"description":"Start seamless database operations"},{"id":839,"title":"Serverless SQL Database","menuAttached":false,"order":2,"path":"/data/Databases/SQL_database","type":"INTERNAL","uiRouterKey":"serverless-sql-database-1","slug":"data-databases-sql-database","external":false,"related":{"id":823,"title":"Serverless Sql Database","path":"/serverless-sql-database/","scheduledAt":null,"createdAt":"2023-05-11T22:46:48.805Z","updatedAt":"2025-02-21T08:33:55.172Z","publishedAt":"2023-05-11T22:47:00.320Z","locale":"en","__contentType":"api::page.page","navigationItemId":839,"__templateName":"Generic"},"items":[],"description":"Go full serverless and take the complexity out of PostgreSQL database"},{"id":840,"title":"Managed Database for Redis®","menuAttached":false,"order":3,"path":"/data/Databases/redis","type":"INTERNAL","uiRouterKey":"managed-database-for-redis-1","slug":"data-databases-redis","external":false,"related":{"id":427,"title":"Managed Database for Redis™","path":"/managed-database-for-redistm/","scheduledAt":null,"createdAt":"2022-06-10T13:30:28.356Z","updatedAt":"2024-12-02T13:13:32.070Z","publishedAt":"2022-07-27T15:29:59.282Z","locale":"en","__contentType":"api::page.page","navigationItemId":840,"__templateName":"Generic"},"items":[],"description":"Fully managed Redis®* in seconds"},{"id":841,"title":"Managed MongoDB®","menuAttached":false,"order":4,"path":"/data/Databases/MongoDB","type":"INTERNAL","uiRouterKey":"managed-mongo-db-1","slug":"data-databases-mongo-db","external":false,"related":{"id":890,"title":"Managed MongoDB","path":"/managed-mongodb/","scheduledAt":null,"createdAt":"2023-07-25T07:58:39.536Z","updatedAt":"2025-02-21T08:27:45.300Z","publishedAt":"2023-10-03T08:31:21.477Z","locale":"en","__contentType":"api::page.page","navigationItemId":841,"__templateName":"Generic"},"items":[],"description":"Create a scalable, secure, and fully managed NoSQL solution"}],"description":""},{"id":843,"title":"Messaging and Queuing","menuAttached":false,"order":2,"path":"/data/mq","type":"WRAPPER","uiRouterKey":"messaging-and-queuing-1","slug":"data-mq","external":false,"items":[{"id":846,"title":"NATS","menuAttached":false,"order":1,"path":"/data/mq/NATS","type":"INTERNAL","uiRouterKey":"nats","slug":"data-mq-nats","external":false,"related":{"id":1506,"title":"NATS","path":"/nats/","scheduledAt":null,"createdAt":"2024-12-02T16:34:48.084Z","updatedAt":"2025-02-06T15:43:48.739Z","publishedAt":"2024-12-04T14:30:28.012Z","locale":"en","__contentType":"api::page.page","navigationItemId":846,"__templateName":"Generic"},"items":[],"description":"Build distributed and scalable client-server applications"},{"id":844,"title":"Queues","menuAttached":false,"order":2,"path":"/data/mq/Queues","type":"INTERNAL","uiRouterKey":"queues","slug":"data-mq-queues","external":false,"related":{"id":1505,"title":"Queues","path":"/queues/","scheduledAt":null,"createdAt":"2024-12-02T15:40:46.474Z","updatedAt":"2024-12-04T14:31:05.795Z","publishedAt":"2024-12-04T14:31:05.505Z","locale":"en","__contentType":"api::page.page","navigationItemId":844,"__templateName":"Generic"},"items":[],"description":"Create a queue, configure its delivery and message parameters"},{"id":845,"title":"Topics and Events","menuAttached":false,"order":3,"path":"/data/mq/TopicsEvents","type":"INTERNAL","uiRouterKey":"topics-and-events","slug":"data-mq-topics-events","external":false,"related":{"id":1509,"title":"Topics \u0026 Events","path":"/topics-and-events/","scheduledAt":null,"createdAt":"2024-12-02T17:09:09.294Z","updatedAt":"2025-02-06T15:45:39.733Z","publishedAt":"2024-12-04T14:30:14.535Z","locale":"en","__contentType":"api::page.page","navigationItemId":845,"__templateName":"Generic"},"items":[],"description":"Sent to a variety of devices and platforms through a single code interface"}],"description":""}],"description":""},{"id":596,"title":"Tools","menuAttached":false,"order":7,"path":"/ManagedServices","type":"WRAPPER","uiRouterKey":"tools","slug":"managed-services-2","external":false,"items":[{"id":619,"title":"Managed Services","menuAttached":false,"order":1,"path":"/ManagedServices/ManagedServices","type":"WRAPPER","uiRouterKey":"managed-services","slug":"managed-services-managed-services","external":false,"items":[{"id":623,"title":"Cockpit","menuAttached":false,"order":1,"path":"/ManagedServices/ManagedServices/Cockpit","type":"INTERNAL","uiRouterKey":"cockpit-2","slug":"managed-services-managed-services-cockpit","external":false,"related":{"id":814,"title":"Cockpit","path":"/cockpit/","scheduledAt":null,"createdAt":"2023-05-02T08:04:46.085Z","updatedAt":"2024-12-02T08:25:58.250Z","publishedAt":"2023-05-04T16:18:10.562Z","locale":"en","__contentType":"api::page.page","navigationItemId":623,"__templateName":"Generic"},"items":[],"description":"Monitor infrastructures in minutes with a fully managed observability solution"},{"id":620,"title":"Web Hosting","menuAttached":false,"order":2,"path":"/ManagedServices/ManagedServices/hosting","type":"INTERNAL","uiRouterKey":"web-hosting-4","slug":"managed-services-managed-services-hosting","external":false,"related":{"id":47,"title":"Web hosting","path":"/web-hosting/","scheduledAt":null,"createdAt":"2022-04-21T11:51:48.689Z","updatedAt":"2024-11-20T15:59:55.910Z","publishedAt":"2022-04-28T13:34:58.879Z","locale":"en","__contentType":"api::page.page","navigationItemId":620,"__templateName":"Generic"},"items":[],"description":"Hosting for individuals, professionals, and everyone in between."},{"id":621,"title":"Web Platform","menuAttached":false,"order":3,"path":"/ManagedServices/ManagedServices/WebPlatform","type":"INTERNAL","uiRouterKey":"web-platform-2","slug":"managed-services-managed-services-web-platform","external":false,"related":{"id":576,"title":"Web Platform - powered by Clever Cloud","path":"/web-platform-powered-by-clever-cloud/","scheduledAt":null,"createdAt":"2022-12-07T14:07:50.856Z","updatedAt":"2023-11-16T15:19:36.970Z","publishedAt":"2022-12-13T08:01:42.916Z","locale":"en","__contentType":"api::page.page","navigationItemId":621,"__templateName":"Generic"},"items":[],"description":"Ship your applications only in a few clicks."