CINXE.COM
Transformers without Normalization | alphaXiv
<!DOCTYPE html><html lang="en" data-sentry-component="RootLayout" data-sentry-source-file="layout.tsx"><head><meta charSet="utf-8"/><meta name="viewport" content="width=device-width, initial-scale=1, viewport-fit=cover"/><link rel="stylesheet" href="/_next/static/css/a6f6df2afb96a7a2.css" data-precedence="next"/><link rel="stylesheet" href="/_next/static/css/1baa833b56016a20.css" data-precedence="next"/><link rel="stylesheet" href="/_next/static/css/b57b729bdae0dee2.css" data-precedence="next"/><link rel="stylesheet" href="/_next/static/css/acdaad1d23646914.css" data-precedence="next"/><link rel="stylesheet" href="/_next/static/css/a7815692be819096.css" data-precedence="next"/><link rel="preload" as="script" fetchPriority="low" href="/_next/static/chunks/webpack-a7b03224847ab321.js"/><script src="/_next/static/chunks/24480ae8-f7eadf6356abbabd.js" async=""></script><script src="/_next/static/chunks/04193fb2-6310b42f4fefcea1.js" async=""></script><script src="/_next/static/chunks/3385-30a6dde6d98bcdf4.js" async=""></script><script src="/_next/static/chunks/main-app-26a5b9526d3a8f0d.js" async=""></script><script src="/_next/static/chunks/1da0d171-1f9041fa20b0f780.js" async=""></script><script src="/_next/static/chunks/6117-41689ef6ff9b033c.js" async=""></script><script src="/_next/static/chunks/1350-a1024eb8f8a6859e.js" async=""></script><script src="/_next/static/chunks/1447-9124dd5e537fe7a8.js" async=""></script><script src="/_next/static/chunks/666-78ffa3c0d0a5a2a3.js" async=""></script><script src="/_next/static/chunks/5872-dd1cde135170beb6.js" async=""></script><script src="/_next/static/chunks/7362-50e5d1ac2abc44a0.js" async=""></script><script src="/_next/static/chunks/2749-95477708edcb2a1e.js" async=""></script><script src="/_next/static/chunks/1615-1f6676350ab288e8.js" async=""></script><script src="/_next/static/chunks/4964-2cba8177dbece8d4.js" async=""></script><script src="/_next/static/chunks/app/layout-a39c2199527f5444.js" async=""></script><script src="/_next/static/chunks/app/global-error-57044b847d6c9be6.js" async=""></script><script src="/_next/static/chunks/8951-bcdd0e91584e856e.js" async=""></script><script src="/_next/static/chunks/932-76dfd6658f5c91af.js" async=""></script><script src="/_next/static/chunks/4765-88aa2d5b19cb25bc.js" async=""></script><script src="/_next/static/chunks/9832-86d2bf8ed3fa1d9e.js" async=""></script><script src="/_next/static/chunks/8545-1bc4c81889a95ea0.js" async=""></script><script src="/_next/static/chunks/7446-1a273b84bf468616.js" async=""></script><script src="/_next/static/chunks/app/(paper)/%5Bid%5D/abs/page-17f212a359917e65.js" async=""></script><script src="https://accounts.google.com/gsi/client" async="" defer=""></script><script src="/_next/static/chunks/62420ecc-ba068cf8c61f9a07.js" async=""></script><script src="/_next/static/chunks/9d987bc4-d447aa4b86ffa8da.js" async=""></script><script src="/_next/static/chunks/c386c4a4-4ae2baf83c93de20.js" async=""></script><script src="/_next/static/chunks/7299-64abce2685056cd4.js" async=""></script><script src="/_next/static/chunks/2068-7fbc56857b0cc3b1.js" async=""></script><script src="/_next/static/chunks/2755-54255117838ce4e4.js" async=""></script><script src="/_next/static/chunks/4505-4fe5d5f302c56050.js" async=""></script><script src="/_next/static/chunks/8273-4cb3558ea58359d7.js" async=""></script><script src="/_next/static/chunks/6681-13aed21c8bb47aa3.js" async=""></script><script src="/_next/static/chunks/4005-6fe3c26cb25644be.js" async=""></script><script src="/_next/static/chunks/4785-5dbc1af26cd46ec5.js" async=""></script><script src="/_next/static/chunks/6335-5d291246680ceb4d.js" async=""></script><script src="/_next/static/chunks/2642-b497e0f313459fb9.js" async=""></script><script src="/_next/static/chunks/5145-f10798defa0dde88.js" async=""></script><script src="/_next/static/chunks/8114-2172b7ef97f83184.js" async=""></script><script src="/_next/static/chunks/9392-5fab98d8656406c4.js" async=""></script><script src="/_next/static/chunks/app/(paper)/%5Bid%5D/layout-3e11c64ff66d737f.js" async=""></script><script src="/_next/static/chunks/app/error-a92d22105c18293c.js" async=""></script><link rel="preload" href="https://www.googletagmanager.com/gtag/js?id=G-94SEL844DQ" as="script"/><meta name="next-size-adjust" content=""/><link rel="preconnect" href="https://fonts.googleapis.com"/><link rel="preconnect" href="https://fonts.gstatic.com" crossorigin="anonymous"/><link rel="apple-touch-icon" sizes="1024x1024" href="/assets/pwa/alphaxiv_app_1024.png"/><meta name="theme-color" content="#FFFFFF" data-sentry-element="meta" data-sentry-source-file="layout.tsx"/><title>Transformers without Normalization | alphaXiv</title><meta name="description" content="View 2 comments: Should be α"/><link rel="manifest" href="/manifest.webmanifest"/><meta name="keywords" content="alphaxiv, arxiv, forum, discussion, explore, trending papers"/><meta name="robots" content="index, follow"/><meta name="googlebot" content="index, follow"/><link rel="canonical" href="https://www.alphaxiv.org/abs/2503.10622"/><meta property="og:title" content="Transformers without Normalization | alphaXiv"/><meta property="og:description" content="View 2 comments: Should be α"/><meta property="og:url" content="https://www.alphaxiv.org/abs/2503.10622"/><meta property="og:site_name" content="alphaXiv"/><meta property="og:locale" content="en_US"/><meta property="og:image" content="https://paper-assets.alphaxiv.org/image/2503.10622v1.png"/><meta property="og:image:width" content="816"/><meta property="og:image:height" content="1056"/><meta property="og:type" content="website"/><meta name="twitter:card" content="summary_large_image"/><meta name="twitter:creator" content="@askalphaxiv"/><meta name="twitter:title" content="Transformers without Normalization | alphaXiv"/><meta name="twitter:description" content="View 2 comments: Should be α"/><meta name="twitter:image" content="https://www.alphaxiv.org/nextapi/og?paperTitle=Transformers+without+Normalization&authors=Kaiming+He%2C+Yann+LeCun%2C+Xinlei+Chen%2C+Zhuang+Liu%2C+Jiachen+Zhu"/><meta name="twitter:image:alt" content="Transformers without Normalization | alphaXiv"/><link rel="icon" href="/icon.ico?ba7039e153811708" type="image/x-icon" sizes="16x16"/><link href="https://fonts.googleapis.com/css2?family=Inter:wght@100..900&family=Onest:wght@100..900&family=Rubik:ital,wght@0,300..900;1,300..900&display=swap" rel="stylesheet"/><meta name="sentry-trace" content="2fbbd50b96373eedcf1ceeedbaadbbc7-81b232d40460ce6d-1"/><meta name="baggage" content="sentry-environment=prod,sentry-release=1f008bf4b59f8db479fb4e06a55a89e4bc6d748d,sentry-public_key=85030943fbd87a51036e3979c1f6c797,sentry-trace_id=2fbbd50b96373eedcf1ceeedbaadbbc7,sentry-sample_rate=1,sentry-transaction=GET%20%2F%5Bid%5D%2Fabs,sentry-sampled=true"/><script src="/_next/static/chunks/polyfills-42372ed130431b0a.js" noModule=""></script></head><body class="h-screen overflow-hidden"><!--$--><!--/$--><div id="root"><section aria-label="Notifications alt+T" tabindex="-1" aria-live="polite" aria-relevant="additions text" aria-atomic="false"></section><script data-alphaxiv-id="json-ld-paper-detail-view" type="application/ld+json">{"@context":"https://schema.org","@type":"ScholarlyArticle","headline":"Transformers without Normalization","abstract":"Normalization layers are ubiquitous in modern neural networks and have long\nbeen considered essential. This work demonstrates that Transformers without\nnormalization can achieve the same or better performance using a remarkably\nsimple technique. We introduce Dynamic Tanh (DyT), an element-wise operation\n$DyT($x$) = \\tanh(\\alpha $x$)$, as a drop-in replacement for normalization\nlayers in Transformers. DyT is inspired by the observation that layer\nnormalization in Transformers often produces tanh-like, $S$-shaped input-output\nmappings. By incorporating DyT, Transformers without normalization can match or\nexceed the performance of their normalized counterparts, mostly without\nhyperparameter tuning. We validate the effectiveness of Transformers with DyT\nacross diverse settings, ranging from recognition to generation, supervised to\nself-supervised learning, and computer vision to language models. These\nfindings challenge the conventional understanding that normalization layers are\nindispensable in modern neural networks, and offer new insights into their role\nin deep networks.","author":[{"@type":"Person","name":"Kaiming He"},{"@type":"Person","name":"Yann LeCun"},{"@type":"Person","name":"Xinlei Chen"},{"@type":"Person","name":"Zhuang Liu"},{"@type":"Person","name":"Jiachen Zhu"}],"datePublished":"2025-03-13T17:59:06.000Z","url":"https://www.alphaxiv.org/abs/67d3840793513844c2f69c11","citation":{"@type":"CreativeWork","identifier":"67d3840793513844c2f69c11"},"publisher":{"@type":"Organization","name":"arXiv"},"discussionUrl":"https://www.alphaxiv.org/abs/67d3840793513844c2f69c11","interactionStatistic":[{"@type":"InteractionCounter","interactionType":{"@type":"ViewAction","url":"https://schema.org/ViewAction"},"userInteractionCount":203215},{"@type":"InteractionCounter","interactionType":{"@type":"LikeAction","url":"https://schema.org/LikeAction"},"userInteractionCount":1580}],"commentCount":2,"comment":[{"@type":"Comment","text":"Should be α","dateCreated":"2025-03-19T13:53:39.469Z","author":{"@type":"Person","name":"Facundo Quiroga"},"upvoteCount":2},{"@type":"Comment","text":"Awesome work!Transformers without Normalization podcast","dateCreated":"2025-03-16T22:24:19.304Z","author":{"@type":"Person","name":"ZEN PUNK"},"upvoteCount":1}]}</script><div class="z-50 flex h-9 border-b border-[#ddd] bg-white px-4 dark:border-[#333333] dark:bg-[#1F1F1F] mt-0" data-sentry-component="TopNavigation" data-sentry-source-file="TopNavigation.tsx"><div class="flex h-full flex-1 items-center" data-sentry-component="LeftSection" data-sentry-source-file="TopNavigation.tsx"><div class="flex h-full items-center gap-2"><button aria-label="Open navigation sidebar" class="rounded-full p-2 hover:bg-gray-100 dark:hover:bg-gray-800"><svg xmlns="http://www.w3.org/2000/svg" width="24" height="24" viewBox="0 0 24 24" fill="none" stroke="currentColor" stroke-width="2" stroke-linecap="round" stroke-linejoin="round" class="lucide lucide-menu dark:text-gray-300"><line x1="4" x2="20" y1="12" y2="12"></line><line x1="4" x2="20" y1="6" y2="6"></line><line x1="4" x2="20" y1="18" y2="18"></line></svg></button><div class="fixed inset-y-0 left-0 z-40 flex w-64 transform flex-col border-r border-gray-200 bg-white transition-transform duration-300 ease-in-out dark:border-gray-800 dark:bg-gray-900 -translate-x-full"><div class="flex items-center border-b border-gray-200 p-4 dark:border-gray-800"><button aria-label="Close navigation sidebar" class="rounded-full p-2 hover:bg-gray-100 dark:hover:bg-gray-800"><svg xmlns="http://www.w3.org/2000/svg" width="24" height="24" viewBox="0 0 24 24" fill="none" stroke="currentColor" stroke-width="2" stroke-linecap="round" stroke-linejoin="round" class="lucide lucide-x dark:text-gray-300" data-sentry-element="X" data-sentry-source-file="HamburgerNav.tsx"><path d="M18 6 6 18"></path><path d="m6 6 12 12"></path></svg></button><a class="ml-2 flex items-center space-x-3" data-sentry-element="Link" data-sentry-source-file="HamburgerNav.tsx" href="/"><svg xmlns="http://www.w3.org/2000/svg" viewBox="0 0 718.41 504.47" width="718.41" height="504.47" class="h-8 w-8 text-customRed dark:text-white" data-sentry-element="svg" data-sentry-source-file="AlphaXivLogo.tsx" data-sentry-component="AlphaXivLogo"><polygon fill="currentColor" points="591.15 258.54 718.41 385.73 663.72 440.28 536.57 313.62 591.15 258.54" data-sentry-element="polygon" data-sentry-source-file="AlphaXivLogo.tsx"></polygon><path fill="currentColor" d="M273.86.3c34.56-2.41,67.66,9.73,92.51,33.54l94.64,94.63-55.11,54.55-96.76-96.55c-16.02-12.7-37.67-12.1-53.19,1.11L54.62,288.82,0,234.23,204.76,29.57C223.12,13.31,249.27,2.02,273.86.3Z" data-sentry-element="path" data-sentry-source-file="AlphaXivLogo.tsx"></path><path fill="currentColor" d="M663.79,1.29l54.62,54.58-418.11,417.9c-114.43,95.94-263.57-53.49-167.05-167.52l160.46-160.33,54.62,54.58-157.88,157.77c-33.17,40.32,18.93,91.41,58.66,57.48L663.79,1.29Z" data-sentry-element="path" data-sentry-source-file="AlphaXivLogo.tsx"></path></svg><span class="hidden text-customRed dark:text-white lg:block lg:text-lg">alphaXiv</span></a></div><div class="flex flex-grow flex-col space-y-2 px-4 py-8"><button class="flex items-center rounded-full px-4 py-3 text-lg transition-colors w-full text-gray-500 hover:bg-gray-100 dark:text-gray-300 dark:hover:bg-gray-800" data-sentry-component="NavButton" data-sentry-source-file="HamburgerNav.tsx"><svg xmlns="http://www.w3.org/2000/svg" width="22" height="22" viewBox="0 0 24 24" fill="none" stroke="currentColor" stroke-width="2" stroke-linecap="round" stroke-linejoin="round" class="lucide lucide-message-square mr-3"><path d="M21 15a2 2 0 0 1-2 2H7l-4 4V5a2 2 0 0 1 2-2h14a2 2 0 0 1 2 2z"></path></svg><span>Explore</span></button><button class="flex items-center rounded-full px-4 py-3 text-lg transition-colors w-full text-gray-500 hover:bg-gray-100 dark:text-gray-300 dark:hover:bg-gray-800" data-sentry-component="NavButton" data-sentry-source-file="HamburgerNav.tsx"><svg xmlns="http://www.w3.org/2000/svg" width="22" height="22" viewBox="0 0 24 24" fill="none" stroke="currentColor" stroke-width="2" stroke-linecap="round" stroke-linejoin="round" class="lucide lucide-users mr-3"><path d="M16 21v-2a4 4 0 0 0-4-4H6a4 4 0 0 0-4 4v2"></path><circle cx="9" cy="7" r="4"></circle><path d="M22 21v-2a4 4 0 0 0-3-3.87"></path><path d="M16 3.13a4 4 0 0 1 0 7.75"></path></svg><span>People</span></button><a href="https://chromewebstore.google.com/detail/alphaxiv-open-research-di/liihfcjialakefgidmaadhajjikbjjab" target="_blank" rel="noopener noreferrer" class="flex items-center rounded-full px-4 py-3 text-lg text-gray-500 transition-colors hover:bg-gray-100 dark:text-gray-300 dark:hover:bg-gray-800"><svg xmlns="http://www.w3.org/2000/svg" width="22" height="22" viewBox="0 0 24 24" fill="none" stroke="currentColor" stroke-width="2" stroke-linecap="round" stroke-linejoin="round" class="lucide lucide-chrome mr-3" data-sentry-element="unknown" data-sentry-source-file="HamburgerNav.tsx"><circle cx="12" cy="12" r="10"></circle><circle cx="12" cy="12" r="4"></circle><line x1="21.17" x2="12" y1="8" y2="8"></line><line x1="3.95" x2="8.54" y1="6.06" y2="14"></line><line x1="10.88" x2="15.46" y1="21.94" y2="14"></line></svg><span>Get extension</span><svg xmlns="http://www.w3.org/2000/svg" width="16" height="16" viewBox="0 0 24 24" fill="none" stroke="currentColor" stroke-width="2" stroke-linecap="round" stroke-linejoin="round" class="lucide lucide-external-link ml-1" data-sentry-element="ExternalLink" data-sentry-source-file="HamburgerNav.tsx"><path d="M15 3h6v6"></path><path d="M10 14 21 3"></path><path d="M18 13v6a2 2 0 0 1-2 2H5a2 2 0 0 1-2-2V8a2 2 0 0 1 2-2h6"></path></svg></a><button class="flex items-center rounded-full px-4 py-3 text-lg transition-colors w-full text-gray-500 hover:bg-gray-100 dark:text-gray-300 dark:hover:bg-gray-800" data-sentry-component="NavButton" data-sentry-source-file="HamburgerNav.tsx"><svg xmlns="http://www.w3.org/2000/svg" width="22" height="22" viewBox="0 0 24 24" fill="none" stroke="currentColor" stroke-width="2" stroke-linecap="round" stroke-linejoin="round" class="lucide lucide-log-in mr-3"><path d="M15 3h4a2 2 0 0 1 2 2v14a2 2 0 0 1-2 2h-4"></path><polyline points="10 17 15 12 10 7"></polyline><line x1="15" x2="3" y1="12" y2="12"></line></svg><span>Login</span></button></div><div class="mt-auto p-8 pt-2"><div class="flex flex-col space-y-4"><div class="mb-2 flex flex-col space-y-3 text-[15px]"><a class="text-gray-500 hover:underline dark:text-gray-400" data-sentry-element="Link" data-sentry-source-file="HamburgerNav.tsx" href="/blog">Blog</a><a target="_blank" rel="noopener noreferrer" class="inline-flex items-center text-gray-500 dark:text-gray-400" href="https://alphaxiv.io"><span class="hover:underline">Research Site</span></a><a class="text-gray-500 hover:underline dark:text-gray-400" data-sentry-element="Link" data-sentry-source-file="HamburgerNav.tsx" href="/commentguidelines">Comment Guidelines</a><a class="text-gray-500 hover:underline dark:text-gray-400" data-sentry-element="Link" data-sentry-source-file="HamburgerNav.tsx" href="/about">About Us</a></div><img alt="ArXiv Labs Logo" data-sentry-element="Image" data-sentry-source-file="HamburgerNav.tsx" loading="lazy" width="120" height="40" decoding="async" data-nimg="1" style="color:transparent;object-fit:contain" srcSet="/_next/image?url=%2Fassets%2Farxivlabs.png&w=128&q=75 1x, /_next/image?url=%2Fassets%2Farxivlabs.png&w=256&q=75 2x" src="/_next/image?url=%2Fassets%2Farxivlabs.png&w=256&q=75"/></div></div></div><a class="ml-2 flex items-center space-x-3" data-loading-trigger="true" data-sentry-element="Link" data-sentry-source-file="TopNavigation.tsx" href="/"><svg xmlns="http://www.w3.org/2000/svg" viewBox="0 0 718.41 504.47" width="718.41" height="504.47" class="h-8 w-8 text-customRed dark:text-white" data-sentry-element="svg" data-sentry-source-file="AlphaXivLogo.tsx" data-sentry-component="AlphaXivLogo"><polygon fill="currentColor" points="591.15 258.54 718.41 385.73 663.72 440.28 536.57 313.62 591.15 258.54" data-sentry-element="polygon" data-sentry-source-file="AlphaXivLogo.tsx"></polygon><path fill="currentColor" d="M273.86.3c34.56-2.41,67.66,9.73,92.51,33.54l94.64,94.63-55.11,54.55-96.76-96.55c-16.02-12.7-37.67-12.1-53.19,1.11L54.62,288.82,0,234.23,204.76,29.57C223.12,13.31,249.27,2.02,273.86.3Z" data-sentry-element="path" data-sentry-source-file="AlphaXivLogo.tsx"></path><path fill="currentColor" d="M663.79,1.29l54.62,54.58-418.11,417.9c-114.43,95.94-263.57-53.49-167.05-167.52l160.46-160.33,54.62,54.58-157.88,157.77c-33.17,40.32,18.93,91.41,58.66,57.48L663.79,1.29Z" data-sentry-element="path" data-sentry-source-file="AlphaXivLogo.tsx"></path></svg><span class="hidden text-customRed dark:text-white lg:block lg:text-lg">alphaXiv</span></a></div></div><div class="flex h-full items-center" data-sentry-component="TabsSection" data-sentry-source-file="TopNavigation.tsx"><div class="relative flex h-full"><button class="inline-flex items-center justify-center whitespace-nowrap ring-offset-white transition-all duration-200 outline-none focus-visible:outline-none disabled:pointer-events-none disabled:opacity-50 dark:ring-offset-neutral-950 hover:bg-[#9a20360a] hover:text-customRed dark:hover:bg-customRed/25 enabled:active:ring-2 enabled:active:ring-[#9a20360a] py-1.5 h-full rounded-none border-0 px-5 text-xs relative bg-white font-medium text-gray-900 dark:bg-[#2A2A2A] dark:text-white before:absolute before:inset-0 before:border-l before:border-r before:border-[#ddd] dark:before:border-[#333333] before:-z-0 after:absolute after:bottom-[-1px] after:left-0 after:right-0 after:h-[2px] after:border-l after:border-r after:border-[#ddd] after:bg-white dark:after:border-[#333333] dark:after:bg-[#2A2A2A] !py-0" data-loading-trigger="true"><span class="relative z-10 flex items-center gap-2"><svg xmlns="http://www.w3.org/2000/svg" width="24" height="24" viewBox="0 0 24 24" fill="none" stroke="currentColor" stroke-width="2" stroke-linecap="round" stroke-linejoin="round" class="lucide lucide-file-text h-4 w-4"><path d="M15 2H6a2 2 0 0 0-2 2v16a2 2 0 0 0 2 2h12a2 2 0 0 0 2-2V7Z"></path><path d="M14 2v4a2 2 0 0 0 2 2h4"></path><path d="M10 9H8"></path><path d="M16 13H8"></path><path d="M16 17H8"></path></svg>Paper</span></button><button class="inline-flex items-center justify-center whitespace-nowrap ring-offset-white transition-all duration-200 outline-none focus-visible:outline-none disabled:pointer-events-none disabled:opacity-50 dark:ring-offset-neutral-950 enabled:active:ring-2 enabled:active:ring-[#9a20360a] py-1.5 h-full rounded-none border-0 px-5 text-xs relative text-gray-500 hover:text-gray-900 dark:text-gray-400 dark:hover:text-white hover:bg-gray-100 dark:hover:bg-[#2A2A2A] !py-0" data-loading-trigger="true"><span class="relative z-10 flex items-center gap-2"><svg xmlns="http://www.w3.org/2000/svg" width="24" height="24" viewBox="0 0 24 24" fill="none" stroke="currentColor" stroke-width="2" stroke-linecap="round" stroke-linejoin="round" class="lucide lucide-book-open h-4 w-4"><path d="M2 3h6a4 4 0 0 1 4 4v14a3 3 0 0 0-3-3H2z"></path><path d="M22 3h-6a4 4 0 0 0-4 4v14a3 3 0 0 1 3-3h7z"></path></svg>Overview</span></button></div><div class="absolute bottom-0 left-0 right-0 h-[1px] bg-[#ddd] dark:bg-[#333333]"></div></div><div class="flex h-full flex-1 items-center justify-end" data-sentry-component="RightSection" data-sentry-source-file="TopNavigation.tsx"><div class="flex h-full items-center space-x-2"><div class="flex items-center space-x-2"><button class="inline-flex items-center justify-center whitespace-nowrap rounded-md text-sm ring-offset-white transition-all duration-200 outline-none focus-visible:outline-none disabled:pointer-events-none disabled:opacity-50 dark:ring-offset-neutral-950 hover:bg-[#9a20360a] hover:text-customRed dark:text-white dark:hover:bg-customRed/25 enabled:active:ring-2 enabled:active:ring-[#9a20360a] h-10 w-10 !rounded-full relative" data-state="closed"><div><svg xmlns="http://www.w3.org/2000/svg" width="24" height="24" viewBox="0 0 24 24" fill="none" stroke="currentColor" stroke-width="2" stroke-linecap="round" stroke-linejoin="round" class="lucide lucide-panel-right-close h-4 w-4"><rect width="18" height="18" x="3" y="3" rx="2"></rect><path d="M15 3v18"></path><path d="m8 9 3 3-3 3"></path></svg></div></button><button class="justify-center whitespace-nowrap text-sm ring-offset-white transition-all duration-200 outline-none focus-visible:outline-none disabled:pointer-events-none disabled:opacity-50 dark:ring-offset-neutral-950 hover:bg-[#9a20360a] hover:text-customRed dark:text-white dark:hover:bg-customRed/25 enabled:active:ring-2 enabled:active:ring-[#9a20360a] h-10 px-2 py-1.5 hidden items-center gap-2 rounded-full md:flex" disabled="" data-sentry-element="Button" data-sentry-source-file="TopNavigation.tsx"><svg xmlns="http://www.w3.org/2000/svg" width="24" height="24" viewBox="0 0 24 24" fill="none" stroke="currentColor" stroke-width="2" stroke-linecap="round" stroke-linejoin="round" class="lucide lucide-thumbs-up h-4 w-4 fill-none" data-sentry-element="ThumbsUpIcon" data-sentry-source-file="TopNavigation.tsx"><path d="M7 10v12"></path><path d="M15 5.88 14 10h5.83a2 2 0 0 1 1.92 2.56l-2.33 8A2 2 0 0 1 17.5 22H4a2 2 0 0 1-2-2v-8a2 2 0 0 1 2-2h2.76a2 2 0 0 0 1.79-1.11L12 2a3.13 3.13 0 0 1 3 3.88Z"></path></svg></button><button class="inline-flex items-center justify-center whitespace-nowrap rounded-md text-sm ring-offset-white transition-all duration-200 outline-none focus-visible:outline-none disabled:pointer-events-none disabled:opacity-50 dark:ring-offset-neutral-950 hover:bg-[#9a20360a] hover:text-customRed dark:text-white dark:hover:bg-customRed/25 enabled:active:ring-2 enabled:active:ring-[#9a20360a] !rounded-full h-8 w-8" aria-label="Download from arXiv" data-sentry-element="Button" data-sentry-source-file="TopNavigation.tsx"><svg xmlns="http://www.w3.org/2000/svg" width="24" height="24" viewBox="0 0 24 24" fill="none" stroke="currentColor" stroke-width="2" stroke-linecap="round" stroke-linejoin="round" class="lucide lucide-download h-4 w-4" data-sentry-element="DownloadIcon" data-sentry-source-file="TopNavigation.tsx"><path d="M21 15v4a2 2 0 0 1-2 2H5a2 2 0 0 1-2-2v-4"></path><polyline points="7 10 12 15 17 10"></polyline><line x1="12" x2="12" y1="15" y2="3"></line></svg></button><div class="relative" data-sentry-component="PaperFeedBookmarks" data-sentry-source-file="PaperFeedBookmarks.tsx"><button class="group flex h-8 w-8 items-center justify-center rounded-full text-gray-900 transition-all hover:bg-customRed/10 dark:text-white dark:hover:bg-customRed/10"><svg xmlns="http://www.w3.org/2000/svg" width="24" height="24" viewBox="0 0 24 24" fill="none" stroke="currentColor" stroke-width="2" stroke-linecap="round" stroke-linejoin="round" class="lucide lucide-bookmark h-4 w-4 text-gray-900 transition-colors group-hover:text-customRed dark:text-white dark:group-hover:text-customRed" data-sentry-element="Bookmark" data-sentry-component="renderBookmarkContent" data-sentry-source-file="PaperFeedBookmarks.tsx"><path d="m19 21-7-4-7 4V5a2 2 0 0 1 2-2h10a2 2 0 0 1 2 2v16z"></path></svg></button></div><button class="inline-flex items-center justify-center whitespace-nowrap rounded-md text-sm ring-offset-white transition-all duration-200 outline-none focus-visible:outline-none disabled:pointer-events-none disabled:opacity-50 dark:ring-offset-neutral-950 hover:bg-[#9a20360a] hover:text-customRed dark:text-white dark:hover:bg-customRed/25 enabled:active:ring-2 enabled:active:ring-[#9a20360a] !rounded-full focus-visible:outline-0 h-8 w-8" type="button" id="radix-:R3drrulb:" aria-haspopup="menu" aria-expanded="false" data-state="closed"><svg xmlns="http://www.w3.org/2000/svg" width="24" height="24" viewBox="0 0 24 24" fill="none" stroke="currentColor" stroke-width="2" stroke-linecap="round" stroke-linejoin="round" class="lucide lucide-info h-4 w-4"><circle cx="12" cy="12" r="10"></circle><path d="M12 16v-4"></path><path d="M12 8h.01"></path></svg></button></div></div></div></div><div class="!relative !flex !h-[calc(100dvh-48px)] !flex-col overflow-hidden md:!flex-row" data-sentry-component="CommentsProvider" data-sentry-source-file="CommentsProvider.tsx"><div class="Viewer relative flex h-full flex-col" style="width:60%;height:100%"><h1 class="hidden">Transformers without Normalization</h1><div class="paperBody absolute h-full w-full flex-col overflow-auto" data-sentry-component="PDFViewerContainer" data-sentry-source-file="PaperPane.tsx"><div class="absolute flex h-svh w-full flex-[4] flex-col items-center justify-center"><svg xmlns="http://www.w3.org/2000/svg" width="24" height="24" viewBox="0 0 24 24" fill="none" stroke="currentColor" stroke-width="1.5" stroke-linecap="round" stroke-linejoin="round" class="lucide lucide-loader-circle size-20 animate-spin text-customRed"><path d="M21 12a9 9 0 1 1-6.219-8.56"></path></svg></div><!--$!--><template data-dgst="BAILOUT_TO_CLIENT_SIDE_RENDERING"></template><!--/$--></div></div><div id="rightSidePane" class="flex flex-1 flex-grow flex-col overflow-x-hidden overflow-y-scroll h-[calc(100dvh-100%px)]" data-sentry-component="RightSidePane" data-sentry-source-file="RightSidePane.tsx"><div class="flex h-full flex-col"><div id="rightSidePaneContent" class="flex min-h-0 flex-1 flex-col overflow-hidden"><div class="sticky top-0 z-10"><div class="border-b-1 sticky top-0 z-10 flex h-12 items-center justify-between border-b bg-white/80 dark:border-b-gray-700 dark:bg-transparent" data-sentry-component="CreateQuestionPane" data-sentry-source-file="CreateQuestionPane.tsx"><div class="flex w-full items-center justify-between px-1"><div class="flex min-w-0 items-center"><div class="scrollbar-hide flex min-w-0 items-center space-x-2 overflow-x-auto"><button class="radius-2 relative flex items-center gap-1.5 whitespace-nowrap px-4 py-1.5 text-base transition-all duration-200 hover:bg-gray-50 dark:hover:bg-transparent dark:hover:text-gray-200 font-semibold text-customRed dark:text-white"><span class="mr-1.5">Comments</span></button><button class="radius-2 relative flex items-center gap-1.5 whitespace-nowrap px-4 py-1.5 text-base text-gray-900 transition-all duration-200 hover:bg-gray-50 dark:text-gray-400 dark:hover:bg-transparent dark:hover:text-gray-200"><span class="mr-1.5">My Notes</span></button><button class="radius-2 relative flex items-center gap-1.5 whitespace-nowrap px-4 py-1.5 text-base text-gray-900 transition-all duration-200 hover:bg-gray-50 dark:text-gray-400 dark:hover:bg-transparent dark:hover:text-gray-200">Chat</button><button class="radius-2 relative flex items-center gap-1.5 whitespace-nowrap px-4 py-1.5 text-base text-gray-900 transition-all duration-200 hover:bg-gray-50 dark:text-gray-400 dark:hover:bg-transparent dark:hover:text-gray-200">Similar</button></div></div><div class="ml-4 flex shrink-0 md:hidden"><button class="flex items-center gap-2 rounded-full px-4 py-2 text-sm text-gray-700 transition-all duration-200 hover:bg-gray-50 dark:text-gray-200 dark:hover:bg-gray-800/50" disabled=""><svg xmlns="http://www.w3.org/2000/svg" width="24" height="24" viewBox="0 0 24 24" fill="none" stroke="currentColor" stroke-width="2" stroke-linecap="round" stroke-linejoin="round" class="lucide lucide-thumbs-up h-4 w-4 transition-transform hover:scale-110 fill-none" data-sentry-element="ThumbsUpIcon" data-sentry-source-file="CreateQuestionPane.tsx"><path d="M7 10v12"></path><path d="M15 5.88 14 10h5.83a2 2 0 0 1 1.92 2.56l-2.33 8A2 2 0 0 1 17.5 22H4a2 2 0 0 1-2-2v-8a2 2 0 0 1 2-2h2.76a2 2 0 0 0 1.79-1.11L12 2a3.13 3.13 0 0 1 3 3.88Z"></path></svg></button></div></div></div></div><div class="flex-1 overflow-y-auto"><!--$!--><template data-dgst="BAILOUT_TO_CLIENT_SIDE_RENDERING"></template><!--/$--><div id="scrollablePane" class="z-0 h-full flex-shrink flex-grow basis-auto overflow-y-scroll bg-transparent pt-2 dark:bg-[#1F1F1F]" data-sentry-component="ScrollableQuestionPane" data-sentry-source-file="ScrollableQuestionPane.tsx"><div class="relative bg-inherit pb-2 pl-2 pr-2 pt-1 md:pb-3 md:pl-3 md:pr-3" data-sentry-component="EmptyQuestionBox" data-sentry-source-file="EmptyQuestionBox.tsx"><div class="w-auto overflow-visible rounded-lg border border-gray-200 bg-white p-3 dark:border-gray-700 dark:bg-[#1f1f1f]"><div class="relative flex flex-col gap-3"><textarea class="w-full resize-none border-none bg-transparent p-2 text-gray-800 placeholder-gray-400 focus:outline-none dark:text-gray-200" placeholder="Leave a public question" rows="2"></textarea><div class="flex items-center gap-2 border-t border-gray-100 px-2 pt-2 dark:border-gray-800"><span class="text-xs text-gray-500 dark:text-gray-400">Authors will be notified</span><div class="flex -space-x-2"><button class="whitespace-nowrap rounded-md text-sm ring-offset-white duration-200 outline-none focus-visible:outline-none disabled:pointer-events-none disabled:opacity-50 dark:ring-offset-neutral-950 enabled:active:ring-2 enabled:active:ring-[#9a20360a] !rounded-full flex h-6 w-6 transform cursor-pointer items-center justify-center border-2 border-white bg-gray-200 text-gray-500 transition-all hover:bg-gray-300 hover:text-gray-600 dark:border-[#1f1f1f] dark:bg-gray-700 dark:text-gray-400 dark:hover:bg-gray-700 dark:hover:text-gray-200" data-sentry-element="Button" data-sentry-source-file="AuthorVerifyDialog.tsx" data-state="closed"><svg xmlns="http://www.w3.org/2000/svg" width="24" height="24" viewBox="0 0 24 24" fill="none" stroke="currentColor" stroke-width="2" stroke-linecap="round" stroke-linejoin="round" class="lucide lucide-plus size-4" data-sentry-element="PlusIcon" data-sentry-source-file="AuthorVerifyDialog.tsx"><path d="M5 12h14"></path><path d="M12 5v14"></path></svg></button></div></div></div></div></div><div><div class="hidden flex-row px-3 text-gray-500 md:flex"><div class="flex gap-2" data-sentry-component="MutateQuestion" data-sentry-source-file="MutateQuestion.tsx"><button class="inline-flex items-center justify-center whitespace-nowrap rounded-md text-sm ring-offset-white transition-all duration-200 outline-none focus-visible:outline-none disabled:opacity-50 dark:ring-offset-neutral-950 dark:text-white dark:hover:bg-customRed/25 enabled:active:ring-2 enabled:active:ring-[#9a20360a] h-10 w-10 !rounded-full focus-visible:outline-0 hover:bg-gray-100 hover:text-inherit disabled:pointer-events-auto" aria-label="Filter comments" data-sentry-element="Button" data-sentry-source-file="MutateQuestion.tsx" type="button" id="radix-:Rqqlabrulb:" aria-haspopup="menu" aria-expanded="false" data-state="closed"><svg xmlns="http://www.w3.org/2000/svg" width="24" height="24" viewBox="0 0 24 24" fill="none" stroke="currentColor" stroke-width="2" stroke-linecap="round" stroke-linejoin="round" class="lucide lucide-filter h-5 w-5" data-sentry-element="FilterIcon" data-sentry-source-file="MutateQuestion.tsx"><polygon points="22 3 2 3 10 12.46 10 19 14 21 14 12.46 22 3"></polygon></svg></button><button class="inline-flex items-center justify-center whitespace-nowrap rounded-md text-sm ring-offset-white transition-all duration-200 outline-none focus-visible:outline-none disabled:opacity-50 dark:ring-offset-neutral-950 dark:text-white dark:hover:bg-customRed/25 enabled:active:ring-2 enabled:active:ring-[#9a20360a] h-10 w-10 !rounded-full focus-visible:outline-0 hover:bg-gray-100 hover:text-inherit disabled:pointer-events-auto" aria-label="Sort comments" data-sentry-element="Button" data-sentry-source-file="MutateQuestion.tsx" type="button" id="radix-:Rsqlabrulb:" aria-haspopup="menu" aria-expanded="false" data-state="closed"><svg xmlns="http://www.w3.org/2000/svg" width="24" height="24" viewBox="0 0 24 24" fill="none" stroke="currentColor" stroke-width="2" stroke-linecap="round" stroke-linejoin="round" class="lucide lucide-arrow-down-wide-narrow h-5 w-5" data-sentry-element="ArrowDownWideNarrowIcon" data-sentry-source-file="MutateQuestion.tsx"><path d="m3 16 4 4 4-4"></path><path d="M7 20V4"></path><path d="M11 4h10"></path><path d="M11 8h7"></path><path d="M11 12h4"></path></svg></button></div></div><!--$!--><template data-dgst="BAILOUT_TO_CLIENT_SIDE_RENDERING"></template><!--/$--><!--$!--><template data-dgst="BAILOUT_TO_CLIENT_SIDE_RENDERING"></template><!--/$--></div></div></div></div></div></div></div></div><script src="/_next/static/chunks/webpack-a7b03224847ab321.js" async=""></script><script>(self.__next_f=self.__next_f||[]).push([0])</script><script>self.__next_f.push([1,"1:\"$Sreact.fragment\"\n2:I[85963,[\"3110\",\"static/chunks/1da0d171-1f9041fa20b0f780.js\",\"6117\",\"static/chunks/6117-41689ef6ff9b033c.js\",\"1350\",\"static/chunks/1350-a1024eb8f8a6859e.js\",\"1447\",\"static/chunks/1447-9124dd5e537fe7a8.js\",\"666\",\"static/chunks/666-78ffa3c0d0a5a2a3.js\",\"5872\",\"static/chunks/5872-dd1cde135170beb6.js\",\"7362\",\"static/chunks/7362-50e5d1ac2abc44a0.js\",\"2749\",\"static/chunks/2749-95477708edcb2a1e.js\",\"1615\",\"static/chunks/1615-1f6676350ab288e8.js\",\"4964\",\"static/chunks/4964-2cba8177dbece8d4.js\",\"7177\",\"static/chunks/app/layout-a39c2199527f5444.js\"],\"GoogleAnalytics\"]\n3:\"$Sreact.suspense\"\n4:I[6877,[\"3110\",\"static/chunks/1da0d171-1f9041fa20b0f780.js\",\"6117\",\"static/chunks/6117-41689ef6ff9b033c.js\",\"1350\",\"static/chunks/1350-a1024eb8f8a6859e.js\",\"1447\",\"static/chunks/1447-9124dd5e537fe7a8.js\",\"666\",\"static/chunks/666-78ffa3c0d0a5a2a3.js\",\"5872\",\"static/chunks/5872-dd1cde135170beb6.js\",\"7362\",\"static/chunks/7362-50e5d1ac2abc44a0.js\",\"2749\",\"static/chunks/2749-95477708edcb2a1e.js\",\"1615\",\"static/chunks/1615-1f6676350ab288e8.js\",\"4964\",\"static/chunks/4964-2cba8177dbece8d4.js\",\"7177\",\"static/chunks/app/layout-a39c2199527f5444.js\"],\"ProgressBar\"]\n5:I[58117,[\"3110\",\"static/chunks/1da0d171-1f9041fa20b0f780.js\",\"6117\",\"static/chunks/6117-41689ef6ff9b033c.js\",\"1350\",\"static/chunks/1350-a1024eb8f8a6859e.js\",\"1447\",\"static/chunks/1447-9124dd5e537fe7a8.js\",\"666\",\"static/chunks/666-78ffa3c0d0a5a2a3.js\",\"5872\",\"static/chunks/5872-dd1cde135170beb6.js\",\"7362\",\"static/chunks/7362-50e5d1ac2abc44a0.js\",\"2749\",\"static/chunks/2749-95477708edcb2a1e.js\",\"1615\",\"static/chunks/1615-1f6676350ab288e8.js\",\"4964\",\"static/chunks/4964-2cba8177dbece8d4.js\",\"7177\",\"static/chunks/app/layout-a39c2199527f5444.js\"],\"default\"]\n7:I[43202,[],\"\"]\n8:I[24560,[],\"\"]\nb:I[77179,[],\"OutletBoundary\"]\nd:I[77179,[],\"MetadataBoundary\"]\nf:I[77179,[],\"ViewportBoundary\"]\n11:I[74997,[\"4219\",\"static/chunks/app/global-error-57044b847d6c9be6.js\"],\"default\"]\n12:I[78357,[\"3110\",\"static/chunks/1da0d171-1f9041fa20b0f780.js\",\"6117\",\"static/chunks/6117-41689ef6ff9b"])</script><script>self.__next_f.push([1,"033c.js\",\"1350\",\"static/chunks/1350-a1024eb8f8a6859e.js\",\"8951\",\"static/chunks/8951-bcdd0e91584e856e.js\",\"1447\",\"static/chunks/1447-9124dd5e537fe7a8.js\",\"666\",\"static/chunks/666-78ffa3c0d0a5a2a3.js\",\"932\",\"static/chunks/932-76dfd6658f5c91af.js\",\"4765\",\"static/chunks/4765-88aa2d5b19cb25bc.js\",\"7362\",\"static/chunks/7362-50e5d1ac2abc44a0.js\",\"9832\",\"static/chunks/9832-86d2bf8ed3fa1d9e.js\",\"4964\",\"static/chunks/4964-2cba8177dbece8d4.js\",\"8545\",\"static/chunks/8545-1bc4c81889a95ea0.js\",\"7446\",\"static/chunks/7446-1a273b84bf468616.js\",\"7977\",\"static/chunks/app/(paper)/%5Bid%5D/abs/page-17f212a359917e65.js\"],\"default\"]\n:HL[\"/_next/static/css/a6f6df2afb96a7a2.css\",\"style\"]\n:HL[\"/_next/static/media/a34f9d1faa5f3315-s.p.woff2\",\"font\",{\"crossOrigin\":\"\",\"type\":\"font/woff2\"}]\n:HL[\"/_next/static/css/1baa833b56016a20.css\",\"style\"]\n:HL[\"/_next/static/css/b57b729bdae0dee2.css\",\"style\"]\n:HL[\"/_next/static/css/acdaad1d23646914.css\",\"style\"]\n:HL[\"/_next/static/css/a7815692be819096.css\",\"style\"]\n"])</script><script>self.__next_f.push([1,"0:{\"P\":null,\"b\":\"Z1Y-2Dr4q-jwn9aCFlXNi\",\"p\":\"\",\"c\":[\"\",\"abs\",\"2503.10622\"],\"i\":false,\"f\":[[[\"\",{\"children\":[\"(paper)\",{\"children\":[[\"id\",\"2503.10622\",\"d\"],{\"children\":[\"abs\",{\"children\":[\"__PAGE__\",{}]}]}]}]},\"$undefined\",\"$undefined\",true],[\"\",[\"$\",\"$1\",\"c\",{\"children\":[[[\"$\",\"link\",\"0\",{\"rel\":\"stylesheet\",\"href\":\"/_next/static/css/a6f6df2afb96a7a2.css\",\"precedence\":\"next\",\"crossOrigin\":\"$undefined\",\"nonce\":\"$undefined\"}]],[\"$\",\"html\",null,{\"lang\":\"en\",\"data-sentry-component\":\"RootLayout\",\"data-sentry-source-file\":\"layout.tsx\",\"children\":[[\"$\",\"head\",null,{\"children\":[[\"$\",\"$L2\",null,{\"gaId\":\"G-94SEL844DQ\",\"data-sentry-element\":\"GoogleAnalytics\",\"data-sentry-source-file\":\"layout.tsx\"}],[\"$\",\"link\",null,{\"rel\":\"preconnect\",\"href\":\"https://fonts.googleapis.com\"}],[\"$\",\"link\",null,{\"rel\":\"preconnect\",\"href\":\"https://fonts.gstatic.com\",\"crossOrigin\":\"anonymous\"}],[\"$\",\"link\",null,{\"href\":\"https://fonts.googleapis.com/css2?family=Inter:wght@100..900\u0026family=Onest:wght@100..900\u0026family=Rubik:ital,wght@0,300..900;1,300..900\u0026display=swap\",\"rel\":\"stylesheet\"}],[\"$\",\"script\",null,{\"src\":\"https://accounts.google.com/gsi/client\",\"async\":true,\"defer\":true}],[\"$\",\"link\",null,{\"rel\":\"apple-touch-icon\",\"sizes\":\"1024x1024\",\"href\":\"/assets/pwa/alphaxiv_app_1024.png\"}],[\"$\",\"meta\",null,{\"name\":\"theme-color\",\"content\":\"#FFFFFF\",\"data-sentry-element\":\"meta\",\"data-sentry-source-file\":\"layout.tsx\"}]]}],[\"$\",\"body\",null,{\"className\":\"h-screen overflow-hidden\",\"children\":[[\"$\",\"$3\",null,{\"data-sentry-element\":\"Suspense\",\"data-sentry-source-file\":\"layout.tsx\",\"children\":[\"$\",\"$L4\",null,{\"data-sentry-element\":\"ProgressBar\",\"data-sentry-source-file\":\"layout.tsx\"}]}],[\"$\",\"div\",null,{\"id\":\"root\",\"children\":[\"$\",\"$L5\",null,{\"data-sentry-element\":\"Providers\",\"data-sentry-source-file\":\"layout.tsx\",\"children\":\"$L6\"}]}]]}]]}]]}],{\"children\":[\"(paper)\",[\"$\",\"$1\",\"c\",{\"children\":[null,[\"$\",\"$L7\",null,{\"parallelRouterKey\":\"children\",\"segmentPath\":[\"children\",\"(paper)\",\"children\"],\"error\":\"$undefined\",\"errorStyles\":\"$undefined\",\"errorScripts\":\"$undefined\",\"template\":[\"$\",\"$L8\",null,{}],\"templateStyles\":\"$undefined\",\"templateScripts\":\"$undefined\",\"notFound\":\"$undefined\",\"forbidden\":\"$undefined\",\"unauthorized\":\"$undefined\"}]]}],{\"children\":[[\"id\",\"2503.10622\",\"d\"],[\"$\",\"$1\",\"c\",{\"children\":[[[\"$\",\"link\",\"0\",{\"rel\":\"stylesheet\",\"href\":\"/_next/static/css/1baa833b56016a20.css\",\"precedence\":\"next\",\"crossOrigin\":\"$undefined\",\"nonce\":\"$undefined\"}],[\"$\",\"link\",\"1\",{\"rel\":\"stylesheet\",\"href\":\"/_next/static/css/b57b729bdae0dee2.css\",\"precedence\":\"next\",\"crossOrigin\":\"$undefined\",\"nonce\":\"$undefined\"}],[\"$\",\"link\",\"2\",{\"rel\":\"stylesheet\",\"href\":\"/_next/static/css/acdaad1d23646914.css\",\"precedence\":\"next\",\"crossOrigin\":\"$undefined\",\"nonce\":\"$undefined\"}],[\"$\",\"link\",\"3\",{\"rel\":\"stylesheet\",\"href\":\"/_next/static/css/a7815692be819096.css\",\"precedence\":\"next\",\"crossOrigin\":\"$undefined\",\"nonce\":\"$undefined\"}]],\"$L9\"]}],{\"children\":[\"abs\",[\"$\",\"$1\",\"c\",{\"children\":[null,[\"$\",\"$L7\",null,{\"parallelRouterKey\":\"children\",\"segmentPath\":[\"children\",\"(paper)\",\"children\",\"$0:f:0:1:2:children:2:children:0\",\"children\",\"abs\",\"children\"],\"error\":\"$undefined\",\"errorStyles\":\"$undefined\",\"errorScripts\":\"$undefined\",\"template\":[\"$\",\"$L8\",null,{}],\"templateStyles\":\"$undefined\",\"templateScripts\":\"$undefined\",\"notFound\":\"$undefined\",\"forbidden\":\"$undefined\",\"unauthorized\":\"$undefined\"}]]}],{\"children\":[\"__PAGE__\",[\"$\",\"$1\",\"c\",{\"children\":[\"$La\",null,[\"$\",\"$Lb\",null,{\"children\":\"$Lc\"}]]}],{},null,false]},null,false]},null,false]},null,false]},null,false],[\"$\",\"$1\",\"h\",{\"children\":[null,[\"$\",\"$1\",\"c7-Ua2GvpPgw0RVnpFZ0a\",{\"children\":[[\"$\",\"$Ld\",null,{\"children\":\"$Le\"}],[\"$\",\"$Lf\",null,{\"children\":\"$L10\"}],[\"$\",\"meta\",null,{\"name\":\"next-size-adjust\",\"content\":\"\"}]]}]]}],false]],\"m\":\"$undefined\",\"G\":[\"$11\",[]],\"s\":false,\"S\":false}\n"])</script><script>self.__next_f.push([1,"a:[\"$\",\"$L12\",null,{\"paperId\":\"2503.10622\",\"searchParams\":{},\"data-sentry-element\":\"DetailView\",\"data-sentry-source-file\":\"page.tsx\"}]\n10:[[\"$\",\"meta\",\"0\",{\"name\":\"viewport\",\"content\":\"width=device-width, initial-scale=1, viewport-fit=cover\"}]]\n"])</script><script>self.__next_f.push([1,"13:I[50709,[\"3110\",\"static/chunks/1da0d171-1f9041fa20b0f780.js\",\"6906\",\"static/chunks/62420ecc-ba068cf8c61f9a07.js\",\"2029\",\"static/chunks/9d987bc4-d447aa4b86ffa8da.js\",\"7701\",\"static/chunks/c386c4a4-4ae2baf83c93de20.js\",\"6117\",\"static/chunks/6117-41689ef6ff9b033c.js\",\"1350\",\"static/chunks/1350-a1024eb8f8a6859e.js\",\"8951\",\"static/chunks/8951-bcdd0e91584e856e.js\",\"1447\",\"static/chunks/1447-9124dd5e537fe7a8.js\",\"666\",\"static/chunks/666-78ffa3c0d0a5a2a3.js\",\"932\",\"static/chunks/932-76dfd6658f5c91af.js\",\"5872\",\"static/chunks/5872-dd1cde135170beb6.js\",\"7299\",\"static/chunks/7299-64abce2685056cd4.js\",\"4765\",\"static/chunks/4765-88aa2d5b19cb25bc.js\",\"7362\",\"static/chunks/7362-50e5d1ac2abc44a0.js\",\"2068\",\"static/chunks/2068-7fbc56857b0cc3b1.js\",\"2755\",\"static/chunks/2755-54255117838ce4e4.js\",\"4505\",\"static/chunks/4505-4fe5d5f302c56050.js\",\"8273\",\"static/chunks/8273-4cb3558ea58359d7.js\",\"6681\",\"static/chunks/6681-13aed21c8bb47aa3.js\",\"4005\",\"static/chunks/4005-6fe3c26cb25644be.js\",\"4785\",\"static/chunks/4785-5dbc1af26cd46ec5.js\",\"6335\",\"static/chunks/6335-5d291246680ceb4d.js\",\"2642\",\"static/chunks/2642-b497e0f313459fb9.js\",\"5145\",\"static/chunks/5145-f10798defa0dde88.js\",\"8114\",\"static/chunks/8114-2172b7ef97f83184.js\",\"9392\",\"static/chunks/9392-5fab98d8656406c4.js\",\"9305\",\"static/chunks/app/(paper)/%5Bid%5D/layout-3e11c64ff66d737f.js\"],\"Hydrate\"]\n87:I[44029,[\"3110\",\"static/chunks/1da0d171-1f9041fa20b0f780.js\",\"6117\",\"static/chunks/6117-41689ef6ff9b033c.js\",\"1350\",\"static/chunks/1350-a1024eb8f8a6859e.js\",\"1447\",\"static/chunks/1447-9124dd5e537fe7a8.js\",\"666\",\"static/chunks/666-78ffa3c0d0a5a2a3.js\",\"5872\",\"static/chunks/5872-dd1cde135170beb6.js\",\"7362\",\"static/chunks/7362-50e5d1ac2abc44a0.js\",\"2749\",\"static/chunks/2749-95477708edcb2a1e.js\",\"1615\",\"static/chunks/1615-1f6676350ab288e8.js\",\"4964\",\"static/chunks/4964-2cba8177dbece8d4.js\",\"7177\",\"static/chunks/app/layout-a39c2199527f5444.js\"],\"default\"]\n88:I[93727,[\"3110\",\"static/chunks/1da0d171-1f9041fa20b0f780.js\",\"6117\",\"static/chunks/6117-41689ef6ff9b033c.js\",\"1350\",\"static/chunks"])</script><script>self.__next_f.push([1,"/1350-a1024eb8f8a6859e.js\",\"1447\",\"static/chunks/1447-9124dd5e537fe7a8.js\",\"666\",\"static/chunks/666-78ffa3c0d0a5a2a3.js\",\"5872\",\"static/chunks/5872-dd1cde135170beb6.js\",\"7362\",\"static/chunks/7362-50e5d1ac2abc44a0.js\",\"2749\",\"static/chunks/2749-95477708edcb2a1e.js\",\"1615\",\"static/chunks/1615-1f6676350ab288e8.js\",\"4964\",\"static/chunks/4964-2cba8177dbece8d4.js\",\"7177\",\"static/chunks/app/layout-a39c2199527f5444.js\"],\"default\"]\n89:I[43761,[\"6117\",\"static/chunks/6117-41689ef6ff9b033c.js\",\"8951\",\"static/chunks/8951-bcdd0e91584e856e.js\",\"8039\",\"static/chunks/app/error-a92d22105c18293c.js\"],\"default\"]\n8a:I[68951,[\"3110\",\"static/chunks/1da0d171-1f9041fa20b0f780.js\",\"6906\",\"static/chunks/62420ecc-ba068cf8c61f9a07.js\",\"2029\",\"static/chunks/9d987bc4-d447aa4b86ffa8da.js\",\"7701\",\"static/chunks/c386c4a4-4ae2baf83c93de20.js\",\"6117\",\"static/chunks/6117-41689ef6ff9b033c.js\",\"1350\",\"static/chunks/1350-a1024eb8f8a6859e.js\",\"8951\",\"static/chunks/8951-bcdd0e91584e856e.js\",\"1447\",\"static/chunks/1447-9124dd5e537fe7a8.js\",\"666\",\"static/chunks/666-78ffa3c0d0a5a2a3.js\",\"932\",\"static/chunks/932-76dfd6658f5c91af.js\",\"5872\",\"static/chunks/5872-dd1cde135170beb6.js\",\"7299\",\"static/chunks/7299-64abce2685056cd4.js\",\"4765\",\"static/chunks/4765-88aa2d5b19cb25bc.js\",\"7362\",\"static/chunks/7362-50e5d1ac2abc44a0.js\",\"2068\",\"static/chunks/2068-7fbc56857b0cc3b1.js\",\"2755\",\"static/chunks/2755-54255117838ce4e4.js\",\"4505\",\"static/chunks/4505-4fe5d5f302c56050.js\",\"8273\",\"static/chunks/8273-4cb3558ea58359d7.js\",\"6681\",\"static/chunks/6681-13aed21c8bb47aa3.js\",\"4005\",\"static/chunks/4005-6fe3c26cb25644be.js\",\"4785\",\"static/chunks/4785-5dbc1af26cd46ec5.js\",\"6335\",\"static/chunks/6335-5d291246680ceb4d.js\",\"2642\",\"static/chunks/2642-b497e0f313459fb9.js\",\"5145\",\"static/chunks/5145-f10798defa0dde88.js\",\"8114\",\"static/chunks/8114-2172b7ef97f83184.js\",\"9392\",\"static/chunks/9392-5fab98d8656406c4.js\",\"9305\",\"static/chunks/app/(paper)/%5Bid%5D/layout-3e11c64ff66d737f.js\"],\"\"]\n14:T529,CLIP has enabled new and exciting joint vision-language applications, one of which is open-v"])</script><script>self.__next_f.push([1,"ocabulary segmentation, which can locate any segment given an arbitrary text query. In our research, we ask whether it is possible to discover semantic segments without any user guidance in the form of text queries or predefined classes, and label them using natural language automatically? We propose a novel problem zero-guidance segmentation and the first baseline that leverages two pre-trained generalist models, DINO and CLIP, to solve this problem without any fine-tuning or segmentation dataset. The general idea is to first segment an image into small over-segments, encode them into CLIP's visual-language space, translate them into text labels, and merge semantically similar segments together. The key challenge, however, is how to encode a visual segment into a segment-specific embedding that balances global and local context information, both useful for recognition. Our main contribution is a novel attention-masking technique that balances the two contexts by analyzing the attention layers inside CLIP. We also introduce several metrics for the evaluation of this new task. With CLIP's innate knowledge, our method can precisely locate the Mona Lisa painting among a museum crowd. Project page: this https URL.15:T529,CLIP has enabled new and exciting joint vision-language applications, one of which is open-vocabulary segmentation, which can locate any segment given an arbitrary text query. In our research, we ask whether it is possible to discover semantic segments without any user guidance in the form of text queries or predefined classes, and label them using natural language automatically? We propose a novel problem zero-guidance segmentation and the first baseline that leverages two pre-trained generalist models, DINO and CLIP, to solve this problem without any fine-tuning or segmentation dataset. The general idea is to first segment an image into small over-segments, encode them into CLIP's visual-language space, translate them into text labels, and merge semantically similar segments together. The key chall"])</script><script>self.__next_f.push([1,"enge, however, is how to encode a visual segment into a segment-specific embedding that balances global and local context information, both useful for recognition. Our main contribution is a novel attention-masking technique that balances the two contexts by analyzing the attention layers inside CLIP. We also introduce several metrics for the evaluation of this new task. With CLIP's innate knowledge, our method can precisely locate the Mona Lisa painting among a museum crowd. Project page: this https URL.16:T72e,We describe several shortcomings of a study by Patone et al, whose findings\nwere recently published in the American Heart Association Journal Circulation,\nincluding the following:\n * The study's principal conclusion, as initially stated, begins \"Overall, the\nrisk of myocarditis is greater after SARS-CoV-2 infection than after COVID-19\nvaccination ....\" However, Patone et al never attempt to assess the incidence\nof myocarditis in their study population following SARS-CoV-2 infection.\nRather, they make an untenable assumption that all infections occurring in\ntheir study population are associated with (reported) positive COVID-19 tests.\nUsing publicly available data from the UK's ONS and NHS, we show that Patone et\nal's estimates, for the unvaccinated, of myocarditis incidence associated with\ninfection are likely overestimated by a factor of at least 1.58.\n * The method Patone et al use to compute the incidence of myocarditis among\nthe unvaccinated after a positive COVID test may overestimate risk. The authors\nassume, without justification, that unvaccinated persons hospitalized during\nthe study period with positive-test-associated myocarditis would later choose\nto vaccinate with the same probability as unvaccinated persons who have had a\npositive COVID test. We present a plausibility argument that suggests a\npossible further exaggeration of myocarditis risk post infection by a factor of\n1.5.\n * Patone et al fail to discuss important limitations of their study with\nrespect to guiding public health recomme"])</script><script>self.__next_f.push([1,"ndations. For instance, an\ninsignificant number of cases contributing to the study's findings were\nOmicron-variant cases. Thus, the study's estimates of myocarditis risk\nfollowing infection do not speak to the risk following Omicron infection, which\nis recognized to be milder than that of previous variants.17:T72e,We describe several shortcomings of a study by Patone et al, whose findings\nwere recently published in the American Heart Association Journal Circulation,\nincluding the following:\n * The study's principal conclusion, as initially stated, begins \"Overall, the\nrisk of myocarditis is greater after SARS-CoV-2 infection than after COVID-19\nvaccination ....\" However, Patone et al never attempt to assess the incidence\nof myocarditis in their study population following SARS-CoV-2 infection.\nRather, they make an untenable assumption that all infections occurring in\ntheir study population are associated with (reported) positive COVID-19 tests.\nUsing publicly available data from the UK's ONS and NHS, we show that Patone et\nal's estimates, for the unvaccinated, of myocarditis incidence associated with\ninfection are likely overestimated by a factor of at least 1.58.\n * The method Patone et al use to compute the incidence of myocarditis among\nthe unvaccinated after a positive COVID test may overestimate risk. The authors\nassume, without justification, that unvaccinated persons hospitalized during\nthe study period with positive-test-associated myocarditis would later choose\nto vaccinate with the same probability as unvaccinated persons who have had a\npositive COVID test. We present a plausibility argument that suggests a\npossible further exaggeration of myocarditis risk post infection by a factor of\n1.5.\n * Patone et al fail to discuss important limitations of their study with\nrespect to guiding public health recommendations. For instance, an\ninsignificant number of cases contributing to the study's findings were\nOmicron-variant cases. Thus, the study's estimates of myocarditis risk\nfollowing infection do not speak"])</script><script>self.__next_f.push([1," to the risk following Omicron infection, which\nis recognized to be milder than that of previous variants.18:T4bb,The goal of building a benchmark (suite of datasets) is to provide a unified\nprotocol for fair evaluation and thus facilitate the evolution of a specific\narea. Nonetheless, we point out that existing protocols of action recognition\ncould yield partial evaluations due to several limitations. To comprehensively\nprobe the effectiveness of spatiotemporal representation learning, we introduce\nBEAR, a new BEnchmark on video Action Recognition. BEAR is a collection of 18\nvideo datasets grouped into 5 categories (anomaly, gesture, daily, sports, and\ninstructional), which covers a diverse set of real-world applications. With\nBEAR, we thoroughly evaluate 6 common spatiotemporal models pre-trained by both\nsupervised and self-supervised learning. We also report transfer performance\nvia standard finetuning, few-shot finetuning, and unsupervised domain\nadaptation. Our observation suggests that current state-of-the-art cannot\nsolidly guarantee high performance on datasets close to real-world\napplications, and we hope BEAR can serve as a fair and challenging evaluation\nbenchmark to gain insights on building next-generation spatiotemporal learners.\nOur dataset, code, and models are released at:\nthis https URL19:T4bb,The goal of building a benchmark (suite of datasets) is to provide a unified\nprotocol for fair evaluation and thus facilitate the evolution of a specific\narea. Nonetheless, we point out that existing protocols of action recognition\ncould yield partial evaluations due to several limitations. To comprehensively\nprobe the effectiveness of spatiotemporal representation learning, we introduce\nBEAR, a new BEnchmark on video Action Recognition. BEAR is a collection of 18\nvideo datasets grouped into 5 categories (anomaly, gesture, daily, sports, and\ninstructional), which covers a diverse set of real-world applications. With\nBEAR, we thoroughly evaluate 6 common spatiotemporal models pre-trained by both\nsupervised "])</script><script>self.__next_f.push([1,"and self-supervised learning. We also report transfer performance\nvia standard finetuning, few-shot finetuning, and unsupervised domain\nadaptation. Our observation suggests that current state-of-the-art cannot\nsolidly guarantee high performance on datasets close to real-world\napplications, and we hope BEAR can serve as a fair and challenging evaluation\nbenchmark to gain insights on building next-generation spatiotemporal learners.\nOur dataset, code, and models are released at:\nthis https URL1a:T4aa,We review here some aspects of our recent works about the geometric\nengineering of heterotic little string theories using F-theory. Building on the\nseminal work by Aspinwall and Morrison as well as Intrilligator and Blum, we\nsolve some longstanding open questions thanks to recent progress in our\nunderstanding of 6D (1,0) theories and their generalized symmetries. On the\ngeometry side, these systems correspond to non-compact elliptically fibered\nCalabi-Yau varieties that must admit the structure of an elliptic K3 fibration.\nFrom fiberwise F-theory/Heterotic duality the K3 plays a central role - it\ndetermines the 6D flavor group, as well as different T-dual LSTs via\ninequivalent elliptic fibration structures. The geometries we obtain are some\nfiner versions of Kulikov degenerations: the point where the K3 fiber\ndegenerates is the locus where the LST arises. This structure serve on one hand\nto check our field theory predictions on LST dualities via the match of Coulomb\nbranch dimension, flavor symmetries, and 2-group structure constants, and also\non the other hand to deduce novel LST models and their networks of dualities,\nthus allowing exploring non-geometric Heterotic regimes.1b:T4aa,We review here some aspects of our recent works about the geometric\nengineering of heterotic little string theories using F-theory. Building on the\nseminal work by Aspinwall and Morrison as well as Intrilligator and Blum, we\nsolve some longstanding open questions thanks to recent progress in our\nunderstanding of 6D (1,0) theories and their "])</script><script>self.__next_f.push([1,"generalized symmetries. On the\ngeometry side, these systems correspond to non-compact elliptically fibered\nCalabi-Yau varieties that must admit the structure of an elliptic K3 fibration.\nFrom fiberwise F-theory/Heterotic duality the K3 plays a central role - it\ndetermines the 6D flavor group, as well as different T-dual LSTs via\ninequivalent elliptic fibration structures. The geometries we obtain are some\nfiner versions of Kulikov degenerations: the point where the K3 fiber\ndegenerates is the locus where the LST arises. This structure serve on one hand\nto check our field theory predictions on LST dualities via the match of Coulomb\nbranch dimension, flavor symmetries, and 2-group structure constants, and also\non the other hand to deduce novel LST models and their networks of dualities,\nthus allowing exploring non-geometric Heterotic regimes.1c:T41c,A potential application for spectral computed tomography (CT) with multi-energy-window photon-counting detectors is quantitative medical imaging with K-edge contrast agents. Image reconstruction for spectral CT with such contrast agents necessitates expression of the X-ray linear attenuation map in at least three expansion functions, for example, bone/water/K-edge-material or photo-electric- process/Compton-process/K-edge-material. The use of three expansion functions can result in slow convergence for iterative image reconstruction (IIR) algorithms applied to spectral CT. We propose a block-diagonal step-preconditioner for use with a primal-dual iterative image reconstruction framework that we have been developing for spectral CT. We demonstrate the advantage of the new step-preconditioner on a sensitive spectral CT simulation where the test object has low concentration of Gadolinium (Gd) contrast agent and the X-ray attenuation map is represented by three materials - PMMA, a soft-tissue equivalent, Aluminum, a bone equivalent, and Gd.1d:T41c,A potential application for spectral computed tomography (CT) with multi-energy-window photon-counting detectors is quantitative"])</script><script>self.__next_f.push([1," medical imaging with K-edge contrast agents. Image reconstruction for spectral CT with such contrast agents necessitates expression of the X-ray linear attenuation map in at least three expansion functions, for example, bone/water/K-edge-material or photo-electric- process/Compton-process/K-edge-material. The use of three expansion functions can result in slow convergence for iterative image reconstruction (IIR) algorithms applied to spectral CT. We propose a block-diagonal step-preconditioner for use with a primal-dual iterative image reconstruction framework that we have been developing for spectral CT. We demonstrate the advantage of the new step-preconditioner on a sensitive spectral CT simulation where the test object has low concentration of Gadolinium (Gd) contrast agent and the X-ray attenuation map is represented by three materials - PMMA, a soft-tissue equivalent, Aluminum, a bone equivalent, and Gd.1e:T660,Diffusion models gain increasing popularity for their generative\ncapabilities. Recently, there have been surging needs to generate customized\nimages by inverting diffusion models from exemplar images, and existing\ninversion methods mainly focus on capturing object appearances (i.e., the\n\"look\"). However, how to invert object relations, another important pillar in\nthe visual world, remains unexplored. In this work, we propose the Relation\nInversion task, which aims to learn a specific relation (represented as\n\"relation prompt\") from exemplar images. Specifically, we learn a relation\nprompt with a frozen pre-trained text-to-image diffusion model. The learned\nrelation prompt can then be applied to generate relation-specific images with\nnew objects, backgrounds, and styles.\nTo tackle the Relation Inversion task, we propose the ReVersion Framework.\nSpecifically, we propose a novel \"relation-steering contrastive learning\"\nscheme to steer the relation prompt towards relation-dense regions, and\ndisentangle it away from object appearances. We further devise \"relation-focal\nimportance sampling\" to emphasize h"])</script><script>self.__next_f.push([1,"igh-level interactions over low-level\nappearances (e.g., texture, color). To comprehensively evaluate this new task,\nwe contribute the ReVersion Benchmark, which provides various exemplar images\nwith diverse relations. Extensive experiments validate the superiority of our\napproach over existing methods across a wide range of visual relations. Our\nproposed task and method could be good inspirations for future research in\nvarious domains like generative inversion, few-shot learning, and visual\nrelation detection.1f:T660,Diffusion models gain increasing popularity for their generative\ncapabilities. Recently, there have been surging needs to generate customized\nimages by inverting diffusion models from exemplar images, and existing\ninversion methods mainly focus on capturing object appearances (i.e., the\n\"look\"). However, how to invert object relations, another important pillar in\nthe visual world, remains unexplored. In this work, we propose the Relation\nInversion task, which aims to learn a specific relation (represented as\n\"relation prompt\") from exemplar images. Specifically, we learn a relation\nprompt with a frozen pre-trained text-to-image diffusion model. The learned\nrelation prompt can then be applied to generate relation-specific images with\nnew objects, backgrounds, and styles.\nTo tackle the Relation Inversion task, we propose the ReVersion Framework.\nSpecifically, we propose a novel \"relation-steering contrastive learning\"\nscheme to steer the relation prompt towards relation-dense regions, and\ndisentangle it away from object appearances. We further devise \"relation-focal\nimportance sampling\" to emphasize high-level interactions over low-level\nappearances (e.g., texture, color). To comprehensively evaluate this new task,\nwe contribute the ReVersion Benchmark, which provides various exemplar images\nwith diverse relations. Extensive experiments validate the superiority of our\napproach over existing methods across a wide range of visual relations. Our\nproposed task and method could be good inspirations for fut"])</script><script>self.__next_f.push([1,"ure research in\nvarious domains like generative inversion, few-shot learning, and visual\nrelation detection.20:T568,Collusive fraud, in which multiple fraudsters collude to defraud health\ninsurance funds, threatens the operation of the healthcare system. However,\nexisting statistical and machine learning-based methods have limited ability to\ndetect fraud in the scenario of health insurance due to the high similarity of\nfraudulent behaviors to normal medical visits and the lack of labeled data. To\nensure the accuracy of the detection results, expert knowledge needs to be\nintegrated with the fraud detection process. By working closely with health\ninsurance audit experts, we propose FraudAuditor, a three-stage visual\nanalytics approach to collusive fraud detection in health insurance.\nSpecifically, we first allow users to interactively construct a co-visit\nnetwork to holistically model the visit relationships of different patients.\nSecond, an improved community detection algorithm that considers the strength\nof fraud likelihood is designed to detect suspicious fraudulent groups.\nFinally, through our visual interface, users can compare, investigate, and\nverify suspicious patient behavior with tailored visualizations that support\ndifferent time scales. We conducted case studies in a real-world healthcare\nscenario, i.e., to help locate the actual fraud group and exclude the false\npositive group. The results and expert feedback proved the effectiveness and\nusability of the approach.21:T568,Collusive fraud, in which multiple fraudsters collude to defraud health\ninsurance funds, threatens the operation of the healthcare system. However,\nexisting statistical and machine learning-based methods have limited ability to\ndetect fraud in the scenario of health insurance due to the high similarity of\nfraudulent behaviors to normal medical visits and the lack of labeled data. To\nensure the accuracy of the detection results, expert knowledge needs to be\nintegrated with the fraud detection process. By working closely with health\nins"])</script><script>self.__next_f.push([1,"urance audit experts, we propose FraudAuditor, a three-stage visual\nanalytics approach to collusive fraud detection in health insurance.\nSpecifically, we first allow users to interactively construct a co-visit\nnetwork to holistically model the visit relationships of different patients.\nSecond, an improved community detection algorithm that considers the strength\nof fraud likelihood is designed to detect suspicious fraudulent groups.\nFinally, through our visual interface, users can compare, investigate, and\nverify suspicious patient behavior with tailored visualizations that support\ndifferent time scales. We conducted case studies in a real-world healthcare\nscenario, i.e., to help locate the actual fraud group and exclude the false\npositive group. The results and expert feedback proved the effectiveness and\nusability of the approach.22:T483,We study metric spaces homeomorphic to a closed oriented manifold from both\ngeometric and analytic perspectives. We show that such spaces (which are\nsometimes called metric manifolds) admit a non-trivial integral current without\nboundary, provided they satisfy some weak assumptions. The existence of such an\nobject should be thought of as an analytic analog of the fundamental class of\nthe space and can also be interpreted as giving a way to make sense of Stokes'\ntheorem in this setting. Using our existence result, we establish that\nRiemannian manifolds are Lipschitz-volume rigid among certain metric manifolds\nand we show the validity of (relative) isoperimetric inequalities in metric\n$n$-manifolds that are Ahlfors $n$-regular and linearly locally contractible.\nThe former statement is a generalization of a well-known Lipschitz-volume\nrigidity result in Riemannian geometry and the latter yields a relatively short\nand conceptually simple proof of a deep theorem of Semmes about the validity of\nPoincar\\'e inequalities in these spaces. Finally, as a further application, we\nalso give sufficient conditions for a metric manifold to be rectifiable.23:T483,We study metric spaces homeomorph"])</script><script>self.__next_f.push([1,"ic to a closed oriented manifold from both\ngeometric and analytic perspectives. We show that such spaces (which are\nsometimes called metric manifolds) admit a non-trivial integral current without\nboundary, provided they satisfy some weak assumptions. The existence of such an\nobject should be thought of as an analytic analog of the fundamental class of\nthe space and can also be interpreted as giving a way to make sense of Stokes'\ntheorem in this setting. Using our existence result, we establish that\nRiemannian manifolds are Lipschitz-volume rigid among certain metric manifolds\nand we show the validity of (relative) isoperimetric inequalities in metric\n$n$-manifolds that are Ahlfors $n$-regular and linearly locally contractible.\nThe former statement is a generalization of a well-known Lipschitz-volume\nrigidity result in Riemannian geometry and the latter yields a relatively short\nand conceptually simple proof of a deep theorem of Semmes about the validity of\nPoincar\\'e inequalities in these spaces. Finally, as a further application, we\nalso give sufficient conditions for a metric manifold to be rectifiable.24:T565,Humans naturally perceive surrounding scenes by unifying sound and sight in a first-person view. Likewise, machines are advanced to approach human intelligence by learning with multisensory inputs from an egocentric perspective. In this paper, we explore the challenging egocentric audio-visual object localization task and observe that 1) egomotion commonly exists in first-person recordings, even within a short duration; 2) The out-of-view sound components can be created while wearers shift their attention. To address the first problem, we propose a geometry-aware temporal aggregation module to handle the egomotion explicitly. The effect of egomotion is mitigated by estimating the temporal geometry transformation and exploiting it to update visual representations. Moreover, we propose a cascaded feature enhancement module to tackle the second issue. It improves cross-modal localization robustness by disent"])</script><script>self.__next_f.push([1,"angling visually-indicated audio representation. During training, we take advantage of the naturally available audio-visual temporal synchronization as the ``free'' self-supervision to avoid costly labeling. We also annotate and create the Epic Sounding Object dataset for evaluation purposes. Extensive experiments show that our method achieves state-of-the-art localization performance in egocentric videos and can be generalized to diverse audio-visual scenes.25:T565,Humans naturally perceive surrounding scenes by unifying sound and sight in a first-person view. Likewise, machines are advanced to approach human intelligence by learning with multisensory inputs from an egocentric perspective. In this paper, we explore the challenging egocentric audio-visual object localization task and observe that 1) egomotion commonly exists in first-person recordings, even within a short duration; 2) The out-of-view sound components can be created while wearers shift their attention. To address the first problem, we propose a geometry-aware temporal aggregation module to handle the egomotion explicitly. The effect of egomotion is mitigated by estimating the temporal geometry transformation and exploiting it to update visual representations. Moreover, we propose a cascaded feature enhancement module to tackle the second issue. It improves cross-modal localization robustness by disentangling visually-indicated audio representation. During training, we take advantage of the naturally available audio-visual temporal synchronization as the ``free'' self-supervision to avoid costly labeling. We also annotate and create the Epic Sounding Object dataset for evaluation purposes. Extensive experiments show that our method achieves state-of-the-art localization performance in egocentric videos and can be generalized to diverse audio-visual scenes.26:T53e,Recent breakthroughs in text-guided image generation have led to remarkable progress in the field of 3D synthesis from text. By optimizing neural radiance fields (NeRF) directly from text,"])</script><script>self.__next_f.push([1," recent methods are able to produce remarkable results. Yet, these methods are limited in their control of each object's placement or appearance, as they represent the scene as a whole. This can be a major issue in scenarios that require refining or manipulating objects in the scene. To remedy this deficit, we propose a novel GlobalLocal training framework for synthesizing a 3D scene using object proxies. A proxy represents the object's placement in the generated scene and optionally defines its coarse geometry. The key to our approach is to represent each object as an independent NeRF. We alternate between optimizing each NeRF on its own and as part of the full scene. Thus, a complete representation of each object can be learned, while also creating a harmonious scene with style and lighting match. We show that using proxies allows a wide variety of editing options, such as adjusting the placement of each independent object, removing objects from a scene, or refining an object. Our results show that Set-the-Scene offers a powerful solution for scene synthesis and manipulation, filling a crucial gap in controllable text-to-3D synthesis.27:T53e,Recent breakthroughs in text-guided image generation have led to remarkable progress in the field of 3D synthesis from text. By optimizing neural radiance fields (NeRF) directly from text, recent methods are able to produce remarkable results. Yet, these methods are limited in their control of each object's placement or appearance, as they represent the scene as a whole. This can be a major issue in scenarios that require refining or manipulating objects in the scene. To remedy this deficit, we propose a novel GlobalLocal training framework for synthesizing a 3D scene using object proxies. A proxy represents the object's placement in the generated scene and optionally defines its coarse geometry. The key to our approach is to represent each object as an independent NeRF. We alternate between optimizing each NeRF on its own and as part of the full scene. Thus, a complete rep"])</script><script>self.__next_f.push([1,"resentation of each object can be learned, while also creating a harmonious scene with style and lighting match. We show that using proxies allows a wide variety of editing options, such as adjusting the placement of each independent object, removing objects from a scene, or refining an object. Our results show that Set-the-Scene offers a powerful solution for scene synthesis and manipulation, filling a crucial gap in controllable text-to-3D synthesis.28:T549,The field of vision and language has witnessed a proliferation of pre-trained foundation models. Most existing methods are independently pre-trained with contrastive objective like CLIP, image-to-text generative objective like PaLI, or text-to-image generative objective like Parti. However, the three objectives can be pre-trained on the same data, image-text pairs, and intuitively they complement each other as contrasting provides global alignment capacity and generation grants fine-grained understanding. In this work, we present a Contrastive Bi-directional Image-Text generation model (CoBIT), which attempts to unify the three pre-training objectives in one framework. Specifically, CoBIT employs a novel unicoder-decoder structure, consisting of an image unicoder, a text unicoder and a cross-modal decoder. The image/text unicoders can switch between encoding and decoding in different tasks, enabling flexibility and shared knowledge that benefits both image-to-text and text-to-image generations. CoBIT achieves superior performance in image understanding, image-text understanding (Retrieval, Captioning, VQA, SNLI-VE) and text-based content creation, particularly in zero-shot scenarios. For instance, 82.7% in zero-shot ImageNet classification, 9.37 FID score in zero-shot text-to-image generation and 44.8 CIDEr in zero-shot captioning.29:T549,The field of vision and language has witnessed a proliferation of pre-trained foundation models. Most existing methods are independently pre-trained with contrastive objective like CLIP, image-to-text generative objective l"])</script><script>self.__next_f.push([1,"ike PaLI, or text-to-image generative objective like Parti. However, the three objectives can be pre-trained on the same data, image-text pairs, and intuitively they complement each other as contrasting provides global alignment capacity and generation grants fine-grained understanding. In this work, we present a Contrastive Bi-directional Image-Text generation model (CoBIT), which attempts to unify the three pre-training objectives in one framework. Specifically, CoBIT employs a novel unicoder-decoder structure, consisting of an image unicoder, a text unicoder and a cross-modal decoder. The image/text unicoders can switch between encoding and decoding in different tasks, enabling flexibility and shared knowledge that benefits both image-to-text and text-to-image generations. CoBIT achieves superior performance in image understanding, image-text understanding (Retrieval, Captioning, VQA, SNLI-VE) and text-based content creation, particularly in zero-shot scenarios. For instance, 82.7% in zero-shot ImageNet classification, 9.37 FID score in zero-shot text-to-image generation and 44.8 CIDEr in zero-shot captioning.2a:T46e,Graph neural network (GNN) is a promising approach to learning and predicting physical phenomena described in boundary value problems, such as partial differential equations (PDEs) with boundary conditions. However, existing models inadequately treat boundary conditions essential for the reliable prediction of such problems. In addition, because of the locally connected nature of GNNs, it is difficult to accurately predict the state after a long time, where interaction between vertices tends to be global. We present our approach termed physics-embedded neural networks that considers boundary conditions and predicts the state after a long time using an implicit method. It is built based on an E(n)-equivariant GNN, resulting in high generalization performance on various shapes. We demonstrate that our model learns flow phenomena in complex shapes and outperforms a well-optimized classical solver and"])</script><script>self.__next_f.push([1," a state-of-the-art machine learning model in speed-accuracy trade-off. Therefore, our model can be a useful standard for realizing reliable, fast, and accurate GNN-based PDE solvers. The code is available at this https URL.2b:T46e,Graph neural network (GNN) is a promising approach to learning and predicting physical phenomena described in boundary value problems, such as partial differential equations (PDEs) with boundary conditions. However, existing models inadequately treat boundary conditions essential for the reliable prediction of such problems. In addition, because of the locally connected nature of GNNs, it is difficult to accurately predict the state after a long time, where interaction between vertices tends to be global. We present our approach termed physics-embedded neural networks that considers boundary conditions and predicts the state after a long time using an implicit method. It is built based on an E(n)-equivariant GNN, resulting in high generalization performance on various shapes. We demonstrate that our model learns flow phenomena in complex shapes and outperforms a well-optimized classical solver and a state-of-the-art machine learning model in speed-accuracy trade-off. Therefore, our model can be a useful standard for realizing reliable, fast, and accurate GNN-based PDE solvers. The code is available at this https URL.2c:T407,The coupling between a pseudo-scalar inflaton and a gauge field leads to an\namount of additional density perturbations and gravitational waves (GWs) that\nis strongly sensitive to the inflaton speed. This naturally results in enhanced\nGWs at (relatively) small scales that exited the horizon well after the CMB\nones, and that can be probed by a variety of GW observatories (from pulsar\ntiming arrays, to astrometry, to space-borne and ground-based interferometers).\nThis production occurs in a regime in which the gauge field significantly\nbackreacts on the inflaton motion. Contrary to earlier assumptions, it has been\nrecently shown that this regime is characterized by an "])</script><script>self.__next_f.push([1,"oscillatory behavior of\nthe inflaton speed, with a period of~${\\rm O } \\left( 5 \\right)$ e-folds.\nBursts of GWs are produced at the maxima of the speed, imprinting nearly\nperiodic bumps in the frequency-dependent spectrum of GWs produced during\ninflation. This can potentially generate correlated peaks appearing in the same\nor in different GWs experiments.2d:T407,The coupling between a pseudo-scalar inflaton and a gauge field leads to an\namount of additional density perturbations and gravitational waves (GWs) that\nis strongly sensitive to the inflaton speed. This naturally results in enhanced\nGWs at (relatively) small scales that exited the horizon well after the CMB\nones, and that can be probed by a variety of GW observatories (from pulsar\ntiming arrays, to astrometry, to space-borne and ground-based interferometers).\nThis production occurs in a regime in which the gauge field significantly\nbackreacts on the inflaton motion. Contrary to earlier assumptions, it has been\nrecently shown that this regime is characterized by an oscillatory behavior of\nthe inflaton speed, with a period of~${\\rm O } \\left( 5 \\right)$ e-folds.\nBursts of GWs are produced at the maxima of the speed, imprinting nearly\nperiodic bumps in the frequency-dependent spectrum of GWs produced during\ninflation. This can potentially generate correlated peaks appearing in the same\nor in different GWs experiments.2e:T41e,Symptom information is primarily documented in free-text clinical notes and is not directly accessible for downstream applications. To address this challenge, information extraction approaches that can handle clinical language variation across different institutions and specialties are needed. In this paper, we present domain generalization for symptom extraction using pretraining and fine-tuning data that differs from the target domain in terms of institution and/or specialty and patient population. We extract symptom events using a transformer-based joint entity and relation extraction method. To reduce reliance on domain-specific fea"])</script><script>self.__next_f.push([1,"tures, we propose a domain generalization method that dynamically masks frequent symptoms words in the source domain. Additionally, we pretrain the transformer language model (LM) on task-related unlabeled texts for better representation. Our experiments indicate that masking and adaptive pretraining methods can significantly improve performance when the source domain is more distant from the target domain.2f:T41e,Symptom information is primarily documented in free-text clinical notes and is not directly accessible for downstream applications. To address this challenge, information extraction approaches that can handle clinical language variation across different institutions and specialties are needed. In this paper, we present domain generalization for symptom extraction using pretraining and fine-tuning data that differs from the target domain in terms of institution and/or specialty and patient population. We extract symptom events using a transformer-based joint entity and relation extraction method. To reduce reliance on domain-specific features, we propose a domain generalization method that dynamically masks frequent symptoms words in the source domain. Additionally, we pretrain the transformer language model (LM) on task-related unlabeled texts for better representation. Our experiments indicate that masking and adaptive pretraining methods can significantly improve performance when the source domain is more distant from the target domain.30:T48c,Quantum information scrambling, typically explored in closed quantum systems, describes the spread of initially localized information throughout a system and can be quantified by measures such as the Loschmidt echo (LE) and out-of-time-order correlator (OTOC). In this paper, we explore information scrambling in the presence of dissipation by generalizing the concepts of LE and OTOC to open quantum systems governed by Lindblad dynamics. We investigate the universal dynamics of the generalized LE across regimes of weak and strong dissipation. In the weak dissipati"])</script><script>self.__next_f.push([1,"on regime, we identify a universal structure, while in the strong dissipation regime, we observe a distinctive two-local-minima structure, which we interpret through an analysis of the Lindblad spectrum. Furthermore, we establish connections between the thermal averages of LE and OTOC and prove a general relation between OTOC and Rényi entropy in open systems. Finally, we propose an experimental protocol for measuring OTOC in open systems. These findings provide deeper insights into information scrambling under dissipation and pave the way for experimental studies in open quantum systems.31:T48c,Quantum information scrambling, typically explored in closed quantum systems, describes the spread of initially localized information throughout a system and can be quantified by measures such as the Loschmidt echo (LE) and out-of-time-order correlator (OTOC). In this paper, we explore information scrambling in the presence of dissipation by generalizing the concepts of LE and OTOC to open quantum systems governed by Lindblad dynamics. We investigate the universal dynamics of the generalized LE across regimes of weak and strong dissipation. In the weak dissipation regime, we identify a universal structure, while in the strong dissipation regime, we observe a distinctive two-local-minima structure, which we interpret through an analysis of the Lindblad spectrum. Furthermore, we establish connections between the thermal averages of LE and OTOC and prove a general relation between OTOC and Rényi entropy in open systems. Finally, we propose an experimental protocol for measuring OTOC in open systems. These findings provide deeper insights into information scrambling under dissipation and pave the way for experimental studies in open quantum systems.32:T572,Learned sparse retrieval (LSR) is a family of first-stage retrieval methods that are trained to generate sparse lexical representations of queries and documents for use with an inverted index. Many LSR methods have been recently introduced, with Splade models achieving st"])</script><script>self.__next_f.push([1,"ate-of-the-art performance on MSMarco. Despite similarities in their model architectures, many LSR methods show substantial differences in effectiveness and efficiency. Differences in the experimental setups and configurations used make it difficult to compare the methods and derive insights. In this work, we analyze existing LSR methods and identify key components to establish an LSR framework that unifies all LSR methods under the same perspective. We then reproduce all prominent methods using a common codebase and re-train them in the same environment, which allows us to quantify how components of the framework affect effectiveness and efficiency. We find that (1) including document term weighting is most important for a method's effectiveness, (2) including query weighting has a small positive impact, and (3) document expansion and query expansion have a cancellation effect. As a result, we show how removing query expansion from a state-of-the-art model can reduce latency significantly while maintaining effectiveness on MSMarco and TripClick benchmarks. Our code is publicly available at this https URL33:T572,Learned sparse retrieval (LSR) is a family of first-stage retrieval methods that are trained to generate sparse lexical representations of queries and documents for use with an inverted index. Many LSR methods have been recently introduced, with Splade models achieving state-of-the-art performance on MSMarco. Despite similarities in their model architectures, many LSR methods show substantial differences in effectiveness and efficiency. Differences in the experimental setups and configurations used make it difficult to compare the methods and derive insights. In this work, we analyze existing LSR methods and identify key components to establish an LSR framework that unifies all LSR methods under the same perspective. We then reproduce all prominent methods using a common codebase and re-train them in the same environment, which allows us to quantify how components of the framework affect effectiveness and"])</script><script>self.__next_f.push([1," efficiency. We find that (1) including document term weighting is most important for a method's effectiveness, (2) including query weighting has a small positive impact, and (3) document expansion and query expansion have a cancellation effect. As a result, we show how removing query expansion from a state-of-the-art model can reduce latency significantly while maintaining effectiveness on MSMarco and TripClick benchmarks. Our code is publicly available at this https URL34:T4db,We investigate a symmetric logarithmic derivative (SLD) Fisher information\nfor kinetic uncertainty relations (KURs) of open quantum systems described by\nthe GKSL quantum master equation with and without the detailed balance\ncondition. In a quantum kinetic uncertainty relation derived by Vu and Saito\n[Phys. Rev. Lett. 128, 140602 (2022)], the Fisher information of probability of\nquantum trajectory with a time-rescaling parameter plays an essential role.\nThis Fisher information is upper bounded by the SLD Fisher information. For a\nfinite time and arbitrary initial state, we derive a concise expression of the\nSLD Fisher information, which is a double time integral and can be calculated\nby solving coupled first-order differential equations. We also derive a simple\nlower bound of the Fisher information of quantum trajectory. We point out that\nthe SLD Fisher information also appears in the speed limit based on the\nMandelstam-Tamm relation by Hasegawa [Nat. Commun. 14, 2828 (2023)]. When the\njump operators connect eigenstates of the system Hamiltonian, we show that the\nBures angle in the interaction picture is upper bounded by the square root of\nthe dynamical activity at short times, which contrasts with the classical\ncounterpart.35:T4db,We investigate a symmetric logarithmic derivative (SLD) Fisher information\nfor kinetic uncertainty relations (KURs) of open quantum systems described by\nthe GKSL quantum master equation with and without the detailed balance\ncondition. In a quantum kinetic uncertainty relation derived by Vu and Saito\n[Phys. Rev. "])</script><script>self.__next_f.push([1,"Lett. 128, 140602 (2022)], the Fisher information of probability of\nquantum trajectory with a time-rescaling parameter plays an essential role.\nThis Fisher information is upper bounded by the SLD Fisher information. For a\nfinite time and arbitrary initial state, we derive a concise expression of the\nSLD Fisher information, which is a double time integral and can be calculated\nby solving coupled first-order differential equations. We also derive a simple\nlower bound of the Fisher information of quantum trajectory. We point out that\nthe SLD Fisher information also appears in the speed limit based on the\nMandelstam-Tamm relation by Hasegawa [Nat. Commun. 14, 2828 (2023)]. When the\njump operators connect eigenstates of the system Hamiltonian, we show that the\nBures angle in the interaction picture is upper bounded by the square root of\nthe dynamical activity at short times, which contrasts with the classical\ncounterpart.36:T413,With recent advancements in computer vision as well as machine learning (ML),\nvideo-based at-home exercise evaluation systems have become a popular topic of\ncurrent research. However, performance depends heavily on the amount of\navailable training data. Since labeled datasets specific to exercising are\nrare, we propose a method that makes use of the abundance of fitness videos\navailable online. Specifically, we utilize the advantage that videos often not\nonly show the exercises, but also provide language as an additional source of\ninformation. With push-ups as an example, we show that through the analysis of\nsubtitle data using natural language processing (NLP), it is possible to create\na labeled (irrelevant, relevant correct, relevant incorrect) dataset containing\nrelevant information for pose analysis. In particular, we show that irrelevant\nclips ($n=332$) have significantly different joint visibility values compared\nto relevant clips ($n=298$). Inspecting cluster centroids also show different\nposes for the different classes.37:T413,With recent advancements in computer vision as well as mach"])</script><script>self.__next_f.push([1,"ine learning (ML),\nvideo-based at-home exercise evaluation systems have become a popular topic of\ncurrent research. However, performance depends heavily on the amount of\navailable training data. Since labeled datasets specific to exercising are\nrare, we propose a method that makes use of the abundance of fitness videos\navailable online. Specifically, we utilize the advantage that videos often not\nonly show the exercises, but also provide language as an additional source of\ninformation. With push-ups as an example, we show that through the analysis of\nsubtitle data using natural language processing (NLP), it is possible to create\na labeled (irrelevant, relevant correct, relevant incorrect) dataset containing\nrelevant information for pose analysis. In particular, we show that irrelevant\nclips ($n=332$) have significantly different joint visibility values compared\nto relevant clips ($n=298$). Inspecting cluster centroids also show different\nposes for the different classes.38:T544,Pixel binning based Quad sensors have emerged as a promising solution to overcome the hardware limitations of compact cameras in low-light imaging. However, binning results in lower spatial resolution and non-Bayer CFA artifacts. To address these challenges, we propose a dual-head joint remosaicing and denoising network (DJRD), which enables the conversion of noisy Quad Bayer and standard noise-free Bayer pattern without any resolution loss. DJRD includes a newly designed Quad Bayer remosaicing (QB-Re) block, integrated denoising modules based on Swin-transformer and multi-scale wavelet transform. The QB-Re block constructs the convolution kernel based on the CFA pattern to achieve a periodic color distribution in the perceptual field, which is used to extract exact spectral information and reduce color misalignment. The integrated Swin-Transformer and multi-scale wavelet transform capture non-local dependencies, frequency and location information to effectively reduce practical noise. By identifying challenging patches utilizing Moire and "])</script><script>self.__next_f.push([1,"zipper detection metrics, we enable our model to concentrate on difficult patches during the post-training phase, which enhances the model's performance in hard cases. Our proposed model outperforms competing models by approximately 3dB, without additional complexity in hardware or software.39:T544,Pixel binning based Quad sensors have emerged as a promising solution to overcome the hardware limitations of compact cameras in low-light imaging. However, binning results in lower spatial resolution and non-Bayer CFA artifacts. To address these challenges, we propose a dual-head joint remosaicing and denoising network (DJRD), which enables the conversion of noisy Quad Bayer and standard noise-free Bayer pattern without any resolution loss. DJRD includes a newly designed Quad Bayer remosaicing (QB-Re) block, integrated denoising modules based on Swin-transformer and multi-scale wavelet transform. The QB-Re block constructs the convolution kernel based on the CFA pattern to achieve a periodic color distribution in the perceptual field, which is used to extract exact spectral information and reduce color misalignment. The integrated Swin-Transformer and multi-scale wavelet transform capture non-local dependencies, frequency and location information to effectively reduce practical noise. By identifying challenging patches utilizing Moire and zipper detection metrics, we enable our model to concentrate on difficult patches during the post-training phase, which enhances the model's performance in hard cases. Our proposed model outperforms competing models by approximately 3dB, without additional complexity in hardware or software.3a:T657,Recently, vision transformers have shown great success in a set of human reconstruction tasks such as 2D human pose estimation (2D HPE), 3D human pose estimation (3D HPE), and human mesh reconstruction (HMR) tasks. In these tasks, feature map representations of the human structural information are often extracted first from the image by a CNN (such as HRNet), and then further processed by "])</script><script>self.__next_f.push([1,"transformer to predict the heatmaps (encodes each joint's location into a feature map with a Gaussian distribution) for HPE or HMR. However, existing transformer architectures are not able to process these feature map inputs directly, forcing an unnatural flattening of the location-sensitive human structural information. Furthermore, much of the performance benefit in recent HPE and HMR methods has come at the cost of ever-increasing computation and memory needs. Therefore, to simultaneously address these problems, we propose FeatER, a novel transformer design that preserves the inherent structure of feature map representations when modeling attention while reducing memory and computational costs. Taking advantage of FeatER, we build an efficient network for a set of human reconstruction tasks including 2D HPE, 3D HPE, and HMR. A feature map reconstruction module is applied to improve the performance of the estimated human pose and mesh. Extensive experiments demonstrate the effectiveness of FeatER on various human pose and mesh datasets. For instance, FeatER outperforms the SOTA method MeshGraphormer by requiring 5% of Params and 16% of MACs on Human3.6M and 3DPW datasets. The project webpage is this https URL.3b:T657,Recently, vision transformers have shown great success in a set of human reconstruction tasks such as 2D human pose estimation (2D HPE), 3D human pose estimation (3D HPE), and human mesh reconstruction (HMR) tasks. In these tasks, feature map representations of the human structural information are often extracted first from the image by a CNN (such as HRNet), and then further processed by transformer to predict the heatmaps (encodes each joint's location into a feature map with a Gaussian distribution) for HPE or HMR. However, existing transformer architectures are not able to process these feature map inputs directly, forcing an unnatural flattening of the location-sensitive human structural information. Furthermore, much of the performance benefit in recent HPE and HMR methods has come at the cos"])</script><script>self.__next_f.push([1,"t of ever-increasing computation and memory needs. Therefore, to simultaneously address these problems, we propose FeatER, a novel transformer design that preserves the inherent structure of feature map representations when modeling attention while reducing memory and computational costs. Taking advantage of FeatER, we build an efficient network for a set of human reconstruction tasks including 2D HPE, 3D HPE, and HMR. A feature map reconstruction module is applied to improve the performance of the estimated human pose and mesh. Extensive experiments demonstrate the effectiveness of FeatER on various human pose and mesh datasets. For instance, FeatER outperforms the SOTA method MeshGraphormer by requiring 5% of Params and 16% of MACs on Human3.6M and 3DPW datasets. The project webpage is this https URL.3c:T655,The segmentation and automatic identification of histological regions of diagnostic interest offer a valuable aid to pathologists. However, segmentation methods are hampered by the difficulty of obtaining pixel-level annotations, which are tedious and expensive to obtain for Whole-Slide images (WSI). To remedy this, weakly supervised methods have been developed to exploit the annotations directly available at the image level. However, to our knowledge, none of these techniques is adapted to deal with WSIs. In this paper, we propose WholeSIGHT, a weakly-supervised method, to simultaneously segment and classify WSIs of arbitrary shapes and sizes. Formally, WholeSIGHT first constructs a tissue-graph representation of the WSI, where the nodes and edges depict tissue regions and their interactions, respectively. During training, a graph classification head classifies the WSI and produces node-level pseudo labels via post-hoc feature attribution. These pseudo labels are then used to train a node classification head for WSI segmentation. During testing, both heads simultaneously render class prediction and segmentation for an input WSI. We evaluated WholeSIGHT on three public prostate cancer WSI datasets. Our meth"])</script><script>self.__next_f.push([1,"od achieved state-of-the-art weakly-supervised segmentation performance on all datasets while resulting in better or comparable classification with respect to state-of-the-art weakly-supervised WSI classification methods. Additionally, we quantify the generalization capability of our method in terms of segmentation and classification performance, uncertainty estimation, and model calibration.3d:T655,The segmentation and automatic identification of histological regions of diagnostic interest offer a valuable aid to pathologists. However, segmentation methods are hampered by the difficulty of obtaining pixel-level annotations, which are tedious and expensive to obtain for Whole-Slide images (WSI). To remedy this, weakly supervised methods have been developed to exploit the annotations directly available at the image level. However, to our knowledge, none of these techniques is adapted to deal with WSIs. In this paper, we propose WholeSIGHT, a weakly-supervised method, to simultaneously segment and classify WSIs of arbitrary shapes and sizes. Formally, WholeSIGHT first constructs a tissue-graph representation of the WSI, where the nodes and edges depict tissue regions and their interactions, respectively. During training, a graph classification head classifies the WSI and produces node-level pseudo labels via post-hoc feature attribution. These pseudo labels are then used to train a node classification head for WSI segmentation. During testing, both heads simultaneously render class prediction and segmentation for an input WSI. We evaluated WholeSIGHT on three public prostate cancer WSI datasets. Our method achieved state-of-the-art weakly-supervised segmentation performance on all datasets while resulting in better or comparable classification with respect to state-of-the-art weakly-supervised WSI classification methods. Additionally, we quantify the generalization capability of our method in terms of segmentation and classification performance, uncertainty estimation, and model calibration.3e:T620,Overparameteriza"])</script><script>self.__next_f.push([1,"tion in deep learning typically refers to settings where a trained neural network (NN) has representational capacity to fit the training data in many ways, some of which generalize well, while others do not. In the case of Recurrent Neural Networks (RNNs), there exists an additional layer of overparameterization, in the sense that a model may exhibit many solutions that generalize well for sequence lengths seen in training, some of which extrapolate to longer sequences, while others do not. Numerous works have studied the tendency of Gradient Descent (GD) to fit overparameterized NNs with solutions that generalize well. On the other hand, its tendency to fit overparameterized RNNs with solutions that extrapolate has been discovered only recently and is far less understood. In this paper, we analyze the extrapolation properties of GD when applied to overparameterized linear RNNs. In contrast to recent arguments suggesting an implicit bias towards short-term memory, we provide theoretical evidence for learning low-dimensional state spaces, which can also model long-term memory. Our result relies on a dynamical characterization which shows that GD (with small step size and near-zero initialization) strives to maintain a certain form of balancedness, as well as on tools developed in the context of the moment problem from statistics (recovery of a probability distribution from its moments). Experiments corroborate our theory, demonstrating extrapolation via learning low-dimensional state spaces with both linear and non-linear RNNs.3f:T620,Overparameterization in deep learning typically refers to settings where a trained neural network (NN) has representational capacity to fit the training data in many ways, some of which generalize well, while others do not. In the case of Recurrent Neural Networks (RNNs), there exists an additional layer of overparameterization, in the sense that a model may exhibit many solutions that generalize well for sequence lengths seen in training, some of which extrapolate to longer sequence"])</script><script>self.__next_f.push([1,"s, while others do not. Numerous works have studied the tendency of Gradient Descent (GD) to fit overparameterized NNs with solutions that generalize well. On the other hand, its tendency to fit overparameterized RNNs with solutions that extrapolate has been discovered only recently and is far less understood. In this paper, we analyze the extrapolation properties of GD when applied to overparameterized linear RNNs. In contrast to recent arguments suggesting an implicit bias towards short-term memory, we provide theoretical evidence for learning low-dimensional state spaces, which can also model long-term memory. Our result relies on a dynamical characterization which shows that GD (with small step size and near-zero initialization) strives to maintain a certain form of balancedness, as well as on tools developed in the context of the moment problem from statistics (recovery of a probability distribution from its moments). Experiments corroborate our theory, demonstrating extrapolation via learning low-dimensional state spaces with both linear and non-linear RNNs.40:T6c8,The goal of this work is to build flexible video-language models that can generalize to various video-to-text tasks from few examples, such as domain-specific captioning, question answering, and future event prediction. Existing few-shot video-language learners focus exclusively on the encoder, resulting in the absence of a video-to-text decoder to handle generative tasks. Video captioners have been pretrained on large-scale video-language datasets, but they rely heavily on finetuning and lack the ability to generate text for unseen tasks in a few-shot setting. We propose VidIL, a few-shot Video-language Learner via Image and Language models, which demonstrates strong performance on few-shot video-to-text tasks without the necessity of pretraining or finetuning on any video datasets. We use the image-language models to translate the video content into frame captions, object, attribute, and event phrases, and compose them into a temporal structure"])</script><script>self.__next_f.push([1," template. We then instruct a language model, with a prompt containing a few in-context examples, to generate a target output from the composed content. The flexibility of prompting allows the model to capture any form of text input, such as automatic speech recognition (ASR) transcripts. Our experiments demonstrate the power of language models in understanding videos on a wide variety of video-language tasks, including video captioning, video question answering, video caption retrieval, and video future event prediction. Especially, on video future event prediction, our few-shot model significantly outperforms state-of-the-art supervised models trained on large-scale video datasets. Code and resources are publicly available for research purposes at this https URL .41:T6c8,The goal of this work is to build flexible video-language models that can generalize to various video-to-text tasks from few examples, such as domain-specific captioning, question answering, and future event prediction. Existing few-shot video-language learners focus exclusively on the encoder, resulting in the absence of a video-to-text decoder to handle generative tasks. Video captioners have been pretrained on large-scale video-language datasets, but they rely heavily on finetuning and lack the ability to generate text for unseen tasks in a few-shot setting. We propose VidIL, a few-shot Video-language Learner via Image and Language models, which demonstrates strong performance on few-shot video-to-text tasks without the necessity of pretraining or finetuning on any video datasets. We use the image-language models to translate the video content into frame captions, object, attribute, and event phrases, and compose them into a temporal structure template. We then instruct a language model, with a prompt containing a few in-context examples, to generate a target output from the composed content. The flexibility of prompting allows the model to capture any form of text input, such as automatic speech recognition (ASR) transcripts. Our experiment"])</script><script>self.__next_f.push([1,"s demonstrate the power of language models in understanding videos on a wide variety of video-language tasks, including video captioning, video question answering, video caption retrieval, and video future event prediction. Especially, on video future event prediction, our few-shot model significantly outperforms state-of-the-art supervised models trained on large-scale video datasets. Code and resources are publicly available for research purposes at this https URL .42:T5ed,In the context of tomographic cosmic shear surveys, a theoretical model for the one-point statistics of the aperture mass (Map) is developed. This formalism is based on the application of the large deviation principle to the projected matter density field and more specifically to the angular aperture masses. The latter holds the advantage of being an observable that can be directly extracted from the observed shear field and to be, by construction, independent from the long wave modes. Furthermore we show that, with the help of a nulling procedure based on the so-called BNT transform, it is possible to build observables that depend only on a finite range of redshifts making them also independent from the small-scale modes. This procedure makes predictions for the shape of the one-point Probability Distribution Function of such an observable very accurate, comparable to what had been previously obtained for 3D observables. Comparisons with specific simulations reveal however inconsistent results showing that synthetic lensing maps were not accurate enough for such refined observables. It points to the need for more precise dedicated numerical developments whose performances could be benchmarked with such observables. We furthermore review the possible systematics that could affect such a formalism in future weak-lensing surveys like Euclid, notably the impact of shape noise as well as leading corrections coming from lens-lens couplings, geodesic deviation, reduced shear and magnification bias.43:T5ed,In the context of tomographic cosmic shear "])</script><script>self.__next_f.push([1,"surveys, a theoretical model for the one-point statistics of the aperture mass (Map) is developed. This formalism is based on the application of the large deviation principle to the projected matter density field and more specifically to the angular aperture masses. The latter holds the advantage of being an observable that can be directly extracted from the observed shear field and to be, by construction, independent from the long wave modes. Furthermore we show that, with the help of a nulling procedure based on the so-called BNT transform, it is possible to build observables that depend only on a finite range of redshifts making them also independent from the small-scale modes. This procedure makes predictions for the shape of the one-point Probability Distribution Function of such an observable very accurate, comparable to what had been previously obtained for 3D observables. Comparisons with specific simulations reveal however inconsistent results showing that synthetic lensing maps were not accurate enough for such refined observables. It points to the need for more precise dedicated numerical developments whose performances could be benchmarked with such observables. We furthermore review the possible systematics that could affect such a formalism in future weak-lensing surveys like Euclid, notably the impact of shape noise as well as leading corrections coming from lens-lens couplings, geodesic deviation, reduced shear and magnification bias.44:T586,Immigration is often blamed for increasing unemployment among local workers.\nThis sentiment is reflected in the rise of anti-immigration parties and\npolicies in Western democracies. And in fact, numerous studies estimate that in\nthe short run, the arrival of new workers in a labor market raises the\nunemployment rate of local workers. Yet, standard migration models, such as the\nWalrasian model and the Diamond-Mortensen-Pissarides model, inherently assume\nthat immigrants are absorbed into the labor market without affecting local\nunemployment. This paper presents"])</script><script>self.__next_f.push([1," a more general model of migration that allows\nfor the possibility that not only the wages but also the unemployment rate of\nlocal workers may be affected by the arrival of newcomers. This extension is\nessential to capture the full range of potential impacts of labor migration on\nlabor markets. The model blends a matching framework with job rationing. In it,\nthe arrival of new workers raises the unemployment rate among local workers,\nparticularly in a depressed labor market where job opportunities are limited.\nOn the positive side, in-migration helps firms fill vacancies more easily,\nboosting their profits. The overall impact of in-migration on local welfare\nvaries with labor market conditions: in-migration reduces welfare when the\nlabor market is inefficiently slack, but it enhances welfare when the labor\nmarket is inefficiently tight.45:T586,Immigration is often blamed for increasing unemployment among local workers.\nThis sentiment is reflected in the rise of anti-immigration parties and\npolicies in Western democracies. And in fact, numerous studies estimate that in\nthe short run, the arrival of new workers in a labor market raises the\nunemployment rate of local workers. Yet, standard migration models, such as the\nWalrasian model and the Diamond-Mortensen-Pissarides model, inherently assume\nthat immigrants are absorbed into the labor market without affecting local\nunemployment. This paper presents a more general model of migration that allows\nfor the possibility that not only the wages but also the unemployment rate of\nlocal workers may be affected by the arrival of newcomers. This extension is\nessential to capture the full range of potential impacts of labor migration on\nlabor markets. The model blends a matching framework with job rationing. In it,\nthe arrival of new workers raises the unemployment rate among local workers,\nparticularly in a depressed labor market where job opportunities are limited.\nOn the positive side, in-migration helps firms fill vacancies more easily,\nboosting their profits. The overall"])</script><script>self.__next_f.push([1," impact of in-migration on local welfare\nvaries with labor market conditions: in-migration reduces welfare when the\nlabor market is inefficiently slack, but it enhances welfare when the labor\nmarket is inefficiently tight.46:T573,This paper presents an implementation of radio astronomy imaging algorithms on modern High Performance Computing (HPC) infrastructures, exploiting distributed memory parallelism and acceleration throughout multiple GPUs. Our code, called RICK (Radio Imaging Code Kernels), is capable of performing the major steps of the w-stacking algorithm presented in Offringa et al. (2014) both inter- and intra-node, and in particular has the possibility to run entirely on the GPU memory, minimising the number of data transfers between CPU and GPU. This feature, especially among multiple GPUs, is critical given the huge sizes of radio datasets involved. After a detailed description of the new implementations of the code with respect to the first version presented in Gheller et al. (2023), we analyse the performances of the code for each step involved in its execution. We also discuss the pros and cons related to an accelerated approach to this problem and its impact on the overall behaviour of the code. Such approach to the problem results in a significant improvement in terms of runtime with respect to the CPU version of the code, as long as the amount of computational resources does not exceed the one requested by the size of the problem: the code, in fact, is now limited by the communication costs, with the computation that gets heavily reduced by the capabilities of the accelerators.47:T573,This paper presents an implementation of radio astronomy imaging algorithms on modern High Performance Computing (HPC) infrastructures, exploiting distributed memory parallelism and acceleration throughout multiple GPUs. Our code, called RICK (Radio Imaging Code Kernels), is capable of performing the major steps of the w-stacking algorithm presented in Offringa et al. (2014) both inter- and intra-node, and in pa"])</script><script>self.__next_f.push([1,"rticular has the possibility to run entirely on the GPU memory, minimising the number of data transfers between CPU and GPU. This feature, especially among multiple GPUs, is critical given the huge sizes of radio datasets involved. After a detailed description of the new implementations of the code with respect to the first version presented in Gheller et al. (2023), we analyse the performances of the code for each step involved in its execution. We also discuss the pros and cons related to an accelerated approach to this problem and its impact on the overall behaviour of the code. Such approach to the problem results in a significant improvement in terms of runtime with respect to the CPU version of the code, as long as the amount of computational resources does not exceed the one requested by the size of the problem: the code, in fact, is now limited by the communication costs, with the computation that gets heavily reduced by the capabilities of the accelerators.48:T655,Feature representation learning is the key recipe for learning-based\nMulti-View Stereo (MVS). As the common feature extractor of learning-based MVS,\nvanilla Feature Pyramid Networks (FPNs) suffer from discouraged feature\nrepresentations for reflection and texture-less areas, which limits the\ngeneralization of MVS. Even FPNs worked with pre-trained Convolutional Neural\nNetworks (CNNs) fail to tackle these issues. On the other hand, Vision\nTransformers (ViTs) have achieved prominent success in many 2D vision tasks.\nThus we ask whether ViTs can facilitate feature learning in MVS? In this paper,\nwe propose a pre-trained ViT enhanced MVS network called MVSFormer, which can\nlearn more reliable feature representations benefited by informative priors\nfrom ViT. The finetuned MVSFormer with hierarchical ViTs of efficient attention\nmechanisms can achieve prominent improvement based on FPNs. Besides, the\nalternative MVSFormer with frozen ViT weights is further proposed. This largely\nalleviates the training cost with competitive performance strengthened by "])</script><script>self.__next_f.push([1,"the\nattention map from the self-distillation pre-training. MVSFormer can be\ngeneralized to various input resolutions with efficient multi-scale training\nstrengthened by gradient accumulation. Moreover, we discuss the merits and\ndrawbacks of classification and regression-based MVS methods, and further\npropose to unify them with a temperature-based strategy. MVSFormer achieves\nstate-of-the-art performance on the DTU dataset. Particularly, MVSFormer ranks\nas Top-1 on both intermediate and advanced sets of the highly competitive\nTanks-and-Temples leaderboard.49:T655,Feature representation learning is the key recipe for learning-based\nMulti-View Stereo (MVS). As the common feature extractor of learning-based MVS,\nvanilla Feature Pyramid Networks (FPNs) suffer from discouraged feature\nrepresentations for reflection and texture-less areas, which limits the\ngeneralization of MVS. Even FPNs worked with pre-trained Convolutional Neural\nNetworks (CNNs) fail to tackle these issues. On the other hand, Vision\nTransformers (ViTs) have achieved prominent success in many 2D vision tasks.\nThus we ask whether ViTs can facilitate feature learning in MVS? In this paper,\nwe propose a pre-trained ViT enhanced MVS network called MVSFormer, which can\nlearn more reliable feature representations benefited by informative priors\nfrom ViT. The finetuned MVSFormer with hierarchical ViTs of efficient attention\nmechanisms can achieve prominent improvement based on FPNs. Besides, the\nalternative MVSFormer with frozen ViT weights is further proposed. This largely\nalleviates the training cost with competitive performance strengthened by the\nattention map from the self-distillation pre-training. MVSFormer can be\ngeneralized to various input resolutions with efficient multi-scale training\nstrengthened by gradient accumulation. Moreover, we discuss the merits and\ndrawbacks of classification and regression-based MVS methods, and further\npropose to unify them with a temperature-based strategy. MVSFormer achieves\nstate-of-the-art performance on the DTU d"])</script><script>self.__next_f.push([1,"ataset. Particularly, MVSFormer ranks\nas Top-1 on both intermediate and advanced sets of the highly competitive\nTanks-and-Temples leaderboard.4a:T519,A recent series of theoretical works showed that the dynamics of neural networks with a certain initialisation are well-captured by kernel methods. Concurrent empirical work demonstrated that kernel methods can come close to the performance of neural networks on some image classification tasks. These results raise the question of whether neural networks only learn successfully if kernels also learn successfully, despite neural networks being more expressive. Here, we show theoretically that two-layer neural networks (2LNN) with only a few hidden neurons can beat the performance of kernel learning on a simple Gaussian mixture classification task. We study the high-dimensional limit where the number of samples is linearly proportional to the input dimension, and show that while small 2LNN achieve near-optimal performance on this task, lazy training approaches such as random features and kernel methods do not. Our analysis is based on the derivation of a closed set of equations that track the learning dynamics of the 2LNN and thus allow to extract the asymptotic performance of the network as a function of signal-to-noise ratio and other hyperparameters. We finally illustrate how over-parametrising the neural network leads to faster convergence, but does not improve its final performance.4b:T519,A recent series of theoretical works showed that the dynamics of neural networks with a certain initialisation are well-captured by kernel methods. Concurrent empirical work demonstrated that kernel methods can come close to the performance of neural networks on some image classification tasks. These results raise the question of whether neural networks only learn successfully if kernels also learn successfully, despite neural networks being more expressive. Here, we show theoretically that two-layer neural networks (2LNN) with only a few hidden neurons can beat the performance "])</script><script>self.__next_f.push([1,"of kernel learning on a simple Gaussian mixture classification task. We study the high-dimensional limit where the number of samples is linearly proportional to the input dimension, and show that while small 2LNN achieve near-optimal performance on this task, lazy training approaches such as random features and kernel methods do not. Our analysis is based on the derivation of a closed set of equations that track the learning dynamics of the 2LNN and thus allow to extract the asymptotic performance of the network as a function of signal-to-noise ratio and other hyperparameters. We finally illustrate how over-parametrising the neural network leads to faster convergence, but does not improve its final performance.4c:T3d2c,"])</script><script>self.__next_f.push([1,"{\"folders\":[],\"unorganizedPapers\":[{\"link\":\"1109.3041v2\",\"title\":\"Asymptotic analysis of the stochastic block model for modular networks\\n and its algorithmic applications\"},{\"link\":\"1511.02476v5\",\"title\":\"Statistical physics of inference: Thresholds and algorithms\"},{\"link\":\"1109.4424v4\",\"title\":\"Statistical physics-based reconstruction in compressed sensing\"},{\"link\":\"arXiv:1909.11500v4\",\"title\":\"Modelling the influence of data structure on learning in neural networks: the hidden manifold model\"},{\"link\":\"1708.03395v3\",\"title\":\"Optimal Errors and Phase Transitions in High-Dimensional Generalized Linear Models\"},{\"link\":\"1907.00657v2\",\"title\":\"Optical Reservoir Computing using multiple light scattering for chaotic systems prediction\"},{\"link\":\"1701.00858v3\",\"title\":\"Constrained Low-rank Matrix Estimation: Phase Transitions, Approximate Message Passing and Applications\"},{\"link\":\"0901.2130v2\",\"title\":\"Hiding Quiet Solutions in Random Constraint Satisfaction Problems\"},{\"link\":\"1406.1880v2\",\"title\":\"Spectral Clustering of Graphs with the Bethe Hessian\"},{\"link\":\"1402.1298v3\",\"title\":\"Phase transitions and sample complexity in Bayes-optimal matrix factorization\"},{\"link\":\"1510.06664v2\",\"title\":\"Random Projections through multiple optical scattering: Approximating kernels at the speed of light\"},{\"link\":\"1906.08632v2\",\"title\":\"Dynamics of stochastic gradient descent for two-layer neural networks in the teacher-student setup\"},{\"link\":\"2002.09339v2\",\"title\":\"Generalisation error in learning with random features and the hidden manifold model\"},{\"link\":\"2006.14709v3\",\"title\":\"The Gaussian equivalence of generative models for learning with shallow neural networks\"},{\"link\":\"1603.08447v1\",\"title\":\"Mutual Information in Rank-One Matrix Estimation\"},{\"link\":\"1306.5550v2\",\"title\":\"Spectral redemption: clustering sparse networks\"},{\"link\":\"1701.08010v2\",\"title\":\"Statistical and computational phase transitions in spiked tensor estimation\"},{\"link\":\"1507.04113v1\",\"title\":\"Spectral Detection on Sparse Hypergraphs\"},{\"link\":\"cond-mat_0606180v2\",\"title\":\"Temperature and Disorder Chaos in Three-Dimensional Ising Spin Glasses\"},{\"link\":\"1503.08040v4\",\"title\":\"Approximate message-passing decoder and capacity-achieving sparse superposition codes\"},{\"link\":\"1506.02914v2\",\"title\":\"Training Restricted Boltzmann Machines via the Thouless-Anderson-Palmer Free Energy\"},{\"link\":\"0911.1551v2\",\"title\":\"Elusive Glassy Phase in the Random Field Ising Model\"},{\"link\":\"1003.2748v1\",\"title\":\"Generalization of the cavity method for adiabatic evolution of Gibbs states\"},{\"link\":\"0807.2553v2\",\"title\":\"On the path integral representation for quantum spin models and its application to the quantum cavity method and to Monte Carlo simulations\"},{\"link\":\"cond-mat_0212070v2\",\"title\":\"Energy exponents and corrections to scaling in Ising spin glasses\"},{\"link\":\"1701.05823v2\",\"title\":\"Mutual Information and Optimality of Approximate Message-Passing in Random Linear Estimation\"},{\"link\":\"1607.02335v2\",\"title\":\"The Mutual Information in Random Linear Estimation\"},{\"link\":\"2006.06098v2\",\"title\":\"Dynamical mean-field theory for stochastic gradient descent in Gaussian mixture classification\"},{\"link\":\"2105.15004v2\",\"title\":\"Generalization Error Rates in Kernel Regression: The Crossover from the Noiseless to Noisy Regime\"},{\"link\":\"1905.12385v2\",\"title\":\"The spiked matrix model with generative priors\"},{\"link\":\"2102.11742v2\",\"title\":\"Classifying high-dimensional Gaussian mixtures: Where kernel methods fail and neural networks succeed\"},{\"link\":\"cond-mat_0002055v2\",\"title\":\"Spin and link overlaps in 3-dimensional spin glasses\"},{\"link\":\"1701.06981v1\",\"title\":\"Multi-Layer Generalized Linear Estimation\"},{\"link\":\"1801.01593v2\",\"title\":\"Estimation in the spiked Wigner model: A short proof of the replica formula\"},{\"link\":\"2006.06560v2\",\"title\":\"Generalization error in high-dimensional perceptrons: Approaching Bayes error with convex optimization\"},{\"link\":\"cond-mat_0107366v2\",\"title\":\"Zero-temperature responses of a 3D spin glass in a field\"},{\"link\":\"2006.06581v6\",\"title\":\"Asymptotic Errors for Teacher-Student Convex Generalized Linear Models (or : How to Prove Kabashima's Replica Formula)\"},{\"link\":\"1312.1740v5\",\"title\":\"Approximate message-passing with spatially coupled structured operators, with applications to compressed sensing and sparse superposition codes\"},{\"link\":\"1806.09588v1\",\"title\":\"Fundamental limits of detection in the spiked Wigner model\"},{\"link\":\"1907.08226v3\",\"title\":\"Who is Afraid of Big Bad Minima? Analysis of Gradient-Flow in a Spiked Matrix-Tensor Model\"},{\"link\":\"1306.4121v2\",\"title\":\"The hard-core model on random graphs revisited\"},{\"link\":\"0910.3008v2\",\"title\":\"Fragility and hysteretic creep in frictional granular jamming\"},{\"link\":\"1609.05204v3\",\"title\":\"Scaling up Echo-State Networks with multiple light scattering\"},{\"link\":\"2006.05228v2\",\"title\":\"Phase retrieval in high dimensions: Statistical and computational phase transitions\"},{\"link\":\"1211.2379v2\",\"title\":\"Belief Propagation Reconstruction for Discrete Tomography\"},{\"link\":\"2002.04372v1\",\"title\":\"Asymptotic errors for convex penalized linear regression beyond Gaussian matrices\"},{\"link\":\"1301.5898v1\",\"title\":\"Phase Diagram and Approximate Message Passing for Blind Calibration and Dictionary Learning\"},{\"link\":\"1610.02918v1\",\"title\":\"Phase transitions and optimal algorithms in high-dimensional Gaussian mixture clustering\"},{\"link\":\"0909.3820v3\",\"title\":\"Following Gibbs States Adiabatically - The Energy Landscape of Mean Field Glassy Systems\"},{\"link\":\"1702.03260v3\",\"title\":\"A Deterministic and Generalized Framework for Unsupervised Learning with Restricted Boltzmann Machines\"},{\"link\":\"0805.3509v2\",\"title\":\"A Lattice Model for Colloidal Gels and Glasses\"},{\"link\":\"1207.2328v2\",\"title\":\"Comparative Study for Inference of Hidden Classes in Stochastic Block Models\"},{\"link\":\"0902.4185v3\",\"title\":\"Quiet Planting in the Locked Constraint Satisfaction Problems\"},{\"link\":\"2106.03791v2\",\"title\":\"Learning Gaussian Mixtures with Generalised Linear Models: Precise Asymptotics in High-dimensions\"},{\"link\":\"1510.01098v2\",\"title\":\"Intensity-only optical compressive imaging using a multiply scattering material and a double phase retrieval approach\"},{\"link\":\"2110.08775v3\",\"title\":\"Perturbative construction of mean-field equations in extensive-rank matrix factorization and denoising\"},{\"link\":\"2102.08127v3\",\"title\":\"Learning curves of generic features maps for realistic datasets with a teacher-student model\"},{\"link\":\"1006.2479v2\",\"title\":\"Glassy dynamics as a melting process (On melting dynamics and the glass transition, Part II)\"},{\"link\":\"2202.00293v4\",\"title\":\"Phase diagram of Stochastic Gradient Descent in high-dimensional two-layer neural networks\"},{\"link\":\"cond-mat_0403053v3\",\"title\":\"Spin glass models with ferromagnetically biased couplings on the Bethe lattice: analytic solutions and numerical simulations\"},{\"link\":\"2006.07310v2\",\"title\":\"Reservoir Computing meets Recurrent Kernels and Structured Transforms\"},{\"link\":\"1203.5521v3\",\"title\":\"Reweighted belief propagation and quiet planting for random K-SAT\"},{\"link\":\"2305.18270v3\",\"title\":\"How Two-Layer Neural Networks Learn, One (Giant) Step at a Time\"},{\"link\":\"2302.00375v2\",\"title\":\"Bayes-optimal Learning of Deep Random Networks of Extensive-width\"},{\"link\":\"1812.02537v1\",\"title\":\"Rank-one matrix estimation: analysis of algorithmic and information theoretic limits by the spatial coupling method\"},{\"link\":\"2006.06997v1\",\"title\":\"Complex Dynamics in Simple Neural Networks: Understanding Gradient Flow in Phase Retrieval\"},{\"link\":\"1404.7787v1\",\"title\":\"Spectral density of the non-backtracking operator\"},{\"link\":\"1006.2480v2\",\"title\":\"Glassy aspects of melting dynamics (On melting dynamics and the glass transition, Part I)\"},{\"link\":\"1008.4497v1\",\"title\":\"No spin glass phase in ferromagnetic random-field random-temperature scalar Ginzburg-Landau model\"},{\"link\":\"2210.06591v3\",\"title\":\"Rigorous dynamical mean field theory for stochastic gradient descent methods\"},{\"link\":\"1710.02903v1\",\"title\":\"Finite Size Corrections and Likelihood Ratio Fluctuations in the Spiked Wigner Model\"},{\"link\":\"1207.2079v1\",\"title\":\"Compressed Sensing of Approximately-Sparse Signals: Phase Transitions and Optimal Reconstruction\"},{\"link\":\"1203.3166v2\",\"title\":\"On the relation between kinetically constrained models of glass dynamics and the random first-order transition theory\"},{\"link\":\"2012.06373v1\",\"title\":\"Hardware Beyond Backpropagation: a Photonic Co-Processor for Direct Feedback Alignment\"},{\"link\":\"1604.02475v2\",\"title\":\"Performance Limits for Noisy Multi-Measurement Vector Problems\"},{\"link\":\"1506.03498v3\",\"title\":\"Matrix Completion from Fewer Entries: Spectral Detectability and Rank Estimation\"},{\"link\":\"2302.08923v1\",\"title\":\"Are Gaussian data all you need? Extents and limits of universality in high-dimensional generalized linear estimation\"},{\"link\":\"2302.08933v1\",\"title\":\"Universality laws for Gaussian mixtures in generalized linear models\"},{\"link\":\"1301.0901v1\",\"title\":\"Compressed Sensing under Matrix Uncertainty: Optimum Thresholds and Robust Approximate Message Passing\"},{\"link\":\"1809.06304v1\",\"title\":\"Approximate message-passing for convex optimization with non-separable penalties\"},{\"link\":\"1605.07516v1\",\"title\":\"Robust phase retrieval with the swept approximate message passing (prSAMP) algorithm\"},{\"link\":\"1302.0189v1\",\"title\":\"Non-adaptive pooling strategies for detection of rare faulty items\"},{\"link\":\"cond-mat_0512309v3\",\"title\":\"Aging, memory and rejuvenation: some lessons from simple models\"},{\"link\":\"1601.06683v2\",\"title\":\"Clustering from Sparse Pairwise Measurements\"},{\"link\":\"2203.07752v1\",\"title\":\"Optimal denoising of rotationally invariant rectangular matrices\"},{\"link\":\"2308.14085v1\",\"title\":\"Sampling with flows, diffusion and autoregressive neural networks: A spin-glass perspective\"},{\"link\":\"0910.5644v1\",\"title\":\"Quantum Annealing of Hard Problems\"},{\"link\":\"1810.13038v1\",\"title\":\"Spectral Method for Multiplexed Phase Retrieval and Application in Optical Imaging in Complex Media\"},{\"link\":\"2402.03220v3\",\"title\":\"The Benefits of Reusing Batches for Gradient Descent in Two-Layer Networks: Breaking the Curse of Information and Leap Exponents\"},{\"link\":\"1204.3734v1\",\"title\":\"Following states in temperature in the spherical s+p-spin glass model\"},{\"link\":\"2210.12760v4\",\"title\":\"On double-descent in uncertainty quantification in overparametrized models\"},{\"link\":\"1101.5863v1\",\"title\":\"Random-field p-spin glass model on regular random graphs\"},{\"link\":\"2208.05918v1\",\"title\":\"Low-rank Matrix Estimation with Inhomogeneous Noise\"},{\"link\":\"1901.09085v1\",\"title\":\"Generalisation dynamics of online learning in over-parameterised neural networks\"},{\"link\":\"cond-mat_0211106v2\",\"title\":\"Hiking through glassy phases: physics beyond aging\"},{\"link\":\"1906.04735v1\",\"title\":\"On the Universality of Noiseless Linear Estimation with Respect to the Measurement Matrix\"},{\"link\":\"2107.11814v1\",\"title\":\"LightOn Optical Processing Unit: Scaling-up AI and HPC with a Non von Neumann co-processor\"},{\"link\":\"1706.00705v1\",\"title\":\"Streaming Bayesian inference: theoretical limits and mini-batch approximate message-passing\"},{\"link\":\"1605.06422v3\",\"title\":\"Fast Randomized Semi-Supervised Clustering\"},{\"link\":\"2202.03295v2\",\"title\":\"Theoretical characterization of uncertainty in high-dimensional linear classification\"},{\"link\":\"cond-mat_0208566v2\",\"title\":\"Absence of an equilibrium ferromagnetic spin glass phase in three dimensions\"},{\"link\":\"2302.05882v1\",\"title\":\"From high-dimensional \u0026 mean-field dynamics to dimensionless ODEs: A unifying approach to SGD in two-layers networks\"},{\"link\":\"1307.7846v3\",\"title\":\"Belief-Propagation Guided Monte-Carlo Sampling\"},{\"link\":\"2302.06665v1\",\"title\":\"Optimal Algorithms for the Inhomogeneous Spiked Wigner Model\"},{\"link\":\"2310.14055v1\",\"title\":\"Spectral Phase Transitions in Non-Linear Wigner Spiked Models\"},{\"link\":\"0709.0894v1\",\"title\":\"Comment on \\\"Ultrametricity in the Edwards-Anderson Model\\\"\"},{\"link\":\"1602.02944v2\",\"title\":\"Fast phase retrieval for high dimensions: A block-based approach\"},{\"link\":\"2402.04980v2\",\"title\":\"Asymptotics of feature learning in two-layer networks after one gradient-step\"},{\"link\":\"2310.03575v2\",\"title\":\"Analysis of learning a flow-based generative model from limited sample complexity\"},{\"link\":\"2006.01475v2\",\"title\":\"Light-in-the-loop: using a photonics co-processor for scalable training of neural networks\"},{\"link\":\"1304.6599v2\",\"title\":\"Robust error correction for real-valued signals via message-passing decoding and spatial coupling\"},{\"link\":\"2303.02644v2\",\"title\":\"Expectation consistency for calibration of neural networks\"},{\"link\":\"2402.03902v1\",\"title\":\"A phase transition between positional and semantic learning in a solvable model of dot-product attention\"},{\"link\":\"0709.1023v1\",\"title\":\"Constraint optimization and landscapes\"},{\"link\":\"1609.08269v1\",\"title\":\"Spectral Bounds for the Ising Ferromagnet on an Arbitrary Given Graph\"},{\"link\":\"2310.02850v2\",\"title\":\"On the Atypical Solutions of the Symmetric Binary Perceptron\"},{\"link\":\"cond-mat_0409448v2\",\"title\":\"How many colors to color a random graph? Cavity, Complexity, Stability and all that\"},{\"link\":\"2402.13622v1\",\"title\":\"Analysis of Bootstrap and Subsampling in High-dimensional Regularized Regression\"},{\"link\":\"2201.09986v3\",\"title\":\"Bayesian Inference with Nonlinear Generative Models: Comments on Secure Learning\"},{\"link\":\"2405.15459v1\",\"title\":\"Repetita Iuvant: Data Repetition Allows SGD to Learn High-Dimensional Multi-Index Functions\"},{\"link\":\"2205.13527v2\",\"title\":\"Subspace clustering in high-dimensions: Phase transitions \u0026 Statistical-to-Computational gap\"},{\"link\":\"1511.05860v2\",\"title\":\"Scampi: a robust approximate message-passing framework for compressive imaging\"},{\"link\":\"2101.02115v1\",\"title\":\"Adversarial Robustness by Design through Analog Computing and Synthetic Gradients\"},{\"link\":\"2306.09283v1\",\"title\":\"Estimating rank-one matrices with mismatched prior and noise: universality and large deviations\"},{\"link\":\"2405.15480v2\",\"title\":\"Fundamental computational limits of weak learnability in high-dimensional multi-index models\"},{\"link\":\"2406.02157v1\",\"title\":\"Online Learning and Information Exponents: On The Importance of Batch size, and Time/Complexity Tradeoffs\"},{\"link\":\"2405.04267v2\",\"title\":\"Quenches in the Sherrington-Kirkpatrick model\"},{\"link\":\"2205.08782v1\",\"title\":\"Secure Coding via Gaussian Random Fields\"},{\"link\":\"2408.03733v1\",\"title\":\"Bayes-optimal learning of an extensive-width neural network from\\n quadratically many samples\"},{\"link\":\"2205.13503v2\",\"title\":\"Multi-layer State Evolution Under Random Convolutional Design\"},{\"link\":\"2403.04234v1\",\"title\":\"Fundamental limits of Non-Linear Low-Rank Matrix Estimation\"},{\"link\":\"1910.00285v2\",\"title\":\"Blind calibration for compressed sensing: State evolution and an online algorithm\"},{\"link\":\"2402.05674v2\",\"title\":\"A High Dimensional Statistical Model for Adversarial Training: Geometry and Trade-Offs\"},{\"link\":\"1104.0921v1\",\"title\":\"The nature of the different zero-temperature phases in discrete two-dimensional spin glasses: Entropy, universality, chaos and cascades in the renormalization group flow\"},{\"link\":\"0710.3336v2\",\"title\":\"Potts Glass on Random Graphs\"},{\"link\":\"1502.03324v1\",\"title\":\"Reference-less measurement of the transmission matrix of a highly scattering material using a DMD and phase retrieval techniques\"},{\"link\":\"1205.4200v4\",\"title\":\"Ultrametric probe of the spin-glass state in a field\"}]}"])</script><script>self.__next_f.push([1,"4d:T3d2c,"])</script><script>self.