},{"id":622,"title":"Transactional Email","menuAttached":false,"order":4,"path":"/ManagedServices/ManagedServices/tem","type":"INTERNAL","uiRouterKey":"transactional-email-2","slug":"managed-services-managed-services-tem","external":false,"related":{"id":776,"title":"Transactional Email (TEM)","path":"/transactional-email-tem/","scheduledAt":null,"createdAt":"2023-04-05T16:33:35.536Z","updatedAt":"2024-10-21T14:45:56.496Z","publishedAt":"2023-04-06T10:30:43.491Z","locale":"en","__contentType":"api::page.page","navigationItemId":622,"__templateName":"Generic"},"items":[],"description":"Instant delivery of your transactional emails"},{"id":842,"title":"Distributed Data Lab","menuAttached":false,"order":5,"path":"/ManagedServices/ManagedServices/DataLab","type":"INTERNAL","uiRouterKey":"distributed-data-lab-1","slug":"managed-services-managed-services-data-lab","external":false,"related":{"id":949,"title":"Distributed Data Lab ","path":"/distributed-data-lab/","scheduledAt":null,"createdAt":"2023-09-21T11:57:12.802Z","updatedAt":"2025-01-03T13:55:54.202Z","publishedAt":"2024-09-27T15:10:48.257Z","locale":"en","__contentType":"api::page.page","navigationItemId":842,"__templateName":"Generic"},"items":[],"description":"Speed up data processing over very large volumes of data with an Apache Spark™ managed solution"},{"id":784,"title":"IoT Hub","menuAttached":false,"order":6,"path":"/ManagedServices/ManagedServices/iot","type":"INTERNAL","uiRouterKey":"io-t-hub","slug":"managed-services-managed-services-iot","external":false,"related":{"id":31,"title":"Iot hub","path":"/iot-hub/","scheduledAt":null,"createdAt":"2022-04-20T04:58:03.085Z","updatedAt":"2023-11-15T15:42:53.313Z","publishedAt":"2022-04-28T17:13:21.005Z","locale":"en","__contentType":"api::page.page","navigationItemId":784,"__templateName":"Generic"},"items":[],"description":"A purpose-built bridge between connected hardware and cloud."}],"description":""},{"id":615,"title":"Security \u0026 Organization","menuAttached":false,"order":2,"path":"/ManagedServices/SecurityandAccount","type":"WRAPPER","uiRouterKey":"security-3","slug":"managed-services-securityand-account","external":false,"items":[{"id":618,"title":"Identity and Access Management (IAM)","menuAttached":false,"order":1,"path":"/ManagedServices/SecurityandAccount/iam","type":"INTERNAL","uiRouterKey":"identity-and-access-management-iam-1","slug":"managed-services-securityand-account-iam","external":false,"related":{"id":569,"title":"IAM","path":"/iam/","scheduledAt":null,"createdAt":"2022-12-02T16:25:06.762Z","updatedAt":"2025-01-10T14:30:40.377Z","publishedAt":"2022-12-06T15:27:30.794Z","locale":"en","__contentType":"api::page.page","navigationItemId":618,"__templateName":"Generic"},"items":[],"description":"The easiest way to safely collaborate in the cloud"},{"id":616,"title":"Secret Manager","menuAttached":false,"order":2,"path":"/ManagedServices/SecurityandAccount/secretmanager","type":"INTERNAL","uiRouterKey":"secret-manager-1","slug":"managed-services-securityand-account-secretmanager","external":false,"related":{"id":779,"title":"Secret Manager","path":"/secret-manager/","scheduledAt":null,"createdAt":"2023-04-11T11:04:18.808Z","updatedAt":"2024-08-28T09:57:43.021Z","publishedAt":"2023-04-26T07:47:45.718Z","locale":"en","__contentType":"api::page.page","navigationItemId":616,"__templateName":"Generic"},"items":[],"description":"Protect your sensitive data across your cloud infrastructure"},{"id":617,"title":"Cost Manager","menuAttached":false,"order":3,"path":"/ManagedServices/SecurityandAccount/cost-manager","type":"INTERNAL","uiRouterKey":"cost-manager-1","slug":"managed-services-securityand-account-cost-manager","external":false,"related":{"id":1186,"title":"Cost Manager","path":"/cost-manager/","scheduledAt":null,"createdAt":"2024-04-08T07:36:07.839Z","updatedAt":"2024-04-08T09:14:21.699Z","publishedAt":"2024-04-08T09:14:21.666Z","locale":"en","__contentType":"api::page.page","navigationItemId":617,"__templateName":"Generic"},"items":[],"description":"Easily track your consumption in an all-in-one tool"},{"id":830,"title":"Environmental Footprint Calculator","menuAttached":false,"order":4,"path":"/ManagedServices/SecurityandAccount/Footprint","type":"INTERNAL","uiRouterKey":"environmental-footprint-calculator","slug":"managed-services-securityand-account-footprint","external":false,"related":{"id":1450,"title":"Environmental Footprint Calculator","path":"/environmental-footprint-calculator/","scheduledAt":null,"createdAt":"2024-10-28T14:47:30.518Z","updatedAt":"2025-01-27T14:26:21.239Z","publishedAt":"2024-11-04T12:12:34.311Z","locale":"en","__contentType":"api::page.page","navigationItemId":830,"__templateName":"Generic"},"items":[],"description":"Accurately track your environmental impact and make informed choices"}],"description":""},{"id":624,"title":"Developer Tools","menuAttached":false,"order":3,"path":"/ManagedServices/DeveloperTools","type":"WRAPPER","uiRouterKey":"developer-tools","slug":"managed-services-developer-tools","external":false,"items":[{"id":625,"title":"Scaleway API","menuAttached":false,"order":1,"path":"https://www.scaleway.com/en/developers/api/","type":"EXTERNAL","uiRouterKey":"scaleway-api-2","slug":{},"external":true,"description":"The Public Interface for developers"},{"id":626,"title":"CLI","menuAttached":false,"order":2,"path":"/ManagedServices/DeveloperTools/cli","type":"INTERNAL","uiRouterKey":"cli-2","slug":"managed-services-developer-tools-cli","external":false,"related":{"id":187,"title":"CLI","path":"/cli/","scheduledAt":null,"createdAt":"2022-05-03T08:37:17.214Z","updatedAt":"2024-08-22T05:35:23.543Z","publishedAt":"2022-05-03T11:43:09.246Z","locale":"en","__contentType":"api::page.page","navigationItemId":626,"__templateName":"Generic"},"items":[],"description":"Deploy and manage your infrastructure directly from the command line"},{"id":627,"title":"Terraform","menuAttached":false,"order":3,"path":"/ManagedServices/DeveloperTools/terraform","type":"INTERNAL","uiRouterKey":"terraform-1","slug":"managed-services-developer-tools-terraform","external":false,"related":{"id":40,"title":"Terraform","path":"/terraform/","scheduledAt":null,"createdAt":"2022-04-20T14:37:30.508Z","updatedAt":"2023-11-15T08:32:57.793Z","publishedAt":"2022-04-28T17:05:15.208Z","locale":"en","__contentType":"api::page.page","navigationItemId":627,"__templateName":"Generic"},"items":[],"description":"Securely and efficiently provision and manage Infrastructure as Code with Terraform"}],"description":""}],"description":""},{"id":597,"title":"Solutions","menuAttached":false,"order":8,"path":"/Solutions","type":"WRAPPER","uiRouterKey":"solutions-2","slug":"solutions-2","external":false,"items":[{"id":628,"title":"Industries","menuAttached":false,"order":1,"path":"/Solutions/Industries","type":"WRAPPER","uiRouterKey":"industries-1","slug":"solutions-industries","external":false,"items":[{"id":631,"title":"Gaming","menuAttached":false,"order":1,"path":"/Solutions/Industries/Gaming","type":"INTERNAL","uiRouterKey":"gaming-1","slug":"solutions-industries-gaming","external":false,"related":{"id":1024,"title":"Gaming Cloud Solutions","path":"/gaming-cloud-solutions/","scheduledAt":null,"createdAt":"2023-11-29T17:06:47.458Z","updatedAt":"2024-09-24T13:29:47.657Z","publishedAt":"2023-12-13T16:53:50.074Z","locale":"en","__contentType":"api::page.page","navigationItemId":631,"__templateName":"Generic"},"items":[],"description":""},{"id":630,"title":"Public Sector","menuAttached":false,"order":2,"path":"/Solutions/Industries/PublicSector","type":"INTERNAL","uiRouterKey":"public-sector","slug":"solutions-industries-public-sector","external":false,"related":{"id":986,"title":"Public sector solutions","path":"/public-sector-solutions/","scheduledAt":null,"createdAt":"2023-10-20T14:23:52.057Z","updatedAt":"2024-09-30T17:00:38.498Z","publishedAt":"2023-11-30T14:58:23.419Z","locale":"en","__contentType":"api::page.page","navigationItemId":630,"__templateName":"Generic"},"items":[],"description":""},{"id":633,"title":"Media and Entertainment","menuAttached":false,"order":3,"path":"/Solutions/Industries/MediaandEntertainment","type":"INTERNAL","uiRouterKey":"media-and-entertainment","slug":"solutions-industries-mediaand-entertainment","external":false,"related":{"id":1048,"title":"Media and Entertainment","path":"/media-and-entertainment/","scheduledAt":null,"createdAt":"2023-12-13T16:23:27.055Z","updatedAt":"2024-09-24T13:30:40.809Z","publishedAt":"2024-01-02T18:08:08.725Z","locale":"en","__contentType":"api::page.page","navigationItemId":633,"__templateName":"Generic"},"items":[],"description":""},{"id":632,"title":"Retail and E-commerce","menuAttached":false,"order":4,"path":"/Solutions/Industries/Retail","type":"INTERNAL","uiRouterKey":"retail-and-e-commerce-2","slug":"solutions-industries-retail","external":false,"related":{"id":1105,"title":"E-commerce retail Solutions","path":"/e-commerce-retail-solutions/","scheduledAt":null,"createdAt":"2024-02-28T09:44:45.583Z","updatedAt":"2025-02-20T16:47:32.650Z","publishedAt":"2024-04-02T14:56:24.762Z","locale":"en","__contentType":"api::page.page","navigationItemId":632,"__templateName":"Generic"},"items":[],"description":""},{"id":794,"title":"Financial Services","menuAttached":false,"order":5,"path":"/Solutions/Industries/FinancialServices","type":"INTERNAL","uiRouterKey":"financial-services","slug":"solutions-industries-financial-services","external":false,"related":{"id":1381,"title":"Financial services solutions","path":"/financial-services-solutions/","scheduledAt":null,"createdAt":"2024-08-06T12:19:51.917Z","updatedAt":"2024-11-12T09:58:52.666Z","publishedAt":"2024-08-06T12:31:25.580Z","locale":"en","__contentType":"api::page.page","navigationItemId":794,"__templateName":"Generic"},"items":[],"description":""},{"id":826,"title":"Industrial","menuAttached":false,"order":6,"path":"/Solutions/Industries/Industrial","type":"INTERNAL","uiRouterKey":"industrial","slug":"solutions-industries-industrial","external":false,"related":{"id":1411,"title":"Industrial solutions","path":"/industrial-solutions/","scheduledAt":null,"createdAt":"2024-10-02T10:14:37.728Z","updatedAt":"2025-01-27T09:37:50.233Z","publishedAt":"2024-10-03T16:29:42.042Z","locale":"en","__contentType":"api::page.page","navigationItemId":826,"__templateName":"Generic"},"items":[],"description":""},{"id":875,"title":"Technology","menuAttached":false,"order":7,"path":"/Solutions/Industries/Technology","type":"INTERNAL","uiRouterKey":"technology","slug":"solutions-industries-technology","external":false,"related":{"id":1572,"title":"tech-solutions","path":"/tech-solutions/","scheduledAt":null,"createdAt":"2024-12-23T10:44:13.921Z","updatedAt":"2024-12-27T13:27:25.098Z","publishedAt":"2024-12-23T10:49:09.338Z","locale":"en","__contentType":"api::page.page","navigationItemId":875,"__templateName":"Generic"},"items":[],"description":""},{"id":876,"title":"Healthcare","menuAttached":false,"order":8,"path":"/Solutions/Industries/Healthcare","type":"INTERNAL","uiRouterKey":"healthcare","slug":"solutions-industries-healthcare","external":false,"related":{"id":1579,"title":"healthcare and life sciences solutions","path":"/healthcare-and-life-sciences-solutions/","scheduledAt":null,"createdAt":"2025-01-03T15:32:23.751Z","updatedAt":"2025-01-08T15:53:36.314Z","publishedAt":"2025-01-08T14:08:25.957Z","locale":"en","__contentType":"api::page.page","navigationItemId":876,"__templateName":"Generic"},"items":[],"description":""}],"description":""},{"id":635,"title":"Use