__next_f.push([1,"{\"folders\":[],\"unorganizedPapers\":[{\"link\":\"1109.3041v2\",\"title\":\"Asymptotic analysis of the stochastic block model for modular networks\\n and its algorithmic applications\"},{\"link\":\"1511.02476v5\",\"title\":\"Statistical physics of inference: Thresholds and algorithms\"},{\"link\":\"1109.4424v4\",\"title\":\"Statistical physics-based reconstruction in compressed sensing\"},{\"link\":\"arXiv:1909.11500v4\",\"title\":\"Modelling the influence of data structure on learning in neural networks: the hidden manifold model\"},{\"link\":\"1708.03395v3\",\"title\":\"Optimal Errors and Phase Transitions in High-Dimensional Generalized Linear Models\"},{\"link\":\"1907.00657v2\",\"title\":\"Optical Reservoir Computing using multiple light scattering for chaotic systems prediction\"},{\"link\":\"1701.00858v3\",\"title\":\"Constrained Low-rank Matrix Estimation: Phase Transitions, Approximate Message Passing and Applications\"},{\"link\":\"0901.2130v2\",\"title\":\"Hiding Quiet Solutions in Random Constraint Satisfaction Problems\"},{\"link\":\"1406.1880v2\",\"title\":\"Spectral Clustering of Graphs with the Bethe Hessian\"},{\"link\":\"1402.1298v3\",\"title\":\"Phase transitions and sample complexity in Bayes-optimal matrix factorization\"},{\"link\":\"1510.06664v2\",\"title\":\"Random Projections through multiple optical scattering: Approximating kernels at the speed of light\"},{\"link\":\"1906.08632v2\",\"title\":\"Dynamics of stochastic gradient descent for two-layer neural networks in the teacher-student setup\"},{\"link\":\"2002.09339v2\",\"title\":\"Generalisation error in learning with random features and the hidden manifold model\"},{\"link\":\"2006.14709v3\",\"title\":\"The Gaussian equivalence of generative models for learning with shallow neural networks\"},{\"link\":\"1603.08447v1\",\"title\":\"Mutual Information in Rank-One Matrix Estimation\"},{\"link\":\"1306.5550v2\",\"title\":\"Spectral redemption: clustering sparse networks\"},{\"link\":\"1701.08010v2\",\"title\":\"Statistical and computational phase transitions in spiked tensor estimation\"},{\"link\":\"1507.04113v1\",\"title\":\"Spectral Detection on Sparse Hypergraphs\"},{\"link\":\"cond-mat_0606180v2\",\"title\":\"Temperature and Disorder Chaos in Three-Dimensional Ising Spin Glasses\"},{\"link\":\"1503.08040v4\",\"title\":\"Approximate message-passing decoder and capacity-achieving sparse superposition codes\"},{\"link\":\"1506.02914v2\",\"title\":\"Training Restricted Boltzmann Machines via the Thouless-Anderson-Palmer Free Energy\"},{\"link\":\"0911.1551v2\",\"title\":\"Elusive Glassy Phase in the Random Field Ising Model\"},{\"link\":\"1003.2748v1\",\"title\":\"Generalization of the cavity method for adiabatic evolution of Gibbs states\"},{\"link\":\"0807.2553v2\",\"title\":\"On the path integral representation for quantum spin models and its application to the quantum cavity method and to Monte Carlo simulations\"},{\"link\":\"cond-mat_0212070v2\",\"title\":\"Energy exponents and corrections to scaling in Ising spin glasses\"},{\"link\":\"1701.05823v2\",\"title\":\"Mutual Information and Optimality of Approximate Message-Passing in Random Linear Estimation\"},{\"link\":\"1607.02335v2\",\"title\":\"The Mutual Information in Random Linear Estimation\"},{\"link\":\"2006.06098v2\",\"title\":\"Dynamical mean-field theory for stochastic gradient descent in Gaussian mixture classification\"},{\"link\":\"2105.15004v2\",\"title\":\"Generalization Error Rates in Kernel Regression: The Crossover from the Noiseless to Noisy Regime\"},{\"link\":\"1905.12385v2\",\"title\":\"The spiked matrix model with generative priors\"},{\"link\":\"2102.11742v2\",\"title\":\"Classifying high-dimensional Gaussian mixtures: Where kernel methods fail and neural networks succeed\"},{\"link\":\"cond-mat_0002055v2\",\"title\":\"Spin and link overlaps in 3-dimensional spin glasses\"},{\"link\":\"1701.06981v1\",\"title\":\"Multi-Layer Generalized Linear Estimation\"},{\"link\":\"1801.01593v2\",\"title\":\"Estimation in the spiked Wigner model: A short proof of the replica formula\"},{\"link\":\"2006.06560v2\",\"title\":\"Generalization error in high-dimensional perceptrons: Approaching Bayes error with convex optimization\"},{\"link\":\"cond-mat_0107366v2\",\"title\":\"Zero-temperature responses of a 3D spin glass in a field\"},{\"link\":\"2006.06581v6\",\"title\":\"Asymptotic Errors for Teacher-Student Convex Generalized Linear Models (or : How to Prove Kabashima's Replica Formula)\"},{\"link\":\"1312.1740v5\",\"title\":\"Approximate message-passing with spatially coupled structured operators, with applications to compressed sensing and sparse superposition codes\"},{\"link\":\"1806.09588v1\",\"title\":\"Fundamental limits of detection in the spiked Wigner model\"},{\"link\":\"1907.08226v3\",\"title\":\"Who is Afraid of Big Bad Minima? Analysis of Gradient-Flow in a Spiked Matrix-Tensor Model\"},{\"link\":\"1306.4121v2\",\"title\":\"The hard-core model on random graphs revisited\"},{\"link\":\"0910.3008v2\",\"title\":\"Fragility and hysteretic creep in frictional granular jamming\"},{\"link\":\"1609.05204v3\",\"title\":\"Scaling up Echo-State Networks with multiple light scattering\"},{\"link\":\"2006.05228v2\",\"title\":\"Phase retrieval in high dimensions: Statistical and computational phase transitions\"},{\"link\":\"1211.2379v2\",\"title\":\"Belief Propagation Reconstruction for Discrete Tomography\"},{\"link\":\"2002.04372v1\",\"title\":\"Asymptotic errors for convex penalized linear regression beyond Gaussian matrices\"},{\"link\":\"1301.5898v1\",\"title\":\"Phase Diagram and Approximate Message Passing for Blind Calibration and Dictionary Learning\"},{\"link\":\"1610.02918v1\",\"title\":\"Phase transitions and optimal algorithms in high-dimensional Gaussian mixture clustering\"},{\"link\":\"0909.3820v3\",\"title\":\"Following Gibbs States Adiabatically - The Energy Landscape of Mean Field Glassy Systems\"},{\"link\":\"1702.03260v3\",\"title\":\"A Deterministic and Generalized Framework for Unsupervised Learning with Restricted Boltzmann Machines\"},{\"link\":\"0805.3509v2\",\"title\":\"A Lattice Model for Colloidal Gels and Glasses\"},{\"link\":\"1207.2328v2\",\"title\":\"Comparative Study for Inference of Hidden Classes in Stochastic Block Models\"},{\"link\":\"0902.4185v3\",\"title\":\"Quiet Planting in the Locked Constraint Satisfaction Problems\"},{\"link\":\"2106.03791v2\",\"title\":\"Learning Gaussian Mixtures with Generalised Linear Models: Precise Asymptotics in High-dimensions\"},{\"link\":\"1510.01098v2\",\"title\":\"Intensity-only optical compressive imaging using a multiply scattering material and a double phase retrieval approach\"},{\"link\":\"2110.08775v3\",\"title\":\"Perturbative construction of mean-field equations in extensive-rank matrix factorization and denoising\"},{\"link\":\"2102.08127v3\",\"title\":\"Learning curves of generic features maps for realistic datasets with a teacher-student model\"},{\"link\":\"1006.2479v2\",\"title\":\"Glassy dynamics as a melting process (On melting dynamics and the glass transition, Part II)\"},{\"link\":\"2202.00293v4\",\"title\":\"Phase diagram of Stochastic Gradient Descent in high-dimensional two-layer neural networks\"},{\"link\":\"cond-mat_0403053v3\",\"title\":\"Spin glass models with ferromagnetically biased couplings on the Bethe lattice: analytic solutions and numerical simulations\"},{\"link\":\"2006.07310v2\",\"title\":\"Reservoir Computing meets Recurrent Kernels and Structured Transforms\"},{\"link\":\"1203.5521v3\",\"title\":\"Reweighted belief propagation and quiet planting for random K-SAT\"},{\"link\":\"2305.18270v3\",\"title\":\"How Two-Layer Neural Networks Learn, One (Giant) Step at a Time\"},{\"link\":\"2302.00375v2\",\"title\":\"Bayes-optimal Learning of Deep Random Networks of Extensive-width\"},{\"link\":\"1812.02537v1\",\"title\":\"Rank-one matrix estimation: analysis of algorithmic and information theoretic limits by the spatial coupling method\"},{\"link\":\"2006.06997v1\",\"title\":\"Complex Dynamics in Simple Neural Networks: Understanding Gradient Flow in Phase Retrieval\"},{\"link\":\"1404.7787v1\",\"title\":\"Spectral density of the non-backtracking operator\"},{\"link\":\"1006.2480v2\",\"title\":\"Glassy aspects of melting dynamics (On melting dynamics and the glass transition, Part I)\"},{\"link\":\"1008.4497v1\",\"title\":\"No spin glass phase in ferromagnetic random-field random-temperature scalar Ginzburg-Landau model\"},{\"link\":\"2210.06591v3\",\"title\":\"Rigorous dynamical mean field theory for stochastic gradient descent methods\"},{\"link\":\"1710.02903v1\",\"title\":\"Finite Size Corrections and Likelihood Ratio Fluctuations in the Spiked Wigner Model\"},{\"link\":\"1207.2079v1\",\"title\":\"Compressed Sensing of Approximately-Sparse Signals: Phase Transitions and Optimal Reconstruction\"},{\"link\":\"1203.3166v2\",\"title\":\"On the relation between kinetically constrained models of glass dynamics and the random first-order transition theory\"},{\"link\":\"2012.06373v1\",\"title\":\"Hardware Beyond Backpropagation: a Photonic Co-Processor for Direct Feedback Alignment\"},{\"link\":\"1604.02475v2\",\"title\":\"Performance Limits for Noisy Multi-Measurement Vector Problems\"},{\"link\":\"1506.03498v3\",\"title\":\"Matrix Completion from Fewer Entries: Spectral Detectability and Rank Estimation\"},{\"link\":\"2302.08923v1\",\"title\":\"Are Gaussian data all you need? Extents and limits of universality in high-dimensional generalized linear estimation\"},{\"link\":\"2302.08933v1\",\"title\":\"Universality laws for Gaussian mixtures in generalized linear models\"},{\"link\":\"1301.0901v1\",\"title\":\"Compressed Sensing under Matrix Uncertainty: Optimum Thresholds and Robust Approximate Message Passing\"},{\"link\":\"1809.06304v1\",\"title\":\"Approximate message-passing for convex optimization with non-separable penalties\"},{\"link\":\"1605.07516v1\",\"title\":\"Robust phase retrieval with the swept approximate message passing (prSAMP) algorithm\"},{\"link\":\"1302.0189v1\",\"title\":\"Non-adaptive pooling strategies for detection of rare faulty items\"},{\"link\":\"cond-mat_0512309v3\",\"title\":\"Aging, memory and rejuvenation: some lessons from simple models\"},{\"link\":\"1601.06683v2\",\"title\":\"Clustering from Sparse Pairwise Measurements\"},{\"link\":\"2203.07752v1\",\"title\":\"Optimal denoising of rotationally invariant rectangular matrices\"},{\"link\":\"2308.14085v1\",\"title\":\"Sampling with flows, diffusion and autoregressive neural networks: A spin-glass perspective\"},{\"link\":\"0910.5644v1\",\"title\":\"Quantum Annealing of Hard Problems\"},{\"link\":\"1810.13038v1\",\"title\":\"Spectral Method for Multiplexed Phase Retrieval and Application in Optical Imaging in Complex Media\"},{\"link\":\"2402.03220v3\",\"title\":\"The Benefits of Reusing Batches for Gradient Descent in Two-Layer Networks: Breaking the Curse of Information and Leap Exponents\"},{\"link\":\"1204.3734v1\",\"title\":\"Following states in temperature in the spherical s+p-spin glass model\"},{\"link\":\"2210.12760v4\",\"title\":\"On double-descent in uncertainty quantification in overparametrized models\"},{\"link\":\"1101.5863v1\",\"title\":\"Random-field p-spin glass model on regular random graphs\"},{\"link\":\"2208.05918v1\",\"title\":\"Low-rank Matrix Estimation with Inhomogeneous Noise\"},{\"link\":\"1901.09085v1\",\"title\":\"Generalisation dynamics of online learning in over-parameterised neural networks\"},{\"link\":\"cond-mat_0211106v2\",\"title\":\"Hiking through glassy phases: physics beyond aging\"},{\"link\":\"1906.04735v1\",\"title\":\"On the Universality of Noiseless Linear Estimation with Respect to the Measurement Matrix\"},{\"link\":\"2107.11814v1\",\"title\":\"LightOn Optical Processing Unit: Scaling-up AI and HPC with a Non von Neumann co-processor\"},{\"link\":\"1706.00705v1\",\"title\":\"Streaming Bayesian inference: theoretical limits and mini-batch approximate message-passing\"},{\"link\":\"1605.06422v3\",\"title\":\"Fast Randomized Semi-Supervised Clustering\"},{\"link\":\"2202.03295v2\",\"title\":\"Theoretical characterization of uncertainty in high-dimensional linear classification\"},{\"link\":\"cond-mat_0208566v2\",\"title\":\"Absence of an equilibrium ferromagnetic spin glass phase in three dimensions\"},{\"link\":\"2302.05882v1\",\"title\":\"From high-dimensional \u0026 mean-field dynamics to dimensionless ODEs: A unifying approach to SGD in two-layers networks\"},{\"link\":\"1307.7846v3\",\"title\":\"Belief-Propagation Guided Monte-Carlo Sampling\"},{\"link\":\"2302.06665v1\",\"title\":\"Optimal Algorithms for the Inhomogeneous Spiked Wigner Model\"},{\"link\":\"2310.14055v1\",\"title\":\"Spectral Phase Transitions in Non-Linear Wigner Spiked Models\"},{\"link\":\"0709.0894v1\",\"title\":\"Comment on \\\"Ultrametricity in the Edwards-Anderson Model\\\"\"},{\"link\":\"1602.02944v2\",\"title\":\"Fast phase retrieval for high dimensions: A block-based approach\"},{\"link\":\"2402.04980v2\",\"title\":\"Asymptotics of feature learning in two-layer networks after one gradient-step\"},{\"link\":\"2310.03575v2\",\"title\":\"Analysis of learning a flow-based generative model from limited sample complexity\"},{\"link\":\"2006.01475v2\",\"title\":\"Light-in-the-loop: using a photonics co-processor for scalable training of neural networks\"},{\"link\":\"1304.6599v2\",\"title\":\"Robust error correction for real-valued signals via message-passing decoding and spatial coupling\"},{\"link\":\"2303.02644v2\",\"title\":\"Expectation consistency for calibration of neural networks\"},{\"link\":\"2402.03902v1\",\"title\":\"A phase transition between positional and semantic learning in a solvable model of dot-product attention\"},{\"link\":\"0709.1023v1\",\"title\":\"Constraint optimization and landscapes\"},{\"link\":\"1609.08269v1\",\"title\":\"Spectral Bounds for the Ising Ferromagnet on an Arbitrary Given Graph\"},{\"link\":\"2310.02850v2\",\"title\":\"On the Atypical Solutions of the Symmetric Binary Perceptron\"},{\"link\":\"cond-mat_0409448v2\",\"title\":\"How many colors to color a random graph? Cavity, Complexity, Stability and all that\"},{\"link\":\"2402.13622v1\",\"title\":\"Analysis of Bootstrap and Subsampling in High-dimensional Regularized Regression\"},{\"link\":\"2201.09986v3\",\"title\":\"Bayesian Inference with Nonlinear Generative Models: Comments on Secure Learning\"},{\"link\":\"2405.15459v1\",\"title\":\"Repetita Iuvant: Data Repetition Allows SGD to Learn High-Dimensional Multi-Index Functions\"},{\"link\":\"2205.13527v2\",\"title\":\"Subspace clustering in high-dimensions: Phase transitions \u0026 Statistical-to-Computational gap\"},{\"link\":\"1511.05860v2\",\"title\":\"Scampi: a robust approximate message-passing framework for compressive imaging\"},{\"link\":\"2101.02115v1\",\"title\":\"Adversarial Robustness by Design through Analog Computing and Synthetic Gradients\"},{\"link\":\"2306.09283v1\",\"title\":\"Estimating rank-one matrices with mismatched prior and noise: universality and large deviations\"},{\"link\":\"2405.15480v2\",\"title\":\"Fundamental computational limits of weak learnability in high-dimensional multi-index models\"},{\"link\":\"2406.02157v1\",\"title\":\"Online Learning and Information Exponents: On The Importance of Batch size, and Time/Complexity Tradeoffs\"},{\"link\":\"2405.04267v2\",\"title\":\"Quenches in the Sherrington-Kirkpatrick model\"},{\"link\":\"2205.08782v1\",\"title\":\"Secure Coding via Gaussian Random Fields\"},{\"link\":\"2408.03733v1\",\"title\":\"Bayes-optimal learning of an extensive-width neural network from\\n quadratically many samples\"},{\"link\":\"2205.13503v2\",\"title\":\"Multi-layer State Evolution Under Random Convolutional Design\"},{\"link\":\"2403.04234v1\",\"title\":\"Fundamental limits of Non-Linear Low-Rank Matrix Estimation\"},{\"link\":\"1910.00285v2\",\"title\":\"Blind calibration for compressed sensing: State evolution and an online algorithm\"},{\"link\":\"2402.05674v2\",\"title\":\"A High Dimensional Statistical Model for Adversarial Training: Geometry and Trade-Offs\"},{\"link\":\"1104.0921v1\",\"title\":\"The nature of the different zero-temperature phases in discrete two-dimensional spin glasses: Entropy, universality, chaos and cascades in the renormalization group flow\"},{\"link\":\"0710.3336v2\",\"title\":\"Potts Glass on Random Graphs\"},{\"link\":\"1502.03324v1\",\"title\":\"Reference-less measurement of the transmission matrix of a highly scattering material using a DMD and phase retrieval techniques\"},{\"link\":\"1205.4200v4\",\"title\":\"Ultrametric probe of the spin-glass state in a field\"}]}"])</script><script>self.__next_f.push([1,"4e:T54b,The Wasserstein barycenter is a geometric construct which captures the notion of centrality among probability distributions, and which has found many applications in machine learning. However, most algorithms for finding even an approximate barycenter suffer an exponential dependence on the dimension $d$ of the underlying space of the distributions. In order to cope with this \"curse of dimensionality,\" we study dimensionality reduction techniques for the Wasserstein barycenter problem. When the barycenter is restricted to support of size $n$, we show that randomized dimensionality reduction can be used to map the problem to a space of dimension $O(\\log n)$ independent of both $d$ and $k$, and that \\emph{any} solution found in the reduced dimension will have its cost preserved up to arbitrary small error in the original space. We provide matching upper and lower bounds on the size of the reduced dimension, showing that our methods are optimal up to constant factors. We also provide a coreset construction for the Wasserstein barycenter problem that significantly decreases the number of input distributions. The coresets can be used in conjunction with random projections and thus further improve computation time. Lastly, our experimental results validate the speedup provided by dimensionality reduction while maintaining solution quality.4f:T54b,The Wasserstein barycenter is a geometric construct which captures the notion of centrality among probability distributions, and which has found many applications in machine learning. However, most algorithms for finding even an approximate barycenter suffer an exponential dependence on the dimension $d$ of the underlying space of the distributions. In order to cope with this \"curse of dimensionality,\" we study dimensionality reduction techniques for the Wasserstein barycenter problem. When the barycenter is restricted to support of size $n$, we show that randomized dimensionality reduction can be used to map the problem to a space of dimension $O(\\log n)$ independent "])</script><script>self.__next_f.push([1,"of both $d$ and $k$, and that \\emph{any} solution found in the reduced dimension will have its cost preserved up to arbitrary small error in the original space. We provide matching upper and lower bounds on the size of the reduced dimension, showing that our methods are optimal up to constant factors. We also provide a coreset construction for the Wasserstein barycenter problem that significantly decreases the number of input distributions. The coresets can be used in conjunction with random projections and thus further improve computation time. Lastly, our experimental results validate the speedup provided by dimensionality reduction while maintaining solution quality.50:T640,We consider a minimax problem motivated by distributionally robust optimization (DRO) when the worst-case distribution is continuous, leading to significant computational challenges due to the infinite-dimensional nature of the optimization problem. Recent research has explored learning the worst-case distribution using neural network-based generative models to address these computational challenges but lacks algorithmic convergence guarantees. This paper bridges this theoretical gap by presenting an iterative algorithm to solve such a minimax problem, achieving global convergence under mild assumptions and leveraging technical tools from vector space minimax optimization and convex analysis in the space of continuous probability densities. In particular, leveraging Brenier's theorem, we represent the worst-case distribution as a transport map applied to a continuous reference measure and reformulate the regularized discrepancy-based DRO as a minimax problem in the Wasserstein space. Furthermore, we demonstrate that the worst-case distribution can be efficiently computed using a modified Jordan-Kinderlehrer-Otto (JKO) scheme with sufficiently large regularization parameters for commonly used discrepancy functions, linked to the radius of the ambiguity set. Additionally, we derive the global convergence rate and quantify the total number of "])</script><script>self.__next_f.push([1,"subgradient and inexact modified JKO iterations required to obtain approximate stationary points. These results are potentially applicable to nonconvex and nonsmooth scenarios, with broad relevance to modern machine learning applications.51:T5e0e,"])</script><script>self.__next_f.push([1,"Classification - Machine Learning This is ‘Classification’ tutorial which is a part of the Machine Learning course offered by Simplilearn. We will learn Classification algorithms, types of classification algorithms, support vector machines(SVM), Naive Bayes, Decision Tree and Random Forest Classifier in this tutorial. Objectives Let us look at some of the objectives covered under this section of Machine Learning tutorial. Define Classification and list its algorithms Describe Logistic Regression and Sigmoid Probability Explain K-Nearest Neighbors and KNN classification Understand Support Vector Machines, Polynomial Kernel, and Kernel Trick Analyze Kernel Support Vector Machines with an example Implement the Naïve Bayes Classifier Demonstrate Decision Tree Classifier Describe Random Forest Classifier Classification: Meaning Classification is a type of supervised learning. It specifies the class to which data elements belong to and is best used when the output has finite and discrete values. It predicts a class for an input variable as well. There are 2 types of Classification: Binomial Multi-Class Classification: Use Cases Some of the key areas where classification cases are being used: To find whether an email received is a spam or ham To identify customer segments To find if a bank loan is granted To identify if a kid will pass or fail in an examination Classification: Example Social media sentiment analysis has two potential outcomes, positive or negative, as displayed by the chart given below. https://www.simplilearn.com/ice9/free_resources_article_thumb/classification-example-machine-learning.JPG This chart shows the classification of the Iris flower dataset into its three sub-species indicated by codes 0, 1, and 2. https://www.simplilearn.com/ice9/free_resources_article_thumb/iris-flower-dataset-graph.JPG The test set dots represent the assignment of new test data points to one class or the other based on the trained classifier model. Types of Classification Algorithms Let’s have a quick look into the types of Classification Algorithm below. Linear Models Logistic Regression Support Vector Machines Nonlinear models K-nearest Neighbors (KNN) Kernel Support Vector Machines (SVM) Naïve Bayes Decision Tree Classification Random Forest Classification Logistic Regression: Meaning Let us understand the Logistic Regression model below. This refers to a regression model that is used for classification. This method is widely used for binary classification problems. It can also be extended to multi-class classification problems. Here, the dependent variable is categorical: y ϵ {0, 1} A binary dependent variable can have only two values, like 0 or 1, win or lose, pass or fail, healthy or sick, etc In this case, you model the probability distribution of output y as 1 or 0. This is called the sigmoid probability (σ). If σ(θ Tx) \u003e 0.5, set y = 1, else set y = 0 Unlike Linear Regression (and its Normal Equation solution), there is no closed form solution for finding optimal weights of Logistic Regression. Instead, you must solve this with maximum likelihood estimation (a probability model to detect the maximum likelihood of something happening). It can be used to calculate the probability of a given outcome in a binary model, like the probability of being classified as sick or passing an exam. https://www.simplilearn.com/ice9/free_resources_article_thumb/logistic-regression-example-graph.JPG Sigmoid Probability The probability in the logistic regression is often represented by the Sigmoid function (also called the logistic function or the S-curve): https://www.simplilearn.com/ice9/free_resources_article_thumb/sigmoid-function-machine-learning.JPG In this equation, t represents data values * the number of hours studied and S(t) represents the probability of passing the exam. Assume sigmoid function: https://www.simplilearn.com/ice9/free_resources_article_thumb/sigmoid-probability-machine-learning.JPG g(z) tends toward 1 as z -\u003e infinity , and g(z) tends toward 0 as z -\u003e infinity K-nearest Neighbors (KNN) K-nearest Neighbors algorithm is used to assign a data point to clusters based on similarity measurement. It uses a supervised method for classification. The steps to writing a k-means algorithm are as given below: https://www.simplilearn.com/ice9/free_resources_article_thumb/knn-distribution-graph-machine-learning.JPG Choose the number of k and a distance metric. (k = 5 is common) Find k-nearest neighbors of the sample that you want to classify Assign the class label by majority vote. KNN Classification A new input point is classified in the category such that it has the most number of neighbors from that category. For example: https://www.simplilearn.com/ice9/free_resources_article_thumb/knn-classification-machine-learning.JPG Classify a patient as high risk or low risk. Mark email as spam or ham. Keen on learning about Classification Algorithms in Machine Learning? Click here! Support Vector Machine (SVM) Let us understand Support Vector Machine (SVM) in detail below. SVMs are classification algorithms used to assign data to various classes. They involve detecting hyperplanes which segregate data into classes. SVMs are very versatile and are also capable of performing linear or nonlinear classification, regression, and outlier detection. Once ideal hyperplanes are discovered, new data points can be easily classified. https://www.simplilearn.com/ice9/free_resources_article_thumb/support-vector-machines-graph-machine-learning.JPG The optimization objective is to find “maximum margin hyperplane” that is farthest from the closest points in the two classes (these points are called support vectors). In the given figure, the middle line represents the hyperplane. SVM Example Let’s look at this image below and have an idea about SVM in general. Hyperplanes with larger margins have lower generalization error. The positive and negative hyperplanes are represented by: https://www.simplilearn.com/ice9/free_resources_article_thumb/positive-negative-hyperplanes-machine-learning.JPG Classification of any new input sample xtest : If w0 + wTxtest \u003e 1, the sample xtest is said to be in the class toward the right of the positive hyperplane. If w0 + wTxtest \u003c -1, the sample xtest is said to be in the class toward the left of the negative hyperplane. When you subtract the two equations, you get: https://www.simplilearn.com/ice9/free_resources_article_thumb/equation-subtraction-machine-learning.JPG Length of vector w is (L2 norm length): https://www.simplilearn.com/ice9/free_resources_article_thumb/length-of-vector-machine-learning.JPG You normalize with the length of w to arrive at: https://www.simplilearn.com/ice9/free_resources_article_thumb/normalize-equation-machine-learning.JPG SVM: Hard Margin Classification Given below are some points to understand Hard Margin Classification. The left side of equation SVM-1 given above can be interpreted as the distance between the positive (+ve) and negative (-ve) hyperplanes; in other words, it is the margin that can be maximized. Hence the objective of the function is to maximize with the constraint that the samples are classified correctly, which is represented as : https://www.simplilearn.com/ice9/free_resources_article_thumb/hard-margin-classification-machine-learning.JPG This means that you are minimizing ‖w‖. This also means that all positive samples are on one side of the positive hyperplane and all negative samples are on the other side of the negative hyperplane. This can be written concisely as : https://www.simplilearn.com/ice9/free_resources_article_thumb/hard-margin-classification-formula.JPG Minimizing ‖w‖ is the same as minimizing. This figure is better as it is differentiable even at w = 0. The approach listed above is called “hard margin linear SVM classifier.” SVM: Soft Margin Classification Given below are some points to understand Soft Margin Classification. To allow for linear constraints to be relaxed for nonlinearly separable data, a slack variable is introduced. (i) measures how much ith instance is allowed to violate the margin. The slack variable is simply added to the linear constraints. https://www.simplilearn.com/ice9/free_resources_article_thumb/soft-margin-calculation-machine-learning.JPG Subject to the above constraints, the new objective to be minimized becomes: https://www.simplilearn.com/ice9/free_resources_article_thumb/soft-margin-calculation-formula.JPG You have two conflicting objectives now—minimizing slack variable to reduce margin violations and minimizing to increase the margin. The hyperparameter C allows us to define this trade-off. Large values of C correspond to larger error penalties (so smaller margins), whereas smaller values of C allow for higher misclassification errors and larger margins. https://www.simplilearn.com/ice9/free_resources_article_thumb/machine-learning-certification-video-preview.jpg SVM: Regularization The concept of C is the reverse of regularization. Higher C means lower regularization, which increases bias and lowers the variance (causing overfitting). https://www.simplilearn.com/ice9/free_resources_article_thumb/concept-of-c-graph-machine-learning.JPG IRIS Data Set The Iris dataset contains measurements of 150 IRIS flowers from three different species: Setosa Versicolor Viriginica Each row represents one sample. Flower measurements in centimeters are stored as columns. These are called features. IRIS Data Set: SVM Let’s train an SVM model using sci-kit-learn for the Iris dataset: https://www.simplilearn.com/ice9/free_resources_article_thumb/svm-model-graph-machine-learning.JPG Nonlinear SVM Classification There are two ways to solve nonlinear SVMs: by adding polynomial features by adding similarity features Polynomial features can be added to datasets; in some cases, this can create a linearly separable dataset. https://www.simplilearn.com/ice9/free_resources_article_thumb/nonlinear-classification-svm-machine-learning.JPG In the figure on the left, there is only 1 feature x1. This dataset is not linearly separable. If you add x2 = (x1)2 (figure on the right), the data becomes linearly separable. Polynomial Kernel In sci-kit-learn, one can use a Pipeline class for creating polynomial features. Classification results for the Moons dataset are shown in the figure. https://www.simplilearn.com/ice9/free_resources_article_thumb/polynomial-kernel-machine-learning.JPG Polynomial Kernel with Kernel Trick Let us look at the image below and understand Kernel Trick in detail. https://www.simplilearn.com/ice9/free_resources_article_thumb/polynomial-kernel-with-kernel-trick.JPG For large dimensional datasets, adding too many polynomial features can slow down the model. You can apply a kernel trick with the effect of polynomial features without actually adding them. The code is shown (SVC class) below trains an SVM classifier using a 3rd-degree polynomial kernel but with a kernel trick. https://www.simplilearn.com/ice9/free_resources_article_thumb/polynomial-kernel-equation-machine-learning.JPG The hyperparameter coefθ controls the influence of high-degree polynomials. Kernel SVM Let us understand in detail about Kernel SVM. Kernel SVMs are used for classification of nonlinear data. In the chart, nonlinear data is projected into a higher dimensional space via a mapping function where it becomes linearly separable. https://www.simplilearn.com/ice9/free_resources_article_thumb/kernel-svm-machine-learning.JPG In the higher dimension, a linear separating hyperplane can be derived and used for classification. A reverse projection of the higher dimension back to original feature space takes it back to nonlinear shape. As mentioned previously, SVMs can be kernelized to solve nonlinear classification problems. You can create a sample dataset for XOR gate (nonlinear problem) from NumPy. 100 samples will be assigned the class sample 1, and 100 samples will be assigned the class label -1. https://www.simplilearn.com/ice9/free_resources_article_thumb/kernel-svm-graph-machine-learning.JPG As you can see, this data is not linearly separable. https://www.simplilearn.com/ice9/free_resources_article_thumb/kernel-svm-non-separable.JPG You now use the kernel trick to classify XOR dataset created earlier. https://www.simplilearn.com/ice9/free_resources_article_thumb/kernel-svm-xor-machine-learning.JPG Naïve Bayes Classifier What is Naive Bayes Classifier? Have you ever wondered how your mail provider implements spam filtering or how online news channels perform news text classification or even how companies perform sentiment analysis of their audience on social media? All of this and more are done through a machine learning algorithm called Naive Bayes Classifier. Naive Bayes Named after Thomas Bayes from the 1700s who first coined this in the Western literature. Naive Bayes classifier works on the principle of conditional probability as given by the Bayes theorem. Advantages of Naive Bayes Classifier Listed below are six benefits of Naive Bayes Classifier. Very simple and easy to implement Needs less training data Handles both continuous and discrete data Highly scalable with the number of predictors and data points As it is fast, it can be used in real-time predictions Not sensitive to irrelevant features Bayes Theorem We will understand Bayes Theorem in detail from the points mentioned below. According to the Bayes model, the conditional probability P(Y|X) can be calculated as: P(Y|X) = P(X|Y)P(Y) / P(X) This means you have to estimate a very large number of P(X|Y) probabilities for a relatively small vector space X. For example, for a Boolean Y and 30 possible Boolean attributes in the X vector, you will have to estimate 3 billion probabilities P(X|Y). To make it practical, a Naïve Bayes classifier is used, which assumes conditional independence of P(X) to each other, with a given value of Y. This reduces the number of probability estimates to 2*30=60 in the above example. Naïve Bayes Classifier for SMS Spam Detection Consider a labeled SMS database having 5574 messages. It has messages as given below: https://www.simplilearn.com/ice9/free_resources_article_thumb/naive-bayes-spam-machine-learning.JPG Each message is marked as spam or ham in the data set. Let’s train a model with Naïve Bayes algorithm to detect spam from ham. The message lengths and their frequency (in the training dataset) are as shown below: https://www.simplilearn.com/ice9/free_resources_article_thumb/naive-bayes-spam-spam-detection.JPG Analyze the logic you use to train an algorithm to detect spam: Split each message into individual words/tokens (bag of words). Lemmatize the data (each word takes its base form, like “walking” or “walked” is replaced with “walk”). Convert data to vectors using scikit-learn module CountVectorizer. Run TFIDF to remove common words like “is,” “are,” “and.” Now apply scikit-learn module for Naïve Bayes MultinomialNB to get the Spam Detector. This spam detector can then be used to classify a random new message as spam or ham. Next, the accuracy of the spam detector is checked using the Confusion Matrix. For the SMS spam example above, the confusion matrix is shown on the right. Accuracy Rate = Correct / Total = (4827 + 592)/5574 = 97.21% Error Rate = Wrong / Total = (155 + 0)/5574 = 2.78% https://www.simplilearn.com/ice9/free_resources_article_thumb/confusion-matrix-machine-learning.JPG Although confusion Matrix is useful, some more precise metrics are provided by Precision and Recall. https://www.simplilearn.com/ice9/free_resources_article_thumb/precision-recall-matrix-machine-learning.JPG Precision refers to the accuracy of positive predictions. https://www.simplilearn.com/ice9/free_resources_article_thumb/precision-formula-machine-learning.JPG Recall refers to the ratio of positive instances that are correctly detected by the classifier (also known as True positive rate or TPR). https://www.simplilearn.com/ice9/free_resources_article_thumb/recall-formula-machine-learning.JPG Precision/Recall Trade-off To detect age-appropriate videos for kids, you need high precision (low recall) to ensure that only safe videos make the cut (even though a few safe videos may be left out). The high recall is needed (low precision is acceptable) in-store surveillance to catch shoplifters; a few false alarms are acceptable, but all shoplifters must be caught. Learn about Naive Bayes in detail. Click here! Decision Tree Classifier Some aspects of the Decision Tree Classifier mentioned below are. Decision Trees (DT) can be used both for classification and regression. The advantage of decision trees is that they require very little data preparation. They do not require feature scaling or centering at all. They are also the fundamental components of Random Forests, one of the most powerful ML algorithms. Unlike Random Forests and Neural Networks (which do black-box modeling), Decision Trees are white box models, which means that inner workings of these models are clearly understood. In the case of classification, the data is segregated based on a series of questions. Any new data point is assigned to the selected leaf node. https://www.simplilearn.com/ice9/free_resources_article_thumb/decision-tree-classifier-machine-learning.JPG Start at the tree root and split the data on the feature using the decision algorithm, resulting in the largest information gain (IG). This splitting procedure is then repeated in an iterative process at each child node until the leaves are pure. This means that the samples at each node belonging to the same class. In practice, you can set a limit on the depth of the tree to prevent overfitting. The purity is compromised here as the final leaves may still have some impurity. The figure shows the classification of the Iris dataset. https://www.simplilearn.com/ice9/free_resources_article_thumb/decision-tree-classifier-graph.JPG IRIS Decision Tree Let’s build a Decision Tree using scikit-learn for the Iris flower dataset and also visualize it using export_graphviz API. https://www.simplilearn.com/ice9/free_resources_article_thumb/iris-decision-tree-machine-learning.JPG The output of export_graphviz can be converted into png format: https://www.simplilearn.com/ice9/free_resources_article_thumb/iris-decision-tree-output.JPG Sample attribute stands for the number of training instances the node applies to. Value attribute stands for the number of training instances of each class the node applies to. Gini impurity measures the node’s impurity. A node is “pure” (gini=0) if all training instances it applies to belong to the same class. https://www.simplilearn.com/ice9/free_resources_article_thumb/impurity-formula-machine-learning.JPG For example, for Versicolor (green color node), the Gini is 1-(0/54)2 -(49/54)2 -(5/54) 2 ≈ 0.168 https://www.simplilearn.com/ice9/free_resources_article_thumb/iris-decision-tree-sample.JPG Decision Boundaries Let us learn to create decision boundaries below. For the first node (depth 0), the solid line splits the data (Iris-Setosa on left). Gini is 0 for Setosa node, so no further split is possible. The second node (depth 1) splits the data into Versicolor and Virginica. If max_depth were set as 3, a third split would happen (vertical dotted line). https://www.simplilearn.com/ice9/free_resources_article_thumb/decision-tree-boundaries.JPG For a sample with petal length 5 cm and petal width 1.5 cm, the tree traverses to depth 2 left node, so the probability predictions for this sample are 0% for Iris-Setosa (0/54), 90.7% for Iris-Versicolor (49/54), and 9.3% for Iris-Virginica (5/54) CART Training Algorithm Scikit-learn uses Classification and Regression Trees (CART) algorithm to train Decision Trees. CART algorithm: Split the data into two subsets using a single feature k and threshold tk (example, petal length \u003c “2.45 cm”). This is done recursively for each node. k and tk are chosen such that they produce the purest subsets (weighted by their size). The objective is to minimize the cost function as given below: https://www.simplilearn.com/ice9/free_resources_article_thumb/cart-training-algorithm-machine-learning.JPG The algorithm stops executing if one of the following situations occurs: max_depth is reached No further splits are found for each node Other hyperparameters may be used to stop the tree: min_samples_split min_samples_leaf min_weight_fraction_leaf max_leaf_nodes Gini Impurity or Entropy Entropy is one more measure of impurity and can be used in place of Gini. https://www.simplilearn.com/ice9/free_resources_article_thumb/gini-impurity-entrophy.JPG It is a degree of uncertainty, and Information Gain is the reduction that occurs in entropy as one traverses down the tree. Entropy is zero for a DT node when the node contains instances of only one class. Entropy for depth 2 left node in the example given above is: https://www.simplilearn.com/ice9/free_resources_article_thumb/entrophy-for-depth-2.JPG Gini and Entropy both lead to similar trees. DT: Regularization The following figure shows two decision trees on the moons dataset. https://www.simplilearn.com/ice9/free_resources_article_thumb/dt-regularization-machine-learning.JPG The decision tree on the right is restricted by min_samples_leaf = 4. The model on the left is overfitting, while the model on the right generalizes better. Random Forest Classifier Let us have an understanding of Random Forest Classifier below. A random forest can be considered an ensemble of decision trees (Ensemble learning). Random Forest algorithm: Draw a random bootstrap sample of size n (randomly choose n samples from the training set). Grow a decision tree from the bootstrap sample. At each node, randomly select d features. Split the node using the feature that provides the best split according to the objective function, for instance by maximizing the information gain. Repeat the steps 1 to 2 k times. (k is the number of trees you want to create, using a subset of samples) Aggregate the prediction by each tree for a new data point to assign the class label by majority vote (pick the group selected by the most number of trees and assign new data point to that group). Random Forests are opaque, which means it is difficult to visualize their inner workings. https://www.simplilearn.com/ice9/free_resources_article_thumb/random-forest-classifier-graph.JPG However, the advantages outweigh their limitations since you do not have to worry about hyperparameters except k, which stands for the number of decision trees to be created from a subset of samples. RF is quite robust to noise from the individual decision trees. Hence, you need not prune individual decision trees. The larger the number of decision trees, the more accurate the Random Forest prediction is. (This, however, comes with higher computation cost). Key Takeaways Let us quickly run through what we have learned so far in this Classification tutorial. Classification algorithms are supervised learning methods to split data into classes. They can work on Linear Data as well as Nonlinear Data. Logistic Regression can classify data based on weighted parameters and sigmoid conversion to calculate the probability of classes. K-nearest Neighbors (KNN) algorithm uses similar features to classify data. Support Vector Machines (SVMs) classify data by detecting the maximum margin hyperplane between data classes. Naïve Bayes, a simplified Bayes Model, can help classify data using conditional probability models. Decision Trees are powerful classifiers and use tree splitting logic until pure or somewhat pure leaf node classes are attained. Random Forests apply Ensemble Learning to Decision Trees for more accurate classification predictions. Conclusion This completes ‘Classification’ tutorial. In the next tutorial, we will learn 'Unsupervised Learning with Clustering.'"])</script><script>self.__next_f.push([1,"52:T640,We consider a minimax problem motivated by distributionally robust optimization (DRO) when the worst-case distribution is continuous, leading to significant computational challenges due to the infinite-dimensional nature of the optimization problem. Recent research has explored learning the worst-case distribution using neural network-based generative models to address these computational challenges but lacks algorithmic convergence guarantees. This paper bridges this theoretical gap by presenting an iterative algorithm to solve such a minimax problem, achieving global convergence under mild assumptions and leveraging technical tools from vector space minimax optimization and convex analysis in the space of continuous probability densities. In particular, leveraging Brenier's theorem, we represent the worst-case distribution as a transport map applied to a continuous reference measure and reformulate the regularized discrepancy-based DRO as a minimax problem in the Wasserstein space. Furthermore, we demonstrate that the worst-case distribution can be efficiently computed using a modified Jordan-Kinderlehrer-Otto (JKO) scheme with sufficiently large regularization parameters for commonly used discrepancy functions, linked to the radius of the ambiguity set. Additionally, we derive the global convergence rate and quantify the total number of subgradient and inexact modified JKO iterations required to obtain approximate stationary points. These results are potentially applicable to nonconvex and nonsmooth scenarios, with broad relevance to modern machine learning applications.53:T4c6,Multilingual Large Language Models are capable of using powerful Large Language Models to handle and respond to queries in multiple languages, which achieves remarkable success in multilingual natural language processing tasks. Despite these breakthroughs, there still remains a lack of a comprehensive survey to summarize existing approaches and recent developments in this field. To this end, in this paper, we present a thorough "])</script><script>self.__next_f.push([1,"review and provide a unified perspective to summarize the recent progress as well as emerging trends in multilingual large language models (MLLMs) literature. The contributions of this paper can be summarized: (1) First survey: to our knowledge, we take the first step and present a thorough review in MLLMs research field according to multi-lingual alignment; (2) New taxonomy: we offer a new and unified perspective to summarize the current progress of MLLMs; (3) New frontiers: we highlight several emerging frontiers and discuss the corresponding challenges; (4) Abundant resources: we collect abundant open-source resources, including relevant papers, data corpora, and leaderboards. We hope our work can provide the community with quick access and spur breakthrough research in MLLMs.54:T4c6,Multilingual Large Language Models are capable of using powerful Large Language Models to handle and respond to queries in multiple languages, which achieves remarkable success in multilingual natural language processing tasks. Despite these breakthroughs, there still remains a lack of a comprehensive survey to summarize existing approaches and recent developments in this field. To this end, in this paper, we present a thorough review and provide a unified perspective to summarize the recent progress as well as emerging trends in multilingual large language models (MLLMs) literature. The contributions of this paper can be summarized: (1) First survey: to our knowledge, we take the first step and present a thorough review in MLLMs research field according to multi-lingual alignment; (2) New taxonomy: we offer a new and unified perspective to summarize the current progress of MLLMs; (3) New frontiers: we highlight several emerging frontiers and discuss the corresponding challenges; (4) Abundant resources: we collect abundant open-source resources, including relevant papers, data corpora, and leaderboards. We hope our work can provide the community with quick access and spur breakthrough research in MLLMs.55:T40f,We introduce a theor"])</script><script>self.__next_f.push([1,"y of $*$-structures for bialgebroids and Hopf algebroids over a $*$-algebra, defined in such a way that the relevant category of (co)modules is a bar category. We show that if $H$ is a Hopf $*$-algebra then the action Hopf algebroid $A\\# H$ associated to a braided-commutative algebra in the category of $H$-crossed modules is a full $*$-Hopf algebroid and the Ehresmann-Schauenburg Hopf algebroid $\\mathcal{L}(P,H)$ associated to a Hopf-Galois extension or quantum group principal bundle $P$ with fibre $H$ forms a $*$-Hopf algebroid pair, when the relevant (co)action respects $*$. We also show that Ghobadi's bialgebroid associated to a $*$-differential structure $(\\Omega^{1},\\rm d)$ on $A$ forms a $*$-bialgebroid pair and its quotient in the pivotal case a $*$-Hopf algebroid pair when the pivotal structure is compatible with $*$. We show that when $\\Omega^1$ is simultaneously free on both sides, Ghobadi's Hopf algebroid is isomorphic to $\\mathcal{L}(A\\#H,H)$ for a smash product by a certain Hopf algebra $H$.56:T40f,We introduce a theory of $*$-structures for bialgebroids and Hopf algebroids over a $*$-algebra, defined in such a way that the relevant category of (co)modules is a bar category. We show that if $H$ is a Hopf $*$-algebra then the action Hopf algebroid $A\\# H$ associated to a braided-commutative algebra in the category of $H$-crossed modules is a full $*$-Hopf algebroid and the Ehresmann-Schauenburg Hopf algebroid $\\mathcal{L}(P,H)$ associated to a Hopf-Galois extension or quantum group principal bundle $P$ with fibre $H$ forms a $*$-Hopf algebroid pair, when the relevant (co)action respects $*$. We also show that Ghobadi's bialgebroid associated to a $*$-differential structure $(\\Omega^{1},\\rm d)$ on $A$ forms a $*$-bialgebroid pair and its quotient in the pivotal case a $*$-Hopf algebroid pair when the pivotal structure is compatible with $*$. We show that when $\\Omega^1$ is simultaneously free on both sides, Ghobadi's Hopf algebroid is isomorphic to $\\mathcal{L}(A\\#H,H)$ for a smash product by a certain"])</script><script>self.__next_f.push([1," Hopf algebra $H$.57:T5a2,Evaluating models on large benchmarks is very resource-intensive, especially\nduring the period of rapid model evolution. Existing efficient evaluation\nmethods estimate the performance of target models by testing them only on a\nsmall and static coreset of the benchmark, which is derived from the publicly\navailable evaluation results of source models. These methods rely on the\nassumption that target models have high prediction consistency with source\nmodels. However, we demonstrate that it doesn't generalize well in practice. To\nalleviate the inconsistency issue, we present TailoredBench, a method that\nconducts customized evaluation tailored to each target model. Specifically, a\nGlobal-coreset is first constructed as a probe to identify the most consistent\nsource models for each target model with an adaptive source model selection\nstrategy. Afterwards, a scalable K-Medoids clustering algorithm is proposed to\nextend the Global-coreset to a tailored Native-coreset for each target model.\nAccording to the predictions on Native-coresets, we obtain the performance of\ntarget models on the whole benchmark with a calibrated estimation strategy.\nComprehensive experiments on 5 benchmarks across over 300 models demonstrate\nthat compared to best performing baselines, TailoredBench achieves an average\nreduction of 31.4% in MAE of accuracy estimates under the same inference\nbudgets, showcasing strong effectiveness and generalizability.58:T5a2,Evaluating models on large benchmarks is very resource-intensive, especially\nduring the period of rapid model evolution. Existing efficient evaluation\nmethods estimate the performance of target models by testing them only on a\nsmall and static coreset of the benchmark, which is derived from the publicly\navailable evaluation results of source models. These methods rely on the\nassumption that target models have high prediction consistency with source\nmodels. However, we demonstrate that it doesn't generalize well in practice. To\nalleviate the inconsistency issue, we p"])</script><script>self.__next_f.push([1,"resent TailoredBench, a method that\nconducts customized evaluation tailored to each target model. Specifically, a\nGlobal-coreset is first constructed as a probe to identify the most consistent\nsource models for each target model with an adaptive source model selection\nstrategy. Afterwards, a scalable K-Medoids clustering algorithm is proposed to\nextend the Global-coreset to a tailored Native-coreset for each target model.\nAccording to the predictions on Native-coresets, we obtain the performance of\ntarget models on the whole benchmark with a calibrated estimation strategy.\nComprehensive experiments on 5 benchmarks across over 300 models demonstrate\nthat compared to best performing baselines, TailoredBench achieves an average\nreduction of 31.4% in MAE of accuracy estimates under the same inference\nbudgets, showcasing strong effectiveness and generalizability.59:T437,Many methods that build powerful variational distributions based on unadjusted Langevin transitions exist. Most of these were developed using a wide range of different approaches and techniques. Unfortunately, the lack of a unified analysis and derivation makes developing new methods and reasoning about existing ones a challenging task. We address this giving a single analysis that unifies and generalizes these existing techniques. The main idea is to augment the target and variational by numerically simulating the underdamped Langevin diffusion process and its time reversal. The benefits of this approach are twofold: it provides a unified formulation for many existing methods, and it simplifies the development of new ones. In fact, using our formulation we propose a new method that combines the strengths of previously existing algorithms; it uses underdamped Langevin transitions and powerful augmentations parameterized by a score network. Our empirical evaluation shows that our proposed method consistently outperforms relevant baselines in a wide range of tasks.5a:T437,Many methods that build powerful variational distributions based on unadjusted Lang"])</script><script>self.__next_f.push([1,"evin transitions exist. Most of these were developed using a wide range of different approaches and techniques. Unfortunately, the lack of a unified analysis and derivation makes developing new methods and reasoning about existing ones a challenging task. We address this giving a single analysis that unifies and generalizes these existing techniques. The main idea is to augment the target and variational by numerically simulating the underdamped Langevin diffusion process and its time reversal. The benefits of this approach are twofold: it provides a unified formulation for many existing methods, and it simplifies the development of new ones. In fact, using our formulation we propose a new method that combines the strengths of previously existing algorithms; it uses underdamped Langevin transitions and powerful augmentations parameterized by a score network. Our empirical evaluation shows that our proposed method consistently outperforms relevant baselines in a wide range of tasks.5b:T6a9,Today, many systems use artificial intelligence (AI) to solve complex problems. While this often increases system effectiveness, developing a production-ready AI-based system is a difficult task. Thus, solid AI engineering practices are required to ensure the quality of the resulting system and to improve the development process. While several practices have already been proposed for the development of AI-based systems, detailed practical experiences of applying these practices are rare.\nIn this paper, we aim to address this gap by collecting such experiences during a case study, namely the development of an autonomous stock trading system that uses machine learning functionality to invest in stocks. We selected 10 AI engineering practices from the literature and systematically applied them during development, with the goal to collect evidence about their applicability and effectiveness. Using structured field notes, we documented our experiences. Furthermore, we also used field notes to document challenges that occurred during "])</script><script>self.__next_f.push([1,"the development, and the solutions we applied to overcome them. Afterwards, we analyzed the collected field notes, and evaluated how each practice improved the development. Lastly, we compared our evidence with existing literature.\nMost applied practices improved our system, albeit to varying extent, and we were able to overcome all major challenges. The qualitative results provide detailed accounts about 10 AI engineering practices, as well as challenges and solutions associated with such a project. Our experiences therefore enrich the emerging body of evidence in this field, which may be especially helpful for practitioner teams new to AI engineering.5c:T6a9,Today, many systems use artificial intelligence (AI) to solve complex problems. While this often increases system effectiveness, developing a production-ready AI-based system is a difficult task. Thus, solid AI engineering practices are required to ensure the quality of the resulting system and to improve the development process. While several practices have already been proposed for the development of AI-based systems, detailed practical experiences of applying these practices are rare.\nIn this paper, we aim to address this gap by collecting such experiences during a case study, namely the development of an autonomous stock trading system that uses machine learning functionality to invest in stocks. We selected 10 AI engineering practices from the literature and systematically applied them during development, with the goal to collect evidence about their applicability and effectiveness. Using structured field notes, we documented our experiences. Furthermore, we also used field notes to document challenges that occurred during the development, and the solutions we applied to overcome them. Afterwards, we analyzed the collected field notes, and evaluated how each practice improved the development. Lastly, we compared our evidence with existing literature.\nMost applied practices improved our system, albeit to varying extent, and we were able to overcome all "])</script><script>self.__next_f.push([1,"major challenges. The qualitative results provide detailed accounts about 10 AI engineering practices, as well as challenges and solutions associated with such a project. Our experiences therefore enrich the emerging body of evidence in this field, which may be especially helpful for practitioner teams new to AI engineering.5d:T57a,Structural pruning enables model acceleration by removing structurally-grouped parameters from neural networks. However, the parameter-grouping patterns vary widely across different models, making architecture-specific pruners, which rely on manually-designed grouping schemes, non-generalizable to new architectures. In this work, we study a highly-challenging yet barely-explored task, any structural pruning, to tackle general structural pruning of arbitrary architecture like CNNs, RNNs, GNNs and Transformers. The most prominent obstacle towards this goal lies in the structural coupling, which not only forces different layers to be pruned simultaneously, but also expects all removed parameters to be consistently unimportant, thereby avoiding structural issues and significant performance degradation after pruning. To address this problem, we propose a general and {fully automatic} method, \\emph{Dependency Graph} (DepGraph), to explicitly model the dependency between layers and comprehensively group coupled parameters for pruning. In this work, we extensively evaluate our method on several architectures and tasks, including ResNe(X)t, DenseNet, MobileNet and Vision transformer for images, GAT for graph, DGCNN for 3D point cloud, alongside LSTM for language, and demonstrate that, even with a simple norm-based criterion, the proposed method consistently yields gratifying performances.5e:T57a,Structural pruning enables model acceleration by removing structurally-grouped parameters from neural networks. However, the parameter-grouping patterns vary widely across different models, making architecture-specific pruners, which rely on manually-designed grouping schemes, non-generalizable to new a"])</script><script>self.__next_f.push([1,"rchitectures. In this work, we study a highly-challenging yet barely-explored task, any structural pruning, to tackle general structural pruning of arbitrary architecture like CNNs, RNNs, GNNs and Transformers. The most prominent obstacle towards this goal lies in the structural coupling, which not only forces different layers to be pruned simultaneously, but also expects all removed parameters to be consistently unimportant, thereby avoiding structural issues and significant performance degradation after pruning. To address this problem, we propose a general and {fully automatic} method, \\emph{Dependency Graph} (DepGraph), to explicitly model the dependency between layers and comprehensively group coupled parameters for pruning. In this work, we extensively evaluate our method on several architectures and tasks, including ResNe(X)t, DenseNet, MobileNet and Vision transformer for images, GAT for graph, DGCNN for 3D point cloud, alongside LSTM for language, and demonstrate that, even with a simple norm-based criterion, the proposed method consistently yields gratifying performances.5f:T4bc,Nowadays automated dynamic analysis frameworks for continuous testing are in\nhigh demand to ensure software safety and satisfy the security development\nlifecycle (SDL) requirements. The security bug hunting efficiency of\ncutting-edge hybrid fuzzing techniques outperforms widely utilized\ncoverage-guided fuzzing. We propose an enhanced dynamic analysis pipeline to\nleverage productivity of automated bug detection based on hybrid fuzzing. We\nimplement the proposed pipeline in the continuous fuzzing toolset Sydr-Fuzz\nwhich is powered by hybrid fuzzing orchestrator, integrating our DSE tool Sydr\nwith libFuzzer and AFL++. Sydr-Fuzz also incorporates security predicate\ncheckers, crash triaging tool Casr, and utilities for corpus minimization and\ncoverage gathering. The benchmarking of our hybrid fuzzer against alternative\nstate-of-the-art solutions demonstrates its superiority over coverage-guided\nfuzzers while remaining on the same lev"])</script><script>self.__next_f.push([1,"el with advanced hybrid fuzzers.\nFurthermore, we approve the relevance of our approach by discovering 85 new\nreal-world software flaws within the OSS-Sydr-Fuzz project. Finally, we open\nCasr source code to the community to facilitate examination of the existing\ncrashes.60:T4bc,Nowadays automated dynamic analysis frameworks for continuous testing are in\nhigh demand to ensure software safety and satisfy the security development\nlifecycle (SDL) requirements. The security bug hunting efficiency of\ncutting-edge hybrid fuzzing techniques outperforms widely utilized\ncoverage-guided fuzzing. We propose an enhanced dynamic analysis pipeline to\nleverage productivity of automated bug detection based on hybrid fuzzing. We\nimplement the proposed pipeline in the continuous fuzzing toolset Sydr-Fuzz\nwhich is powered by hybrid fuzzing orchestrator, integrating our DSE tool Sydr\nwith libFuzzer and AFL++. Sydr-Fuzz also incorporates security predicate\ncheckers, crash triaging tool Casr, and utilities for corpus minimization and\ncoverage gathering. The benchmarking of our hybrid fuzzer against alternative\nstate-of-the-art solutions demonstrates its superiority over coverage-guided\nfuzzers while remaining on the same level with advanced hybrid fuzzers.\nFurthermore, we approve the relevance of our approach by discovering 85 new\nreal-world software flaws within the OSS-Sydr-Fuzz project. Finally, we open\nCasr source code to the community to facilitate examination of the existing\ncrashes.61:T449,In this paper, we investigate an open research task of generating controllable 3D textured shapes from the given textual descriptions. Previous works either require ground truth caption labeling or extensive optimization time. To resolve these issues, we present a novel framework, TAPS3D, to train a text-guided 3D shape generator with pseudo captions. Specifically, based on rendered 2D images, we retrieve relevant words from the CLIP vocabulary and construct pseudo captions using templates. Our constructed captions provide high-level semantic s"])</script><script>self.__next_f.push([1,"upervision for generated 3D shapes. Further, in order to produce fine-grained textures and increase geometry diversity, we propose to adopt low-level image regularization to enable fake-rendered images to align with the real ones. During the inference phase, our proposed model can generate 3D textured shapes from the given text without any additional optimization. We conduct extensive experiments to analyze each of our proposed components and show the efficacy of our framework in generating high-fidelity 3D textured and text-relevant shapes.62:T449,In this paper, we investigate an open research task of generating controllable 3D textured shapes from the given textual descriptions. Previous works either require ground truth caption labeling or extensive optimization time. To resolve these issues, we present a novel framework, TAPS3D, to train a text-guided 3D shape generator with pseudo captions. Specifically, based on rendered 2D images, we retrieve relevant words from the CLIP vocabulary and construct pseudo captions using templates. Our constructed captions provide high-level semantic supervision for generated 3D shapes. Further, in order to produce fine-grained textures and increase geometry diversity, we propose to adopt low-level image regularization to enable fake-rendered images to align with the real ones. During the inference phase, our proposed model can generate 3D textured shapes from the given text without any additional optimization. We conduct extensive experiments to analyze each of our proposed components and show the efficacy of our framework in generating high-fidelity 3D textured and text-relevant shapes.63:T454,There is a limited amount of publicly available data to support research in malware analysis technology. Particularly, there are virtually no publicly available datasets generated from rich sandboxes such as Cuckoo/CAPE. The benefit of using dynamic sandboxes is the realistic simulation of file execution in the target machine and obtaining a log of such execution. The machine can be in"])</script><script>self.__next_f.push([1,"fected by malware hence there is a good chance of capturing the malicious behavior in the execution logs, thus allowing researchers to study such behavior in detail. Although the subsequent analysis of log information is extensively covered in industrial cybersecurity backends, to our knowledge there has been only limited effort invested in academia to advance such log analysis capabilities using cutting edge techniques. We make this sample dataset available to support designing new machine learning methods for malware detection, especially for automatic detection of generic malicious behavior. The dataset has been collected in cooperation between Avast Software and Czech Technical University - AI Center (AIC).64:T454,There is a limited amount of publicly available data to support research in malware analysis technology. Particularly, there are virtually no publicly available datasets generated from rich sandboxes such as Cuckoo/CAPE. The benefit of using dynamic sandboxes is the realistic simulation of file execution in the target machine and obtaining a log of such execution. The machine can be infected by malware hence there is a good chance of capturing the malicious behavior in the execution logs, thus allowing researchers to study such behavior in detail. Although the subsequent analysis of log information is extensively covered in industrial cybersecurity backends, to our knowledge there has been only limited effort invested in academia to advance such log analysis capabilities using cutting edge techniques. We make this sample dataset available to support designing new machine learning methods for malware detection, especially for automatic detection of generic malicious behavior. The dataset has been collected in cooperation between Avast Software and Czech Technical University - AI Center (AIC).65:T5b9,The Internet has turned the entire world into a small village;this is because\nit has made it possible to share millions of images and videos. However,\nsending and receiving a huge amount of data is consid"])</script><script>self.__next_f.push([1,"ered to be a main\nchallenge. To address this issue, a new algorithm is required to reduce image\nbits and represent the data in a compressed form. Nevertheless, image\ncompression is an important application for transferring large files and\nimages. This requires appropriate and efficient transfers in this field to\nachieve the task and reach the best results. In this work, we propose a new\nalgorithm based on discrete Hermite wavelets transformation (DHWT) that shows\nthe efficiency and quality of the color images. By compressing the color image,\nthis method analyzes it and divides it into approximate coefficients and detail\ncoefficients after adding the wavelets into MATLAB. With Multi-Resolution\nAnalyses (MRA), the appropriate filter is derived, and the mathematical aspects\nprove to be validated by testing a new filter and performing its operation.\nAfter the decomposition of the rows and upon the process of the reconstruction,\ntaking the inverse of the filter and dealing with the columns of the matrix,\nthe original matrix is improved by measuring the parameters of the image to\nachieve the best quality of the resulting image, such as the peak\nsignal-to-noise ratio (PSNR), compression ratio (CR), bits per pixel (BPP), and\nmean square error (MSE).66:T5b9,The Internet has turned the entire world into a small village;this is because\nit has made it possible to share millions of images and videos. However,\nsending and receiving a huge amount of data is considered to be a main\nchallenge. To address this issue, a new algorithm is required to reduce image\nbits and represent the data in a compressed form. Nevertheless, image\ncompression is an important application for transferring large files and\nimages. This requires appropriate and efficient transfers in this field to\nachieve the task and reach the best results. In this work, we propose a new\nalgorithm based on discrete Hermite wavelets transformation (DHWT) that shows\nthe efficiency and quality of the color images. By compressing the color image,\nthis method analyzes it and"])</script><script>self.__next_f.push([1," divides it into approximate coefficients and detail\ncoefficients after adding the wavelets into MATLAB. With Multi-Resolution\nAnalyses (MRA), the appropriate filter is derived, and the mathematical aspects\nprove to be validated by testing a new filter and performing its operation.\nAfter the decomposition of the rows and upon the process of the reconstruction,\ntaking the inverse of the filter and dealing with the columns of the matrix,\nthe original matrix is improved by measuring the parameters of the image to\nachieve the best quality of the resulting image, such as the peak\nsignal-to-noise ratio (PSNR), compression ratio (CR), bits per pixel (BPP), and\nmean square error (MSE).67:T401,To benefit the complementary information between heterogeneous data, we introduce a new Multimodal Transformer (MMFormer) for Remote Sensing (RS) image classification using Hyperspectral Image (HSI) accompanied by another source of data such as Light Detection and Ranging (LiDAR). Compared with traditional Vision Transformer (ViT) lacking inductive biases of convolutions, we first introduce convolutional layers to our MMFormer to tokenize patches from multimodal data of HSI and LiDAR. Then we propose a Multi-scale Multi-head Self-Attention (MSMHSA) module to address the problem of compatibility which often limits to fuse HSI with high spectral resolution and LiDAR with relatively low spatial resolution. The proposed MSMHSA module can incorporate HSI to LiDAR data in a coarse-to-fine manner enabling us to learn a fine-grained representation. Extensive experiments on widely used benchmarks (e.g., Trento and MUUFL) demonstrate the effectiveness and superiority of our proposed MMFormer for RS image classification.68:T401,To benefit the complementary information between heterogeneous data, we introduce a new Multimodal Transformer (MMFormer) for Remote Sensing (RS) image classification using Hyperspectral Image (HSI) accompanied by another source of data such as Light Detection and Ranging (LiDAR). Compared with traditional Vision Transf"])</script><script>self.__next_f.push([1,"ormer (ViT) lacking inductive biases of convolutions, we first introduce convolutional layers to our MMFormer to tokenize patches from multimodal data of HSI and LiDAR. Then we propose a Multi-scale Multi-head Self-Attention (MSMHSA) module to address the problem of compatibility which often limits to fuse HSI with high spectral resolution and LiDAR with relatively low spatial resolution. The proposed MSMHSA module can incorporate HSI to LiDAR data in a coarse-to-fine manner enabling us to learn a fine-grained representation. Extensive experiments on widely used benchmarks (e.g., Trento and MUUFL) demonstrate the effectiveness and superiority of our proposed MMFormer for RS image classification.69:T462,Reinforcement learning has been applied in operation research and has shown promise in solving large combinatorial optimization problems. However, existing works focus on developing neural network architectures for certain problems. These works lack the flexibility to incorporate recent advances in reinforcement learning, as well as the flexibility of customizing model architectures for operation research problems. In this work, we analyze the end-to-end autoregressive models for vehicle routing problems and show that these models can benefit from the recent advances in reinforcement learning with a careful re-implementation of the model architecture. In particular, we re-implemented the Attention Model and trained it with Proximal Policy Optimization (PPO) in CleanRL, showing at least 8 times speed up in training time. We hereby introduce RLOR, a flexible framework for Deep Reinforcement Learning for Operation Research. We believe that a flexible framework is key to developing deep reinforcement learning models for operation research problems. The code of our work is publicly available at this https URL.6a:T462,Reinforcement learning has been applied in operation research and has shown promise in solving large combinatorial optimization problems. However, existing works focus on developing neural network architect"])</script><script>self.__next_f.push([1,"ures for certain problems. These works lack the flexibility to incorporate recent advances in reinforcement learning, as well as the flexibility of customizing model architectures for operation research problems. In this work, we analyze the end-to-end autoregressive models for vehicle routing problems and show that these models can benefit from the recent advances in reinforcement learning with a careful re-implementation of the model architecture. In particular, we re-implemented the Attention Model and trained it with Proximal Policy Optimization (PPO) in CleanRL, showing at least 8 times speed up in training time. We hereby introduce RLOR, a flexible framework for Deep Reinforcement Learning for Operation Research. We believe that a flexible framework is key to developing deep reinforcement learning models for operation research problems. The code of our work is publicly available at this https URL.6b:T523,Although several methods were proposed to address the problem of automated essay scoring (AES) in the last 50 years, there is still much to desire in terms of effectiveness. Large Language Models (LLMs) are transformer-based models that demonstrate extraordinary capabilities on various tasks. In this paper, we test the ability of LLMs, given their powerful linguistic knowledge, to analyze and effectively score written essays. We experimented with two popular LLMs, namely ChatGPT and Llama. We aim to check if these models can do this task and, if so, how their performance is positioned among the state-of-the-art (SOTA) models across two levels, holistically and per individual writing trait. We utilized prompt-engineering tactics in designing four different prompts to bring their maximum potential to this task. Our experiments conducted on the ASAP dataset revealed several interesting observations. First, choosing the right prompt depends highly on the model and nature of the task. Second, the two LLMs exhibited comparable average performance in AES, with a slight advantage for ChatGPT. Finally, despite the p"])</script><script>self.__next_f.push([1,"erformance gap between the two LLMs and SOTA models in terms of predictions, they provide feedback to enhance the quality of the essays, which can potentially help both teachers and students.6c:T523,Although several methods were proposed to address the problem of automated essay scoring (AES) in the last 50 years, there is still much to desire in terms of effectiveness. Large Language Models (LLMs) are transformer-based models that demonstrate extraordinary capabilities on various tasks. In this paper, we test the ability of LLMs, given their powerful linguistic knowledge, to analyze and effectively score written essays. We experimented with two popular LLMs, namely ChatGPT and Llama. We aim to check if these models can do this task and, if so, how their performance is positioned among the state-of-the-art (SOTA) models across two levels, holistically and per individual writing trait. We utilized prompt-engineering tactics in designing four different prompts to bring their maximum potential to this task. Our experiments conducted on the ASAP dataset revealed several interesting observations. First, choosing the right prompt depends highly on the model and nature of the task. Second, the two LLMs exhibited comparable average performance in AES, with a slight advantage for ChatGPT. Finally, despite the performance gap between the two LLMs and SOTA models in terms of predictions, they provide feedback to enhance the quality of the essays, which can potentially help both teachers and students.6d:T488,The higher topological complexity of a space $X$, $\\text{TC}_r(X)$, $r=2,3,\\ldots$, and the topological complexity of a map $f$, $\\text{TC}(f)$, have been introduced by Rudyak and Pavešić, respectively, as natural extensions of Farber's topological complexity of a space. In this paper we introduce a notion of higher topological complexity of a map~$f$, $\\text{TC}_{r,s}(f)$, for $1\\leq s\\leq r\\geq2$, which simultaneously extends Rudyak's and Pavešić's notions. Our unified concept is relevant in the $r$-multitasking mo"])</script><script>self.__next_f.push([1,"tion planning problem associated to a robot devise when the forward kinematics map plays a role in $s$ prescribed stages of the motion task. We study the homotopy invariance and the behavior of $\\text{TC}_{r,s}$ under products and compositions of maps, as well as the dependence of $\\text{TC}_{r,s}$ on $r$ and $s$. We draw general estimates for $\\text{TC}_{r,s}(f\\colon X\\to Y)$ in terms of categorical invariants associated to $X$, $Y$ and $f$. In particular, we describe within one the value of $\\text{TC}_{r,s}$ in the case of the non-trivial double covering over real projective spaces, as well as for their complex counterparts.6e:T488,The higher topological complexity of a space $X$, $\\text{TC}_r(X)$, $r=2,3,\\ldots$, and the topological complexity of a map $f$, $\\text{TC}(f)$, have been introduced by Rudyak and Pavešić, respectively, as natural extensions of Farber's topological complexity of a space. In this paper we introduce a notion of higher topological complexity of a map~$f$, $\\text{TC}_{r,s}(f)$, for $1\\leq s\\leq r\\geq2$, which simultaneously extends Rudyak's and Pavešić's notions. Our unified concept is relevant in the $r$-multitasking motion planning problem associated to a robot devise when the forward kinematics map plays a role in $s$ prescribed stages of the motion task. We study the homotopy invariance and the behavior of $\\text{TC}_{r,s}$ under products and compositions of maps, as well as the dependence of $\\text{TC}_{r,s}$ on $r$ and $s$. We draw general estimates for $\\text{TC}_{r,s}(f\\colon X\\to Y)$ in terms of categorical invariants associated to $X$, $Y$ and $f$. In particular, we describe within one the value of $\\text{TC}_{r,s}$ in the case of the non-trivial double covering over real projective spaces, as well as for their complex counterparts.6f:T549,Intrusion detection systems (IDS) for the Internet of Things (IoT) systems\ncan use AI-based models to ensure secure communications. IoT systems tend to\nhave many connected devices producing massive amounts of data with high\ndimensionality"])</script><script>self.__next_f.push([1,", which requires complex models. Complex models have notorious\nproblems such as overfitting, low interpretability, and high computational\ncomplexity. Adding model complexity penalty (i.e., regularization) can ease\noverfitting, but it barely helps interpretability and computational efficiency.