Cases","menuAttached":false,"order":2,"path":"/Solutions/usecases","type":"WRAPPER","uiRouterKey":"use-cases","slug":"solutions-usecases","external":false,"items":[{"id":868,"title":"Artificial Intelligence","menuAttached":false,"order":1,"path":"/Solutions/usecases/ai","type":"INTERNAL","uiRouterKey":"artificial-intelligence-2","slug":"solutions-usecases-ai","external":false,"related":{"id":1443,"title":"ai solutions","path":"/ai-solutions/","scheduledAt":null,"createdAt":"2024-10-25T08:04:00.807Z","updatedAt":"2024-11-04T10:57:00.737Z","publishedAt":"2024-10-28T08:49:11.873Z","locale":"en","__contentType":"api::page.page","navigationItemId":868,"__templateName":"Generic"},"items":[],"description":""},{"id":638,"title":"Cloud Storage Solutions","menuAttached":false,"order":2,"path":"/Solutions/usecases/cloudstorage","type":"INTERNAL","uiRouterKey":"cloud-storage-solutions","slug":"solutions-usecases-cloudstorage","external":false,"related":{"id":595,"title":"Cloud Storage Solutions","path":"/cloud-storage-solutions/","scheduledAt":null,"createdAt":"2022-12-19T13:31:12.676Z","updatedAt":"2024-10-25T13:40:34.304Z","publishedAt":"2023-01-31T10:48:28.580Z","locale":"en","__contentType":"api::page.page","navigationItemId":638,"__templateName":"Generic"},"items":[],"description":""},{"id":637,"title":"Kubernetes Solutions","menuAttached":false,"order":3,"path":"/Solutions/usecases/kub-sol","type":"INTERNAL","uiRouterKey":"kubernetes-solutions-1","slug":"solutions-usecases-kub-sol","external":false,"related":{"id":616,"title":"Kubernetes Solutions","path":"/kubernetes-solutions/","scheduledAt":null,"createdAt":"2023-01-10T16:25:48.652Z","updatedAt":"2024-11-20T16:45:40.105Z","publishedAt":"2023-03-28T07:49:24.834Z","locale":"en","__contentType":"api::page.page","navigationItemId":637,"__templateName":"Generic"},"items":[],"description":""},{"id":636,"title":"Serverless Applications","menuAttached":false,"order":4,"path":"/Solutions/usecases/ServerlessApplications","type":"INTERNAL","uiRouterKey":"serverless-applications-1","slug":"solutions-usecases-serverless-applications","external":false,"related":{"id":780,"title":"Build Scalable Applications With Serverless","path":"/build-scalable-applications-with-serverless/","scheduledAt":null,"createdAt":"2023-04-12T08:42:06.395Z","updatedAt":"2024-05-15T13:59:21.827Z","publishedAt":"2023-05-12T06:59:34.924Z","locale":"en","__contentType":"api::page.page","navigationItemId":636,"__templateName":"Generic"},"items":[],"description":""},{"id":869,"title":"Managed Web Hosting","menuAttached":false,"order":5,"path":"/Solutions/usecases/wenhosting","type":"INTERNAL","uiRouterKey":"managed-web-hosting-1","slug":"solutions-usecases-wenhosting","external":false,"related":{"id":827,"title":"Managed Web Hosting","path":"/managed-web-hosting/","scheduledAt":null,"createdAt":"2023-05-15T09:39:39.531Z","updatedAt":"2024-08-28T06:42:02.109Z","publishedAt":"2023-05-15T12:31:13.810Z","locale":"en","__contentType":"api::page.page","navigationItemId":869,"__templateName":"Generic"},"items":[],"description":""}],"description":""},{"id":870,"title":"For Startups","menuAttached":false,"order":3,"path":"/Solutions/Startups","type":"WRAPPER","uiRouterKey":"for-startups","slug":"solutions-startups","external":false,"items":[{"id":873,"title":"Apply for Startup Program","menuAttached":false,"order":1,"path":"/Solutions/Startups/Apply","type":"INTERNAL","uiRouterKey":"apply-for-startup-program","slug":"solutions-startups-apply","external":false,"related":{"id":82,"title":"Startup program","path":"/startup-program/","scheduledAt":null,"createdAt":"2022-04-27T19:14:18.251Z","updatedAt":"2025-02-18T10:31:31.568Z","publishedAt":"2022-05-11T15:19:00.591Z","locale":"en","__contentType":"api::page.page","navigationItemId":873,"__templateName":"Generic"},"items":[],"description":""},{"id":871,"title":"Founders Program","menuAttached":false,"order":2,"path":"/Solutions/Startups/Founders","type":"INTERNAL","uiRouterKey":"founders-program","slug":"solutions-startups-founders","external":false,"related":{"id":805,"title":"Founders Program","path":"/startup-program/founders-program/","scheduledAt":null,"createdAt":"2023-04-26T15:15:16.052Z","updatedAt":"2025-02-18T10:31:32.123Z","publishedAt":"2023-04-26T15:30:48.551Z","locale":"en","__contentType":"api::page.page","navigationItemId":871,"__templateName":"Generic"},"items":[],"description":""},{"id":874,"title":"Early Stage Program","menuAttached":false,"order":3,"path":"/Solutions/Startups/Early","type":"INTERNAL","uiRouterKey":"early-stage-program","slug":"solutions-startups-early","external":false,"related":{"id":806,"title":"Early Stage Program","path":"/startup-program/early-stage-program/","scheduledAt":null,"createdAt":"2023-04-26T15:38:44.183Z","updatedAt":"2025-02-18T10:31:32.162Z","publishedAt":"2023-04-26T15:41:51.729Z","locale":"en","__contentType":"api::page.page","navigationItemId":874,"__templateName":"Generic"},"items":[],"description":""},{"id":872,"title":"Growth Stage","menuAttached":false,"order":4,"path":"/Solutions/Startups/Growth","type":"INTERNAL","uiRouterKey":"growth-stage","slug":"solutions-startups-growth","external":false,"related":{"id":807,"title":"Growth Stage Program","path":"/startup-program/growth-stage-program/","scheduledAt":null,"createdAt":"2023-04-26T15:50:16.870Z","updatedAt":"2025-02-18T10:31:32.172Z","publishedAt":"2023-04-26T15:52:22.068Z","locale":"en","__contentType":"api::page.page","navigationItemId":872,"__templateName":"Generic"},"items":[],"description":""}],"description":""}],"description":""},{"id":744,"title":"Resources","menuAttached":false,"order":9,"path":"/Resources","type":"WRAPPER","uiRouterKey":"resources-2","slug":"resources-3","external":false,"items":[{"id":746,"title":"Ecosystem","menuAttached":false,"order":1,"path":"/Resources/Ecosystem","type":"WRAPPER","uiRouterKey":"ecosystem","slug":"resources-ecosystem","external":false,"items":[{"id":751,"title":"All products","menuAttached":false,"order":1,"path":"/Resources/Ecosystem/All_products","type":"INTERNAL","uiRouterKey":"all-products-2","slug":"resources-ecosystem-all-products","external":false,"related":{"id":223,"title":"All Products","path":"/all-products/","scheduledAt":null,"createdAt":"2022-05-09T13:56:36.517Z","updatedAt":"2025-01-27T10:23:16.899Z","publishedAt":"2022-05-09T14:37:46.378Z","locale":"en","__contentType":"api::page.page","navigationItemId":751,"__templateName":"Generic"},"items":[],"description":""},{"id":828,"title":"Product updates","menuAttached":false,"order":2,"path":"/Resources/Ecosystem/Productupdates","type":"INTERNAL","uiRouterKey":"product-updates","slug":"resources-ecosystem-productupdates","external":false,"related":{"id":1451,"title":"Product updates","path":"/product-updates/","scheduledAt":null,"createdAt":"2024-10-28T16:25:15.626Z","updatedAt":"2025-01-07T09:57:23.124Z","publishedAt":"2024-10-30T16:21:39.156Z","locale":"en","__contentType":"api::page.page","navigationItemId":828,"__templateName":"Generic"},"items":[],"description":""},{"id":750,"title":"Betas","menuAttached":false,"order":3,"path":"/Resources/Ecosystem/betas","type":"INTERNAL","uiRouterKey":"betas","slug":"resources-ecosystem-betas","external":false,"related":{"id":90,"title":"Betas","path":"/betas/","scheduledAt":null,"createdAt":"2022-04-28T14:06:08.789Z","updatedAt":"2025-02-05T15:06:36.492Z","publishedAt":"2022-04-28T14:39:18.717Z","locale":"en","__contentType":"api::page.page","navigationItemId":750,"__templateName":"Generic"},"items":[],"description":""},{"id":747,"title":"Changelog","menuAttached":false,"order":4,"path":"https://www.scaleway.com/en/docs/changelog/","type":"EXTERNAL","uiRouterKey":"changelog-2","slug":{},"external":true,"description":""},{"id":758,"title":"Blog","menuAttached":false,"order":5,"path":"https://www.scaleway.com/en/blog/","type":"EXTERNAL","uiRouterKey":"blog-2","slug":{},"external":true,"description":""}],"description":""},{"id":745,"title":"Community","menuAttached":false,"order":2,"path":"/Resources/Community","type":"WRAPPER","uiRouterKey":"community","slug":"resources-community","external":false,"items":[{"id":748,"title":"Slack Community","menuAttached":false,"order":1,"path":"https://slack.scaleway.com/","type":"EXTERNAL","uiRouterKey":"slack-community-2","slug":{},"external":true,"description":""},{"id":749,"title":"Feature Requests","menuAttached":false,"order":2,"path":"https://feature-request.scaleway.com/","type":"EXTERNAL","uiRouterKey":"feature-requests-2","slug":{},"external":true,"description":""},{"id":757,"title":"Scaleway Learning","menuAttached":false,"order":3,"path":"/Resources/Community/Scaleway_Learning","type":"INTERNAL","uiRouterKey":"scaleway-learning-2","slug":"resources-community-scaleway-learning","external":false,"related":{"id":597,"title":"Scaleway Learning","path":"/scaleway-learning/","scheduledAt":null,"createdAt":"2022-12-20T08:57:37.886Z","updatedAt":"2024-12-11T09:57:09.345Z","publishedAt":"2023-01-02T21:14:10.049Z","locale":"en","__contentType":"api::page.page","navigationItemId":757,"__templateName":"Generic"},"items":[],"description":""}],"description":""},{"id":752,"title":"Company","menuAttached":false,"order":3,"path":"/Resources/Company","type":"WRAPPER","uiRouterKey":"company-1","slug":"resources-company","external":false,"items":[{"id":756,"title":"Events","menuAttached":false,"order":1,"path":"/Resources/Company/Events","type":"INTERNAL","uiRouterKey":"events-1","slug":"resources-company-events","external":false,"related":{"id":699,"title":"Events","path":"/events/","scheduledAt":null,"createdAt":"2023-03-13T09:14:30.830Z","updatedAt":"2025-02-17T10:12:28.627Z","publishedAt":"2023-03-13T09:14:41.552Z","locale":"en","__contentType":"api::page.page","navigationItemId":756,"__templateName":"Generic"},"items":[],"description":""},{"id":796,"title":"Marketplace","menuAttached":false,"order":2,"path":"https://www.scaleway.com/en/marketplace/","type":"EXTERNAL","uiRouterKey":"marketplace","slug":{},"external":true,"description":""},{"id":755,"title":"Careers","menuAttached":false,"order":3,"path":"/Resources/Company/Careers","type":"INTERNAL","uiRouterKey":"careers-1","slug":"resources-company-careers","external":false,"related":{"id":766,"title":"Careers","path":"/careers/","scheduledAt":null,"createdAt":"2023-03-31T14:17:38.589Z","updatedAt":"2024-07-16T10:08:23.648Z","publishedAt":"2024-02-12T15:39:28.684Z","locale":"en","__contentType":"api::page.page","navigationItemId":755,"__templateName":"Generic"},"items":[],"description":""},{"id":753,"title":"About us","menuAttached":false,"order":4,"path":"/Resources/Company/Aboutus","type":"INTERNAL","uiRouterKey":"about-us-1","slug":"resources-company-aboutus","external":false,"related":{"id":195,"title":"About us","path":"/about-us/","scheduledAt":null,"createdAt":"2022-05-03T13:05:13.546Z","updatedAt":"2023-12-14T09:00:58.075Z","publishedAt":"2022-05-11T12:26:40.217Z","locale":"en","__contentType":"api::page.page","navigationItemId":753,"__templateName":"Generic"},"items":[],"description":""},{"id":754,"title":"Customer Testimonials","menuAttached":false,"order":5,"path":"/Resources/Company/customer-testimonials","type":"INTERNAL","uiRouterKey":"customer-testimonials","slug":"resources-company-customer-testimonials","external":false,"related":{"id":294,"title":"Customer testimonials","path":"/customer-testimonials/","scheduledAt":null,"createdAt":"2022-05-19T15:33:42.418Z","updatedAt":"2024-07-08T12:41:04.663Z","publishedAt":"2022-05-19T15:37:23.202Z","locale":"en","__contentType":"api::page.page","navigationItemId":754,"__templateName":"Generic"},"items":[],"description":""}],"description":""},{"id":860,"title":"Partnership","menuAttached":false,"order":4,"path":"/Resources/Partnership","type":"WRAPPER","uiRouterKey":"partnership","slug":"resources-partnership","external":false,"items":[{"id":861,"title":"Partners