\nFeature engineering can solve these issues; hence, it has become critical for\nIDS in large-scale IoT systems to reduce the size and dimensionality of data,\nresulting in less complex models with excellent performance, smaller data\nstorage, and fast detection. This paper proposes a new feature engineering\nmethod called LEMDA (Light feature Engineering based on the Mean Decrease in\nAccuracy). LEMDA applies exponential decay and an optional sensitivity factor\nto select and create the most informative features. The proposed method has\nbeen evaluated and compared to other feature engineering methods using three\nIoT datasets and four AI/ML models. The results show that LEMDA improves the F1\nscore performance of all the IDS models by an average of 34% and reduces the\naverage training and detection times in most cases.70:T549,Intrusion detection systems (IDS) for the Internet of Things (IoT) systems\ncan use AI-based models to ensure secure communications. IoT systems tend to\nhave many connected devices producing massive amounts of data with high\ndimensionality, which requires complex models. Complex models have notorious\nproblems such as overfitting, low interpretability, and high computational\ncomplexity. Adding model complexity penalty (i.e., regularization) can ease\noverfitting, but it barely helps interpretability and computational efficiency.\nFeature engineering can solve these issues; hence, it has become critical for\nIDS in large-scale IoT systems to reduce the size and dimensionality of data,\nresulting in less complex models with excellent performance, smaller data\nstorage, and fast detection. This paper proposes a new feature engineering\nmethod called LEMDA (Light feature Engineering based on the Mean Decrease in\nAccuracy). "])</script><script>self.__next_f.push([1,"LEMDA applies exponential decay and an optional sensitivity factor\nto select and create the most informative features. The proposed method has\nbeen evaluated and compared to other feature engineering methods using three\nIoT datasets and four AI/ML models. The results show that LEMDA improves the F1\nscore performance of all the IDS models by an average of 34% and reduces the\naverage training and detection times in most cases.71:T55c,In this work, we propose a novel end-to-end sinkhorn autoencoder with noise\ngenerator for efficient data collection simulation. Simulating processes that\naim at collecting experimental data is crucial for multiple real-life\napplications, including nuclear medicine, astronomy and high energy physics.\nContemporary methods, such as Monte Carlo algorithms, provide high-fidelity\nresults at a price of high computational cost. Multiple attempts are taken to\nreduce this burden, e.g. using generative approaches based on Generative\nAdversarial Networks or Variational Autoencoders. Although such methods are\nmuch faster, they are often unstable in training and do not allow sampling from\nan entire data distribution. To address these shortcomings, we introduce a\nnovel method dubbed end-to-end Sinkhorn Autoencoder, that leverages sinkhorn\nalgorithm to explicitly align distribution of encoded real data examples and\ngenerated noise. More precisely, we extend autoencoder architecture by adding a\ndeterministic neural network trained to map noise from a known distribution\nonto autoencoder latent space representing data distribution. We optimise the\nentire model jointly. Our method outperforms competing approaches on a\nchallenging dataset of simulation data from Zero Degree Calorimeters of ALICE\nexperiment in LHC. as well as standard benchmarks, such as MNIST and CelebA.72:T55c,In this work, we propose a novel end-to-end sinkhorn autoencoder with noise\ngenerator for efficient data collection simulation. Simulating processes that\naim at collecting experimental data is crucial for multiple real-life\napplicat"])</script><script>self.__next_f.push([1,"ions, including nuclear medicine, astronomy and high energy physics.\nContemporary methods, such as Monte Carlo algorithms, provide high-fidelity\nresults at a price of high computational cost. Multiple attempts are taken to\nreduce this burden, e.g. using generative approaches based on Generative\nAdversarial Networks or Variational Autoencoders. Although such methods are\nmuch faster, they are often unstable in training and do not allow sampling from\nan entire data distribution. To address these shortcomings, we introduce a\nnovel method dubbed end-to-end Sinkhorn Autoencoder, that leverages sinkhorn\nalgorithm to explicitly align distribution of encoded real data examples and\ngenerated noise. More precisely, we extend autoencoder architecture by adding a\ndeterministic neural network trained to map noise from a known distribution\nonto autoencoder latent space representing data distribution. We optimise the\nentire model jointly. Our method outperforms competing approaches on a\nchallenging dataset of simulation data from Zero Degree Calorimeters of ALICE\nexperiment in LHC. as well as standard benchmarks, such as MNIST and CelebA.73:T599,Open-vocabulary detection (OVD) is an object detection task aiming at detecting objects from novel categories beyond the base categories on which the detector is trained. Recent OVD methods rely on large-scale visual-language pre-trained models, such as CLIP, for recognizing novel objects. We identify the two core obstacles that need to be tackled when incorporating these models into detector training: (1) the distribution mismatch that happens when applying a VL-model trained on whole images to region recognition tasks; (2) the difficulty of localizing objects of unseen classes. To overcome these obstacles, we propose CORA, a DETR-style framework that adapts CLIP for Open-vocabulary detection by Region prompting and Anchor pre-matching. Region prompting mitigates the whole-to-region distribution gap by prompting the region features of the CLIP-based region classifier. Anchor pre-matchin"])</script><script>self.__next_f.push([1,"g helps learning generalizable object localization by a class-aware matching mechanism. We evaluate CORA on the COCO OVD benchmark, where we achieve 41.7 AP50 on novel classes, which outperforms the previous SOTA by 2.4 AP50 even without resorting to extra training data. When extra training data is available, we train CORA$^+$ on both ground-truth base-category annotations and additional pseudo bounding box labels computed by CORA. CORA$^+$ achieves 43.1 AP50 on the COCO OVD benchmark and 28.1 box APr on the LVIS OVD benchmark.74:T599,Open-vocabulary detection (OVD) is an object detection task aiming at detecting objects from novel categories beyond the base categories on which the detector is trained. Recent OVD methods rely on large-scale visual-language pre-trained models, such as CLIP, for recognizing novel objects. We identify the two core obstacles that need to be tackled when incorporating these models into detector training: (1) the distribution mismatch that happens when applying a VL-model trained on whole images to region recognition tasks; (2) the difficulty of localizing objects of unseen classes. To overcome these obstacles, we propose CORA, a DETR-style framework that adapts CLIP for Open-vocabulary detection by Region prompting and Anchor pre-matching. Region prompting mitigates the whole-to-region distribution gap by prompting the region features of the CLIP-based region classifier. Anchor pre-matching helps learning generalizable object localization by a class-aware matching mechanism. We evaluate CORA on the COCO OVD benchmark, where we achieve 41.7 AP50 on novel classes, which outperforms the previous SOTA by 2.4 AP50 even without resorting to extra training data. When extra training data is available, we train CORA$^+$ on both ground-truth base-category annotations and additional pseudo bounding box labels computed by CORA. CORA$^+$ achieves 43.1 AP50 on the COCO OVD benchmark and 28.1 box APr on the LVIS OVD benchmark.75:T561,The beam squint effect, which manifests in different steering matr"])</script><script>self.__next_f.push([1,"ices in different sub-bands, has been widely considered a challenge in millimeter wave (mmWave) multiinput multi-output (MIMO) channel estimation. Existing methods either require specific forms of the precoding/combining matrix, which restrict their general practicality, or simply ignore the beam squint effect by only making use of a single sub-band for channel estimation. Recognizing that different steering matrices are coupled by the same set of unknown channel parameters, this paper proposes to exploit the common sparsity structure of the virtual channel model so that signals from different subbands can be jointly utilized to enhance the performance of channel estimation. A probabilistic model is built to induce the common sparsity in the spatial domain, and the first-order Taylor expansion is adopted to get rid of the grid mismatch in the dictionaries. To learn the model parameters, a variational expectation-maximization (EM) algorithm is derived, which automatically obtains the balance between the likelihood function and the common sparsity prior information, and is applicable to arbitrary forms of precoding/combining matrices. Simulation results show the superior estimation accuracy of the proposed algorithm over existing methods under different noise powers and system configurations.76:T561,The beam squint effect, which manifests in different steering matrices in different sub-bands, has been widely considered a challenge in millimeter wave (mmWave) multiinput multi-output (MIMO) channel estimation. Existing methods either require specific forms of the precoding/combining matrix, which restrict their general practicality, or simply ignore the beam squint effect by only making use of a single sub-band for channel estimation. Recognizing that different steering matrices are coupled by the same set of unknown channel parameters, this paper proposes to exploit the common sparsity structure of the virtual channel model so that signals from different subbands can be jointly utilized to enhance the performance of"])</script><script>self.__next_f.push([1," channel estimation. A probabilistic model is built to induce the common sparsity in the spatial domain, and the first-order Taylor expansion is adopted to get rid of the grid mismatch in the dictionaries. To learn the model parameters, a variational expectation-maximization (EM) algorithm is derived, which automatically obtains the balance between the likelihood function and the common sparsity prior information, and is applicable to arbitrary forms of precoding/combining matrices. Simulation results show the superior estimation accuracy of the proposed algorithm over existing methods under different noise powers and system configurations.77:T547,Electronic health records (EHRs) store an extensive array of patient\ninformation, encompassing medical histories, diagnoses, treatments, and test\noutcomes. These records are crucial for enabling healthcare providers to make\nwell-informed decisions regarding patient care. Summarizing clinical notes\nfurther assists healthcare professionals in pinpointing potential health risks\nand making better-informed decisions. This process contributes to reducing\nerrors and enhancing patient outcomes by ensuring providers have access to the\nmost pertinent and current patient data. Recent research has shown that\nincorporating prompts with large language models (LLMs) substantially boosts\nthe efficacy of summarization tasks. However, we show that this approach also\nleads to increased output variance, resulting in notably divergent outputs even\nwhen prompts share similar meanings. To tackle this challenge, we introduce a\nmodel-agnostic Soft Prompt-Based Calibration (SPeC) pipeline that employs soft\nprompts to diminish variance while preserving the advantages of prompt-based\nsummarization. Experimental findings on multiple clinical note tasks and LLMs\nindicate that our method not only bolsters performance but also effectively\ncurbs variance for various LLMs, providing a more uniform and dependable\nsolution for summarizing vital medical information.78:T547,Electronic health records (EHRs) "])</script><script>self.__next_f.push([1,"store an extensive array of patient\ninformation, encompassing medical histories, diagnoses, treatments, and test\noutcomes. These records are crucial for enabling healthcare providers to make\nwell-informed decisions regarding patient care. Summarizing clinical notes\nfurther assists healthcare professionals in pinpointing potential health risks\nand making better-informed decisions. This process contributes to reducing\nerrors and enhancing patient outcomes by ensuring providers have access to the\nmost pertinent and current patient data. Recent research has shown that\nincorporating prompts with large language models (LLMs) substantially boosts\nthe efficacy of summarization tasks. However, we show that this approach also\nleads to increased output variance, resulting in notably divergent outputs even\nwhen prompts share similar meanings. To tackle this challenge, we introduce a\nmodel-agnostic Soft Prompt-Based Calibration (SPeC) pipeline that employs soft\nprompts to diminish variance while preserving the advantages of prompt-based\nsummarization. Experimental findings on multiple clinical note tasks and LLMs\nindicate that our method not only bolsters performance but also effectively\ncurbs variance for various LLMs, providing a more uniform and dependable\nsolution for summarizing vital medical information.79:T684,The complexity and ambiguity of financial and economic systems, along with frequent changes in the economic environment, have made it difficult to make precise predictions that are supported by theory-consistent explanations. Interpreting the prediction models used for forecasting important macroeconomic indicators is highly valuable for understanding relations among different factors, increasing trust towards the prediction models, and making predictions more actionable. In this study, we develop a fundamental-based model for the Canadian-U.S. dollar exchange rate within an interpretative framework. We propose a comprehensive approach using machine learning to predict the exchange rate and employ interpretabilit"])</script><script>self.__next_f.push([1,"y methods to accurately analyze the relationships among macroeconomic variables. Moreover, we implement an ablation study based on the output of the interpretations to improve the predictive accuracy of the models. Our empirical results show that crude oil, as Canada's main commodity export, is the leading factor that determines the exchange rate dynamics with time-varying effects. The changes in the sign and magnitude of the contributions of crude oil to the exchange rate are consistent with significant events in the commodity and energy markets and the evolution of the crude oil trend in Canada. Gold and the TSX stock index are found to be the second and third most important variables that influence the exchange rate. Accordingly, this analysis provides trustworthy and practical insights for policymakers and economists and accurate knowledge about the predictive model's decisions, which are supported by theoretical considerations.7a:T3db5,"])</script><script>self.__next_f.push([1,"# Explaining Exchange Rate Forecasts with Macroeconomic Fundamentals Using Interpretive Machine Learning\n\n## Table of Contents\n- [Introduction](#introduction)\n- [Research Context and Objectives](#research-context-and-objectives)\n- [Methodology](#methodology)\n- [Key Findings](#key-findings)\n- [Economic Interpretation](#economic-interpretation)\n- [Time-Varying Effects](#time-varying-effects)\n- [Model Performance Analysis](#model-performance-analysis)\n- [Practical Implications](#practical-implications)\n- [Conclusion](#conclusion)\n\n## Introduction\n\nExchange rate forecasting is one of the most challenging problems in financial economics. Traditional economic models often struggle to outperform simple random walk forecasts, a phenomenon known as the Meese-Rogoff puzzle. This challenge is particularly significant for commodity currencies like the Canadian dollar (CAD), whose value is heavily influenced by commodity prices and macroeconomic fundamentals.\n\nIn their paper, researchers Davood Pirayesh Neghab, Mucahit Cevik, and M.I.M. Wahab from Toronto Metropolitan University address this challenge by combining machine learning techniques with interpretability methods to create a model that not only forecasts exchange rates accurately but also explains how different macroeconomic variables influence these forecasts.\n\n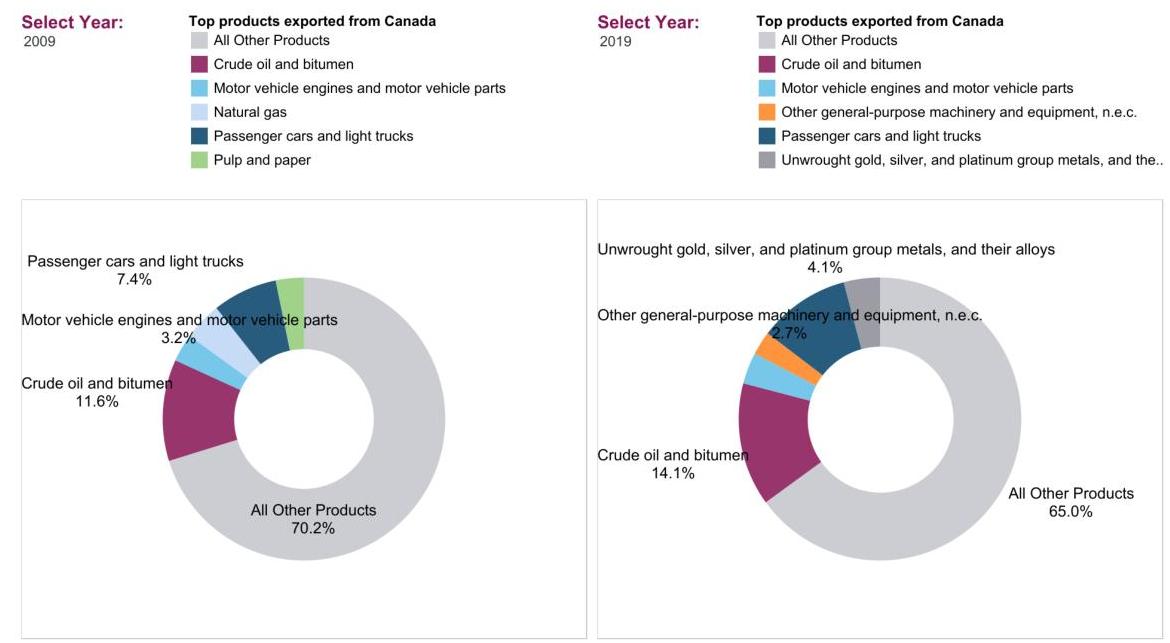\n*Figure 1: Comparison of top products exported from Canada in 2009 and 2019, highlighting the significant role of crude oil in Canada's export economy.*\n\nAs shown in Figure 1, crude oil and bitumen represent a significant portion of Canada's exports (11.6% in 2009 increasing to 14.1% in 2019), making the Canadian dollar a classic example of a \"commodity currency.\" This economic reality provides a theoretical foundation for incorporating oil prices and other macroeconomic fundamentals into exchange rate models.\n\n## Research Context and Objectives\n\nThe paper bridges several active research areas:\n- Exchange rate forecasting using macroeconomic fundamentals\n- Machine learning applications in financial economics\n- Explainable AI (XAI) in finance\n\nTraditional exchange rate models face several challenges:\n1. Nonlinearity in relationships between variables\n2. Multicollinearity among predictors\n3. Noise in financial data\n4. Time-varying relationships\n5. Difficulty in incorporating both backward and forward-looking analyses\n\nMachine learning offers the potential to overcome these challenges by capturing complex nonlinear relationships, but often at the cost of interpretability. The \"black box\" nature of many machine learning models limits their usefulness in economic contexts where understanding relationships is as important as prediction accuracy.\n\n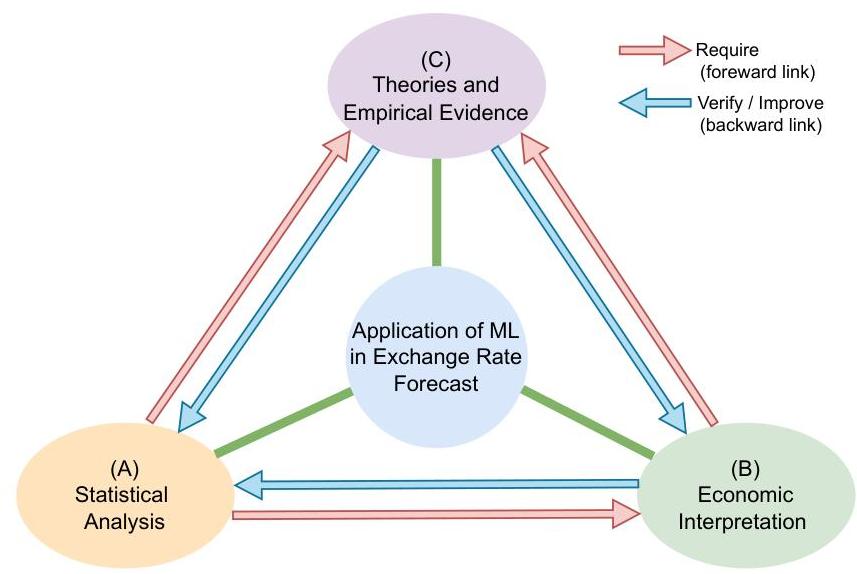\n*Figure 2: The research framework showing the interconnected objectives: Statistical Analysis, Economic Interpretation, and Theories and Empirical Evidence.*\n\nAs illustrated in Figure 2, the authors adopt a comprehensive approach with three interconnected objectives:\n1. **Statistical Analysis**: Developing accurate prediction models\n2. **Economic Interpretation**: Understanding factor relationships\n3. **Theories and Empirical Evidence**: Validating findings against economic theory\n\n## Methodology\n\nThe authors employ a systematic approach to develop their interpretable machine learning framework for exchange rate forecasting:\n\n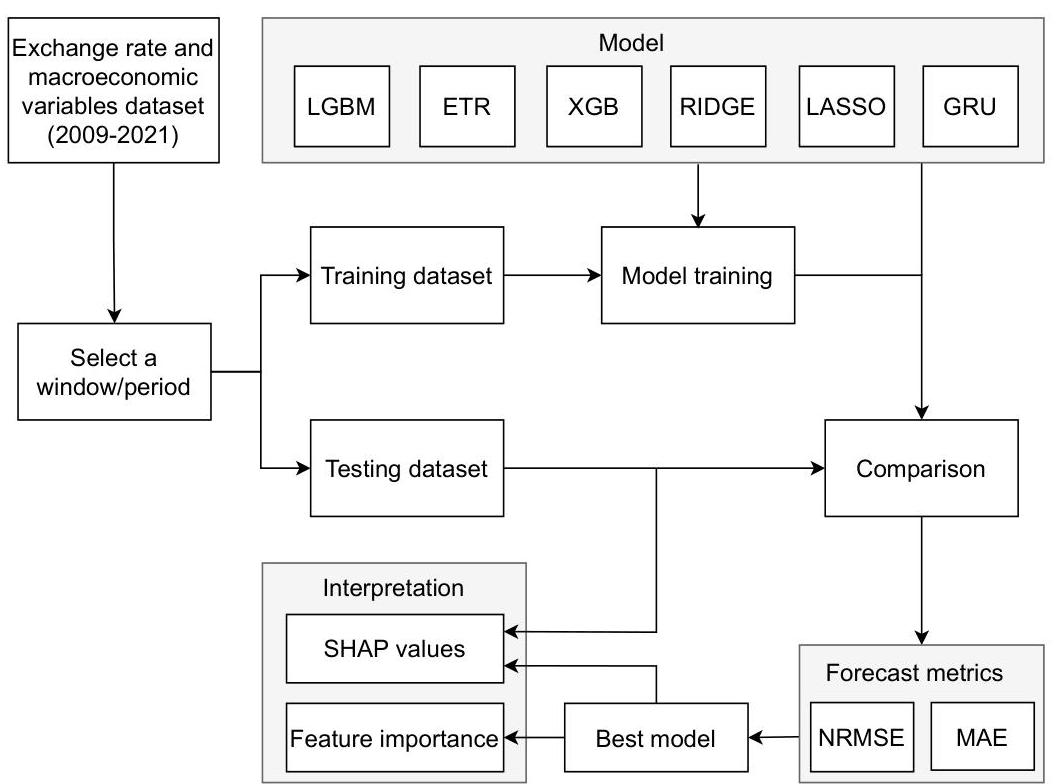\n*Figure 3: The methodological framework showing the workflow from data collection to model interpretation.*\n\n### Data Collection\n\nThe study uses monthly data on the CAD/USD exchange rate and ten macroeconomic variables from January 2009 to December 2021, including:\n- Crude oil prices\n- Gold prices\n- Stock indices (S\u0026P 500, TSX)\n- Interest rates\n- Producer Price Index (PPI)\n- Industrial Production\n- Money Supply (M1)\n- Unemployment Rate\n- Economic Direction (ED)\n\n### Model Selection and Training\n\nThe authors implement and compare several machine learning models:\n- **Linear models**: LASSO and RIDGE regression\n- **Tree-based models**: Extra Trees Regressor (ETR), Extreme Gradient Boosting (XGB), Light Gradient Boosting Machine (LGBM)\n- **Deep learning**: Gated Recurrent Unit (GRU)\n\nThe data is divided into an 80:20 training-testing split, and a rolling window approach is used for evaluation. The models are assessed across different economic periods:\n- Economic Expansion (2009-2014)\n- Economic Stagnation (2015-2019)\n- Covid (2020-2021)\n\nPerformance metrics include Normalized Root Mean Squared Error (NRMSE) and Mean Absolute Error (MAE).\n\n### Interpretability Methods\n\nTwo key interpretability techniques are employed:\n1. **Feature Importance**: Identifying which variables contribute most to predictions\n2. **SHAP (SHapley Additive exPlanations)**: Quantifying the contribution of each feature to individual predictions\n\n## Key Findings\n\n### Feature Importance and SHAP Analysis\n\n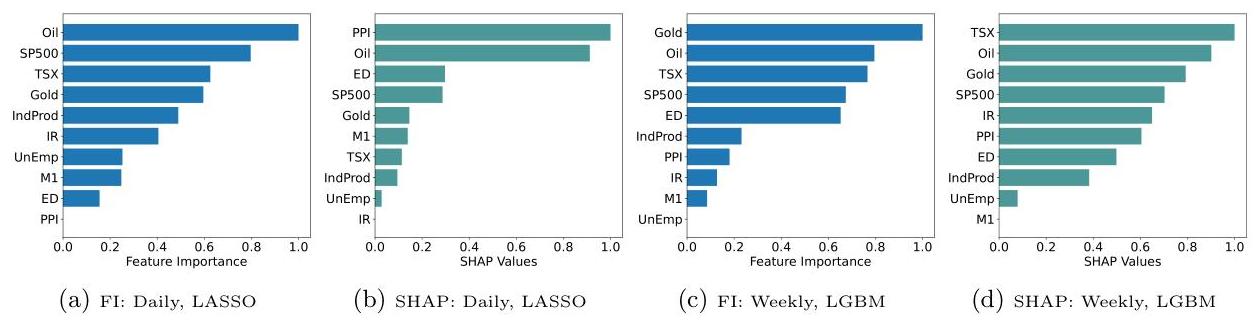\n*Figure 4: Feature importance and SHAP values for daily and weekly models, showing the relative influence of different macroeconomic variables.*\n\nAs shown in Figure 4, both feature importance and SHAP analyses consistently identified several key variables driving exchange rate predictions:\n\n1. **Crude Oil**: Consistently identified as a leading factor influencing the exchange rate\n2. **Gold**: Another significant commodity affecting the CAD/USD rate\n3. **Stock Indices**: Both TSX (Canadian) and S\u0026P 500 (US) indices show substantial influence\n4. **Producer Price Index (PPI)**: Important particularly in certain time periods\n\nThe relative importance of these factors varies between daily and weekly forecasts and across different model types, highlighting the complex, time-varying nature of exchange rate determinants.\n\n## Economic Interpretation\n\nThe findings align well with economic theory regarding commodity currencies. Canada, as a major oil exporter, has an exchange rate that is strongly influenced by oil prices. When oil prices rise, Canada's export revenues increase, creating higher demand for CAD and strengthening it against the USD.\n\nThe significant role of gold prices is also theoretically sound. Gold often acts as a safe-haven asset during periods of uncertainty, and its price movements can influence investor sentiment and capital flows between currencies.\n\nThe importance of stock indices (TSX and S\u0026P 500) reflects the integration of capital markets between the US and Canada. These indices serve as proxies for economic performance and investor sentiment in both countries, affecting capital flows and, consequently, exchange rates.\n\n## Time-Varying Effects\n\nOne of the most valuable insights from the paper is the demonstration of how the influence of different factors varies over time, particularly during significant economic events.\n\n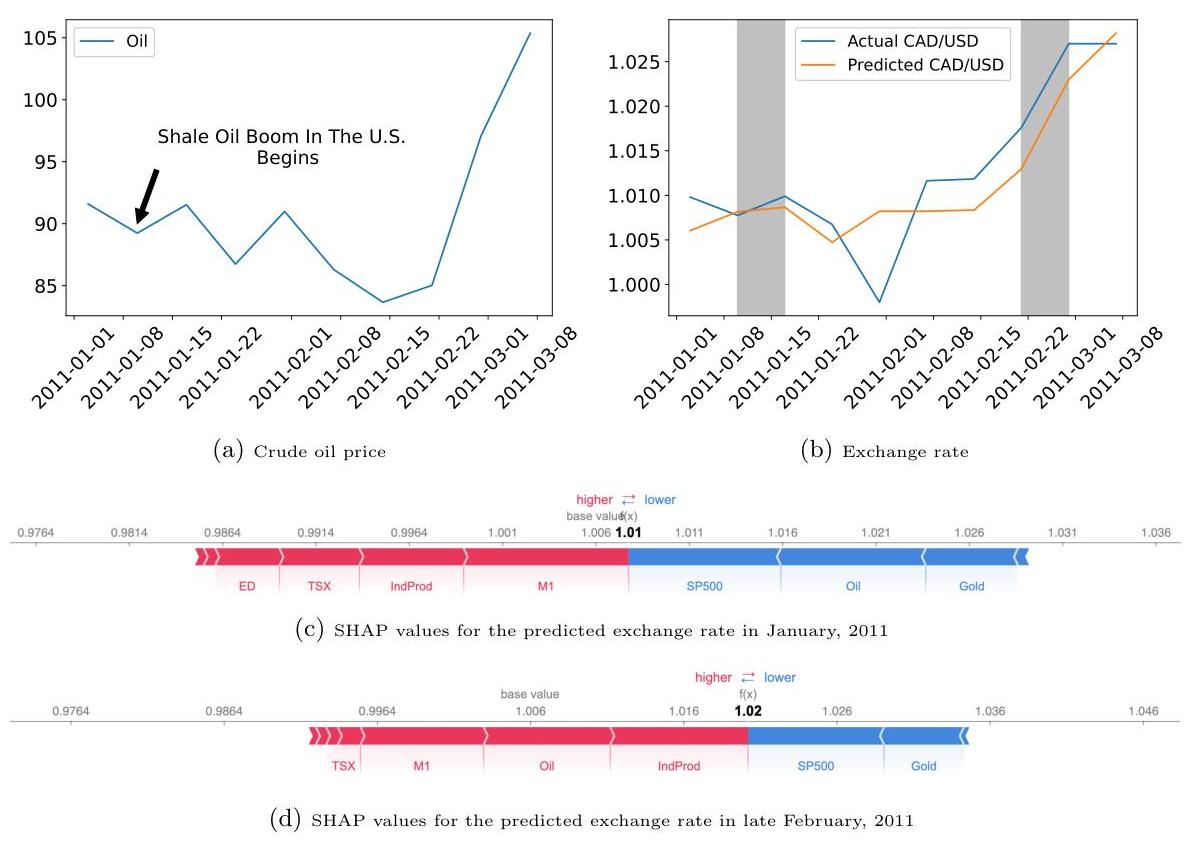\n*Figure 5: The relationship between crude oil prices and CAD/USD exchange rate during the 2011 US shale oil boom, with corresponding SHAP values showing variable impacts.*\n\nFigure 5 illustrates how the US shale oil boom in early 2011 affected the relationship between oil prices and the exchange rate. The SHAP values in panels (c) and (d) show how the contribution of different variables shifted during this period, with oil becoming more influential as its price increased.\n\n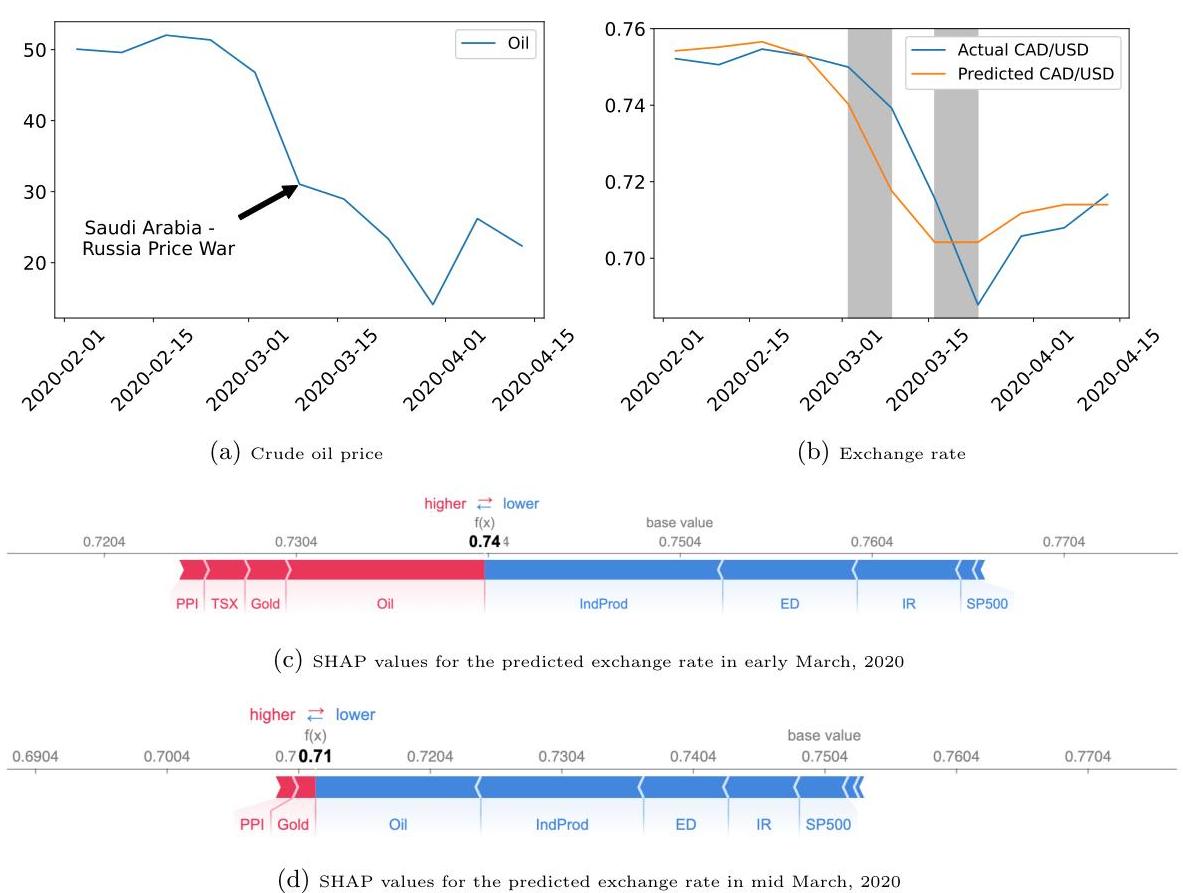\n*Figure 6: The relationship between crude oil prices and CAD/USD exchange rate during the 2020 Saudi Arabia-Russia price war, with corresponding SHAP values.*\n\nSimilarly, Figure 6 demonstrates the impact of the Saudi Arabia-Russia price war in March 2020, which coincided with the beginning of the COVID-19 pandemic. The dramatic drop in oil prices led to a corresponding depreciation of the Canadian dollar, and the SHAP values show how oil's contribution to the exchange rate prediction shifted during this period.\n\nThese examples highlight a key advantage of the interpretable machine learning approach: it can capture and explain complex, time-varying relationships that traditional economic models might miss.\n\n## Model Performance Analysis\n\nThe authors conducted a comprehensive ablation study to assess how different combinations of input variables affect model performance. This analysis revealed several important insights:\n\n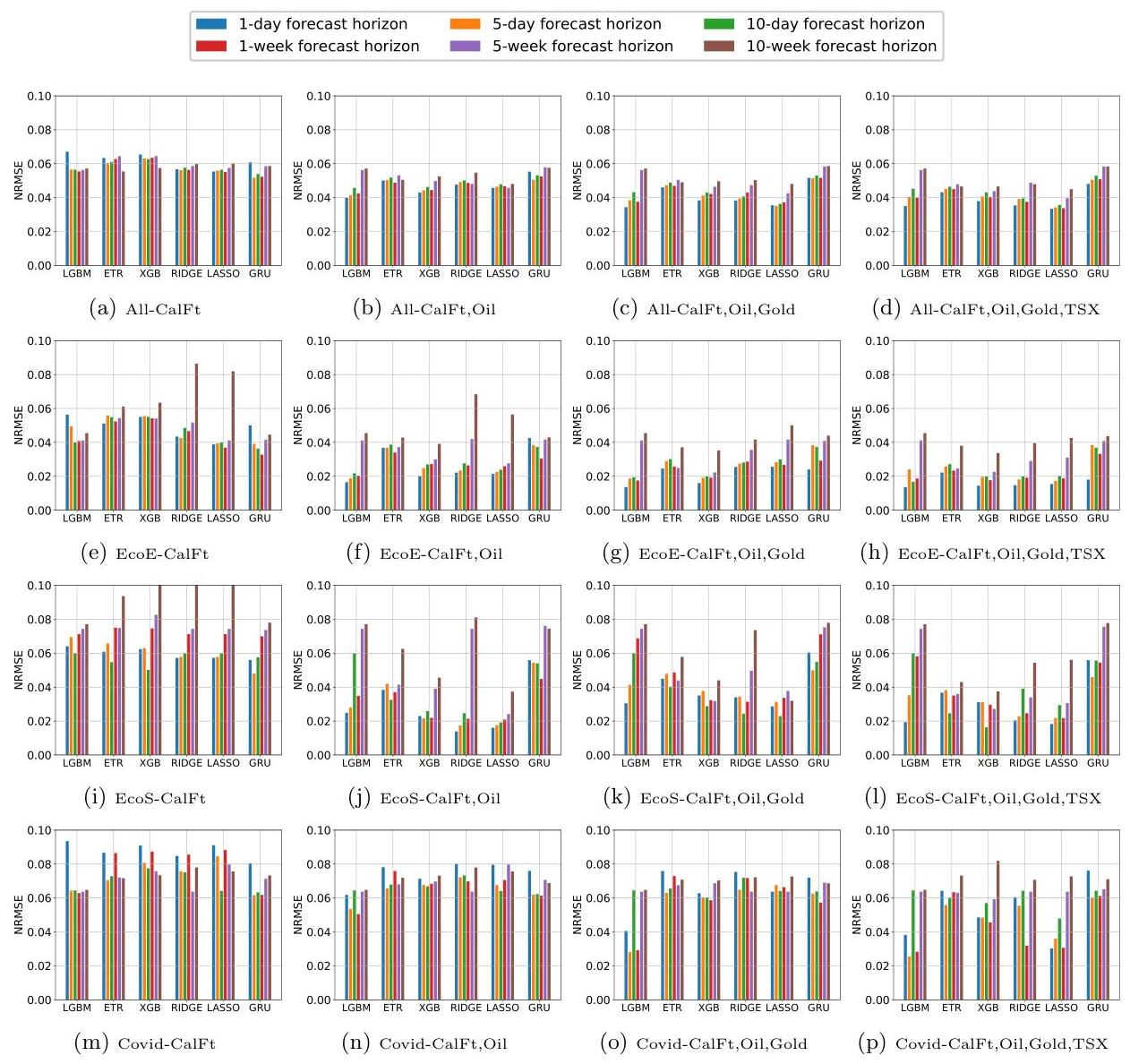\n*Figure 7: Comparison of model performance across different economic periods and variable combinations.*\n\nAs shown in Figure 7, the model performance varied significantly across different economic periods and with different combinations of input variables:\n\n1. **Tree-based models** (LGBM, ETR, XGB) generally outperformed linear models and the GRU deep learning model across most scenarios.\n\n2. **Including key commodities** (oil and gold) substantially improved forecast accuracy, particularly during the Economic Stagnation period.\n\n3. **Adding the TSX index** further enhanced performance in many scenarios, confirming the importance of stock market indicators.\n\n4. **During the COVID-19 period**, linear models (particularly RIDGE) showed improved performance relative to tree-based models, suggesting that the extreme volatility of this period might have simplified some relationships.\n\n5. **Forecast horizon** impacts model performance, with shorter horizons generally yielding better results, though the pattern varies across models and economic periods.\n\nAn interesting finding is that selectively removing some variables that showed low importance actually improved model performance in many cases, highlighting the value of feature selection in exchange rate forecasting.\n\n## Practical Implications\n\nThe research has several important practical implications:\n\n1. **For Policymakers**: Understanding how different macroeconomic factors influence the exchange rate can help in designing more effective monetary and fiscal policies. The time-varying nature of these relationships is particularly important for policy timing.\n\n2. **For Investors and Traders**: The insights about which factors drive exchange rates during different economic conditions can inform trading and hedging strategies. The ability to interpret model predictions adds a layer of confidence that purely black-box approaches lack.\n\n3. **For Economists**: The methodology demonstrates how machine learning can be used in a way that respects economic theory while capturing complex relationships that traditional models might miss.\n\n4. **For Data Scientists**: The paper provides a framework for applying interpretable machine learning to financial time series that balances predictive power with explainability.\n\nThe Canadian economy's dependence on oil exports makes these insights particularly valuable. As shown in Figures 6 and 8, fluctuations in oil production, exports, and prices have significant economic implications beyond just exchange rates.\n\n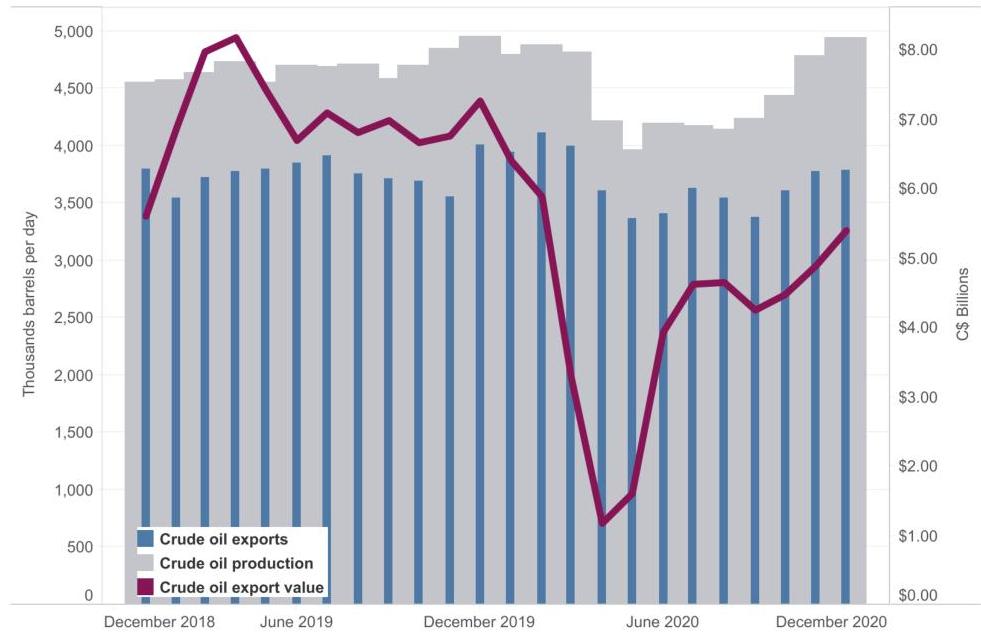\n*Figure 8: Canadian crude oil exports, production, and export value showing the impact of market conditions, particularly during 2020.*\n\n## Conclusion\n\nThis research makes a significant contribution to the field of exchange rate forecasting by successfully bridging machine learning, economic theory, and interpretability. By developing a fundamental-based model within an interpretative machine learning framework, the authors have created an approach that not only predicts the CAD/USD exchange rate with good accuracy but also provides valuable insights into the complex relationships between macroeconomic variables.\n\nKey contributions include:\n\n1. **Methodological Innovation**: Combining statistical rigor, economic theory, and interpretability techniques to create a comprehensive forecasting framework.\n\n2. **Economic Insights**: Confirming and quantifying the importance of crude oil for the Canadian dollar while highlighting how this relationship varies over time and economic conditions.\n\n3. **Model Selection Guidance**: Demonstrating that tree-based models generally outperform other approaches for this task, though performance varies by economic period.\n\n4. **Interpretability**: Showing how techniques like SHAP can make complex machine learning models more transparent and useful in economic contexts.\n\nThe findings have implications for policymakers, investors, and researchers interested in exchange rate dynamics, particularly for commodity currencies. Future research could extend this approach to other currency pairs, develop more specialized interpretability methods for time series data, or incorporate additional variables like sentiment indicators or geopolitical factors.\n\nBy making machine learning models both more accurate and more interpretable, this research helps advance the field of financial economics in a direction that embraces the power of modern analytical techniques while maintaining the economic understanding that gives predictions their context and meaning.\n## Relevant Citations\n\n\n\nFerraro, D., K. Rogoff, and B. Rossi (2015). Can oil prices forecast exchange rates? an empirical analysis of the relationship between commodity prices and exchange rates.Journal of International Money and Finance 54, 116–141.\n\n * This paper empirically investigates the relationship between commodity prices (including crude oil) and exchange rates, providing evidence that crude oil can be a strong predictor for the Canadian exchange rate. It also examines other commodity/exchange rate pairs like gold/South African rand and copper/Chilean peso.\n\nIssa, R., R. Lafrance, and J. Murray (2008). The turning black tide: energy prices and the canadian dollar. Canadian Journal of Economics/Revue Canadienne d’Economique 41(3), 737–759.\n\n * This study examines the relationship between energy prices and the Canadian dollar. It analyzes changes in this relationship using structural break tests and links them to shifts in energy policies and cross-border trade/investment.\n\nBeckmann, J., R. L. Czudaj, and V. Arora (2020). The relationship between oil prices and exchange rates: Revisiting theory and evidence.Energy Economics 88, 104772.\n\n * This paper provides a comprehensive overview of the theoretical and empirical research on the relationship between oil prices and exchange rates, covering multiple perspectives on this complex issue. It revisits the existing theories and empirical findings on the link between oil prices and exchange rates.\n\nChen, S.-S. and H.-C. Chen (2007). Oil prices and real exchange rates.Energy Economics 29(3), 390–404.\n\n * This work investigates the link between oil prices and real exchange rates, providing further context for the dynamic relationship examined in the main paper. It studies the connection between oil prices and real exchange rates across different economies.\n\nZhang, H. J., J.-M. Dufour, and J. W. Galbraith (2016). Exchange rates and commodity prices: Measuring causality at multiple horizons.Journal of Empirical Finance 36, 100–120.\n\n * This paper explores the causal relationship between exchange rates and commodity prices at different time horizons, supporting the main paper's emphasis on the importance of considering time-varying effects in exchange rate modeling. It looks specifically at the impact of commodity prices on exchange rates, using causality tests at multiple horizons.\n\n"])</script><script>self.__next_f.push([1,"7b:T684,The complexity and ambiguity of financial and economic systems, along with frequent changes in the economic environment, have made it difficult to make precise predictions that are supported by theory-consistent explanations. Interpreting the prediction models used for forecasting important macroeconomic indicators is highly valuable for understanding relations among different factors, increasing trust towards the prediction models, and making predictions more actionable. In this study, we develop a fundamental-based model for the Canadian-U.S. dollar exchange rate within an interpretative framework. We propose a comprehensive approach using machine learning to predict the exchange rate and employ interpretability methods to accurately analyze the relationships among macroeconomic variables. Moreover, we implement an ablation study based on the output of the interpretations to improve the predictive accuracy of the models. Our empirical results show that crude oil, as Canada's main commodity export, is the leading factor that determines the exchange rate dynamics with time-varying effects. The changes in the sign and magnitude of the contributions of crude oil to the exchange rate are consistent with significant events in the commodity and energy markets and the evolution of the crude oil trend in Canada. Gold and the TSX stock index are found to be the second and third most important variables that influence the exchange rate. Accordingly, this analysis provides trustworthy and practical insights for policymakers and economists and accurate knowledge about the predictive model's decisions, which are supported by theoretical considerations.7c:T495,To accommodate the growing memory footprints of today's applications, CPU\nvendors have employed large DRAM caches, backed by large non-volatile memories\nlike Intel Optane (e.g., Intel's Cascade Lake). The existing computer\narchitecture simulators do not provide support to model and evaluate systems\nwhich use DRAM devices as a cache to the non-volatile main memor"])</script><script>self.__next_f.push([1,"y. In this\nwork, we present a cycle-level DRAM cache model which is integrated with gem5.\nThis model leverages the flexibility of gem5's memory devices models and full\nsystem support to enable exploration of many different DRAM cache designs. We\ndemonstrate the usefulness of this new tool by exploring the design space of a\nDRAM cache controller through several case studies including the impact of\nscheduling policies, required buffering, combining different memory\ntechnologies (e.g., HBM, DDR3/4/5, 3DXPoint, High latency) as the cache and\nmain memory, and the effect of wear-leveling when DRAM cache is backed by NVM\nmain memory. We also perform experiments with real workloads in full-system\nsimulations to validate the proposed model and show the sensitivity of these\nworkloads to the DRAM cache sizes.7d:T386f,"])</script><script>self.__next_f.push([1,"# A Cycle-level Unified DRAM Cache Controller Model for 3DXPoint Memory Systems in gem5\n\n## Table of Contents\n- [Introduction](#introduction)\n- [Background on DRAM Caches](#background-on-dram-caches)\n- [The Unified DRAM Cache Controller Model](#the-unified-dram-cache-controller-model)\n- [Buffer Design and Request Handling](#buffer-design-and-request-handling)\n- [State Machine Implementation](#state-machine-implementation)\n- [Validation](#validation)\n- [Performance Analysis](#performance-analysis)\n- [Impact of Different Memory Technologies](#impact-of-different-memory-technologies)\n- [Buffer Size Requirements](#buffer-size-requirements)\n- [NVM Performance and Wear Leveling](#nvm-performance-and-wear-leveling)\n- [Real Application Performance](#real-application-performance)\n- [Conclusion](#conclusion)\n\n## Introduction\n\nModern computing systems face increasing memory demands that traditional DRAM alone cannot efficiently satisfy. A promising approach is the use of heterogeneous memory systems that pair DRAM with larger-capacity, non-volatile memories (NVMs) such as Intel's 3DXPoint (Optane). In these systems, DRAM often serves as a hardware-managed cache for the NVM backing store, providing faster access to frequently used data while leveraging the larger capacity of NVM.\n\n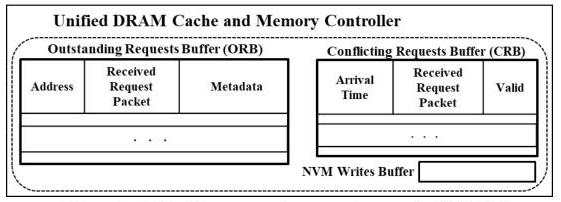\n\nTo effectively research and optimize these heterogeneous memory systems, detailed simulation tools are required. While various memory simulators exist, there has been a notable gap in cycle-level simulation models specifically for DRAM caches backed by NVM. This paper presents a Unified DRAM Cache Controller (UDCC) model implemented in the gem5 simulator, a widely-used open-source platform for computer architecture research.\n\n## Background on DRAM Caches\n\nDRAM caches serve as an intermediate layer between the processor and slower backing memory. They are hardware-managed, meaning the memory controller automatically handles data movement between the DRAM cache and backing memory without explicit software intervention. Unlike traditional CPU caches, DRAM caches operate at the granularity of entire cache lines (typically 64 bytes) and must manage the complexities of DRAM timing constraints and NVM access characteristics.\n\nCommercial implementations of DRAM caches are found in systems like Intel's Cascade Lake and Sapphire Rapids processors, which can use DRAM to cache data stored in Optane memory. These implementations require specialized memory controllers that coordinate access to both the DRAM cache and NVM backing store.\n\n## The Unified DRAM Cache Controller Model\n\nThe UDCC model implemented in gem5 extends the existing memory controller infrastructure to provide a cycle-accurate simulation of a DRAM cache backed by NVM. The model captures critical aspects including:\n\n1. Request buffering and scheduling\n2. DRAM timing constraints\n3. Cache hit/miss handling\n4. Coordinated access to both DRAM and NVM\n\nThe model is designed to be flexible and configurable, allowing researchers to explore different design parameters such as scheduling policies, buffer sizes, memory technologies, and NVM characteristics.\n\n## Buffer Design and Request Handling\n\nThe UDCC implements three key buffer components as shown in Figure 1:\n\n1. **Outstanding Requests Buffer (ORB)**: Stores incoming memory requests along with metadata\n2. **Conflicting Requests Buffer (CRB)**: Manages requests that target the same cache line\n3. **NVM Writes Buffer**: Holds write requests destined for NVM backing storage\n\nThese buffers enable the controller to track and manage multiple concurrent requests while maintaining correctness and optimizing performance. The model supports different scheduling policies, including First-Come-First-Served (FCFS) and First-Ready-First-Come-First-Served (FR-FCFS), which significantly impact performance based on access patterns and hit rates.\n\n## State Machine Implementation\n\nThe UDCC employs a state machine to manage the lifecycle of memory requests. Figure 2 illustrates this state machine:\n\n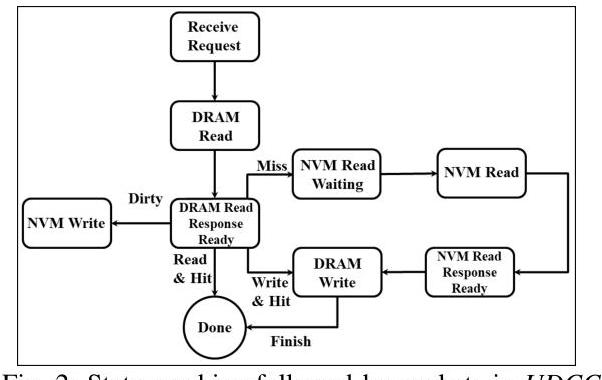\n\nUpon receiving a request, the controller first checks the DRAM cache. For a hit, the data is returned directly. For a miss, the controller must retrieve data from NVM, potentially evicting and writing back dirty data from the DRAM cache. This state machine captures the complex interactions between the DRAM cache and NVM backing store, including handling of read and write operations, cache fills, and evictions.\n\n## Validation\n\nThe authors validated their model against both theoretical expectations and prior research. Figure 7 compares bandwidth measurements between the UDCC, a direct memory controller (DMC), and theoretical peak bandwidth:\n\n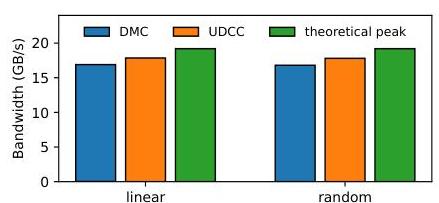\n\nThe model closely approaches theoretical peak bandwidth for both linear and random access patterns, demonstrating its accuracy. Further validation compared the model's request amplification ratios (the number of actual memory accesses generated per processor request) with published data from Hildebrand et al.:\n\n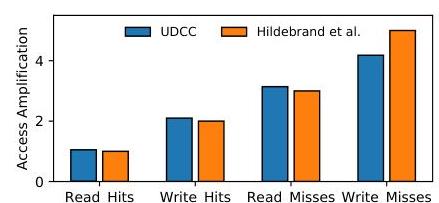\n\nThe consistent results across read hits, write hits, read misses, and write misses confirm that the UDCC model accurately reflects the behavior of real-world DRAM cache implementations.\n\n## Performance Analysis\n\nThe authors conducted extensive experiments to analyze how different parameters affect DRAM cache performance. One significant finding relates to the impact of scheduling policies. Figure 4 compares FCFS and FR-FCFS scheduling under different workloads:\n\n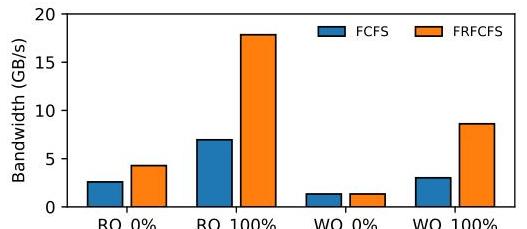\n\nFR-FCFS provides substantial performance benefits for read-only (RO) workloads with high hit rates, showing up to 3x improvement over FCFS when the hit rate is 100%. The benefits are less pronounced for write-only (WO) workloads and workloads with low hit rates, where NVM bandwidth becomes the limiting factor.\n\nFurther analysis examined how cache hit rates affect performance across different scheduling policies. Figure 5 shows bandwidth scaling as hit rates increase:\n\n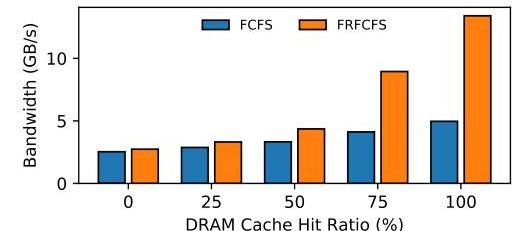\n\nThe performance advantage of FR-FCFS becomes more significant as the hit rate increases, with the greatest benefit at 100% hit rate. This highlights the importance of considering both scheduling policy and expected cache hit rate when designing memory controllers for heterogeneous memory systems.\n\n## Impact of Different Memory Technologies\n\nThe model allows exploration of how different DRAM technologies affect cache performance. Figure 7 compares DDR3, DDR4, and DDR5:\n\n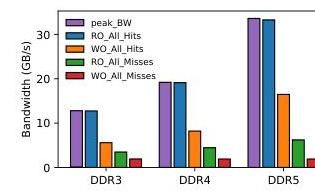\n\nNewer technologies like DDR5 provide higher peak bandwidth, especially for read-heavy workloads with high hit rates. However, the technological benefits diminish for workloads with high miss rates, where NVM access becomes the bottleneck.\n\n## Buffer Size Requirements\n\nAn important design consideration for DRAM cache controllers is buffer sizing. Figure 8 shows the relationship between buffer size requirements and DRAM technology:\n\n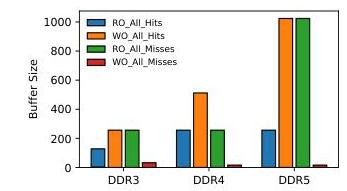\n\nHigher-performing memory technologies like DDR5 require larger buffers to achieve peak performance, particularly for write operations. The authors found that buffer requirements vary significantly based on access patterns and cache hit rates, with some configurations requiring up to 1000 entries to maximize bandwidth.\n\nA more detailed analysis shows the direct relationship between buffer size and bandwidth at different miss rates:\n\n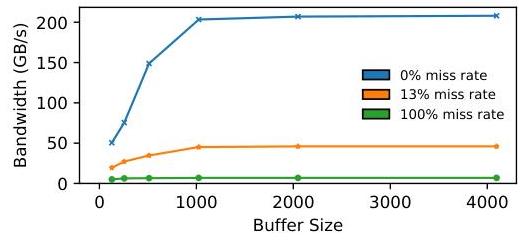\n\nFor workloads with 0% miss rate, bandwidth increases steadily with buffer size until reaching a plateau at approximately 1000 entries. In contrast, workloads with 100% miss rate see minimal benefit from increased buffering, as they are limited by NVM performance.\n\n## NVM Performance and Wear Leveling\n\nThe performance of the NVM backing store significantly impacts overall system performance, especially for workloads with low cache hit rates. Figure 10 compares different NVM configurations:\n\n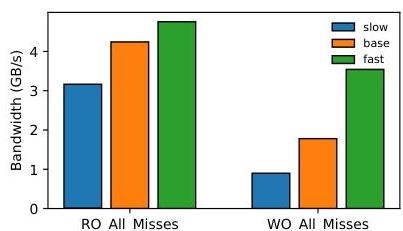\n\nFaster NVM provides substantial benefits for miss-heavy workloads, with the \"fast\" configuration delivering up to 3x higher bandwidth compared to the \"slow\" configuration for read operations with all misses.\n\nThe authors also investigated the impact of NVM wear leveling—a critical feature that distributes writes across the NVM to prevent premature wear-out. They found that while only a small fraction of requests experience wear-leveling delays, these events can have a disproportionate impact on performance in DRAM cache configurations.\n\n## Real Application Performance\n\nTo demonstrate the model's utility for real-world workloads, the authors conducted simulations with both computational benchmarks and data-intensive applications. Figures 11 and 12 show performance and cache miss rates for various applications:\n\n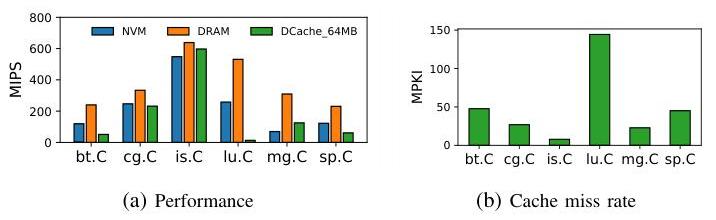\n\n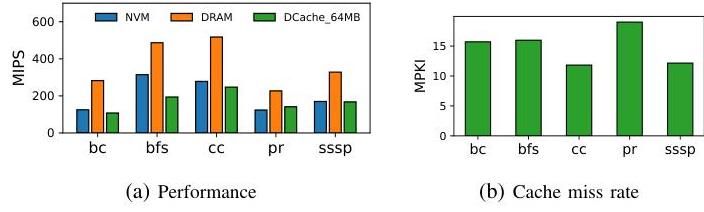\n\nThe results show that applications benefit differently from DRAM caching based on their memory access patterns. For example, the \"lu.C\" benchmark shows high performance with pure DRAM but suffers when using a DRAM cache due to high miss rates (approximately 150 MPKI - misses per thousand instructions). Other applications like \"bt.C\" see modest improvements with DRAM caching due to more favorable access patterns.\n\n## Conclusion\n\nThe Unified DRAM Cache Controller model presented in this paper provides a valuable tool for researching heterogeneous memory systems. The cycle-accurate implementation captures the complex interactions between DRAM caches and NVM backing stores, enabling detailed exploration of design parameters and their impact on performance.\n\nKey findings from the research include:\n\n1. Scheduling policies significantly impact performance, with FR-FCFS providing up to 3x better bandwidth than FCFS for high-hit-rate workloads\n2. Buffer requirements increase with newer DRAM technologies, with DDR5 requiring larger buffers than DDR3/4\n3. NVM performance remains critical for workloads with low cache hit rates\n4. Wear leveling has a disproportionate performance impact in DRAM cache configurations\n5. Application performance with DRAM caches varies widely based on memory access patterns\n\nBy providing this detailed simulation model as an open-source contribution to gem5, the authors enable the research community to further explore and optimize heterogeneous memory systems. This work is particularly timely as commercial systems increasingly adopt heterogeneous memory architectures to address the growing memory demands of data-intensive applications.\n## Relevant Citations\n\n\n\nM. Hildebrand, J. T. Angeles, J. Lowe-Power, and V. Akella, “A case against hardware managed dram caches for nvram based systems,” in2021 IEEE International Symposium on Performance Analysis of Systems and Software (ISPASS). IEEE, 2021, pp. 194–204.\n\n * This paper is highly relevant as it provides a counter-argument to the main paper's focus, highlighting the inefficiencies of hardware-managed DRAM caches for NVRAM-based systems and advocating for manual data movement. It directly motivates the need for detailed cycle-level simulation models like the one proposed in the main paper to better understand the performance trade-offs.\n\nJ. Izraelevitz, J. Yang, L. Zhang, J. Kim, X. Liu, A. Memaripour, Y. J. Soh, Z. Wang, Y. Xu, S. R. Dullooret al., “Basic performance measurements of the intel optane dc persistent memory module,”arXiv preprint arXiv:1903.05714, 2019.\n\n * This citation provides crucial background information on the performance characteristics of Intel Optane DC persistent memory modules, which are relevant to the study as they represent the type of NVRAM technology being considered for use with DRAM caches.\n\nZ. Wang, X. Liu, J. Yang, T. Michailidis, S. Swanson, and J. Zhao, “Characterizing and modeling non-volatile memory systems,” in2020 53rd Annual IEEE/ACM International Symposium on Microarchitecture (MICRO). IEEE, 2020, pp. 496–508.\n\n * This citation introduces VANS, a cycle-level NVRAM simulator. It's relevant because the main paper leverages gem5's NVM model, and discusses plans to integrate VANS for more detailed NVRAM simulation, showing a potential area of future work and comparison for the proposed model.\n\nA. Hansson, N. Agarwal, A. Kolli, T. Wenisch, and A. N. Udipi, “Simulating dram controllers for future system architecture exploration,” in2014 IEEE International Symposium on Performance Analysis of Systems and Software (ISPASS). IEEE, 2014, pp. 201–210.\n\n * This work describes the original implementation of the memory controller model in gem5, which forms the foundation of the proposed DRAM cache controller. The main paper builds upon and extends this work, making it an essential reference for understanding the baseline memory system model in gem5.\n\nW. Elsasser and N. Nikoleris, “Memory controller updates for new DRAM technologies, NVM interfaces and flexible memory topologies,” in3rd gem5 Users’ Workshop with ISCA 2020, 2020.\n\n * This paper describes more recent refactoring of gem5's memory controller, which is important as the UDCC model presented relies on this newer architecture. It explains the separation of memory controllers and interfaces, a key aspect leveraged in the main paper's design.\n\n"])</script><script>self.__next_f.push([1,"7e:T495,To accommodate the growing memory footprints of today's applications, CPU\nvendors have employed large DRAM caches, backed by large non-volatile memories\nlike Intel Optane (e.g., Intel's Cascade Lake). The existing computer\narchitecture simulators do not provide support to model and evaluate systems\nwhich use DRAM devices as a cache to the non-volatile main memory. In this\nwork, we present a cycle-level DRAM cache model which is integrated with gem5.\nThis model leverages the flexibility of gem5's memory devices models and full\nsystem support to enable exploration of many different DRAM cache designs. We\ndemonstrate the usefulness of this new tool by exploring the design space of a\nDRAM cache controller through several case studies including the impact of\nscheduling policies, required buffering, combining different memory\ntechnologies (e.g., HBM, DDR3/4/5, 3DXPoint, High latency) as the cache and\nmain memory, and the effect of wear-leveling when DRAM cache is backed by NVM\nmain memory. We also perform experiments with real workloads in full-system\nsimulations to validate the proposed model and show the sensitivity of these\nworkloads to the DRAM cache sizes.7f:T50c,This paper addresses the problem of constrained multi-objective optimization over black-box objective functions with practitioner-specified preferences over the objectives when a large fraction of the input space is infeasible (i.e., violates constraints). This problem arises in many engineering design problems including analog circuits and electric power system design. Our overall goal is to approximate the optimal Pareto set over the small fraction of feasible input designs. The key challenges include the huge size of the design space, multiple objectives and large number of constraints, and the small fraction of feasible input designs which can be identified only after performing expensive simulations. We propose a novel and efficient preference-aware constrained multi-objective Bayesian optimization approach referred to as PAC-MOO to address t"])</script><script>self.__next_f.push([1,"hese challenges. The key idea is to learn surrogate models for both output objectives and constraints, and select the candidate input for evaluation in each iteration that maximizes the information gained about the optimal constrained Pareto front while factoring in the preferences over objectives. Our experiments on two real-world analog circuit design optimization problems demonstrate the efficacy of PAC-MOO over prior methods.80:T50c,This paper addresses the problem of constrained multi-objective optimization over black-box objective functions with practitioner-specified preferences over the objectives when a large fraction of the input space is infeasible (i.e., violates constraints). This problem arises in many engineering design problems including analog circuits and electric power system design. Our overall goal is to approximate the optimal Pareto set over the small fraction of feasible input designs. The key challenges include the huge size of the design space, multiple objectives and large number of constraints, and the small fraction of feasible input designs which can be identified only after performing expensive simulations. We propose a novel and efficient preference-aware constrained multi-objective Bayesian optimization approach referred to as PAC-MOO to address these challenges. The key idea is to learn surrogate models for both output objectives and constraints, and select the candidate input for evaluation in each iteration that maximizes the information gained about the optimal constrained Pareto front while factoring in the preferences over objectives. Our experiments on two real-world analog circuit design optimization problems demonstrate the efficacy of PAC-MOO over prior methods.81:T505,Purpose: RR Lyrae stars are important distance indicators. They are usually\npresent in globular clusters where they were first discovered. The study of\ntheir properties and distribution in our Galaxy and external galaxies\nconstitutes a modern field of astrophysical research. The aim of this paper is\nchecki"])</script><script>self.__next_f.push([1,"ng the possibility that the observed distribution of RR Lyrae stars in\nthe Galactic bulge derives from orbitally decayed globular clusters (GCs).\nMethods: To reach the aim of the paper I made use of the comparison of\nobservational data of RR Lyrae in the Galactic bulge with the distribution of\nGCs in the Milky Way (MW) as coming from theoretical models under a set of\nassumptions.\nResults: I obtain the expected numbers and distributions of RR Lyrae in the\nGalactic bulge as coming from an initial population of globular clusters at\nvarying some characteristic parameters of the GC population and compare to\nobservational data.\nConclusion: The abundance of RR Lyrae distribution in the Galactic bulge and\ntheir radial distribution is likely still too uncertain to provide a straight\ncomparison with theoretical models. Despite this, it can be stated that a\nsignificant fraction of the `foreground' RR Lyrae present in the MW originate\nfrom orbitally evolved and dissolved GCs.82:T505,Purpose: RR Lyrae stars are important distance indicators. They are usually\npresent in globular clusters where they were first discovered. The study of\ntheir properties and distribution in our Galaxy and external galaxies\nconstitutes a modern field of astrophysical research. The aim of this paper is\nchecking the possibility that the observed distribution of RR Lyrae stars in\nthe Galactic bulge derives from orbitally decayed globular clusters (GCs).\nMethods: To reach the aim of the paper I made use of the comparison of\nobservational data of RR Lyrae in the Galactic bulge with the distribution of\nGCs in the Milky Way (MW) as coming from theoretical models under a set of\nassumptions.\nResults: I obtain the expected numbers and distributions of RR Lyrae in the\nGalactic bulge as coming from an initial population of globular clusters at\nvarying some characteristic parameters of the GC population and compare to\nobservational data.\nConclusion: The abundance of RR Lyrae distribution in the Galactic bulge and\ntheir radial distribution is likely still too un"])</script><script>self.__next_f.push([1,"certain to provide a straight\ncomparison with theoretical models. Despite this, it can be stated that a\nsignificant fraction of the `foreground' RR Lyrae present in the MW originate\nfrom orbitally evolved and dissolved GCs.83:T614,Autonomous and self-driving vehicles are appearing on the public highways. These vehicles commonly use wireless communication techniques for both vehicle-to-vehicle and vehicle-to-infrastructure communications. Manufacturers, regulators and the public are understandably concerned about large-scale systems failure or malicious attack via these wireless vehicular networks. This paper explores the use of sensing and signalling devices that are commonly integrated into modern vehicles for side-channel communication purposes. Visible light (using a CMOS camera) and acoustic (ultrasonic audio) side-channel encoding techniques are proposed, developed and evaluated in this context. The side-channels are examined both theoretically and experimentally and an upper bound on the line code modulation rate that is achievable with these side channel schemes in the vehicular networking context is established. A novel inter-vehicle session key establishment protocol, leveraging both side-channels and a blockchain public key infrastructure, is then presented. In light of the limited channel capacity and the interoperability/security requirements for vehicular communications, techniques for constraining the throughput requirement, providing device independence and validating the location of the intended recipient vehicle, are presented. These reduce the necessary device handshake throughput to 176 bits for creating symmetric encryption and message authentication keys and in verifying a vehicle's certificate with a recognised certification authority.84:T614,Autonomous and self-driving vehicles are appearing on the public highways. These vehicles commonly use wireless communication techniques for both vehicle-to-vehicle and vehicle-to-infrastructure communications. Manufacturers, regulators and the public a"])</script><script>self.__next_f.push([1,"re understandably concerned about large-scale systems failure or malicious attack via these wireless vehicular networks. This paper explores the use of sensing and signalling devices that are commonly integrated into modern vehicles for side-channel communication purposes. Visible light (using a CMOS camera) and acoustic (ultrasonic audio) side-channel encoding techniques are proposed, developed and evaluated in this context. The side-channels are examined both theoretically and experimentally and an upper bound on the line code modulation rate that is achievable with these side channel schemes in the vehicular networking context is established. A novel inter-vehicle session key establishment protocol, leveraging both side-channels and a blockchain public key infrastructure, is then presented. In light of the limited channel capacity and the interoperability/security requirements for vehicular communications, techniques for constraining the throughput requirement, providing device independence and validating the location of the intended recipient vehicle, are presented. These reduce the necessary device handshake throughput to 176 bits for creating symmetric encryption and message authentication keys and in verifying a vehicle's certificate with a recognised certification authority.85:T54e,The best arm identification problem requires identifying the best alternative (i.e., arm) in active experimentation using the smallest number of experiments (i.e., arm pulls), which is crucial for cost-efficient and timely decision-making processes. In the fixed confidence setting, an algorithm must stop data-dependently and return the estimated best arm with a correctness guarantee. Since this stopping time is random, we desire its distribution to have light tails. Unfortunately, many existing studies focus on high probability or in expectation bounds on the stopping time, which allow heavy tails and, for high probability bounds, even not stopping at all. We first prove that this never-stopping event can indeed happen for some"])</script><script>self.__next_f.push([1," popular algorithms. Motivated by this, we propose algorithms that provably enjoy an exponential-tailed stopping time, which improves upon the polynomial tail bound reported by Kalyanakrishnan et al. (2012). The first algorithm is based on a fixed budget algorithm called Sequential Halving along with a doubling trick. The second algorithm is a meta algorithm that takes in any fixed confidence algorithm with a high probability stopping guarantee and turns it into one that enjoys an exponential-tailed stopping time. Our results imply that there is much more to be desired for contemporary fixed confidence algorithms.86:T54e,The best arm identification problem requires identifying the best alternative (i.e., arm) in active experimentation using the smallest number of experiments (i.e., arm pulls), which is crucial for cost-efficient and timely decision-making processes. In the fixed confidence setting, an algorithm must stop data-dependently and return the estimated best arm with a correctness guarantee. Since this stopping time is random, we desire its distribution to have light tails. Unfortunately, many existing studies focus on high probability or in expectation bounds on the stopping time, which allow heavy tails and, for high probability bounds, even not stopping at all. We first prove that this never-stopping event can indeed happen for some popular algorithms. Motivated by this, we propose algorithms that provably enjoy an exponential-tailed stopping time, which improves upon the polynomial tail bound reported by Kalyanakrishnan et al. (2012). The first algorithm is based on a fixed budget algorithm called Sequential Halving along with a doubling trick. The second algorithm is a meta algorithm that takes in any fixed confidence algorithm with a high probability stopping guarantee and turns it into one that enjoys an exponential-tailed stopping time. Our results imply that there is much more to be desired for contemporary fixed confidence algorithms."])</script><script>self.__next_f.push([1,"6:[\"$\",\"$L13\",null,{\"state\":{\"mutations\":[],\"queries\":[{\"state\":{\"data\":[],\"dataUpdateCount\":77,\"dataUpdatedAt\":1744228241606,\"error\":null,\"errorUpdateCount\":0,\"errorUpdatedAt\":0,\"fetchFailureCount\":0,\"fetchFailureReason\":null,\"fetchMeta\":null,\"isInvalidated\":false,\"status\":\"success\",\"fetchStatus\":\"idle\"},\"queryKey\":[\"my_communities\"],\"queryHash\":\"[\\\"my_communities\\\"]\"},{\"state\":{\"data\":null,\"dataUpdateCount\":77,\"dataUpdatedAt\":1744228241606,\"error\":null,\"errorUpdateCount\":0,\"errorUpdatedAt\":0,\"fetchFailureCount\":0,\"fetchFailureReason\":null,\"fetchMeta\":null,\"isInvalidated\":false,\"status\":\"success\",\"fetchStatus\":\"idle\"},\"queryKey\":[\"user\"],\"queryHash\":\"[\\\"user\\\"]\"},{\"state\":{\"data\":{\"data\":{\"paper_version\":{\"_id\":\"67ab1f8162e9208b74ab1ac3\",\"paper_group_id\":\"67ab1f8062e9208b74ab1ac0\",\"version_label\":\"v1\",\"version_order\":1,\"title\":\"Multimodal Adaptive Fusion of Face and Gait Features using Keyless attention based Deep Neural Networks for Human Identification\",\"abstract\":\"Biometrics plays a significant role in vision-based surveillance\\napplications. Soft biometrics such as gait is widely used with face in\\nsurveillance tasks like person recognition and re-identification. Nevertheless,\\nin practical scenarios, classical fusion techniques respond poorly to changes\\nin individual users and in the external environment. To this end, we propose a\\nnovel adaptive multi-biometric fusion strategy for the dynamic incorporation of\\ngait and face biometric cues by leveraging keyless attention deep neural\\nnetworks. Various external factors such as viewpoint and distance to the\\ncamera, are investigated in this study. Extensive experiments have shown\\nsuperior performanceof the proposed model compared with the state-of-the-art\\nmodel.\",\"author_ids\":[\"67ab1f8162e9208b74ab1ac1\",\"67ab1f8162e9208b74ab1ac2\",\"6733f32729b032f3570991fc\",\"6732223ecd1e32a6e7efcf87\"],\"publication_date\":\"2023-03-24T05:28:35.000Z\",\"license\":\"http://arxiv.org/licenses/nonexclusive-distrib/1.0/\",\"created_at\":\"2025-02-11T09:59:29.796Z\",\"updated_at\":\"2025-02-11T09:59:29.796Z\",\"is_deleted\":false,\"is_hidden\":false,\"universal_paper_id\":\"2303.13814\",\"imageURL\":\"image/2303.13814v1.png\"},\"paper_group\":{\"_id\":\"67ab1f8062e9208b74ab1ac0\",\"universal_paper_id\":\"2303.13814\",\"title\":\"Multimodal Adaptive Fusion of Face and Gait Features using Keyless attention based Deep Neural Networks for Human Identification\",\"created_at\":\"2025-02-11T09:59:28.359Z\",\"updated_at\":\"2025-03-03T20:18:35.610Z\",\"categories\":[\"Computer Science\"],\"subcategories\":[\"cs.CV\"],\"custom_categories\":[\"multi-modal-learning\",\"attention-mechanisms\",\"facial-recognition\",\"computer-vision-security\"],\"author_user_ids\":[],\"source\":{\"name\":\"alphaXiv\",\"url\":\"https://arxiv.org/abs/2303.13814\"},\"metrics\":{\"activity_rank\":0,\"questions_count\":0,\"responses_count\":0,\"upvotes_count\":0,\"downvotes_count\":0,\"total_votes\":0,\"public_total_votes\":7,\"visits_count\":{\"last24Hours\":6,\"last7Days\":6,\"last30Days\":7,\"last90Days\":8,\"all\":24},\"weighted_visits\":{\"last24Hours\":9.123594699977585e-129,\"last7Days\":2.3745533235456017e-18,\"last30Days\":0.0003557666200576786,\"last90Days\":0.2963323325848345,\"hot\":2.3745533235456017e-18},\"timeline\":[{\"date\":\"2025-04-03T01:57:35.819Z\",\"views\":20},{\"date\":\"2025-03-30T13:57:35.819Z\",\"views\":2},{\"date\":\"2025-03-27T01:57:35.819Z\",\"views\":0},{\"date\":\"2025-03-23T13:57:35.819Z\",\"views\":0},{\"date\":\"2025-03-20T01:57:35.819Z\",\"views\":0},{\"date\":\"2025-03-16T13:57:35.819Z\",\"views\":4},{\"date\":\"2025-03-13T01:57:35.819Z\",\"views\":2},{\"date\":\"2025-03-09T13:57:35.819Z\",\"views\":0},{\"date\":\"2025-03-06T01:57:35.819Z\",\"views\":2},{\"date\":\"2025-03-02T13:57:35.819Z\",\"views\":2},{\"date\":\"2025-02-27T01:57:35.819Z\",\"views\":2},{\"date\":\"2025-02-23T13:57:35.819Z\",\"views\":2},{\"date\":\"2025-02-20T01:57:35.832Z\",\"views\":2},{\"date\":\"2025-02-16T13:57:35.854Z\",\"views\":2},{\"date\":\"2025-02-13T01:57:35.875Z\",\"views\":1},{\"date\":\"2025-02-09T13:57:35.897Z\",\"views\":3},{\"date\":\"2025-02-06T01:57:35.923Z\",\"views\":2},{\"date\":\"2025-02-02T13:57:35.947Z\",\"views\":0},{\"date\":\"2025-01-30T01:57:35.981Z\",\"views\":1},{\"date\":\"2025-01-26T13:57:36.001Z\",\"views\":0},{\"date\":\"2025-01-23T01:57:36.023Z\",\"views\":1},{\"date\":\"2025-01-19T13:57:36.048Z\",\"views\":1},{\"date\":\"2025-01-16T01:57:36.074Z\",\"views\":1},{\"date\":\"2025-01-12T13:57:36.109Z\",\"views\":0},{\"date\":\"2025-01-09T01:57:36.131Z\",\"views\":1},{\"date\":\"2025-01-05T13:57:36.152Z\",\"views\":0},{\"date\":\"2025-01-02T01:57:36.176Z\",\"views\":1},{\"date\":\"2024-12-29T13:57:36.195Z\",\"views\":1},{\"date\":\"2024-12-26T01:57:36.224Z\",\"views\":0},{\"date\":\"2024-12-22T13:57:36.260Z\",\"views\":1},{\"date\":\"2024-12-19T01:57:36.286Z\",\"views\":2},{\"date\":\"2024-12-15T13:57:36.307Z\",\"views\":2},{\"date\":\"2024-12-12T01:57:36.335Z\",\"views\":2},{\"date\":\"2024-12-08T13:57:36.360Z\",\"views\":2},{\"date\":\"2024-12-05T01:57:36.385Z\",\"views\":0},{\"date\":\"2024-12-01T13:57:36.413Z\",\"views\":1},{\"date\":\"2024-11-28T01:57:36.436Z\",\"views\":1},{\"date\":\"2024-11-24T13:57:36.456Z\",\"views\":0},{\"date\":\"2024-11-21T01:57:36.478Z\",\"views\":0},{\"date\":\"2024-11-17T13:57:36.508Z\",\"views\":2},{\"date\":\"2024-11-14T01:57:36.528Z\",\"views\":2},{\"date\":\"2024-11-10T13:57:36.550Z\",\"views\":2},{\"date\":\"2024-11-07T01:57:36.570Z\",\"views\":0},{\"date\":\"2024-11-03T13:57:36.591Z\",\"views\":0},{\"date\":\"2024-10-31T00:57:36.616Z\",\"views\":1},{\"date\":\"2024-10-27T12:57:36.636Z\",\"views\":2},{\"date\":\"2024-10-24T00:57:36.658Z\",\"views\":2},{\"date\":\"2024-10-20T12:57:36.679Z\",\"views\":0},{\"date\":\"2024-10-17T00:57:36.700Z\",\"views\":2},{\"date\":\"2024-10-13T12:57:36.720Z\",\"views\":0},{\"date\":\"2024-10-10T00:57:36.744Z\",\"views\":1},{\"date\":\"2024-10-06T12:57:36.768Z\",\"views\":0},{\"date\":\"2024-10-03T00:57:36.788Z\",\"views\":1},{\"date\":\"2024-09-29T12:57:36.808Z\",\"views\":1},{\"date\":\"2024-09-26T00:57:36.827Z\",\"views\":2},{\"date\":\"2024-09-22T12:57:36.848Z\",\"views\":0},{\"date\":\"2024-09-19T00:57:36.868Z\",\"views\":0},{\"date\":\"2024-09-15T12:57:36.887Z\",\"views\":1},{\"date\":\"2024-09-12T00:57:36.908Z\",\"views\":1},{\"date\":\"2024-09-08T12:57:36.929Z\",\"views\":2},{\"date\":\"2024-09-05T00:57:36.955Z\",\"views\":1},{\"date\":\"2024-09-01T12:57:36.977Z\",\"views\":0},{\"date\":\"2024-08-29T00:57:36.995Z\",\"views\":1}]},\"is_hidden\":false,\"first_publication_date\":\"2023-03-24T05:28:35.000Z\",\"paperVersions\":{\"_id\":\"67ab1f8162e9208b74ab1ac3\",\"paper_group_id\":\"67ab1f8062e9208b74ab1ac0\",\"version_label\":\"v1\",\"version_order\":1,\"title\":\"Multimodal Adaptive Fusion of Face and Gait Features using Keyless attention based Deep Neural Networks for Human Identification\",\"abstract\":\"Biometrics plays a significant role in vision-based surveillance\\napplications. Soft biometrics such as gait is widely used with face in\\nsurveillance tasks like person recognition and re-identification. Nevertheless,\\nin practical scenarios, classical fusion techniques respond poorly to changes\\nin individual users and in the external environment. To this end, we propose a\\nnovel adaptive multi-biometric fusion strategy for the dynamic incorporation of\\ngait and face biometric cues by leveraging keyless attention deep neural\\nnetworks. Various external factors such as viewpoint and distance to the\\ncamera, are investigated in this study. Extensive experiments have shown\\nsuperior performanceof the proposed model compared with the state-of-the-art\\nmodel.