Program","menuAttached":false,"order":1,"path":"/Resources/Partnership/PartnersProgram","type":"INTERNAL","uiRouterKey":"partners-program","slug":"resources-partnership-partners-program","external":false,"related":{"id":1350,"title":"Partners Program","path":"/partners-program/","scheduledAt":null,"createdAt":"2024-07-16T15:02:57.413Z","updatedAt":"2024-12-03T15:57:20.933Z","publishedAt":"2024-09-23T10:47:55.235Z","locale":"en","__contentType":"api::page.page","navigationItemId":861,"__templateName":"Generic"},"items":[],"description":""},{"id":862,"title":"Find your partner","menuAttached":false,"order":2,"path":"/Resources/Partnership/Find","type":"INTERNAL","uiRouterKey":"find-your-partner","slug":"resources-partnership-find","external":false,"related":{"id":1490,"title":"Find partner","path":"/find-partner/","scheduledAt":null,"createdAt":"2024-11-26T13:32:45.578Z","updatedAt":"2025-01-13T10:32:23.025Z","publishedAt":"2024-12-01T16:19:11.068Z","locale":"en","__contentType":"api::page.page","navigationItemId":862,"__templateName":"Generic"},"items":[],"description":""},{"id":863,"title":"Become a Partner","menuAttached":false,"order":3,"path":"/Resources/Partnership/become","type":"INTERNAL","uiRouterKey":"become-a-partner-1","slug":"resources-partnership-become","external":false,"related":{"id":1495,"title":"Partner Application","path":"/partner-application/","scheduledAt":null,"createdAt":"2024-11-27T13:07:23.267Z","updatedAt":"2025-02-18T16:14:09.502Z","publishedAt":"2024-11-27T13:07:24.432Z","locale":"en","__contentType":"api::page.page","navigationItemId":863,"__templateName":"Generic"},"items":[],"description":""}],"description":""}],"description":""},{"id":598,"title":"Pricing","menuAttached":false,"order":10,"path":"/pricing","type":"INTERNAL","uiRouterKey":"pricing-2","slug":"pricing-1","external":false,"related":{"id":1236,"title":"Pricing","path":"/pricing/","scheduledAt":null,"createdAt":"2024-05-14T07:33:54.370Z","updatedAt":"2025-01-24T08:42:07.875Z","publishedAt":"2024-05-14T13:19:03.795Z","locale":"en","__contentType":"api::page.page","navigationItemId":598,"__templateName":"Generic"},"items":[],"description":""}],"topBarNavigationItems":[{"id":425,"title":"Docs","menuAttached":false,"order":1,"path":"https://www.scaleway.com/en/docs/","type":"EXTERNAL","uiRouterKey":"docs","slug":{},"external":true},{"id":427,"title":"Contact","menuAttached":false,"order":3,"path":"https://www.scaleway.com/en/contact/","type":"EXTERNAL","uiRouterKey":"contact-2","slug":{},"external":true,"description":""}],"MOTD":{"id":7803,"label":"Deepseek R1 Distilled Llama 70B is now available!","url":"https://console.scaleway.com/generative-api/models","page":{"data":null}},"ctaList":{"dediboxCTAList":[{"id":6611,"label":"Log in","url":"https://console.online.net/en/login","page":{"data":null}},{"id":6612,"label":"Sign up","url":"https://console.online.net/en/user/subscribe","page":{"data":null}}],"defaultCTAList":[{"id":6610,"label":"Log in","url":"https://console.scaleway.com/login","page":{"data":null}},{"id":6609,"label":"Sign up","url":"https://console.scaleway.com/register","page":{"data":null}}]}},"footer":[{"id":276,"title":"Products","menuAttached":false,"order":1,"path":"/products","type":"WRAPPER","uiRouterKey":"products","slug":"products-2","external":false,"items":[{"id":283,"title":"All Products","menuAttached":false,"order":1,"path":"/products/AllProducts","type":"INTERNAL","uiRouterKey":"all-products","slug":"products-all-products","external":false,"related":{"id":223,"title":"All Products","path":"/all-products/","scheduledAt":null,"createdAt":"2022-05-09T13:56:36.517Z","updatedAt":"2025-01-27T10:23:16.899Z","publishedAt":"2022-05-09T14:37:46.378Z","locale":"en","__contentType":"api::page.page","navigationItemId":283,"__templateName":"Generic"},"items":[],"description":""},{"id":759,"title":"Betas","menuAttached":false,"order":2,"path":"/products/betas","type":"INTERNAL","uiRouterKey":"betas-1","slug":"products-betas","external":false,"related":{"id":90,"title":"Betas","path":"/betas/","scheduledAt":null,"createdAt":"2022-04-28T14:06:08.789Z","updatedAt":"2025-02-05T15:06:36.492Z","publishedAt":"2022-04-28T14:39:18.717Z","locale":"en","__contentType":"api::page.page","navigationItemId":759,"__templateName":"Generic"},"items":[],"description":""},{"id":281,"title":"Bare Metal","menuAttached":false,"order":3,"path":"/products/BareMetal","type":"INTERNAL","uiRouterKey":"bare-metal-2","slug":"products-bare-metal","external":false,"related":{"id":961,"title":"Bare Metal","path":"/bare-metal/","scheduledAt":null,"createdAt":"2023-09-27T07:45:06.975Z","updatedAt":"2025-01-24T08:21:16.687Z","publishedAt":"2023-10-17T12:08:02.344Z","locale":"en","__contentType":"api::page.page","navigationItemId":281,"__templateName":"Generic"},"items":[],"description":""},{"id":284,"title":"Dedibox","menuAttached":false,"order":4,"path":"/products/Dedibox","type":"INTERNAL","uiRouterKey":"dedibox-4","slug":"products-dedibox","external":false,"related":{"id":29,"title":"Dedibox","path":"/dedibox/","scheduledAt":null,"createdAt":"2022-04-19T15:29:02.488Z","updatedAt":"2024-12-02T21:42:14.962Z","publishedAt":"2022-04-28T17:05:07.122Z","locale":"en","__contentType":"api::page.page","navigationItemId":284,"__templateName":"Generic"},"items":[],"description":""},{"id":282,"title":"Elastic Metal","menuAttached":false,"order":5,"path":"/products/ElasticMetal","type":"INTERNAL","uiRouterKey":"elastic-metal-4","slug":"products-elastic-metal","external":false,"related":{"id":87,"title":"Elastic Metal","path":"/elastic-metal/","scheduledAt":null,"createdAt":"2022-04-28T12:45:28.696Z","updatedAt":"2025-01-24T13:35:03.496Z","publishedAt":"2022-04-28T13:22:46.501Z","locale":"en","__contentType":"api::page.page","navigationItemId":282,"__templateName":"Generic"},"items":[],"description":""},{"id":285,"title":"Compute Instances","menuAttached":false,"order":6,"path":"/products/Compute","type":"INTERNAL","uiRouterKey":"compute-instances","slug":"products-compute","external":false,"related":{"id":655,"title":"Virtual Instances","path":"/virtual-instances/","scheduledAt":null,"createdAt":"2023-02-20T10:48:52.279Z","updatedAt":"2025-02-11T13:16:39.501Z","publishedAt":"2023-02-28T08:32:03.960Z","locale":"en","__contentType":"api::page.page","navigationItemId":285,"__templateName":"Generic"},"items":[],"description":""},{"id":286,"title":"GPU","menuAttached":false,"order":7,"path":"/products/GPu","type":"INTERNAL","uiRouterKey":"gpu-6","slug":"products-g-pu","external":false,"related":{"id":1025,"title":"GPU Instances","path":"/gpu-instances/","scheduledAt":null,"createdAt":"2023-11-30T13:15:51.769Z","updatedAt":"2024-11-19T16:38:15.121Z","publishedAt":"2023-12-12T12:52:20.083Z","locale":"en","__contentType":"api::page.page","navigationItemId":286,"__templateName":"Generic"},"items":[],"description":""},{"id":287,"title":"Containers","menuAttached":false,"order":8,"path":"/products/Containers","type":"INTERNAL","uiRouterKey":"containers-6","slug":"products-containers","external":false,"related":{"id":465,"title":"Containers","path":"/containers/","scheduledAt":null,"createdAt":"2022-07-29T15:09:20.535Z","updatedAt":"2024-08-28T07:05:23.005Z","publishedAt":"2023-02-27T13:53:48.270Z","locale":"en","__contentType":"api::page.page","navigationItemId":287,"__templateName":"Generic"},"items":[],"description":""},{"id":288,"title":"Object Storage","menuAttached":false,"order":9,"path":"/products/ObjectStorage","type":"INTERNAL","uiRouterKey":"object-storage-4","slug":"products-object-storage","external":false,"related":{"id":652,"title":"Object Storage","path":"/object-storage/","scheduledAt":null,"createdAt":"2023-02-16T09:44:56.414Z","updatedAt":"2024-12-02T14:09:58.690Z","publishedAt":"2023-03-07T18:05:15.061Z","locale":"en","__contentType":"api::page.page","navigationItemId":288,"__templateName":"Generic"},"items":[],"description":""},{"id":289,"title":"Block Storage","menuAttached":false,"order":10,"path":"/products/BlockStorage","type":"INTERNAL","uiRouterKey":"block-storage-4","slug":"products-block-storage","external":false,"related":{"id":141,"title":"Block Storage","path":"/block-storage/","scheduledAt":null,"createdAt":"2022-05-02T08:20:39.280Z","updatedAt":"2025-02-05T14:21:18.667Z","publishedAt":"2022-05-02T08:28:12.783Z","locale":"en","__contentType":"api::page.page","navigationItemId":289,"__templateName":"Generic"},"items":[],"description":""}],"description":""},{"id":275,"title":"Resources","menuAttached":false,"order":2,"path":"/resources","type":"WRAPPER","uiRouterKey":"resources","slug":"resources-3","external":false,"items":[{"id":290,"title":"Documentation","menuAttached":false,"order":1,"path":"https://www.scaleway.com/en/docs/","type":"EXTERNAL","uiRouterKey":"documentation","slug":{},"external":true,"description":""},{"id":292,"title":"Changelog","menuAttached":false,"order":2,"path":"https://www.scaleway.com/en/docs/changelog/","type":"EXTERNAL","uiRouterKey":"changelog","slug":{},"external":true,"description":""},{"id":291,"title":"Blog","menuAttached":false,"order":3,"path":"https://www.scaleway.com/en/blog/","type":"EXTERNAL","uiRouterKey":"blog","slug":{},"external":true,"description":""},{"id":293,"title":"Feature Requests","menuAttached":false,"order":4,"path":"https://feature-request.scaleway.com/","type":"EXTERNAL","uiRouterKey":"feature-requests","slug":{},"external":true,"description":""},{"id":321,"title":"Slack Community","menuAttached":false,"order":5,"path":"https://slack.scaleway.com/","type":"EXTERNAL","uiRouterKey":"slack-community-2","slug":{},"external":true,"description":""}],"description":""},{"id":280,"title":"Contact","menuAttached":false,"order":3,"path":"/Contact","type":"WRAPPER","uiRouterKey":"contact-2","slug":"contact-4","external":false,"items":[{"id":294,"title":"Create a ticket","menuAttached":false,"order":1,"path":"https://console.scaleway.com/support/create/","type":"EXTERNAL","uiRouterKey":"create-a-ticket","slug":{},"external":true,"description":""},{"id":296,"title":"Report Abuse","menuAttached":false,"order":2,"path":"https://console.scaleway.com/support/abuses/create/","type":"EXTERNAL","uiRouterKey":"report-abuse","slug":{},"external":true,"description":""},{"id":295,"title":"Status","menuAttached":false,"order":3,"path":"https://status.scaleway.com/","type":"EXTERNAL","uiRouterKey":"status","slug":{},"external":true,"description":""},{"id":298,"title":"Dedibox Console online.net","menuAttached":false,"order":4,"path":"https://console.online.net/fr/login","type":"EXTERNAL","uiRouterKey":"dedibox-console-online-net","slug":{},"external":true,"description":""},{"id":407,"title":"Support plans","menuAttached":false,"order":5,"path":"/Contact/Support","type":"INTERNAL","uiRouterKey":"support-plans","slug":"contact-support","external":false,"related":{"id":493,"title":"Assistance","path":"/assistance/","scheduledAt":null,"createdAt":"2022-09-26T15:14:28.440Z","updatedAt":"2024-08-28T07:19:37.841Z","publishedAt":"2022-10-03T12:20:34.441Z","locale":"en","__contentType":"api::page.page","navigationItemId":407,"__templateName":"Generic"},"items":[],"description":""},{"id":409,"title":"Brand resources","menuAttached":false,"order":6,"path":"https://ultraviolet.scaleway.com/6dd9b5c45/p/62b4e2-ultraviolet","type":"EXTERNAL","uiRouterKey":"brand-resources","slug":{},"external":true,"description":""}],"description":""},{"id":436,"title":"Company","menuAttached":false,"order":4,"path":"/scw","type":"WRAPPER","uiRouterKey":"company","slug":"scw","external":false,"items":[{"id":440,"title":"About