\",\"author_ids\":[\"67ab1f8162e9208b74ab1ac1\",\"67ab1f8162e9208b74ab1ac2\",\"6733f32729b032f3570991fc\",\"6732223ecd1e32a6e7efcf87\"],\"publication_date\":\"2023-03-24T05:28:35.000Z\",\"license\":\"http://arxiv.org/licenses/nonexclusive-distrib/1.0/\",\"created_at\":\"2025-02-11T09:59:29.796Z\",\"updated_at\":\"2025-02-11T09:59:29.796Z\",\"is_deleted\":false,\"is_hidden\":false,\"universal_paper_id\":\"2303.13814\",\"imageURL\":\"image/2303.13814v1.png\"},\"verifiedAuthors\":[],\"authors\":[{\"_id\":\"6732223ecd1e32a6e7efcf87\",\"full_name\":\"Alexandre Bernardino\",\"affiliation\":null,\"orcid\":null,\"semantic_scholarid\":null,\"user_id\":null},{\"_id\":\"6733f32729b032f3570991fc\",\"full_name\":\"Athira Nambiar\",\"affiliation\":null,\"orcid\":null,\"semantic_scholarid\":null,\"user_id\":null},{\"_id\":\"67ab1f8162e9208b74ab1ac1\",\"full_name\":\"Ashwin Prakash\",\"affiliation\":null,\"orcid\":null,\"semantic_scholarid\":null,\"user_id\":null},{\"_id\":\"67ab1f8162e9208b74ab1ac2\",\"full_name\":\"Thejaswin S\",\"affiliation\":null,\"orcid\":null,\"semantic_scholarid\":null,\"user_id\":null}]},\"max_version_order\":1,\"verified_authors\":[],\"authors\":[{\"_id\":\"6732223ecd1e32a6e7efcf87\",\"full_name\":\"Alexandre Bernardino\",\"affiliation\":null,\"orcid\":null,\"semantic_scholarid\":null,\"user_id\":null},{\"_id\":\"6733f32729b032f3570991fc\",\"full_name\":\"Athira Nambiar\",\"affiliation\":null,\"orcid\":null,\"semantic_scholarid\":null,\"user_id\":null},{\"_id\":\"67ab1f8162e9208b74ab1ac1\",\"full_name\":\"Ashwin Prakash\",\"affiliation\":null,\"orcid\":null,\"semantic_scholarid\":null,\"user_id\":null},{\"_id\":\"67ab1f8162e9208b74ab1ac2\",\"full_name\":\"Thejaswin S\",\"affiliation\":null,\"orcid\":null,\"semantic_scholarid\":null,\"user_id\":null}],\"pdf_info\":{\"fetcher_url\":\"https://fetcher.alphaxiv.org/v2/pdf/2303.13814v1\"}}},\"dataUpdateCount\":1,\"dataUpdatedAt\":1744228129063,\"error\":null,\"errorUpdateCount\":0,\"errorUpdatedAt\":0,\"fetchFailureCount\":0,\"fetchFailureReason\":null,\"fetchMeta\":null,\"isInvalidated\":false,\"status\":\"success\",\"fetchStatus\":\"idle\"},\"queryKey\":[\"paper\",\"2303.13814\",\"metadata\"],\"queryHash\":\"[\\\"paper\\\",\\\"2303.13814\\\",\\\"metadata\\\"]\"},{\"state\":{\"data\":{\"data\":[]},\"dataUpdateCount\":1,\"dataUpdatedAt\":1744228129063,\"error\":null,\"errorUpdateCount\":0,\"errorUpdatedAt\":0,\"fetchFailureCount\":0,\"fetchFailureReason\":null,\"fetchMeta\":null,\"isInvalidated\":false,\"status\":\"success\",\"fetchStatus\":\"idle\"},\"queryKey\":[\"paper\",\"2303.13814\",\"comments\"],\"queryHash\":\"[\\\"paper\\\",\\\"2303.13814\\\",\\\"comments\\\"]\"},{\"state\":{\"data\":\"Mozilla/5.0 (Windows NT 6.0; Win64; x64) AppleWebKit/537.36 (KHTML, like Gecko) Chrome/61.0.2060.97 Safari/537.36\",\"dataUpdateCount\":76,\"dataUpdatedAt\":1744228240242,\"error\":null,\"errorUpdateCount\":0,\"errorUpdatedAt\":0,\"fetchFailureCount\":0,\"fetchFailureReason\":null,\"fetchMeta\":null,\"isInvalidated\":false,\"status\":\"success\",\"fetchStatus\":\"idle\"},\"queryKey\":[\"user-agent\"],\"queryHash\":\"[\\\"user-agent\\\"]\"},{\"state\":{\"data\":{\"data\":{\"paper_version\":{\"_id\":\"6792404290464e127b734677\",\"paper_group_id\":\"6792404290464e127b734675\",\"version_label\":\"v3\",\"version_order\":3,\"title\":\"Zero-guidance Segmentation Using Zero Segment Labels\",\"abstract\":\"$14\",\"author_ids\":[\"6792404290464e127b734676\",\"673226d2cd1e32a6e7f01b01\",\"67322a8ccd1e32a6e7f05dce\",\"67322651cd1e32a6e7f01208\"],\"publication_date\":\"2023-09-05T10:50:24.000Z\",\"license\":\"http://creativecommons.org/licenses/by/4.0/\",\"created_at\":\"2025-01-23T13:12:34.982Z\",\"updated_at\":\"2025-01-23T13:12:34.982Z\",\"is_deleted\":false,\"is_hidden\":false,\"universal_paper_id\":\"2303.13396\",\"imageURL\":\"image/2303.13396v3.png\"},\"paper_group\":{\"_id\":\"6792404290464e127b734675\",\"universal_paper_id\":\"2303.13396\",\"title\":\"Zero-guidance Segmentation Using Zero Segment Labels\",\"created_at\":\"2025-01-23T13:12:34.126Z\",\"updated_at\":\"2025-03-03T20:18:37.612Z\",\"categories\":[\"Computer Science\"],\"subcategories\":[\"cs.CV\"],\"custom_categories\":[\"image-segmentation\",\"self-supervised-learning\",\"vision-language-models\",\"attention-mechanisms\",\"zero-shot-learning\"],\"author_user_ids\":[],\"source\":{\"name\":\"alphaXiv\",\"url\":\"https://arxiv.org/paper/2303.13396\"},\"metrics\":{\"activity_rank\":0,\"questions_count\":0,\"responses_count\":0,\"upvotes_count\":0,\"downvotes_count\":0,\"total_votes\":0,\"public_total_votes\":0,\"visits_count\":{\"last24Hours\":0,\"last7Days\":0,\"last30Days\":0,\"last90Days\":3,\"all\":3},\"weighted_visits\":{\"last24Hours\":0,\"last7Days\":0,\"last30Days\":0,\"last90Days\":0.11099659588438043,\"hot\":0},\"timeline\":[{\"date\":\"2025-04-03T01:57:38.300Z\",\"views\":2},{\"date\":\"2025-03-30T13:57:38.300Z\",\"views\":2},{\"date\":\"2025-03-27T01:57:38.300Z\",\"views\":2},{\"date\":\"2025-03-23T13:57:38.300Z\",\"views\":2},{\"date\":\"2025-03-20T01:57:38.300Z\",\"views\":0},{\"date\":\"2025-03-16T13:57:38.300Z\",\"views\":1},{\"date\":\"2025-03-13T01:57:38.300Z\",\"views\":1},{\"date\":\"2025-03-09T13:57:38.300Z\",\"views\":0},{\"date\":\"2025-03-06T01:57:38.300Z\",\"views\":2},{\"date\":\"2025-03-02T13:57:38.300Z\",\"views\":0},{\"date\":\"2025-02-27T01:57:38.300Z\",\"views\":2},{\"date\":\"2025-02-23T13:57:38.300Z\",\"views\":2},{\"date\":\"2025-02-20T01:57:38.311Z\",\"views\":2},{\"date\":\"2025-02-16T13:57:38.334Z\",\"views\":2},{\"date\":\"2025-02-13T01:57:38.369Z\",\"views\":1},{\"date\":\"2025-02-09T13:57:38.392Z\",\"views\":1},{\"date\":\"2025-02-06T01:57:38.413Z\",\"views\":0},{\"date\":\"2025-02-02T13:57:38.436Z\",\"views\":0},{\"date\":\"2025-01-30T01:57:38.457Z\",\"views\":4},{\"date\":\"2025-01-26T13:57:38.478Z\",\"views\":0},{\"date\":\"2025-01-23T01:57:38.498Z\",\"views\":6},{\"date\":\"2025-01-19T13:57:38.522Z\",\"views\":0},{\"date\":\"2025-01-16T01:57:38.541Z\",\"views\":1},{\"date\":\"2025-01-12T13:57:38.565Z\",\"views\":2},{\"date\":\"2025-01-09T01:57:38.583Z\",\"views\":0},{\"date\":\"2025-01-05T13:57:38.608Z\",\"views\":2},{\"date\":\"2025-01-02T01:57:38.632Z\",\"views\":0},{\"date\":\"2024-12-29T13:57:38.653Z\",\"views\":0},{\"date\":\"2024-12-26T01:57:38.688Z\",\"views\":1},{\"date\":\"2024-12-22T13:57:38.710Z\",\"views\":0},{\"date\":\"2024-12-19T01:57:38.730Z\",\"views\":2},{\"date\":\"2024-12-15T13:57:38.763Z\",\"views\":2},{\"date\":\"2024-12-12T01:57:38.785Z\",\"views\":2},{\"date\":\"2024-12-08T13:57:38.807Z\",\"views\":1},{\"date\":\"2024-12-05T01:57:38.841Z\",\"views\":1},{\"date\":\"2024-12-01T13:57:38.861Z\",\"views\":2},{\"date\":\"2024-11-28T01:57:38.895Z\",\"views\":1},{\"date\":\"2024-11-24T13:57:38.917Z\",\"views\":1},{\"date\":\"2024-11-21T01:57:38.953Z\",\"views\":2},{\"date\":\"2024-11-17T13:57:38.978Z\",\"views\":0},{\"date\":\"2024-11-14T01:57:39.002Z\",\"views\":2},{\"date\":\"2024-11-10T13:57:39.026Z\",\"views\":1},{\"date\":\"2024-11-07T01:57:39.053Z\",\"views\":0},{\"date\":\"2024-11-03T13:57:39.075Z\",\"views\":2},{\"date\":\"2024-10-31T00:57:39.102Z\",\"views\":1},{\"date\":\"2024-10-27T12:57:39.122Z\",\"views\":1},{\"date\":\"2024-10-24T00:57:39.148Z\",\"views\":0},{\"date\":\"2024-10-20T12:57:39.169Z\",\"views\":2},{\"date\":\"2024-10-17T00:57:39.194Z\",\"views\":2},{\"date\":\"2024-10-13T12:57:39.213Z\",\"views\":0},{\"date\":\"2024-10-10T00:57:39.245Z\",\"views\":0},{\"date\":\"2024-10-06T12:57:39.268Z\",\"views\":0},{\"date\":\"2024-10-03T00:57:39.289Z\",\"views\":0},{\"date\":\"2024-09-29T12:57:39.316Z\",\"views\":2},{\"date\":\"2024-09-26T00:57:39.343Z\",\"views\":2},{\"date\":\"2024-09-22T12:57:39.367Z\",\"views\":0},{\"date\":\"2024-09-19T00:57:39.408Z\",\"views\":2},{\"date\":\"2024-09-15T12:57:39.428Z\",\"views\":0},{\"date\":\"2024-09-12T00:57:39.448Z\",\"views\":0},{\"date\":\"2024-09-08T12:57:39.474Z\",\"views\":1},{\"date\":\"2024-09-05T00:57:39.495Z\",\"views\":0},{\"date\":\"2024-09-01T12:57:39.521Z\",\"views\":2},{\"date\":\"2024-08-29T00:57:39.536Z\",\"views\":1}]},\"is_hidden\":false,\"first_publication_date\":\"2023-03-23T23:15:07.000Z\",\"paperVersions\":{\"_id\":\"6792404290464e127b734677\",\"paper_group_id\":\"6792404290464e127b734675\",\"version_label\":\"v3\",\"version_order\":3,\"title\":\"Zero-guidance Segmentation Using Zero Segment Labels\",\"abstract\":\"$15\",\"author_ids\":[\"6792404290464e127b734676\",\"673226d2cd1e32a6e7f01b01\",\"67322a8ccd1e32a6e7f05dce\",\"67322651cd1e32a6e7f01208\"],\"publication_date\":\"2023-09-05T10:50:24.000Z\",\"license\":\"http://creativecommons.org/licenses/by/4.0/\",\"created_at\":\"2025-01-23T13:12:34.982Z\",\"updated_at\":\"2025-01-23T13:12:34.982Z\",\"is_deleted\":false,\"is_hidden\":false,\"universal_paper_id\":\"2303.13396\",\"imageURL\":\"image/2303.13396v3.png\"},\"verifiedAuthors\":[],\"authors\":[{\"_id\":\"67322651cd1e32a6e7f01208\",\"full_name\":\"Supasorn Suwajanakorn\",\"affiliation\":null,\"orcid\":null,\"semantic_scholarid\":null,\"user_id\":null},{\"_id\":\"673226d2cd1e32a6e7f01b01\",\"full_name\":\"Nattanat Chatthee\",\"affiliation\":null,\"orcid\":null,\"semantic_scholarid\":null,\"user_id\":null},{\"_id\":\"67322a8ccd1e32a6e7f05dce\",\"full_name\":\"Ekapol Chuangsuwanich\",\"affiliation\":null,\"orcid\":null,\"semantic_scholarid\":null,\"user_id\":null},{\"_id\":\"6792404290464e127b734676\",\"full_name\":\"Pitchaporn Rewatbowornwong\",\"affiliation\":null,\"orcid\":null,\"semantic_scholarid\":null,\"user_id\":null}]},\"max_version_order\":3,\"verified_authors\":[],\"authors\":[{\"_id\":\"67322651cd1e32a6e7f01208\",\"full_name\":\"Supasorn Suwajanakorn\",\"affiliation\":null,\"orcid\":null,\"semantic_scholarid\":null,\"user_id\":null},{\"_id\":\"673226d2cd1e32a6e7f01b01\",\"full_name\":\"Nattanat Chatthee\",\"affiliation\":null,\"orcid\":null,\"semantic_scholarid\":null,\"user_id\":null},{\"_id\":\"67322a8ccd1e32a6e7f05dce\",\"full_name\":\"Ekapol Chuangsuwanich\",\"affiliation\":null,\"orcid\":null,\"semantic_scholarid\":null,\"user_id\":null},{\"_id\":\"6792404290464e127b734676\",\"full_name\":\"Pitchaporn Rewatbowornwong\",\"affiliation\":null,\"orcid\":null,\"semantic_scholarid\":null,\"user_id\":null}],\"pdf_info\":{\"fetcher_url\":\"https://fetcher.alphaxiv.org/v2/pdf/2303.13396v3\"}}},\"dataUpdateCount\":1,\"dataUpdatedAt\":1744228150402,\"error\":null,\"errorUpdateCount\":0,\"errorUpdatedAt\":0,\"fetchFailureCount\":0,\"fetchFailureReason\":null,\"fetchMeta\":null,\"isInvalidated\":false,\"status\":\"success\",\"fetchStatus\":\"idle\"},\"queryKey\":[\"paper\",\"2303.13396\",\"metadata\"],\"queryHash\":\"[\\\"paper\\\",\\\"2303.13396\\\",\\\"metadata\\\"]\"},{\"state\":{\"data\":{\"data\":[]},\"dataUpdateCount\":1,\"dataUpdatedAt\":1744228150402,\"error\":null,\"errorUpdateCount\":0,\"errorUpdatedAt\":0,\"fetchFailureCount\":0,\"fetchFailureReason\":null,\"fetchMeta\":null,\"isInvalidated\":false,\"status\":\"success\",\"fetchStatus\":\"idle\"},\"queryKey\":[\"paper\",\"2303.13396\",\"comments\"],\"queryHash\":\"[\\\"paper\\\",\\\"2303.13396\\\",\\\"comments\\\"]\"},{\"state\":{\"data\":{\"data\":{\"paper_version\":{\"_id\":\"673414c629b032f35709a61f\",\"paper_group_id\":\"673414c429b032f35709a61c\",\"version_label\":\"v3\",\"version_order\":3,\"title\":\"Misleading assertions, unjustified assumptions, and additional\\n limitations of a study by Patone et al., described in the article \\\"Risk of\\n Myocarditis After Sequential Doses of COVID-19 Vaccine and SARS-CoV-2\\n Infection by Age and Sex\\\"\",\"abstract\":\"$16\",\"author_ids\":[\"673414c529b032f35709a61d\",\"673414c529b032f35709a61e\"],\"publication_date\":\"2023-03-23T18:15:12.000Z\",\"license\":\"http://creativecommons.org/licenses/by-nc-nd/4.0/\",\"created_at\":\"2024-11-13T02:53:58.069Z\",\"updated_at\":\"2024-11-13T02:53:58.069Z\",\"is_deleted\":false,\"is_hidden\":false,\"universal_paper_id\":\"2210.14955\",\"imageURL\":\"image/2210.14955v3.png\"},\"paper_group\":{\"_id\":\"673414c429b032f35709a61c\",\"universal_paper_id\":\"2210.14955\",\"source\":{\"name\":\"alphaXiv\",\"url\":\"https://alphaxiv.org/paper/2210.14955\"},\"title\":\"Misleading assertions, unjustified assumptions, and additional\\n limitations of a study by Patone et al., described in the article \\\"Risk of\\n Myocarditis After Sequential Doses of COVID-19 Vaccine and SARS-CoV-2\\n Infection by Age and Sex\\\"\",\"created_at\":\"1970-01-01T00:00:00.000Z\",\"updated_at\":\"2025-03-03T20:18:37.689Z\",\"categories\":[\"Quantitative Biology\"],\"subcategories\":[\"q-bio.QM\"],\"metrics\":{\"activity_rank\":0,\"questions_count\":0,\"responses_count\":0,\"upvotes_count\":null,\"downvotes_count\":0,\"total_votes\":0,\"visits_count\":{\"last24Hours\":0,\"last7Days\":0,\"last30Days\":0,\"last90Days\":1,\"all\":3},\"weighted_visits\":{\"last24Hours\":0,\"last7Days\":0,\"last30Days\":0,\"last90Days\":0.0369646281173831,\"hot\":0},\"public_total_votes\":0,\"timeline\":[{\"date\":\"2025-04-02T02:39:23.843Z\",\"views\":2},{\"date\":\"2025-03-29T14:39:23.843Z\",\"views\":1},{\"date\":\"2025-03-26T02:39:23.843Z\",\"views\":1},{\"date\":\"2025-03-22T14:39:23.843Z\",\"views\":0},{\"date\":\"2025-03-19T02:39:23.843Z\",\"views\":2},{\"date\":\"2025-03-15T14:39:23.843Z\",\"views\":2},{\"date\":\"2025-03-12T02:39:23.843Z\",\"views\":1},{\"date\":\"2025-03-08T14:39:23.843Z\",\"views\":0},{\"date\":\"2025-03-05T02:39:23.843Z\",\"views\":1},{\"date\":\"2025-03-01T14:39:23.843Z\",\"views\":1},{\"date\":\"2025-02-26T02:39:23.843Z\",\"views\":0},{\"date\":\"2025-02-22T14:39:23.843Z\",\"views\":0},{\"date\":\"2025-02-19T02:39:23.854Z\",\"views\":2},{\"date\":\"2025-02-15T14:39:23.865Z\",\"views\":2},{\"date\":\"2025-02-12T02:39:23.882Z\",\"views\":0},{\"date\":\"2025-02-08T14:39:23.898Z\",\"views\":0},{\"date\":\"2025-02-05T02:39:23.915Z\",\"views\":1},{\"date\":\"2025-02-01T14:39:23.931Z\",\"views\":2},{\"date\":\"2025-01-29T02:39:23.947Z\",\"views\":1},{\"date\":\"2025-01-25T14:39:23.963Z\",\"views\":2},{\"date\":\"2025-01-22T02:39:23.979Z\",\"views\":0},{\"date\":\"2025-01-18T14:39:23.999Z\",\"views\":1},{\"date\":\"2025-01-15T02:39:24.016Z\",\"views\":4},{\"date\":\"2025-01-11T14:39:24.039Z\",\"views\":2},{\"date\":\"2025-01-08T02:39:24.062Z\",\"views\":1},{\"date\":\"2025-01-04T14:39:24.078Z\",\"views\":2},{\"date\":\"2025-01-01T02:39:24.091Z\",\"views\":1},{\"date\":\"2024-12-28T14:39:24.110Z\",\"views\":3},{\"date\":\"2024-12-25T02:39:24.130Z\",\"views\":2},{\"date\":\"2024-12-21T14:39:24.146Z\",\"views\":1},{\"date\":\"2024-12-18T02:39:24.163Z\",\"views\":1},{\"date\":\"2024-12-14T14:39:24.179Z\",\"views\":1},{\"date\":\"2024-12-11T02:39:24.198Z\",\"views\":2},{\"date\":\"2024-12-07T14:39:24.213Z\",\"views\":2},{\"date\":\"2024-12-04T02:39:24.231Z\",\"views\":0},{\"date\":\"2024-11-30T14:39:24.249Z\",\"views\":2},{\"date\":\"2024-11-27T02:39:24.264Z\",\"views\":2},{\"date\":\"2024-11-23T14:39:24.280Z\",\"views\":1},{\"date\":\"2024-11-20T02:39:24.297Z\",\"views\":2},{\"date\":\"2024-11-16T14:39:24.313Z\",\"views\":1},{\"date\":\"2024-11-13T02:39:24.326Z\",\"views\":0},{\"date\":\"2024-11-09T14:39:24.344Z\",\"views\":1},{\"date\":\"2024-11-06T02:39:24.366Z\",\"views\":2},{\"date\":\"2024-11-02T13:39:24.388Z\",\"views\":0},{\"date\":\"2024-10-30T01:39:24.403Z\",\"views\":4},{\"date\":\"2024-10-26T13:39:24.419Z\",\"views\":1},{\"date\":\"2024-10-23T01:39:24.434Z\",\"views\":1},{\"date\":\"2024-10-19T13:39:24.562Z\",\"views\":1},{\"date\":\"2024-10-16T01:39:24.587Z\",\"views\":1},{\"date\":\"2024-10-12T13:39:24.607Z\",\"views\":2},{\"date\":\"2024-10-09T01:39:24.631Z\",\"views\":2},{\"date\":\"2024-10-05T13:39:24.700Z\",\"views\":1},{\"date\":\"2024-10-02T01:39:24.715Z\",\"views\":2},{\"date\":\"2024-09-28T13:39:24.746Z\",\"views\":2},{\"date\":\"2024-09-25T01:39:24.762Z\",\"views\":0},{\"date\":\"2024-09-21T13:39:24.777Z\",\"views\":2},{\"date\":\"2024-09-18T01:39:24.792Z\",\"views\":1},{\"date\":\"2024-09-14T13:39:24.808Z\",\"views\":1},{\"date\":\"2024-09-11T01:39:24.823Z\",\"views\":0},{\"date\":\"2024-09-07T13:39:24.839Z\",\"views\":0},{\"date\":\"2024-09-04T01:39:24.858Z\",\"views\":1},{\"date\":\"2024-08-31T13:39:24.876Z\",\"views\":0},{\"date\":\"2024-08-28T01:39:24.890Z\",\"views\":1}]},\"ranking\":{\"current_rank\":60098,\"previous_rank\":26098,\"activity_score\":0,\"paper_score\":0},\"is_hidden\":false,\"custom_categories\":null,\"first_publication_date\":\"2023-03-23T18:15:12.000Z\",\"author_user_ids\":[],\"citation\":{\"bibtex\":\"@Inproceedings{Bourdon2022MisleadingAU,\\n author = {P. Bourdon and S. Pantazatos},\\n title = {Misleading assertions, unjustified assumptions, and additional limitations of a study by Patone et al., described in the article\\\"Risk of Myocarditis After Sequential Doses of COVID-19 Vaccine and SARS-CoV-2 Infection by Age and Sex\\\"},\\n year = {2022}\\n}\\n\"},\"paperVersions\":{\"_id\":\"673414c629b032f35709a61f\",\"paper_group_id\":\"673414c429b032f35709a61c\",\"version_label\":\"v3\",\"version_order\":3,\"title\":\"Misleading assertions, unjustified assumptions, and additional\\n limitations of a study by Patone et al., described in the article \\\"Risk of\\n Myocarditis After Sequential Doses of COVID-19 Vaccine and SARS-CoV-2\\n Infection by Age and Sex\\\"\",\"abstract\":\"$17\",\"author_ids\":[\"673414c529b032f35709a61d\",\"673414c529b032f35709a61e\"],\"publication_date\":\"2023-03-23T18:15:12.000Z\",\"license\":\"http://creativecommons.org/licenses/by-nc-nd/4.0/\",\"created_at\":\"2024-11-13T02:53:58.069Z\",\"updated_at\":\"2024-11-13T02:53:58.069Z\",\"is_deleted\":false,\"is_hidden\":false,\"universal_paper_id\":\"2210.14955\",\"imageURL\":\"image/2210.14955v3.png\"},\"verifiedAuthors\":[],\"authors\":[{\"_id\":\"673414c529b032f35709a61d\",\"full_name\":\"Paul S. Bourdon\",\"affiliation\":null,\"orcid\":null,\"semantic_scholarid\":null,\"user_id\":null},{\"_id\":\"673414c529b032f35709a61e\",\"full_name\":\"Spiro Pantazatos\",\"affiliation\":null,\"orcid\":null,\"semantic_scholarid\":null,\"user_id\":null}]},\"max_version_order\":3,\"verified_authors\":[],\"authors\":[{\"_id\":\"673414c529b032f35709a61d\",\"full_name\":\"Paul S. Bourdon\",\"affiliation\":null,\"orcid\":null,\"semantic_scholarid\":null,\"user_id\":null},{\"_id\":\"673414c529b032f35709a61e\",\"full_name\":\"Spiro Pantazatos\",\"affiliation\":null,\"orcid\":null,\"semantic_scholarid\":null,\"user_id\":null}],\"pdf_info\":{\"fetcher_url\":\"https://fetcher.alphaxiv.org/v2/pdf/2210.14955v3\"}}},\"dataUpdateCount\":1,\"dataUpdatedAt\":1744228170915,\"error\":null,\"errorUpdateCount\":0,\"errorUpdatedAt\":0,\"fetchFailureCount\":0,\"fetchFailureReason\":null,\"fetchMeta\":null,\"isInvalidated\":false,\"status\":\"success\",\"fetchStatus\":\"idle\"},\"queryKey\":[\"paper\",\"2210.14955\",\"metadata\"],\"queryHash\":\"[\\\"paper\\\",\\\"2210.14955\\\",\\\"metadata\\\"]\"},{\"state\":{\"data\":{\"data\":[]},\"dataUpdateCount\":1,\"dataUpdatedAt\":1744228170915,\"error\":null,\"errorUpdateCount\":0,\"errorUpdatedAt\":0,\"fetchFailureCount\":0,\"fetchFailureReason\":null,\"fetchMeta\":null,\"isInvalidated\":false,\"status\":\"success\",\"fetchStatus\":\"idle\"},\"queryKey\":[\"paper\",\"2210.14955\",\"comments\"],\"queryHash\":\"[\\\"paper\\\",\\\"2210.14955\\\",\\\"comments\\\"]\"},{\"state\":{\"data\":{\"data\":{\"paper_version\":{\"_id\":\"67de001d591ba47ea649d3df\",\"paper_group_id\":\"67de001d591ba47ea649d3de\",\"version_label\":\"v2\",\"version_order\":2,\"title\":\"A Large-scale Study of Spatiotemporal Representation Learning with a New Benchmark on Action Recognition\",\"abstract\":\"$18\",\"author_ids\":[\"672bc7c4986a1370676d71c7\",\"672bcb43986a1370676da1c5\",\"672bbf78986a1370676d5ecc\"],\"publication_date\":\"2023-08-18T22:06:04.000Z\",\"license\":\"http://arxiv.org/licenses/nonexclusive-distrib/1.0/\",\"created_at\":\"2025-03-22T00:11:09.077Z\",\"updated_at\":\"2025-03-22T00:11:09.077Z\",\"is_deleted\":false,\"is_hidden\":false,\"universal_paper_id\":\"2303.13505\",\"imageURL\":\"image/2303.13505v2.png\"},\"paper_group\":{\"_id\":\"67de001d591ba47ea649d3de\",\"universal_paper_id\":\"2303.13505\",\"title\":\"A Large-scale Study of Spatiotemporal Representation Learning with a New Benchmark on Action Recognition\",\"created_at\":\"2025-03-22T00:11:09.045Z\",\"updated_at\":\"2025-03-22T00:11:09.045Z\",\"categories\":[\"Computer Science\"],\"subcategories\":[\"cs.CV\"],\"custom_categories\":[\"representation-learning\",\"self-supervised-learning\",\"video-understanding\",\"domain-adaptation\",\"transfer-learning\"],\"author_user_ids\":[],\"source\":{\"name\":\"alphaXiv\",\"url\":\"https://arxiv.org/abs/2303.13505\"},\"metrics\":{\"activity_rank\":0,\"questions_count\":0,\"responses_count\":0,\"upvotes_count\":0,\"downvotes_count\":0,\"total_votes\":0,\"public_total_votes\":2,\"visits_count\":{\"last24Hours\":0,\"last7Days\":1,\"last30Days\":2,\"last90Days\":2,\"all\":2},\"timeline\":[{\"date\":\"2025-04-01T14:45:55.110Z\",\"views\":4},{\"date\":\"2025-03-29T02:45:55.110Z\",\"views\":1},{\"date\":\"2025-03-25T14:45:55.110Z\",\"views\":1},{\"date\":\"2025-03-22T02:45:55.110Z\",\"views\":0},{\"date\":\"2025-03-18T14:45:55.110Z\",\"views\":5},{\"date\":\"2025-03-15T02:45:55.243Z\",\"views\":2},{\"date\":\"2025-03-11T14:45:55.313Z\",\"views\":2},{\"date\":\"2025-03-08T02:45:55.349Z\",\"views\":2},{\"date\":\"2025-03-04T14:45:55.426Z\",\"views\":1},{\"date\":\"2025-03-01T02:45:55.450Z\",\"views\":0},{\"date\":\"2025-02-25T14:45:55.578Z\",\"views\":2},{\"date\":\"2025-02-22T02:45:55.602Z\",\"views\":0},{\"date\":\"2025-02-18T14:45:56.090Z\",\"views\":1},{\"date\":\"2025-02-15T02:45:56.114Z\",\"views\":1},{\"date\":\"2025-02-11T14:45:56.139Z\",\"views\":2},{\"date\":\"2025-02-08T02:45:56.206Z\",\"views\":2},{\"date\":\"2025-02-04T14:45:56.231Z\",\"views\":0},{\"date\":\"2025-02-01T02:45:56.255Z\",\"views\":1},{\"date\":\"2025-01-28T14:45:56.283Z\",\"views\":1},{\"date\":\"2025-01-25T02:45:56.306Z\",\"views\":0},{\"date\":\"2025-01-21T14:45:56.329Z\",\"views\":2},{\"date\":\"2025-01-18T02:45:56.353Z\",\"views\":1},{\"date\":\"2025-01-14T14:45:56.376Z\",\"views\":2},{\"date\":\"2025-01-11T02:45:56.399Z\",\"views\":1},{\"date\":\"2025-01-07T14:45:56.422Z\",\"views\":0},{\"date\":\"2025-01-04T02:45:57.100Z\",\"views\":0},{\"date\":\"2024-12-31T14:45:57.122Z\",\"views\":2},{\"date\":\"2024-12-28T02:45:57.145Z\",\"views\":2},{\"date\":\"2024-12-24T14:45:57.168Z\",\"views\":2},{\"date\":\"2024-12-21T02:45:57.191Z\",\"views\":2},{\"date\":\"2024-12-17T14:45:57.213Z\",\"views\":0},{\"date\":\"2024-12-14T02:45:57.237Z\",\"views\":1},{\"date\":\"2024-12-10T14:45:57.260Z\",\"views\":2},{\"date\":\"2024-12-07T02:45:57.283Z\",\"views\":2},{\"date\":\"2024-12-03T14:45:57.305Z\",\"views\":0},{\"date\":\"2024-11-30T02:45:57.329Z\",\"views\":2},{\"date\":\"2024-11-26T14:45:57.352Z\",\"views\":1},{\"date\":\"2024-11-23T02:45:57.375Z\",\"views\":2},{\"date\":\"2024-11-19T14:45:57.398Z\",\"views\":0},{\"date\":\"2024-11-16T02:45:57.421Z\",\"views\":0},{\"date\":\"2024-11-12T14:45:57.444Z\",\"views\":1},{\"date\":\"2024-11-09T02:45:57.467Z\",\"views\":1},{\"date\":\"2024-11-05T14:45:57.490Z\",\"views\":0},{\"date\":\"2024-11-02T02:45:57.513Z\",\"views\":0},{\"date\":\"2024-10-29T14:45:57.535Z\",\"views\":2},{\"date\":\"2024-10-26T02:45:57.558Z\",\"views\":2},{\"date\":\"2024-10-22T14:45:57.584Z\",\"views\":2},{\"date\":\"2024-10-19T02:45:57.651Z\",\"views\":2},{\"date\":\"2024-10-15T14:45:57.674Z\",\"views\":2},{\"date\":\"2024-10-12T02:45:57.697Z\",\"views\":1},{\"date\":\"2024-10-08T14:45:57.720Z\",\"views\":1},{\"date\":\"2024-10-05T02:45:57.744Z\",\"views\":1},{\"date\":\"2024-10-01T14:45:57.767Z\",\"views\":1},{\"date\":\"2024-09-28T02:45:57.790Z\",\"views\":2},{\"date\":\"2024-09-24T14:45:57.813Z\",\"views\":2},{\"date\":\"2024-09-21T02:45:57.836Z\",\"views\":0}],\"weighted_visits\":{\"last24Hours\":0,\"last7Days\":3.850607503328095e-19,\"last30Days\":0.00010099971647571655,\"last90Days\":0.07392534874330627,\"hot\":3.850607503328095e-19}},\"is_hidden\":false,\"first_publication_date\":\"2023-03-23T17:58:05.000Z\",\"organizations\":[\"67be6389aa92218ccd8b1590\"],\"paperVersions\":{\"_id\":\"67de001d591ba47ea649d3df\",\"paper_group_id\":\"67de001d591ba47ea649d3de\",\"version_label\":\"v2\",\"version_order\":2,\"title\":\"A Large-scale Study of Spatiotemporal Representation Learning with a New Benchmark on Action Recognition\",\"abstract\":\"$19\",\"author_ids\":[\"672bc7c4986a1370676d71c7\",\"672bcb43986a1370676da1c5\",\"672bbf78986a1370676d5ecc\"],\"publication_date\":\"2023-08-18T22:06:04.000Z\",\"license\":\"http://arxiv.org/licenses/nonexclusive-distrib/1.0/\",\"created_at\":\"2025-03-22T00:11:09.077Z\",\"updated_at\":\"2025-03-22T00:11:09.077Z\",\"is_deleted\":false,\"is_hidden\":false,\"universal_paper_id\":\"2303.13505\",\"imageURL\":\"image/2303.13505v2.png\"},\"verifiedAuthors\":[],\"authors\":[{\"_id\":\"672bbf78986a1370676d5ecc\",\"full_name\":\"Chen Chen\",\"affiliation\":null,\"orcid\":null,\"semantic_scholarid\":null,\"user_id\":null},{\"_id\":\"672bc7c4986a1370676d71c7\",\"full_name\":\"Andong Deng\",\"affiliation\":null,\"orcid\":null,\"semantic_scholarid\":null,\"user_id\":\"661890bbbf61076bc3fde213\"},{\"_id\":\"672bcb43986a1370676da1c5\",\"full_name\":\"Taojiannan Yang\",\"affiliation\":null,\"orcid\":null,\"semantic_scholarid\":null,\"user_id\":null}]},\"max_version_order\":2,\"verified_authors\":[],\"authors\":[{\"_id\":\"672bbf78986a1370676d5ecc\",\"full_name\":\"Chen Chen\",\"affiliation\":null,\"orcid\":null,\"semantic_scholarid\":null,\"user_id\":null},{\"_id\":\"672bc7c4986a1370676d71c7\",\"full_name\":\"Andong Deng\",\"affiliation\":null,\"orcid\":null,\"semantic_scholarid\":null,\"user_id\":\"661890bbbf61076bc3fde213\"},{\"_id\":\"672bcb43986a1370676da1c5\",\"full_name\":\"Taojiannan Yang\",\"affiliation\":null,\"orcid\":null,\"semantic_scholarid\":null,\"user_id\":null}],\"pdf_info\":{\"fetcher_url\":\"https://fetcher.alphaxiv.org/v2/pdf/2303.13505v2\"}}},\"dataUpdateCount\":1,\"dataUpdatedAt\":1744228171177,\"error\":null,\"errorUpdateCount\":0,\"errorUpdatedAt\":0,\"fetchFailureCount\":0,\"fetchFailureReason\":null,\"fetchMeta\":null,\"isInvalidated\":false,\"status\":\"success\",\"fetchStatus\":\"idle\"},\"queryKey\":[\"paper\",\"2303.13505\",\"metadata\"],\"queryHash\":\"[\\\"paper\\\",\\\"2303.13505\\\",\\\"metadata\\\"]\"},{\"state\":{\"data\":{\"data\":[]},\"dataUpdateCount\":1,\"dataUpdatedAt\":1744228171177,\"error\":null,\"errorUpdateCount\":0,\"errorUpdatedAt\":0,\"fetchFailureCount\":0,\"fetchFailureReason\":null,\"fetchMeta\":null,\"isInvalidated\":false,\"status\":\"success\",\"fetchStatus\":\"idle\"},\"queryKey\":[\"paper\",\"2303.13505\",\"comments\"],\"queryHash\":\"[\\\"paper\\\",\\\"2303.13505\\\",\\\"comments\\\"]\"},{\"state\":{\"data\":{\"data\":{\"paper_version\":{\"_id\":\"67f51eebfecfcd081bd601dc\",\"paper_group_id\":\"67f51ee9fecfcd081bd601db\",\"version_label\":\"v2\",\"version_order\":2,\"title\":\"6D Heterotic Little String Theories and F-theory Geometry: An Introduction\",\"abstract\":\"$1a\",\"author_ids\":[\"673477b993ee43749600e401\",\"6773a9f9890de0f04d4358f4\",\"67d39fa569b2b4176893f07c\"],\"publication_date\":\"2023-03-24T14:29:45.000Z\",\"license\":\"http://arxiv.org/licenses/nonexclusive-distrib/1.0/\",\"created_at\":\"2025-04-08T13:04:43.066Z\",\"updated_at\":\"2025-04-08T13:04:43.066Z\",\"is_deleted\":false,\"is_hidden\":false,\"universal_paper_id\":\"2303.13502\",\"imageURL\":\"image/2303.13502v2.png\"},\"paper_group\":{\"_id\":\"67f51ee9fecfcd081bd601db\",\"universal_paper_id\":\"2303.13502\",\"title\":\"6D Heterotic Little String Theories and F-theory Geometry: An Introduction\",\"created_at\":\"2025-04-08T13:04:41.506Z\",\"updated_at\":\"2025-04-08T13:04:41.506Z\",\"categories\":[\"Physics\"],\"subcategories\":[\"hep-th\"],\"custom_categories\":null,\"author_user_ids\":[],\"source\":{\"name\":\"alphaXiv\",\"url\":\"https://arxiv.org/abs/2303.13502\"},\"metrics\":{\"activity_rank\":0,\"questions_count\":0,\"responses_count\":0,\"upvotes_count\":0,\"downvotes_count\":0,\"total_votes\":0,\"public_total_votes\":3,\"visits_count\":{\"last24Hours\":0,\"last7Days\":2,\"last30Days\":2,\"last90Days\":2,\"all\":2},\"timeline\":[{\"date\":\"2025-04-05T02:54:42.380Z\",\"views\":6},{\"date\":\"2025-04-01T14:54:42.381Z\",\"views\":0},{\"date\":\"2025-03-29T02:54:42.382Z\",\"views\":2},{\"date\":\"2025-03-25T14:54:42.383Z\",\"views\":1},{\"date\":\"2025-03-22T02:54:42.383Z\",\"views\":2},{\"date\":\"2025-03-18T14:54:42.384Z\",\"views\":2},{\"date\":\"2025-03-15T02:54:42.384Z\",\"views\":0},{\"date\":\"2025-03-11T14:54:42.385Z\",\"views\":0},{\"date\":\"2025-03-08T02:54:42.385Z\",\"views\":0},{\"date\":\"2025-03-04T14:54:42.386Z\",\"views\":2},{\"date\":\"2025-03-01T02:54:42.386Z\",\"views\":2},{\"date\":\"2025-02-25T14:54:42.387Z\",\"views\":2},{\"date\":\"2025-02-22T02:54:42.387Z\",\"views\":2},{\"date\":\"2025-02-18T14:54:42.388Z\",\"views\":0},{\"date\":\"2025-02-15T02:54:42.389Z\",\"views\":0},{\"date\":\"2025-02-11T14:54:42.389Z\",\"views\":0},{\"date\":\"2025-02-08T02:54:42.390Z\",\"views\":2},{\"date\":\"2025-02-04T14:54:42.390Z\",\"views\":2},{\"date\":\"2025-02-01T02:54:42.391Z\",\"views\":2},{\"date\":\"2025-01-28T14:54:42.391Z\",\"views\":2},{\"date\":\"2025-01-25T02:54:42.392Z\",\"views\":2},{\"date\":\"2025-01-21T14:54:42.392Z\",\"views\":0},{\"date\":\"2025-01-18T02:54:42.393Z\",\"views\":2},{\"date\":\"2025-01-14T14:54:42.393Z\",\"views\":0},{\"date\":\"2025-01-11T02:54:42.394Z\",\"views\":2},{\"date\":\"2025-01-07T14:54:42.395Z\",\"views\":2},{\"date\":\"2025-01-04T02:54:42.396Z\",\"views\":0},{\"date\":\"2024-12-31T14:54:42.396Z\",\"views\":1},{\"date\":\"2024-12-28T02:54:42.397Z\",\"views\":2},{\"date\":\"2024-12-24T14:54:42.397Z\",\"views\":2},{\"date\":\"2024-12-21T02:54:42.398Z\",\"views\":0},{\"date\":\"2024-12-17T14:54:42.398Z\",\"views\":0},{\"date\":\"2024-12-14T02:54:42.399Z\",\"views\":1},{\"date\":\"2024-12-10T14:54:42.399Z\",\"views\":0},{\"date\":\"2024-12-07T02:54:42.400Z\",\"views\":1},{\"date\":\"2024-12-03T14:54:42.401Z\",\"views\":0},{\"date\":\"2024-11-30T02:54:42.401Z\",\"views\":1},{\"date\":\"2024-11-26T14:54:42.402Z\",\"views\":0},{\"date\":\"2024-11-23T02:54:42.402Z\",\"views\":0},{\"date\":\"2024-11-19T14:54:42.403Z\",\"views\":0},{\"date\":\"2024-11-16T02:54:42.403Z\",\"views\":1},{\"date\":\"2024-11-12T14:54:42.404Z\",\"views\":2},{\"date\":\"2024-11-09T02:54:42.404Z\",\"views\":1},{\"date\":\"2024-11-05T14:54:42.405Z\",\"views\":1},{\"date\":\"2024-11-02T02:54:42.405Z\",\"views\":0},{\"date\":\"2024-10-29T14:54:42.406Z\",\"views\":0},{\"date\":\"2024-10-26T02:54:42.406Z\",\"views\":2},{\"date\":\"2024-10-22T14:54:42.407Z\",\"views\":1},{\"date\":\"2024-10-19T02:54:42.407Z\",\"views\":2},{\"date\":\"2024-10-15T14:54:42.408Z\",\"views\":0},{\"date\":\"2024-10-12T02:54:42.408Z\",\"views\":1},{\"date\":\"2024-10-08T14:54:42.409Z\",\"views\":2}],\"weighted_visits\":{\"last24Hours\":0,\"last7Days\":6.549921454504403e-19,\"last30Days\":0.00009725488354406391,\"last90Days\":0.07300015851446506,\"hot\":6.549921454504403e-19}},\"is_hidden\":false,\"first_publication_date\":\"2023-03-23T17:57:44.000Z\",\"organizations\":[],\"paperVersions\":{\"_id\":\"67f51eebfecfcd081bd601dc\",\"paper_group_id\":\"67f51ee9fecfcd081bd601db\",\"version_label\":\"v2\",\"version_order\":2,\"title\":\"6D Heterotic Little String Theories and F-theory Geometry: An Introduction\",\"abstract\":\"$1b\",\"author_ids\":[\"673477b993ee43749600e401\",\"6773a9f9890de0f04d4358f4\",\"67d39fa569b2b4176893f07c\"],\"publication_date\":\"2023-03-24T14:29:45.000Z\",\"license\":\"http://arxiv.org/licenses/nonexclusive-distrib/1.0/\",\"created_at\":\"2025-04-08T13:04:43.066Z\",\"updated_at\":\"2025-04-08T13:04:43.066Z\",\"is_deleted\":false,\"is_hidden\":false,\"universal_paper_id\":\"2303.13502\",\"imageURL\":\"image/2303.13502v2.png\"},\"verifiedAuthors\":[],\"authors\":[{\"_id\":\"673477b993ee43749600e401\",\"full_name\":\"Michele Del Zotto\",\"affiliation\":null,\"orcid\":null,\"semantic_scholarid\":null,\"user_id\":null},{\"_id\":\"6773a9f9890de0f04d4358f4\",\"full_name\":\"Muyang Liu\",\"affiliation\":null,\"orcid\":null,\"semantic_scholarid\":null,\"user_id\":null},{\"_id\":\"67d39fa569b2b4176893f07c\",\"full_name\":\"Paul-Konstantin Oehlmann\",\"affiliation\":null,\"orcid\":null,\"semantic_scholarid\":null,\"user_id\":null}]},\"max_version_order\":2,\"verified_authors\":[],\"authors\":[{\"_id\":\"673477b993ee43749600e401\",\"full_name\":\"Michele Del Zotto\",\"affiliation\":null,\"orcid\":null,\"semantic_scholarid\":null,\"user_id\":null},{\"_id\":\"6773a9f9890de0f04d4358f4\",\"full_name\":\"Muyang Liu\",\"affiliation\":null,\"orcid\":null,\"semantic_scholarid\":null,\"user_id\":null},{\"_id\":\"67d39fa569b2b4176893f07c\",\"full_name\":\"Paul-Konstantin Oehlmann\",\"affiliation\":null,\"orcid\":null,\"semantic_scholarid\":null,\"user_id\":null}],\"pdf_info\":{\"fetcher_url\":\"https://fetcher.alphaxiv.org/v2/pdf/2303.13502v2\"}}},\"dataUpdateCount\":1,\"dataUpdatedAt\":1744228171577,\"error\":null,\"errorUpdateCount\":0,\"errorUpdatedAt\":0,\"fetchFailureCount\":0,\"fetchFailureReason\":null,\"fetchMeta\":null,\"isInvalidated\":false,\"status\":\"success\",\"fetchStatus\":\"idle\"},\"queryKey\":[\"paper\",\"2303.13502\",\"metadata\"],\"queryHash\":\"[\\\"paper\\\",\\\"2303.13502\\\",\\\"metadata\\\"]\"},{\"state\":{\"data\":{\"data\":[]},\"dataUpdateCount\":1,\"dataUpdatedAt\":1744228171577,\"error\":null,\"errorUpdateCount\":0,\"errorUpdatedAt\":0,\"fetchFailureCount\":0,\"fetchFailureReason\":null,\"fetchMeta\":null,\"isInvalidated\":false,\"status\":\"success\",\"fetchStatus\":\"idle\"},\"queryKey\":[\"paper\",\"2303.13502\",\"comments\"],\"queryHash\":\"[\\\"paper\\\",\\\"2303.13502\\\",\\\"comments\\\"]\"},{\"state\":{\"data\":{\"data\":{\"paper_version\":{\"_id\":\"677032c590f035bff487a005\",\"paper_group_id\":\"677032c490f035bff487a003\",\"version_label\":\"v1\",\"version_order\":1,\"title\":\"Three material decomposition for spectral computed tomography enabled by block-diagonal step-preconditioning\",\"abstract\":\"$1c\",\"author_ids\":[\"673d020e615941b897fba2bd\",\"672bd37f986a1370676e434e\",\"677032c590f035bff487a004\",\"673d0212615941b897fba2cf\"],\"publication_date\":\"2018-01-19T00:36:02.000Z\",\"license\":\"http://arxiv.org/licenses/nonexclusive-distrib/1.0/\",\"created_at\":\"2024-12-28T17:17:57.219Z\",\"updated_at\":\"2024-12-28T17:17:57.219Z\",\"is_deleted\":false,\"is_hidden\":false,\"universal_paper_id\":\"1801.06263\",\"imageURL\":\"image/1801.06263v1.png\"},\"paper_group\":{\"_id\":\"677032c490f035bff487a003\",\"universal_paper_id\":\"1801.06263\",\"title\":\"Three material decomposition for spectral computed tomography enabled by block-diagonal step-preconditioning\",\"created_at\":\"2024-12-28T17:17:56.507Z\",\"updated_at\":\"2025-03-03T21:13:16.055Z\",\"categories\":[\"Physics\"],\"subcategories\":[\"physics.med-ph\"],\"custom_categories\":null,\"author_user_ids\":[],\"source\":{\"name\":\"alphaXiv\",\"url\":\"https://arxiv.org/paper/1801.06263\"},\"metrics\":{\"activity_rank\":0,\"questions_count\":0,\"responses_count\":0,\"upvotes_count\":0,\"downvotes_count\":0,\"total_votes\":0,\"visits_count\":{\"last24Hours\":0,\"last7Days\":0,\"last30Days\":1,\"last90Days\":3,\"all\":19},\"weighted_visits\":{\"last24Hours\":0,\"last7Days\":0,\"last30Days\":5.755863416306111e-16,\"last90Days\":0.000024955029139521427,\"hot\":0},\"public_total_votes\":0,\"timeline\":[{\"date\":\"2025-03-30T21:17:00.573Z\",\"views\":2},{\"date\":\"2025-03-27T09:17:00.573Z\",\"views\":4},{\"date\":\"2025-03-23T21:17:00.573Z\",\"views\":2},{\"date\":\"2025-03-20T09:17:00.573Z\",\"views\":2},{\"date\":\"2025-03-16T21:17:00.573Z\",\"views\":1},{\"date\":\"2025-03-13T09:17:00.573Z\",\"views\":0},{\"date\":\"2025-03-09T21:17:00.573Z\",\"views\":1},{\"date\":\"2025-03-06T09:17:00.573Z\",\"views\":1},{\"date\":\"2025-03-02T21:17:00.573Z\",\"views\":1},{\"date\":\"2025-02-27T09:17:00.573Z\",\"views\":0},{\"date\":\"2025-02-23T21:17:00.573Z\",\"views\":0},{\"date\":\"2025-02-20T09:17:00.608Z\",\"views\":0},{\"date\":\"2025-02-16T21:17:00.622Z\",\"views\":1},{\"date\":\"2025-02-13T09:17:00.654Z\",\"views\":2},{\"date\":\"2025-02-09T21:17:00.673Z\",\"views\":1},{\"date\":\"2025-02-06T09:17:00.689Z\",\"views\":2},{\"date\":\"2025-02-02T21:17:00.704Z\",\"views\":1},{\"date\":\"2025-01-30T09:17:00.716Z\",\"views\":2},{\"date\":\"2025-01-26T21:17:00.735Z\",\"views\":6},{\"date\":\"2025-01-23T09:17:00.751Z\",\"views\":0},{\"date\":\"2025-01-19T21:17:00.771Z\",\"views\":1},{\"date\":\"2025-01-16T09:17:00.792Z\",\"views\":2},{\"date\":\"2025-01-12T21:17:00.814Z\",\"views\":2},{\"date\":\"2025-01-09T09:17:00.838Z\",\"views\":0},{\"date\":\"2025-01-05T21:17:00.862Z\",\"views\":2},{\"date\":\"2025-01-02T09:17:00.885Z\",\"views\":0},{\"date\":\"2024-12-29T21:17:00.913Z\",\"views\":4},{\"date\":\"2024-12-26T09:17:00.933Z\",\"views\":6},{\"date\":\"2024-12-22T21:17:00.952Z\",\"views\":0},{\"date\":\"2024-12-19T09:17:00.970Z\",\"views\":2},{\"date\":\"2024-12-15T21:17:00.990Z\",\"views\":2},{\"date\":\"2024-12-12T09:17:01.009Z\",\"views\":1},{\"date\":\"2024-12-08T21:17:01.036Z\",\"views\":0},{\"date\":\"2024-12-05T09:17:01.057Z\",\"views\":1},{\"date\":\"2024-12-01T21:17:01.076Z\",\"views\":2},{\"date\":\"2024-11-28T09:17:01.098Z\",\"views\":2},{\"date\":\"2024-11-24T21:17:01.121Z\",\"views\":2},{\"date\":\"2024-11-21T09:17:01.146Z\",\"views\":0},{\"date\":\"2024-11-17T21:17:01.176Z\",\"views\":2},{\"date\":\"2024-11-14T09:17:01.194Z\",\"views\":0},{\"date\":\"2024-11-10T21:17:01.215Z\",\"views\":2},{\"date\":\"2024-11-07T09:17:01.235Z\",\"views\":2},{\"date\":\"2024-11-03T21:17:01.254Z\",\"views\":0},{\"date\":\"2024-10-31T08:17:01.276Z\",\"views\":2},{\"date\":\"2024-10-27T20:17:01.295Z\",\"views\":1},{\"date\":\"2024-10-24T08:17:01.319Z\",\"views\":0},{\"date\":\"2024-10-20T20:17:01.343Z\",\"views\":1},{\"date\":\"2024-10-17T08:17:01.374Z\",\"views\":2},{\"date\":\"2024-10-13T20:17:01.392Z\",\"views\":0},{\"date\":\"2024-10-10T08:17:01.418Z\",\"views\":1},{\"date\":\"2024-10-06T20:17:01.441Z\",\"views\":0},{\"date\":\"2024-10-03T08:17:01.460Z\",\"views\":2},{\"date\":\"2024-09-29T20:17:01.478Z\",\"views\":1},{\"date\":\"2024-09-26T08:17:01.503Z\",\"views\":0},{\"date\":\"2024-09-22T20:17:01.529Z\",\"views\":2},{\"date\":\"2024-09-19T08:17:01.548Z\",\"views\":1},{\"date\":\"2024-09-15T20:17:01.568Z\",\"views\":0},{\"date\":\"2024-09-12T08:17:01.589Z\",\"views\":2},{\"date\":\"2024-09-08T20:17:01.608Z\",\"views\":0},{\"date\":\"2024-09-05T08:17:01.628Z\",\"views\":0},{\"date\":\"2024-09-01T20:17:01.647Z\",\"views\":2},{\"date\":\"2024-08-29T08:17:01.666Z\",\"views\":1}]},\"is_hidden\":false,\"first_publication_date\":\"2018-01-19T00:36:02.000Z\",\"paperVersions\":{\"_id\":\"677032c590f035bff487a005\",\"paper_group_id\":\"677032c490f035bff487a003\",\"version_label\":\"v1\",\"version_order\":1,\"title\":\"Three material decomposition for spectral computed tomography enabled by block-diagonal step-preconditioning\",\"abstract\":\"$1d\",\"author_ids\":[\"673d020e615941b897fba2bd\",\"672bd37f986a1370676e434e\",\"677032c590f035bff487a004\",\"673d0212615941b897fba2cf\"],\"publication_date\":\"2018-01-19T00:36:02.000Z\",\"license\":\"http://arxiv.org/licenses/nonexclusive-distrib/1.0/\",\"created_at\":\"2024-12-28T17:17:57.219Z\",\"updated_at\":\"2024-12-28T17:17:57.219Z\",\"is_deleted\":false,\"is_hidden\":false,\"universal_paper_id\":\"1801.06263\",\"imageURL\":\"image/1801.06263v1.png\"},\"verifiedAuthors\":[],\"authors\":[{\"_id\":\"672bd37f986a1370676e434e\",\"full_name\":\"Rina Foygel Barber\",\"affiliation\":null,\"orcid\":null,\"semantic_scholarid\":null,\"user_id\":null},{\"_id\":\"673d020e615941b897fba2bd\",\"full_name\":\"Emil Y. Sidky\",\"affiliation\":null,\"orcid\":null,\"semantic_scholarid\":null,\"user_id\":null},{\"_id\":\"673d0212615941b897fba2cf\",\"full_name\":\"Xiaochuan Pan\",\"affiliation\":null,\"orcid\":null,\"semantic_scholarid\":null,\"user_id\":null},{\"_id\":\"677032c590f035bff487a004\",\"full_name\":\"Taly Gilat-Schmidt\",\"affiliation\":null,\"orcid\":null,\"semantic_scholarid\":null,\"user_id\":null}]},\"max_version_order\":1,\"verified_authors\":[],\"authors\":[{\"_id\":\"672bd37f986a1370676e434e\",\"full_name\":\"Rina Foygel Barber\",\"affiliation\":null,\"orcid\":null,\"semantic_scholarid\":null,\"user_id\":null},{\"_id\":\"673d020e615941b897fba2bd\",\"full_name\":\"Emil Y. Sidky\",\"affiliation\":null,\"orcid\":null,\"semantic_scholarid\":null,\"user_id\":null},{\"_id\":\"673d0212615941b897fba2cf\",\"full_name\":\"Xiaochuan Pan\",\"affiliation\":null,\"orcid\":null,\"semantic_scholarid\":null,\"user_id\":null},{\"_id\":\"677032c590f035bff487a004\",\"full_name\":\"Taly Gilat-Schmidt\",\"affiliation\":null,\"orcid\":null,\"semantic_scholarid\":null,\"user_id\":null}],\"pdf_info\":{\"fetcher_url\":\"https://fetcher.alphaxiv.org/v2/pdf/1801.06263v1\"}}},\"dataUpdateCount\":1,\"dataUpdatedAt\":1744228172768,\"error\":null,\"errorUpdateCount\":0,\"errorUpdatedAt\":0,\"fetchFailureCount\":0,\"fetchFailureReason\":null,\"fetchMeta\":null,\"isInvalidated\":false,\"status\":\"success\",\"fetchStatus\":\"idle\"},\"queryKey\":[\"paper\",\"1801.06263\",\"metadata\"],\"queryHash\":\"[\\\"paper\\\",\\\"1801.06263\\\",\\\"metadata\\\"]\"},{\"state\":{\"data\":{\"data\":[]},\"dataUpdateCount\":1,\"dataUpdatedAt\":1744228172768,\"error\":null,\"errorUpdateCount\":0,\"errorUpdatedAt\":0,\"fetchFailureCount\":0,\"fetchFailureReason\":null,\"fetchMeta\":null,\"isInvalidated\":false,\"status\":\"success\",\"fetchStatus\":\"idle\"},\"queryKey\":[\"paper\",\"1801.06263\",\"comments\"],\"queryHash\":\"[\\\"paper\\\",\\\"1801.06263\\\",\\\"comments\\\"]\"},{\"state\":{\"data\":{\"data\":{\"paper_version\":{\"_id\":\"6751d1bc7546122de639dda8\",\"paper_group_id\":\"6751d1bc7546122de639dda6\",\"version_label\":\"v1\",\"version_order\":1,\"title\":\"Reconstructing Neutrino Mass Spectrum\",\"abstract\":\"Reconstruction of the neutrino mass spectrum and lepton mixing is one of the fundamental problems of particle physics. In this connection we consider two central topics: (i) the origin of large lepton mixing, (ii) possible existence of new (sterile) neutrino states. We discuss also possible relation between large mixing and existence of sterile neutrinos.\",\"author_ids\":[\"6751d1bc7546122de639dda7\"],\"publication_date\":\"1999-01-03T13:15:43.000Z\",\"license\":\"http://arxiv.org/licenses/assumed-1991-2003/\",\"created_at\":\"2024-12-05T16:15:56.938Z\",\"updated_at\":\"2024-12-05T16:15:56.938Z\",\"is_deleted\":false,\"is_hidden\":false,\"universal_paper_id\":\"hep-ph/9901208\"},\"paper_group\":{\"_id\":\"6751d1bc7546122de639dda6\",\"universal_paper_id\":\"hep-ph/9901208\",\"source\":{\"name\":\"arXiv\",\"url\":\"https://arXiv.org/paper/hep-ph_9901208\"},\"title\":\"Reconstructing Neutrino Mass Spectrum\",\"created_at\":\"2024-12-05T16:15:45.279Z\",\"updated_at\":\"2025-03-03T19:39:17.284Z\",\"categories\":[\"Physics\"],\"subcategories\":[\"hep-ph\"],\"custom_categories\":null,\"author_user_ids\":[],\"metrics\":{\"activity_rank\":0,\"questions_count\":0,\"responses_count\":0,\"upvotes_count\":null,\"downvotes_count\":null,\"total_votes\":0,\"visits_count\":{\"last24Hours\":0,\"last7Days\":5,\"last30Days\":6,\"last90Days\":8,\"all\":25},\"weighted_visits\":{\"last24Hours\":0,\"last7Days\":0.005582180809256903,\"last30Days\":1.2283227414704339,\"last90Days\":4.714949740782471,\"hot\":0.005582180809256903},\"public_total_votes\":0,\"timeline\":[{\"date\":\"2025-04-02T23:48:48.858Z\",\"views\":14},{\"date\":\"2025-03-30T11:48:48.858Z\",\"views\":3},{\"date\":\"2025-03-26T23:48:48.858Z\",\"views\":4},{\"date\":\"2025-03-23T11:48:48.858Z\",\"views\":1},{\"date\":\"2025-03-19T23:48:48.858Z\",\"views\":1},{\"date\":\"2025-03-16T11:48:48.858Z\",\"views\":2},{\"date\":\"2025-03-12T23:48:48.858Z\",\"views\":0},{\"date\":\"2025-03-09T11:48:48.858Z\",\"views\":1},{\"date\":\"2025-03-05T23:48:48.858Z\",\"views\":1},{\"date\":\"2025-03-02T11:48:48.858Z\",\"views\":0},{\"date\":\"2025-02-26T23:48:48.858Z\",\"views\":1},{\"date\":\"2025-02-23T11:48:48.858Z\",\"views\":1},{\"date\":\"2025-02-19T23:48:48.868Z\",\"views\":2},{\"date\":\"2025-02-16T11:48:48.953Z\",\"views\":1},{\"date\":\"2025-02-12T23:48:48.986Z\",\"views\":1},{\"date\":\"2025-02-09T11:48:49.043Z\",\"views\":2},{\"date\":\"2025-02-05T23:48:49.068Z\",\"views\":2},{\"date\":\"2025-02-02T11:48:49.093Z\",\"views\":1},{\"date\":\"2025-01-29T23:48:49.112Z\",\"views\":2},{\"date\":\"2025-01-26T11:48:49.141Z\",\"views\":0},{\"date\":\"2025-01-22T23:48:49.166Z\",\"views\":5},{\"date\":\"2025-01-19T11:48:49.192Z\",\"views\":2},{\"date\":\"2025-01-15T23:48:49.208Z\",\"views\":1},{\"date\":\"2025-01-12T11:48:49.234Z\",\"views\":4},{\"date\":\"2025-01-08T23:48:49.283Z\",\"views\":1},{\"date\":\"2025-01-05T11:48:49.330Z\",\"views\":2},{\"date\":\"2025-01-01T23:48:49.356Z\",\"views\":2},{\"date\":\"2024-12-29T11:48:49.390Z\",\"views\":1},{\"date\":\"2024-12-25T23:48:49.440Z\",\"views\":2},{\"date\":\"2024-12-22T11:48:49.465Z\",\"views\":1},{\"date\":\"2024-12-18T23:48:49.490Z\",\"views\":1},{\"date\":\"2024-12-15T11:48:49.514Z\",\"views\":0},{\"date\":\"2024-12-11T23:48:49.550Z\",\"views\":1},{\"date\":\"2024-12-08T11:48:49.580Z\",\"views\":0},{\"date\":\"2024-12-04T23:48:49.605Z\",\"views\":2}]},\"ranking\":{\"current_rank\":0,\"previous_rank\":0,\"activity_score\":0,\"paper_score\":0},\"is_hidden\":false,\"first_publication_date\":\"2024-12-05T16:15:56.550Z\",\"citation\":{\"bibtex\":\"@misc{smirnov1999reconstructingneutrinomass,\\n title={Reconstructing Neutrino Mass Spectrum}, \\n author={A. Yu. Smirnov},\\n year={1999},\\n eprint={hep-ph/9901208},\\n archivePrefix={arXiv},\\n primaryClass={hep-ph},\\n url={https://arxiv.org/abs/hep-ph/9901208}, \\n}\"},\"paperVersions\":{\"_id\":\"6751d1bc7546122de639dda8\",\"paper_group_id\":\"6751d1bc7546122de639dda6\",\"version_label\":\"v1\",\"version_order\":1,\"title\":\"Reconstructing Neutrino Mass Spectrum\",\"abstract\":\"Reconstruction of the neutrino mass spectrum and lepton mixing is one of the fundamental problems of particle physics. In this connection we consider two central topics: (i) the origin of large lepton mixing, (ii) possible existence of new (sterile) neutrino states. We discuss also possible relation between large mixing and existence of sterile neutrinos.\",\"author_ids\":[\"6751d1bc7546122de639dda7\"],\"publication_date\":\"1999-01-03T13:15:43.000Z\",\"license\":\"http://arxiv.org/licenses/assumed-1991-2003/\",\"created_at\":\"2024-12-05T16:15:56.938Z\",\"updated_at\":\"2024-12-05T16:15:56.938Z\",\"is_deleted\":false,\"is_hidden\":false,\"universal_paper_id\":\"hep-ph/9901208\"},\"verifiedAuthors\":[],\"authors\":[{\"_id\":\"6751d1bc7546122de639dda7\",\"full_name\":\"A. Yu. Smirnov\",\"affiliation\":null,\"orcid\":null,\"semantic_scholarid\":null,\"user_id\":null}]},\"max_version_order\":1,\"verified_authors\":[],\"authors\":[{\"_id\":\"6751d1bc7546122de639dda7\",\"full_name\":\"A. Yu. Smirnov\",\"affiliation\":null,\"orcid\":null,\"semantic_scholarid\":null,\"user_id\":null}],\"pdf_info\":{\"fetcher_url\":\"https://fetcher.alphaxiv.org/v2/pdf/hep-ph%2F9901208v1\"}}},\"dataUpdateCount\":2,\"dataUpdatedAt\":1744228173451,\"error\":null,\"errorUpdateCount\":0,\"errorUpdatedAt\":0,\"fetchFailureCount\":0,\"fetchFailureReason\":null,\"fetchMeta\":null,\"isInvalidated\":false,\"status\":\"success\",\"fetchStatus\":\"idle\"},\"queryKey\":[\"paper\",\"hep-ph/9901208\",\"metadata\"],\"queryHash\":\"[\\\"paper\\\",\\\"hep-ph/9901208\\\",\\\"metadata\\\"]\"},{\"state\":{\"data\":{\"data\":[]},\"dataUpdateCount\":2,\"dataUpdatedAt\":1744228173451,\"error\":null,\"errorUpdateCount\":0,\"errorUpdatedAt\":0,\"fetchFailureCount\":0,\"fetchFailureReason\":null,\"fetchMeta\":null,\"isInvalidated\":false,\"status\":\"success\",\"fetchStatus\":\"idle\"},\"queryKey\":[\"paper\",\"hep-ph/9901208\",\"comments\"],\"queryHash\":\"[\\\"paper\\\",\\\"hep-ph/9901208\\\",\\\"comments\\\"]\"},{\"state\":{\"data\":{\"data\":{\"paper_version\":{\"_id\":\"67a8df6665d4a2a0b6d30aba\",\"paper_group_id\":\"673ba6b1ee7cdcdc03b18de8\",\"version_label\":\"v2\",\"version_order\":2,\"title\":\"ReVersion: Diffusion-Based Relation Inversion from Images\",\"abstract\":\"$1e\",\"author_ids\":[\"6733262ac48bba476d7884b4\",\"6733262dc48bba476d7884b6\",\"6733262cc48bba476d7884b5\",\"6732250ccd1e32a6e7effba3\",\"672bbe10986a1370676d5619\"],\"publication_date\":\"2024-12-01T14:04:22.000Z\",\"license\":\"http://arxiv.org/licenses/nonexclusive-distrib/1.0/\",\"created_at\":\"2025-02-09T17:01:26.567Z\",\"updated_at\":\"2025-02-09T17:01:26.567Z\",\"is_deleted\":false,\"is_hidden\":false,\"universal_paper_id\":\"2303.13495\",\"imageURL\":\"image/2303.13495v2.png\"},\"paper_group\":{\"_id\":\"673ba6b1ee7cdcdc03b18de8\",\"universal_paper_id\":\"2303.13495\",\"source\":{\"name\":\"arXiv\",\"url\":\"https://arXiv.org/paper/2303.13495\"},\"title\":\"ReVersion: Diffusion-Based Relation Inversion from Images\",\"created_at\":\"2024-11-17T03:35:49.534Z\",\"updated_at\":\"2025-03-03T20:18:37.694Z\",\"categories\":[\"Computer Science\"],\"subcategories\":[\"cs.CV\"],\"metrics\":{\"activity_rank\":0,\"questions_count\":0,\"responses_count\":0,\"upvotes_count\":null,\"downvotes_count\":null,\"total_votes\":0,\"visits_count\":{\"last24Hours\":0,\"last7Days\":2,\"last30Days\":8,\"last90Days\":14,\"all\":112},\"weighted_visits\":{\"last24Hours\":0,\"last7Days\":7.700629298557463e-19,\"last30Days\":0.0004039916963582148,\"last90Days\":0.5174743800562933,\"hot\":7.700629298557463e-19},\"public_total_votes\":2,\"timeline\":[{\"date\":\"2025-04-02T02:39:24.914Z\",\"views\":1},{\"date\":\"2025-03-29T14:39:24.914Z\",\"views\":7},{\"date\":\"2025-03-26T02:39:24.914Z\",\"views\":7},{\"date\":\"2025-03-22T14:39:24.914Z\",\"views\":8},{\"date\":\"2025-03-19T02:39:24.914Z\",\"views\":1},{\"date\":\"2025-03-15T14:39:24.914Z\",\"views\":4},{\"date\":\"2025-03-12T02:39:24.914Z\",\"views\":4},{\"date\":\"2025-03-08T14:39:24.914Z\",\"views\":1},{\"date\":\"2025-03-05T02:39:24.914Z\",\"views\":1},{\"date\":\"2025-03-01T14:39:24.914Z\",\"views\":2},{\"date\":\"2025-02-26T02:39:24.914Z\",\"views\":0},{\"date\":\"2025-02-22T14:39:24.914Z\",\"views\":2},{\"date\":\"2025-02-19T02:39:24.926Z\",\"views\":0},{\"date\":\"2025-02-15T14:39:24.936Z\",\"views\":1},{\"date\":\"2025-02-12T02:39:24.952Z\",\"views\":5},{\"date\":\"2025-02-08T14:39:24.965Z\",\"views\":6},{\"date\":\"2025-02-05T02:39:24.976Z\",\"views\":1},{\"date\":\"2025-02-01T14:39:24.991Z\",\"views\":1},{\"date\":\"2025-01-29T02:39:25.014Z\",\"views\":2},{\"date\":\"2025-01-25T14:39:25.029Z\",\"views\":1},{\"date\":\"2025-01-22T02:39:25.047Z\",\"views\":1},{\"date\":\"2025-01-18T14:39:25.063Z\",\"views\":0},{\"date\":\"2025-01-15T02:39:25.080Z\",\"views\":2},{\"date\":\"2025-01-11T14:39:25.095Z\",\"views\":5},{\"date\":\"2025-01-08T02:39:25.114Z\",\"views\":4},{\"date\":\"2025-01-04T14:39:25.131Z\",\"views\":6},{\"date\":\"2025-01-01T02:39:25.149Z\",\"views\":0},{\"date\":\"2024-12-28T14:39:25.165Z\",\"views\":4},{\"date\":\"2024-12-25T02:39:25.183Z\",\"views\":3},{\"date\":\"2024-12-21T14:39:25.198Z\",\"views\":1},{\"date\":\"2024-12-18T02:39:25.218Z\",\"views\":2},{\"date\":\"2024-12-14T14:39:25.233Z\",\"views\":1},{\"date\":\"2024-12-11T02:39:25.251Z\",\"views\":1},{\"date\":\"2024-12-07T14:39:25.267Z\",\"views\":1},{\"date\":\"2024-12-04T02:39:25.281Z\",\"views\":5},{\"date\":\"2024-11-30T14:39:25.298Z\",\"views\":13},{\"date\":\"2024-11-27T02:39:25.317Z\",\"views\":3},{\"date\":\"2024-11-23T14:39:25.334Z\",\"views\":1},{\"date\":\"2024-11-20T02:39:25.349Z\",\"views\":30},{\"date\":\"2024-11-16T14:39:25.379Z\",\"views\":13},{\"date\":\"2024-11-13T02:39:25.397Z\",\"views\":1},{\"date\":\"2024-11-09T14:39:25.412Z\",\"views\":2},{\"date\":\"2024-11-06T02:39:25.433Z\",\"views\":0},{\"date\":\"2024-11-02T13:39:25.452Z\",\"views\":2},{\"date\":\"2024-10-30T01:39:25.469Z\",\"views\":2},{\"date\":\"2024-10-26T13:39:25.486Z\",\"views\":2},{\"date\":\"2024-10-23T01:39:25.503Z\",\"views\":1},{\"date\":\"2024-10-19T13:39:25.520Z\",\"views\":0},{\"date\":\"2024-10-16T01:39:25.535Z\",\"views\":0},{\"date\":\"2024-10-12T13:39:25.554Z\",\"views\":2},{\"date\":\"2024-10-09T01:39:25.570Z\",\"views\":1},{\"date\":\"2024-10-05T13:39:25.595Z\",\"views\":0},{\"date\":\"2024-10-02T01:39:25.615Z\",\"views\":1},{\"date\":\"2024-09-28T13:39:25.641Z\",\"views\":2},{\"date\":\"2024-09-25T01:39:25.666Z\",\"views\":0},{\"date\":\"2024-09-21T13:39:25.687Z\",\"views\":2},{\"date\":\"2024-09-18T01:39:25.706Z\",\"views\":1},{\"date\":\"2024-09-14T13:39:25.726Z\",\"views\":1},{\"date\":\"2024-09-11T01:39:25.743Z\",\"views\":1},{\"date\":\"2024-09-07T13:39:25.762Z\",\"views\":0},{\"date\":\"2024-09-04T01:39:25.782Z\",\"views\":0},{\"date\":\"2024-08-31T13:39:25.798Z\",\"views\":2},{\"date\":\"2024-08-28T01:39:25.819Z\",\"views\":0}]},\"ranking\":{\"current_rank\":6873,\"previous_rank\":46634,\"activity_score\":0,\"paper_score\":0.8047189562170501},\"is_hidden\":false,\"custom_categories\":null,\"first_publication_date\":\"2023-03-23T17:56:10.000Z\",\"author_user_ids\":[],\"resources\":{\"github\":{\"url\":\"https://github.com/ziqihuangg/ReVersion\",\"description\":\"[SIGGRAPH Asia 2024] ReVersion: Diffusion-Based Relation Inversion from Images\",\"language\":\"Python\",\"stars\":500}},\"organizations\":[\"67be6379aa92218ccd8b10c5\"],\"paperVersions\":{\"_id\":\"67a8df6665d4a2a0b6d30aba\",\"paper_group_id\":\"673ba6b1ee7cdcdc03b18de8\",\"version_label\":\"v2\",\"version_order\":2,\"title\":\"ReVersion: Diffusion-Based Relation Inversion from Images\",\"abstract\":\"$1f\",\"author_ids\":[\"6733262ac48bba476d7884b4\",\"6733262dc48bba476d7884b6\",\"6733262cc48bba476d7884b5\",\"6732250ccd1e32a6e7effba3\",\"672bbe10986a1370676d5619\"],\"publication_date\":\"2024-12-01T14:04:22.000Z\",\"license\":\"http://arxiv.org/licenses/nonexclusive-distrib/1.0/\",\"created_at\":\"2025-02-09T17:01:26.567Z\",\"updated_at\":\"2025-02-09T17:01:26.567Z\",\"is_deleted\":false,\"is_hidden\":false,\"universal_paper_id\":\"2303.13495\",\"imageURL\":\"image/2303.13495v2.png\"},\"verifiedAuthors\":[],\"authors\":[{\"_id\":\"672bbe10986a1370676d5619\",\"full_name\":\"Ziwei Liu\",\"affiliation\":null,\"orcid\":null,\"semantic_scholarid\":null,\"user_id\":null},{\"_id\":\"6732250ccd1e32a6e7effba3\",\"full_name\":\"Kelvin C.K. Chan\",\"affiliation\":null,\"orcid\":null,\"semantic_scholarid\":null,\"user_id\":null},{\"_id\":\"6733262ac48bba476d7884b4\",\"full_name\":\"Ziqi Huang\",\"affiliation\":null,\"orcid\":null,\"semantic_scholarid\":null,\"user_id\":null},{\"_id\":\"6733262cc48bba476d7884b5\",\"full_name\":\"Yuming Jiang\",\"affiliation\":null,\"orcid\":null,\"semantic_scholarid\":null,\"user_id\":null},{\"_id\":\"6733262dc48bba476d7884b6\",\"full_name\":\"Tianxing Wu\",\"affiliation\":null,\"orcid\":null,\"semantic_scholarid\":null,\"user_id\":null}]},\"max_version_order\":2,\"verified_authors\":[],\"authors\":[{\"_id\":\"672bbe10986a1370676d5619\",\"full_name\":\"Ziwei Liu\",\"affiliation\":null,\"orcid\":null,\"semantic_scholarid\":null,\"user_id\":null},{\"_id\":\"6732250ccd1e32a6e7effba3\",\"full_name\":\"Kelvin C.K. Chan\",\"affiliation\":null,\"orcid\":null,\"semantic_scholarid\":null,\"user_id\":null},{\"_id\":\"6733262ac48bba476d7884b4\",\"full_name\":\"Ziqi Huang\",\"affiliation\":null,\"orcid\":null,\"semantic_scholarid\":null,\"user_id\":null},{\"_id\":\"6733262cc48bba476d7884b5\",\"full_name\":\"Yuming Jiang\",\"affiliation\":null,\"orcid\":null,\"semantic_scholarid\":null,\"user_id\":null},{\"_id\":\"6733262dc48bba476d7884b6\",\"full_name\":\"Tianxing Wu\",\"affiliation\":null,\"orcid\":null,\"semantic_scholarid\":null,\"user_id\":null}],\"pdf_info\":{\"fetcher_url\":\"https://fetcher.alphaxiv.org/v2/pdf/2303.13495v2\"}}},\"dataUpdateCount\":1,\"dataUpdatedAt\":1744228173944,\"error\":null,\"errorUpdateCount\":0,\"errorUpdatedAt\":0,\"fetchFailureCount\":0,\"fetchFailureReason\":null,\"fetchMeta\":null,\"isInvalidated\":false,\"status\":\"success\",\"fetchStatus\":\"idle\"},\"queryKey\":[\"paper\",\"2303.13495\",\"metadata\"],\"queryHash\":\"[\\\"paper\\\",\\\"2303.13495\\\",\\\"metadata\\\"]\"},{\"state\":{\"data\":{\"data\":[]},\"dataUpdateCount\":1,\"dataUpdatedAt\":1744228173944,\"error\":null,\"errorUpdateCount\":0,\"errorUpdatedAt\":0,\"fetchFailureCount\":0,\"fetchFailureReason\":null,\"fetchMeta\":null,\"isInvalidated\":false,\"status\":\"success\",\"fetchStatus\":\"idle\"},\"queryKey\":[\"paper\",\"2303.13495\",\"comments\"],\"queryHash\":\"[\\\"paper\\\",\\\"2303.13495\\\",\\\"comments\\\"]\"},{\"state\":{\"data\":{\"data\":{\"paper_version\":{\"_id\":\"67d397ba3adf9432fbc0f2d3\",\"paper_group_id\":\"67d397b93adf9432fbc0f2d1\",\"version_label\":\"v1\",\"version_order\":1,\"title\":\"FraudAuditor: A Visual Analytics Approach for Collusive Fraud in Health Insurance\",\"abstract\":\"$20\",\"author_ids\":[\"67778d3b31430e4d1bbf154c\",\"67656372bf51f1cfd1e2f304\",\"672bc625986a1370676d68e1\",\"673b79ddbf626fe16b8a868b\",\"67d397ba3adf9432fbc0f2d2\",\"672bcd31986a1370676dc3d2\",\"673324dac48bba476d78831f\",\"673cbc4c7d2b7ed9dd51ab80\",\"672bbf76986a1370676d5eb3\",\"672bbf7d986a1370676d5eea\"],\"publication_date\":\"2023-03-23T17:53:58.000Z\",\"license\":\"http://arxiv.org/licenses/nonexclusive-distrib/1.0/\",\"created_at\":\"2025-03-14T02:43:06.510Z\",\"updated_at\":\"2025-03-14T02:43:06.510Z\",\"is_deleted\":false,\"is_hidden\":false,\"universal_paper_id\":\"2303.13491\",\"imageURL\":\"image/2303.13491v1.png\"},\"paper_group\":{\"_id\":\"67d397b93adf9432fbc0f2d1\",\"universal_paper_id\":\"2303.13491\",\"title\":\"FraudAuditor: A Visual Analytics Approach for Collusive Fraud in Health Insurance\",\"created_at\":\"2025-03-14T02:43:05.174Z\",\"updated_at\":\"2025-03-14T02:43:05.174Z\",\"categories\":[\"Computer Science\"],\"subcategories\":[\"cs.HC\"],\"custom_categories\":null,\"author_user_ids\":[],\"source\":{\"name\":\"alphaXiv\",\"url\":\"https://arxiv.org/abs/2303.13491\"},\"metrics\":{\"activity_rank\":0,\"questions_count\":0,\"responses_count\":0,\"upvotes_count\":0,\"downvotes_count\":0,\"total_votes\":0,\"public_total_votes\":0,\"visits_count\":{\"last24Hours\":0,\"last7Days\":2,\"last30Days\":3,\"last90Days\":3,\"all\":3},\"timeline\":[{\"date\":\"2025-03-31T20:42:42.329Z\",\"views\":4},{\"date\":\"2025-03-28T08:42:42.329Z\",\"views\":2},{\"date\":\"2025-03-24T20:42:42.329Z\",\"views\":2},{\"date\":\"2025-03-21T08:42:42.329Z\",\"views\":0},{\"date\":\"2025-03-17T20:42:42.329Z\",\"views\":2},{\"date\":\"2025-03-14T08:42:42.329Z\",\"views\":2},{\"date\":\"2025-03-10T20:42:42.329Z\",\"views\":4},{\"date\":\"2025-03-07T08:42:42.356Z\",\"views\":2},{\"date\":\"2025-03-03T20:42:42.382Z\",\"views\":0},{\"date\":\"2025-02-28T08:42:42.407Z\",\"views\":0},{\"date\":\"2025-02-24T20:42:42.436Z\",\"views\":2},{\"date\":\"2025-02-21T08:42:42.467Z\",\"views\":0},{\"date\":\"2025-02-17T20:42:42.493Z\",\"views\":2},{\"date\":\"2025-02-14T08:42:42.520Z\",\"views\":1},{\"date\":\"2025-02-10T20:42:42.545Z\",\"views\":1},{\"date\":\"2025-02-07T08:42:42.572Z\",\"views\":2},{\"date\":\"2025-02-03T20:42:42.666Z\",\"views\":1},{\"date\":\"2025-01-31T08:42:42.692Z\",\"views\":0},{\"date\":\"2025-01-27T20:42:42.719Z\",\"views\":1},{\"date\":\"2025-01-24T08:42:42.744Z\",\"views\":1},{\"date\":\"2025-01-20T20:42:42.771Z\",\"views\":1},{\"date\":\"2025-01-17T08:42:42.795Z\",\"views\":0},{\"date\":\"2025-01-13T20:42:42.819Z\",\"views\":2},{\"date\":\"2025-01-10T08:42:42.846Z\",\"views\":2},{\"date\":\"2025-01-06T20:42:42.870Z\",\"views\":0},{\"date\":\"2025-01-03T08:42:42.896Z\",\"views\":0},{\"date\":\"2024-12-30T20:42:42.921Z\",\"views\":2},{\"date\":\"2024-12-27T08:42:42.950Z\",\"views\":0},{\"date\":\"2024-12-23T20:42:42.976Z\",\"views\":0},{\"date\":\"2024-12-20T08:42:43.003Z\",\"views\":2},{\"date\":\"2024-12-16T20:42:43.028Z\",\"views\":2},{\"date\":\"2024-12-13T08:42:43.052Z\",\"views\":0},{\"date\":\"2024-12-09T20:42:43.077Z\",\"views\":1},{\"date\":\"2024-12-06T08:42:43.106Z\",\"views\":0},{\"date\":\"2024-12-02T20:42:43.132Z\",\"views\":2},{\"date\":\"2024-11-29T08:42:43.159Z\",\"views\":2},{\"date\":\"2024-11-25T20:42:43.185Z\",\"views\":0},{\"date\":\"2024-11-22T08:42:43.210Z\",\"views\":2},{\"date\":\"2024-11-18T20:42:43.234Z\",\"views\":1},{\"date\":\"2024-11-15T08:42:43.261Z\",\"views\":0},{\"date\":\"2024-11-11T20:42:43.287Z\",\"views\":2},{\"date\":\"2024-11-08T08:42:43.313Z\",\"views\":0},{\"date\":\"2024-11-04T20:42:43.339Z\",\"views\":2},{\"date\":\"2024-11-01T08:42:43.362Z\",\"views\":1},{\"date\":\"2024-10-28T20:42:43.386Z\",\"views\":2},{\"date\":\"2024-10-25T08:42:43.412Z\",\"views\":0},{\"date\":\"2024-10-21T20:42:43.437Z\",\"views\":0},{\"date\":\"2024-10-18T08:42:43.464Z\",\"views\":0},{\"date\":\"2024-10-14T20:42:43.489Z\",\"views\":1},{\"date\":\"2024-10-11T08:42:43.514Z\",\"views\":1},{\"date\":\"2024-10-07T20:42:43.541Z\",\"views\":2},{\"date\":\"2024-10-04T08:42:43.568Z\",\"views\":2},{\"date\":\"2024-09-30T20:42:43.595Z\",\"views\":0},{\"date\":\"2024-09-27T08:42:43.620Z\",\"views\":2},{\"date\":\"2024-09-23T20:42:43.646Z\",\"views\":0},{\"date\":\"2024-09-20T08:42:43.670Z\",\"views\":0},{\"date\":\"2024-09-16T20:42:43.697Z\",\"views\":2},{\"date\":\"2024-09-13T08:42:43.724Z\",\"views\":2}],\"weighted_visits\":{\"last24Hours\":0,\"last7Days\":7.699957570182654e-19,\"last30Days\":0.0001514938025027067,\"last90Days\":0.11088661480037604,\"hot\":7.699957570182654e-19}},\"is_hidden\":false,\"first_publication_date\":\"2023-03-23T17:53:58.000Z\",\"organizations\":[\"67be6376aa92218ccd8b0fa4\",\"67be6376aa92218ccd8b0f6c\",\"67be6377aa92218ccd8b100a\",\"67be6383aa92218ccd8b1406\"],\"paperVersions\":{\"_id\":\"67d397ba3adf9432fbc0f2d3\",\"paper_group_id\":\"67d397b93adf9432fbc0f2d1\",\"version_label\":\"v1\",\"version_order\":1,\"title\":\"FraudAuditor: A Visual Analytics Approach for Collusive Fraud in Health Insurance\",\"abstract\":\"$21\",\"author_ids\":[\"67778d3b31430e4d1bbf154c\",\"67656372bf51f1cfd1e2f304\",\"672bc625986a1370676d68e1\",\"673b79ddbf626fe16b8a868b\",\"67d397ba3adf9432fbc0f2d2\",\"672bcd31986a1370676dc3d2\",\"673324dac48bba476d78831f\",\"673cbc4c7d2b7ed9dd51ab80\",\"672bbf76986a1370676d5eb3\",\"672bbf7d986a1370676d5eea\"],\"publication_date\":\"2023-03-23T17:53:58.000Z\",\"license\":\"http://arxiv.org/licenses/nonexclusive-distrib/1.0/\",\"created_at\":\"2025-03-14T02:43:06.510Z\",\"updated_at\":\"2025-03-14T02:43:06.510Z\",\"is_deleted\":false,\"is_hidden\":false,\"universal_paper_id\":\"2303.13491\",\"imageURL\":\"image/2303.13491v1.png\"},\"verifiedAuthors\":[],\"authors\":[{\"_id\":\"672bbf76986a1370676d5eb3\",\"full_name\":\"Jian Wu\",\"affiliation\":null,\"orcid\":null,\"semantic_scholarid\":null,\"user_id\":null},{\"_id\":\"672bbf7d986a1370676d5eea\",\"full_name\":\"Wei Chen\",\"affiliation\":null,\"orcid\":null,\"semantic_scholarid\":null,\"user_id\":null},{\"_id\":\"672bc625986a1370676d68e1\",\"full_name\":\"Jie Wang\",\"affiliation\":null,\"orcid\":null,\"semantic_scholarid\":null,\"user_id\":null},{\"_id\":\"672bcd31986a1370676dc3d2\",\"full_name\":\"Zihan Zhou\",\"affiliation\":null,\"orcid\":null,\"semantic_scholarid\":null,\"user_id\":null},{\"_id\":\"673324dac48bba476d78831f\",\"full_name\":\"Dongming Han\",\"affiliation\":null,\"orcid\":null,\"semantic_scholarid\":null,\"user_id\":null},{\"_id\":\"673b79ddbf626fe16b8a868b\",\"full_name\":\"Hui Ye\",\"affiliation\":null,\"orcid\":null,\"semantic_scholarid\":null,\"user_id\":null},{\"_id\":\"673cbc4c7d2b7ed9dd51ab80\",\"full_name\":\"Haochao Ying\",\"affiliation\":null,\"orcid\":null,\"semantic_scholarid\":null,\"user_id\":null},{\"_id\":\"67656372bf51f1cfd1e2f304\",\"full_name\":\"Xumeng Wang\",\"affiliation\":null,\"orcid\":null,\"semantic_scholarid\":null,\"user_id\":null},{\"_id\":\"67778d3b31430e4d1bbf154c\",\"full_name\":\"Jiehui Zhou\",\"affiliation\":null,\"orcid\":null,\"semantic_scholarid\":null,\"user_id\":null},{\"_id\":\"67d397ba3adf9432fbc0f2d2\",\"full_name\":\"Huanliang Wang\",\"affiliation\":null,\"orcid\":null,\"semantic_scholarid\":null,\"user_id\":null}]},\"max_version_order\":1,\"verified_authors\":[],\"authors\":[{\"_id\":\"672bbf76986a1370676d5eb3\",\"full_name\":\"Jian Wu\",\"affiliation\":null,\"orcid\":null,\"semantic_scholarid\":null,\"user_id\":null},{\"_id\":\"672bbf7d986a1370676d5eea\",\"full_name\":\"Wei Chen\",\"affiliation\":null,\"orcid\":null,\"semantic_scholarid\":null,\"user_id\":null},{\"_id\":\"672bc625986a1370676d68e1\",\"full_name\":\"Jie Wang\",\"affiliation\":null,\"orcid\":null,\"semantic_scholarid\":null,\"user_id\":null},{\"_id\":\"672bcd31986a1370676dc3d2\",\"full_name\":\"Zihan Zhou\",\"affiliation\":null,\"orcid\":null,\"semantic_scholarid\":null,\"user_id\":null},{\"_id\":\"673324dac48bba476d78831f\",\"full_name\":\"Dongming Han\",\"affiliation\":null,\"orcid\":null,\"semantic_scholarid\":null,\"user_id\":null},{\"_id\":\"673b79ddbf626fe16b8a868b\",\"full_name\":\"Hui Ye\",\"affiliation\":null,\"orcid\":null,\"semantic_scholarid\":null,\"user_id\":null},{\"_id\":\"673cbc4c7d2b7ed9dd51ab80\",\"full_name\":\"Haochao Ying\",\"affiliation\":null,\"orcid\":null,\"semantic_scholarid\":null,\"user_id\":null},{\"_id\":\"67656372bf51f1cfd1e2f304\",\"full_name\":\"Xumeng Wang\",\"affiliation\":null,\"orcid\":null,\"semantic_scholarid\":null,\"user_id\":null},{\"_id\":\"67778d3b31430e4d1bbf154c\",\"full_name\":\"Jiehui Zhou\",\"affiliation\":null,\"orcid\":null,\"semantic_scholarid\":null,\"user_id\":null},{\"_id\":\"67d397ba3adf9432fbc0f2d2\",\"full_name\":\"Huanliang Wang\",\"affiliation\":null,\"orcid\":null,\"semantic_scholarid\":null,\"user_id\":null}],\"pdf_info\":{\"fetcher_url\":\"https://fetcher.alphaxiv.org/v2/pdf/2303.13491v1\"}}},\"dataUpdateCount\":1,\"dataUpdatedAt\":1744228174083,\"error\":null,\"errorUpdateCount\":0,\"errorUpdatedAt\":0,\"fetchFailureCount\":0,\"fetchFailureReason\":null,\"fetchMeta\":null,\"isInvalidated\":false,\"status\":\"success\",\"fetchStatus\":\"idle\"},\"queryKey\":[\"paper\",\"2303.13491\",\"metadata\"],\"queryHash\":\"[\\\"paper\\\",\\\"2303.13491\\\",\\\"metadata\\\"]\"},{\"state\":{\"data\":{\"data\":[]},\"dataUpdateCount\":1,\"dataUpdatedAt\":1744228174083,\"error\":null,\"errorUpdateCount\":0,\"errorUpdatedAt\":0,\"fetchFailureCount\":0,\"fetchFailureReason\":null,\"fetchMeta\":null,\"isInvalidated\":false,\"status\":\"success\",\"fetchStatus\":\"idle\"},\"queryKey\":[\"paper\",\"2303.13491\",\"comments\"],\"queryHash\":\"[\\\"paper\\\",\\\"2303.13491\\\",\\\"comments\\\"]\"},{\"state\":{\"data\":{\"data\":{\"paper_version\":{\"_id\":\"67eb8ccce1e17bb9df79333b\",\"paper_group_id\":\"67eb8ccbe1e17bb9df79333a\",\"version_label\":\"v2\",\"version_order\":2,\"title\":\"Geometric and analytic structures on metric spaces homeomorphic to a manifold\",\"abstract\":\"$22\",\"author_ids\":[\"67daa9e9682dc31851f8c3c2\",\"6775f505b06648e5c8e1d47e\",\"67a38dc8ee262751fe28baaa\"],\"publication_date\":\"2023-09-22T09:18:24.000Z\",\"license\":\"http://arxiv.org/licenses/nonexclusive-distrib/1.0/\",\"created_at\":\"2025-04-01T06:50:52.193Z\",\"updated_at\":\"2025-04-01T06:50:52.193Z\",\"is_deleted\":false,\"is_hidden\":false,\"universal_paper_id\":\"2303.13490\",\"imageURL\":\"image/2303.13490v2.png\"},\"paper_group\":{\"_id\":\"67eb8ccbe1e17bb9df79333a\",\"universal_paper_id\":\"2303.13490\",\"title\":\"Geometric and analytic structures on metric spaces homeomorphic to a manifold\",\"created_at\":\"2025-04-01T06:50:51.134Z\",\"updated_at\":\"2025-04-01T06:50:51.134Z\",\"categories\":[\"Mathematics\"],\"subcategories\":[\"math.MG\",\"math.DG\"],\"custom_categories\":null,\"author_user_ids\":[],\"source\":{\"name\":\"alphaXiv\",\"url\":\"https://arxiv.org/abs/2303.13490\"},\"metrics\":{\"activity_rank\":0,\"questions_count\":0,\"responses_count\":0,\"upvotes_count\":0,\"downvotes_count\":0,\"total_votes\":0,\"public_total_votes\":7,\"visits_count\":{\"last24Hours\":0,\"last7Days\":1,\"last30Days\":3,\"last90Days\":3,\"all\":3},\"timeline\":[{\"date\":\"2025-04-04T20:46:33.464Z\",\"views\":3},{\"date\":\"2025-04-01T08:46:33.464Z\",\"views\":3},{\"date\":\"2025-03-28T20:46:33.464Z\",\"views\":5},{\"date\":\"2025-03-25T08:46:33.489Z\",\"views\":1},{\"date\":\"2025-03-21T20:46:33.513Z\",\"views\":0},{\"date\":\"2025-03-18T08:46:33.536Z\",\"views\":2},{\"date\":\"2025-03-14T20:46:33.559Z\",\"views\":1},{\"date\":\"2025-03-11T08:46:33.582Z\",\"views\":2},{\"date\":\"2025-03-07T20:46:33.605Z\",\"views\":2},{\"date\":\"2025-03-04T08:46:33.628Z\",\"views\":2},{\"date\":\"2025-02-28T20:46:33.651Z\",\"views\":0},{\"date\":\"2025-02-25T08:46:33.674Z\",\"views\":1},{\"date\":\"2025-02-21T20:46:33.697Z\",\"views\":0},{\"date\":\"2025-02-18T08:46:33.721Z\",\"views\":0},{\"date\":\"2025-02-14T20:46:33.745Z\",\"views\":2},{\"date\":\"2025-02-11T08:46:33.768Z\",\"views\":1},{\"date\":\"2025-02-07T20:46:33.793Z\",\"views\":2},{\"date\":\"2025-02-04T08:46:33.816Z\",\"views\":0},{\"date\":\"2025-01-31T20:46:33.839Z\",\"views\":0},{\"date\":\"2025-01-28T08:46:33.865Z\",\"views\":1},{\"date\":\"2025-01-24T20:46:33.964Z\",\"views\":2},{\"date\":\"2025-01-21T08:46:35.352Z\",\"views\":0},{\"date\":\"2025-01-17T20:46:35.376Z\",\"views\":1},{\"date\":\"2025-01-14T08:46:35.413Z\",\"views\":1},{\"date\":\"2025-01-10T20:46:35.484Z\",\"views\":2},{\"date\":\"2025-01-07T08:46:35.509Z\",\"views\":0},{\"date\":\"2025-01-03T20:46:35.532Z\",\"views\":1},{\"date\":\"2024-12-31T08:46:35.557Z\",\"views\":1},{\"date\":\"2024-12-27T20:46:35.581Z\",\"views\":1},{\"date\":\"2024-12-24T08:46:35.604Z\",\"views\":2},{\"date\":\"2024-12-20T20:46:35.632Z\",\"views\":2},{\"date\":\"2024-12-17T08:46:35.655Z\",\"views\":2},{\"date\":\"2024-12-13T20:46:35.678Z\",\"views\":1},{\"date\":\"2024-12-10T08:46:35.703Z\",\"views\":0},{\"date\":\"2024-12-06T20:46:35.726Z\",\"views\":0},{\"date\":\"2024-12-03T08:46:35.750Z\",\"views\":0},{\"date\":\"2024-11-29T20:46:35.773Z\",\"views\":2},{\"date\":\"2024-11-26T08:46:35.797Z\",\"views\":2},{\"date\":\"2024-11-22T20:46:35.821Z\",\"views\":0},{\"date\":\"2024-11-19T08:46:35.844Z\",\"views\":1},{\"date\":\"2024-11-15T20:46:35.869Z\",\"views\":2},{\"date\":\"2024-11-12T08:46:35.892Z\",\"views\":0},{\"date\":\"2024-11-08T20:46:35.927Z\",\"views\":2},{\"date\":\"2024-11-05T08:46:35.956Z\",\"views\":0},{\"date\":\"2024-11-01T20:46:35.986Z\",\"views\":1},{\"date\":\"2024-10-29T08:46:36.010Z\",\"views\":0},{\"date\":\"2024-10-25T20:46:36.033Z\",\"views\":0},{\"date\":\"2024-10-22T08:46:36.093Z\",\"views\":2},{\"date\":\"2024-10-18T20:46:36.132Z\",\"views\":2},{\"date\":\"2024-10-15T08:46:36.156Z\",\"views\":1},{\"date\":\"2024-10-11T20:46:36.179Z\",\"views\":1},{\"date\":\"2024-10-08T08:46:36.203Z\",\"views\":1},{\"date\":\"2024-10-04T20:46:36.226Z\",\"views\":0},{\"date\":\"2024-10-01T08:46:36.274Z\",\"views\":0}],\"weighted_visits\":{\"last24Hours\":0,\"last7Days\":3.2744516533841607e-19,\"last30Days\":0.00014587703380086788,\"last90Days\":0.10949891380691937,\"hot\":3.2744516533841607e-19}},\"is_hidden\":false,\"first_publication_date\":\"2023-03-23T17:53:50.000Z\",\"organizations\":[\"67be6423aa92218ccd8b306b\",\"67be657caa92218ccd8b5296\"],\"paperVersions\":{\"_id\":\"67eb8ccce1e17bb9df79333b\",\"paper_group_id\":\"67eb8ccbe1e17bb9df79333a\",\"version_label\":\"v2\",\"version_order\":2,\"title\":\"Geometric and analytic structures on metric spaces homeomorphic to a manifold\",\"abstract\":\"$23\",\"author_ids\":[\"67daa9e9682dc31851f8c3c2\",\"6775f505b06648e5c8e1d47e\",\"67a38dc8ee262751fe28baaa\"],\"publication_date\":\"2023-09-22T09:18:24.000Z\",\"license\":\"http://arxiv.org/licenses/nonexclusive-distrib/1.0/\",\"created_at\":\"2025-04-01T06:50:52.193Z\",\"updated_at\":\"2025-04-01T06:50:52.193Z\",\"is_deleted\":false,\"is_hidden\":false,\"universal_paper_id\":\"2303.13490\",\"imageURL\":\"image/2303.13490v2.png\"},\"verifiedAuthors\":[],\"authors\":[{\"_id\":\"6775f505b06648e5c8e1d47e\",\"full_name\":\"Denis Marti\",\"affiliation\":null,\"orcid\":null,\"semantic_scholarid\":null,\"user_id\":null},{\"_id\":\"67a38dc8ee262751fe28baaa\",\"full_name\":\"Stefan Wenger\",\"affiliation\":null,\"orcid\":null,\"semantic_scholarid\":null,\"user_id\":null},{\"_id\":\"67daa9e9682dc31851f8c3c2\",\"full_name\":\"Giuliano Basso\",\"affiliation\":null,\"orcid\":null,\"semantic_scholarid\":null,\"user_id\":null}]},\"max_version_order\":2,\"verified_authors\":[],\"authors\":[{\"_id\":\"6775f505b06648e5c8e1d47e\",\"full_name\":\"Denis Marti\",\"affiliation\":null,\"orcid\":null,\"semantic_scholarid\":null,\"user_id\":null},{\"_id\":\"67a38dc8ee262751fe28baaa\",\"full_name\":\"Stefan Wenger\",\"affiliation\":null,\"orcid\":null,\"semantic_scholarid\":null,\"user_id\":null},{\"_id\":\"67daa9e9682dc31851f8c3c2\",\"full_name\":\"Giuliano Basso\",\"affiliation\":null,\"orcid\":null,\"semantic_scholarid\":null,\"user_id\":null}],\"pdf_info\":{\"fetcher_url\":\"https://fetcher.alphaxiv.org/v2/pdf/2303.13490v2\"}}},\"dataUpdateCount\":1,\"dataUpdatedAt\":1744228174336,\"error\":null,\"errorUpdateCount\":0,\"errorUpdatedAt\":0,\"fetchFailureCount\":0,\"fetchFailureReason\":null,\"fetchMeta\":null,\"isInvalidated\":false,\"status\":\"success\",\"fetchStatus\":\"idle\"},\"queryKey\":[\"paper\",\"2303.13490\",\"metadata\"],\"queryHash\":\"[\\\"paper\\\",\\\"2303.13490\\\",\\\"metadata\\\"]\"},{\"state\":{\"data\":{\"data\":[]},\"dataUpdateCount\":1,\"dataUpdatedAt\":1744228174336,\"error\":null,\"errorUpdateCount\":0,\"errorUpdatedAt\":0,\"fetchFailureCount\":0,\"fetchFailureReason\":null,\"fetchMeta\":null,\"isInvalidated\":false,\"status\":\"success\",\"fetchStatus\":\"idle\"},\"queryKey\":[\"paper\",\"2303.13490\",\"comments\"],\"queryHash\":\"[\\\"paper\\\",\\\"2303.13490\\\",\\\"comments\\\"]\"},{\"state\":{\"data\":{\"data\":{\"paper_version\":{\"_id\":\"673d85f3181e8ac859336c0e\",\"paper_group_id\":\"673d85f2181e8ac859336c0d\",\"version_label\":\"v1\",\"version_order\":1,\"title\":\"Egocentric Audio-Visual Object Localization\",\"abstract\":\"$24\",\"author_ids\":[\"672bc883986a1370676d7b4b\",\"672bd49a986a1370676e587c\",\"6732224acd1e32a6e7efd05d\",\"6732237ccd1e32a6e7efe53d\"],\"publication_date\":\"2023-03-23T17:43:11.000Z\",\"license\":\"http://creativecommons.org/licenses/by/4.0/\",\"created_at\":\"2024-11-20T06:47:15.217Z\",\"updated_at\":\"2024-11-20T06:47:15.217Z\",\"is_deleted\":false,\"is_hidden\":false,\"universal_paper_id\":\"2303.13471\",\"imageURL\":\"image/2303.13471v1.png\"},\"paper_group\":{\"_id\":\"673d85f2181e8ac859336c0d\",\"universal_paper_id\":\"2303.13471\",\"source\":{\"name\":\"arXiv\",\"url\":\"https://arXiv.org/paper/2303.13471\"},\"title\":\"Egocentric Audio-Visual Object Localization\",\"created_at\":\"2024-11-10T11:08:27.910Z\",\"updated_at\":\"2025-03-03T20:18:37.710Z\",\"categories\":[\"Computer Science\",\"Electrical Engineering and Systems Science\"],\"subcategories\":[\"cs.CV\",\"cs.MM\",\"cs.SD\",\"eess.AS\"],\"metrics\":{\"activity_rank\":0,\"questions_count\":0,\"responses_count\":0,\"upvotes_count\":null,\"downvotes_count\":null,\"total_votes\":0,\"visits_count\":{\"last24Hours\":0,\"last7Days\":4,\"last30Days\":5,\"last90Days\":9,\"all\":43},\"weighted_visits\":{\"last24Hours\":0,\"last7Days\":1.53933239616851e-18,\"last30Days\":0.0002524644513406131,\"last90Days\":0.33264876831322765,\"hot\":1.53933239616851e-18},\"public_total_votes\":0,\"timeline\":[{\"date\":\"2025-04-02T02:39:24.941Z\",\"views\":14},{\"date\":\"2025-03-29T14:39:24.941Z\",\"views\":0},{\"date\":\"2025-03-26T02:39:24.941Z\",\"views\":2},{\"date\":\"2025-03-22T14:39:24.941Z\",\"views\":5},{\"date\":\"2025-03-19T02:39:24.941Z\",\"views\":1},{\"date\":\"2025-03-15T14:39:24.941Z\",\"views\":0},{\"date\":\"2025-03-12T02:39:24.941Z\",\"views\":0},{\"date\":\"2025-03-08T14:39:24.941Z\",\"views\":1},{\"date\":\"2025-03-05T02:39:24.941Z\",\"views\":1},{\"date\":\"2025-03-01T14:39:24.941Z\",\"views\":1},{\"date\":\"2025-02-26T02:39:24.941Z\",\"views\":2},{\"date\":\"2025-02-22T14:39:24.941Z\",\"views\":7},{\"date\":\"2025-02-19T02:39:24.952Z\",\"views\":8},{\"date\":\"2025-02-15T14:39:24.966Z\",\"views\":2},{\"date\":\"2025-02-12T02:39:24.981Z\",\"views\":0},{\"date\":\"2025-02-08T14:39:25.000Z\",\"views\":0},{\"date\":\"2025-02-05T02:39:25.019Z\",\"views\":1},{\"date\":\"2025-02-01T14:39:25.036Z\",\"views\":1},{\"date\":\"2025-01-29T02:39:25.054Z\",\"views\":1},{\"date\":\"2025-01-25T14:39:25.070Z\",\"views\":2},{\"date\":\"2025-01-22T02:39:25.087Z\",\"views\":2},{\"date\":\"2025-01-18T14:39:25.103Z\",\"views\":1},{\"date\":\"2025-01-15T02:39:25.121Z\",\"views\":0},{\"date\":\"2025-01-11T14:39:25.136Z\",\"views\":1},{\"date\":\"2025-01-08T02:39:25.155Z\",\"views\":0},{\"date\":\"2025-01-04T14:39:25.169Z\",\"views\":0},{\"date\":\"2025-01-01T02:39:25.186Z\",\"views\":4},{\"date\":\"2024-12-28T14:39:25.202Z\",\"views\":1},{\"date\":\"2024-12-25T02:39:25.222Z\",\"views\":5},{\"date\":\"2024-12-21T14:39:25.238Z\",\"views\":0},{\"date\":\"2024-12-18T02:39:25.252Z\",\"views\":1},{\"date\":\"2024-12-14T14:39:25.270Z\",\"views\":1},{\"date\":\"2024-12-11T02:39:25.288Z\",\"views\":0},{\"date\":\"2024-12-07T14:39:25.306Z\",\"views\":1},{\"date\":\"2024-12-04T02:39:25.324Z\",\"views\":0},{\"date\":\"2024-11-30T14:39:25.339Z\",\"views\":1},{\"date\":\"2024-11-27T02:39:25.364Z\",\"views\":0},{\"date\":\"2024-11-23T14:39:25.388Z\",\"views\":0},{\"date\":\"2024-11-20T02:39:25.407Z\",\"views\":2},{\"date\":\"2024-11-16T14:39:25.426Z\",\"views\":1},{\"date\":\"2024-11-13T02:39:25.442Z\",\"views\":2},{\"date\":\"2024-11-09T14:39:25.457Z\",\"views\":10},{\"date\":\"2024-11-06T02:39:25.472Z\",\"views\":0},{\"date\":\"2024-11-02T13:39:25.487Z\",\"views\":2},{\"date\":\"2024-10-30T01:39:25.504Z\",\"views\":0},{\"date\":\"2024-10-26T13:39:25.521Z\",\"views\":0},{\"date\":\"2024-10-23T01:39:25.535Z\",\"views\":1},{\"date\":\"2024-10-19T13:39:25.554Z\",\"views\":2},{\"date\":\"2024-10-16T01:39:25.572Z\",\"views\":1},{\"date\":\"2024-10-12T13:39:25.595Z\",\"views\":1},{\"date\":\"2024-10-09T01:39:25.615Z\",\"views\":0},{\"date\":\"2024-10-05T13:39:25.642Z\",\"views\":0},{\"date\":\"2024-10-02T01:39:25.667Z\",\"views\":2},{\"date\":\"2024-09-28T13:39:25.687Z\",\"views\":1},{\"date\":\"2024-09-25T01:39:25.706Z\",\"views\":0},{\"date\":\"2024-09-21T13:39:25.728Z\",\"views\":0},{\"date\":\"2024-09-18T01:39:25.744Z\",\"views\":2},{\"date\":\"2024-09-14T13:39:25.762Z\",\"views\":0},{\"date\":\"2024-09-11T01:39:25.783Z\",\"views\":1},{\"date\":\"2024-09-07T13:39:25.805Z\",\"views\":0},{\"date\":\"2024-09-04T01:39:25.828Z\",\"views\":0},{\"date\":\"2024-08-31T13:39:25.845Z\",\"views\":2},{\"date\":\"2024-08-28T01:39:25.865Z\",\"views\":0}]},\"ranking\":{\"current_rank\":141712,\"previous_rank\":140524,\"activity_score\":0,\"paper_score\":0},\"is_hidden\":false,\"custom_categories\":[\"multi-modal-learning\",\"object-detection\",\"self-supervised-learning\",\"visual-reasoning\",\"robotics-perception\"],\"first_publication_date\":\"2023-03-23T17:43:11.000Z\",\"author_user_ids\":[],\"resources\":{\"github\":{\"url\":\"https://github.com/WikiChao/Ego-AV-Loc\",\"description\":\"[CVPR 2023] Egocentric Audio-Visual Object Localization\",\"language\":\"Python\",\"stars\":24}},\"organizations\":[\"67be637baa92218ccd8b119d\",\"67be637faa92218ccd8b12b7\"],\"paperVersions\":{\"_id\":\"673d85f3181e8ac859336c0e\",\"paper_group_id\":\"673d85f2181e8ac859336c0d\",\"version_label\":\"v1\",\"version_order\":1,\"title\":\"Egocentric Audio-Visual Object Localization\",\"abstract\":\"$25\",\"author_ids\":[\"672bc883986a1370676d7b4b\",\"672bd49a986a1370676e587c\",\"6732224acd1e32a6e7efd05d\",\"6732237ccd1e32a6e7efe53d\"],\"publication_date\":\"2023-03-23T17:43:11.000Z\",\"license\":\"http://creativecommons.org/licenses/by/4.0/\",\"created_at\":\"2024-11-20T06:47:15.217Z\",\"updated_at\":\"2024-11-20T06:47:15.217Z\",\"is_deleted\":false,\"is_hidden\":false,\"universal_paper_id\":\"2303.13471\",\"imageURL\":\"image/2303.13471v1.png\"},\"verifiedAuthors\":[],\"authors\":[{\"_id\":\"672bc883986a1370676d7b4b\",\"full_name\":\"Chao Huang\",\"affiliation\":null,\"orcid\":null,\"semantic_scholarid\":null,\"user_id\":null},{\"_id\":\"672bd49a986a1370676e587c\",\"full_name\":\"Yapeng Tian\",\"affiliation\":null,\"orcid\":null,\"semantic_scholarid\":null,\"user_id\":null},{\"_id\":\"6732224acd1e32a6e7efd05d\",\"full_name\":\"Anurag Kumar\",\"affiliation\":null,\"orcid\":null,\"semantic_scholarid\":null,\"user_id\":null},{\"_id\":\"6732237ccd1e32a6e7efe53d\",\"full_name\":\"Chenliang Xu\",\"affiliation\":null,\"orcid\":null,\"semantic_scholarid\":null,\"user_id\":null}]},\"max_version_order\":1,\"verified_authors\":[],\"authors\":[{\"_id\":\"672bc883986a1370676d7b4b\",\"full_name\":\"Chao Huang\",\"affiliation\":null,\"orcid\":null,\"semantic_scholarid\":null,\"user_id\":null},{\"_id\":\"672bd49a986a1370676e587c\",\"full_name\":\"Yapeng Tian\",\"affiliation\":null,\"orcid\":null,\"semantic_scholarid\":null,\"user_id\":null},{\"_id\":\"6732224acd1e32a6e7efd05d\",\"full_name\":\"Anurag Kumar\",\"affiliation\":null,\"orcid\":null,\"semantic_scholarid\":null,\"user_id\":null},{\"_id\":\"6732237ccd1e32a6e7efe53d\",\"full_name\":\"Chenliang Xu\",\"affiliation\":null,\"orcid\":null,\"semantic_scholarid\":null,\"user_id\":null}],\"pdf_info\":{\"fetcher_url\":\"https://fetcher.alphaxiv.org/v2/pdf/2303.13471v1\"}}},\"dataUpdateCount\":1,\"dataUpdatedAt\":1744228174958,\"error\":null,\"errorUpdateCount\":0,\"errorUpdatedAt\":0,\"fetchFailureCount\":0,\"fetchFailureReason\":null,\"fetchMeta\":null,\"isInvalidated\":false,\"status\":\"success\",\"fetchStatus\":\"idle\"},\"queryKey\":[\"paper\",\"2303.13471\",\"metadata\"],\"queryHash\":\"[\\\"paper\\\",\\\"2303.13471\\\",\\\"metadata\\\"]\"},{\"state\":{\"data\":{\"data\":[]},\"dataUpdateCount\":1,\"dataUpdatedAt\":1744228174958,\"error\":null,\"errorUpdateCount\":0,\"errorUpdatedAt\":0,\"fetchFailureCount\":0,\"fetchFailureReason\":null,\"fetchMeta\":null,\"isInvalidated\":false,\"status\":\"success\",\"fetchStatus\":\"idle\"},\"queryKey\":[\"paper\",\"2303.13471\",\"comments\"],\"queryHash\":\"[\\\"paper\\\",\\\"2303.13471\\\",\\\"comments\\\"]\"},{\"state\":{\"data\":{\"data\":{\"paper_version\":{\"_id\":\"67a2e936ee262751fe28b196\",\"paper_group_id\":\"67a2e935ee262751fe28b195\",\"version_label\":\"v3\",\"version_order\":3,\"title\":\"Generalization of Quantum Machine Learning Models Using Quantum Fisher Information Metric\",\"abstract\":\"Generalization is the ability of machine learning models to make accurate predictions on new data by learning from training data. However, understanding generalization of quantum machine learning models has been a major challenge. Here, we introduce the data quantum Fisher information metric (DQFIM). It describes the capacity of variational quantum algorithms depending on variational ansatz, training data and their symmetries. We apply the DQFIM to quantify circuit parameters and training data needed to successfully train and generalize. Using the dynamical Lie algebra, we explain how to generalize using a low number of training states. Counter-intuitively, breaking symmetries of the training data can help to improve generalization. Finally, we find that out-of-distribution generalization, where training and testing data are drawn from different data distributions, can be better than using the same distribution. Our work provides a useful framework to explore the power of quantum machine learning models.\",\"author_ids\":[\"67323005cd1e32a6e7f0af99\",\"6762e98a8455f19bd6e508a4\"],\"publication_date\":\"2024-07-27T11:57:52.000Z\",\"license\":\"http://arxiv.org/licenses/nonexclusive-distrib/1.0/\",\"created_at\":\"2025-02-05T04:29:42.155Z\",\"updated_at\":\"2025-02-05T04:29:42.155Z\",\"is_deleted\":false,\"is_hidden\":false,\"universal_paper_id\":\"2303.13462\",\"imageURL\":\"image/2303.13462v3.png\"},\"paper_group\":{\"_id\":\"67a2e935ee262751fe28b195\",\"universal_paper_id\":\"2303.13462\",\"title\":\"Generalization of Quantum Machine Learning Models Using Quantum Fisher Information Metric\",\"created_at\":\"2025-02-05T04:29:41.319Z\",\"updated_at\":\"2025-03-03T20:18:37.717Z\",\"categories\":[\"Physics\",\"Computer Science\",\"Statistics\"],\"subcategories\":[\"quant-ph\",\"cs.LG\",\"stat.ML\"],\"custom_categories\":[\"quantum-machine-learning\",\"statistical-learning\",\"representation-learning\"],\"author_user_ids\":[],\"source\":{\"name\":\"alphaXiv\",\"url\":\"https://arxiv.org/abs/2303.13462\"},\"metrics\":{\"activity_rank\":0,\"questions_count\":0,\"responses_count\":0,\"upvotes_count\":0,\"downvotes_count\":0,\"total_votes\":0,\"public_total_votes\":6,\"visits_count\":{\"last24Hours\":6,\"last7Days\":8,\"last30Days\":10,\"last90Days\":13,\"all\":39},\"weighted_visits\":{\"last24Hours\":7.477364404057587e-129,\"last7Days\":3.0773388168658284e-18,\"last30Days\":0.0005048781508384321,\"last90Days\":0.48047656623961216,\"hot\":3.0773388168658284e-18},\"timeline\":[{\"date\":\"2025-04-02T02:39:24.947Z\",\"views\":7},{\"date\":\"2025-03-29T14:39:24.947Z\",\"views\":1},{\"date\":\"2025-03-26T02:39:24.947Z\",\"views\":1},{\"date\":\"2025-03-22T14:39:24.947Z\",\"views\":0},{\"date\":\"2025-03-19T02:39:24.947Z\",\"views\":1},{\"date\":\"2025-03-15T14:39:24.947Z\",\"views\":3},{\"date\":\"2025-03-12T02:39:24.947Z\",\"views\":4},{\"date\":\"2025-03-08T14:39:24.947Z\",\"views\":0},{\"date\":\"2025-03-05T02:39:24.947Z\",\"views\":1},{\"date\":\"2025-03-01T14:39:24.947Z\",\"views\":1},{\"date\":\"2025-02-26T02:39:24.947Z\",\"views\":0},{\"date\":\"2025-02-22T14:39:24.947Z\",\"views\":1},{\"date\":\"2025-02-19T02:39:24.959Z\",\"views\":1},{\"date\":\"2025-02-15T14:39:24.973Z\",\"views\":2},{\"date\":\"2025-02-12T02:39:24.990Z\",\"views\":2},{\"date\":\"2025-02-08T14:39:25.009Z\",\"views\":0},{\"date\":\"2025-02-05T02:39:25.022Z\",\"views\":10},{\"date\":\"2025-02-01T14:39:25.039Z\",\"views\":2},{\"date\":\"2025-01-29T02:39:25.056Z\",\"views\":2},{\"date\":\"2025-01-25T14:39:25.073Z\",\"views\":2},{\"date\":\"2025-01-22T02:39:25.087Z\",\"views\":0},{\"date\":\"2025-01-18T14:39:25.104Z\",\"views\":2},{\"date\":\"2025-01-15T02:39:25.123Z\",\"views\":0},{\"date\":\"2025-01-11T14:39:25.137Z\",\"views\":2},{\"date\":\"2025-01-08T02:39:25.158Z\",\"views\":0},{\"date\":\"2025-01-04T14:39:25.175Z\",\"views\":1},{\"date\":\"2025-01-01T02:39:25.191Z\",\"views\":1},{\"date\":\"2024-12-28T14:39:25.211Z\",\"views\":0},{\"date\":\"2024-12-25T02:39:25.231Z\",\"views\":2},{\"date\":\"2024-12-21T14:39:25.249Z\",\"views\":0},{\"date\":\"2024-12-18T02:39:25.263Z\",\"views\":1},{\"date\":\"2024-12-14T14:39:25.280Z\",\"views\":0},{\"date\":\"2024-12-11T02:39:25.295Z\",\"views\":0},{\"date\":\"2024-12-07T14:39:25.315Z\",\"views\":2},{\"date\":\"2024-12-04T02:39:25.331Z\",\"views\":0},{\"date\":\"2024-11-30T14:39:25.346Z\",\"views\":2},{\"date\":\"2024-11-27T02:39:25.367Z\",\"views\":1},{\"date\":\"2024-11-23T14:39:25.390Z\",\"views\":2},{\"date\":\"2024-11-20T02:39:25.411Z\",\"views\":1},{\"date\":\"2024-11-16T14:39:25.433Z\",\"views\":0},{\"date\":\"2024-11-13T02:39:25.450Z\",\"views\":1},{\"date\":\"2024-11-09T14:39:25.465Z\",\"views\":1},{\"date\":\"2024-11-06T02:39:25.482Z\",\"views\":2},{\"date\":\"2024-11-02T13:39:25.499Z\",\"views\":1},{\"date\":\"2024-10-30T01:39:25.525Z\",\"views\":2},{\"date\":\"2024-10-26T13:39:25.543Z\",\"views\":1},{\"date\":\"2024-10-23T01:39:25.558Z\",\"views\":1},{\"date\":\"2024-10-19T13:39:25.573Z\",\"views\":0},{\"date\":\"2024-10-16T01:39:25.594Z\",\"views\":0},{\"date\":\"2024-10-12T13:39:25.614Z\",\"views\":1},{\"date\":\"2024-10-09T01:39:25.638Z\",\"views\":2},{\"date\":\"2024-10-05T13:39:25.666Z\",\"views\":1},{\"date\":\"2024-10-02T01:39:25.686Z\",\"views\":2},{\"date\":\"2024-09-28T13:39:25.703Z\",\"views\":0},{\"date\":\"2024-09-25T01:39:25.719Z\",\"views\":0},{\"date\":\"2024-09-21T13:39:25.741Z\",\"views\":0},{\"date\":\"2024-09-18T01:39:25.760Z\",\"views\":0},{\"date\":\"2024-09-14T13:39:25.780Z\",\"views\":1},{\"date\":\"2024-09-11T01:39:25.798Z\",\"views\":1},{\"date\":\"2024-09-07T13:39:25.821Z\",\"views\":0},{\"date\":\"2024-09-04T01:39:25.838Z\",\"views\":0},{\"date\":\"2024-08-31T13:39:25.857Z\",\"views\":1},{\"date\":\"2024-08-28T01:39:25.877Z\",\"views\":1}]},\"is_hidden\":false,\"first_publication_date\":\"2023-03-23T17:32:20.000Z\",\"paperVersions\":{\"_id\":\"67a2e936ee262751fe28b196\",\"paper_group_id\":\"67a2e935ee262751fe28b195\",\"version_label\":\"v3\",\"version_order\":3,\"title\":\"Generalization of Quantum Machine Learning Models Using Quantum Fisher Information Metric\",\"abstract\":\"Generalization is the ability of machine learning models to make accurate predictions on new data by learning from training data. However, understanding generalization of quantum machine learning models has been a major challenge. Here, we introduce the data quantum Fisher information metric (DQFIM). It describes the capacity of variational quantum algorithms depending on variational ansatz, training data and their symmetries. We apply the DQFIM to quantify circuit parameters and training data needed to successfully train and generalize. Using the dynamical Lie algebra, we explain how to generalize using a low number of training states. Counter-intuitively, breaking symmetries of the training data can help to improve generalization. Finally, we find that out-of-distribution generalization, where training and testing data are drawn from different data distributions, can be better than using the same distribution. Our work provides a useful framework to explore the power of quantum machine learning models.\",\"author_ids\":[\"67323005cd1e32a6e7f0af99\",\"6762e98a8455f19bd6e508a4\"],\"publication_date\":\"2024-07-27T11:57:52.000Z\",\"license\":\"http://arxiv.org/licenses/nonexclusive-distrib/1.0/\",\"created_at\":\"2025-02-05T04:29:42.155Z\",\"updated_at\":\"2025-02-05T04:29:42.155Z\",\"is_deleted\":false,\"is_hidden\":false,\"universal_paper_id\":\"2303.13462\",\"imageURL\":\"image/2303.13462v3.png\"},\"verifiedAuthors\":[],\"authors\":[{\"_id\":\"67323005cd1e32a6e7f0af99\",\"full_name\":\"Tobias Haug\",\"affiliation\":null,\"orcid\":null,\"semantic_scholarid\":null,\"user_id\":null},{\"_id\":\"6762e98a8455f19bd6e508a4\",\"full_name\":\"M.S. Kim\",\"affiliation\":null,\"orcid\":null,\"semantic_scholarid\":null,\"user_id\":null}]},\"max_version_order\":3,\"verified_authors\":[],\"authors\":[{\"_id\":\"67323005cd1e32a6e7f0af99\",\"full_name\":\"Tobias Haug\",\"affiliation\":null,\"orcid\":null,\"semantic_scholarid\":null,\"user_id\":null},{\"_id\":\"6762e98a8455f19bd6e508a4\",\"full_name\":\"M.S. Kim\",\"affiliation\":null,\"orcid\":null,\"semantic_scholarid\":null,\"user_id\":null}],\"pdf_info\":{\"fetcher_url\":\"https://fetcher.alphaxiv.org/v2/pdf/2303.13462v3\"}}},\"dataUpdateCount\":1,\"dataUpdatedAt\":1744228174997,\"error\":null,\"errorUpdateCount\":0,\"errorUpdatedAt\":0,\"fetchFailureCount\":0,\"fetchFailureReason\":null,\"fetchMeta\":null,\"isInvalidated\":false,\"status\":\"success\",\"fetchStatus\":\"idle\"},\"queryKey\":[\"paper\",\"2303.13462\",\"metadata\"],\"queryHash\":\"[\\\"paper\\\",\\\"2303.13462\\\",\\\"metadata\\\"]\"},{\"state\":{\"data\":{\"data\":[]},\"dataUpdateCount\":1,\"dataUpdatedAt\":1744228174997,\"error\":null,\"errorUpdateCount\":0,\"errorUpdatedAt\":0,\"fetchFailureCount\":0,\"fetchFailureReason\":null,\"fetchMeta\":null,\"isInvalidated\":false,\"status\":\"success\",\"fetchStatus\":\"idle\"},\"queryKey\":[\"paper\",\"2303.13462\",\"comments\"],\"queryHash\":\"[\\\"paper\\\",\\\"2303.13462\\\",\\\"comments\\\"]\"},{\"state\":{\"data\":{\"data\":{\"paper_version\":{\"_id\":\"673cccd47d2b7ed9dd51d77d\",\"paper_group_id\":\"673cccd47d2b7ed9dd51d77a\",\"version_label\":\"v1\",\"version_order\":1,\"title\":\"Set-the-Scene: Global-Local Training for Generating Controllable NeRF Scenes\",\"abstract\":\"$26\",\"author_ids\":[\"673cccd47d2b7ed9dd51d77c\",\"67322ffbcd1e32a6e7f0af06\",\"673b7886ee7cdcdc03b14fff\",\"672bcf69986a1370676deb9c\",\"672bbdf0986a1370676d5562\"],\"publication_date\":\"2023-03-23T17:17:29.000Z\",\"license\":\"http://arxiv.org/licenses/nonexclusive-distrib/1.0/\",\"created_at\":\"2024-11-19T17:37:24.754Z\",\"updated_at\":\"2024-11-19T17:37:24.754Z\",\"is_deleted\":false,\"is_hidden\":false,\"universal_paper_id\":\"2303.13450\",\"imageURL\":\"image/2303.13450v1.png\"},\"paper_group\":{\"_id\":\"673cccd47d2b7ed9dd51d77a\",\"universal_paper_id\":\"2303.13450\",\"source\":{\"name\":\"arXiv\",\"url\":\"https://arXiv.org/paper/2303.13450\"},\"title\":\"Set-the-Scene: Global-Local Training for Generating Controllable NeRF Scenes\",\"created_at\":\"2024-10-21T21:17:17.387Z\",\"updated_at\":\"2025-03-03T20:18:37.721Z\",\"categories\":[\"Computer Science\"],\"subcategories\":[\"cs.CV\",\"cs.GR\",\"cs.LG\"],\"metrics\":{\"activity_rank\":0,\"questions_count\":0,\"responses_count\":0,\"upvotes_count\":null,\"downvotes_count\":null,\"total_votes\":0,\"visits_count\":{\"last24Hours\":0,\"last7Days\":1,\"last30Days\":5,\"last90Days\":6,\"all\":48},\"weighted_visits\":{\"last24Hours\":0,\"last7Days\":3.8444065702043394e-19,\"last30Days\":0.00025240435473445407,\"last90Days\":0.22174824777445246,\"hot\":3.8444065702043394e-19},\"public_total_votes\":0,\"timeline\":[{\"date\":\"2025-04-02T02:39:24.951Z\",\"views\":2},{\"date\":\"2025-03-29T14:39:24.951Z\",\"views\":3},{\"date\":\"2025-03-26T02:39:24.951Z\",\"views\":8},{\"date\":\"2025-03-22T14:39:24.951Z\",\"views\":2},{\"date\":\"2025-03-19T02:39:24.951Z\",\"views\":2},{\"date\":\"2025-03-15T14:39:24.951Z\",\"views\":5},{\"date\":\"2025-03-12T02:39:24.951Z\",\"views\":2},{\"date\":\"2025-03-08T14:39:24.951Z\",\"views\":3},{\"date\":\"2025-03-05T02:39:24.951Z\",\"views\":2},{\"date\":\"2025-03-01T14:39:24.951Z\",\"views\":0},{\"date\":\"2025-02-26T02:39:24.951Z\",\"views\":0},{\"date\":\"2025-02-22T14:39:24.951Z\",\"views\":2},{\"date\":\"2025-02-19T02:39:24.966Z\",\"views\":1},{\"date\":\"2025-02-15T14:39:25.006Z\",\"views\":1},{\"date\":\"2025-02-12T02:39:25.021Z\",\"views\":0},{\"date\":\"2025-02-08T14:39:25.040Z\",\"views\":2},{\"date\":\"2025-02-05T02:39:25.057Z\",\"views\":0},{\"date\":\"2025-02-01T14:39:25.075Z\",\"views\":0},{\"date\":\"2025-01-29T02:39:25.091Z\",\"views\":0},{\"date\":\"2025-01-25T14:39:25.109Z\",\"views\":2},{\"date\":\"2025-01-22T02:39:25.125Z\",\"views\":4},{\"date\":\"2025-01-18T14:39:25.145Z\",\"views\":1},{\"date\":\"2025-01-15T02:39:25.161Z\",\"views\":1},{\"date\":\"2025-01-11T14:39:25.176Z\",\"views\":2},{\"date\":\"2025-01-08T02:39:25.191Z\",\"views\":2},{\"date\":\"2025-01-04T14:39:25.210Z\",\"views\":0},{\"date\":\"2025-01-01T02:39:25.228Z\",\"views\":1},{\"date\":\"2024-12-28T14:39:25.242Z\",\"views\":1},{\"date\":\"2024-12-25T02:39:25.259Z\",\"views\":2},{\"date\":\"2024-12-21T14:39:25.275Z\",\"views\":1},{\"date\":\"2024-12-18T02:39:25.290Z\",\"views\":1},{\"date\":\"2024-12-14T14:39:25.307Z\",\"views\":1},{\"date\":\"2024-12-11T02:39:25.326Z\",\"views\":3},{\"date\":\"2024-12-07T14:39:25.342Z\",\"views\":1},{\"date\":\"2024-12-04T02:39:25.364Z\",\"views\":1},{\"date\":\"2024-11-30T14:39:25.389Z\",\"views\":0},{\"date\":\"2024-11-27T02:39:25.408Z\",\"views\":2},{\"date\":\"2024-11-23T14:39:25.426Z\",\"views\":1},{\"date\":\"2024-11-20T02:39:25.444Z\",\"views\":0},{\"date\":\"2024-11-16T14:39:25.464Z\",\"views\":0},{\"date\":\"2024-11-13T02:39:25.479Z\",\"views\":0},{\"date\":\"2024-11-09T14:39:25.496Z\",\"views\":13},{\"date\":\"2024-11-06T02:39:25.525Z\",\"views\":2},{\"date\":\"2024-11-02T13:39:25.540Z\",\"views\":0},{\"date\":\"2024-10-30T01:39:25.555Z\",\"views\":2},{\"date\":\"2024-10-26T13:39:25.574Z\",\"views\":0},{\"date\":\"2024-10-23T01:39:25.596Z\",\"views\":0},{\"date\":\"2024-10-19T13:39:25.624Z\",\"views\":3},{\"date\":\"2024-10-16T01:39:25.647Z\",\"views\":13},{\"date\":\"2024-10-12T13:39:25.670Z\",\"views\":0},{\"date\":\"2024-10-09T01:39:25.690Z\",\"views\":0},{\"date\":\"2024-10-05T13:39:25.707Z\",\"views\":0},{\"date\":\"2024-10-02T01:39:25.729Z\",\"views\":1},{\"date\":\"2024-09-28T13:39:25.751Z\",\"views\":0},{\"date\":\"2024-09-25T01:39:25.771Z\",\"views\":2},{\"date\":\"2024-09-21T13:39:25.789Z\",\"views\":1},{\"date\":\"2024-09-18T01:39:25.811Z\",\"views\":0},{\"date\":\"2024-09-14T13:39:25.835Z\",\"views\":2},{\"date\":\"2024-09-11T01:39:25.853Z\",\"views\":1},{\"date\":\"2024-09-07T13:39:25.871Z\",\"views\":0},{\"date\":\"2024-09-04T01:39:25.888Z\",\"views\":1},{\"date\":\"2024-08-31T13:39:25.902Z\",\"views\":1},{\"date\":\"2024-08-28T01:39:25.914Z\",\"views\":1}]},\"ranking\":{\"current_rank\":91773,\"previous_rank\":91432,\"activity_score\":0,\"paper_score\":0},\"is_hidden\":false,\"custom_categories\":[\"neural-rendering\",\"generative-models\",\"multi-task-learning\",\"computer-vision-security\",\"representation-learning\"],\"first_publication_date\":\"2023-03-23T17:17:29.000Z\",\"author_user_ids\":[],\"organizations\":[\"67be6376aa92218ccd8b0f9a\"],\"paperVersions\":{\"_id\":\"673cccd47d2b7ed9dd51d77d\",\"paper_group_id\":\"673cccd47d2b7ed9dd51d77a\",\"version_label\":\"v1\",\"version_order\":1,\"title\":\"Set-the-Scene: Global-Local Training for Generating Controllable NeRF Scenes\",\"abstract\":\"$27\",\"author_ids\":[\"673cccd47d2b7ed9dd51d77c\",\"67322ffbcd1e32a6e7f0af06\",\"673b7886ee7cdcdc03b14fff\",\"672bcf69986a1370676deb9c\",\"672bbdf0986a1370676d5562\"],\"publication_date\":\"2023-03-23T17:17:29.000Z\",\"license\":\"http://arxiv.org/licenses/nonexclusive-distrib/1.0/\",\"created_at\":\"2024-11-19T17:37:24.754Z\",\"updated_at\":\"2024-11-19T17:37:24.754Z\",\"is_deleted\":false,\"is_hidden\":false,\"universal_paper_id\":\"2303.13450\",\"imageURL\":\"image/2303.13450v1.png\"},\"verifiedAuthors\":[],\"authors\":[{\"_id\":\"672bbdf0986a1370676d5562\",\"full_name\":\"Daniel Cohen-Or\",\"affiliation\":null,\"orcid\":null,\"semantic_scholarid\":null,\"user_id\":null},{\"_id\":\"672bcf69986a1370676deb9c\",\"full_name\":\"Raja Giryes\",\"affiliation\":null,\"orcid\":null,\"semantic_scholarid\":null,\"user_id\":null},{\"_id\":\"67322ffbcd1e32a6e7f0af06\",\"full_name\":\"Elad Richardson\",\"affiliation\":null,\"orcid\":null,\"semantic_scholarid\":null,\"user_id\":null},{\"_id\":\"673b7886ee7cdcdc03b14fff\",\"full_name\":\"Gal Metzer\",\"affiliation\":null,\"orcid\":null,\"semantic_scholarid\":null,\"user_id\":null},{\"_id\":\"673cccd47d2b7ed9dd51d77c\",\"full_name\":\"Dana Cohen-Bar\",\"affiliation\":null,\"orcid\":null,\"semantic_scholarid\":null,\"user_id\":null}]},\"max_version_order\":1,\"verified_authors\":[],\"authors\":[{\"_id\":\"672bbdf0986a1370676d5562\",\"full_name\":\"Daniel Cohen-Or\",\"affiliation\":null,\"orcid\":null,\"semantic_scholarid\":null,\"user_id\":null},{\"_id\":\"672bcf69986a1370676deb9c\",\"full_name\":\"Raja Giryes\",\"affiliation\":null,\"orcid\":null,\"semantic_scholarid\":null,\"user_id\":null},{\"_id\":\"67322ffbcd1e32a6e7f0af06\",\"full_name\":\"Elad Richardson\",\"affiliation\":null,\"orcid\":null,\"semantic_scholarid\":null,\"user_id\":null},{\"_id\":\"673b7886ee7cdcdc03b14fff\",\"full_name\":\"Gal Metzer\",\"affiliation\":null,\"orcid\":null,\"semantic_scholarid\":null,\"user_id\":null},{\"_id\":\"673cccd47d2b7ed9dd51d77c\",\"full_name\":\"Dana Cohen-Bar\",\"affiliation\":null,\"orcid\":null,\"semantic_scholarid\":null,\"user_id\":null}],\"pdf_info\":{\"fetcher_url\":\"https://fetcher.alphaxiv.org/v2/pdf/2303.13450v1\"}}},\"dataUpdateCount\":1,\"dataUpdatedAt\":1744228175454,\"error\":null,\"errorUpdateCount\":0,\"errorUpdatedAt\":0,\"fetchFailureCount\":0,\"fetchFailureReason\":null,\"fetchMeta\":null,\"isInvalidated\":false,\"status\":\"success\",\"fetchStatus\":\"idle\"},\"queryKey\":[\"paper\",\"2303.13450\",\"metadata\"],\"queryHash\":\"[\\\"paper\\\",\\\"2303.13450\\\",\\\"metadata\\\"]\"},{\"state\":{\"data\":{\"data\":[]},\"dataUpdateCount\":1,\"dataUpdatedAt\":1744228175454,\"error\":null,\"errorUpdateCount\":0,\"errorUpdatedAt\":0,\"fetchFailureCount\":0,\"fetchFailureReason\":null,\"fetchMeta\":null,\"isInvalidated\":false,\"status\":\"success\",\"fetchStatus\":\"idle\"},\"queryKey\":[\"paper\",\"2303.13450\",\"comments\"],\"queryHash\":\"[\\\"paper\\\",\\\"2303.13450\\\",\\\"comments\\\"]\"},{\"state\":{\"data\":{\"data\":{\"paper_version\":{\"_id\":\"673b7e5eee7cdcdc03b15c30\",\"paper_group_id\":\"673b7e5eee7cdcdc03b15c2e\",\"version_label\":\"v1\",\"version_order\":1,\"title\":\"CoBIT: A Contrastive Bi-directional Image-Text Generation Model\",\"abstract\":\"$28\",\"author_ids\":[\"672bc5ce986a1370676d6807\",\"672bc0b7986a1370676d657e\",\"673b773bee7cdcdc03b14a80\",\"672bbc9d986a1370676d4ffc\",\"672bc0b8986a1370676d6584\",\"672bbd2b986a1370676d51ff\"],\"publication_date\":\"2023-03-23T17:24:31.000Z\",\"license\":\"http://arxiv.org/licenses/nonexclusive-distrib/1.0/\",\"created_at\":\"2024-11-18T17:50:22.455Z\",\"updated_at\":\"2024-11-18T17:50:22.455Z\",\"is_deleted\":false,\"is_hidden\":false,\"universal_paper_id\":\"2303.13455\",\"imageURL\":\"image/2303.13455v1.png\"},\"paper_group\":{\"_id\":\"673b7e5eee7cdcdc03b15c2e\",\"universal_paper_id\":\"2303.13455\",\"source\":{\"name\":\"arXiv\",\"url\":\"https://arXiv.org/paper/2303.13455\"},\"title\":\"CoBIT: A Contrastive Bi-directional Image-Text Generation Model\",\"created_at\":\"2024-10-26T01:54:04.820Z\",\"updated_at\":\"2025-03-03T20:18:37.720Z\",\"categories\":[\"Computer Science\"],\"subcategories\":[\"cs.CV\",\"cs.CL\"],\"metrics\":{\"activity_rank\":0,\"questions_count\":0,\"responses_count\":0,\"upvotes_count\":null,\"downvotes_count\":null,\"total_votes\":0,\"visits_count\":{\"last24Hours\":0,\"last7Days\":9,\"last30Days\":10,\"last90Days\":13,\"all\":45},\"weighted_visits\":{\"last24Hours\":0,\"last7Days\":3.4609326647544507e-18,\"last30Days\":0.0005048416173697116,\"last90Days\":0.48046497671120336,\"hot\":3.4609326647544507e-18},\"public_total_votes\":0,\"timeline\":[{\"date\":\"2025-04-02T02:39:24.948Z\",\"views\":24},{\"date\":\"2025-03-29T14:39:24.948Z\",\"views\":5},{\"date\":\"2025-03-26T02:39:24.948Z\",\"views\":3},{\"date\":\"2025-03-22T14:39:24.948Z\",\"views\":1},{\"date\":\"2025-03-19T02:39:24.948Z\",\"views\":0},{\"date\":\"2025-03-15T14:39:24.948Z\",\"views\":1},{\"date\":\"2025-03-12T02:39:24.948Z\",\"views\":2},{\"date\":\"2025-03-08T14:39:24.948Z\",\"views\":2},{\"date\":\"2025-03-05T02:39:24.948Z\",\"views\":0},{\"date\":\"2025-03-01T14:39:24.948Z\",\"views\":2},{\"date\":\"2025-02-26T02:39:24.948Z\",\"views\":2},{\"date\":\"2025-02-22T14:39:24.948Z\",\"views\":2},{\"date\":\"2025-02-19T02:39:24.960Z\",\"views\":1},{\"date\":\"2025-02-15T14:39:24.977Z\",\"views\":4},{\"date\":\"2025-02-12T02:39:24.997Z\",\"views\":0},{\"date\":\"2025-02-08T14:39:25.014Z\",\"views\":2},{\"date\":\"2025-02-05T02:39:25.031Z\",\"views\":1},{\"date\":\"2025-02-01T14:39:25.049Z\",\"views\":3},{\"date\":\"2025-01-29T02:39:25.066Z\",\"views\":3},{\"date\":\"2025-01-25T14:39:25.083Z\",\"views\":0},{\"date\":\"2025-01-22T02:39:25.102Z\",\"views\":0},{\"date\":\"2025-01-18T14:39:25.117Z\",\"views\":1},{\"date\":\"2025-01-15T02:39:25.136Z\",\"views\":2},{\"date\":\"2025-01-11T14:39:25.155Z\",\"views\":0},{\"date\":\"2025-01-08T02:39:25.169Z\",\"views\":1},{\"date\":\"2025-01-04T14:39:25.185Z\",\"views\":2},{\"date\":\"2025-01-01T02:39:25.203Z\",\"views\":1},{\"date\":\"2024-12-28T14:39:25.223Z\",\"views\":2},{\"date\":\"2024-12-25T02:39:25.241Z\",\"views\":0},{\"date\":\"2024-12-21T14:39:25.260Z\",\"views\":0},{\"date\":\"2024-12-18T02:39:25.279Z\",\"views\":2},{\"date\":\"2024-12-14T14:39:25.293Z\",\"views\":2},{\"date\":\"2024-12-11T02:39:25.310Z\",\"views\":1},{\"date\":\"2024-12-07T14:39:25.331Z\",\"views\":2},{\"date\":\"2024-12-04T02:39:25.347Z\",\"views\":1},{\"date\":\"2024-11-30T14:39:25.369Z\",\"views\":0},{\"date\":\"2024-11-27T02:39:25.388Z\",\"views\":2},{\"date\":\"2024-11-23T14:39:25.411Z\",\"views\":0},{\"date\":\"2024-11-20T02:39:25.428Z\",\"views\":2},{\"date\":\"2024-11-16T14:39:25.444Z\",\"views\":0},{\"date\":\"2024-11-13T02:39:25.462Z\",\"views\":4},{\"date\":\"2024-11-09T14:39:25.478Z\",\"views\":1},{\"date\":\"2024-11-06T02:39:25.492Z\",\"views\":1},{\"date\":\"2024-11-02T13:39:25.506Z\",\"views\":1},{\"date\":\"2024-10-30T01:39:25.528Z\",\"views\":2},{\"date\":\"2024-10-26T13:39:25.544Z\",\"views\":1},{\"date\":\"2024-10-23T01:39:25.562Z\",\"views\":5},{\"date\":\"2024-10-19T13:39:25.579Z\",\"views\":0},{\"date\":\"2024-10-16T01:39:25.604Z\",\"views\":2},{\"date\":\"2024-10-12T13:39:25.625Z\",\"views\":2},{\"date\":\"2024-10-09T01:39:25.650Z\",\"views\":2},{\"date\":\"2024-10-05T13:39:25.676Z\",\"views\":2},{\"date\":\"2024-10-02T01:39:25.696Z\",\"views\":1},{\"date\":\"2024-09-28T13:39:25.718Z\",\"views\":1},{\"date\":\"2024-09-25T01:39:25.737Z\",\"views\":0},{\"date\":\"2024-09-21T13:39:25.752Z\",\"views\":0},{\"date\":\"2024-09-18T01:39:25.771Z\",\"views\":2},{\"date\":\"2024-09-14T13:39:25.789Z\",\"views\":0},{\"date\":\"2024-09-11T01:39:25.811Z\",\"views\":2},{\"date\":\"2024-09-07T13:39:25.832Z\",\"views\":1},{\"date\":\"2024-09-04T01:39:25.853Z\",\"views\":0},{\"date\":\"2024-08-31T13:39:25.870Z\",\"views\":2},{\"date\":\"2024-08-28T01:39:25.886Z\",\"views\":0}]},\"ranking\":{\"current_rank\":29322,\"previous_rank\":32434,\"activity_score\":0,\"paper_score\":0.34657359027997264},\"is_hidden\":false,\"custom_categories\":null,\"first_publication_date\":\"2023-03-23T17:24:31.000Z\",\"author_user_ids\":[],\"paperVersions\":{\"_id\":\"673b7e5eee7cdcdc03b15c30\",\"paper_group_id\":\"673b7e5eee7cdcdc03b15c2e\",\"version_label\":\"v1\",\"version_order\":1,\"title\":\"CoBIT: A Contrastive Bi-directional Image-Text Generation Model\",\"abstract\":\"$29\",\"author_ids\":[\"672bc5ce986a1370676d6807\",\"672bc0b7986a1370676d657e\",\"673b773bee7cdcdc03b14a80\",\"672bbc9d986a1370676d4ffc\",\"672bc0b8986a1370676d6584\",\"672bbd2b986a1370676d51ff\"],\"publication_date\":\"2023-03-23T17:24:31.000Z\",\"license\":\"http://arxiv.org/licenses/nonexclusive-distrib/1.0/\",\"created_at\":\"2024-11-18T17:50:22.455Z\",\"updated_at\":\"2024-11-18T17:50:22.455Z\",\"is_deleted\":false,\"is_hidden\":false,\"universal_paper_id\":\"2303.13455\",\"imageURL\":\"image/2303.13455v1.png\"},\"verifiedAuthors\":[],\"authors\":[{\"_id\":\"672bbc9d986a1370676d4ffc\",\"full_name\":\"Kai-Wei Chang\",\"affiliation\":null,\"orcid\":null,\"semantic_scholarid\":null,\"user_id\":null},{\"_id\":\"672bbd2b986a1370676d51ff\",\"full_name\":\"Jiahui Yu\",\"affiliation\":null,\"orcid\":null,\"semantic_scholarid\":null,\"user_id\":null},{\"_id\":\"672bc0b7986a1370676d657e\",\"full_name\":\"Mandy Guo\",\"affiliation\":null,\"orcid\":null,\"semantic_scholarid\":null,\"user_id\":null},{\"_id\":\"672bc0b8986a1370676d6584\",\"full_name\":\"Jason Baldridge\",\"affiliation\":null,\"orcid\":null,\"semantic_scholarid\":null,\"user_id\":null},{\"_id\":\"672bc5ce986a1370676d6807\",\"full_name\":\"Haoxuan You\",\"affiliation\":null,\"orcid\":null,\"semantic_scholarid\":null,\"user_id\":null},{\"_id\":\"673b773bee7cdcdc03b14a80\",\"full_name\":\"Zhecan Wang\",\"affiliation\":null,\"orcid\":null,\"semantic_scholarid\":null,\"user_id\":null}]},\"max_version_order\":1,\"verified_authors\":[],\"authors\":[{\"_id\":\"672bbc9d986a1370676d4ffc\",\"full_name\":\"Kai-Wei Chang\",\"affiliation\":null,\"orcid\":null,\"semantic_scholarid\":null,\"user_id\":null},{\"_id\":\"672bbd2b986a1370676d51ff\",\"full_name\":\"Jiahui Yu\",\"affiliation\":null,\"orcid\":null,\"semantic_scholarid\":null,\"user_id\":null},{\"_id\":\"672bc0b7986a1370676d657e\",\"full_name\":\"Mandy Guo\",\"affiliation\":null,\"orcid\":null,\"semantic_scholarid\":null,\"user_id\":null},{\"_id\":\"672bc0b8986a1370676d6584\",\"full_name\":\"Jason Baldridge\",\"affiliation\":null,\"orcid\":null,\"semantic_scholarid\":null,\"user_id\":null},{\"_id\":\"672bc5ce986a1370676d6807\",\"full_name\":\"Haoxuan You\",\"affiliation\":null,\"orcid\":null,\"semantic_scholarid\":null,\"user_id\":null},{\"_id\":\"673b773bee7cdcdc03b14a80\",\"full_name\":\"Zhecan Wang\",\"affiliation\":null,\"orcid\":null,\"semantic_scholarid\":null,\"user_id\":null}],\"pdf_info\":{\"fetcher_url\":\"https://fetcher.alphaxiv.org/v2/pdf/2303.13455v1\"}}},\"dataUpdateCount\":1,\"dataUpdatedAt\":1744228175998,\"error\":null,\"errorUpdateCount\":0,\"errorUpdatedAt\":0,\"fetchFailureCount\":0,\"fetchFailureReason\":null,\"fetchMeta\":null,\"isInvalidated\":false,\"status\":\"success\",\"fetchStatus\":\"idle\"},\"queryKey\":[\"paper\",\"2303.13455\",\"metadata\"],\"queryHash\":\"[\\\"paper\\\",\\\"2303.13455\\\",\\\"metadata\\\"]\"},{\"state\":{\"data\":{\"data\":[]},\"dataUpdateCount\":1,\"dataUpdatedAt\":1744228175998,\"error\":null,\"errorUpdateCount\":0,\"errorUpdatedAt\":0,\"fetchFailureCount\":0,\"fetchFailureReason\":null,\"fetchMeta\":null,\"isInvalidated\":false,\"status\":\"success\",\"fetchStatus\":\"idle\"},\"queryKey\":[\"paper\",\"2303.13455\",\"comments\"],\"queryHash\":\"[\\\"paper\\\",\\\"2303.13455\\\",\\\"comments\\\"]\"},{\"state\":{\"data\":{\"data\":{\"paper_version\":{\"_id\":\"673d916b1e502f9ec7d26982\",\"paper_group_id\":\"673d916b1e502f9ec7d2697f\",\"version_label\":\"v2\",\"version_order\":2,\"title\":\"Physics-Embedded Neural Networks: Graph Neural PDE Solvers with Mixed Boundary Conditions\",\"abstract\":\"$2a\",\"author_ids\":[\"673d916b1e502f9ec7d26980\",\"673d916b1e502f9ec7d26981\"],\"publication_date\":\"2023-03-23T17:24:04.000Z\",\"license\":\"http://arxiv.org/licenses/nonexclusive-distrib/1.0/\",\"created_at\":\"2024-11-20T07:36:11.810Z\",\"updated_at\":\"2024-11-20T07:36:11.810Z\",\"is_deleted\":false,\"is_hidden\":false,\"imageURL\":\"image/2205.11912v2.png\",\"universal_paper_id\":\"2205.11912\"},\"paper_group\":{\"_id\":\"673d916b1e502f9ec7d2697f\",\"universal_paper_id\":\"2205.11912\",\"source\":{\"name\":\"arXiv\",\"url\":\"https://arXiv.org/paper/2205.11912\"},\"title\":\"Physics-Embedded Neural Networks: Graph Neural PDE Solvers with Mixed Boundary Conditions\",\"created_at\":\"2024-11-11T08:32:43.448Z\",\"updated_at\":\"2025-03-03T20:18:37.721Z\",\"categories\":[\"Computer Science\"],\"subcategories\":[\"cs.LG\",\"cs.CE\"],\"metrics\":{\"activity_rank\":0,\"questions_count\":0,\"responses_count\":0,\"upvotes_count\":null,\"downvotes_count\":null,\"total_votes\":0,\"visits_count\":{\"last24Hours\":5,\"last7Days\":7,\"last30Days\":9,\"last90Days\":18,\"all\":60},\"weighted_visits\":{\"last24Hours\":6.216845227691489e-129,\"last7Days\":2.69178832077077e-18,\"last30Days\":0.0004543555574322238,\"last90Days\":0.6652582720888176,\"hot\":2.69178832077077e-18},\"public_total_votes\":6,\"timeline\":[{\"date\":\"2025-04-02T02:39:24.949Z\",\"views\":7},{\"date\":\"2025-03-29T14:39:24.949Z\",\"views\":0},{\"date\":\"2025-03-26T02:39:24.949Z\",\"views\":2},{\"date\":\"2025-03-22T14:39:24.949Z\",\"views\":0},{\"date\":\"2025-03-19T02:39:24.949Z\",\"views\":0},{\"date\":\"2025-03-15T14:39:24.949Z\",\"views\":8},{\"date\":\"2025-03-12T02:39:24.949Z\",\"views\":1},{\"date\":\"2025-03-08T14:39:24.949Z\",\"views\":1},{\"date\":\"2025-03-05T02:39:24.949Z\",\"views\":1},{\"date\":\"2025-03-01T14:39:24.949Z\",\"views\":1},{\"date\":\"2025-02-26T02:39:24.949Z\",\"views\":6},{\"date\":\"2025-02-22T14:39:24.949Z\",\"views\":2},{\"date\":\"2025-02-19T02:39:24.960Z\",\"views\":11},{\"date\":\"2025-02-15T14:39:25.004Z\",\"views\":2},{\"date\":\"2025-02-12T02:39:25.020Z\",\"views\":2},{\"date\":\"2025-02-08T14:39:25.037Z\",\"views\":1},{\"date\":\"2025-02-05T02:39:25.055Z\",\"views\":7},{\"date\":\"2025-02-01T14:39:25.073Z\",\"views\":2},{\"date\":\"2025-01-29T02:39:25.088Z\",\"views\":0},{\"date\":\"2025-01-25T14:39:25.104Z\",\"views\":2},{\"date\":\"2025-01-22T02:39:25.125Z\",\"views\":8},{\"date\":\"2025-01-18T14:39:25.142Z\",\"views\":0},{\"date\":\"2025-01-15T02:39:25.157Z\",\"views\":0},{\"date\":\"2025-01-11T14:39:25.173Z\",\"views\":0},{\"date\":\"2025-01-08T02:39:25.191Z\",\"views\":0},{\"date\":\"2025-01-04T14:39:25.205Z\",\"views\":1},{\"date\":\"2025-01-01T02:39:25.223Z\",\"views\":2},{\"date\":\"2024-12-28T14:39:25.240Z\",\"views\":0},{\"date\":\"2024-12-25T02:39:25.259Z\",\"views\":1},{\"date\":\"2024-12-21T14:39:25.274Z\",\"views\":0},{\"date\":\"2024-12-18T02:39:25.289Z\",\"views\":1},{\"date\":\"2024-12-14T14:39:25.307Z\",\"views\":0},{\"date\":\"2024-12-11T02:39:25.326Z\",\"views\":2},{\"date\":\"2024-12-07T14:39:25.341Z\",\"views\":1},{\"date\":\"2024-12-04T02:39:25.363Z\",\"views\":2},{\"date\":\"2024-11-30T14:39:25.380Z\",\"views\":0},{\"date\":\"2024-11-27T02:39:25.398Z\",\"views\":1},{\"date\":\"2024-11-23T14:39:25.423Z\",\"views\":5},{\"date\":\"2024-11-20T02:39:25.441Z\",\"views\":2},{\"date\":\"2024-11-16T14:39:25.457Z\",\"views\":2},{\"date\":\"2024-11-13T02:39:25.472Z\",\"views\":1},{\"date\":\"2024-11-09T14:39:25.487Z\",\"views\":5},{\"date\":\"2024-11-06T02:39:25.503Z\",\"views\":1},{\"date\":\"2024-11-02T13:39:25.521Z\",\"views\":0},{\"date\":\"2024-10-30T01:39:25.534Z\",\"views\":1},{\"date\":\"2024-10-26T13:39:25.553Z\",\"views\":1},{\"date\":\"2024-10-23T01:39:25.569Z\",\"views\":2},{\"date\":\"2024-10-19T13:39:25.595Z\",\"views\":0},{\"date\":\"2024-10-16T01:39:25.614Z\",\"views\":2},{\"date\":\"2024-10-12T13:39:25.637Z\",\"views\":1},{\"date\":\"2024-10-09T01:39:25.666Z\",\"views\":0},{\"date\":\"2024-10-05T13:39:25.686Z\",\"views\":0},{\"date\":\"2024-10-02T01:39:25.704Z\",\"views\":2},{\"date\":\"2024-09-28T13:39:25.725Z\",\"views\":2},{\"date\":\"2024-09-25T01:39:25.741Z\",\"views\":2},{\"date\":\"2024-09-21T13:39:25.760Z\",\"views\":2},{\"date\":\"2024-09-18T01:39:25.779Z\",\"views\":2},{\"date\":\"2024-09-14T13:39:25.797Z\",\"views\":1},{\"date\":\"2024-09-11T01:39:25.813Z\",\"views\":2},{\"date\":\"2024-09-07T13:39:25.837Z\",\"views\":2},{\"date\":\"2024-09-04T01:39:25.855Z\",\"views\":1},{\"date\":\"2024-08-31T13:39:25.872Z\",\"views\":0},{\"date\":\"2024-08-28T01:39:25.888Z\",\"views\":0}]},\"ranking\":{\"current_rank\":143574,\"previous_rank\":142426,\"activity_score\":0,\"paper_score\":0},\"is_hidden\":false,\"custom_categories\":null,\"first_publication_date\":\"2023-03-23T17:24:04.000Z\",\"author_user_ids\":[],\"paperVersions\":{\"_id\":\"673d916b1e502f9ec7d26982\",\"paper_group_id\":\"673d916b1e502f9ec7d2697f\",\"version_label\":\"v2\",\"version_order\":2,\"title\":\"Physics-Embedded Neural Networks: Graph Neural PDE Solvers with Mixed Boundary Conditions\",\"abstract\":\"$2b\",\"author_ids\":[\"673d916b1e502f9ec7d26980\",\"673d916b1e502f9ec7d26981\"],\"publication_date\":\"2023-03-23T17:24:04.000Z\",\"license\":\"http://arxiv.org/licenses/nonexclusive-distrib/1.0/\",\"created_at\":\"2024-11-20T07:36:11.810Z\",\"updated_at\":\"2024-11-20T07:36:11.810Z\",\"is_deleted\":false,\"is_hidden\":false,\"imageURL\":\"image/2205.11912v2.png\",\"universal_paper_id\":\"2205.11912\"},\"verifiedAuthors\":[],\"authors\":[{\"_id\":\"673d916b1e502f9ec7d26980\",\"full_name\":\"Masanobu Horie\",\"affiliation\":null,\"orcid\":null,\"semantic_scholarid\":null,\"user_id\":null},{\"_id\":\"673d916b1e502f9ec7d26981\",\"full_name\":\"Naoto Mitsume\",\"affiliation\":null,\"orcid\":null,\"semantic_scholarid\":null,\"user_id\":null}]},\"max_version_order\":2,\"verified_authors\":[],\"authors\":[{\"_id\":\"673d916b1e502f9ec7d26980\",\"full_name\":\"Masanobu Horie\",\"affiliation\":null,\"orcid\":null,\"semantic_scholarid\":null,\"user_id\":null},{\"_id\":\"673d916b1e502f9ec7d26981\",\"full_name\":\"Naoto Mitsume\",\"affiliation\":null,\"orcid\":null,\"semantic_scholarid\":null,\"user_id\":null}],\"pdf_info\":{\"fetcher_url\":\"https://fetcher.alphaxiv.org/v2/pdf/2205.11912v2\"}}},\"dataUpdateCount\":1,\"dataUpdatedAt\":1744228176027,\"error\":null,\"errorUpdateCount\":0,\"errorUpdatedAt\":0,\"fetchFailureCount\":0,\"fetchFailureReason\":null,\"fetchMeta\":null,\"isInvalidated\":false,\"status\":\"success\",\"fetchStatus\":\"idle\"},\"queryKey\":[\"paper\",\"2205.11912\",\"metadata\"],\"queryHash\":\"[\\\"paper\\\",\\\"2205.11912\\\",\\\"metadata\\\"]\"},{\"state\":{\"data\":{\"data\":[]},\"dataUpdateCount\":1,\"dataUpdatedAt\":1744228176027,\"error\":null,\"errorUpdateCount\":0,\"errorUpdatedAt\":0,\"fetchFailureCount\":0,\"fetchFailureReason\":null,\"fetchMeta\":null,\"isInvalidated\":false,\"status\":\"success\",\"fetchStatus\":\"idle\"},\"queryKey\":[\"paper\",\"2205.11912\",\"comments\"],\"queryHash\":\"[\\\"paper\\\",\\\"2205.11912\\\",\\\"comments\\\"]\"},{\"state\":{\"data\":{\"data\":{\"paper_version\":{\"_id\":\"67e90245879c7672ace33e9a\",\"paper_group_id\":\"67e90245879c7672ace33e99\",\"version_label\":\"v1\",\"version_order\":1,\"title\":\"A flashing beacon in axion inflation: recurring bursts of gravitational waves in the strong backreaction regime\",\"abstract\":\"$2c\",\"author_ids\":[\"67323405cd1e32a6e7f0e30c\",\"6732570b2aa08508fa76637a\",\"67324b362aa08508fa76536d\",\"6744c86aa36f1403bb85da9b\"],\"publication_date\":\"2023-03-23T16:45:32.000Z\",\"license\":\"http://arxiv.org/licenses/nonexclusive-distrib/1.0/\",\"created_at\":\"2025-03-30T08:35:17.830Z\",\"updated_at\":\"2025-03-30T08:35:17.830Z\",\"is_deleted\":false,\"is_hidden\":false,\"universal_paper_id\":\"2303.13425\",\"imageURL\":\"image/2303.13425v1.png\"},\"paper_group\":{\"_id\":\"67e90245879c7672ace33e99\",\"universal_paper_id\":\"2303.13425\",\"title\":\"A flashing beacon in axion inflation: recurring bursts of gravitational waves in the strong backreaction regime\",\"created_at\":\"2025-03-30T08:35:17.074Z\",\"updated_at\":\"2025-03-30T08:35:17.074Z\",\"categories\":[\"Physics\"],\"subcategories\":[\"astro-ph.CO\",\"hep-ph\"],\"custom_categories\":null,\"author_user_ids\":[],\"source\":{\"name\":\"alphaXiv\",\"url\":\"https://arxiv.org/abs/2303.13425\"},\"metrics\":{\"activity_rank\":0,\"questions_count\":0,\"responses_count\":0,\"upvotes_count\":0,\"downvotes_count\":0,\"total_votes\":0,\"public_total_votes\":2,\"visits_count\":{\"last24Hours\":0,\"last7Days\":0,\"last30Days\":3,\"last90Days\":3,\"all\":3},\"timeline\":[{\"date\":\"2025-04-03T08:24:34.671Z\",\"views\":2},{\"date\":\"2025-03-30T20:24:34.671Z\",\"views\":4},{\"date\":\"2025-03-27T08:24:34.671Z\",\"views\":8},{\"date\":\"2025-03-23T20:24:34.692Z\",\"views\":0},{\"date\":\"2025-03-20T08:24:34.713Z\",\"views\":2},{\"date\":\"2025-03-16T20:24:34.734Z\",\"views\":2},{\"date\":\"2025-03-13T08:24:34.799Z\",\"views\":0},{\"date\":\"2025-03-09T20:24:34.846Z\",\"views\":1},{\"date\":\"2025-03-06T08:24:34.870Z\",\"views\":1},{\"date\":\"2025-03-02T20:24:34.891Z\",\"views\":1},{\"date\":\"2025-02-27T08:24:34.912Z\",\"views\":1},{\"date\":\"2025-02-23T20:24:34.934Z\",\"views\":1},{\"date\":\"2025-02-20T08:24:34.955Z\",\"views\":0},{\"date\":\"2025-02-16T20:24:34.976Z\",\"views\":0},{\"date\":\"2025-02-13T08:24:34.997Z\",\"views\":0},{\"date\":\"2025-02-09T20:24:35.018Z\",\"views\":1},{\"date\":\"2025-02-06T08:24:35.040Z\",\"views\":2},{\"date\":\"2025-02-02T20:24:35.061Z\",\"views\":2},{\"date\":\"2025-01-30T08:24:35.082Z\",\"views\":0},{\"date\":\"2025-01-26T20:24:35.104Z\",\"views\":0},{\"date\":\"2025-01-23T08:24:35.125Z\",\"views\":1},{\"date\":\"2025-01-19T20:24:35.147Z\",\"views\":1},{\"date\":\"2025-01-16T08:24:35.168Z\",\"views\":0},{\"date\":\"2025-01-12T20:24:35.189Z\",\"views\":2},{\"date\":\"2025-01-09T08:24:35.210Z\",\"views\":1},{\"date\":\"2025-01-05T20:24:35.231Z\",\"views\":2},{\"date\":\"2025-01-02T08:24:35.253Z\",\"views\":1},{\"date\":\"2024-12-29T20:24:35.274Z\",\"views\":2},{\"date\":\"2024-12-26T08:24:35.295Z\",\"views\":1},{\"date\":\"2024-12-22T20:24:35.316Z\",\"views\":1},{\"date\":\"2024-12-19T08:24:35.338Z\",\"views\":1},{\"date\":\"2024-12-15T20:24:35.359Z\",\"views\":1},{\"date\":\"2024-12-12T08:24:35.381Z\",\"views\":0},{\"date\":\"2024-12-08T20:24:35.402Z\",\"views\":1},{\"date\":\"2024-12-05T08:24:35.423Z\",\"views\":1},{\"date\":\"2024-12-01T20:24:35.445Z\",\"views\":0},{\"date\":\"2024-11-28T08:24:35.585Z\",\"views\":1},{\"date\":\"2024-11-24T20:24:35.606Z\",\"views\":2},{\"date\":\"2024-11-21T08:24:35.627Z\",\"views\":2},{\"date\":\"2024-11-17T20:24:35.665Z\",\"views\":2},{\"date\":\"2024-11-14T08:24:35.687Z\",\"views\":0},{\"date\":\"2024-11-10T20:24:35.708Z\",\"views\":1},{\"date\":\"2024-11-07T08:24:35.784Z\",\"views\":1},{\"date\":\"2024-11-03T20:24:35.805Z\",\"views\":2},{\"date\":\"2024-10-31T08:24:35.826Z\",\"views\":0},{\"date\":\"2024-10-27T20:24:35.847Z\",\"views\":0},{\"date\":\"2024-10-24T08:24:35.868Z\",\"views\":1},{\"date\":\"2024-10-20T20:24:35.890Z\",\"views\":2},{\"date\":\"2024-10-17T08:24:35.918Z\",\"views\":1},{\"date\":\"2024-10-13T20:24:35.939Z\",\"views\":1},{\"date\":\"2024-10-10T08:24:35.960Z\",\"views\":2},{\"date\":\"2024-10-06T20:24:35.982Z\",\"views\":2},{\"date\":\"2024-10-03T08:24:36.003Z\",\"views\":2},{\"date\":\"2024-09-29T20:24:36.024Z\",\"views\":2}],\"weighted_visits\":{\"last24Hours\":0,\"last7Days\":0,\"last30Days\":0.00014627471549829966,\"last90Days\":0.10959832676676957,\"hot\":0}},\"is_hidden\":false,\"first_publication_date\":\"2023-03-23T16:45:32.000Z\",\"organizations\":[\"67be643daa92218ccd8b33bb\",\"67be6394aa92218ccd8b1881\",\"67be637baa92218ccd8b11be\",\"67be63b8aa92218ccd8b1fdd\",\"67be6378aa92218ccd8b1053\",\"67be6396aa92218ccd8b1911\"],\"paperVersions\":{\"_id\":\"67e90245879c7672ace33e9a\",\"paper_group_id\":\"67e90245879c7672ace33e99\",\"version_label\":\"v1\",\"version_order\":1,\"title\":\"A flashing beacon in axion inflation: recurring bursts of gravitational waves in the strong backreaction regime\",\"abstract\":\"$2d\",\"author_ids\":[\"67323405cd1e32a6e7f0e30c\",\"6732570b2aa08508fa76637a\",\"67324b362aa08508fa76536d\",\"6744c86aa36f1403bb85da9b\"],\"publication_date\":\"2023-03-23T16:45:32.000Z\",\"license\":\"http://arxiv.org/licenses/nonexclusive-distrib/1.0/\",\"created_at\":\"2025-03-30T08:35:17.830Z\",\"updated_at\":\"2025-03-30T08:35:17.830Z\",\"is_deleted\":false,\"is_hidden\":false,\"universal_paper_id\":\"2303.13425\",\"imageURL\":\"image/2303.13425v1.png\"},\"verifiedAuthors\":[],\"authors\":[{\"_id\":\"67323405cd1e32a6e7f0e30c\",\"full_name\":\"Juan Garcia-Bellido\",\"affiliation\":null,\"orcid\":null,\"semantic_scholarid\":null,\"user_id\":null},{\"_id\":\"67324b362aa08508fa76536d\",\"full_name\":\"Marco Peloso\",\"affiliation\":null,\"orcid\":null,\"semantic_scholarid\":null,\"user_id\":null},{\"_id\":\"6732570b2aa08508fa76637a\",\"full_name\":\"Alexandros Papageorgiou\",\"affiliation\":null,\"orcid\":null,\"semantic_scholarid\":null,\"user_id\":null},{\"_id\":\"6744c86aa36f1403bb85da9b\",\"full_name\":\"Lorenzo Sorbo\",\"affiliation\":null,\"orcid\":null,\"semantic_scholarid\":null,\"user_id\":null}]},\"max_version_order\":1,\"verified_authors\":[],\"authors\":[{\"_id\":\"67323405cd1e32a6e7f0e30c\",\"full_name\":\"Juan Garcia-Bellido\",\"affiliation\":null,\"orcid\":null,\"semantic_scholarid\":null,\"user_id\":null},{\"_id\":\"67324b362aa08508fa76536d\",\"full_name\":\"Marco Peloso\",\"affiliation\":null,\"orcid\":null,\"semantic_scholarid\":null,\"user_id\":null},{\"_id\":\"6732570b2aa08508fa76637a\",\"full_name\":\"Alexandros Papageorgiou\",\"affiliation\":null,\"orcid\":null,\"semantic_scholarid\":null,\"user_id\":null},{\"_id\":\"6744c86aa36f1403bb85da9b\",\"full_name\":\"Lorenzo Sorbo\",\"affiliation\":null,\"orcid\":null,\"semantic_scholarid\":null,\"user_id\":null}],\"pdf_info\":{\"fetcher_url\":\"https://fetcher.alphaxiv.org/v2/pdf/2303.13425v1\"}}},\"dataUpdateCount\":1,\"dataUpdatedAt\":1744228176751,\"error\":null,\"errorUpdateCount\":0,\"errorUpdatedAt\":0,\"fetchFailureCount\":0,\"fetchFailureReason\":null,\"fetchMeta\":null,\"isInvalidated\":false,\"status\":\"success\",\"fetchStatus\":\"idle\"},\"queryKey\":[\"paper\",\"2303.13425\",\"metadata\"],\"queryHash\":\"[\\\"paper\\\",\\\"2303.13425\\\",\\\"metadata\\\"]\"},{\"state\":{\"data\":{\"data\":[]},\"dataUpdateCount\":1,\"dataUpdatedAt\":1744228176751,\"error\":null,\"errorUpdateCount\":0,\"errorUpdatedAt\":0,\"fetchFailureCount\":0,\"fetchFailureReason\":null,\"fetchMeta\":null,\"isInvalidated\":false,\"status\":\"success\",\"fetchStatus\":\"idle\"},\"queryKey\":[\"paper\",\"2303.13425\",\"comments\"],\"queryHash\":\"[\\\"paper\\\",\\\"2303.13425\\\",\\\"comments\\\"]\"},{\"state\":{\"data\":{\"data\":{\"paper_version\":{\"_id\":\"6784add2782c46110c9061f2\",\"paper_group_id\":\"6784add1782c46110c9061ef\",\"version_label\":\"v2\",\"version_order\":2,\"title\":\"General solution for the response of materials under radiation and tilted magnetic field: semi-classical regime\",\"abstract\":\"The Berry curvature dipole is well-known to cause Hall conductivity. This study expands on previous results to demonstrate how two- and three-dimensional materials react under a tilted magnetic field in the linear and nonlinear regimes. We show how the Hall effect has a quantum origin by deriving the general form of intrinsic and extrinsic currents in materials under a tilted magnetic field. Our focus is on determining the linear and nonlinear response of two-dimensional materials. We also demonstrate that as the result of the perpendicular component of the magnetic field a current resulted by both velocity and Berry curvature can occur in two-dimensional materials and topological crystalline insulators in second harmonic generation and ratchet responses. The findings of this research may provide insight into the transport characteristics of materials in the semi-classical regime and initiate a new chapter in linear and nonlinear Hall effects.\",\"author_ids\":[\"6784add1782c46110c9061f0\",\"6784add2782c46110c9061f1\"],\"publication_date\":\"2025-01-10T08:04:43.000Z\",\"license\":\"http://creativecommons.org/licenses/by/4.0/\",\"created_at\":\"2025-01-13T06:08:18.256Z\",\"updated_at\":\"2025-01-13T06:08:18.256Z\",\"is_deleted\":false,\"is_hidden\":false,\"universal_paper_id\":\"2406.07987\",\"imageURL\":\"image/2406.07987v2.png\"},\"paper_group\":{\"_id\":\"6784add1782c46110c9061ef\",\"universal_paper_id\":\"2406.07987\",\"title\":\"General solution for the response of materials under radiation and tilted magnetic field: semi-classical regime\",\"created_at\":\"2025-01-13T06:08:17.588Z\",\"updated_at\":\"2025-03-03T19:37:42.387Z\",\"categories\":[\"Physics\"],\"subcategories\":[\"cond-mat.mes-hall\"],\"custom_categories\":null,\"author_user_ids\":[],\"source\":{\"name\":\"alphaXiv\",\"url\":\"https://arxiv.org/paper/2406.07987\"},\"metrics\":{\"activity_rank\":0,\"questions_count\":0,\"responses_count\":0,\"upvotes_count\":0,\"downvotes_count\":0,\"total_votes\":0,\"visits_count\":{\"last24Hours\":0,\"last7Days\":3,\"last30Days\":6,\"last90Days\":7,\"all\":21},\"public_total_votes\":0,\"weighted_visits\":{\"last24Hours\":0,\"last7Days\":0.02571687047195366,\"last30Days\":1.976395478632135,\"last90Days\":7,\"hot\":0.02571687047195366},\"timeline\":[{\"date\":\"2025-04-02T23:41:27.202Z\",\"views\":10},{\"date\":\"2025-03-30T11:41:27.202Z\",\"views\":1},{\"date\":\"2025-03-26T23:41:27.202Z\",\"views\":5},{\"date\":\"2025-03-23T11:41:27.202Z\",\"views\":2},{\"date\":\"2025-03-19T23:41:27.202Z\",\"views\":2},{\"date\":\"2025-03-16T11:41:27.202Z\",\"views\":5},{\"date\":\"2025-03-12T23:41:27.202Z\",\"views\":3},{\"date\":\"2025-03-09T11:41:27.202Z\",\"views\":2},{\"date\":\"2025-03-05T23:41:27.202Z\",\"views\":0},{\"date\":\"2025-03-02T11:41:27.202Z\",\"views\":1},{\"date\":\"2025-02-26T23:41:27.202Z\",\"views\":1},{\"date\":\"2025-02-23T11:41:27.202Z\",\"views\":1},{\"date\":\"2025-02-19T23:41:27.219Z\",\"views\":1},{\"date\":\"2025-02-16T11:41:27.251Z\",\"views\":2},{\"date\":\"2025-02-12T23:41:27.271Z\",\"views\":0},{\"date\":\"2025-02-09T11:41:27.301Z\",\"views\":1},{\"date\":\"2025-02-05T23:41:27.333Z\",\"views\":2},{\"date\":\"2025-02-02T11:41:27.359Z\",\"views\":1},{\"date\":\"2025-01-29T23:41:27.384Z\",\"views\":2},{\"date\":\"2025-01-26T11:41:27.411Z\",\"views\":0},{\"date\":\"2025-01-22T23:41:27.434Z\",\"views\":0},{\"date\":\"2025-01-19T11:41:27.458Z\",\"views\":0},{\"date\":\"2025-01-15T23:41:27.483Z\",\"views\":0},{\"date\":\"2025-01-12T11:41:27.498Z\",\"views\":3},{\"date\":\"2025-01-08T23:41:27.517Z\",\"views\":1}]},\"is_hidden\":false,\"first_publication_date\":\"2025-01-10T08:04:43.000Z\",\"organizations\":[\"67be6388aa92218ccd8b155a\",\"67be6396aa92218ccd8b1915\",\"67be6377aa92218ccd8b1022\",\"67be6376aa92218ccd8b0f68\"],\"citation\":{\"bibtex\":\"@misc{kheirabadi2025generalsolutionresponse,\\n title={General solution for the response of materials under radiation and tilted magnetic field: semi-classical regime}, \\n author={Narjes Kheirabadi and YuanDong Wang},\\n year={2025},\\n eprint={2406.07987},\\n archivePrefix={arXiv},\\n primaryClass={cond-mat.mes-hall},\\n url={https://arxiv.org/abs/2406.07987}, \\n}\"},\"paperVersions\":{\"_id\":\"6784add2782c46110c9061f2\",\"paper_group_id\":\"6784add1782c46110c9061ef\",\"version_label\":\"v2\",\"version_order\":2,\"title\":\"General solution for the response of materials under radiation and tilted magnetic field: semi-classical regime\",\"abstract\":\"The Berry curvature dipole is well-known to cause Hall conductivity. This study expands on previous results to demonstrate how two- and three-dimensional materials react under a tilted magnetic field in the linear and nonlinear regimes. We show how the Hall effect has a quantum origin by deriving the general form of intrinsic and extrinsic currents in materials under a tilted magnetic field. Our focus is on determining the linear and nonlinear response of two-dimensional materials. We also demonstrate that as the result of the perpendicular component of the magnetic field a current resulted by both velocity and Berry curvature can occur in two-dimensional materials and topological crystalline insulators in second harmonic generation and ratchet responses. The findings of this research may provide insight into the transport characteristics of materials in the semi-classical regime and initiate a new chapter in linear and nonlinear Hall effects.\",\"author_ids\":[\"6784add1782c46110c9061f0\",\"6784add2782c46110c9061f1\"],\"publication_date\":\"2025-01-10T08:04:43.000Z\",\"license\":\"http://creativecommons.org/licenses/by/4.0/\",\"created_at\":\"2025-01-13T06:08:18.256Z\",\"updated_at\":\"2025-01-13T06:08:18.256Z\",\"is_deleted\":false,\"is_hidden\":false,\"universal_paper_id\":\"2406.07987\",\"imageURL\":\"image/2406.07987v2.png\"},\"verifiedAuthors\":[],\"authors\":[{\"_id\":\"6784add1782c46110c9061f0\",\"full_name\":\"Narjes Kheirabadi\",\"affiliation\":null,\"orcid\":null,\"semantic_scholarid\":null,\"user_id\":null},{\"_id\":\"6784add2782c46110c9061f1\",\"full_name\":\"YuanDong Wang\",\"affiliation\":null,\"orcid\":null,\"semantic_scholarid\":null,\"user_id\":null}]},\"max_version_order\":2,\"verified_authors\":[],\"authors\":[{\"_id\":\"6784add1782c46110c9061f0\",\"full_name\":\"Narjes Kheirabadi\",\"affiliation\":null,\"orcid\":null,\"semantic_scholarid\":null,\"user_id\":null},{\"_id\":\"6784add2782c46110c9061f1\",\"full_name\":\"YuanDong Wang\",\"affiliation\":null,\"orcid\":null,\"semantic_scholarid\":null,\"user_id\":null}],\"pdf_info\":{\"fetcher_url\":\"https://fetcher.alphaxiv.org/v2/pdf/2406.07987v2\"}}},\"dataUpdateCount\":1,\"dataUpdatedAt\":1744228177771,\"error\":null,\"errorUpdateCount\":0,\"errorUpdatedAt\":0,\"fetchFailureCount\":0,\"fetchFailureReason\":null,\"fetchMeta\":null,\"isInvalidated\":false,\"status\":\"success\",\"fetchStatus\":\"idle\"},\"queryKey\":[\"paper\",\"2406.07987\",\"metadata\"],\"queryHash\":\"[\\\"paper\\\",\\\"2406.07987\\\",\\\"metadata\\\"]\"},{\"state\":{\"data\":{\"data\":[]},\"dataUpdateCount\":1,\"dataUpdatedAt\":1744228177771,\"error\":null,\"errorUpdateCount\":0,\"errorUpdatedAt\":0,\"fetchFailureCount\":0,\"fetchFailureReason\":null,\"fetchMeta\":null,\"isInvalidated\":false,\"status\":\"success\",\"fetchStatus\":\"idle\"},\"queryKey\":[\"paper\",\"2406.07987\",\"comments\"],\"queryHash\":\"[\\\"paper\\\",\\\"2406.07987\\\",\\\"comments\\\"]\"},{\"state\":{\"data\":{\"data\":{\"paper_version\":{\"_id\":\"67a2ea9301641c8699893736\",\"paper_group_id\":\"67a2ea9201641c8699893735\",\"version_label\":\"v2\",\"version_order\":2,\"title\":\"Generalizing through Forgetting -- Domain Generalization for Symptom Event Extraction in Clinical Notes\",\"abstract\":\"$2e\",\"author_ids\":[\"676bb4c4750c5b0e879b74f1\",\"673cb67d8a52218f8bc916ed\",\"67322c15cd1e32a6e7f07866\",\"67322cebcd1e32a6e7f085f7\"],\"publication_date\":\"2023-02-23T20:57:37.000Z\",\"license\":\"http://creativecommons.org/licenses/by/4.0/\",\"created_at\":\"2025-02-05T04:35:31.104Z\",\"updated_at\":\"2025-02-05T04:35:31.104Z\",\"is_deleted\":false,\"is_hidden\":false,\"universal_paper_id\":\"2209.09485\",\"imageURL\":\"image/2209.09485v2.png\"},\"paper_group\":{\"_id\":\"67a2ea9201641c8699893735\",\"universal_paper_id\":\"2209.09485\",\"title\":\"Generalizing through Forgetting -- Domain Generalization for Symptom Event Extraction in Clinical Notes\",\"created_at\":\"2025-02-05T04:35:30.135Z\",\"updated_at\":\"2025-03-03T20:27:05.000Z\",\"categories\":[\"Computer Science\"],\"subcategories\":[\"cs.CL\"],\"custom_categories\":[\"domain-adaptation\",\"information-extraction\",\"transfer-learning\",\"transformers\",\"self-supervised-learning\"],\"author_user_ids\":[],\"source\":{\"name\":\"alphaXiv\",\"url\":\"https://arxiv.org/abs/2209.09485\"},\"metrics\":{\"activity_rank\":0,\"questions_count\":0,\"responses_count\":0,\"upvotes_count\":0,\"downvotes_count\":0,\"total_votes\":0,\"public_total_votes\":0,\"visits_count\":{\"last24Hours\":0,\"last7Days\":1,\"last30Days\":4,\"last90Days\":7,\"all\":21},\"weighted_visits\":{\"last24Hours\":0,\"last7Days\":1.0147139035515231e-23,\"last30Days\":0.000017253352706032247,\"last90Days\":0.11394690752689712,\"hot\":1.0147139035515231e-23},\"timeline\":[{\"date\":\"2025-04-02T02:52:40.154Z\",\"views\":4},{\"date\":\"2025-03-29T14:52:40.154Z\",\"views\":3},{\"date\":\"2025-03-26T02:52:40.154Z\",\"views\":0},{\"date\":\"2025-03-22T14:52:40.154Z\",\"views\":0},{\"date\":\"2025-03-19T02:52:40.154Z\",\"views\":2},{\"date\":\"2025-03-15T14:52:40.154Z\",\"views\":0},{\"date\":\"2025-03-12T02:52:40.154Z\",\"views\":0},{\"date\":\"2025-03-08T14:52:40.154Z\",\"views\":7},{\"date\":\"2025-03-05T02:52:40.154Z\",\"views\":3},{\"date\":\"2025-03-01T14:52:40.154Z\",\"views\":4},{\"date\":\"2025-02-26T02:52:40.154Z\",\"views\":1},{\"date\":\"2025-02-22T14:52:40.154Z\",\"views\":0},{\"date\":\"2025-02-19T02:52:40.174Z\",\"views\":0},{\"date\":\"2025-02-15T14:52:40.192Z\",\"views\":1},{\"date\":\"2025-02-12T02:52:40.208Z\",\"views\":1},{\"date\":\"2025-02-08T14:52:40.225Z\",\"views\":2},{\"date\":\"2025-02-05T02:52:40.240Z\",\"views\":3},{\"date\":\"2025-02-01T14:52:40.256Z\",\"views\":2},{\"date\":\"2025-01-29T02:52:40.273Z\",\"views\":0},{\"date\":\"2025-01-25T14:52:40.289Z\",\"views\":1},{\"date\":\"2025-01-22T02:52:40.304Z\",\"views\":0},{\"date\":\"2025-01-18T14:52:40.319Z\",\"views\":1},{\"date\":\"2025-01-15T02:52:40.345Z\",\"views\":0},{\"date\":\"2025-01-11T14:52:40.385Z\",\"views\":0},{\"date\":\"2025-01-08T02:52:40.408Z\",\"views\":1},{\"date\":\"2025-01-04T14:52:40.425Z\",\"views\":2},{\"date\":\"2025-01-01T02:52:40.443Z\",\"views\":1},{\"date\":\"2024-12-28T14:52:40.462Z\",\"views\":0},{\"date\":\"2024-12-25T02:52:40.476Z\",\"views\":2},{\"date\":\"2024-12-21T14:52:40.490Z\",\"views\":2},{\"date\":\"2024-12-18T02:52:40.509Z\",\"views\":1},{\"date\":\"2024-12-14T14:52:40.527Z\",\"views\":1},{\"date\":\"2024-12-11T02:52:40.543Z\",\"views\":1},{\"date\":\"2024-12-07T14:52:40.559Z\",\"views\":2},{\"date\":\"2024-12-04T02:52:40.575Z\",\"views\":1},{\"date\":\"2024-11-30T14:52:40.598Z\",\"views\":0},{\"date\":\"2024-11-27T02:52:40.625Z\",\"views\":1},{\"date\":\"2024-11-23T14:52:40.669Z\",\"views\":2},{\"date\":\"2024-11-20T02:52:40.707Z\",\"views\":0},{\"date\":\"2024-11-16T14:52:40.733Z\",\"views\":2},{\"date\":\"2024-11-13T02:52:40.788Z\",\"views\":1},{\"date\":\"2024-11-09T14:52:40.821Z\",\"views\":2},{\"date\":\"2024-11-06T02:52:40.837Z\",\"views\":0},{\"date\":\"2024-11-02T13:52:40.882Z\",\"views\":0},{\"date\":\"2024-10-30T01:52:40.918Z\",\"views\":1},{\"date\":\"2024-10-26T13:52:40.936Z\",\"views\":2},{\"date\":\"2024-10-23T01:52:40.951Z\",\"views\":2},{\"date\":\"2024-10-19T13:52:40.967Z\",\"views\":0},{\"date\":\"2024-10-16T01:52:40.987Z\",\"views\":1},{\"date\":\"2024-10-12T13:52:41.004Z\",\"views\":1},{\"date\":\"2024-10-09T01:52:41.022Z\",\"views\":2},{\"date\":\"2024-10-05T13:52:41.041Z\",\"views\":2},{\"date\":\"2024-10-02T01:52:41.055Z\",\"views\":1},{\"date\":\"2024-09-28T13:52:41.073Z\",\"views\":0},{\"date\":\"2024-09-25T01:52:41.089Z\",\"views\":0},{\"date\":\"2024-09-21T13:52:41.105Z\",\"views\":1},{\"date\":\"2024-09-18T01:52:41.126Z\",\"views\":0},{\"date\":\"2024-09-14T13:52:41.145Z\",\"views\":1},{\"date\":\"2024-09-11T01:52:41.164Z\",\"views\":2},{\"date\":\"2024-09-07T13:52:41.181Z\",\"views\":0},{\"date\":\"2024-09-04T01:52:41.199Z\",\"views\":1},{\"date\":\"2024-08-31T13:52:41.216Z\",\"views\":2},{\"date\":\"2024-08-28T01:52:41.235Z\",\"views\":1}]},\"is_hidden\":false,\"first_publication_date\":\"2022-09-20T05:53:22.000Z\",\"organizations\":[\"67be6377aa92218ccd8b0ff9\",\"67be6384aa92218ccd8b1448\"],\"citation\":{\"bibtex\":\"@misc{yetisgen2023generalizingforgettingdomain,\\n title={Generalizing through Forgetting -- Domain Generalization for Symptom Event Extraction in Clinical Notes}, \\n author={Meliha Yetisgen and Mari Ostendorf and Kevin Lybarger and Sitong Zhou},\\n year={2023},\\n eprint={2209.09485},\\n archivePrefix={arXiv},\\n primaryClass={cs.CL},\\n url={https://arxiv.org/abs/2209.09485}, \\n}\"},\"paperVersions\":{\"_id\":\"67a2ea9301641c8699893736\",\"paper_group_id\":\"67a2ea9201641c8699893735\",\"version_label\":\"v2\",\"version_order\":2,\"title\":\"Generalizing through Forgetting -- Domain Generalization for Symptom Event Extraction in Clinical Notes\",\"abstract\":\"$2f\",\"author_ids\":[\"676bb4c4750c5b0e879b74f1\",\"673cb67d8a52218f8bc916ed\",\"67322c15cd1e32a6e7f07866\",\"67322cebcd1e32a6e7f085f7\"],\"publication_date\":\"2023-02-23T20:57:37.000Z\",\"license\":\"http://creativecommons.org/licenses/by/4.0/\",\"created_at\":\"2025-02-05T04:35:31.104Z\",\"updated_at\":\"2025-02-05T04:35:31.104Z\",\"is_deleted\":false,\"is_hidden\":false,\"universal_paper_id\":\"2209.09485\",\"imageURL\":\"image/2209.09485v2.png\"},\"verifiedAuthors\":[],\"authors\":[{\"_id\":\"67322c15cd1e32a6e7f07866\",\"full_name\":\"Meliha Yetisgen\",\"affiliation\":null,\"orcid\":null,\"semantic_scholarid\":null,\"user_id\":null},{\"_id\":\"67322cebcd1e32a6e7f085f7\",\"full_name\":\"Mari Ostendorf\",\"affiliation\":null,\"orcid\":null,\"semantic_scholarid\":null,\"user_id\":null},{\"_id\":\"673cb67d8a52218f8bc916ed\",\"full_name\":\"Kevin Lybarger\",\"affiliation\":null,\"orcid\":null,\"semantic_scholarid\":null,\"user_id\":null},{\"_id\":\"676bb4c4750c5b0e879b74f1\",\"full_name\":\"Sitong Zhou\",\"affiliation\":null,\"orcid\":null,\"semantic_scholarid\":null,\"user_id\":null}]},\"max_version_order\":2,\"verified_authors\":[],\"authors\":[{\"_id\":\"67322c15cd1e32a6e7f07866\",\"full_name\":\"Meliha Yetisgen\",\"affiliation\":null,\"orcid\":null,\"semantic_scholarid\":null,\"user_id\":null},{\"_id\":\"67322cebcd1e32a6e7f085f7\",\"full_name\":\"Mari Ostendorf\",\"affiliation\":null,\"orcid\":null,\"semantic_scholarid\":null,\"user_id\":null},{\"_id\":\"673cb67d8a52218f8bc916ed\",\"full_name\":\"Kevin Lybarger\",\"affiliation\":null,\"orcid\":null,\"semantic_scholarid\":null,\"user_id\":null},{\"_id\":\"676bb4c4750c5b0e879b74f1\",\"full_name\":\"Sitong Zhou\",\"affiliation\":null,\"orcid\":null,\"semantic_scholarid\":null,\"user_id\":null}],\"pdf_info\":{\"fetcher_url\":\"https://fetcher.alphaxiv.org/v2/pdf/2209.09485v2\"}}},\"dataUpdateCount\":1,\"dataUpdatedAt\":1744228177861,\"error\":null,\"errorUpdateCount\":0,\"errorUpdatedAt\":0,\"fetchFailureCount\":0,\"fetchFailureReason\":null,\"fetchMeta\":null,\"isInvalidated\":false,\"status\":\"success\",\"fetchStatus\":\"idle\"},\"queryKey\":[\"paper\",\"2209.09485\",\"metadata\"],\"queryHash\":\"[\\\"paper\\\",\\\"2209.09485\\\",\\\"metadata\\\"]\"},{\"state\":{\"data\":{\"data\":[]},\"dataUpdateCount\":1,\"dataUpdatedAt\":1744228177861,\"error\":null,\"errorUpdateCount\":0,\"errorUpdatedAt\":0,\"fetchFailureCount\":0,\"fetchFailureReason\":null,\"fetchMeta\":null,\"isInvalidated\":false,\"status\":\"success\",\"fetchStatus\":\"idle\"},\"queryKey\":[\"paper\",\"2209.09485\",\"comments\"],\"queryHash\":\"[\\\"paper\\\",\\\"2209.09485\\\",\\\"comments\\\"]\"},{\"state\":{\"data\":{\"data\":{\"paper_version\":{\"_id\":\"675042cf196a35053f127808\",\"paper_group_id\":\"675042cf196a35053f127807\",\"version_label\":\"v1\",\"version_order\":1,\"title\":\"Generalized Loschmidt echo and information scrambling in open systems\",\"abstract\":\"$30\",\"author_ids\":[\"673cbeb17d2b7ed9dd51b7b8\",\"672bc7a6986a1370676d704b\"],\"publication_date\":\"2024-11-29T10:00:00.000Z\",\"license\":\"http://creativecommons.org/licenses/by/4.0/\",\"created_at\":\"2024-12-04T11:53:51.798Z\",\"updated_at\":\"2024-12-04T11:53:51.798Z\",\"is_deleted\":false,\"is_hidden\":false,\"universal_paper_id\":\"2412.01851\",\"imageURL\":\"image/2412.01851v1.png\"},\"paper_group\":{\"_id\":\"675042cf196a35053f127807\",\"universal_paper_id\":\"2412.01851\",\"source\":{\"name\":\"arXiv\",\"url\":\"https://arXiv.org/paper/2412.01851\"},\"title\":\"Generalized Loschmidt echo and information scrambling in open systems\",\"created_at\":\"2024-12-04T10:13:38.283Z\",\"updated_at\":\"2025-03-03T19:39:46.297Z\",\"categories\":[\"Physics\"],\"subcategories\":[\"quant-ph\",\"cond-mat.dis-nn\",\"cond-mat.quant-gas\",\"cond-mat.stat-mech\",\"cond-mat.str-el\"],\"custom_categories\":null,\"metrics\":{\"activity_rank\":0,\"questions_count\":0,\"responses_count\":0,\"upvotes_count\":null,\"downvotes_count\":null,\"total_votes\":0,\"visits_count\":{\"last24Hours\":0,\"last7Days\":2,\"last30Days\":3,\"last90Days\":4,\"all\":31},\"weighted_visits\":{\"last24Hours\":0,\"last7Days\":0.0020866422097579394,\"last30Days\":0.6045312925861992,\"last90Days\":2.3450881305037905,\"hot\":0.0020866422097579394},\"public_total_votes\":0,\"timeline\":[{\"date\":\"2025-04-02T01:12:50.030Z\",\"views\":4},{\"date\":\"2025-03-29T13:12:50.030Z\",\"views\":8},{\"date\":\"2025-03-26T01:12:50.030Z\",\"views\":0},{\"date\":\"2025-03-22T13:12:50.030Z\",\"views\":0},{\"date\":\"2025-03-19T01:12:50.030Z\",\"views\":0},{\"date\":\"2025-03-15T13:12:50.030Z\",\"views\":2},{\"date\":\"2025-03-12T01:12:50.030Z\",\"views\":0},{\"date\":\"2025-03-08T13:12:50.030Z\",\"views\":0},{\"date\":\"2025-03-05T01:12:50.030Z\",\"views\":2},{\"date\":\"2025-03-01T13:12:50.030Z\",\"views\":1},{\"date\":\"2025-02-26T01:12:50.030Z\",\"views\":0},{\"date\":\"2025-02-22T13:12:50.030Z\",\"views\":1},{\"date\":\"2025-02-19T01:12:50.051Z\",\"views\":0},{\"date\":\"2025-02-15T13:12:50.070Z\",\"views\":0},{\"date\":\"2025-02-12T01:12:50.088Z\",\"views\":0},{\"date\":\"2025-02-08T13:12:50.103Z\",\"views\":0},{\"date\":\"2025-02-05T01:12:50.123Z\",\"views\":0},{\"date\":\"2025-02-01T13:12:50.143Z\",\"views\":1},{\"date\":\"2025-01-29T01:12:50.166Z\",\"views\":0},{\"date\":\"2025-01-25T13:12:50.183Z\",\"views\":2},{\"date\":\"2025-01-22T01:12:50.201Z\",\"views\":1},{\"date\":\"2025-01-18T13:12:50.216Z\",\"views\":0},{\"date\":\"2025-01-15T01:12:50.246Z\",\"views\":2},{\"date\":\"2025-01-11T13:12:50.267Z\",\"views\":0},{\"date\":\"2025-01-08T01:12:50.283Z\",\"views\":4},{\"date\":\"2025-01-04T13:12:50.301Z\",\"views\":1},{\"date\":\"2025-01-01T01:12:50.326Z\",\"views\":1},{\"date\":\"2024-12-28T13:12:50.341Z\",\"views\":1},{\"date\":\"2024-12-25T01:12:50.362Z\",\"views\":0},{\"date\":\"2024-12-21T13:12:50.386Z\",\"views\":1},{\"date\":\"2024-12-18T01:12:50.402Z\",\"views\":1},{\"date\":\"2024-12-14T13:12:50.419Z\",\"views\":0},{\"date\":\"2024-12-11T01:12:50.436Z\",\"views\":6},{\"date\":\"2024-12-07T13:12:50.450Z\",\"views\":5},{\"date\":\"2024-12-04T01:12:50.475Z\",\"views\":11}]},\"ranking\":{\"current_rank\":0,\"previous_rank\":0,\"activity_score\":0,\"paper_score\":0},\"is_hidden\":false,\"first_publication_date\":\"2024-12-04T11:53:51.509Z\",\"author_user_ids\":[],\"organizations\":[\"67be6376aa92218ccd8b0f6f\",\"67c2d20c6238d4c4ef210f0e\",\"67be6377aa92218ccd8b0fc3\"],\"citation\":{\"bibtex\":\"@misc{liu2024generalizedloschmidtecho,\\n title={Generalized Loschmidt echo and information scrambling in open systems}, \\n author={Chang Liu and Yi-Neng Zhou},\\n year={2024},\\n eprint={2412.01851},\\n archivePrefix={arXiv},\\n primaryClass={quant-ph},\\n url={https://arxiv.org/abs/2412.01851}, \\n}\"},\"paperVersions\":{\"_id\":\"675042cf196a35053f127808\",\"paper_group_id\":\"675042cf196a35053f127807\",\"version_label\":\"v1\",\"version_order\":1,\"title\":\"Generalized Loschmidt echo and information scrambling in open systems\",\"abstract\":\"$31\",\"author_ids\":[\"673cbeb17d2b7ed9dd51b7b8\",\"672bc7a6986a1370676d704b\"],\"publication_date\":\"2024-11-29T10:00:00.000Z\",\"license\":\"http://creativecommons.org/licenses/by/4.0/\",\"created_at\":\"2024-12-04T11:53:51.798Z\",\"updated_at\":\"2024-12-04T11:53:51.798Z\",\"is_deleted\":false,\"is_hidden\":false,\"universal_paper_id\":\"2412.01851\",\"imageURL\":\"image/2412.01851v1.png\"},\"verifiedAuthors\":[],\"authors\":[{\"_id\":\"672bc7a6986a1370676d704b\",\"full_name\":\"Chang Liu\",\"affiliation\":null,\"orcid\":null,\"semantic_scholarid\":null,\"user_id\":null},{\"_id\":\"673cbeb17d2b7ed9dd51b7b8\",\"full_name\":\"Yi-Neng Zhou\",\"affiliation\":null,\"orcid\":null,\"semantic_scholarid\":null,\"user_id\":null}]},\"max_version_order\":1,\"verified_authors\":[],\"authors\":[{\"_id\":\"672bc7a6986a1370676d704b\",\"full_name\":\"Chang Liu\",\"affiliation\":null,\"orcid\":null,\"semantic_scholarid\":null,\"user_id\":null},{\"_id\":\"673cbeb17d2b7ed9dd51b7b8\",\"full_name\":\"Yi-Neng Zhou\",\"affiliation\":null,\"orcid\":null,\"semantic_scholarid\":null,\"user_id\":null}],\"pdf_info\":{\"fetcher_url\":\"https://fetcher.alphaxiv.org/v2/pdf/2412.01851v1\"}}},\"dataUpdateCount\":1,\"dataUpdatedAt\":1744228184136,\"error\":null,\"errorUpdateCount\":0,\"errorUpdatedAt\":0,\"fetchFailureCount\":0,\"fetchFailureReason\":null,\"fetchMeta\":null,\"isInvalidated\":false,\"status\":\"success\",\"fetchStatus\":\"idle\"},\"queryKey\":[\"paper\",\"2412.01851\",\"metadata\"],\"queryHash\":\"[\\\"paper\\\",\\\"2412.01851\\\",\\\"metadata\\\"]\"},{\"state\":{\"data\":{\"data\":[]},\"dataUpdateCount\":1,\"dataUpdatedAt\":1744228184136,\"error\":null,\"errorUpdateCount\":0,\"errorUpdatedAt\":0,\"fetchFailureCount\":0,\"fetchFailureReason\":null,\"fetchMeta\":null,\"isInvalidated\":false,\"status\":\"success\",\"fetchStatus\":\"idle\"},\"queryKey\":[\"paper\",\"2412.01851\",\"comments\"],\"queryHash\":\"[\\\"paper\\\",\\\"2412.01851\\\",\\\"comments\\\"]\"},{\"state\":{\"data\":{\"data\":{\"paper_version\":{\"_id\":\"673e5dcfb608990f7088bfd8\",\"paper_group_id\":\"673e5dcfb608990f7088bfd7\",\"version_label\":\"v1\",\"version_order\":1,\"title\":\"A Unified Framework for Learned Sparse Retrieval\",\"abstract\":\"$32\",\"author_ids\":[\"672bc9ac986a1370676d8c1c\",\"673cb5c97d2b7ed9dd518ce3\",\"6732298ccd1e32a6e7f04c3e\"],\"publication_date\":\"2023-03-23T16:38:18.000Z\",\"license\":\"http://creativecommons.org/licenses/by/4.0/\",\"created_at\":\"2024-11-20T22:08:15.501Z\",\"updated_at\":\"2024-11-20T22:08:15.501Z\",\"is_deleted\":false,\"is_hidden\":false,\"universal_paper_id\":\"2303.13416\"},\"paper_group\":{\"_id\":\"673e5dcfb608990f7088bfd7\",\"universal_paper_id\":\"2303.13416\",\"source\":{\"name\":\"arXiv\",\"url\":\"https://arXiv.org/paper/2303.13416\"},\"title\":\"A Unified Framework for Learned Sparse Retrieval\",\"created_at\":\"2024-11-19T14:35:31.035Z\",\"updated_at\":\"2025-03-03T20:18:37.725Z\",\"categories\":[\"Computer Science\"],\"subcategories\":[\"cs.IR\"],\"metrics\":{\"activity_rank\":0,\"questions_count\":0,\"responses_count\":0,\"upvotes_count\":null,\"downvotes_count\":null,\"total_votes\":0,\"visits_count\":{\"last24Hours\":0,\"last7Days\":8,\"last30Days\":8,\"last90Days\":9,\"all\":40},\"weighted_visits\":{\"last24Hours\":0,\"last7Days\":3.070746465822671e-18,\"last30Days\":0.00040370046301975616,\"last90Days\":0.3325821447188966,\"hot\":3.070746465822671e-18},\"public_total_votes\":0,\"timeline\":[{\"date\":\"2025-04-02T02:39:25.853Z\",\"views\":25},{\"date\":\"2025-03-29T14:39:25.853Z\",\"views\":2},{\"date\":\"2025-03-26T02:39:25.853Z\",\"views\":2},{\"date\":\"2025-03-22T14:39:25.853Z\",\"views\":1},{\"date\":\"2025-03-19T02:39:25.853Z\",\"views\":2},{\"date\":\"2025-03-15T14:39:25.853Z\",\"views\":1},{\"date\":\"2025-03-12T02:39:25.853Z\",\"views\":0},{\"date\":\"2025-03-08T14:39:25.853Z\",\"views\":0},{\"date\":\"2025-03-05T02:39:25.853Z\",\"views\":0},{\"date\":\"2025-03-01T14:39:25.853Z\",\"views\":2},{\"date\":\"2025-02-26T02:39:25.853Z\",\"views\":2},{\"date\":\"2025-02-22T14:39:25.853Z\",\"views\":3},{\"date\":\"2025-02-19T02:39:25.871Z\",\"views\":1},{\"date\":\"2025-02-15T14:39:25.888Z\",\"views\":2},{\"date\":\"2025-02-12T02:39:25.904Z\",\"views\":1},{\"date\":\"2025-02-08T14:39:25.914Z\",\"views\":2},{\"date\":\"2025-02-05T02:39:25.925Z\",\"views\":2},{\"date\":\"2025-02-01T14:39:25.938Z\",\"views\":0},{\"date\":\"2025-01-29T02:39:25.950Z\",\"views\":1},{\"date\":\"2025-01-25T14:39:25.968Z\",\"views\":2},{\"date\":\"2025-01-22T02:39:25.983Z\",\"views\":0},{\"date\":\"2025-01-18T14:39:26.000Z\",\"views\":2},{\"date\":\"2025-01-15T02:39:26.018Z\",\"views\":2},{\"date\":\"2025-01-11T14:39:26.036Z\",\"views\":0},{\"date\":\"2025-01-08T02:39:26.052Z\",\"views\":0},{\"date\":\"2025-01-04T14:39:26.072Z\",\"views\":2},{\"date\":\"2025-01-01T02:39:26.094Z\",\"views\":2},{\"date\":\"2024-12-28T14:39:26.116Z\",\"views\":3},{\"date\":\"2024-12-25T02:39:26.137Z\",\"views\":0},{\"date\":\"2024-12-21T14:39:26.151Z\",\"views\":0},{\"date\":\"2024-12-18T02:39:26.169Z\",\"views\":1},{\"date\":\"2024-12-14T14:39:26.190Z\",\"views\":2},{\"date\":\"2024-12-11T02:39:26.208Z\",\"views\":2},{\"date\":\"2024-12-07T14:39:26.232Z\",\"views\":1},{\"date\":\"2024-12-04T02:39:26.251Z\",\"views\":0},{\"date\":\"2024-11-30T14:39:26.269Z\",\"views\":2},{\"date\":\"2024-11-27T02:39:26.286Z\",\"views\":0},{\"date\":\"2024-11-23T14:39:26.302Z\",\"views\":1},{\"date\":\"2024-11-20T02:39:26.324Z\",\"views\":8},{\"date\":\"2024-11-16T14:39:26.344Z\",\"views\":5},{\"date\":\"2024-11-13T02:39:26.365Z\",\"views\":1},{\"date\":\"2024-11-09T14:39:26.383Z\",\"views\":1},{\"date\":\"2024-11-06T02:39:26.400Z\",\"views\":1},{\"date\":\"2024-11-02T13:39:26.415Z\",\"views\":2},{\"date\":\"2024-10-30T01:39:26.434Z\",\"views\":0},{\"date\":\"2024-10-26T13:39:26.453Z\",\"views\":2},{\"date\":\"2024-10-23T01:39:26.469Z\",\"views\":0},{\"date\":\"2024-10-19T13:39:26.487Z\",\"views\":0},{\"date\":\"2024-10-16T01:39:26.506Z\",\"views\":2},{\"date\":\"2024-10-12T13:39:26.523Z\",\"views\":0},{\"date\":\"2024-10-09T01:39:26.539Z\",\"views\":0},{\"date\":\"2024-10-05T13:39:26.559Z\",\"views\":1},{\"date\":\"2024-10-02T01:39:26.586Z\",\"views\":1},{\"date\":\"2024-09-28T13:39:26.601Z\",\"views\":1},{\"date\":\"2024-09-25T01:39:26.619Z\",\"views\":0},{\"date\":\"2024-09-21T13:39:26.635Z\",\"views\":2},{\"date\":\"2024-09-18T01:39:26.654Z\",\"views\":0},{\"date\":\"2024-09-14T13:39:26.670Z\",\"views\":0},{\"date\":\"2024-09-11T01:39:26.689Z\",\"views\":1},{\"date\":\"2024-09-07T13:39:26.713Z\",\"views\":2},{\"date\":\"2024-09-04T01:39:26.730Z\",\"views\":0},{\"date\":\"2024-08-31T13:39:26.752Z\",\"views\":2},{\"date\":\"2024-08-28T01:39:26.768Z\",\"views\":1}]},\"ranking\":{\"current_rank\":46060,\"previous_rank\":0,\"activity_score\":0,\"paper_score\":0.34657359027997264},\"is_hidden\":false,\"custom_categories\":null,\"first_publication_date\":\"2023-03-23T16:38:18.000Z\",\"author_user_ids\":[],\"paperVersions\":{\"_id\":\"673e5dcfb608990f7088bfd8\",\"paper_group_id\":\"673e5dcfb608990f7088bfd7\",\"version_label\":\"v1\",\"version_order\":1,\"title\":\"A Unified Framework for Learned Sparse Retrieval\",\"abstract\":\"$33\",\"author_ids\":[\"672bc9ac986a1370676d8c1c\",\"673cb5c97d2b7ed9dd518ce3\",\"6732298ccd1e32a6e7f04c3e\"],\"publication_date\":\"2023-03-23T16:38:18.000Z\",\"license\":\"http://creativecommons.org/licenses/by/4.0/\",\"created_at\":\"2024-11-20T22:08:15.501Z\",\"updated_at\":\"2024-11-20T22:08:15.501Z\",\"is_deleted\":false,\"is_hidden\":false,\"universal_paper_id\":\"2303.13416\"},\"verifiedAuthors\":[],\"authors\":[{\"_id\":\"672bc9ac986a1370676d8c1c\",\"full_name\":\"Thong Nguyen\",\"affiliation\":null,\"orcid\":null,\"semantic_scholarid\":null,\"user_id\":null},{\"_id\":\"6732298ccd1e32a6e7f04c3e\",\"full_name\":\"Andrew Yates\",\"affiliation\":null,\"orcid\":null,\"semantic_scholarid\":null,\"user_id\":null},{\"_id\":\"673cb5c97d2b7ed9dd518ce3\",\"full_name\":\"Sean MacAvaney\",\"affiliation\":null,\"orcid\":null,\"semantic_scholarid\":null,\"user_id\":null}]},\"max_version_order\":1,\"verified_authors\":[],\"authors\":[{\"_id\":\"672bc9ac986a1370676d8c1c\",\"full_name\":\"Thong Nguyen\",\"affiliation\":null,\"orcid\":null,\"semantic_scholarid\":null,\"user_id\":null},{\"_id\":\"6732298ccd1e32a6e7f04c3e\",\"full_name\":\"Andrew Yates\",\"affiliation\":null,\"orcid\":null,\"semantic_scholarid\":null,\"user_id\":null},{\"_id\":\"673cb5c97d2b7ed9dd518ce3\",\"full_name\":\"Sean MacAvaney\",\"affiliation\":null,\"orcid\":null,\"semantic_scholarid\":null,\"user_id\":null}],\"pdf_info\":{\"fetcher_url\":\"https://fetcher.alphaxiv.org/v2/pdf/2303.13416v1\"}}},\"dataUpdateCount\":1,\"dataUpdatedAt\":1744228184929,\"error\":null,\"errorUpdateCount\":0,\"errorUpdatedAt\":0,\"fetchFailureCount\":0,\"fetchFailureReason\":null,\"fetchMeta\":null,\"isInvalidated\":false,\"status\":\"success\",\"fetchStatus\":\"idle\"},\"queryKey\":[\"paper\",\"2303.13416\",\"metadata\"],\"queryHash\":\"[\\\"paper\\\",\\\"2303.13416\\\",\\\"metadata\\\"]\"},{\"state\":{\"data\":{\"data\":[]},\"dataUpdateCount\":1,\"dataUpdatedAt\":1744228184929,\"error\":null,\"errorUpdateCount\":0,\"errorUpdatedAt\":0,\"fetchFailureCount\":0,\"fetchFailureReason\":null,\"fetchMeta\":null,\"isInvalidated\":false,\"status\":\"success\",\"fetchStatus\":\"idle\"},\"queryKey\":[\"paper\",\"2303.13416\",\"comments\"],\"queryHash\":\"[\\\"paper\\\",\\\"2303.13416\\\",\\\"comments\\\"]\"},{\"state\":{\"data\":{\"data\":{\"paper_version\":{\"_id\":\"67dd421df2db88aa3a290ba0\",\"paper_group_id\":\"67dd421cf2db88aa3a290b9f\",\"version_label\":\"v3\",\"version_order\":3,\"title\":\"SLD Fisher information for kinetic uncertainty relations\",\"abstract\":\"$34\",\"author_ids\":[\"67322fb0cd1e32a6e7f0aaf6\",\"67d472b968d0290b21e37e65\"],\"publication_date\":\"2023-08-20T12:35:43.000Z\",\"license\":\"http://arxiv.org/licenses/nonexclusive-distrib/1.0/\",\"created_at\":\"2025-03-21T10:40:29.502Z\",\"updated_at\":\"2025-03-21T10:40:29.502Z\",\"is_deleted\":false,\"is_hidden\":false,\"universal_paper_id\":\"2303.13417\",\"imageURL\":\"image/2303.13417v3.png\"},\"paper_group\":{\"_id\":\"67dd421cf2db88aa3a290b9f\",\"universal_paper_id\":\"2303.13417\",\"title\":\"SLD Fisher information for kinetic uncertainty relations\",\"created_at\":\"2025-03-21T10:40:28.980Z\",\"updated_at\":\"2025-03-21T10:40:28.980Z\",\"categories\":[\"Physics\"],\"subcategories\":[\"cond-mat.stat-mech\",\"quant-ph\"],\"custom_categories\":null,\"author_user_ids\":[],\"source\":{\"name\":\"alphaXiv\",\"url\":\"https://arxiv.org/abs/2303.13417\"},\"metrics\":{\"activity_rank\":0,\"questions_count\":0,\"responses_count\":0,\"upvotes_count\":0,\"downvotes_count\":0,\"total_votes\":0,\"public_total_votes\":3,\"visits_count\":{\"last24Hours\":0,\"last7Days\":1,\"last30Days\":6,\"last90Days\":6,\"all\":18},\"timeline\":[{\"date\":\"2025-04-01T02:41:21.405Z\",\"views\":5},{\"date\":\"2025-03-28T14:41:21.405Z\",\"views\":1},{\"date\":\"2025-03-25T02:41:21.405Z\",\"views\":2},{\"date\":\"2025-03-21T14:41:21.405Z\",\"views\":8},{\"date\":\"2025-03-18T02:41:21.405Z\",\"views\":0},{\"date\":\"2025-03-14T14:41:21.540Z\",\"views\":2},{\"date\":\"2025-03-11T02:41:21.563Z\",\"views\":2},{\"date\":\"2025-03-07T14:41:21.718Z\",\"views\":0},{\"date\":\"2025-03-04T02:41:21.741Z\",\"views\":0},{\"date\":\"2025-02-28T14:41:21.767Z\",\"views\":2},{\"date\":\"2025-02-25T02:41:21.794Z\",\"views\":0},{\"date\":\"2025-02-21T14:41:21.820Z\",\"views\":0},{\"date\":\"2025-02-18T02:41:21.846Z\",\"views\":2},{\"date\":\"2025-02-14T14:41:21.869Z\",\"views\":0},{\"date\":\"2025-02-11T02:41:21.893Z\",\"views\":0},{\"date\":\"2025-02-07T14:41:22.988Z\",\"views\":2},{\"date\":\"2025-02-04T02:41:23.014Z\",\"views\":0},{\"date\":\"2025-01-31T14:41:23.112Z\",\"views\":1},{\"date\":\"2025-01-28T02:41:23.287Z\",\"views\":0},{\"date\":\"2025-01-24T14:41:23.314Z\",\"views\":2},{\"date\":\"2025-01-21T02:41:23.349Z\",\"views\":2},{\"date\":\"2025-01-17T14:41:23.437Z\",\"views\":2},{\"date\":\"2025-01-14T02:41:23.468Z\",\"views\":2},{\"date\":\"2025-01-10T14:41:23.492Z\",\"views\":1},{\"date\":\"2025-01-07T02:41:23.518Z\",\"views\":1},{\"date\":\"2025-01-03T14:41:23.542Z\",\"views\":1},{\"date\":\"2024-12-31T02:41:23.568Z\",\"views\":1},{\"date\":\"2024-12-27T14:41:23.592Z\",\"views\":2},{\"date\":\"2024-12-24T02:41:23.617Z\",\"views\":0},{\"date\":\"2024-12-20T14:41:23.645Z\",\"views\":1},{\"date\":\"2024-12-17T02:41:23.669Z\",\"views\":0},{\"date\":\"2024-12-13T14:41:23.693Z\",\"views\":1},{\"date\":\"2024-12-10T02:41:23.719Z\",\"views\":2},{\"date\":\"2024-12-06T14:41:23.763Z\",\"views\":0},{\"date\":\"2024-12-03T02:41:23.787Z\",\"views\":2},{\"date\":\"2024-11-29T14:41:23.813Z\",\"views\":0},{\"date\":\"2024-11-26T02:41:23.838Z\",\"views\":2},{\"date\":\"2024-11-22T14:41:23.865Z\",\"views\":0},{\"date\":\"2024-11-19T02:41:23.889Z\",\"views\":1},{\"date\":\"2024-11-15T14:41:23.916Z\",\"views\":2},{\"date\":\"2024-11-12T02:41:23.940Z\",\"views\":0},{\"date\":\"2024-11-08T14:41:23.964Z\",\"views\":1},{\"date\":\"2024-11-05T02:41:23.990Z\",\"views\":0},{\"date\":\"2024-11-01T14:41:24.015Z\",\"views\":2},{\"date\":\"2024-10-29T02:41:24.050Z\",\"views\":2},{\"date\":\"2024-10-25T14:41:24.075Z\",\"views\":2},{\"date\":\"2024-10-22T02:41:24.101Z\",\"views\":2},{\"date\":\"2024-10-18T14:41:24.124Z\",\"views\":2},{\"date\":\"2024-10-15T02:41:24.151Z\",\"views\":1},{\"date\":\"2024-10-11T14:41:24.176Z\",\"views\":0},{\"date\":\"2024-10-08T02:41:24.199Z\",\"views\":2},{\"date\":\"2024-10-04T14:41:24.225Z\",\"views\":1},{\"date\":\"2024-10-01T02:41:24.276Z\",\"views\":0},{\"date\":\"2024-09-27T14:41:24.316Z\",\"views\":1},{\"date\":\"2024-09-24T02:41:24.342Z\",\"views\":0},{\"date\":\"2024-09-20T14:41:24.366Z\",\"views\":2}],\"weighted_visits\":{\"last24Hours\":0,\"last7Days\":3.8385872472044413e-19,\"last30Days\":0.0003027781846756029,\"last90Days\":0.2217221224194175,\"hot\":3.8385872472044413e-19}},\"is_hidden\":false,\"first_publication_date\":\"2023-03-23T16:39:19.000Z\",\"organizations\":[\"67c4f48ebc0069d3c4949ae8\"],\"paperVersions\":{\"_id\":\"67dd421df2db88aa3a290ba0\",\"paper_group_id\":\"67dd421cf2db88aa3a290b9f\",\"version_label\":\"v3\",\"version_order\":3,\"title\":\"SLD