us","menuAttached":false,"order":1,"path":"/scw/About-us","type":"INTERNAL","uiRouterKey":"about-us","slug":"scw-about-us","external":false,"related":{"id":195,"title":"About us","path":"/about-us/","scheduledAt":null,"createdAt":"2022-05-03T13:05:13.546Z","updatedAt":"2023-12-14T09:00:58.075Z","publishedAt":"2022-05-11T12:26:40.217Z","locale":"en","__contentType":"api::page.page","navigationItemId":440,"__templateName":"Generic"},"items":[],"description":""},{"id":441,"title":"Events","menuAttached":false,"order":2,"path":"/scw/events","type":"INTERNAL","uiRouterKey":"events","slug":"scw-events","external":false,"related":{"id":699,"title":"Events","path":"/events/","scheduledAt":null,"createdAt":"2023-03-13T09:14:30.830Z","updatedAt":"2025-02-17T10:12:28.627Z","publishedAt":"2023-03-13T09:14:41.552Z","locale":"en","__contentType":"api::page.page","navigationItemId":441,"__templateName":"Generic"},"items":[],"description":""},{"id":798,"title":"Marketplace","menuAttached":false,"order":3,"path":"https://www.scaleway.com/en/marketplace/","type":"EXTERNAL","uiRouterKey":"marketplace-2","slug":{},"external":true,"description":""},{"id":439,"title":"Environment ","menuAttached":false,"order":4,"path":"/scw/environment","type":"INTERNAL","uiRouterKey":"environment","slug":"scw-environment","external":false,"related":{"id":59,"title":"Environmental leadership ","path":"/environmental-leadership/","scheduledAt":null,"createdAt":"2022-04-26T08:30:15.289Z","updatedAt":"2025-02-04T15:14:39.010Z","publishedAt":"2022-04-28T17:12:24.574Z","locale":"en","__contentType":"api::page.page","navigationItemId":439,"__templateName":"Generic"},"items":[],"description":""},{"id":790,"title":"Social Responsibility","menuAttached":false,"order":5,"path":"/scw/SocialResponsibility","type":"INTERNAL","uiRouterKey":"social-responsibility","slug":"scw-social-responsibility","external":false,"related":{"id":184,"title":"Social responsibility","path":"/social-responsibility/","scheduledAt":null,"createdAt":"2022-05-03T07:48:38.038Z","updatedAt":"2024-08-28T07:08:11.382Z","publishedAt":"2022-05-03T13:08:48.890Z","locale":"en","__contentType":"api::page.page","navigationItemId":790,"__templateName":"Generic"},"items":[],"description":""},{"id":438,"title":"Security","menuAttached":false,"order":6,"path":"/scw/security","type":"INTERNAL","uiRouterKey":"security-4","slug":"scw-security","external":false,"related":{"id":190,"title":"Security and resilience","path":"/security-and-resilience/","scheduledAt":null,"createdAt":"2022-05-03T10:22:40.696Z","updatedAt":"2024-08-28T08:56:56.744Z","publishedAt":"2022-05-11T12:39:01.810Z","locale":"en","__contentType":"api::page.page","navigationItemId":438,"__templateName":"Generic"},"items":[],"description":""},{"id":782,"title":"Shared Responsibility Model","menuAttached":false,"order":7,"path":"/scw/Model","type":"INTERNAL","uiRouterKey":"shared-responsibility-model","slug":"scw-model","external":false,"related":{"id":1180,"title":"Shared Responsibility Model","path":"/shared-responsibility-model/","scheduledAt":null,"createdAt":"2024-04-04T15:54:36.614Z","updatedAt":"2024-11-18T13:28:57.006Z","publishedAt":"2024-04-04T15:56:39.573Z","locale":"en","__contentType":"api::page.page","navigationItemId":782,"__templateName":"Generic"},"items":[],"description":""},{"id":442,"title":"News","menuAttached":false,"order":8,"path":"/scw/news","type":"INTERNAL","uiRouterKey":"news","slug":"scw-news","external":false,"related":{"id":263,"title":"News","path":"/news/","scheduledAt":null,"createdAt":"2022-05-19T10:28:45.212Z","updatedAt":"2022-05-31T07:47:17.728Z","publishedAt":"2022-05-19T10:29:13.394Z","locale":"en","__contentType":"api::page.page","navigationItemId":442,"__templateName":"Generic"},"items":[],"description":""},{"id":443,"title":"Careers","menuAttached":false,"order":9,"path":"/scw/career/","type":"INTERNAL","uiRouterKey":"careers","slug":"scw-career","external":false,"related":{"id":766,"title":"Careers","path":"/careers/","scheduledAt":null,"createdAt":"2023-03-31T14:17:38.589Z","updatedAt":"2024-07-16T10:08:23.648Z","publishedAt":"2024-02-12T15:39:28.684Z","locale":"en","__contentType":"api::page.page","navigationItemId":443,"__templateName":"Generic"},"items":[],"description":""},{"id":445,"title":"Scaleway Learning","menuAttached":false,"order":10,"path":"/scw/learning","type":"INTERNAL","uiRouterKey":"scaleway-learning","slug":"scw-learning","external":false,"related":{"id":597,"title":"Scaleway Learning","path":"/scaleway-learning/","scheduledAt":null,"createdAt":"2022-12-20T08:57:37.886Z","updatedAt":"2024-12-11T09:57:09.345Z","publishedAt":"2023-01-02T21:14:10.049Z","locale":"en","__contentType":"api::page.page","navigationItemId":445,"__templateName":"Generic"},"items":[],"description":""},{"id":444,"title":"Client Success Stories","menuAttached":false,"order":11,"path":"/scw/clientstor/","type":"INTERNAL","uiRouterKey":"client-success-stories","slug":"scw-clientstor","external":false,"related":{"id":294,"title":"Customer testimonials","path":"/customer-testimonials/","scheduledAt":null,"createdAt":"2022-05-19T15:33:42.418Z","updatedAt":"2024-07-08T12:41:04.663Z","publishedAt":"2022-05-19T15:37:23.202Z","locale":"en","__contentType":"api::page.page","navigationItemId":444,"__templateName":"Generic"},"items":[],"description":""},{"id":437,"title":"Labs","menuAttached":false,"order":12,"path":"https://labs.scaleway.com/en/","type":"EXTERNAL","uiRouterKey":"labs","slug":{},"external":true,"description":""}],"description":""}],"pageType":"post","isDraftMode":false},"__N_SSG":true},"page":"/blog/[slug]","query":{"slug":"get-started-ai-cost-emissions-mindmatch"},"buildId":"4xZKwUKlhtIRe3nXE5xXw","isFallback":false,"gsp":true,"locale":"en","locales":["default","en","fr"],"defaultLocale":"default","scriptLoader":[]}</script></body></html>