Fisher information for kinetic uncertainty relations\",\"abstract\":\"$35\",\"author_ids\":[\"67322fb0cd1e32a6e7f0aaf6\",\"67d472b968d0290b21e37e65\"],\"publication_date\":\"2023-08-20T12:35:43.000Z\",\"license\":\"http://arxiv.org/licenses/nonexclusive-distrib/1.0/\",\"created_at\":\"2025-03-21T10:40:29.502Z\",\"updated_at\":\"2025-03-21T10:40:29.502Z\",\"is_deleted\":false,\"is_hidden\":false,\"universal_paper_id\":\"2303.13417\",\"imageURL\":\"image/2303.13417v3.png\"},\"verifiedAuthors\":[],\"authors\":[{\"_id\":\"67322fb0cd1e32a6e7f0aaf6\",\"full_name\":\"Satoshi Nakajima\",\"affiliation\":null,\"orcid\":null,\"semantic_scholarid\":null,\"user_id\":null},{\"_id\":\"67d472b968d0290b21e37e65\",\"full_name\":\"Yasuhiro Utsumi\",\"affiliation\":null,\"orcid\":null,\"semantic_scholarid\":null,\"user_id\":null}]},\"max_version_order\":3,\"verified_authors\":[],\"authors\":[{\"_id\":\"67322fb0cd1e32a6e7f0aaf6\",\"full_name\":\"Satoshi Nakajima\",\"affiliation\":null,\"orcid\":null,\"semantic_scholarid\":null,\"user_id\":null},{\"_id\":\"67d472b968d0290b21e37e65\",\"full_name\":\"Yasuhiro Utsumi\",\"affiliation\":null,\"orcid\":null,\"semantic_scholarid\":null,\"user_id\":null}],\"pdf_info\":{\"fetcher_url\":\"https://fetcher.alphaxiv.org/v2/pdf/2303.13417v3\"}}},\"dataUpdateCount\":1,\"dataUpdatedAt\":1744228185265,\"error\":null,\"errorUpdateCount\":0,\"errorUpdatedAt\":0,\"fetchFailureCount\":0,\"fetchFailureReason\":null,\"fetchMeta\":null,\"isInvalidated\":false,\"status\":\"success\",\"fetchStatus\":\"idle\"},\"queryKey\":[\"paper\",\"2303.13417\",\"metadata\"],\"queryHash\":\"[\\\"paper\\\",\\\"2303.13417\\\",\\\"metadata\\\"]\"},{\"state\":{\"data\":{\"data\":[]},\"dataUpdateCount\":1,\"dataUpdatedAt\":1744228185265,\"error\":null,\"errorUpdateCount\":0,\"errorUpdatedAt\":0,\"fetchFailureCount\":0,\"fetchFailureReason\":null,\"fetchMeta\":null,\"isInvalidated\":false,\"status\":\"success\",\"fetchStatus\":\"idle\"},\"queryKey\":[\"paper\",\"2303.13417\",\"comments\"],\"queryHash\":\"[\\\"paper\\\",\\\"2303.13417\\\",\\\"comments\\\"]\"},{\"state\":{\"data\":{\"data\":{\"paper_version\":{\"_id\":\"67ab78d9914c9db2f85380ea\",\"paper_group_id\":\"67ab78d7914c9db2f85380e4\",\"version_label\":\"v2\",\"version_order\":2,\"title\":\"Automatic Generation of Labeled Data for Video-Based Human Pose Analysis via NLP applied to YouTube Subtitles\",\"abstract\":\"$36\",\"author_ids\":[\"67ab78d7914c9db2f85380e5\",\"67ab78d8914c9db2f85380e6\",\"67ab78d8914c9db2f85380e7\",\"67ab78d9914c9db2f85380e8\",\"67ab78d9914c9db2f85380e9\"],\"publication_date\":\"2023-05-02T08:54:06.000Z\",\"license\":\"http://creativecommons.org/publicdomain/zero/1.0/\",\"created_at\":\"2025-02-11T16:20:41.468Z\",\"updated_at\":\"2025-02-11T16:20:41.468Z\",\"is_deleted\":false,\"is_hidden\":false,\"universal_paper_id\":\"2304.14489\",\"imageURL\":\"image/2304.14489v2.png\"},\"paper_group\":{\"_id\":\"67ab78d7914c9db2f85380e4\",\"universal_paper_id\":\"2304.14489\",\"title\":\"Automatic Generation of Labeled Data for Video-Based Human Pose Analysis via NLP applied to YouTube Subtitles\",\"created_at\":\"2025-02-11T16:20:39.305Z\",\"updated_at\":\"2025-03-03T20:18:37.754Z\",\"categories\":[\"Computer Science\",\"Electrical Engineering and Systems Science\"],\"subcategories\":[\"cs.CV\",\"cs.LG\",\"eess.IV\"],\"custom_categories\":[\"computer-vision-security\",\"synthetic-data\",\"weak-supervision\",\"multi-modal-learning\"],\"author_user_ids\":[],\"source\":{\"name\":\"alphaXiv\",\"url\":\"https://arxiv.org/abs/2304.14489\"},\"metrics\":{\"activity_rank\":0,\"questions_count\":0,\"responses_count\":0,\"upvotes_count\":0,\"downvotes_count\":0,\"total_votes\":0,\"public_total_votes\":0,\"visits_count\":{\"last24Hours\":0,\"last7Days\":0,\"last30Days\":2,\"last90Days\":4,\"all\":4},\"weighted_visits\":{\"last24Hours\":0,\"last7Days\":0,\"last30Days\":0.00010088516703625963,\"last90Days\":0.1477947810876396,\"hot\":0},\"timeline\":[{\"date\":\"2025-04-02T02:39:25.942Z\",\"views\":2},{\"date\":\"2025-03-29T14:39:25.942Z\",\"views\":2},{\"date\":\"2025-03-26T02:39:25.942Z\",\"views\":6},{\"date\":\"2025-03-22T14:39:25.942Z\",\"views\":1},{\"date\":\"2025-03-19T02:39:25.942Z\",\"views\":0},{\"date\":\"2025-03-15T14:39:25.942Z\",\"views\":0},{\"date\":\"2025-03-12T02:39:25.942Z\",\"views\":0},{\"date\":\"2025-03-08T14:39:25.942Z\",\"views\":2},{\"date\":\"2025-03-05T02:39:25.942Z\",\"views\":0},{\"date\":\"2025-03-01T14:39:25.942Z\",\"views\":4},{\"date\":\"2025-02-26T02:39:25.942Z\",\"views\":2},{\"date\":\"2025-02-22T14:39:25.942Z\",\"views\":0},{\"date\":\"2025-02-19T02:39:25.957Z\",\"views\":2},{\"date\":\"2025-02-15T14:39:25.974Z\",\"views\":0},{\"date\":\"2025-02-12T02:39:25.991Z\",\"views\":1},{\"date\":\"2025-02-08T14:39:26.011Z\",\"views\":4},{\"date\":\"2025-02-05T02:39:26.029Z\",\"views\":0},{\"date\":\"2025-02-01T14:39:26.046Z\",\"views\":1},{\"date\":\"2025-01-29T02:39:26.063Z\",\"views\":2},{\"date\":\"2025-01-25T14:39:26.082Z\",\"views\":1},{\"date\":\"2025-01-22T02:39:26.110Z\",\"views\":0},{\"date\":\"2025-01-18T14:39:26.133Z\",\"views\":1},{\"date\":\"2025-01-15T02:39:26.146Z\",\"views\":0},{\"date\":\"2025-01-11T14:39:26.161Z\",\"views\":2},{\"date\":\"2025-01-08T02:39:26.181Z\",\"views\":1},{\"date\":\"2025-01-04T14:39:26.199Z\",\"views\":1},{\"date\":\"2025-01-01T02:39:26.219Z\",\"views\":0},{\"date\":\"2024-12-28T14:39:26.241Z\",\"views\":0},{\"date\":\"2024-12-25T02:39:26.256Z\",\"views\":0},{\"date\":\"2024-12-21T14:39:26.271Z\",\"views\":2},{\"date\":\"2024-12-18T02:39:26.287Z\",\"views\":2},{\"date\":\"2024-12-14T14:39:26.304Z\",\"views\":0},{\"date\":\"2024-12-11T02:39:26.324Z\",\"views\":0},{\"date\":\"2024-12-07T14:39:26.343Z\",\"views\":2},{\"date\":\"2024-12-04T02:39:26.365Z\",\"views\":1},{\"date\":\"2024-11-30T14:39:26.383Z\",\"views\":0},{\"date\":\"2024-11-27T02:39:26.403Z\",\"views\":2},{\"date\":\"2024-11-23T14:39:26.422Z\",\"views\":2},{\"date\":\"2024-11-20T02:39:26.436Z\",\"views\":1},{\"date\":\"2024-11-16T14:39:26.453Z\",\"views\":2},{\"date\":\"2024-11-13T02:39:26.470Z\",\"views\":2},{\"date\":\"2024-11-09T14:39:26.492Z\",\"views\":0},{\"date\":\"2024-11-06T02:39:26.508Z\",\"views\":0},{\"date\":\"2024-11-02T13:39:26.529Z\",\"views\":0},{\"date\":\"2024-10-30T01:39:26.548Z\",\"views\":0},{\"date\":\"2024-10-26T13:39:26.567Z\",\"views\":0},{\"date\":\"2024-10-23T01:39:26.590Z\",\"views\":0},{\"date\":\"2024-10-19T13:39:26.605Z\",\"views\":0},{\"date\":\"2024-10-16T01:39:26.623Z\",\"views\":1},{\"date\":\"2024-10-12T13:39:26.639Z\",\"views\":0},{\"date\":\"2024-10-09T01:39:26.657Z\",\"views\":2},{\"date\":\"2024-10-05T13:39:26.672Z\",\"views\":2},{\"date\":\"2024-10-02T01:39:26.688Z\",\"views\":0},{\"date\":\"2024-09-28T13:39:26.710Z\",\"views\":0},{\"date\":\"2024-09-25T01:39:26.727Z\",\"views\":0},{\"date\":\"2024-09-21T13:39:26.745Z\",\"views\":2},{\"date\":\"2024-09-18T01:39:26.762Z\",\"views\":0},{\"date\":\"2024-09-14T13:39:26.779Z\",\"views\":2},{\"date\":\"2024-09-11T01:39:26.797Z\",\"views\":0},{\"date\":\"2024-09-07T13:39:26.813Z\",\"views\":2},{\"date\":\"2024-09-04T01:39:26.826Z\",\"views\":1},{\"date\":\"2024-08-31T13:39:26.842Z\",\"views\":0},{\"date\":\"2024-08-28T01:39:26.854Z\",\"views\":1}]},\"is_hidden\":false,\"first_publication_date\":\"2023-03-23T15:55:33.000Z\",\"paperVersions\":{\"_id\":\"67ab78d9914c9db2f85380ea\",\"paper_group_id\":\"67ab78d7914c9db2f85380e4\",\"version_label\":\"v2\",\"version_order\":2,\"title\":\"Automatic Generation of Labeled Data for Video-Based Human Pose Analysis via NLP applied to YouTube Subtitles\",\"abstract\":\"$37\",\"author_ids\":[\"67ab78d7914c9db2f85380e5\",\"67ab78d8914c9db2f85380e6\",\"67ab78d8914c9db2f85380e7\",\"67ab78d9914c9db2f85380e8\",\"67ab78d9914c9db2f85380e9\"],\"publication_date\":\"2023-05-02T08:54:06.000Z\",\"license\":\"http://creativecommons.org/publicdomain/zero/1.0/\",\"created_at\":\"2025-02-11T16:20:41.468Z\",\"updated_at\":\"2025-02-11T16:20:41.468Z\",\"is_deleted\":false,\"is_hidden\":false,\"universal_paper_id\":\"2304.14489\",\"imageURL\":\"image/2304.14489v2.png\"},\"verifiedAuthors\":[],\"authors\":[{\"_id\":\"67ab78d7914c9db2f85380e5\",\"full_name\":\"Sebastian Dill\",\"affiliation\":null,\"orcid\":null,\"semantic_scholarid\":null,\"user_id\":null},{\"_id\":\"67ab78d8914c9db2f85380e6\",\"full_name\":\"Susi Zhihan\",\"affiliation\":null,\"orcid\":null,\"semantic_scholarid\":null,\"user_id\":null},{\"_id\":\"67ab78d8914c9db2f85380e7\",\"full_name\":\"Maurice Rohr\",\"affiliation\":null,\"orcid\":null,\"semantic_scholarid\":null,\"user_id\":null},{\"_id\":\"67ab78d9914c9db2f85380e8\",\"full_name\":\"Maziar Sharbafi\",\"affiliation\":null,\"orcid\":null,\"semantic_scholarid\":null,\"user_id\":null},{\"_id\":\"67ab78d9914c9db2f85380e9\",\"full_name\":\"Christoph Hoog Antink\",\"affiliation\":null,\"orcid\":null,\"semantic_scholarid\":null,\"user_id\":null}]},\"max_version_order\":2,\"verified_authors\":[],\"authors\":[{\"_id\":\"67ab78d7914c9db2f85380e5\",\"full_name\":\"Sebastian Dill\",\"affiliation\":null,\"orcid\":null,\"semantic_scholarid\":null,\"user_id\":null},{\"_id\":\"67ab78d8914c9db2f85380e6\",\"full_name\":\"Susi Zhihan\",\"affiliation\":null,\"orcid\":null,\"semantic_scholarid\":null,\"user_id\":null},{\"_id\":\"67ab78d8914c9db2f85380e7\",\"full_name\":\"Maurice Rohr\",\"affiliation\":null,\"orcid\":null,\"semantic_scholarid\":null,\"user_id\":null},{\"_id\":\"67ab78d9914c9db2f85380e8\",\"full_name\":\"Maziar Sharbafi\",\"affiliation\":null,\"orcid\":null,\"semantic_scholarid\":null,\"user_id\":null},{\"_id\":\"67ab78d9914c9db2f85380e9\",\"full_name\":\"Christoph Hoog Antink\",\"affiliation\":null,\"orcid\":null,\"semantic_scholarid\":null,\"user_id\":null}],\"pdf_info\":{\"fetcher_url\":\"https://fetcher.alphaxiv.org/v2/pdf/2304.14489v2\"}}},\"dataUpdateCount\":1,\"dataUpdatedAt\":1744228185627,\"error\":null,\"errorUpdateCount\":0,\"errorUpdatedAt\":0,\"fetchFailureCount\":0,\"fetchFailureReason\":null,\"fetchMeta\":null,\"isInvalidated\":false,\"status\":\"success\",\"fetchStatus\":\"idle\"},\"queryKey\":[\"paper\",\"2304.14489\",\"metadata\"],\"queryHash\":\"[\\\"paper\\\",\\\"2304.14489\\\",\\\"metadata\\\"]\"},{\"state\":{\"data\":{\"data\":[]},\"dataUpdateCount\":1,\"dataUpdatedAt\":1744228185627,\"error\":null,\"errorUpdateCount\":0,\"errorUpdatedAt\":0,\"fetchFailureCount\":0,\"fetchFailureReason\":null,\"fetchMeta\":null,\"isInvalidated\":false,\"status\":\"success\",\"fetchStatus\":\"idle\"},\"queryKey\":[\"paper\",\"2304.14489\",\"comments\"],\"queryHash\":\"[\\\"paper\\\",\\\"2304.14489\\\",\\\"comments\\\"]\"},{\"state\":{\"data\":{\"data\":{\"paper_version\":{\"_id\":\"6756936a6471bd7d8c4ba7d9\",\"paper_group_id\":\"675693696471bd7d8c4ba7d8\",\"version_label\":\"v1\",\"version_order\":1,\"title\":\"Inheriting Bayer's Legacy-Joint Remosaicing and Denoising for Quad Bayer Image Sensor\",\"abstract\":\"$38\",\"author_ids\":[\"672bcdc7986a1370676dcd67\",\"67322697cd1e32a6e7f016ff\",\"672bcf43986a1370676de860\",\"6733a936f4e97503d39f7af1\",\"67322697cd1e32a6e7f01707\",\"6755597bc84394661320a280\",\"674eb2e0e57dd4be770dbd84\",\"673babe4ee7cdcdc03b198f5\"],\"publication_date\":\"2023-03-23T16:16:50.000Z\",\"license\":\"http://arxiv.org/licenses/nonexclusive-distrib/1.0/\",\"created_at\":\"2024-12-09T06:51:22.892Z\",\"updated_at\":\"2024-12-09T06:51:22.892Z\",\"is_deleted\":false,\"is_hidden\":false,\"universal_paper_id\":\"2303.13571\",\"imageURL\":\"image/2303.13571v1.png\"},\"paper_group\":{\"_id\":\"675693696471bd7d8c4ba7d8\",\"universal_paper_id\":\"2303.13571\",\"title\":\"Inheriting Bayer's Legacy-Joint Remosaicing and Denoising for Quad Bayer Image Sensor\",\"created_at\":\"2024-12-09T06:51:21.354Z\",\"updated_at\":\"2025-03-03T20:18:37.744Z\",\"categories\":[\"Computer Science\",\"Electrical Engineering and Systems Science\"],\"subcategories\":[\"cs.CV\",\"eess.IV\"],\"custom_categories\":[\"image-segmentation\",\"computer-vision-security\",\"efficient-transformers\",\"model-compression\",\"image-generation\"],\"author_user_ids\":[],\"source\":{\"name\":\"alphaXiv\",\"url\":\"https://arxiv.org/paper/2303.13571\"},\"metrics\":{\"activity_rank\":0,\"questions_count\":0,\"responses_count\":0,\"upvotes_count\":0,\"downvotes_count\":0,\"total_votes\":0,\"visits_count\":{\"last24Hours\":1,\"last7Days\":1,\"last30Days\":1,\"last90Days\":4,\"all\":18},\"weighted_visits\":{\"last24Hours\":1.2203559174183888e-129,\"last7Days\":3.8351626512936284e-19,\"last30Days\":0.00005045252237890383,\"last90Days\":0.14780448727360207,\"hot\":3.8351626512936284e-19},\"public_total_votes\":3,\"timeline\":[{\"date\":\"2025-04-02T02:39:25.931Z\",\"views\":0},{\"date\":\"2025-03-29T14:39:25.931Z\",\"views\":2},{\"date\":\"2025-03-26T02:39:25.931Z\",\"views\":2},{\"date\":\"2025-03-22T14:39:25.931Z\",\"views\":0},{\"date\":\"2025-03-19T02:39:25.931Z\",\"views\":2},{\"date\":\"2025-03-15T14:39:25.931Z\",\"views\":2},{\"date\":\"2025-03-12T02:39:25.931Z\",\"views\":1},{\"date\":\"2025-03-08T14:39:25.931Z\",\"views\":1},{\"date\":\"2025-03-05T02:39:25.931Z\",\"views\":0},{\"date\":\"2025-03-01T14:39:25.931Z\",\"views\":1},{\"date\":\"2025-02-26T02:39:25.931Z\",\"views\":2},{\"date\":\"2025-02-22T14:39:25.931Z\",\"views\":2},{\"date\":\"2025-02-19T02:39:25.943Z\",\"views\":2},{\"date\":\"2025-02-15T14:39:25.960Z\",\"views\":0},{\"date\":\"2025-02-12T02:39:25.975Z\",\"views\":0},{\"date\":\"2025-02-08T14:39:25.996Z\",\"views\":7},{\"date\":\"2025-02-05T02:39:26.018Z\",\"views\":2},{\"date\":\"2025-02-01T14:39:26.038Z\",\"views\":2},{\"date\":\"2025-01-29T02:39:26.057Z\",\"views\":4},{\"date\":\"2025-01-25T14:39:26.072Z\",\"views\":1},{\"date\":\"2025-01-22T02:39:26.093Z\",\"views\":2},{\"date\":\"2025-01-18T14:39:26.113Z\",\"views\":0},{\"date\":\"2025-01-15T02:39:26.137Z\",\"views\":0},{\"date\":\"2025-01-11T14:39:26.151Z\",\"views\":1},{\"date\":\"2025-01-08T02:39:26.169Z\",\"views\":2},{\"date\":\"2025-01-04T14:39:26.189Z\",\"views\":5},{\"date\":\"2025-01-01T02:39:26.208Z\",\"views\":1},{\"date\":\"2024-12-28T14:39:26.231Z\",\"views\":1},{\"date\":\"2024-12-25T02:39:26.245Z\",\"views\":1},{\"date\":\"2024-12-21T14:39:26.263Z\",\"views\":0},{\"date\":\"2024-12-18T02:39:26.279Z\",\"views\":1},{\"date\":\"2024-12-14T14:39:26.296Z\",\"views\":2},{\"date\":\"2024-12-11T02:39:26.312Z\",\"views\":2},{\"date\":\"2024-12-07T14:39:26.335Z\",\"views\":4},{\"date\":\"2024-12-04T02:39:26.359Z\",\"views\":2},{\"date\":\"2024-11-30T14:39:26.381Z\",\"views\":0},{\"date\":\"2024-11-27T02:39:26.401Z\",\"views\":1},{\"date\":\"2024-11-23T14:39:26.417Z\",\"views\":2},{\"date\":\"2024-11-20T02:39:26.435Z\",\"views\":2},{\"date\":\"2024-11-16T14:39:26.454Z\",\"views\":1},{\"date\":\"2024-11-13T02:39:26.471Z\",\"views\":1},{\"date\":\"2024-11-09T14:39:26.491Z\",\"views\":2},{\"date\":\"2024-11-06T02:39:26.508Z\",\"views\":0},{\"date\":\"2024-11-02T13:39:26.527Z\",\"views\":2},{\"date\":\"2024-10-30T01:39:26.548Z\",\"views\":1},{\"date\":\"2024-10-26T13:39:26.567Z\",\"views\":0},{\"date\":\"2024-10-23T01:39:26.592Z\",\"views\":2},{\"date\":\"2024-10-19T13:39:26.606Z\",\"views\":0},{\"date\":\"2024-10-16T01:39:26.623Z\",\"views\":0},{\"date\":\"2024-10-12T13:39:26.639Z\",\"views\":2},{\"date\":\"2024-10-09T01:39:26.657Z\",\"views\":1},{\"date\":\"2024-10-05T13:39:26.673Z\",\"views\":0},{\"date\":\"2024-10-02T01:39:26.689Z\",\"views\":1},{\"date\":\"2024-09-28T13:39:26.707Z\",\"views\":1},{\"date\":\"2024-09-25T01:39:26.723Z\",\"views\":0},{\"date\":\"2024-09-21T13:39:26.740Z\",\"views\":1},{\"date\":\"2024-09-18T01:39:26.757Z\",\"views\":2},{\"date\":\"2024-09-14T13:39:26.774Z\",\"views\":1},{\"date\":\"2024-09-11T01:39:26.789Z\",\"views\":2},{\"date\":\"2024-09-07T13:39:26.807Z\",\"views\":0},{\"date\":\"2024-09-04T01:39:26.823Z\",\"views\":1},{\"date\":\"2024-08-31T13:39:26.834Z\",\"views\":2},{\"date\":\"2024-08-28T01:39:26.851Z\",\"views\":0}]},\"is_hidden\":false,\"first_publication_date\":\"2023-03-23T16:16:50.000Z\",\"organizations\":[\"67be6385aa92218ccd8b1495\",\"67be63dcaa92218ccd8b263e\",\"67be6563aa92218ccd8b5076\",\"67c5053c50f52819d87c2a8f\",\"67be6377aa92218ccd8b1014\"],\"paperVersions\":{\"_id\":\"6756936a6471bd7d8c4ba7d9\",\"paper_group_id\":\"675693696471bd7d8c4ba7d8\",\"version_label\":\"v1\",\"version_order\":1,\"title\":\"Inheriting Bayer's Legacy-Joint Remosaicing and Denoising for Quad Bayer Image Sensor\",\"abstract\":\"$39\",\"author_ids\":[\"672bcdc7986a1370676dcd67\",\"67322697cd1e32a6e7f016ff\",\"672bcf43986a1370676de860\",\"6733a936f4e97503d39f7af1\",\"67322697cd1e32a6e7f01707\",\"6755597bc84394661320a280\",\"674eb2e0e57dd4be770dbd84\",\"673babe4ee7cdcdc03b198f5\"],\"publication_date\":\"2023-03-23T16:16:50.000Z\",\"license\":\"http://arxiv.org/licenses/nonexclusive-distrib/1.0/\",\"created_at\":\"2024-12-09T06:51:22.892Z\",\"updated_at\":\"2024-12-09T06:51:22.892Z\",\"is_deleted\":false,\"is_hidden\":false,\"universal_paper_id\":\"2303.13571\",\"imageURL\":\"image/2303.13571v1.png\"},\"verifiedAuthors\":[],\"authors\":[{\"_id\":\"672bcdc7986a1370676dcd67\",\"full_name\":\"Haijin Zeng\",\"affiliation\":null,\"orcid\":null,\"semantic_scholarid\":null,\"user_id\":null},{\"_id\":\"672bcf43986a1370676de860\",\"full_name\":\"Jiezhang Cao\",\"affiliation\":null,\"orcid\":null,\"semantic_scholarid\":null,\"user_id\":null},{\"_id\":\"67322697cd1e32a6e7f016ff\",\"full_name\":\"Kai Feng\",\"affiliation\":null,\"orcid\":null,\"semantic_scholarid\":null,\"user_id\":null},{\"_id\":\"67322697cd1e32a6e7f01707\",\"full_name\":\"Yongqiang Zhao\",\"affiliation\":null,\"orcid\":null,\"semantic_scholarid\":null,\"user_id\":null},{\"_id\":\"6733a936f4e97503d39f7af1\",\"full_name\":\"Shaoguang Huang\",\"affiliation\":null,\"orcid\":null,\"semantic_scholarid\":null,\"user_id\":null},{\"_id\":\"673babe4ee7cdcdc03b198f5\",\"full_name\":\"Wilfried Philips\",\"affiliation\":null,\"orcid\":null,\"semantic_scholarid\":null,\"user_id\":null},{\"_id\":\"674eb2e0e57dd4be770dbd84\",\"full_name\":\"Jan Aelterman\",\"affiliation\":null,\"orcid\":null,\"semantic_scholarid\":null,\"user_id\":null},{\"_id\":\"6755597bc84394661320a280\",\"full_name\":\"Hiep Luong\",\"affiliation\":null,\"orcid\":null,\"semantic_scholarid\":null,\"user_id\":null}]},\"max_version_order\":1,\"verified_authors\":[],\"authors\":[{\"_id\":\"672bcdc7986a1370676dcd67\",\"full_name\":\"Haijin Zeng\",\"affiliation\":null,\"orcid\":null,\"semantic_scholarid\":null,\"user_id\":null},{\"_id\":\"672bcf43986a1370676de860\",\"full_name\":\"Jiezhang Cao\",\"affiliation\":null,\"orcid\":null,\"semantic_scholarid\":null,\"user_id\":null},{\"_id\":\"67322697cd1e32a6e7f016ff\",\"full_name\":\"Kai Feng\",\"affiliation\":null,\"orcid\":null,\"semantic_scholarid\":null,\"user_id\":null},{\"_id\":\"67322697cd1e32a6e7f01707\",\"full_name\":\"Yongqiang Zhao\",\"affiliation\":null,\"orcid\":null,\"semantic_scholarid\":null,\"user_id\":null},{\"_id\":\"6733a936f4e97503d39f7af1\",\"full_name\":\"Shaoguang Huang\",\"affiliation\":null,\"orcid\":null,\"semantic_scholarid\":null,\"user_id\":null},{\"_id\":\"673babe4ee7cdcdc03b198f5\",\"full_name\":\"Wilfried Philips\",\"affiliation\":null,\"orcid\":null,\"semantic_scholarid\":null,\"user_id\":null},{\"_id\":\"674eb2e0e57dd4be770dbd84\",\"full_name\":\"Jan Aelterman\",\"affiliation\":null,\"orcid\":null,\"semantic_scholarid\":null,\"user_id\":null},{\"_id\":\"6755597bc84394661320a280\",\"full_name\":\"Hiep Luong\",\"affiliation\":null,\"orcid\":null,\"semantic_scholarid\":null,\"user_id\":null}],\"pdf_info\":{\"fetcher_url\":\"https://fetcher.alphaxiv.org/v2/pdf/2303.13571v1\"}}},\"dataUpdateCount\":1,\"dataUpdatedAt\":1744228185766,\"error\":null,\"errorUpdateCount\":0,\"errorUpdatedAt\":0,\"fetchFailureCount\":0,\"fetchFailureReason\":null,\"fetchMeta\":null,\"isInvalidated\":false,\"status\":\"success\",\"fetchStatus\":\"idle\"},\"queryKey\":[\"paper\",\"2303.13571\",\"metadata\"],\"queryHash\":\"[\\\"paper\\\",\\\"2303.13571\\\",\\\"metadata\\\"]\"},{\"state\":{\"data\":{\"data\":[]},\"dataUpdateCount\":1,\"dataUpdatedAt\":1744228185766,\"error\":null,\"errorUpdateCount\":0,\"errorUpdatedAt\":0,\"fetchFailureCount\":0,\"fetchFailureReason\":null,\"fetchMeta\":null,\"isInvalidated\":false,\"status\":\"success\",\"fetchStatus\":\"idle\"},\"queryKey\":[\"paper\",\"2303.13571\",\"comments\"],\"queryHash\":\"[\\\"paper\\\",\\\"2303.13571\\\",\\\"comments\\\"]\"},{\"state\":{\"data\":{\"data\":{\"paper_version\":{\"_id\":\"673cf1ba615941b897fb57f3\",\"paper_group_id\":\"673cf1ba615941b897fb57f1\",\"version_label\":\"v3\",\"version_order\":3,\"title\":\"FeatER: An Efficient Network for Human Reconstruction via Feature Map-Based TransformER\",\"abstract\":\"$3a\",\"author_ids\":[\"672bbd2c986a1370676d5213\",\"6733e2c829b032f3570982c1\",\"672bcb43986a1370676da1c5\",\"67322590cd1e32a6e7f004e9\",\"672bbf78986a1370676d5ecc\"],\"publication_date\":\"2023-03-23T15:48:05.000Z\",\"license\":\"http://arxiv.org/licenses/nonexclusive-distrib/1.0/\",\"created_at\":\"2024-11-19T20:14:50.373Z\",\"updated_at\":\"2024-11-19T20:14:50.373Z\",\"is_deleted\":false,\"is_hidden\":false,\"universal_paper_id\":\"2205.15448\",\"imageURL\":\"image/2205.15448v3.png\"},\"paper_group\":{\"_id\":\"673cf1ba615941b897fb57f1\",\"universal_paper_id\":\"2205.15448\",\"source\":{\"name\":\"arXiv\",\"url\":\"https://arXiv.org/paper/2205.15448\"},\"title\":\"FeatER: An Efficient Network for Human Reconstruction via Feature Map-Based TransformER\",\"created_at\":\"2024-10-25T15:22:40.783Z\",\"updated_at\":\"2025-03-03T20:18:37.757Z\",\"categories\":[\"Computer Science\"],\"subcategories\":[\"cs.CV\",\"cs.AI\",\"cs.HC\"],\"metrics\":{\"activity_rank\":0,\"questions_count\":0,\"responses_count\":0,\"upvotes_count\":null,\"downvotes_count\":null,\"total_votes\":0,\"visits_count\":{\"last24Hours\":0,\"last7Days\":0,\"last30Days\":0,\"last90Days\":1,\"all\":3},\"weighted_visits\":{\"last24Hours\":0,\"last7Days\":0,\"last30Days\":0,\"last90Days\":0.0369478434508469,\"hot\":0},\"public_total_votes\":0,\"timeline\":[{\"date\":\"2025-04-02T02:39:25.945Z\",\"views\":1},{\"date\":\"2025-03-29T14:39:25.945Z\",\"views\":1},{\"date\":\"2025-03-26T02:39:25.945Z\",\"views\":1},{\"date\":\"2025-03-22T14:39:25.945Z\",\"views\":2},{\"date\":\"2025-03-19T02:39:25.945Z\",\"views\":0},{\"date\":\"2025-03-15T14:39:25.945Z\",\"views\":2},{\"date\":\"2025-03-12T02:39:25.945Z\",\"views\":2},{\"date\":\"2025-03-08T14:39:25.945Z\",\"views\":0},{\"date\":\"2025-03-05T02:39:25.945Z\",\"views\":1},{\"date\":\"2025-03-01T14:39:25.945Z\",\"views\":1},{\"date\":\"2025-02-26T02:39:25.945Z\",\"views\":2},{\"date\":\"2025-02-22T14:39:25.945Z\",\"views\":1},{\"date\":\"2025-02-19T02:39:25.962Z\",\"views\":0},{\"date\":\"2025-02-15T14:39:25.982Z\",\"views\":2},{\"date\":\"2025-02-12T02:39:26.005Z\",\"views\":0},{\"date\":\"2025-02-08T14:39:26.027Z\",\"views\":0},{\"date\":\"2025-02-05T02:39:26.042Z\",\"views\":0},{\"date\":\"2025-02-01T14:39:26.057Z\",\"views\":0},{\"date\":\"2025-01-29T02:39:26.082Z\",\"views\":0},{\"date\":\"2025-01-25T14:39:26.111Z\",\"views\":1},{\"date\":\"2025-01-22T02:39:26.135Z\",\"views\":1},{\"date\":\"2025-01-18T14:39:26.148Z\",\"views\":2},{\"date\":\"2025-01-15T02:39:26.164Z\",\"views\":2},{\"date\":\"2025-01-11T14:39:26.184Z\",\"views\":0},{\"date\":\"2025-01-08T02:39:26.202Z\",\"views\":3},{\"date\":\"2025-01-04T14:39:26.219Z\",\"views\":4},{\"date\":\"2025-01-01T02:39:26.243Z\",\"views\":1},{\"date\":\"2024-12-28T14:39:26.262Z\",\"views\":0},{\"date\":\"2024-12-25T02:39:26.279Z\",\"views\":0},{\"date\":\"2024-12-21T14:39:26.296Z\",\"views\":0},{\"date\":\"2024-12-18T02:39:26.319Z\",\"views\":1},{\"date\":\"2024-12-14T14:39:26.344Z\",\"views\":0},{\"date\":\"2024-12-11T02:39:26.369Z\",\"views\":1},{\"date\":\"2024-12-07T14:39:26.388Z\",\"views\":1},{\"date\":\"2024-12-04T02:39:26.404Z\",\"views\":1},{\"date\":\"2024-11-30T14:39:26.422Z\",\"views\":1},{\"date\":\"2024-11-27T02:39:26.440Z\",\"views\":1},{\"date\":\"2024-11-23T14:39:26.454Z\",\"views\":2},{\"date\":\"2024-11-20T02:39:26.478Z\",\"views\":2},{\"date\":\"2024-11-16T14:39:26.497Z\",\"views\":0},{\"date\":\"2024-11-13T02:39:26.514Z\",\"views\":0},{\"date\":\"2024-11-09T14:39:26.533Z\",\"views\":2},{\"date\":\"2024-11-06T02:39:26.551Z\",\"views\":0},{\"date\":\"2024-11-02T13:39:26.567Z\",\"views\":0},{\"date\":\"2024-10-30T01:39:26.593Z\",\"views\":2},{\"date\":\"2024-10-26T13:39:26.610Z\",\"views\":0},{\"date\":\"2024-10-23T01:39:26.627Z\",\"views\":5},{\"date\":\"2024-10-19T13:39:26.647Z\",\"views\":1},{\"date\":\"2024-10-16T01:39:26.662Z\",\"views\":2},{\"date\":\"2024-10-12T13:39:26.680Z\",\"views\":0},{\"date\":\"2024-10-09T01:39:26.697Z\",\"views\":0},{\"date\":\"2024-10-05T13:39:26.715Z\",\"views\":2},{\"date\":\"2024-10-02T01:39:26.731Z\",\"views\":1},{\"date\":\"2024-09-28T13:39:26.752Z\",\"views\":1},{\"date\":\"2024-09-25T01:39:26.769Z\",\"views\":2},{\"date\":\"2024-09-21T13:39:26.789Z\",\"views\":0},{\"date\":\"2024-09-18T01:39:26.804Z\",\"views\":2},{\"date\":\"2024-09-14T13:39:26.819Z\",\"views\":2},{\"date\":\"2024-09-11T01:39:26.831Z\",\"views\":0},{\"date\":\"2024-09-07T13:39:26.845Z\",\"views\":2},{\"date\":\"2024-09-04T01:39:26.861Z\",\"views\":2},{\"date\":\"2024-08-31T13:39:26.879Z\",\"views\":1},{\"date\":\"2024-08-28T01:39:26.893Z\",\"views\":0}]},\"ranking\":{\"current_rank\":104147,\"previous_rank\":103796,\"activity_score\":0,\"paper_score\":0},\"is_hidden\":false,\"custom_categories\":[\"computer-vision-security\",\"attention-mechanisms\",\"efficient-transformers\",\"model-compression\",\"parameter-efficient-training\"],\"first_publication_date\":\"2023-03-23T15:48:05.000Z\",\"author_user_ids\":[],\"paperVersions\":{\"_id\":\"673cf1ba615941b897fb57f3\",\"paper_group_id\":\"673cf1ba615941b897fb57f1\",\"version_label\":\"v3\",\"version_order\":3,\"title\":\"FeatER: An Efficient Network for Human Reconstruction via Feature Map-Based TransformER\",\"abstract\":\"$3b\",\"author_ids\":[\"672bbd2c986a1370676d5213\",\"6733e2c829b032f3570982c1\",\"672bcb43986a1370676da1c5\",\"67322590cd1e32a6e7f004e9\",\"672bbf78986a1370676d5ecc\"],\"publication_date\":\"2023-03-23T15:48:05.000Z\",\"license\":\"http://arxiv.org/licenses/nonexclusive-distrib/1.0/\",\"created_at\":\"2024-11-19T20:14:50.373Z\",\"updated_at\":\"2024-11-19T20:14:50.373Z\",\"is_deleted\":false,\"is_hidden\":false,\"universal_paper_id\":\"2205.15448\",\"imageURL\":\"image/2205.15448v3.png\"},\"verifiedAuthors\":[],\"authors\":[{\"_id\":\"672bbd2c986a1370676d5213\",\"full_name\":\"Ce Zheng\",\"affiliation\":null,\"orcid\":null,\"semantic_scholarid\":null,\"user_id\":null},{\"_id\":\"672bbf78986a1370676d5ecc\",\"full_name\":\"Chen Chen\",\"affiliation\":null,\"orcid\":null,\"semantic_scholarid\":null,\"user_id\":null},{\"_id\":\"672bcb43986a1370676da1c5\",\"full_name\":\"Taojiannan Yang\",\"affiliation\":null,\"orcid\":null,\"semantic_scholarid\":null,\"user_id\":null},{\"_id\":\"67322590cd1e32a6e7f004e9\",\"full_name\":\"Guo-Jun Qi\",\"affiliation\":null,\"orcid\":null,\"semantic_scholarid\":null,\"user_id\":null},{\"_id\":\"6733e2c829b032f3570982c1\",\"full_name\":\"Matias Mendieta\",\"affiliation\":null,\"orcid\":null,\"semantic_scholarid\":null,\"user_id\":null}]},\"max_version_order\":3,\"verified_authors\":[],\"authors\":[{\"_id\":\"672bbd2c986a1370676d5213\",\"full_name\":\"Ce Zheng\",\"affiliation\":null,\"orcid\":null,\"semantic_scholarid\":null,\"user_id\":null},{\"_id\":\"672bbf78986a1370676d5ecc\",\"full_name\":\"Chen Chen\",\"affiliation\":null,\"orcid\":null,\"semantic_scholarid\":null,\"user_id\":null},{\"_id\":\"672bcb43986a1370676da1c5\",\"full_name\":\"Taojiannan Yang\",\"affiliation\":null,\"orcid\":null,\"semantic_scholarid\":null,\"user_id\":null},{\"_id\":\"67322590cd1e32a6e7f004e9\",\"full_name\":\"Guo-Jun Qi\",\"affiliation\":null,\"orcid\":null,\"semantic_scholarid\":null,\"user_id\":null},{\"_id\":\"6733e2c829b032f3570982c1\",\"full_name\":\"Matias Mendieta\",\"affiliation\":null,\"orcid\":null,\"semantic_scholarid\":null,\"user_id\":null}],\"pdf_info\":{\"fetcher_url\":\"https://fetcher.alphaxiv.org/v2/pdf/2205.15448v3\"}}},\"dataUpdateCount\":1,\"dataUpdatedAt\":1744228185784,\"error\":null,\"errorUpdateCount\":0,\"errorUpdatedAt\":0,\"fetchFailureCount\":0,\"fetchFailureReason\":null,\"fetchMeta\":null,\"isInvalidated\":false,\"status\":\"success\",\"fetchStatus\":\"idle\"},\"queryKey\":[\"paper\",\"2205.15448\",\"metadata\"],\"queryHash\":\"[\\\"paper\\\",\\\"2205.15448\\\",\\\"metadata\\\"]\"},{\"state\":{\"data\":{\"data\":[]},\"dataUpdateCount\":1,\"dataUpdatedAt\":1744228185784,\"error\":null,\"errorUpdateCount\":0,\"errorUpdatedAt\":0,\"fetchFailureCount\":0,\"fetchFailureReason\":null,\"fetchMeta\":null,\"isInvalidated\":false,\"status\":\"success\",\"fetchStatus\":\"idle\"},\"queryKey\":[\"paper\",\"2205.15448\",\"comments\"],\"queryHash\":\"[\\\"paper\\\",\\\"2205.15448\\\",\\\"comments\\\"]\"},{\"state\":{\"data\":{\"data\":{\"paper_version\":{\"_id\":\"673d03d9615941b897fbaa70\",\"paper_group_id\":\"673d03d8615941b897fbaa6a\",\"version_label\":\"v1\",\"version_order\":1,\"title\":\"Weakly Supervised Joint Whole-Slide Segmentation and Classification in Prostate Cancer\",\"abstract\":\"$3c\",\"author_ids\":[\"67322213cd1e32a6e7efcc94\",\"672bc96d986a1370676d8854\",\"673d03d8615941b897fbaa6d\",\"67322213cd1e32a6e7efcc8b\",\"673b8b89ee7cdcdc03b1767a\",\"673cc2eb8a52218f8bc94d6d\",\"67322214cd1e32a6e7efcc9c\"],\"publication_date\":\"2023-01-07T20:38:36.000Z\",\"license\":\"http://creativecommons.org/licenses/by-nc-nd/4.0/\",\"created_at\":\"2024-11-19T21:32:09.562Z\",\"updated_at\":\"2024-11-19T21:32:09.562Z\",\"is_deleted\":false,\"is_hidden\":false,\"universal_paper_id\":\"2301.02933\",\"imageURL\":\"image/2301.02933v1.png\"},\"paper_group\":{\"_id\":\"673d03d8615941b897fbaa6a\",\"universal_paper_id\":\"2301.02933\",\"source\":{\"name\":\"arXiv\",\"url\":\"https://arXiv.org/paper/2301.02933\"},\"title\":\"Weakly Supervised Joint Whole-Slide Segmentation and Classification in Prostate Cancer\",\"created_at\":\"2024-10-30T07:20:20.406Z\",\"updated_at\":\"2025-03-03T20:22:24.451Z\",\"categories\":[\"Electrical Engineering and Systems Science\",\"Computer Science\"],\"subcategories\":[\"eess.IV\",\"cs.CV\"],\"metrics\":{\"activity_rank\":0,\"questions_count\":0,\"responses_count\":0,\"upvotes_count\":null,\"downvotes_count\":null,\"total_votes\":0,\"visits_count\":{\"last24Hours\":0,\"last7Days\":0,\"last30Days\":4,\"last90Days\":4,\"all\":18},\"weighted_visits\":{\"last24Hours\":0,\"last7Days\":0,\"last30Days\":0.00007441460148596415,\"last90Days\":0.10598865500318737,\"hot\":0},\"public_total_votes\":0,\"timeline\":[{\"date\":\"2025-04-02T02:45:30.838Z\",\"views\":0},{\"date\":\"2025-03-29T14:45:30.838Z\",\"views\":5},{\"date\":\"2025-03-26T02:45:30.838Z\",\"views\":0},{\"date\":\"2025-03-22T14:45:30.838Z\",\"views\":10},{\"date\":\"2025-03-19T02:45:30.838Z\",\"views\":0},{\"date\":\"2025-03-15T14:45:30.838Z\",\"views\":0},{\"date\":\"2025-03-12T02:45:30.838Z\",\"views\":2},{\"date\":\"2025-03-08T14:45:30.838Z\",\"views\":2},{\"date\":\"2025-03-05T02:45:30.838Z\",\"views\":0},{\"date\":\"2025-03-01T14:45:30.838Z\",\"views\":1},{\"date\":\"2025-02-26T02:45:30.838Z\",\"views\":1},{\"date\":\"2025-02-22T14:45:30.838Z\",\"views\":2},{\"date\":\"2025-02-19T02:45:30.852Z\",\"views\":0},{\"date\":\"2025-02-15T14:45:30.867Z\",\"views\":0},{\"date\":\"2025-02-12T02:45:30.884Z\",\"views\":0},{\"date\":\"2025-02-08T14:45:30.900Z\",\"views\":0},{\"date\":\"2025-02-05T02:45:30.915Z\",\"views\":2},{\"date\":\"2025-02-01T14:45:30.931Z\",\"views\":1},{\"date\":\"2025-01-29T02:45:30.948Z\",\"views\":0},{\"date\":\"2025-01-25T14:45:30.960Z\",\"views\":0},{\"date\":\"2025-01-22T02:45:30.976Z\",\"views\":0},{\"date\":\"2025-01-18T14:45:30.996Z\",\"views\":1},{\"date\":\"2025-01-15T02:45:31.012Z\",\"views\":2},{\"date\":\"2025-01-11T14:45:31.026Z\",\"views\":0},{\"date\":\"2025-01-08T02:45:31.042Z\",\"views\":0},{\"date\":\"2025-01-04T14:45:31.062Z\",\"views\":5},{\"date\":\"2025-01-01T02:45:31.080Z\",\"views\":0},{\"date\":\"2024-12-28T14:45:31.096Z\",\"views\":0},{\"date\":\"2024-12-25T02:45:31.112Z\",\"views\":2},{\"date\":\"2024-12-21T14:45:31.132Z\",\"views\":1},{\"date\":\"2024-12-18T02:45:31.149Z\",\"views\":0},{\"date\":\"2024-12-14T14:45:31.166Z\",\"views\":1},{\"date\":\"2024-12-11T02:45:31.185Z\",\"views\":1},{\"date\":\"2024-12-07T14:45:31.204Z\",\"views\":1},{\"date\":\"2024-12-04T02:45:31.217Z\",\"views\":0},{\"date\":\"2024-11-30T14:45:31.236Z\",\"views\":2},{\"date\":\"2024-11-27T02:45:31.254Z\",\"views\":0},{\"date\":\"2024-11-23T14:45:31.270Z\",\"views\":0},{\"date\":\"2024-11-20T02:45:31.285Z\",\"views\":2},{\"date\":\"2024-11-16T14:45:31.305Z\",\"views\":1},{\"date\":\"2024-11-13T02:45:31.320Z\",\"views\":0},{\"date\":\"2024-11-09T14:45:31.338Z\",\"views\":2},{\"date\":\"2024-11-06T02:45:31.355Z\",\"views\":2},{\"date\":\"2024-11-02T13:45:31.373Z\",\"views\":2},{\"date\":\"2024-10-30T01:45:31.389Z\",\"views\":3},{\"date\":\"2024-10-26T13:45:31.405Z\",\"views\":0},{\"date\":\"2024-10-23T01:45:31.423Z\",\"views\":0},{\"date\":\"2024-10-19T13:45:31.447Z\",\"views\":1},{\"date\":\"2024-10-16T01:45:31.470Z\",\"views\":1},{\"date\":\"2024-10-12T13:45:31.487Z\",\"views\":0},{\"date\":\"2024-10-09T01:45:31.509Z\",\"views\":1},{\"date\":\"2024-10-05T13:45:31.525Z\",\"views\":2},{\"date\":\"2024-10-02T01:45:31.540Z\",\"views\":0},{\"date\":\"2024-09-28T13:45:31.565Z\",\"views\":0},{\"date\":\"2024-09-25T01:45:31.582Z\",\"views\":1},{\"date\":\"2024-09-21T13:45:31.619Z\",\"views\":2},{\"date\":\"2024-09-18T01:45:31.635Z\",\"views\":1},{\"date\":\"2024-09-14T13:45:31.652Z\",\"views\":0},{\"date\":\"2024-09-11T01:45:31.669Z\",\"views\":2},{\"date\":\"2024-09-07T13:45:31.684Z\",\"views\":0},{\"date\":\"2024-09-04T01:45:31.698Z\",\"views\":1},{\"date\":\"2024-08-31T13:45:31.719Z\",\"views\":2},{\"date\":\"2024-08-28T01:45:31.730Z\",\"views\":0}]},\"ranking\":{\"current_rank\":114301,\"previous_rank\":113945,\"activity_score\":0,\"paper_score\":0},\"is_hidden\":false,\"custom_categories\":[\"weak-supervision\",\"image-segmentation\",\"ai-for-health\",\"uncertainty-estimation\",\"graph-neural-networks\"],\"first_publication_date\":\"2023-01-07T20:38:36.000Z\",\"author_user_ids\":[],\"organizations\":[\"67be63dfaa92218ccd8b26c4\",\"67be63bbaa92218ccd8b2089\",\"67be637aaa92218ccd8b1142\",\"67be64a2aa92218ccd8b3efb\",\"67c39b706238d4c4ef2141e7\",\"67be6377aa92218ccd8b1014\",\"67be637baa92218ccd8b116d\",\"67be637aaa92218ccd8b1167\"],\"paperVersions\":{\"_id\":\"673d03d9615941b897fbaa70\",\"paper_group_id\":\"673d03d8615941b897fbaa6a\",\"version_label\":\"v1\",\"version_order\":1,\"title\":\"Weakly Supervised Joint Whole-Slide Segmentation and Classification in Prostate Cancer\",\"abstract\":\"$3d\",\"author_ids\":[\"67322213cd1e32a6e7efcc94\",\"672bc96d986a1370676d8854\",\"673d03d8615941b897fbaa6d\",\"67322213cd1e32a6e7efcc8b\",\"673b8b89ee7cdcdc03b1767a\",\"673cc2eb8a52218f8bc94d6d\",\"67322214cd1e32a6e7efcc9c\"],\"publication_date\":\"2023-01-07T20:38:36.000Z\",\"license\":\"http://creativecommons.org/licenses/by-nc-nd/4.0/\",\"created_at\":\"2024-11-19T21:32:09.562Z\",\"updated_at\":\"2024-11-19T21:32:09.562Z\",\"is_deleted\":false,\"is_hidden\":false,\"universal_paper_id\":\"2301.02933\",\"imageURL\":\"image/2301.02933v1.png\"},\"verifiedAuthors\":[],\"authors\":[{\"_id\":\"672bc96d986a1370676d8854\",\"full_name\":\"Guillaume Jaume\",\"affiliation\":null,\"orcid\":null,\"semantic_scholarid\":null,\"user_id\":null},{\"_id\":\"67322213cd1e32a6e7efcc8b\",\"full_name\":\"Kevin Thandiackal\",\"affiliation\":null,\"orcid\":null,\"semantic_scholarid\":null,\"user_id\":null},{\"_id\":\"67322213cd1e32a6e7efcc94\",\"full_name\":\"Pushpak Pati\",\"affiliation\":null,\"orcid\":null,\"semantic_scholarid\":null,\"user_id\":null},{\"_id\":\"67322214cd1e32a6e7efcc9c\",\"full_name\":\"Orcun Goksel\",\"affiliation\":null,\"orcid\":null,\"semantic_scholarid\":null,\"user_id\":null},{\"_id\":\"673b8b89ee7cdcdc03b1767a\",\"full_name\":\"Behzad Bozorgtabar\",\"affiliation\":null,\"orcid\":null,\"semantic_scholarid\":null,\"user_id\":null},{\"_id\":\"673cc2eb8a52218f8bc94d6d\",\"full_name\":\"Maria Gabrani\",\"affiliation\":null,\"orcid\":null,\"semantic_scholarid\":null,\"user_id\":null},{\"_id\":\"673d03d8615941b897fbaa6d\",\"full_name\":\"Zeineb Ayadi\",\"affiliation\":null,\"orcid\":null,\"semantic_scholarid\":null,\"user_id\":null}]},\"max_version_order\":1,\"verified_authors\":[],\"authors\":[{\"_id\":\"672bc96d986a1370676d8854\",\"full_name\":\"Guillaume Jaume\",\"affiliation\":null,\"orcid\":null,\"semantic_scholarid\":null,\"user_id\":null},{\"_id\":\"67322213cd1e32a6e7efcc8b\",\"full_name\":\"Kevin Thandiackal\",\"affiliation\":null,\"orcid\":null,\"semantic_scholarid\":null,\"user_id\":null},{\"_id\":\"67322213cd1e32a6e7efcc94\",\"full_name\":\"Pushpak Pati\",\"affiliation\":null,\"orcid\":null,\"semantic_scholarid\":null,\"user_id\":null},{\"_id\":\"67322214cd1e32a6e7efcc9c\",\"full_name\":\"Orcun Goksel\",\"affiliation\":null,\"orcid\":null,\"semantic_scholarid\":null,\"user_id\":null},{\"_id\":\"673b8b89ee7cdcdc03b1767a\",\"full_name\":\"Behzad Bozorgtabar\",\"affiliation\":null,\"orcid\":null,\"semantic_scholarid\":null,\"user_id\":null},{\"_id\":\"673cc2eb8a52218f8bc94d6d\",\"full_name\":\"Maria Gabrani\",\"affiliation\":null,\"orcid\":null,\"semantic_scholarid\":null,\"user_id\":null},{\"_id\":\"673d03d8615941b897fbaa6d\",\"full_name\":\"Zeineb Ayadi\",\"affiliation\":null,\"orcid\":null,\"semantic_scholarid\":null,\"user_id\":null}],\"pdf_info\":{\"fetcher_url\":\"https://fetcher.alphaxiv.org/v2/pdf/2301.02933v1\"}}},\"dataUpdateCount\":1,\"dataUpdatedAt\":1744228185948,\"error\":null,\"errorUpdateCount\":0,\"errorUpdatedAt\":0,\"fetchFailureCount\":0,\"fetchFailureReason\":null,\"fetchMeta\":null,\"isInvalidated\":false,\"status\":\"success\",\"fetchStatus\":\"idle\"},\"queryKey\":[\"paper\",\"2301.02933\",\"metadata\"],\"queryHash\":\"[\\\"paper\\\",\\\"2301.02933\\\",\\\"metadata\\\"]\"},{\"state\":{\"data\":{\"data\":[]},\"dataUpdateCount\":1,\"dataUpdatedAt\":1744228185948,\"error\":null,\"errorUpdateCount\":0,\"errorUpdatedAt\":0,\"fetchFailureCount\":0,\"fetchFailureReason\":null,\"fetchMeta\":null,\"isInvalidated\":false,\"status\":\"success\",\"fetchStatus\":\"idle\"},\"queryKey\":[\"paper\",\"2301.02933\",\"comments\"],\"queryHash\":\"[\\\"paper\\\",\\\"2301.02933\\\",\\\"comments\\\"]\"},{\"state\":{\"data\":{\"data\":{\"paper_version\":{\"_id\":\"67631a318455f19bd6e50d7e\",\"paper_group_id\":\"67631a308455f19bd6e50d7b\",\"version_label\":\"v3\",\"version_order\":3,\"title\":\"Learning Low Dimensional State Spaces with Overparameterized Recurrent Neural Nets\",\"abstract\":\"$3e\",\"author_ids\":[\"672bd6d7e78ce066acf2e212\",\"67631a318455f19bd6e50d7d\",\"672bcf69986a1370676deb9c\",\"67341aee29b032f35709ae79\",\"672bbda0986a1370676d53e2\"],\"publication_date\":\"2023-03-23T15:45:41.000Z\",\"license\":\"http://creativecommons.org/licenses/by/4.0/\",\"created_at\":\"2024-12-18T18:53:37.508Z\",\"updated_at\":\"2024-12-18T18:53:37.508Z\",\"is_deleted\":false,\"is_hidden\":false,\"universal_paper_id\":\"2210.14064\",\"imageURL\":\"image/2210.14064v3.png\"},\"paper_group\":{\"_id\":\"67631a308455f19bd6e50d7b\",\"universal_paper_id\":\"2210.14064\",\"title\":\"Learning Low Dimensional State Spaces with Overparameterized Recurrent Neural Nets\",\"created_at\":\"2024-12-18T18:53:36.929Z\",\"updated_at\":\"2025-03-03T20:18:37.758Z\",\"categories\":[\"Computer Science\"],\"subcategories\":[\"cs.LG\"],\"custom_categories\":[\"sequence-modeling\",\"representation-learning\",\"optimization-methods\",\"statistical-learning\",\"neural-coding\"],\"author_user_ids\":[],\"source\":{\"name\":\"alphaXiv\",\"url\":\"https://arxiv.org/paper/2210.14064\"},\"metrics\":{\"activity_rank\":0,\"questions_count\":0,\"responses_count\":0,\"upvotes_count\":0,\"downvotes_count\":0,\"total_votes\":0,\"visits_count\":{\"last24Hours\":1,\"last7Days\":1,\"last30Days\":5,\"last90Days\":7,\"all\":25},\"weighted_visits\":{\"last24Hours\":1.2098437548934945e-129,\"last7Days\":3.8304256858671152e-19,\"last30Days\":0.0002521898754902932,\"last90Days\":0.258632990211502,\"hot\":3.8304256858671152e-19},\"public_total_votes\":2,\"timeline\":[{\"date\":\"2025-04-02T02:39:25.955Z\",\"views\":0},{\"date\":\"2025-03-29T14:39:25.955Z\",\"views\":12},{\"date\":\"2025-03-26T02:39:25.955Z\",\"views\":2},{\"date\":\"2025-03-22T14:39:25.955Z\",\"views\":0},{\"date\":\"2025-03-19T02:39:25.955Z\",\"views\":2},{\"date\":\"2025-03-15T14:39:25.955Z\",\"views\":2},{\"date\":\"2025-03-12T02:39:25.955Z\",\"views\":2},{\"date\":\"2025-03-08T14:39:25.955Z\",\"views\":0},{\"date\":\"2025-03-05T02:39:25.955Z\",\"views\":1},{\"date\":\"2025-03-01T14:39:25.955Z\",\"views\":2},{\"date\":\"2025-02-26T02:39:25.955Z\",\"views\":2},{\"date\":\"2025-02-22T14:39:25.955Z\",\"views\":5},{\"date\":\"2025-02-19T02:39:25.973Z\",\"views\":2},{\"date\":\"2025-02-15T14:39:25.990Z\",\"views\":0},{\"date\":\"2025-02-12T02:39:26.007Z\",\"views\":2},{\"date\":\"2025-02-08T14:39:26.027Z\",\"views\":1},{\"date\":\"2025-02-05T02:39:26.044Z\",\"views\":1},{\"date\":\"2025-02-01T14:39:26.063Z\",\"views\":5},{\"date\":\"2025-01-29T02:39:26.082Z\",\"views\":1},{\"date\":\"2025-01-25T14:39:26.105Z\",\"views\":2},{\"date\":\"2025-01-22T02:39:26.128Z\",\"views\":2},{\"date\":\"2025-01-18T14:39:26.147Z\",\"views\":1},{\"date\":\"2025-01-15T02:39:26.167Z\",\"views\":0},{\"date\":\"2025-01-11T14:39:26.186Z\",\"views\":2},{\"date\":\"2025-01-08T02:39:26.205Z\",\"views\":2},{\"date\":\"2025-01-04T14:39:26.223Z\",\"views\":2},{\"date\":\"2025-01-01T02:39:26.242Z\",\"views\":2},{\"date\":\"2024-12-28T14:39:26.262Z\",\"views\":1},{\"date\":\"2024-12-25T02:39:26.277Z\",\"views\":2},{\"date\":\"2024-12-21T14:39:26.295Z\",\"views\":2},{\"date\":\"2024-12-18T02:39:26.311Z\",\"views\":5},{\"date\":\"2024-12-14T14:39:26.334Z\",\"views\":2},{\"date\":\"2024-12-11T02:39:26.351Z\",\"views\":1},{\"date\":\"2024-12-07T14:39:26.374Z\",\"views\":0},{\"date\":\"2024-12-04T02:39:26.390Z\",\"views\":2},{\"date\":\"2024-11-30T14:39:26.408Z\",\"views\":2},{\"date\":\"2024-11-27T02:39:26.425Z\",\"views\":0},{\"date\":\"2024-11-23T14:39:26.446Z\",\"views\":2},{\"date\":\"2024-11-20T02:39:26.466Z\",\"views\":1},{\"date\":\"2024-11-16T14:39:26.483Z\",\"views\":2},{\"date\":\"2024-11-13T02:39:26.500Z\",\"views\":1},{\"date\":\"2024-11-09T14:39:26.519Z\",\"views\":1},{\"date\":\"2024-11-06T02:39:26.538Z\",\"views\":0},{\"date\":\"2024-11-02T13:39:26.559Z\",\"views\":0},{\"date\":\"2024-10-30T01:39:26.585Z\",\"views\":1},{\"date\":\"2024-10-26T13:39:26.601Z\",\"views\":1},{\"date\":\"2024-10-23T01:39:26.618Z\",\"views\":2},{\"date\":\"2024-10-19T13:39:26.633Z\",\"views\":2},{\"date\":\"2024-10-16T01:39:26.651Z\",\"views\":2},{\"date\":\"2024-10-12T13:39:26.670Z\",\"views\":1},{\"date\":\"2024-10-09T01:39:26.688Z\",\"views\":1},{\"date\":\"2024-10-05T13:39:26.705Z\",\"views\":1},{\"date\":\"2024-10-02T01:39:26.722Z\",\"views\":2},{\"date\":\"2024-09-28T13:39:26.740Z\",\"views\":1},{\"date\":\"2024-09-25T01:39:26.758Z\",\"views\":1},{\"date\":\"2024-09-21T13:39:26.776Z\",\"views\":0},{\"date\":\"2024-09-18T01:39:26.793Z\",\"views\":0},{\"date\":\"2024-09-14T13:39:26.808Z\",\"views\":1},{\"date\":\"2024-09-11T01:39:26.823Z\",\"views\":2},{\"date\":\"2024-09-07T13:39:26.835Z\",\"views\":2},{\"date\":\"2024-09-04T01:39:26.851Z\",\"views\":0},{\"date\":\"2024-08-31T13:39:26.885Z\",\"views\":0},{\"date\":\"2024-08-28T01:39:26.896Z\",\"views\":1}]},\"is_hidden\":false,\"first_publication_date\":\"2023-03-23T15:45:41.000Z\",\"paperVersions\":{\"_id\":\"67631a318455f19bd6e50d7e\",\"paper_group_id\":\"67631a308455f19bd6e50d7b\",\"version_label\":\"v3\",\"version_order\":3,\"title\":\"Learning Low Dimensional State Spaces with Overparameterized Recurrent Neural Nets\",\"abstract\":\"$3f\",\"author_ids\":[\"672bd6d7e78ce066acf2e212\",\"67631a318455f19bd6e50d7d\",\"672bcf69986a1370676deb9c\",\"67341aee29b032f35709ae79\",\"672bbda0986a1370676d53e2\"],\"publication_date\":\"2023-03-23T15:45:41.000Z\",\"license\":\"http://creativecommons.org/licenses/by/4.0/\",\"created_at\":\"2024-12-18T18:53:37.508Z\",\"updated_at\":\"2024-12-18T18:53:37.508Z\",\"is_deleted\":false,\"is_hidden\":false,\"universal_paper_id\":\"2210.14064\",\"imageURL\":\"image/2210.14064v3.png\"},\"verifiedAuthors\":[],\"authors\":[{\"_id\":\"672bbda0986a1370676d53e2\",\"full_name\":\"Amir Globerson\",\"affiliation\":null,\"orcid\":null,\"semantic_scholarid\":null,\"user_id\":null},{\"_id\":\"672bcf69986a1370676deb9c\",\"full_name\":\"Raja Giryes\",\"affiliation\":null,\"orcid\":null,\"semantic_scholarid\":null,\"user_id\":null},{\"_id\":\"672bd6d7e78ce066acf2e212\",\"full_name\":\"Edo Cohen-Karlik\",\"affiliation\":null,\"orcid\":null,\"semantic_scholarid\":null,\"user_id\":null},{\"_id\":\"67341aee29b032f35709ae79\",\"full_name\":\"Nadav Cohen\",\"affiliation\":null,\"orcid\":null,\"semantic_scholarid\":null,\"user_id\":null},{\"_id\":\"67631a318455f19bd6e50d7d\",\"full_name\":\"Itamar Menuhin-Gruman\",\"affiliation\":null,\"orcid\":null,\"semantic_scholarid\":null,\"user_id\":null}]},\"max_version_order\":3,\"verified_authors\":[],\"authors\":[{\"_id\":\"672bbda0986a1370676d53e2\",\"full_name\":\"Amir Globerson\",\"affiliation\":null,\"orcid\":null,\"semantic_scholarid\":null,\"user_id\":null},{\"_id\":\"672bcf69986a1370676deb9c\",\"full_name\":\"Raja Giryes\",\"affiliation\":null,\"orcid\":null,\"semantic_scholarid\":null,\"user_id\":null},{\"_id\":\"672bd6d7e78ce066acf2e212\",\"full_name\":\"Edo Cohen-Karlik\",\"affiliation\":null,\"orcid\":null,\"semantic_scholarid\":null,\"user_id\":null},{\"_id\":\"67341aee29b032f35709ae79\",\"full_name\":\"Nadav Cohen\",\"affiliation\":null,\"orcid\":null,\"semantic_scholarid\":null,\"user_id\":null},{\"_id\":\"67631a318455f19bd6e50d7d\",\"full_name\":\"Itamar Menuhin-Gruman\",\"affiliation\":null,\"orcid\":null,\"semantic_scholarid\":null,\"user_id\":null}],\"pdf_info\":{\"fetcher_url\":\"https://fetcher.alphaxiv.org/v2/pdf/2210.14064v3\"}}},\"dataUpdateCount\":1,\"dataUpdatedAt\":1744228185991,\"error\":null,\"errorUpdateCount\":0,\"errorUpdatedAt\":0,\"fetchFailureCount\":0,\"fetchFailureReason\":null,\"fetchMeta\":null,\"isInvalidated\":false,\"status\":\"success\",\"fetchStatus\":\"idle\"},\"queryKey\":[\"paper\",\"2210.14064\",\"metadata\"],\"queryHash\":\"[\\\"paper\\\",\\\"2210.14064\\\",\\\"metadata\\\"]\"},{\"state\":{\"data\":{\"data\":[]},\"dataUpdateCount\":1,\"dataUpdatedAt\":1744228185991,\"error\":null,\"errorUpdateCount\":0,\"errorUpdatedAt\":0,\"fetchFailureCount\":0,\"fetchFailureReason\":null,\"fetchMeta\":null,\"isInvalidated\":false,\"status\":\"success\",\"fetchStatus\":\"idle\"},\"queryKey\":[\"paper\",\"2210.14064\",\"comments\"],\"queryHash\":\"[\\\"paper\\\",\\\"2210.14064\\\",\\\"comments\\\"]\"},{\"state\":{\"data\":{\"data\":{\"paper_version\":{\"_id\":\"673d1c99181e8ac85932f6fd\",\"paper_group_id\":\"673d1c99181e8ac85932f6fc\",\"version_label\":\"v4\",\"version_order\":4,\"title\":\"Language Models with Image Descriptors are Strong Few-Shot Video-Language Learners\",\"abstract\":\"$40\",\"author_ids\":[\"672bd0f3986a1370676e0ca9\",\"672bc9d3986a1370676d8e47\",\"67322fe0cd1e32a6e7f0ada6\",\"672bc06d986a1370676d632a\",\"672bcd9d986a1370676dcaba\",\"672bcdd6986a1370676dce68\",\"672bcf2b986a1370676de68a\",\"672bca84986a1370676d9696\",\"672bca85986a1370676d969f\",\"672bcefd986a1370676de2d5\",\"672bc5d0986a1370676d680d\",\"672bbf32986a1370676d5aee\",\"672bc943986a1370676d861c\"],\"publication_date\":\"2022-10-13T06:32:37.000Z\",\"license\":\"http://arxiv.org/licenses/nonexclusive-distrib/1.0/\",\"created_at\":\"2024-11-19T23:17:45.700Z\",\"updated_at\":\"2024-11-19T23:17:45.700Z\",\"is_deleted\":false,\"is_hidden\":false,\"universal_paper_id\":\"2205.10747\",\"imageURL\":\"image/2205.10747v4.png\"},\"paper_group\":{\"_id\":\"673d1c99181e8ac85932f6fc\",\"universal_paper_id\":\"2205.10747\",\"source\":{\"name\":\"arXiv\",\"url\":\"https://arXiv.org/paper/2205.10747\"},\"title\":\"Language Models with Image Descriptors are Strong Few-Shot Video-Language Learners\",\"created_at\":\"2024-11-04T13:00:05.089Z\",\"updated_at\":\"2025-03-03T20:26:06.664Z\",\"categories\":[\"Computer Science\"],\"subcategories\":[\"cs.CV\",\"cs.AI\"],\"metrics\":{\"activity_rank\":0,\"questions_count\":0,\"responses_count\":0,\"upvotes_count\":null,\"downvotes_count\":null,\"total_votes\":0,\"visits_count\":{\"last24Hours\":0,\"last7Days\":0,\"last30Days\":3,\"last90Days\":6,\"all\":21},\"weighted_visits\":{\"last24Hours\":0,\"last7Days\":0,\"last30Days\":0.000017590859117343075,\"last90Days\":0.10819483168188468,\"hot\":0},\"public_total_votes\":0,\"timeline\":[{\"date\":\"2025-04-03T02:17:28.245Z\",\"views\":1},{\"date\":\"2025-03-30T14:17:28.245Z\",\"views\":1},{\"date\":\"2025-03-27T02:17:28.245Z\",\"views\":3},{\"date\":\"2025-03-23T14:17:28.245Z\",\"views\":1},{\"date\":\"2025-03-20T02:17:28.245Z\",\"views\":3},{\"date\":\"2025-03-16T14:17:28.245Z\",\"views\":2},{\"date\":\"2025-03-13T02:17:28.245Z\",\"views\":1},{\"date\":\"2025-03-09T14:17:28.245Z\",\"views\":4},{\"date\":\"2025-03-06T02:17:28.245Z\",\"views\":0},{\"date\":\"2025-03-02T14:17:28.245Z\",\"views\":2},{\"date\":\"2025-02-27T02:17:28.245Z\",\"views\":2},{\"date\":\"2025-02-23T14:17:28.245Z\",\"views\":1},{\"date\":\"2025-02-20T02:17:28.270Z\",\"views\":0},{\"date\":\"2025-02-16T14:17:28.293Z\",\"views\":0},{\"date\":\"2025-02-13T02:17:28.318Z\",\"views\":0},{\"date\":\"2025-02-09T14:17:28.339Z\",\"views\":1},{\"date\":\"2025-02-06T02:17:28.363Z\",\"views\":2},{\"date\":\"2025-02-02T14:17:28.389Z\",\"views\":1},{\"date\":\"2025-01-30T02:17:28.409Z\",\"views\":0},{\"date\":\"2025-01-26T14:17:28.432Z\",\"views\":1},{\"date\":\"2025-01-23T02:17:28.455Z\",\"views\":5},{\"date\":\"2025-01-19T14:17:28.477Z\",\"views\":2},{\"date\":\"2025-01-16T02:17:28.500Z\",\"views\":5},{\"date\":\"2025-01-12T14:17:28.580Z\",\"views\":3},{\"date\":\"2025-01-09T02:17:28.618Z\",\"views\":0},{\"date\":\"2025-01-05T14:17:28.637Z\",\"views\":0},{\"date\":\"2025-01-02T02:17:28.662Z\",\"views\":1},{\"date\":\"2024-12-29T14:17:28.682Z\",\"views\":1},{\"date\":\"2024-12-26T02:17:28.707Z\",\"views\":1},{\"date\":\"2024-12-22T14:17:28.728Z\",\"views\":1},{\"date\":\"2024-12-19T02:17:28.753Z\",\"views\":1},{\"date\":\"2024-12-15T14:17:28.775Z\",\"views\":1},{\"date\":\"2024-12-12T02:17:28.799Z\",\"views\":1},{\"date\":\"2024-12-08T14:17:28.833Z\",\"views\":2},{\"date\":\"2024-12-05T02:17:28.856Z\",\"views\":0},{\"date\":\"2024-12-01T14:17:28.876Z\",\"views\":1},{\"date\":\"2024-11-28T02:17:28.902Z\",\"views\":0},{\"date\":\"2024-11-24T14:17:28.926Z\",\"views\":1},{\"date\":\"2024-11-21T02:17:28.946Z\",\"views\":1},{\"date\":\"2024-11-17T14:17:28.970Z\",\"views\":2},{\"date\":\"2024-11-14T02:17:28.996Z\",\"views\":2},{\"date\":\"2024-11-10T14:17:29.016Z\",\"views\":2},{\"date\":\"2024-11-07T02:17:29.038Z\",\"views\":0},{\"date\":\"2024-11-03T14:17:29.070Z\",\"views\":4},{\"date\":\"2024-10-31T01:17:29.092Z\",\"views\":0},{\"date\":\"2024-10-27T13:17:29.118Z\",\"views\":2},{\"date\":\"2024-10-24T01:17:29.141Z\",\"views\":0},{\"date\":\"2024-10-20T13:17:29.170Z\",\"views\":2},{\"date\":\"2024-10-17T01:17:29.196Z\",\"views\":1},{\"date\":\"2024-10-13T13:17:29.225Z\",\"views\":2},{\"date\":\"2024-10-10T01:17:29.250Z\",\"views\":0},{\"date\":\"2024-10-06T13:17:29.271Z\",\"views\":0},{\"date\":\"2024-10-03T01:17:29.291Z\",\"views\":0},{\"date\":\"2024-09-29T13:17:29.314Z\",\"views\":1},{\"date\":\"2024-09-26T01:17:29.336Z\",\"views\":2},{\"date\":\"2024-09-22T13:17:29.358Z\",\"views\":0},{\"date\":\"2024-09-19T01:17:29.378Z\",\"views\":0},{\"date\":\"2024-09-15T13:17:29.399Z\",\"views\":1},{\"date\":\"2024-09-12T01:17:29.426Z\",\"views\":1},{\"date\":\"2024-09-08T13:17:29.445Z\",\"views\":0},{\"date\":\"2024-09-05T01:17:29.470Z\",\"views\":0},{\"date\":\"2024-09-01T13:17:29.490Z\",\"views\":2},{\"date\":\"2024-08-29T01:17:29.521Z\",\"views\":2}]},\"ranking\":{\"current_rank\":126109,\"previous_rank\":125724,\"activity_score\":0,\"paper_score\":0},\"is_hidden\":false,\"custom_categories\":[\"few-shot-learning\",\"video-understanding\",\"vision-language-models\",\"multi-modal-learning\",\"text-generation\"],\"first_publication_date\":\"2022-10-13T06:32:37.000Z\",\"author_user_ids\":[],\"resources\":{\"github\":{\"url\":\"https://github.com/MikeWangWZHL/VidIL\",\"description\":\"Pytorch code for Language Models with Image Descriptors are Strong Few-Shot Video-Language Learners\",\"language\":\"Python\",\"stars\":113}},\"paperVersions\":{\"_id\":\"673d1c99181e8ac85932f6fd\",\"paper_group_id\":\"673d1c99181e8ac85932f6fc\",\"version_label\":\"v4\",\"version_order\":4,\"title\":\"Language Models with Image Descriptors are Strong Few-Shot Video-Language Learners\",\"abstract\":\"$41\",\"author_ids\":[\"672bd0f3986a1370676e0ca9\",\"672bc9d3986a1370676d8e47\",\"67322fe0cd1e32a6e7f0ada6\",\"672bc06d986a1370676d632a\",\"672bcd9d986a1370676dcaba\",\"672bcdd6986a1370676dce68\",\"672bcf2b986a1370676de68a\",\"672bca84986a1370676d9696\",\"672bca85986a1370676d969f\",\"672bcefd986a1370676de2d5\",\"672bc5d0986a1370676d680d\",\"672bbf32986a1370676d5aee\",\"672bc943986a1370676d861c\"],\"publication_date\":\"2022-10-13T06:32:37.000Z\",\"license\":\"http://arxiv.org/licenses/nonexclusive-distrib/1.0/\",\"created_at\":\"2024-11-19T23:17:45.700Z\",\"updated_at\":\"2024-11-19T23:17:45.700Z\",\"is_deleted\":false,\"is_hidden\":false,\"universal_paper_id\":\"2205.10747\",\"imageURL\":\"image/2205.10747v4.png\"},\"verifiedAuthors\":[],\"authors\":[{\"_id\":\"672bbf32986a1370676d5aee\",\"full_name\":\"Mohit Bansal\",\"affiliation\":null,\"orcid\":null,\"semantic_scholarid\":null,\"user_id\":null},{\"_id\":\"672bc06d986a1370676d632a\",\"full_name\":\"Luowei Zhou\",\"affiliation\":null,\"orcid\":null,\"semantic_scholarid\":null,\"user_id\":null},{\"_id\":\"672bc5d0986a1370676d680d\",\"full_name\":\"Shih-Fu Chang\",\"affiliation\":null,\"orcid\":null,\"semantic_scholarid\":null,\"user_id\":null},{\"_id\":\"672bc943986a1370676d861c\",\"full_name\":\"Heng Ji\",\"affiliation\":null,\"orcid\":null,\"semantic_scholarid\":null,\"user_id\":null},{\"_id\":\"672bc9d3986a1370676d8e47\",\"full_name\":\"Manling Li\",\"affiliation\":null,\"orcid\":null,\"semantic_scholarid\":null,\"user_id\":null},{\"_id\":\"672bca84986a1370676d9696\",\"full_name\":\"Ziyi Yang\",\"affiliation\":null,\"orcid\":null,\"semantic_scholarid\":null,\"user_id\":null},{\"_id\":\"672bca85986a1370676d969f\",\"full_name\":\"Chenguang Zhu\",\"affiliation\":null,\"orcid\":null,\"semantic_scholarid\":null,\"user_id\":null},{\"_id\":\"672bcd9d986a1370676dcaba\",\"full_name\":\"Jie Lei\",\"affiliation\":null,\"orcid\":null,\"semantic_scholarid\":null,\"user_id\":null},{\"_id\":\"672bcdd6986a1370676dce68\",\"full_name\":\"Xudong Lin\",\"affiliation\":null,\"orcid\":null,\"semantic_scholarid\":null,\"user_id\":null},{\"_id\":\"672bcefd986a1370676de2d5\",\"full_name\":\"Derek Hoiem\",\"affiliation\":null,\"orcid\":null,\"semantic_scholarid\":null,\"user_id\":null},{\"_id\":\"672bcf2b986a1370676de68a\",\"full_name\":\"Shuohang Wang\",\"affiliation\":null,\"orcid\":null,\"semantic_scholarid\":null,\"user_id\":null},{\"_id\":\"672bd0f3986a1370676e0ca9\",\"full_name\":\"Zhenhailong Wang\",\"affiliation\":null,\"orcid\":null,\"semantic_scholarid\":null,\"user_id\":null},{\"_id\":\"67322fe0cd1e32a6e7f0ada6\",\"full_name\":\"Ruochen Xu\",\"affiliation\":null,\"orcid\":null,\"semantic_scholarid\":null,\"user_id\":null}]},\"max_version_order\":4,\"verified_authors\":[],\"authors\":[{\"_id\":\"672bbf32986a1370676d5aee\",\"full_name\":\"Mohit Bansal\",\"affiliation\":null,\"orcid\":null,\"semantic_scholarid\":null,\"user_id\":null},{\"_id\":\"672bc06d986a1370676d632a\",\"full_name\":\"Luowei Zhou\",\"affiliation\":null,\"orcid\":null,\"semantic_scholarid\":null,\"user_id\":null},{\"_id\":\"672bc5d0986a1370676d680d\",\"full_name\":\"Shih-Fu Chang\",\"affiliation\":null,\"orcid\":null,\"semantic_scholarid\":null,\"user_id\":null},{\"_id\":\"672bc943986a1370676d861c\",\"full_name\":\"Heng Ji\",\"affiliation\":null,\"orcid\":null,\"semantic_scholarid\":null,\"user_id\":null},{\"_id\":\"672bc9d3986a1370676d8e47\",\"full_name\":\"Manling Li\",\"affiliation\":null,\"orcid\":null,\"semantic_scholarid\":null,\"user_id\":null},{\"_id\":\"672bca84986a1370676d9696\",\"full_name\":\"Ziyi Yang\",\"affiliation\":null,\"orcid\":null,\"semantic_scholarid\":null,\"user_id\":null},{\"_id\":\"672bca85986a1370676d969f\",\"full_name\":\"Chenguang Zhu\",\"affiliation\":null,\"orcid\":null,\"semantic_scholarid\":null,\"user_id\":null},{\"_id\":\"672bcd9d986a1370676dcaba\",\"full_name\":\"Jie Lei\",\"affiliation\":null,\"orcid\":null,\"semantic_scholarid\":null,\"user_id\":null},{\"_id\":\"672bcdd6986a1370676dce68\",\"full_name\":\"Xudong Lin\",\"affiliation\":null,\"orcid\":null,\"semantic_scholarid\":null,\"user_id\":null},{\"_id\":\"672bcefd986a1370676de2d5\",\"full_name\":\"Derek Hoiem\",\"affiliation\":null,\"orcid\":null,\"semantic_scholarid\":null,\"user_id\":null},{\"_id\":\"672bcf2b986a1370676de68a\",\"full_name\":\"Shuohang Wang\",\"affiliation\":null,\"orcid\":null,\"semantic_scholarid\":null,\"user_id\":null},{\"_id\":\"672bd0f3986a1370676e0ca9\",\"full_name\":\"Zhenhailong Wang\",\"affiliation\":null,\"orcid\":null,\"semantic_scholarid\":null,\"user_id\":null},{\"_id\":\"67322fe0cd1e32a6e7f0ada6\",\"full_name\":\"Ruochen Xu\",\"affiliation\":null,\"orcid\":null,\"semantic_scholarid\":null,\"user_id\":null}],\"pdf_info\":{\"fetcher_url\":\"https://fetcher.alphaxiv.org/v2/pdf/2205.10747v4\"}}},\"dataUpdateCount\":1,\"dataUpdatedAt\":1744228186296,\"error\":null,\"errorUpdateCount\":0,\"errorUpdatedAt\":0,\"fetchFailureCount\":0,\"fetchFailureReason\":null,\"fetchMeta\":null,\"isInvalidated\":false,\"status\":\"success\",\"fetchStatus\":\"idle\"},\"queryKey\":[\"paper\",\"2205.10747\",\"metadata\"],\"queryHash\":\"[\\\"paper\\\",\\\"2205.10747\\\",\\\"metadata\\\"]\"},{\"state\":{\"data\":{\"data\":[]},\"dataUpdateCount\":1,\"dataUpdatedAt\":1744228186296,\"error\":null,\"errorUpdateCount\":0,\"errorUpdatedAt\":0,\"fetchFailureCount\":0,\"fetchFailureReason\":null,\"fetchMeta\":null,\"isInvalidated\":false,\"status\":\"success\",\"fetchStatus\":\"idle\"},\"queryKey\":[\"paper\",\"2205.10747\",\"comments\"],\"queryHash\":\"[\\\"paper\\\",\\\"2205.10747\\\",\\\"comments\\\"]\"},{\"state\":{\"data\":{\"data\":{\"paper_version\":{\"_id\":\"67b2d56cce74fa4744372612\",\"paper_group_id\":\"67b2d56bce74fa474437260f\",\"version_label\":\"v1\",\"version_order\":1,\"title\":\"Verified error bounds for the singular values of structured matrices with applications to computer-assisted proofs for differential equations\",\"abstract\":\"This paper introduces two methods for verifying the singular values of the\\nstructured matrix denoted by $R^{-H}AR^{-1}$, where $R$ is a nonsingular matrix\\nand $A$ is a general nonsingular square matrix. The first of the two methods\\nuses the computed factors from a singular value decomposition (SVD) to verify\\nall singular values; the second estimates a lower bound of the minimum singular\\nvalue without performing the SVD. The proposed approach for verifying all\\nsingular values efficiently computes tight error bounds. The method for\\nestimating a lower bound of the minimum singular value is particularly\\neffective for sparse matrices. These methods have proven to be efficient in\\nverifying solutions to differential equation problems, that were previously\\nchallenging due to the extensive computational time and memory requirements.\",\"author_ids\":[\"67b2d56cce74fa4744372610\",\"67b2d56cce74fa4744372611\",\"67333d6dc48bba476d789ca3\"],\"publication_date\":\"2025-02-14T08:12:06.000Z\",\"license\":\"http://arxiv.org/licenses/nonexclusive-distrib/1.0/\",\"created_at\":\"2025-02-17T06:21:32.657Z\",\"updated_at\":\"2025-02-17T06:21:32.657Z\",\"is_deleted\":false,\"is_hidden\":false,\"universal_paper_id\":\"2502.09984\",\"imageURL\":\"image/2502.09984v1.png\"},\"paper_group\":{\"_id\":\"67b2d56bce74fa474437260f\",\"universal_paper_id\":\"2502.09984\",\"title\":\"Verified error bounds for the singular values of structured matrices with applications to computer-assisted proofs for differential equations\",\"created_at\":\"2025-02-17T06:21:31.775Z\",\"updated_at\":\"2025-03-03T19:36:09.677Z\",\"categories\":[\"Mathematics\"],\"subcategories\":[\"math.NA\"],\"custom_categories\":null,\"author_user_ids\":[],\"source\":{\"name\":\"alphaXiv\",\"url\":\"https://arxiv.org/abs/2502.09984\"},\"metrics\":{\"activity_rank\":0,\"questions_count\":0,\"responses_count\":0,\"upvotes_count\":0,\"downvotes_count\":0,\"total_votes\":0,\"public_total_votes\":0,\"visits_count\":{\"last24Hours\":0,\"last7Days\":2,\"last30Days\":3,\"last90Days\":4,\"all\":4},\"weighted_visits\":{\"last24Hours\":0,\"last7Days\":0.12683442410582935,\"last30Days\":1.576290489907939,\"last90Days\":4,\"hot\":0.12683442410582935},\"timeline\":[{\"date\":\"2025-04-02T23:39:09.714Z\",\"views\":3},{\"date\":\"2025-03-30T11:39:09.714Z\",\"views\":3},{\"date\":\"2025-03-26T23:39:09.714Z\",\"views\":5},{\"date\":\"2025-03-23T11:39:09.714Z\",\"views\":0},{\"date\":\"2025-03-19T23:39:09.714Z\",\"views\":1},{\"date\":\"2025-03-16T11:39:09.714Z\",\"views\":1},{\"date\":\"2025-03-12T23:39:09.714Z\",\"views\":0},{\"date\":\"2025-03-09T11:39:09.714Z\",\"views\":0},{\"date\":\"2025-03-05T23:39:09.714Z\",\"views\":1},{\"date\":\"2025-03-02T11:39:09.714Z\",\"views\":2},{\"date\":\"2025-02-26T23:39:09.714Z\",\"views\":1},{\"date\":\"2025-02-23T11:39:09.714Z\",\"views\":1},{\"date\":\"2025-02-19T23:39:09.731Z\",\"views\":1},{\"date\":\"2025-02-16T11:39:09.748Z\",\"views\":4},{\"date\":\"2025-02-12T23:39:09.761Z\",\"views\":1}]},\"is_hidden\":false,\"first_publication_date\":\"2025-02-14T08:12:06.000Z\",\"organizations\":[\"67be6386aa92218ccd8b14d5\",\"67be655caa92218ccd8b4fbe\"],\"citation\":{\"bibtex\":\"@misc{ozaki2025verifiederrorbounds,\\n title={Verified error bounds for the singular values of structured matrices with applications to computer-assisted proofs for differential equations}, \\n author={Katsuhisa Ozaki and Takeshi Terao and Yoshitaka Watanabe},\\n year={2025},\\n eprint={2502.09984},\\n archivePrefix={arXiv},\\n primaryClass={math.NA},\\n url={https://arxiv.org/abs/2502.09984}, \\n}\"},\"paperVersions\":{\"_id\":\"67b2d56cce74fa4744372612\",\"paper_group_id\":\"67b2d56bce74fa474437260f\",\"version_label\":\"v1\",\"version_order\":1,\"title\":\"Verified error bounds for the singular values of structured matrices with applications to computer-assisted proofs for differential equations\",\"abstract\":\"This paper introduces two methods for verifying the singular values of the\\nstructured matrix denoted by $R^{-H}AR^{-1}$, where $R$ is a nonsingular matrix\\nand $A$ is a general nonsingular square matrix. The first of the two methods\\nuses the computed factors from a singular value decomposition (SVD) to verify\\nall singular values; the second estimates a lower bound of the minimum singular\\nvalue without performing the SVD. The proposed approach for verifying all\\nsingular values efficiently computes tight error bounds. The method for\\nestimating a lower bound of the minimum singular value is particularly\\neffective for sparse matrices. These methods have proven to be efficient in\\nverifying solutions to differential equation problems, that were previously\\nchallenging due to the extensive computational time and memory requirements.\",\"author_ids\":[\"67b2d56cce74fa4744372610\",\"67b2d56cce74fa4744372611\",\"67333d6dc48bba476d789ca3\"],\"publication_date\":\"2025-02-14T08:12:06.000Z\",\"license\":\"http://arxiv.org/licenses/nonexclusive-distrib/1.0/\",\"created_at\":\"2025-02-17T06:21:32.657Z\",\"updated_at\":\"2025-02-17T06:21:32.657Z\",\"is_deleted\":false,\"is_hidden\":false,\"universal_paper_id\":\"2502.09984\",\"imageURL\":\"image/2502.09984v1.png\"},\"verifiedAuthors\":[],\"authors\":[{\"_id\":\"67333d6dc48bba476d789ca3\",\"full_name\":\"Katsuhisa Ozaki\",\"affiliation\":null,\"orcid\":null,\"semantic_scholarid\":null,\"user_id\":null},{\"_id\":\"67b2d56cce74fa4744372610\",\"full_name\":\"Takeshi Terao\",\"affiliation\":null,\"orcid\":null,\"semantic_scholarid\":null,\"user_id\":null},{\"_id\":\"67b2d56cce74fa4744372611\",\"full_name\":\"Yoshitaka Watanabe\",\"affiliation\":null,\"orcid\":null,\"semantic_scholarid\":null,\"user_id\":null}]},\"max_version_order\":1,\"verified_authors\":[],\"authors\":[{\"_id\":\"67333d6dc48bba476d789ca3\",\"full_name\":\"Katsuhisa Ozaki\",\"affiliation\":null,\"orcid\":null,\"semantic_scholarid\":null,\"user_id\":null},{\"_id\":\"67b2d56cce74fa4744372610\",\"full_name\":\"Takeshi Terao\",\"affiliation\":null,\"orcid\":null,\"semantic_scholarid\":null,\"user_id\":null},{\"_id\":\"67b2d56cce74fa4744372611\",\"full_name\":\"Yoshitaka Watanabe\",\"affiliation\":null,\"orcid\":null,\"semantic_scholarid\":null,\"user_id\":null}],\"pdf_info\":{\"fetcher_url\":\"https://fetcher.alphaxiv.org/v2/pdf/2502.09984v1\"}}},\"dataUpdateCount\":2,\"dataUpdatedAt\":1744228191028,\"error\":null,\"errorUpdateCount\":0,\"errorUpdatedAt\":0,\"fetchFailureCount\":0,\"fetchFailureReason\":null,\"fetchMeta\":null,\"isInvalidated\":false,\"status\":\"success\",\"fetchStatus\":\"idle\"},\"queryKey\":[\"paper\",\"2502.09984\",\"metadata\"],\"queryHash\":\"[\\\"paper\\\",\\\"2502.09984\\\",\\\"metadata\\\"]\"},{\"state\":{\"data\":{\"data\":[]},\"dataUpdateCount\":2,\"dataUpdatedAt\":1744228191028,\"error\":null,\"errorUpdateCount\":0,\"errorUpdatedAt\":0,\"fetchFailureCount\":0,\"fetchFailureReason\":null,\"fetchMeta\":null,\"isInvalidated\":false,\"status\":\"success\",\"fetchStatus\":\"idle\"},\"queryKey\":[\"paper\",\"2502.09984\",\"comments\"],\"queryHash\":\"[\\\"paper\\\",\\\"2502.09984\\\",\\\"comments\\\"]\"},{\"state\":{\"data\":{\"data\":{\"paper_version\":{\"_id\":\"674c633d0567cfa0fdf61509\",\"paper_group_id\":\"674c633d0567cfa0fdf61508\",\"version_label\":\"v2\",\"version_order\":2,\"title\":\"Quantum Martingale Theory and Entropy Production\",\"abstract\":\"We develop a martingale theory to describe fluctuations of entropy production for open quantum systems in nonequilbrium steady states. Using the formalism of quantum jump trajectories, we identify a decomposition of entropy production into an exponential martingale and a purely quantum term, both obeying integral fluctuation theorems. An important consequence of this approach is the derivation of a set of genuine universal results for stopping-time and infimum statistics of stochastic entropy production. Finally we complement the general formalism with numerical simulations of a qubit system.\",\"author_ids\":[\"674130f0738130185cc1ec22\",\"673215ddcd1e32a6e7efb8d0\",\"673491c893ee43749600fe66\"],\"publication_date\":\"2019-06-11T07:43:34.000Z\",\"license\":\"http://arxiv.org/licenses/nonexclusive-distrib/1.0/\",\"created_at\":\"2024-12-01T13:23:09.901Z\",\"updated_at\":\"2024-12-01T13:23:09.901Z\",\"is_deleted\":false,\"is_hidden\":false,\"universal_paper_id\":\"1903.02925\",\"imageURL\":\"image/1903.02925v2.png\"},\"paper_group\":{\"_id\":\"674c633d0567cfa0fdf61508\",\"universal_paper_id\":\"1903.02925\",\"source\":{\"name\":\"arXiv\",\"url\":\"https://arXiv.org/paper/1903.02925\"},\"title\":\"Quantum Martingale Theory and Entropy Production\",\"created_at\":\"2024-12-01T13:22:57.511Z\",\"updated_at\":\"2025-03-03T21:04:13.704Z\",\"categories\":[\"Physics\"],\"subcategories\":[\"quant-ph\",\"cond-mat.stat-mech\"],\"metrics\":{\"activity_rank\":0,\"questions_count\":0,\"responses_count\":0,\"upvotes_count\":null,\"downvotes_count\":null,\"total_votes\":0,\"visits_count\":{\"last24Hours\":0,\"last7Days\":0,\"last30Days\":1,\"last90Days\":2,\"all\":3},\"weighted_visits\":{\"last24Hours\":0,\"last7Days\":0,\"last30Days\":5.052813378791358e-13,\"last90Days\":0.00015929705556717868,\"hot\":0},\"public_total_votes\":0,\"timeline\":[{\"date\":\"2025-03-30T21:03:06.553Z\",\"views\":1},{\"date\":\"2025-03-27T09:03:06.553Z\",\"views\":3},{\"date\":\"2025-03-23T21:03:06.553Z\",\"views\":2},{\"date\":\"2025-03-20T09:03:06.553Z\",\"views\":2},{\"date\":\"2025-03-16T21:03:06.553Z\",\"views\":2},{\"date\":\"2025-03-13T09:03:06.553Z\",\"views\":1},{\"date\":\"2025-03-09T21:03:06.553Z\",\"views\":0},{\"date\":\"2025-03-06T09:03:06.553Z\",\"views\":1},{\"date\":\"2025-03-02T21:03:06.553Z\",\"views\":0},{\"date\":\"2025-02-27T09:03:06.553Z\",\"views\":0},{\"date\":\"2025-02-23T21:03:06.553Z\",\"views\":2},{\"date\":\"2025-02-20T09:03:06.566Z\",\"views\":1},{\"date\":\"2025-02-16T21:03:06.598Z\",\"views\":0},{\"date\":\"2025-02-13T09:03:06.620Z\",\"views\":0},{\"date\":\"2025-02-09T21:03:06.639Z\",\"views\":2},{\"date\":\"2025-02-06T09:03:06.671Z\",\"views\":5},{\"date\":\"2025-02-02T21:03:06.697Z\",\"views\":2},{\"date\":\"2025-01-30T09:03:06.715Z\",\"views\":2},{\"date\":\"2025-01-26T21:03:06.736Z\",\"views\":0},{\"date\":\"2025-01-23T09:03:06.759Z\",\"views\":0},{\"date\":\"2025-01-19T21:03:06.780Z\",\"views\":2},{\"date\":\"2025-01-16T09:03:06.804Z\",\"views\":0},{\"date\":\"2025-01-12T21:03:06.828Z\",\"views\":1},{\"date\":\"2025-01-09T09:03:06.852Z\",\"views\":1},{\"date\":\"2025-01-05T21:03:06.875Z\",\"views\":1},{\"date\":\"2025-01-02T09:03:06.901Z\",\"views\":0},{\"date\":\"2024-12-29T21:03:06.934Z\",\"views\":1},{\"date\":\"2024-12-26T09:03:06.957Z\",\"views\":2},{\"date\":\"2024-12-22T21:03:06.979Z\",\"views\":2},{\"date\":\"2024-12-19T09:03:07.020Z\",\"views\":1},{\"date\":\"2024-12-15T21:03:07.051Z\",\"views\":0},{\"date\":\"2024-12-12T09:03:07.087Z\",\"views\":1},{\"date\":\"2024-12-08T21:03:07.123Z\",\"views\":0},{\"date\":\"2024-12-05T09:03:07.149Z\",\"views\":1},{\"date\":\"2024-12-01T21:03:07.175Z\",\"views\":2},{\"date\":\"2024-11-28T09:03:07.194Z\",\"views\":3},{\"date\":\"2024-11-24T21:03:07.216Z\",\"views\":0},{\"date\":\"2024-11-21T09:03:07.238Z\",\"views\":0},{\"date\":\"2024-11-17T21:03:07.257Z\",\"views\":2},{\"date\":\"2024-11-14T09:03:07.278Z\",\"views\":1},{\"date\":\"2024-11-10T21:03:07.296Z\",\"views\":1},{\"date\":\"2024-11-07T09:03:07.320Z\",\"views\":1},{\"date\":\"2024-11-03T21:03:07.338Z\",\"views\":0},{\"date\":\"2024-10-31T08:03:07.361Z\",\"views\":2},{\"date\":\"2024-10-27T20:03:07.381Z\",\"views\":1},{\"date\":\"2024-10-24T08:03:07.411Z\",\"views\":2},{\"date\":\"2024-10-20T20:03:07.444Z\",\"views\":1},{\"date\":\"2024-10-17T08:03:07.473Z\",\"views\":2},{\"date\":\"2024-10-13T20:03:07.497Z\",\"views\":1},{\"date\":\"2024-10-10T08:03:07.520Z\",\"views\":1},{\"date\":\"2024-10-06T20:03:07.547Z\",\"views\":1},{\"date\":\"2024-10-03T08:03:07.567Z\",\"views\":1},{\"date\":\"2024-09-29T20:03:07.591Z\",\"views\":2},{\"date\":\"2024-09-26T08:03:07.622Z\",\"views\":1},{\"date\":\"2024-09-22T20:03:07.647Z\",\"views\":2},{\"date\":\"2024-09-19T08:03:07.668Z\",\"views\":2},{\"date\":\"2024-09-15T20:03:07.688Z\",\"views\":1},{\"date\":\"2024-09-12T08:03:07.707Z\",\"views\":2},{\"date\":\"2024-09-08T20:03:07.733Z\",\"views\":1},{\"date\":\"2024-09-05T08:03:07.757Z\",\"views\":2},{\"date\":\"2024-09-01T20:03:07.783Z\",\"views\":1},{\"date\":\"2024-08-29T08:03:07.802Z\",\"views\":1}]},\"ranking\":{\"current_rank\":0,\"previous_rank\":0,\"activity_score\":0,\"paper_score\":0},\"is_hidden\":false,\"first_publication_date\":\"2019-06-11T07:43:34.000Z\",\"author_user_ids\":[],\"paperVersions\":{\"_id\":\"674c633d0567cfa0fdf61509\",\"paper_group_id\":\"674c633d0567cfa0fdf61508\",\"version_label\":\"v2\",\"version_order\":2,\"title\":\"Quantum Martingale Theory and Entropy Production\",\"abstract\":\"We develop a martingale theory to describe fluctuations of entropy production for open quantum systems in nonequilbrium steady states. Using the formalism of quantum jump trajectories, we identify a decomposition of entropy production into an exponential martingale and a purely quantum term, both obeying integral fluctuation theorems. An important consequence of this approach is the derivation of a set of genuine universal results for stopping-time and infimum statistics of stochastic entropy production. Finally we complement the general formalism with numerical simulations of a qubit system.\",\"author_ids\":[\"674130f0738130185cc1ec22\",\"673215ddcd1e32a6e7efb8d0\",\"673491c893ee43749600fe66\"],\"publication_date\":\"2019-06-11T07:43:34.000Z\",\"license\":\"http://arxiv.org/licenses/nonexclusive-distrib/1.0/\",\"created_at\":\"2024-12-01T13:23:09.901Z\",\"updated_at\":\"2024-12-01T13:23:09.901Z\",\"is_deleted\":false,\"is_hidden\":false,\"universal_paper_id\":\"1903.02925\",\"imageURL\":\"image/1903.02925v2.png\"},\"verifiedAuthors\":[],\"authors\":[{\"_id\":\"673215ddcd1e32a6e7efb8d0\",\"full_name\":\"Rosario Fazio\",\"affiliation\":null,\"orcid\":null,\"semantic_scholarid\":null,\"user_id\":null},{\"_id\":\"673491c893ee43749600fe66\",\"full_name\":\"Édgar Roldán\",\"affiliation\":null,\"orcid\":null,\"semantic_scholarid\":null,\"user_id\":null},{\"_id\":\"674130f0738130185cc1ec22\",\"full_name\":\"Gonzalo Manzano\",\"affiliation\":null,\"orcid\":null,\"semantic_scholarid\":null,\"user_id\":null}]},\"max_version_order\":2,\"verified_authors\":[],\"authors\":[{\"_id\":\"673215ddcd1e32a6e7efb8d0\",\"full_name\":\"Rosario Fazio\",\"affiliation\":null,\"orcid\":null,\"semantic_scholarid\":null,\"user_id\":null},{\"_id\":\"673491c893ee43749600fe66\",\"full_name\":\"Édgar Roldán\",\"affiliation\":null,\"orcid\":null,\"semantic_scholarid\":null,\"user_id\":null},{\"_id\":\"674130f0738130185cc1ec22\",\"full_name\":\"Gonzalo Manzano\",\"affiliation\":null,\"orcid\":null,\"semantic_scholarid\":null,\"user_id\":null}],\"pdf_info\":{\"fetcher_url\":\"https://fetcher.alphaxiv.org/v2/pdf/1903.02925v2\"}}},\"dataUpdateCount\":1,\"dataUpdatedAt\":1744228190781,\"error\":null,\"errorUpdateCount\":0,\"errorUpdatedAt\":0,\"fetchFailureCount\":0,\"fetchFailureReason\":null,\"fetchMeta\":null,\"isInvalidated\":false,\"status\":\"success\",\"fetchStatus\":\"idle\"},\"queryKey\":[\"paper\",\"1903.02925\",\"metadata\"],\"queryHash\":\"[\\\"paper\\\",\\\"1903.02925\\\",\\\"metadata\\\"]\"},{\"state\":{\"data\":{\"data\":[]},\"dataUpdateCount\":1,\"dataUpdatedAt\":1744228190781,\"error\":null,\"errorUpdateCount\":0,\"errorUpdatedAt\":0,\"fetchFailureCount\":0,\"fetchFailureReason\":null,\"fetchMeta\":null,\"isInvalidated\":false,\"status\":\"success\",\"fetchStatus\":\"idle\"},\"queryKey\":[\"paper\",\"1903.02925\",\"comments\"],\"queryHash\":\"[\\\"paper\\\",\\\"1903.02925\\\",\\\"comments\\\"]\"},{\"state\":{\"data\":{\"data\":{\"paper_version\":{\"_id\":\"674df524f3629ec53b0ec513\",\"paper_group_id\":\"674df523f3629ec53b0ec512\",\"version_label\":\"v2\",\"version_order\":2,\"title\":\"Probability distribution function of the aperture mass field with large deviation theory\",\"abstract\":\"$42\",\"author_ids\":[\"674d37b2e57dd4be770d6a00\",\"673cb2548a52218f8bc90d85\",\"673d1430bdf5ad128bc1f881\"],\"publication_date\":\"2021-03-16T10:57:15.000Z\",\"license\":\"http://arxiv.org/licenses/nonexclusive-distrib/1.0/\",\"created_at\":\"2024-12-02T17:57:56.475Z\",\"updated_at\":\"2024-12-02T17:57:56.475Z\",\"is_deleted\":false,\"is_hidden\":false,\"universal_paper_id\":\"2012.03831\",\"imageURL\":\"image/2012.03831v2.png\"},\"paper_group\":{\"_id\":\"674df523f3629ec53b0ec512\",\"universal_paper_id\":\"2012.03831\",\"source\":{\"name\":\"arXiv\",\"url\":\"https://arXiv.org/paper/2012.03831\"},\"title\":\"Probability distribution function of the aperture mass field with large deviation theory\",\"created_at\":\"2024-12-02T14:10:11.973Z\",\"updated_at\":\"2025-03-03T20:47:31.669Z\",\"categories\":[\"Physics\"],\"subcategories\":[\"astro-ph.CO\"],\"custom_categories\":null,\"metrics\":{\"activity_rank\":0,\"questions_count\":0,\"responses_count\":0,\"upvotes_count\":null,\"downvotes_count\":null,\"total_votes\":0,\"visits_count\":{\"last24Hours\":0,\"last7Days\":0,\"last30Days\":1,\"last90Days\":3,\"all\":18},\"weighted_visits\":{\"last24Hours\":0,\"last7Days\":0,\"last30Days\":2.7141002202618938e-9,\"last90Days\":0.00418468926419965,\"hot\":0},\"public_total_votes\":0,\"timeline\":[{\"date\":\"2025-04-02T03:20:36.700Z\",\"views\":2},{\"date\":\"2025-03-29T15:20:36.700Z\",\"views\":5},{\"date\":\"2025-03-26T03:20:36.700Z\",\"views\":2},{\"date\":\"2025-03-22T15:20:36.700Z\",\"views\":1},{\"date\":\"2025-03-19T03:20:36.700Z\",\"views\":2},{\"date\":\"2025-03-15T15:20:36.700Z\",\"views\":1},{\"date\":\"2025-03-12T03:20:36.700Z\",\"views\":2},{\"date\":\"2025-03-08T15:20:36.700Z\",\"views\":1},{\"date\":\"2025-03-05T03:20:36.700Z\",\"views\":0},{\"date\":\"2025-03-01T15:20:36.700Z\",\"views\":2},{\"date\":\"2025-02-26T03:20:36.700Z\",\"views\":0},{\"date\":\"2025-02-22T15:20:36.700Z\",\"views\":1},{\"date\":\"2025-02-19T03:20:36.715Z\",\"views\":0},{\"date\":\"2025-02-15T15:20:36.733Z\",\"views\":0},{\"date\":\"2025-02-12T03:20:36.758Z\",\"views\":2},{\"date\":\"2025-02-08T15:20:36.773Z\",\"views\":0},{\"date\":\"2025-02-05T03:20:36.790Z\",\"views\":6},{\"date\":\"2025-02-01T15:20:36.802Z\",\"views\":1},{\"date\":\"2025-01-29T03:20:36.817Z\",\"views\":0},{\"date\":\"2025-01-25T15:20:36.834Z\",\"views\":2},{\"date\":\"2025-01-22T03:20:36.850Z\",\"views\":1},{\"date\":\"2025-01-18T15:20:36.870Z\",\"views\":2},{\"date\":\"2025-01-15T03:20:36.893Z\",\"views\":0},{\"date\":\"2025-01-11T15:20:36.909Z\",\"views\":1},{\"date\":\"2025-01-08T03:20:36.924Z\",\"views\":2},{\"date\":\"2025-01-04T15:20:36.940Z\",\"views\":0},{\"date\":\"2025-01-01T03:20:36.954Z\",\"views\":2},{\"date\":\"2024-12-28T15:20:36.972Z\",\"views\":0},{\"date\":\"2024-12-25T03:20:36.989Z\",\"views\":2},{\"date\":\"2024-12-21T15:20:37.006Z\",\"views\":0},{\"date\":\"2024-12-18T03:20:37.023Z\",\"views\":2},{\"date\":\"2024-12-14T15:20:37.040Z\",\"views\":0},{\"date\":\"2024-12-11T03:20:37.056Z\",\"views\":1},{\"date\":\"2024-12-07T15:20:37.071Z\",\"views\":1},{\"date\":\"2024-12-04T03:20:37.094Z\",\"views\":0},{\"date\":\"2024-11-30T15:20:37.113Z\",\"views\":10},{\"date\":\"2024-11-27T03:20:37.133Z\",\"views\":2},{\"date\":\"2024-11-23T15:20:37.148Z\",\"views\":0},{\"date\":\"2024-11-20T03:20:37.167Z\",\"views\":0},{\"date\":\"2024-11-16T15:20:37.182Z\",\"views\":1},{\"date\":\"2024-11-13T03:20:37.197Z\",\"views\":0},{\"date\":\"2024-11-09T15:20:37.213Z\",\"views\":0},{\"date\":\"2024-11-06T03:20:37.235Z\",\"views\":0},{\"date\":\"2024-11-02T14:20:37.250Z\",\"views\":1},{\"date\":\"2024-10-30T02:20:37.268Z\",\"views\":0},{\"date\":\"2024-10-26T14:20:37.285Z\",\"views\":2},{\"date\":\"2024-10-23T02:20:37.303Z\",\"views\":0},{\"date\":\"2024-10-19T14:20:37.320Z\",\"views\":2},{\"date\":\"2024-10-16T02:20:37.337Z\",\"views\":0},{\"date\":\"2024-10-12T14:20:37.353Z\",\"views\":0},{\"date\":\"2024-10-09T02:20:37.375Z\",\"views\":2},{\"date\":\"2024-10-05T14:20:37.399Z\",\"views\":2},{\"date\":\"2024-10-02T02:20:37.418Z\",\"views\":0},{\"date\":\"2024-09-28T14:20:37.442Z\",\"views\":0},{\"date\":\"2024-09-25T02:20:37.457Z\",\"views\":0},{\"date\":\"2024-09-21T14:20:37.474Z\",\"views\":1},{\"date\":\"2024-09-18T02:20:37.493Z\",\"views\":1},{\"date\":\"2024-09-14T14:20:37.506Z\",\"views\":2},{\"date\":\"2024-09-11T02:20:37.524Z\",\"views\":2},{\"date\":\"2024-09-07T14:20:37.544Z\",\"views\":2},{\"date\":\"2024-09-04T02:20:37.575Z\",\"views\":1},{\"date\":\"2024-08-31T14:20:37.592Z\",\"views\":1},{\"date\":\"2024-08-28T02:20:37.602Z\",\"views\":2}]},\"ranking\":{\"current_rank\":0,\"previous_rank\":0,\"activity_score\":0,\"paper_score\":0},\"is_hidden\":false,\"first_publication_date\":\"2021-03-16T10:57:15.000Z\",\"author_user_ids\":[],\"citation\":{\"bibtex\":\"@misc{codis2021probabilitydistributionfunction,\\n title={Probability distribution function of the aperture mass field with large deviation theory}, \\n author={Sandrine Codis and Francis Bernardeau and Alexandre Barthelemy},\\n year={2021},\\n eprint={2012.03831},\\n archivePrefix={arXiv},\\n primaryClass={astro-ph.CO},\\n url={https://arxiv.org/abs/2012.03831}, \\n}\"},\"paperVersions\":{\"_id\":\"674df524f3629ec53b0ec513\",\"paper_group_id\":\"674df523f3629ec53b0ec512\",\"version_label\":\"v2\",\"version_order\":2,\"title\":\"Probability distribution function of the aperture mass field with large deviation theory\",\"abstract\":\"$43\",\"author_ids\":[\"674d37b2e57dd4be770d6a00\",\"673cb2548a52218f8bc90d85\",\"673d1430bdf5ad128bc1f881\"],\"publication_date\":\"2021-03-16T10:57:15.000Z\",\"license\":\"http://arxiv.org/licenses/nonexclusive-distrib/1.0/\",\"created_at\":\"2024-12-02T17:57:56.475Z\",\"updated_at\":\"2024-12-02T17:57:56.475Z\",\"is_deleted\":false,\"is_hidden\":false,\"universal_paper_id\":\"2012.03831\",\"imageURL\":\"image/2012.03831v2.png\"},\"verifiedAuthors\":[],\"authors\":[{\"_id\":\"673cb2548a52218f8bc90d85\",\"full_name\":\"Sandrine Codis\",\"affiliation\":null,\"orcid\":null,\"semantic_scholarid\":null,\"user_id\":null},{\"_id\":\"673d1430bdf5ad128bc1f881\",\"full_name\":\"Francis Bernardeau\",\"affiliation\":null,\"orcid\":null,\"semantic_scholarid\":null,\"user_id\":null},{\"_id\":\"674d37b2e57dd4be770d6a00\",\"full_name\":\"Alexandre Barthelemy\",\"affiliation\":null,\"orcid\":null,\"semantic_scholarid\":null,\"user_id\":null}]},\"max_version_order\":2,\"verified_authors\":[],\"authors\":[{\"_id\":\"673cb2548a52218f8bc90d85\",\"full_name\":\"Sandrine Codis\",\"affiliation\":null,\"orcid\":null,\"semantic_scholarid\":null,\"user_id\":null},{\"_id\":\"673d1430bdf5ad128bc1f881\",\"full_name\":\"Francis Bernardeau\",\"affiliation\":null,\"orcid\":null,\"semantic_scholarid\":null,\"user_id\":null},{\"_id\":\"674d37b2e57dd4be770d6a00\",\"full_name\":\"Alexandre Barthelemy\",\"affiliation\":null,\"orcid\":null,\"semantic_scholarid\":null,\"user_id\":null}],\"pdf_info\":{\"fetcher_url\":\"https://fetcher.alphaxiv.org/v2/pdf/2012.03831v2\"}}},\"dataUpdateCount\":1,\"dataUpdatedAt\":1744228191148,\"error\":null,\"errorUpdateCount\":0,\"errorUpdatedAt\":0,\"fetchFailureCount\":0,\"fetchFailureReason\":null,\"fetchMeta\":null,\"isInvalidated\":false,\"status\":\"success\",\"fetchStatus\":\"idle\"},\"queryKey\":[\"paper\",\"2012.03831\",\"metadata\"],\"queryHash\":\"[\\\"paper\\\",\\\"2012.03831\\\",\\\"metadata\\\"]\"},{\"state\":{\"data\":{\"data\":[]},\"dataUpdateCount\":1,\"dataUpdatedAt\":1744228191148,\"error\":null,\"errorUpdateCount\":0,\"errorUpdatedAt\":0,\"fetchFailureCount\":0,\"fetchFailureReason\":null,\"fetchMeta\":null,\"isInvalidated\":false,\"status\":\"success\",\"fetchStatus\":\"idle\"},\"queryKey\":[\"paper\",\"2012.03831\",\"comments\"],\"queryHash\":\"[\\\"paper\\\",\\\"2012.03831\\\",\\\"comments\\\"]\"},{\"state\":{\"data\":{\"data\":{\"paper_version\":{\"_id\":\"67e2bc363a581fde71a4822c\",\"paper_group_id\":\"67e2bc363a581fde71a4822b\",\"version_label\":\"v5\",\"version_order\":5,\"title\":\"Modeling Migration-Induced Unemployment\",\"abstract\":\"$44\",\"author_ids\":[\"6732160ccd1e32a6e7efbbce\"],\"publication_date\":\"2024-12-10T16:30:23.000Z\",\"license\":\"http://creativecommons.org/licenses/by/4.0/\",\"created_at\":\"2025-03-25T14:22:46.065Z\",\"updated_at\":\"2025-03-25T14:22:46.065Z\",\"is_deleted\":false,\"is_hidden\":false,\"universal_paper_id\":\"2303.13319\",\"imageURL\":\"image/2303.13319v5.png\"},\"paper_group\":{\"_id\":\"67e2bc363a581fde71a4822b\",\"universal_paper_id\":\"2303.13319\",\"title\":\"Modeling Migration-Induced Unemployment\",\"created_at\":\"2025-03-25T14:22:46.022Z\",\"updated_at\":\"2025-03-25T14:22:46.022Z\",\"categories\":[\"Economics\"],\"subcategories\":[\"econ.GN\"],\"custom_categories\":null,\"author_user_ids\":[],\"source\":{\"name\":\"alphaXiv\",\"url\":\"https://arxiv.org/abs/2303.13319\"},\"metrics\":{\"activity_rank\":0,\"questions_count\":0,\"responses_count\":0,\"upvotes_count\":0,\"downvotes_count\":0,\"total_votes\":0,\"public_total_votes\":12,\"visits_count\":{\"last24Hours\":1,\"last7Days\":3,\"last30Days\":6,\"last90Days\":6,\"all\":18},\"timeline\":[{\"date\":\"2025-04-01T20:30:48.639Z\",\"views\":8},{\"date\":\"2025-03-29T08:30:48.639Z\",\"views\":2},{\"date\":\"2025-03-25T20:30:48.639Z\",\"views\":8},{\"date\":\"2025-03-22T08:30:48.639Z\",\"views\":5},{\"date\":\"2025-03-18T20:30:48.677Z\",\"views\":1},{\"date\":\"2025-03-15T08:30:48.700Z\",\"views\":0},{\"date\":\"2025-03-11T20:30:48.725Z\",\"views\":0},{\"date\":\"2025-03-08T08:30:48.750Z\",\"views\":2},{\"date\":\"2025-03-04T20:30:48.775Z\",\"views\":1},{\"date\":\"2025-03-01T08:30:48.800Z\",\"views\":2},{\"date\":\"2025-02-25T20:30:48.825Z\",\"views\":1},{\"date\":\"2025-02-22T08:30:48.850Z\",\"views\":1},{\"date\":\"2025-02-18T20:30:48.874Z\",\"views\":1},{\"date\":\"2025-02-15T08:30:48.899Z\",\"views\":2},{\"date\":\"2025-02-11T20:30:48.924Z\",\"views\":2},{\"date\":\"2025-02-08T08:30:48.948Z\",\"views\":2},{\"date\":\"2025-02-04T20:30:48.972Z\",\"views\":0},{\"date\":\"2025-02-01T08:30:48.997Z\",\"views\":1},{\"date\":\"2025-01-28T20:30:49.022Z\",\"views\":1},{\"date\":\"2025-01-25T08:30:49.047Z\",\"views\":0},{\"date\":\"2025-01-21T20:30:49.071Z\",\"views\":0},{\"date\":\"2025-01-18T08:30:49.095Z\",\"views\":2},{\"date\":\"2025-01-14T20:30:49.120Z\",\"views\":0},{\"date\":\"2025-01-11T08:30:49.144Z\",\"views\":0},{\"date\":\"2025-01-07T20:30:49.169Z\",\"views\":0},{\"date\":\"2025-01-04T08:30:49.194Z\",\"views\":0},{\"date\":\"2024-12-31T20:30:49.218Z\",\"views\":0},{\"date\":\"2024-12-28T08:30:49.243Z\",\"views\":2},{\"date\":\"2024-12-24T20:30:49.268Z\",\"views\":2},{\"date\":\"2024-12-21T08:30:49.292Z\",\"views\":0},{\"date\":\"2024-12-17T20:30:49.716Z\",\"views\":1},{\"date\":\"2024-12-14T08:30:49.760Z\",\"views\":2},{\"date\":\"2024-12-10T20:30:49.785Z\",\"views\":2},{\"date\":\"2024-12-07T08:30:49.809Z\",\"views\":2},{\"date\":\"2024-12-03T20:30:49.834Z\",\"views\":1},{\"date\":\"2024-11-30T08:30:49.858Z\",\"views\":1},{\"date\":\"2024-11-26T20:30:49.882Z\",\"views\":0},{\"date\":\"2024-11-23T08:30:49.907Z\",\"views\":0},{\"date\":\"2024-11-19T20:30:49.932Z\",\"views\":0},{\"date\":\"2024-11-16T08:30:49.957Z\",\"views\":0},{\"date\":\"2024-11-12T20:30:49.981Z\",\"views\":1},{\"date\":\"2024-11-09T08:30:50.005Z\",\"views\":2},{\"date\":\"2024-11-05T20:30:50.033Z\",\"views\":2},{\"date\":\"2024-11-02T08:30:50.057Z\",\"views\":0},{\"date\":\"2024-10-29T20:30:50.082Z\",\"views\":2},{\"date\":\"2024-10-26T08:30:50.106Z\",\"views\":2},{\"date\":\"2024-10-22T20:30:50.129Z\",\"views\":2},{\"date\":\"2024-10-19T08:30:50.154Z\",\"views\":0},{\"date\":\"2024-10-15T20:30:50.178Z\",\"views\":0},{\"date\":\"2024-10-12T08:30:50.201Z\",\"views\":2},{\"date\":\"2024-10-08T20:30:50.226Z\",\"views\":1},{\"date\":\"2024-10-05T08:30:50.251Z\",\"views\":1},{\"date\":\"2024-10-01T20:30:50.274Z\",\"views\":2},{\"date\":\"2024-09-28T08:30:50.301Z\",\"views\":0},{\"date\":\"2024-09-24T20:30:50.325Z\",\"views\":2}],\"weighted_visits\":{\"last24Hours\":1.1921963780825085e-129,\"last7Days\":1.1467180654256455e-18,\"last30Days\":0.0003024796605555725,\"last90Days\":0.22164922948997803,\"hot\":1.1467180654256455e-18}},\"is_hidden\":false,\"first_publication_date\":\"2023-03-23T14:52:48.000Z\",\"organizations\":[\"67be637faa92218ccd8b12da\"],\"paperVersions\":{\"_id\":\"67e2bc363a581fde71a4822c\",\"paper_group_id\":\"67e2bc363a581fde71a4822b\",\"version_label\":\"v5\",\"version_order\":5,\"title\":\"Modeling Migration-Induced Unemployment\",\"abstract\":\"$45\",\"author_ids\":[\"6732160ccd1e32a6e7efbbce\"],\"publication_date\":\"2024-12-10T16:30:23.000Z\",\"license\":\"http://creativecommons.org/licenses/by/4.0/\",\"created_at\":\"2025-03-25T14:22:46.065Z\",\"updated_at\":\"2025-03-25T14:22:46.065Z\",\"is_deleted\":false,\"is_hidden\":false,\"universal_paper_id\":\"2303.13319\",\"imageURL\":\"image/2303.13319v5.png\"},\"verifiedAuthors\":[],\"authors\":[{\"_id\":\"6732160ccd1e32a6e7efbbce\",\"full_name\":\"Pascal Michaillat\",\"affiliation\":null,\"orcid\":null,\"semantic_scholarid\":null,\"user_id\":null}]},\"max_version_order\":5,\"verified_authors\":[],\"authors\":[{\"_id\":\"6732160ccd1e32a6e7efbbce\",\"full_name\":\"Pascal Michaillat\",\"affiliation\":null,\"orcid\":null,\"semantic_scholarid\":null,\"user_id\":null}],\"pdf_info\":{\"fetcher_url\":\"https://fetcher.alphaxiv.org/v2/pdf/2303.13319v5\"}}},\"dataUpdateCount\":1,\"dataUpdatedAt\":1744228192003,\"error\":null,\"errorUpdateCount\":0,\"errorUpdatedAt\":0,\"fetchFailureCount\":0,\"fetchFailureReason\":null,\"fetchMeta\":null,\"isInvalidated\":false,\"status\":\"success\",\"fetchStatus\":\"idle\"},\"queryKey\":[\"paper\",\"2303.13319\",\"metadata\"],\"queryHash\":\"[\\\"paper\\\",\\\"2303.13319\\\",\\\"metadata\\\"]\"},{\"state\":{\"data\":{\"data\":[]},\"dataUpdateCount\":1,\"dataUpdatedAt\":1744228192003,\"error\":null,\"errorUpdateCount\":0,\"errorUpdatedAt\":0,\"fetchFailureCount\":0,\"fetchFailureReason\":null,\"fetchMeta\":null,\"isInvalidated\":false,\"status\":\"success\",\"fetchStatus\":\"idle\"},\"queryKey\":[\"paper\",\"2303.13319\",\"comments\"],\"queryHash\":\"[\\\"paper\\\",\\\"2303.13319\\\",\\\"comments\\\"]\"},{\"state\":{\"data\":{\"data\":{\"paper_version\":{\"_id\":\"676fee0290f035bff4879c5e\",\"paper_group_id\":\"676fee0090f035bff4879c59\",\"version_label\":\"v1\",\"version_order\":1,\"title\":\"Accelerating radio astronomy imaging with RICK\",\"abstract\":\"$46\",\"author_ids\":[\"676fee0090f035bff4879c5a\",\"676fee0190f035bff4879c5b\",\"676fee0190f035bff4879c5c\",\"676fee0190f035bff4879c5d\",\"673cd4478a52218f8bc98383\"],\"publication_date\":\"2024-11-11T19:30:53.000Z\",\"license\":\"http://arxiv.org/licenses/nonexclusive-distrib/1.0/\",\"created_at\":\"2024-12-28T12:24:34.078Z\",\"updated_at\":\"2024-12-28T12:24:34.078Z\",\"is_deleted\":false,\"is_hidden\":false,\"universal_paper_id\":\"2411.07321\",\"imageURL\":\"image/2411.07321v1.png\"},\"paper_group\":{\"_id\":\"676fee0090f035bff4879c59\",\"universal_paper_id\":\"2411.07321\",\"title\":\"Accelerating radio astronomy imaging with RICK\",\"created_at\":\"2024-12-28T12:24:32.676Z\",\"updated_at\":\"2025-03-03T19:41:11.311Z\",\"categories\":[\"Physics\"],\"subcategories\":[\"astro-ph.IM\"],\"custom_categories\":null,\"author_user_ids\":[],\"source\":{\"name\":\"alphaXiv\",\"url\":\"https://arxiv.org/paper/2411.07321\"},\"metrics\":{\"activity_rank\":0,\"questions_count\":0,\"responses_count\":0,\"upvotes_count\":0,\"downvotes_count\":0,\"total_votes\":0,\"visits_count\":{\"last24Hours\":1,\"last7Days\":3,\"last30Days\":5,\"last90Days\":8,\"all\":37},\"weighted_visits\":{\"last24Hours\":1.5381221477819755e-25,\"last7Days\":0.000855865772164575,\"last30Days\":0.7445085653164918,\"last90Days\":4.24023459542895,\"hot\":0.000855865772164575},\"public_total_votes\":2,\"timeline\":[{\"date\":\"2025-04-02T23:57:10.993Z\",\"views\":7},{\"date\":\"2025-03-30T11:57:10.993Z\",\"views\":4},{\"date\":\"2025-03-26T23:57:10.993Z\",\"views\":5},{\"date\":\"2025-03-23T11:57:10.993Z\",\"views\":3},{\"date\":\"2025-03-19T23:57:10.993Z\",\"views\":2},{\"date\":\"2025-03-16T11:57:10.993Z\",\"views\":2},{\"date\":\"2025-03-12T23:57:10.993Z\",\"views\":1},{\"date\":\"2025-03-09T11:57:10.993Z\",\"views\":0},{\"date\":\"2025-03-05T23:57:10.993Z\",\"views\":1},{\"date\":\"2025-03-02T11:57:10.993Z\",\"views\":2},{\"date\":\"2025-02-26T23:57:10.993Z\",\"views\":4},{\"date\":\"2025-02-23T11:57:10.993Z\",\"views\":2},{\"date\":\"2025-02-19T23:57:11.022Z\",\"views\":2},{\"date\":\"2025-02-16T11:57:11.058Z\",\"views\":1},{\"date\":\"2025-02-12T23:57:11.082Z\",\"views\":1},{\"date\":\"2025-02-09T11:57:11.125Z\",\"views\":2},{\"date\":\"2025-02-05T23:57:11.145Z\",\"views\":2},{\"date\":\"2025-02-02T11:57:11.200Z\",\"views\":2},{\"date\":\"2025-01-29T23:57:11.230Z\",\"views\":1},{\"date\":\"2025-01-26T11:57:11.262Z\",\"views\":0},{\"date\":\"2025-01-22T23:57:11.282Z\",\"views\":6},{\"date\":\"2025-01-19T11:57:11.314Z\",\"views\":0},{\"date\":\"2025-01-15T23:57:11.349Z\",\"views\":1},{\"date\":\"2025-01-12T11:57:11.379Z\",\"views\":0},{\"date\":\"2025-01-08T23:57:11.470Z\",\"views\":0},{\"date\":\"2025-01-05T11:57:11.489Z\",\"views\":1},{\"date\":\"2025-01-01T23:57:11.529Z\",\"views\":0},{\"date\":\"2024-12-29T11:57:11.574Z\",\"views\":4},{\"date\":\"2024-12-25T23:57:11.614Z\",\"views\":10},{\"date\":\"2024-12-22T11:57:11.648Z\",\"views\":0},{\"date\":\"2024-12-18T23:57:11.674Z\",\"views\":2},{\"date\":\"2024-12-15T11:57:11.695Z\",\"views\":0},{\"date\":\"2024-12-11T23:57:11.751Z\",\"views\":1},{\"date\":\"2024-12-08T11:57:11.775Z\",\"views\":2},{\"date\":\"2024-12-04T23:57:11.797Z\",\"views\":1},{\"date\":\"2024-12-01T11:57:11.821Z\",\"views\":2},{\"date\":\"2024-11-27T23:57:11.848Z\",\"views\":0},{\"date\":\"2024-11-24T11:57:11.874Z\",\"views\":2},{\"date\":\"2024-11-20T23:57:11.892Z\",\"views\":0},{\"date\":\"2024-11-17T11:57:11.915Z\",\"views\":2},{\"date\":\"2024-11-13T23:57:11.969Z\",\"views\":1},{\"date\":\"2024-11-10T11:57:12.003Z\",\"views\":2}]},\"is_hidden\":false,\"first_publication_date\":\"2024-11-11T19:30:53.000Z\",\"organizations\":[\"67be6395aa92218ccd8b18b6\",\"67c2eb866238d4c4ef21134f\",\"67c2eb866238d4c4ef211350\",\"67be63bbaa92218ccd8b207a\"],\"citation\":{\"bibtex\":\"@misc{taffoni2024acceleratingradioastronomy,\\n title={Accelerating radio astronomy imaging with RICK}, \\n author={Giuliano Taffoni and Emanuele De Rubeis and Giovanni Lacopo and Claudio Gheller and Luca Tornatore},\\n year={2024},\\n eprint={2411.07321},\\n archivePrefix={arXiv},\\n primaryClass={astro-ph.IM},\\n url={https://arxiv.org/abs/2411.07321}, \\n}\"},\"paperVersions\":{\"_id\":\"676fee0290f035bff4879c5e\",\"paper_group_id\":\"676fee0090f035bff4879c59\",\"version_label\":\"v1\",\"version_order\":1,\"title\":\"Accelerating radio astronomy imaging with RICK\",\"abstract\":\"$47\",\"author_ids\":[\"676fee0090f035bff4879c5a\",\"676fee0190f035bff4879c5b\",\"676fee0190f035bff4879c5c\",\"676fee0190f035bff4879c5d\",\"673cd4478a52218f8bc98383\"],\"publication_date\":\"2024-11-11T19:30:53.000Z\",\"license\":\"http://arxiv.org/licenses/nonexclusive-distrib/1.0/\",\"created_at\":\"2024-12-28T12:24:34.078Z\",\"updated_at\":\"2024-12-28T12:24:34.078Z\",\"is_deleted\":false,\"is_hidden\":false,\"universal_paper_id\":\"2411.07321\",\"imageURL\":\"image/2411.07321v1.png\"},\"verifiedAuthors\":[],\"authors\":[{\"_id\":\"673cd4478a52218f8bc98383\",\"full_name\":\"Giuliano Taffoni\",\"affiliation\":null,\"orcid\":null,\"semantic_scholarid\":null,\"user_id\":null},{\"_id\":\"676fee0090f035bff4879c5a\",\"full_name\":\"Emanuele De Rubeis\",\"affiliation\":null,\"orcid\":null,\"semantic_scholarid\":null,\"user_id\":null},{\"_id\":\"676fee0190f035bff4879c5b\",\"full_name\":\"Giovanni Lacopo\",\"affiliation\":null,\"orcid\":null,\"semantic_scholarid\":null,\"user_id\":null},{\"_id\":\"676fee0190f035bff4879c5c\",\"full_name\":\"Claudio Gheller\",\"affiliation\":null,\"orcid\":null,\"semantic_scholarid\":null,\"user_id\":null},{\"_id\":\"676fee0190f035bff4879c5d\",\"full_name\":\"Luca Tornatore\",\"affiliation\":null,\"orcid\":null,\"semantic_scholarid\":null,\"user_id\":null}]},\"max_version_order\":1,\"verified_authors\":[],\"authors\":[{\"_id\":\"673cd4478a52218f8bc98383\",\"full_name\":\"Giuliano Taffoni\",\"affiliation\":null,\"orcid\":null,\"semantic_scholarid\":null,\"user_id\":null},{\"_id\":\"676fee0090f035bff4879c5a\",\"full_name\":\"Emanuele De Rubeis\",\"affiliation\":null,\"orcid\":null,\"semantic_scholarid\":null,\"user_id\":null},{\"_id\":\"676fee0190f035bff4879c5b\",\"full_name\":\"Giovanni Lacopo\",\"affiliation\":null,\"orcid\":null,\"semantic_scholarid\":null,\"user_id\":null},{\"_id\":\"676fee0190f035bff4879c5c\",\"full_name\":\"Claudio Gheller\",\"affiliation\":null,\"orcid\":null,\"semantic_scholarid\":null,\"user_id\":null},{\"_id\":\"676fee0190f035bff4879c5d\",\"full_name\":\"Luca Tornatore\",\"affiliation\":null,\"orcid\":null,\"semantic_scholarid\":null,\"user_id\":null}],\"pdf_info\":{\"fetcher_url\":\"https://fetcher.alphaxiv.org/v2/pdf/2411.07321v1\"}}},\"dataUpdateCount\":1,\"dataUpdatedAt\":1744228196703,\"error\":null,\"errorUpdateCount\":0,\"errorUpdatedAt\":0,\"fetchFailureCount\":0,\"fetchFailureReason\":null,\"fetchMeta\":null,\"isInvalidated\":false,\"status\":\"success\",\"fetchStatus\":\"idle\"},\"queryKey\":[\"paper\",\"2411.07321\",\"metadata\"],\"queryHash\":\"[\\\"paper\\\",\\\"2411.07321\\\",\\\"metadata\\\"]\"},{\"state\":{\"data\":{\"data\":[]},\"dataUpdateCount\":1,\"dataUpdatedAt\":1744228196703,\"error\":null,\"errorUpdateCount\":0,\"errorUpdatedAt\":0,\"fetchFailureCount\":0,\"fetchFailureReason\":null,\"fetchMeta\":null,\"isInvalidated\":false,\"status\":\"success\",\"fetchStatus\":\"idle\"},\"queryKey\":[\"paper\",\"2411.07321\",\"comments\"],\"queryHash\":\"[\\\"paper\\\",\\\"2411.07321\\\",\\\"comments\\\"]\"},{\"state\":{\"data\":{\"data\":{\"paper_version\":{\"_id\":\"67332e41c48bba476d788d0c\",\"paper_group_id\":\"67332e3fc48bba476d788d0a\",\"version_label\":\"v3\",\"version_order\":3,\"title\":\"MVSFormer: Multi-View Stereo by Learning Robust Image Features and\\n Temperature-based Depth\",\"abstract\":\"$48\",\"author_ids\":[\"672bd2b0986a1370676e30d8\",\"67332e40c48bba476d788d0b\",\"672bcfa3986a1370676df096\"],\"publication_date\":\"2022-12-16T13:42:24.000Z\",\"license\":\"http://creativecommons.org/licenses/by/4.0/\",\"created_at\":\"2024-11-12T10:30:25.306Z\",\"updated_at\":\"2024-11-12T10:30:25.306Z\",\"is_deleted\":false,\"is_hidden\":false,\"universal_paper_id\":\"2208.02541\",\"imageURL\":\"image/2208.02541v3.png\"},\"paper_group\":{\"_id\":\"67332e3fc48bba476d788d0a\",\"universal_paper_id\":\"2208.02541\",\"source\":{\"name\":\"alphaXiv\",\"url\":\"https://alphaxiv.org/paper/2208.02541\"},\"title\":\"MVSFormer: Multi-View Stereo by Learning Robust Image Features and\\n Temperature-based Depth\",\"created_at\":\"1970-01-01T00:00:00.000Z\",\"updated_at\":\"2025-03-03T20:23:08.836Z\",\"categories\":[\"Computer Science\"],\"subcategories\":[\"cs.CV\"],\"metrics\":{\"activity_rank\":0,\"questions_count\":0,\"responses_count\":0,\"upvotes_count\":null,\"downvotes_count\":0,\"total_votes\":0,\"visits_count\":{\"last24Hours\":2,\"last7Days\":2,\"last30Days\":7,\"last90Days\":29,\"all\":90},\"weighted_visits\":{\"last24Hours\":3.286402322674748e-146,\"last7Days\":2.9833217914904206e-21,\"last30Days\":0.0000967433371959472,\"last90Days\":0.6959408662295113,\"hot\":2.9833217914904206e-21},\"public_total_votes\":3,\"timeline\":[{\"date\":\"2025-04-03T02:11:34.241Z\",\"views\":2},{\"date\":\"2025-03-30T14:11:34.241Z\",\"views\":2},{\"date\":\"2025-03-27T02:11:34.241Z\",\"views\":3},{\"date\":\"2025-03-23T14:11:34.241Z\",\"views\":5},{\"date\":\"2025-03-20T02:11:34.241Z\",\"views\":1},{\"date\":\"2025-03-16T14:11:34.241Z\",\"views\":1},{\"date\":\"2025-03-13T02:11:34.241Z\",\"views\":0},{\"date\":\"2025-03-09T14:11:34.241Z\",\"views\":8},{\"date\":\"2025-03-06T02:11:34.241Z\",\"views\":3},{\"date\":\"2025-03-02T14:11:34.241Z\",\"views\":24},{\"date\":\"2025-02-27T02:11:34.241Z\",\"views\":6},{\"date\":\"2025-02-23T14:11:34.241Z\",\"views\":8},{\"date\":\"2025-02-20T02:11:34.261Z\",\"views\":1},{\"date\":\"2025-02-16T14:11:34.281Z\",\"views\":7},{\"date\":\"2025-02-13T02:11:34.303Z\",\"views\":2},{\"date\":\"2025-02-09T14:11:34.325Z\",\"views\":0},{\"date\":\"2025-02-06T02:11:34.355Z\",\"views\":0},{\"date\":\"2025-02-02T14:11:34.404Z\",\"views\":2},{\"date\":\"2025-01-30T02:11:34.428Z\",\"views\":0},{\"date\":\"2025-01-26T14:11:34.449Z\",\"views\":11},{\"date\":\"2025-01-23T02:11:34.470Z\",\"views\":5},{\"date\":\"2025-01-19T14:11:34.497Z\",\"views\":3},{\"date\":\"2025-01-16T02:11:34.522Z\",\"views\":7},{\"date\":\"2025-01-12T14:11:34.540Z\",\"views\":0},{\"date\":\"2025-01-09T02:11:34.561Z\",\"views\":4},{\"date\":\"2025-01-05T14:11:34.581Z\",\"views\":1},{\"date\":\"2025-01-02T02:11:34.609Z\",\"views\":0},{\"date\":\"2024-12-29T14:11:34.633Z\",\"views\":0},{\"date\":\"2024-12-26T02:11:34.656Z\",\"views\":1},{\"date\":\"2024-12-22T14:11:34.683Z\",\"views\":0},{\"date\":\"2024-12-19T02:11:34.708Z\",\"views\":2},{\"date\":\"2024-12-15T14:11:34.735Z\",\"views\":1},{\"date\":\"2024-12-12T02:11:34.760Z\",\"views\":0},{\"date\":\"2024-12-08T14:11:34.784Z\",\"views\":2},{\"date\":\"2024-12-05T02:11:34.803Z\",\"views\":3},{\"date\":\"2024-12-01T14:11:34.829Z\",\"views\":2},{\"date\":\"2024-11-28T02:11:34.864Z\",\"views\":2},{\"date\":\"2024-11-24T14:11:34.891Z\",\"views\":1},{\"date\":\"2024-11-21T02:11:34.917Z\",\"views\":2},{\"date\":\"2024-11-17T14:11:34.949Z\",\"views\":2},{\"date\":\"2024-11-14T02:11:34.975Z\",\"views\":0},{\"date\":\"2024-11-10T14:11:34.993Z\",\"views\":0},{\"date\":\"2024-11-07T02:11:35.017Z\",\"views\":1},{\"date\":\"2024-11-03T14:11:35.045Z\",\"views\":0},{\"date\":\"2024-10-31T01:11:35.069Z\",\"views\":1},{\"date\":\"2024-10-27T13:11:35.099Z\",\"views\":2},{\"date\":\"2024-10-24T01:11:35.125Z\",\"views\":2},{\"date\":\"2024-10-20T13:11:35.146Z\",\"views\":2},{\"date\":\"2024-10-17T01:11:35.166Z\",\"views\":2},{\"date\":\"2024-10-13T13:11:35.193Z\",\"views\":1},{\"date\":\"2024-10-10T01:11:35.221Z\",\"views\":0},{\"date\":\"2024-10-06T13:11:35.249Z\",\"views\":0},{\"date\":\"2024-10-03T01:11:35.272Z\",\"views\":2},{\"date\":\"2024-09-29T13:11:35.292Z\",\"views\":2},{\"date\":\"2024-09-26T01:11:35.314Z\",\"views\":1},{\"date\":\"2024-09-22T13:11:35.337Z\",\"views\":1},{\"date\":\"2024-09-19T01:11:35.358Z\",\"views\":2},{\"date\":\"2024-09-15T13:11:35.380Z\",\"views\":1},{\"date\":\"2024-09-12T01:11:35.399Z\",\"views\":2},{\"date\":\"2024-09-08T13:11:35.419Z\",\"views\":2},{\"date\":\"2024-09-05T01:11:35.442Z\",\"views\":1},{\"date\":\"2024-09-01T13:11:35.499Z\",\"views\":2},{\"date\":\"2024-08-29T01:11:35.508Z\",\"views\":2}]},\"ranking\":{\"current_rank\":50477,\"previous_rank\":54913,\"activity_score\":0,\"paper_score\":0},\"is_hidden\":false,\"custom_categories\":[\"computer-vision-security\",\"transformers\",\"representation-learning\",\"self-supervised-learning\",\"vision-language-models\"],\"first_publication_date\":\"2022-12-16T13:42:24.000Z\",\"author_user_ids\":[],\"citation\":{\"bibtex\":\"@Article{Cao2022MVSFormerMS,\\n author = {Chenjie Cao and Xinlin Ren and Yanwei Fu},\\n booktitle = {Trans. Mach. Learn. Res.},\\n journal = {Trans. Mach. Learn. Res.},\\n title = {MVSFormer: Multi-View Stereo by Learning Robust Image Features and Temperature-based Depth},\\n volume = {2022},\\n year = {2022}\\n}\\n\"},\"resources\":{\"github\":{\"url\":\"https://github.com/ewrfcas/MVSFormer\",\"description\":\"Codes of MVSFormer: Multi-View Stereo by Learning Robust Image Features and Temperature-based Depth (TMLR2023)\",\"language\":\"Python\",\"stars\":193}},\"paperVersions\":{\"_id\":\"67332e41c48bba476d788d0c\",\"paper_group_id\":\"67332e3fc48bba476d788d0a\",\"version_label\":\"v3\",\"version_order\":3,\"title\":\"MVSFormer: Multi-View Stereo by Learning Robust Image Features and\\n Temperature-based Depth\",\"abstract\":\"$49\",\"author_ids\":[\"672bd2b0986a1370676e30d8\",\"67332e40c48bba476d788d0b\",\"672bcfa3986a1370676df096\"],\"publication_date\":\"2022-12-16T13:42:24.000Z\",\"license\":\"http://creativecommons.org/licenses/by/4.0/\",\"created_at\":\"2024-11-12T10:30:25.306Z\",\"updated_at\":\"2024-11-12T10:30:25.306Z\",\"is_deleted\":false,\"is_hidden\":false,\"universal_paper_id\":\"2208.02541\",\"imageURL\":\"image/2208.02541v3.png\"},\"verifiedAuthors\":[],\"authors\":[{\"_id\":\"672bcfa3986a1370676df096\",\"full_name\":\"Yanwei Fu\",\"affiliation\":null,\"orcid\":null,\"semantic_scholarid\":null,\"user_id\":null},{\"_id\":\"672bd2b0986a1370676e30d8\",\"full_name\":\"Chenjie Cao\",\"affiliation\":null,\"orcid\":null,\"semantic_scholarid\":null,\"user_id\":null},{\"_id\":\"67332e40c48bba476d788d0b\",\"full_name\":\"Xinlin Ren\",\"affiliation\":null,\"orcid\":null,\"semantic_scholarid\":null,\"user_id\":null}]},\"max_version_order\":3,\"verified_authors\":[],\"authors\":[{\"_id\":\"672bcfa3986a1370676df096\",\"full_name\":\"Yanwei Fu\",\"affiliation\":null,\"orcid\":null,\"semantic_scholarid\":null,\"user_id\":null},{\"_id\":\"672bd2b0986a1370676e30d8\",\"full_name\":\"Chenjie Cao\",\"affiliation\":null,\"orcid\":null,\"semantic_scholarid\":null,\"user_id\":null},{\"_id\":\"67332e40c48bba476d788d0b\",\"full_name\":\"Xinlin Ren\",\"affiliation\":null,\"orcid\":null,\"semantic_scholarid\":null,\"user_id\":null}],\"pdf_info\":{\"fetcher_url\":\"https://fetcher.alphaxiv.org/v2/pdf/2208.02541v3\"}}},\"dataUpdateCount\":1,\"dataUpdatedAt\":1744228196865,\"error\":null,\"errorUpdateCount\":0,\"errorUpdatedAt\":0,\"fetchFailureCount\":0,\"fetchFailureReason\":null,\"fetchMeta\":null,\"isInvalidated\":false,\"status\":\"success\",\"fetchStatus\":\"idle\"},\"queryKey\":[\"paper\",\"2208.02541\",\"metadata\"],\"queryHash\":\"[\\\"paper\\\",\\\"2208.02541\\\",\\\"metadata\\\"]\"},{\"state\":{\"data\":{\"data\":[]},\"dataUpdateCount\":1,\"dataUpdatedAt\":1744228196865,\"error\":null,\"errorUpdateCount\":0,\"errorUpdatedAt\":0,\"fetchFailureCount\":0,\"fetchFailureReason\":null,\"fetchMeta\":null,\"isInvalidated\":false,\"status\":\"success\",\"fetchStatus\":\"idle\"},\"queryKey\":[\"paper\",\"2208.02541\",\"comments\"],\"queryHash\":\"[\\\"paper\\\",\\\"2208.02541\\\",\\\"comments\\\"]\"},{\"state\":{\"data\":{\"data\":{\"paper_version\":{\"_id\":\"673baba7bf626fe16b8aca7b\",\"paper_group_id\":\"673baba7bf626fe16b8aca79\",\"version_label\":\"v2\",\"version_order\":2,\"title\":\"Classifying high-dimensional Gaussian mixtures: Where kernel methods fail and neural networks succeed\",\"abstract\":\"$4a\",\"author_ids\":[\"672bd17c986a1370676e1825\",\"672bd17d986a1370676e183b\",\"672bce19986a1370676dd2f2\",\"672bce19986a1370676dd2fa\"],\"publication_date\":\"2021-06-10T16:24:03.000Z\",\"license\":\"http://arxiv.org/licenses/nonexclusive-distrib/1.0/\",\"created_at\":\"2024-11-18T21:03:35.344Z\",\"updated_at\":\"2024-11-18T21:03:35.344Z\",\"is_deleted\":false,\"is_hidden\":false,\"universal_paper_id\":\"2102.11742\",\"imageURL\":\"image/2102.11742v2.png\"},\"paper_group\":{\"_id\":\"673baba7bf626fe16b8aca79\",\"universal_paper_id\":\"2102.11742\",\"source\":{\"name\":\"arXiv\",\"url\":\"https://arXiv.org/paper/2102.11742\"},\"title\":\"Classifying high-dimensional Gaussian mixtures: Where kernel methods fail and neural networks succeed\",\"created_at\":\"1970-01-01T00:00:00.000Z\",\"updated_at\":\"2025-03-03T20:44:32.233Z\",\"categories\":[\"Computer Science\",\"Physics\",\"Statistics\"],\"subcategories\":[\"cs.LG\",\"cond-mat.dis-nn\",\"cond-mat.stat-mech\",\"stat.ML\"],\"metrics\":{\"activity_rank\":0,\"questions_count\":0,\"responses_count\":0,\"upvotes_count\":null,\"downvotes_count\":0,\"total_votes\":0,\"visits_count\":{\"last24Hours\":0,\"last7Days\":1,\"last30Days\":2,\"last90Days\":7,\"all\":25},\"weighted_visits\":{\"last24Hours\":0,\"last7Days\":2.6729707655727113e-35,\"last30Days\":1.7139388025907975e-8,\"last90Days\":0.014324731107453813,\"hot\":2.6729707655727113e-35},\"public_total_votes\":0,\"timeline\":[{\"date\":\"2025-04-03T02:53:12.479Z\",\"views\":5},{\"date\":\"2025-03-30T14:53:12.479Z\",\"views\":0},{\"date\":\"2025-03-27T02:53:12.479Z\",\"views\":3},{\"date\":\"2025-03-23T14:53:12.479Z\",\"views\":0},{\"date\":\"2025-03-20T02:53:12.479Z\",\"views\":1},{\"date\":\"2025-03-16T14:53:12.479Z\",\"views\":2},{\"date\":\"2025-03-13T02:53:12.479Z\",\"views\":2},{\"date\":\"2025-03-09T14:53:12.479Z\",\"views\":0},{\"date\":\"2025-03-06T02:53:12.479Z\",\"views\":1},{\"date\":\"2025-03-02T14:53:12.479Z\",\"views\":2},{\"date\":\"2025-02-27T02:53:12.479Z\",\"views\":2},{\"date\":\"2025-02-23T14:53:12.479Z\",\"views\":1},{\"date\":\"2025-02-20T02:53:12.493Z\",\"views\":0},{\"date\":\"2025-02-16T14:53:12.521Z\",\"views\":1},{\"date\":\"2025-02-13T02:53:12.554Z\",\"views\":0},{\"date\":\"2025-02-09T14:53:12.577Z\",\"views\":6},{\"date\":\"2025-02-06T02:53:12.599Z\",\"views\":1},{\"date\":\"2025-02-02T14:53:12.630Z\",\"views\":1},{\"date\":\"2025-01-30T02:53:12.653Z\",\"views\":1},{\"date\":\"2025-01-26T14:53:12.675Z\",\"views\":1},{\"date\":\"2025-01-23T02:53:12.700Z\",\"views\":5},{\"date\":\"2025-01-19T14:53:12.724Z\",\"views\":0},{\"date\":\"2025-01-16T02:53:12.749Z\",\"views\":0},{\"date\":\"2025-01-12T14:53:12.771Z\",\"views\":6},{\"date\":\"2025-01-09T02:53:12.794Z\",\"views\":2},{\"date\":\"2025-01-05T14:53:12.813Z\",\"views\":2},{\"date\":\"2025-01-02T02:53:12.834Z\",\"views\":2},{\"date\":\"2024-12-29T14:53:12.898Z\",\"views\":1},{\"date\":\"2024-12-26T02:53:12.923Z\",\"views\":1},{\"date\":\"2024-12-22T14:53:12.947Z\",\"views\":2},{\"date\":\"2024-12-19T02:53:12.968Z\",\"views\":0},{\"date\":\"2024-12-15T14:53:12.990Z\",\"views\":0},{\"date\":\"2024-12-12T02:53:13.021Z\",\"views\":1},{\"date\":\"2024-12-08T14:53:13.045Z\",\"views\":1},{\"date\":\"2024-12-05T02:53:13.065Z\",\"views\":2},{\"date\":\"2024-12-01T14:53:13.086Z\",\"views\":0},{\"date\":\"2024-11-28T02:53:13.114Z\",\"views\":2},{\"date\":\"2024-11-24T14:53:13.136Z\",\"views\":2},{\"date\":\"2024-11-21T02:53:13.158Z\",\"views\":2},{\"date\":\"2024-11-17T14:53:13.180Z\",\"views\":2},{\"date\":\"2024-11-14T02:53:13.204Z\",\"views\":1},{\"date\":\"2024-11-10T14:53:13.225Z\",\"views\":1},{\"date\":\"2024-11-07T02:53:13.252Z\",\"views\":1},{\"date\":\"2024-11-03T14:53:13.274Z\",\"views\":2},{\"date\":\"2024-10-31T01:53:13.296Z\",\"views\":5},{\"date\":\"2024-10-27T13:53:13.322Z\",\"views\":1},{\"date\":\"2024-10-24T01:53:13.344Z\",\"views\":0},{\"date\":\"2024-10-20T13:53:13.369Z\",\"views\":0},{\"date\":\"2024-10-17T01:53:13.391Z\",\"views\":0},{\"date\":\"2024-10-13T13:53:13.421Z\",\"views\":1},{\"date\":\"2024-10-10T01:53:13.451Z\",\"views\":1},{\"date\":\"2024-10-06T13:53:13.479Z\",\"views\":0},{\"date\":\"2024-10-03T01:53:13.533Z\",\"views\":0},{\"date\":\"2024-09-29T13:53:13.574Z\",\"views\":1},{\"date\":\"2024-09-26T01:53:13.604Z\",\"views\":0},{\"date\":\"2024-09-22T13:53:13.700Z\",\"views\":0},{\"date\":\"2024-09-19T01:53:13.723Z\",\"views\":2},{\"date\":\"2024-09-15T13:53:13.745Z\",\"views\":2},{\"date\":\"2024-09-12T01:53:13.766Z\",\"views\":0},{\"date\":\"2024-09-08T13:53:13.839Z\",\"views\":2},{\"date\":\"2024-09-05T01:53:13.859Z\",\"views\":1},{\"date\":\"2024-09-01T13:53:13.886Z\",\"views\":0},{\"date\":\"2024-08-29T01:53:13.904Z\",\"views\":0}]},\"ranking\":{\"current_rank\":69518,\"previous_rank\":72388,\"activity_score\":0,\"paper_score\":0},\"is_hidden\":false,\"custom_categories\":[\"neural-architecture-search\",\"statistical-learning\",\"optimization-methods\",\"representation-learning\"],\"first_publication_date\":\"2021-06-10T16:24:03.000Z\",\"author_user_ids\":[\"66aa969c94a3f2f6c93dcaef\"],\"resources\":{\"github\":{\"url\":\"https://github.com/ArdaS2012/kernel_nn_gaussian\",\"description\":\"Reproducing paper from Maria Refinetti, Sebastian Goldt, Florent Krzakala and Lenka Zdeborová with the title: \\\"Classifying high-dimensional Gaussian mixtures: Where kernel methods fail and neural networks succeed\\\". Also introducing possible extensions for this paper.\",\"language\":\"Python\",\"stars\":0}},\"citation\":{\"bibtex\":\"@misc{krzakala2021classifyinghighdimensionalgaussian,\\n title={Classifying high-dimensional Gaussian mixtures: Where kernel methods fail and neural networks succeed}, \\n author={Florent Krzakala and Lenka Zdeborová and Maria Refinetti and Sebastian Goldt},\\n year={2021},\\n eprint={2102.11742},\\n archivePrefix={arXiv},\\n primaryClass={cs.LG},\\n url={https://arxiv.org/abs/2102.11742}, \\n}\"},\"paperVersions\":{\"_id\":\"673baba7bf626fe16b8aca7b\",\"paper_group_id\":\"673baba7bf626fe16b8aca79\",\"version_label\":\"v2\",\"version_order\":2,\"title\":\"Classifying high-dimensional Gaussian mixtures: Where kernel methods fail and neural networks succeed\",\"abstract\":\"$4b\",\"author_ids\":[\"672bd17c986a1370676e1825\",\"672bd17d986a1370676e183b\",\"672bce19986a1370676dd2f2\",\"672bce19986a1370676dd2fa\"],\"publication_date\":\"2021-06-10T16:24:03.000Z\",\"license\":\"http://arxiv.org/licenses/nonexclusive-distrib/1.0/\",\"created_at\":\"2024-11-18T21:03:35.344Z\",\"updated_at\":\"2024-11-18T21:03:35.344Z\",\"is_deleted\":false,\"is_hidden\":false,\"universal_paper_id\":\"2102.11742\",\"imageURL\":\"image/2102.11742v2.png\"},\"verifiedAuthors\":[{\"_id\":\"66aa969c94a3f2f6c93dcaef\",\"useremail\":\"florent.krzakala@gmail.com\",\"username\":\"Florent KRZAKALA\",\"realname\":\"Florent KRZAKALA\",\"totalupvotes\":0,\"numquestions\":0,\"numresponses\":0,\"papers\":[],\"activity\":[],\"following\":[],\"followers\":[],\"followingPapers\":[\"1109.3041v2\",\"1511.02476v5\",\"1109.4424v4\",\"arXiv:1909.11500v4\",\"1708.03395v3\",\"1907.00657v2\",\"1701.00858v3\",\"0901.2130v2\",\"1406.1880v2\",\"1402.1298v3\",\"1510.06664v2\",\"1906.08632v2\",\"2002.09339v2\",\"2006.14709v3\",\"1603.08447v1\",\"1306.5550v2\",\"1701.08010v2\",\"1507.04113v1\",\"cond-mat_0606180v2\",\"1503.08040v4\",\"1506.02914v2\",\"0911.1551v2\",\"1003.2748v1\",\"0807.2553v2\",\"cond-mat_0212070v2\",\"1701.05823v2\",\"1607.02335v2\",\"2006.06098v2\",\"2105.15004v2\",\"1905.12385v2\",\"2102.11742v2\",\"cond-mat_0002055v2\",\"1701.06981v1\",\"1801.01593v2\",\"2006.06560v2\",\"cond-mat_0107366v2\",\"2006.06581v6\",\"1312.1740v5\",\"1806.09588v1\",\"1907.08226v3\",\"1306.4121v2\",\"0910.3008v2\",\"1609.05204v3\",\"2006.05228v2\",\"1211.2379v2\",\"2002.04372v1\",\"1301.5898v1\",\"1610.02918v1\",\"0909.3820v3\",\"1702.03260v3\",\"0805.3509v2\",\"1207.2328v2\",\"0902.4185v3\",\"2106.03791v2\",\"1510.01098v2\",\"2110.08775v3\",\"2102.08127v3\",\"1006.2479v2\",\"2202.00293v4\",\"cond-mat_0403053v3\",\"2006.07310v2\",\"1203.5521v3\",\"2305.18270v3\",\"2302.00375v2\",\"1812.02537v1\",\"2006.06997v1\",\"1404.7787v1\",\"1006.2480v2\",\"1008.4497v1\",\"2210.06591v3\",\"1710.02903v1\",\"1207.2079v1\",\"1203.3166v2\",\"2012.06373v1\",\"1604.02475v2\",\"1506.03498v3\",\"2302.08923v1\",\"2302.08933v1\",\"1301.0901v1\",\"1809.06304v1\",\"1605.07516v1\",\"1302.0189v1\",\"cond-mat_0512309v3\",\"1601.06683v2\",\"2203.07752v1\",\"2308.14085v1\",\"0910.5644v1\",\"1810.13038v1\",\"2402.03220v3\",\"1204.3734v1\",\"2210.12760v4\",\"1101.5863v1\",\"2208.05918v1\",\"1901.09085v1\",\"cond-mat_0211106v2\",\"1906.04735v1\",\"2107.11814v1\",\"1706.00705v1\",\"1605.06422v3\",\"2202.03295v2\",\"cond-mat_0208566v2\",\"2302.05882v1\",\"1307.7846v3\",\"2302.06665v1\",\"2310.14055v1\",\"0709.0894v1\",\"1602.02944v2\",\"2402.04980v2\",\"2310.03575v2\",\"2006.01475v2\",\"1304.6599v2\",\"2303.02644v2\",\"2402.03902v1\",\"0709.1023v1\",\"1609.08269v1\",\"2310.02850v2\",\"cond-mat_0409448v2\",\"2402.13622v1\",\"2201.09986v3\",\"2405.15459v1\",\"2205.13527v2\",\"1511.05860v2\",\"2101.02115v1\",\"2306.09283v1\",\"2405.15480v2\",\"2406.02157v1\",\"2405.04267v2\",\"2205.08782v1\",\"2408.03733v1\",\"2205.13503v2\",\"2403.04234v1\",\"1910.00285v2\",\"2402.05674v2\",\"1104.0921v1\",\"0710.3336v2\",\"1502.03324v1\",\"1205.4200v4\"],\"claimedPapers\":[\"1109.3041v2\",\"1511.02476v5\",\"1206.3953v1\",\"1102.1182v1\",\"1109.4424v4\",\"0806.3665v3\",\"arXiv:1909.11500v4\",\"1210.0811v2\",\"1708.03395v3\",\"1907.00657v2\",\"1701.00858v3\",\"0901.2130v2\",\"1406.1880v2\",\"1402.1298v3\",\"cond-mat_0702546v4\",\"1510.06664v2\",\"1906.08632v2\",\"2002.09339v2\",\"2006.14709v3\",\"1603.08447v1\",\"1306.5550v2\",\"1701.08010v2\",\"1507.04113v1\",\"cond-mat_0606180v2\",\"1503.08040v4\",\"1506.02914v2\",\"0911.1551v2\",\"1003.2748v1\",\"0807.2553v2\",\"2006.12878v2\",\"cond-mat_0212070v2\",\"0909.4256v2\",\"1701.05823v2\",\"1607.02335v2\",\"2006.06098v2\",\"2105.15004v2\",\"1802.08963v2\",\"1905.12385v2\",\"2102.11742v2\",\"cond-mat_0002055v2\",\"1701.06981v1\",\"1801.01593v2\",\"2006.06560v2\",\"cond-mat_0107366v2\",\"2006.06581v6\",\"1312.1740v5\",\"1806.09588v1\",\"1403.8024v2\",\"cond-mat_0203449v2\",\"1907.08226v3\",\"1306.4121v2\",\"0910.3008v2\",\"1609.05204v3\",\"2006.05228v2\",\"1211.2379v2\",\"1906.08479v3\",\"2002.04372v1\",\"1301.5898v1\",\"1610.02918v1\",\"1410.1368v2\",\"0909.3820v3\",\"1702.03260v3\",\"0805.3509v2\",\"1207.2328v2\",\"1912.02008v2\",\"0902.4185v3\",\"1906.04554v1\",\"1812.09066v4\",\"2106.03791v2\",\"1502.06470v3\",\"1510.01098v2\",\"2110.08775v3\",\"2102.08127v3\",\"1006.2479v2\",\"2202.00293v4\",\"cond-mat_0403053v3\",\"1910.09880v2\",\"2006.07310v2\",\"1203.5521v3\",\"2205.13303v2\",\"2305.18270v3\",\"2302.00375v2\",\"1812.02537v1\",\"2201.13383v1\",\"2006.06997v1\",\"1404.7787v1\",\"1807.01296v1\",\"1006.2480v2\",\"1606.03956v1\",\"1008.4497v1\",\"2210.06591v3\",\"1710.02903v1\",\"1207.2079v1\",\"1203.3166v2\",\"2012.06373v1\",\"1604.02475v2\",\"1506.03498v3\",\"2302.08923v1\",\"2302.08933v1\",\"1301.0901v1\",\"1809.06304v1\",\"1605.07516v1\",\"1302.0189v1\",\"cond-mat_0512309v3\",\"1601.06683v2\",\"2203.07752v1\",\"2308.14085v1\",\"0910.5644v1\",\"1810.13038v1\",\"cond-mat_0010010v2\",\"2402.03220v3\",\"1204.3734v1\",\"2210.12760v4\",\"1101.5863v1\",\"2208.05918v1\",\"1901.09085v1\",\"cond-mat_0211106v2\",\"2201.12655v3\",\"2012.04524v3\",\"1906.04735v1\",\"2107.11814v1\",\"1706.00705v1\",\"1605.06422v3\",\"2202.03295v2\",\"cond-mat_0208566v2\",\"2302.05882v1\",\"2305.18502v2\",\"1307.7846v3\",\"2302.06665v1\",\"2310.14055v1\",\"0709.0894v1\",\"1602.02944v2\",\"2402.04980v2\",\"2310.03575v2\",\"2006.01475v2\",\"1304.6599v2\",\"2303.02644v2\",\"2402.03902v1\",\"0709.1023v1\",\"1609.08269v1\",\"2310.02850v2\",\"2105.07416v2\",\"cond-mat_0409448v2\",\"2402.13622v1\",\"2201.09986v3\",\"cond-mat_0409449v3\",\"2405.15459v1\",\"2205.13527v2\",\"1511.05860v2\",\"2101.02115v1\",\"2403.03695v1\",\"2306.09283v1\",\"2405.15480v2\",\"2406.02157v1\",\"2405.04267v2\",\"2305.18974v2\",\"2205.08782v1\",\"2408.03733v1\",\"2205.13503v2\",\"2403.04234v1\",\"1910.00285v2\",\"2402.05674v2\",\"0712.2009v2\",\"1104.0921v1\",\"0710.3336v2\",\"1502.03324v1\",\"1205.4200v4\",\"0806.4144v3\"],\"biography\":\"\",\"lastViewedGroup\":\"public\",\"groups\":[],\"todayQ\":0,\"todayR\":0,\"daysActive\":145,\"upvotesGivenToday\":0,\"downvotesGivenToday\":0,\"lastViewOfFollowingPapers\":\"2024-09-20T14:17:55.359Z\",\"usernameChanged\":false,\"firstLogin\":true,\"subscribedPotw\":true,\"orcid_id\":\"0000-0003-2313-2578\",\"role\":\"user\",\"institution\":null,\"gscholar_id\":\"3jDeUlMAAAAJ\",\"reputation\":15,\"bookmarks\":\"$4c\",\"weeklyReputation\":0,\"email_settings\":{\"direct_notifications\":true,\"relevant_activity\":false},\"interests\":{\"categories\":[\"Computer Science\",\"Physics\",\"Mathematics\",\"Statistics\"],\"subcategories\":[{\"name\":\"cond-mat.dis-nn\",\"score\":89},{\"name\":\"cs.LG\",\"score\":70},{\"name\":\"stat.ML\",\"score\":68},{\"name\":\"cond-mat.stat-mech\",\"score\":57},{\"name\":\"cs.IT\",\"score\":47},{\"name\":\"math.ST\",\"score\":28},{\"name\":\"math.PR\",\"score\":21},{\"name\":\"cs.SI\",\"score\":7},{\"name\":\"cs.ET\",\"score\":6},{\"name\":\"math-ph\",\"score\":6},{\"name\":\"physics.soc-ph\",\"score\":5},{\"name\":\"cs.NE\",\"score\":5},{\"name\":\"cs.CC\",\"score\":5},{\"name\":\"quant-ph\",\"score\":5},{\"name\":\"physics.optics\",\"score\":4},{\"name\":\"eess.SP\",\"score\":4},{\"name\":\"cs.AI\",\"score\":3},{\"name\":\"physics.data-an\",\"score\":3},{\"name\":\"cond-mat.soft\",\"score\":3},{\"name\":\"cs.AR\",\"score\":2},{\"name\":\"eess.IV\",\"score\":2},{\"name\":\"cs.CV\",\"score\":2},{\"name\":\"math.NA\",\"score\":2},{\"name\":\"cs.CR\",\"score\":2},{\"name\":\"cs.DS\",\"score\":1},{\"name\":\"cs.IR\",\"score\":1},{\"name\":\"nlin.CD\",\"score\":1},{\"name\":\"physics.flu-dyn\",\"score\":1},{\"name\":\"cs.DM\",\"score\":1},{\"name\":\"stat.AP\",\"score\":1},{\"name\":\"cs.DC\",\"score\":1},{\"name\":\"q-bio.GN\",\"score\":1},{\"name\":\"q-bio.QM\",\"score\":1},{\"name\":\"nlin.AO\",\"score\":1},{\"name\":\"q-bio.NC\",\"score\":1}],\"custom_categories\":[{\"name\":\"statistical-learning\",\"score\":56},{\"name\":\"optimization-methods\",\"score\":38},{\"name\":\"representation-learning\",\"score\":20},{\"name\":\"unsupervised-learning\",\"score\":15},{\"name\":\"neural-coding\",\"score\":10},{\"name\":\"neural-architecture-search\",\"score\":9},{\"name\":\"generative-models\",\"score\":9},{\"name\":\"bayesian-deep-learning\",\"score\":8},{\"name\":\"uncertainty-estimation\",\"score\":8},{\"name\":\"neural-networks\",\"score\":7},{\"name\":\"clustering-algorithms\",\"score\":6},{\"name\":\"deep-reinforcement-learning\",\"score\":5},{\"name\":\"probabilistic-programming\",\"score\":5},{\"name\":\"energy-efficient-ml\",\"score\":4},{\"name\":\"machine-learning\",\"score\":4},{\"name\":\"efficient-transformers\",\"score\":3},{\"name\":\"bayesian-optimization\",\"score\":3},{\"name\":\"transfer-learning\",\"score\":3},{\"name\":\"time-series-analysis\",\"score\":2},{\"name\":\"machine-learning-theory\",\"score\":2},{\"name\":\"machine-translation\",\"score\":2},{\"name\":\"model-compression\",\"score\":2},{\"name\":\"adversarial-robustness\",\"score\":2},{\"name\":\"adversarial-attacks\",\"score\":2},{\"name\":\"distributed-learning\",\"score\":1},{\"name\":\"lightweight-models\",\"score\":1},{\"name\":\"hardware-acceleration\",\"score\":1},{\"name\":\"efficient-training\",\"score\":1},{\"name\":\"optical-computing\",\"score\":1},{\"name\":\"computer-vision-security\",\"score\":1},{\"name\":\"image-generation\",\"score\":1},{\"name\":\"graph-neural-networks\",\"score\":1},{\"name\":\"weak-supervision\",\"score\":1},{\"name\":\"training-efficiency\",\"score\":1},{\"name\":\"dimensionality-reduction\",\"score\":1},{\"name\":\"compressed-sensing\",\"score\":1},{\"name\":\"neural-networks-training\",\"score\":1},{\"name\":\"biologically-inspired-learning\",\"score\":1},{\"name\":\"neural-networks-theory\",\"score\":1},{\"name\":\"kernel-methods\",\"score\":1},{\"name\":\"sequence-modeling\",\"score\":1},{\"name\":\"information-extraction\",\"score\":1},{\"name\":\"ensemble-methods\",\"score\":1},{\"name\":\"deep-learning\",\"score\":1},{\"name\":\"matrix-completion\",\"score\":1},{\"name\":\"semi-supervised-learning\",\"score\":1},{\"name\":\"model-interpretation\",\"score\":1},{\"name\":\"privacy-preserving-ml\",\"score\":1},{\"name\":\"learning-theory\",\"score\":1},{\"name\":\"hardware-efficient-ml\",\"score\":1},{\"name\":\"online-learning\",\"score\":1},{\"name\":\"robotic-control\",\"score\":1},{\"name\":\"matrix-factorization\",\"score\":1}]},\"semantic_scholar\":{\"id\":\"2300473918\"},\"claimed_paper_groups\":[\"672bce18986a1370676dd2e8\",\"67348a6993ee43749600f621\",\"673bab8fee7cdcdc03b197a7\",\"673bab90bf626fe16b8aca32\",\"673bab91ee7cdcdc03b197ab\",\"673bab92ee7cdcdc03b197af\",\"673bab90bf626fe16b8aca33\",\"673bab93bf626fe16b8aca39\",\"6734b38493ee437496011aa7\",\"673bab94ee7cdcdc03b197b4\",\"673bab96ee7cdcdc03b197bc\",\"673bab97bf626fe16b8aca46\",\"673bab97ee7cdcdc03b197bf\",\"6734b36a93ee437496011a91\",\"673bab99bf626fe16b8aca50\",\"673bab99ee7cdcdc03b197c4\",\"673bab9aee7cdcdc03b197c8\",\"673bab9abf626fe16b8aca52\",\"673bab9bee7cdcdc03b197cb\",\"673bab9dee7cdcdc03b197cd\",\"673bab9eee7cdcdc03b197d2\",\"673bab9fbf626fe16b8aca61\",\"673bab9fbf626fe16b8aca64\",\"673baba0ee7cdcdc03b197d7\",\"673baba0ee7cdcdc03b197d8\",\"673baba1bf626fe16b8aca67\",\"673baba1ee7cdcdc03b197db\",\"673baba3bf626fe16b8aca6d\",\"673baba3ee7cdcdc03b197e4\",\"673baba4bf626fe16b8aca73\",\"673baba5ee7cdcdc03b197e6\",\"673baba5ee7cdcdc03b197e7\",\"673baba6ee7cdcdc03b197eb\",\"673baba6bf626fe16b8aca77\",\"673baba7bf626fe16b8aca79\",\"673baba8ee7cdcdc03b197ee\",\"673baba8bf626fe16b8aca7d\",\"673baba9ee7cdcdc03b197f4\",\"673bababee7cdcdc03b197fc\",\"673bababee7cdcdc03b197fd\",\"673babacbf626fe16b8aca85\",\"673babadee7cdcdc03b19805\",\"673babadee7cdcdc03b19807\",\"673babaebf626fe16b8aca89\",\"673babafee7cdcdc03b19811\",\"672bd673e78ce066acf2db98\",\"673babafbf626fe16b8aca8b\",\"673babb0ee7cdcdc03b19816\",\"673babb0ee7cdcdc03b19818\",\"673babb1bf626fe16b8aca8d\",\"673babb1bf626fe16b8aca8e\",\"673babb2bf626fe16b8aca91\",\"673babb2ee7cdcdc03b1981d\",\"673babb2bf626fe16b8aca95\",\"673babb3ee7cdcdc03b1981f\",\"673babb3ee7cdcdc03b19820\",\"673babb4bf626fe16b8aca97\",\"673babb4ee7cdcdc03b19824\",\"673babb4bf626fe16b8aca9a\",\"673babb6bf626fe16b8aca9c\",\"673babb6ee7cdcdc03b1982d\",\"673babb6bf626fe16b8aca9e\",\"673babb7ee7cdcdc03b1982f\",\"6734b3d193ee437496011ae2\",\"673babb7bf626fe16b8acaa1\",\"673bab9cbf626fe16b8aca57\",\"673babb8ee7cdcdc03b19831\",\"673babb8ee7cdcdc03b19833\",\"673babb9ee7cdcdc03b19836\",\"673babb9bf626fe16b8acaa6\",\"673babbabf626fe16b8acaa8\",\"673babbaee7cdcdc03b1983a\",\"673babbbbf626fe16b8acaaa\",\"673babbcee7cdcdc03b1983c\",\"673babbdee7cdcdc03b1983d\",\"673babbdee7cdcdc03b19840\",\"673babbebf626fe16b8acab7\",\"673babbebf626fe16b8acab8\",\"673babbfbf626fe16b8acabb\",\"673babbfee7cdcdc03b19842\",\"673babbfee7cdcdc03b19844\",\"673babc0bf626fe16b8acabe\",\"673babc1bf626fe16b8acac1\",\"673babc1ee7cdcdc03b19848\",\"673babc2ee7cdcdc03b1984a\",\"673babc3bf626fe16b8acac5\",\"672bd65be78ce066acf2da21\",\"673babc3ee7cdcdc03b1984e\",\"673babc4ee7cdcdc03b19852\",\"673babc4bf626fe16b8acac9\",\"67333356c48bba476d789343\",\"673babc5bf626fe16b8acacb\",\"673babc6bf626fe16b8acacd\",\"672bd689e78ce066acf2dcd0\",\"673babc6ee7cdcdc03b19856\",\"673babc7ee7cdcdc03b19858\",\"673babc7bf626fe16b8acad1\",\"673babc7ee7cdcdc03b1985a\",\"673babc7bf626fe16b8acad2\",\"672bd668e78ce066acf2daec\",\"673babc8ee7cdcdc03b1985d\",\"673babc9ee7cdcdc03b1985e\",\"673babc9bf626fe16b8acad8\",\"673babc9bf626fe16b8acadb\",\"673babcaee7cdcdc03b19864\",\"673babcaee7cdcdc03b19865\",\"673babcbee7cdcdc03b19868\",\"673babcbee7cdcdc03b19869\",\"673babcbbf626fe16b8acae3\",\"672bd68ae78ce066acf2dcdc\",\"673babcbbf626fe16b8acae4\",\"673babcbbf626fe16b8acae5\",\"673babccee7cdcdc03b1986c\",\"673babccee7cdcdc03b1986d\",\"673babcdee7cdcdc03b19871\",\"673babcdee7cdcdc03b19870\",\"673babcebf626fe16b8acaeb\",\"673babcebf626fe16b8acaee\",\"673babceee7cdcdc03b19875\",\"6734b42f93ee437496011b24\",\"673babcfbf626fe16b8acaf3\",\"673babcfbf626fe16b8acaf4\",\"672bd682e78ce066acf2dc65\",\"673babcfee7cdcdc03b19877\",\"673babd0bf626fe16b8acaf7\",\"673babd0ee7cdcdc03b19879\",\"673babd0bf626fe16b8acaf8\",\"673babd1bf626fe16b8acafb\",\"673baba7ee7cdcdc03b197ed\",\"673babd1ee7cdcdc03b1987c\",\"673babd2ee7cdcdc03b19887\",\"673babd2ee7cdcdc03b1988a\",\"673babd2bf626fe16b8acaff\",\"673babd3bf626fe16b8acb01\",\"673babd3bf626fe16b8acb02\",\"673babd4ee7cdcdc03b19892\",\"673babd4ee7cdcdc03b19895\",\"673babd4bf626fe16b8acb05\",\"673babd5ee7cdcdc03b19897\",\"673babd5bf626fe16b8acb07\",\"673babd6ee7cdcdc03b1989a\",\"673babd6ee7cdcdc03b1989b\",\"673babd7bf626fe16b8acb13\",\"672bcf44986a1370676de862\",\"673babd7ee7cdcdc03b1989f\",\"673babd7ee7cdcdc03b198a0\",\"673babd8ee7cdcdc03b198a3\",\"673babd8bf626fe16b8acb15\",\"673babd8bf626fe16b8acb16\",\"673babc0ee7cdcdc03b19846\",\"673babd9bf626fe16b8acb1c\",\"673babd9ee7cdcdc03b198a9\",\"673babd8ee7cdcdc03b198a4\",\"673bab9ebf626fe16b8aca5e\"],\"slug\":\"florent-krzakala\",\"following_paper_groups\":[\"672bce18986a1370676dd2e8\",\"67348a6993ee43749600f621\",\"673bab91ee7cdcdc03b197ab\",\"673bab92bf626fe16b8aca37\",\"673bab93bf626fe16b8aca39\",\"6734b38493ee437496011aa7\",\"673bab94ee7cdcdc03b197b4\",\"673bab96ee7cdcdc03b197bc\",\"673bab97bf626fe16b8aca46\",\"673bab97ee7cdcdc03b197bf\",\"6734b36a93ee437496011a91\",\"673bab99bf626fe16b8aca50\",\"673bab99ee7cdcdc03b197c4\",\"673bab9aee7cdcdc03b197c8\",\"673bab9abf626fe16b8aca52\",\"673bab9bee7cdcdc03b197cb\",\"673bab9dee7cdcdc03b197cd\",\"673bab9eee7cdcdc03b197d2\",\"673bab9fee7cdcdc03b197d5\",\"673bab9fbf626fe16b8aca61\",\"673bab9fbf626fe16b8aca64\",\"673baba0ee7cdcdc03b197d7\",\"673baba0ee7cdcdc03b197d8\",\"673baba1bf626fe16b8aca67\",\"673baba2bf626fe16b8aca69\",\"673baba3ee7cdcdc03b197e4\",\"673baba4bf626fe16b8aca73\",\"673baba5ee7cdcdc03b197e6\",\"673baba5ee7cdcdc03b197e7\",\"673baba6bf626fe16b8aca77\",\"673baba7bf626fe16b8aca79\",\"673baba7bf626fe16b8aca7a\",\"673baba8ee7cdcdc03b197ee\",\"673baba8bf626fe16b8aca7d\",\"673baba9ee7cdcdc03b197f4\",\"673babaaee7cdcdc03b197f7\",\"673bababee7cdcdc03b197fc\",\"673bababee7cdcdc03b197fd\",\"673babacbf626fe16b8aca85\",\"673babadee7cdcdc03b19807\",\"673babaebf626fe16b8aca89\",\"673babafee7cdcdc03b19811\",\"672bd673e78ce066acf2db98\",\"673babafbf626fe16b8aca8b\",\"673babb0ee7cdcdc03b19816\",\"673babb1bf626fe16b8aca8d\",\"673babb1bf626fe16b8aca8e\",\"673babb2bf626fe16b8aca91\",\"673babb2bf626fe16b8aca95\",\"673babb3ee7cdcdc03b1981f\",\"673babb3ee7cdcdc03b19820\",\"673babb4bf626fe16b8aca97\",\"673babb4bf626fe16b8aca9a\",\"673babb6bf626fe16b8aca9e\",\"6734b3d193ee437496011ae2\",\"673babb7bf626fe16b8acaa1\",\"673bab9cbf626fe16b8aca57\",\"673babb8ee7cdcdc03b19831\",\"673babb8ee7cdcdc03b19833\",\"673babb9bf626fe16b8acaa3\",\"673babb9bf626fe16b8acaa6\",\"673babbabf626fe16b8acaa8\",\"673babbbbf626fe16b8acaaa\",\"673babbcee7cdcdc03b1983c\",\"673babbdee7cdcdc03b1983d\",\"673babbebf626fe16b8acab7\",\"673babbebf626fe16b8acab8\",\"673babbfee7cdcdc03b19842\",\"673babc0bf626fe16b8acabe\",\"673babc1bf626fe16b8acac1\",\"673babc1ee7cdcdc03b19848\",\"673babc2ee7cdcdc03b1984a\",\"673babc3bf626fe16b8acac5\",\"672bd65be78ce066acf2da21\",\"673babc3ee7cdcdc03b1984e\",\"673babc4ee7cdcdc03b19852\",\"673babc4bf626fe16b8acac9\",\"67333356c48bba476d789343\",\"673babc5bf626fe16b8acacb\",\"673babc6bf626fe16b8acacd\",\"672bd689e78ce066acf2dcd0\",\"673babc6ee7cdcdc03b19856\",\"673babc7bf626fe16b8acacf\",\"673babc7ee7cdcdc03b19858\",\"673babc7bf626fe16b8acad1\",\"673babc7ee7cdcdc03b1985a\",\"673babc7bf626fe16b8acad2\",\"672bd668e78ce066acf2daec\",\"673babc8ee7cdcdc03b1985d\",\"673babc9ee7cdcdc03b1985e\",\"673babc9bf626fe16b8acad8\",\"673babc9bf626fe16b8acadb\",\"673babcaee7cdcdc03b19864\",\"673babcaee7cdcdc03b19865\",\"673babcabf626fe16b8acade\",\"673babcbbf626fe16b8acae3\",\"672bd68ae78ce066acf2dcdc\",\"673babcbbf626fe16b8acae4\",\"673babcbbf626fe16b8acae5\",\"673babccee7cdcdc03b1986c\",\"673babccbf626fe16b8acae9\",\"673babccee7cdcdc03b1986d\",\"673babcdee7cdcdc03b19870\",\"673babcebf626fe16b8acaeb\",\"673babcebf626fe16b8acaee\",\"673babceee7cdcdc03b19875\",\"6734b42f93ee437496011b24\",\"673babcfbf626fe16b8acaf3\",\"673babcfbf626fe16b8acaf4\",\"672bd682e78ce066acf2dc65\",\"673babcfee7cdcdc03b19877\",\"673babd0bf626fe16b8acaf7\",\"673babd0ee7cdcdc03b19879\",\"673babd0bf626fe16b8acaf8\",\"673babd1bf626fe16b8acafb\",\"673baba7ee7cdcdc03b197ed\",\"673babd2bf626fe16b8acafd\",\"673babd2ee7cdcdc03b19887\",\"673babd2ee7cdcdc03b1988a\",\"673babd2bf626fe16b8acaff\",\"673babd3bf626fe16b8acb01\",\"673babd3bf626fe16b8acb02\",\"673babd4ee7cdcdc03b19892\",\"673babd4bf626fe16b8acb05\",\"673babd5ee7cdcdc03b19897\",\"673babd5bf626fe16b8acb07\",\"673babd6ee7cdcdc03b1989a\",\"673babd7bf626fe16b8acb13\",\"672bcf44986a1370676de862\",\"673babd7ee7cdcdc03b1989f\",\"673babd7ee7cdcdc03b198a0\",\"673babd8ee7cdcdc03b198a3\",\"673babd8bf626fe16b8acb15\",\"673babc0ee7cdcdc03b19846\",\"673babd9bf626fe16b8acb1c\",\"673babd9ee7cdcdc03b198a9\",\"673babd8ee7cdcdc03b198a4\"],\"followingUsers\":[],\"created_at\":\"2024-08-01T20:14:12.461Z\",\"voted_paper_groups\":[],\"followerCount\":0,\"preferences\":{\"communities_order\":{\"communities\":[],\"global_community_index\":0},\"model\":\"gemini-2.0-flash\",\"folders\":[{\"folder_id\":\"67ad610dd4568bf90d84a61e\",\"opened\":false},{\"folder_id\":\"67ad610dd4568bf90d84a61f\",\"opened\":false},{\"folder_id\":\"67ad610dd4568bf90d84a620\",\"opened\":false},{\"folder_id\":\"67ad610dd4568bf90d84a621\",\"opened\":false}],\"show_my_communities_in_sidebar\":true,\"enable_dark_mode\":false,\"current_community_slug\":\"global\",\"topic_preferences\":[]},\"following_orgs\":[],\"following_topics\":[]}],\"authors\":[{\"_id\":\"672bce19986a1370676dd2f2\",\"full_name\":\"Florent Krzakala\",\"affiliation\":null,\"orcid\":null,\"semantic_scholarid\":null,\"user_id\":null},{\"_id\":\"672bce19986a1370676dd2fa\",\"full_name\":\"Lenka Zdeborová\",\"affiliation\":null,\"orcid\":null,\"semantic_scholarid\":null,\"user_id\":null},{\"_id\":\"672bd17c986a1370676e1825\",\"full_name\":\"Maria Refinetti\",\"affiliation\":null,\"orcid\":null,\"semantic_scholarid\":null,\"user_id\":null},{\"_id\":\"672bd17d986a1370676e183b\",\"full_name\":\"Sebastian Goldt\",\"affiliation\":null,\"orcid\":null,\"semantic_scholarid\":null,\"user_id\":null}]},\"max_version_order\":2,\"verified_authors\":[{\"_id\":\"66aa969c94a3f2f6c93dcaef\",\"useremail\":\"florent.krzakala@gmail.com\",\"username\":\"Florent KRZAKALA\",\"realname\":\"Florent KRZAKALA\",\"totalupvotes\":0,\"numquestions\":0,\"numresponses\":0,\"papers\":[],\"activity\":[],\"following\":[],\"followers\":[],\"followingPapers\":[\"1109.3041v2\",\"1511.02476v5\",\"1109.4424v4\",\"arXiv:1909.11500v4\",\"1708.03395v3\",\"1907.00657v2\",\"1701.00858v3\",\"0901.2130v2\",\"1406.1880v2\",\"1402.1298v3\",\"1510.06664v2\",\"1906.08632v2\",\"2002.09339v2\",\"2006.14709v3\",\"1603.08447v1\",\"1306.5550v2\",\"1701.08010v2\",\"1507.04113v1\",\"cond-mat_0606180v2\",\"1503.08040v4\",\"1506.02914v2\",\"0911.1551v2\",\"1003.2748v1\",\"0807.2553v2\",\"cond-mat_0212070v2\",\"1701.05823v2\",\"1607.02335v2\",\"2006.06098v2\",\"2105.15004v2\",\"1905.12385v2\",\"2102.11742v2\",\"cond-mat_0002055v2\",\"1701.06981v1\",\"1801.01593v2\",\"2006.06560v2\",\"cond-mat_0107366v2\",\"2006.06581v6\",\"1312.1740v5\",\"1806.09588v1\",\"1907.08226v3\",\"1306.4121v2\",\"0910.3008v2\",\"1609.05204v3\",\"2006.05228v2\",\"1211.2379v2\",\"2002.04372v1\",\"1301.5898v1\",\"1610.02918v1\",\"0909.3820v3\",\"1702.03260v3\",\"0805.3509v2\",\"1207.2328v2\",\"0902.4185v3\",\"2106.03791v2\",\"1510.01098v2\",\"2110.08775v3\",\"2102.08127v3\",\"1006.2479v2\",\"2202.00293v4\",\"cond-mat_0403053v3\",\"2006.07310v2\",\"1203.5521v3\",\"2305.18270v3\",\"2302.00375v2\",\"1812.02537v1\",\"2006.06997v1\",\"1404.7787v1\",\"1006.2480v2\",\"1008.4497v1\",\"2210.06591v3\",\"1710.02903v1\",\"1207.2079v1\",\"1203.3166v2\",\"2012.06373v1\",\"1604.02475v2\",\"1506.03498v3\",\"2302.08923v1\",\"2302.08933v1\",\"1301.0901v1\",\"1809.06304v1\",\"1605.07516v1\",\"1302.0189v1\",\"cond-mat_0512309v3\",\"1601.06683v2\",\"2203.07752v1\",\"2308.14085v1\",\"0910.5644v1\",\"1810.13038v1\",\"2402.03220v3\",\"1204.3734v1\",\"2210.12760v4\",\"1101.5863v1\",\"2208.05918v1\",\"1901.09085v1\",\"cond-mat_0211106v2\",\"1906.04735v1\",\"2107.11814v1\",\"1706.00705v1\",\"1605.06422v3\",\"2202.03295v2\",\"cond-mat_0208566v2\",\"2302.05882v1\",\"1307.7846v3\",\"2302.06665v1\",\"2310.14055v1\",\"0709.0894v1\",\"1602.02944v2\",\"2402.04980v2\",\"2310.03575v2\",\"2006.01475v2\",\"1304.6599v2\",\"2303.02644v2\",\"2402.03902v1\",\"0709.1023v1\",\"1609.08269v1\",\"2310.02850v2\",\"cond-mat_0409448v2\",\"2402.13622v1\",\"2201.09986v3\",\"2405.15459v1\",\"2205.13527v2\",\"1511.05860v2\",\"2101.02115v1\",\"2306.09283v1\",\"2405.15480v2\",\"2406.02157v1\",\"2405.04267v2\",\"2205.08782v1\",\"2408.03733v1\",\"2205.13503v2\",\"2403.04234v1\",\"1910.00285v2\",\"2402.05674v2\",\"1104.0921v1\",\"0710.3336v2\",\"1502.03324v1\",\"1205.4200v4\"],\"claimedPapers\":[\"1109.3041v2\",\"1511.02476v5\",\"1206.3953v1\",\"1102.1182v1\",\"1109.4424v4\",\"0806.3665v3\",\"arXiv:1909.11500v4\",\"1210.0811v2\",\"1708.03395v3\",\"1907.00657v2\",\"1701.00858v3\",\"0901.2130v2\",\"1406.1880v2\",\"1402.1298v3\",\"cond-mat_0702546v4\",\"1510.06664v2\",\"1906.08632v2\",\"2002.09339v2\",\"2006.14709v3\",\"1603.08447v1\",\"1306.5550v2\",\"1701.08010v2\",\"1507.04113v1\",\"cond-mat_0606180v2\",\"1503.08040v4\",\"1506.02914v2\",\"0911.1551v2\",\"1003.2748v1\",\"0807.2553v2\",\"2006.12878v2\",\"cond-mat_0212070v2\",\"0909.4256v2\",\"1701.05823v2\",\"1607.02335v2\",\"2006.06098v2\",\"2105.15004v2\",\"1802.08963v2\",\"1905.12385v2\",\"2102.11742v2\",\"cond-mat_0002055v2\",\"1701.06981v1\",\"1801.01593v2\",\"2006.06560v2\",\"cond-mat_0107366v2\",\"2006.06581v6\",\"1312.1740v5\",\"1806.09588v1\",\"1403.8024v2\",\"cond-mat_0203449v2\",\"1907.08226v3\",\"1306.4121v2\",\"0910.3008v2\",\"1609.05204v3\",\"2006.05228v2\",\"1211.2379v2\",\"1906.08479v3\",\"2002.04372v1\",\"1301.5898v1\",\"1610.02918v1\",\"1410.1368v2\",\"0909.3820v3\",\"1702.03260v3\",\"0805.3509v2\",\"1207.2328v2\",\"1912.02008v2\",\"0902.4185v3\",\"1906.04554v1\",\"1812.09066v4\",\"2106.03791v2\",\"1502.06470v3\",\"1510.01098v2\",\"2110.08775v3\",\"2102.08127v3\",\"1006.2479v2\",\"2202.00293v4\",\"cond-mat_0403053v3\",\"1910.09880v2\",\"2006.07310v2\",\"1203.5521v3\",\"2205.13303v2\",\"2305.18270v3\",\"2302.00375v2\",\"1812.02537v1\",\"2201.13383v1\",\"2006.06997v1\",\"1404.7787v1\",\"1807.01296v1\",\"1006.2480v2\",\"1606.03956v1\",\"1008.4497v1\",\"2210.06591v3\",\"1710.02903v1\",\"1207.2079v1\",\"1203.3166v2\",\"2012.06373v1\",\"1604.02475v2\",\"1506.03498v3\",\"2302.08923v1\",\"2302.08933v1\",\"1301.0901v1\",\"1809.06304v1\",\"1605.07516v1\",\"1302.0189v1\",\"cond-mat_0512309v3\",\"1601.06683v2\",\"2203.07752v1\",\"2308.14085v1\",\"0910.5644v1\",\"1810.13038v1\",\"cond-mat_0010010v2\",\"2402.03220v3\",\"1204.3734v1\",\"2210.12760v4\",\"1101.5863v1\",\"2208.05918v1\",\"1901.09085v1\",\"cond-mat_0211106v2\",\"2201.12655v3\",\"2012.04524v3\",\"1906.04735v1\",\"2107.11814v1\",\"1706.00705v1\",\"1605.06422v3\",\"2202.03295v2\",\"cond-mat_0208566v2\",\"2302.05882v1\",\"2305.18502v2\",\"1307.7846v3\",\"2302.06665v1\",\"2310.14055v1\",\"0709.0894v1\",\"1602.02944v2\",\"2402.04980v2\",\"2310.03575v2\",\"2006.01475v2\",\"1304.6599v2\",\"2303.02644v2\",\"2402.03902v1\",\"0709.1023v1\",\"1609.08269v1\",\"2310.02850v2\",\"2105.07416v2\",\"cond-mat_0409448v2\",\"2402.13622v1\",\"2201.09986v3\",\"cond-mat_0409449v3\",\"2405.15459v1\",\"2205.13527v2\",\"1511.05860v2\",\"2101.02115v1\",\"2403.03695v1\",\"2306.09283v1\",\"2405.15480v2\",\"2406.02157v1\",\"2405.04267v2\",\"2305.18974v2\",\"2205.08782v1\",\"2408.03733v1\",\"2205.13503v2\",\"2403.04234v1\",\"1910.00285v2\",\"2402.05674v2\",\"0712.2009v2\",\"1104.0921v1\",\"0710.3336v2\",\"1502.03324v1\",\"1205.4200v4\",\"0806.4144v3\"],\"biography\":\"\",\"lastViewedGroup\":\"public\",\"groups\":[],\"todayQ\":0,\"todayR\":0,\"daysActive\":145,\"upvotesGivenToday\":0,\"downvotesGivenToday\":0,\"lastViewOfFollowingPapers\":\"2024-09-20T14:17:55.359Z\",\"usernameChanged\":false,\"firstLogin\":true,\"subscribedPotw\":true,\"orcid_id\":\"0000-0003-2313-2578\",\"role\":\"user\",\"institution\":null,\"gscholar_id\":\"3jDeUlMAAAAJ\",\"reputation\":15,\"bookmarks\":\"$4d\",\"weeklyReputation\":0,\"email_settings\":{\"direct_notifications\":true,\"relevant_activity\":false},\"interests\":{\"categories\":[\"Computer Science\",\"Physics\",\"Mathematics\",\"Statistics\"],\"subcategories\":[{\"name\":\"cond-mat.dis-nn\",\"score\":89},{\"name\":\"cs.LG\",\"score\":70},{\"name\":\"stat.ML\",\"score\":68},{\"name\":\"cond-mat.stat-mech\",\"score\":57},{\"name\":\"cs.IT\",\"score\":47},{\"name\":\"math.ST\",\"score\":28},{\"name\":\"math.PR\",\"score\":21},{\"name\":\"cs.SI\",\"score\":7},{\"name\":\"cs.ET\",\"score\":6},{\"name\":\"math-ph\",\"score\":6},{\"name\":\"physics.soc-ph\",\"score\":5},{\"name\":\"cs.NE\",\"score\":5},{\"name\":\"cs.CC\",\"score\":5},{\"name\":\"quant-ph\",\"score\":5},{\"name\":\"physics.optics\",\"score\":4},{\"name\":\"eess.SP\",\"score\":4},{\"name\":\"cs.AI\",\"score\":3},{\"name\":\"physics.data-an\",\"score\":3},{\"name\":\"cond-mat.soft\",\"score\":3},{\"name\":\"cs.AR\",\"score\":2},{\"name\":\"eess.IV\",\"score\":2},{\"name\":\"cs.CV\",\"score\":2},{\"name\":\"math.NA\",\"score\":2},{\"name\":\"cs.CR\",\"score\":2},{\"name\":\"cs.DS\",\"score\":1},{\"name\":\"cs.IR\",\"score\":1},{\"name\":\"nlin.CD\",\"score\":1},{\"name\":\"physics.flu-dyn\",\"score\":1},{\"name\":\"cs.DM\",\"score\":1},{\"name\":\"stat.AP\",\"score\":1},{\"name\":\"cs.DC\",\"score\":1},{\"name\":\"q-bio.GN\",\"score\":1},{\"name\":\"q-bio.QM\",\"score\":1},{\"name\":\"nlin.AO\",\"score\":1},{\"name\":\"q-bio.NC\",\"score\":1}],\"custom_categories\":[{\"name\":\"statistical-learning\",\"score\":56},{\"name\":\"optimization-methods\",\"score\":38},{\"name\":\"representation-learning\",\"score\":20},{\"name\":\"unsupervised-learning\",\"score\":15},{\"name\":\"neural-coding\",\"score\":10},{\"name\":\"neural-architecture-search\",\"score\":9},{\"name\":\"generative-models\",\"score\":9},{\"name\":\"bayesian-deep-learning\",\"score\":8},{\"name\":\"uncertainty-estimation\",\"score\":8},{\"name\":\"neural-networks\",\"score\":7},{\"name\":\"clustering-algorithms\",\"score\":6},{\"name\":\"deep-reinforcement-learning\",\"score\":5},{\"name\":\"probabilistic-programming\",\"score\":5},{\"name\":\"energy-efficient-ml\",\"score\":4},{\"name\":\"machine-learning\",\"score\":4},{\"name\":\"efficient-transformers\",\"score\":3},{\"name\":\"bayesian-optimization\",\"score\":3},{\"name\":\"transfer-learning\",\"score\":3},{\"name\":\"time-series-analysis\",\"score\":2},{\"name\":\"machine-learning-theory\",\"score\":2},{\"name\":\"machine-translation\",\"score\":2},{\"name\":\"model-compression\",\"score\":2},{\"name\":\"adversarial-robustness\",\"score\":2},{\"name\":\"adversarial-attacks\",\"score\":2},{\"name\":\"distributed-learning\",\"score\":1},{\"name\":\"lightweight-models\",\"score\":1},{\"name\":\"hardware-acceleration\",\"score\":1},{\"name\":\"efficient-training\",\"score\":1},{\"name\":\"optical-computing\",\"score\":1},{\"name\":\"computer-vision-security\",\"score\":1},{\"name\":\"image-generation\",\"score\":1},{\"name\":\"graph-neural-networks\",\"score\":1},{\"name\":\"weak-supervision\",\"score\":1},{\"name\":\"training-efficiency\",\"score\":1},{\"name\":\"dimensionality-reduction\",\"score\":1},{\"name\":\"compressed-sensing\",\"score\":1},{\"name\":\"neural-networks-training\",\"score\":1},{\"name\":\"biologically-inspired-learning\",\"score\":1},{\"name\":\"neural-networks-theory\",\"score\":1},{\"name\":\"kernel-methods\",\"score\":1},{\"name\":\"sequence-modeling\",\"score\":1},{\"name\":\"information-extraction\",\"score\":1},{\"name\":\"ensemble-methods\",\"score\":1},{\"name\":\"deep-learning\",\"score\":1},{\"name\":\"matrix-completion\",\"score\":1},{\"name\":\"semi-supervised-learning\",\"score\":1},{\"name\":\"model-interpretation\",\"score\":1},{\"name\":\"privacy-preserving-ml\",\"score\":1},{\"name\":\"learning-theory\",\"score\":1},{\"name\":\"hardware-efficient-ml\",\"score\":1},{\"name\":\"online-learning\",\"score\":1},{\"name\":\"robotic-control\",\"score\":1},{\"name\":\"matrix-factorization\",\"score\":1}]},\"semantic_scholar\":{\"id\":\"2300473918\"},\"claimed_paper_groups\":[\"672bce18986a1370676dd2e8\",\"67348a6993ee43749600f621\",\"673bab8fee7cdcdc03b197a7\",\"673bab90bf626fe16b8aca32\",\"673bab91ee7cdcdc03b197ab\",\"673bab92ee7cdcdc03b197af\",\"673bab90bf626fe16b8aca33\",\"673bab93bf626fe16b8aca39\",\"6734b38493ee437496011aa7\",\"673bab94ee7cdcdc03b197b4\",\"673bab96ee7cdcdc03b197bc\",\"673bab97bf626fe16b8aca46\",\"673bab97ee7cdcdc03b197bf\",\"6734b36a93ee437496011a91\",\"673bab99bf626fe16b8aca50\",\"673bab99ee7cdcdc03b197c4\",\"673bab9aee7cdcdc03b197c8\",\"673bab9abf626fe16b8aca52\",\"673bab9bee7cdcdc03b197cb\",\"673bab9dee7cdcdc03b197cd\",\"673bab9eee7cdcdc03b197d2\",\"673bab9fbf626fe16b8aca61\",\"673bab9fbf626fe16b8aca64\",\"673baba0ee7cdcdc03b197d7\",\"673baba0ee7cdcdc03b197d8\",\"673baba1bf626fe16b8aca67\",\"673baba1ee7cdcdc03b197db\",\"673baba3bf626fe16b8aca6d\",\"673baba3ee7cdcdc03b197e4\",\"673baba4bf626fe16b8aca73\",\"673baba5ee7cdcdc03b197e6\",\"673baba5ee7cdcdc03b197e7\",\"673baba6ee7cdcdc03b197eb\",\"673baba6bf626fe16b8aca77\",\"673baba7bf626fe16b8aca79\",\"673baba8ee7cdcdc03b197ee\",\"673baba8bf626fe16b8aca7d\",\"673baba9ee7cdcdc03b197f4\",\"673bababee7cdcdc03b197fc\",\"673bababee7cdcdc03b197fd\",\"673babacbf626fe16b8aca85\",\"673babadee7cdcdc03b19805\",\"673babadee7cdcdc03b19807\",\"673babaebf626fe16b8aca89\",\"673babafee7cdcdc03b19811\",\"672bd673e78ce066acf2db98\",\"673babafbf626fe16b8aca8b\",\"673babb0ee7cdcdc03b19816\",\"673babb0ee7cdcdc03b19818\",\"673babb1bf626fe16b8aca8d\",\"673babb1bf626fe16b8aca8e\",\"673babb2bf626fe16b8aca91\",\"673babb2ee7cdcdc03b1981d\",\"673babb2bf626fe16b8aca95\",\"673babb3ee7cdcdc03b1981f\",\"673babb3ee7cdcdc03b19820\",\"673babb4bf626fe16b8aca97\",\"673babb4ee7cdcdc03b19824\",\"673babb4bf626fe16b8aca9a\",\"673babb6bf626fe16b8aca9c\",\"673babb6ee7cdcdc03b1982d\",\"673babb6bf626fe16b8aca9e\",\"673babb7ee7cdcdc03b1982f\",\"6734b3d193ee437496011ae2\",\"673babb7bf626fe16b8acaa1\",\"673bab9cbf626fe16b8aca57\",\"673babb8ee7cdcdc03b19831\",\"673babb8ee7cdcdc03b19833\",\"673babb9ee7cdcdc03b19836\",\"673babb9bf626fe16b8acaa6\",\"673babbabf626fe16b8acaa8\",\"673babbaee7cdcdc03b1983a\",\"673babbbbf626fe16b8acaaa\",\"673babbcee7cdcdc03b1983c\",\"673babbdee7cdcdc03b1983d\",\"673babbdee7cdcdc03b19840\",\"673babbebf626fe16b8acab7\",\"673babbebf626fe16b8acab8\",\"673babbfbf626fe16b8acabb\",\"673babbfee7cdcdc03b19842\",\"673babbfee7cdcdc03b19844\",\"673babc0bf626fe16b8acabe\",\"673babc1bf626fe16b8acac1\",\"673babc1ee7cdcdc03b19848\",\"673babc2ee7cdcdc03b1984a\",\"673babc3bf626fe16b8acac5\",\"672bd65be78ce066acf2da21\",\"673babc3ee7cdcdc03b1984e\",\"673babc4ee7cdcdc03b19852\",\"673babc4bf626fe16b8acac9\",\"67333356c48bba476d789343\",\"673babc5bf626fe16b8acacb\",\"673babc6bf626fe16b8acacd\",\"672bd689e78ce066acf2dcd0\",\"673babc6ee7cdcdc03b19856\",\"673babc7ee7cdcdc03b19858\",\"673babc7bf626fe16b8acad1\",\"673babc7ee7cdcdc03b1985a\",\"673babc7bf626fe16b8acad2\",\"672bd668e78ce066acf2daec\",\"673babc8ee7cdcdc03b1985d\",\"673babc9ee7cdcdc03b1985e\",\"673babc9bf626fe16b8acad8\",\"673babc9bf626fe16b8acadb\",\"673babcaee7cdcdc03b19864\",\"673babcaee7cdcdc03b19865\",\"673babcbee7cdcdc03b19868\",\"673babcbee7cdcdc03b19869\",\"673babcbbf626fe16b8acae3\",\"672bd68ae78ce066acf2dcdc\",\"673babcbbf626fe16b8acae4\",\"673babcbbf626fe16b8acae5\",\"673babccee7cdcdc03b1986c\",\"673babccee7cdcdc03b1986d\",\"673babcdee7cdcdc03b19871\",\"673babcdee7cdcdc03b19870\",\"673babcebf626fe16b8acaeb\",\"673babcebf626fe16b8acaee\",\"673babceee7cdcdc03b19875\",\"6734b42f93ee437496011b24\",\"673babcfbf626fe16b8acaf3\",\"673babcfbf626fe16b8acaf4\",\"672bd682e78ce066acf2dc65\",\"673babcfee7cdcdc03b19877\",\"673babd0bf626fe16b8acaf7\",\"673babd0ee7cdcdc03b19879\",\"673babd0bf626fe16b8acaf8\",\"673babd1bf626fe16b8acafb\",\"673baba7ee7cdcdc03b197ed\",\"673babd1ee7cdcdc03b1987c\",\"673babd2ee7cdcdc03b19887\",\"673babd2ee7cdcdc03b1988a\",\"673babd2bf626fe16b8acaff\",\"673babd3bf626fe16b8acb01\",\"673babd3bf626fe16b8acb02\",\"673babd4ee7cdcdc03b19892\",\"673babd4ee7cdcdc03b19895\",\"673babd4bf626fe16b8acb05\",\"673babd5ee7cdcdc03b19897\",\"673babd5bf626fe16b8acb07\",\"673babd6ee7cdcdc03b1989a\",\"673babd6ee7cdcdc03b1989b\",\"673babd7bf626fe16b8acb13\",\"672bcf44986a1370676de862\",\"673babd7ee7cdcdc03b1989f\",\"673babd7ee7cdcdc03b198a0\",\"673babd8ee7cdcdc03b198a3\",\"673babd8bf626fe16b8acb15\",\"673babd8bf626fe16b8acb16\",\"673babc0ee7cdcdc03b19846\",\"673babd9bf626fe16b8acb1c\",\"673babd9ee7cdcdc03b198a9\",\"673babd8ee7cdcdc03b198a4\",\"673bab9ebf626fe16b8aca5e\"],\"slug\":\"florent-krzakala\",\"following_paper_groups\":[\"672bce18986a1370676dd2e8\",\"67348a6993ee43749600f621\",\"673bab91ee7cdcdc03b197ab\",\"673bab92bf626fe16b8aca37\",\"673bab93bf626fe16b8aca39\",\"6734b38493ee437496011aa7\",\"673bab94ee7cdcdc03b197b4\",\"673bab96ee7cdcdc03b197bc\",\"673bab97bf626fe16b8aca46\",\"673bab97ee7cdcdc03b197bf\",\"6734b36a93ee437496011a91\",\"673bab99bf626fe16b8aca50\",\"673bab99ee7cdcdc03b197c4\",\"673bab9aee7cdcdc03b197c8\",\"673bab9abf626fe16b8aca52\",\"673bab9bee7cdcdc03b197cb\",\"673bab9dee7cdcdc03b197cd\",\"673bab9eee7cdcdc03b197d2\",\"673bab9fee7cdcdc03b197d5\",\"673bab9fbf626fe16b8aca61\",\"673bab9fbf626fe16b8aca64\",\"673baba0ee7cdcdc03b197d7\",\"673baba0ee7cdcdc03b197d8\",\"673baba1bf626fe16b8aca67\",\"673baba2bf626fe16b8aca69\",\"673baba3ee7cdcdc03b197e4\",\"673baba4bf626fe16b8aca73\",\"673baba5ee7cdcdc03b197e6\",\"673baba5ee7cdcdc03b197e7\",\"673baba6bf626fe16b8aca77\",\"673baba7bf626fe16b8aca79\",\"673baba7bf626fe16b8aca7a\",\"673baba8ee7cdcdc03b197ee\",\"673baba8bf626fe16b8aca7d\",\"673baba9ee7cdcdc03b197f4\",\"673babaaee7cdcdc03b197f7\",\"673bababee7cdcdc03b197fc\",\"673bababee7cdcdc03b197fd\",\"673babacbf626fe16b8aca85\",\"673babadee7cdcdc03b19807\",\"673babaebf626fe16b8aca89\",\"673babafee7cdcdc03b19811\",\"672bd673e78ce066acf2db98\",\"673babafbf626fe16b8aca8b\",\"673babb0ee7cdcdc03b19816\",\"673babb1bf626fe16b8aca8d\",\"673babb1bf626fe16b8aca8e\",\"673babb2bf626fe16b8aca91\",\"673babb2bf626fe16b8aca95\",\"673babb3ee7cdcdc03b1981f\",\"673babb3ee7cdcdc03b19820\",\"673babb4bf626fe16b8aca97\",\"673babb4bf626fe16b8aca9a\",\"673babb6bf626fe16b8aca9e\",\"6734b3d193ee437496011ae2\",\"673babb7bf626fe16b8acaa1\",\"673bab9cbf626fe16b8aca57\",\"673babb8ee7cdcdc03b19831\",\"673babb8ee7cdcdc03b19833\",\"673babb9bf626fe16b8acaa3\",\"673babb9bf626fe16b8acaa6\",\"673babbabf626fe16b8acaa8\",\"673babbbbf626fe16b8acaaa\",\"673babbcee7cdcdc03b1983c\",\"673babbdee7cdcdc03b1983d\",\"673babbebf626fe16b8acab7\",\"673babbebf626fe16b8acab8\",\"673babbfee7cdcdc03b19842\",\"673babc0bf626fe16b8acabe\",\"673babc1bf626fe16b8acac1\",\"673babc1ee7cdcdc03b19848\",\"673babc2ee7cdcdc03b1984a\",\"673babc3bf626fe16b8acac5\",\"672bd65be78ce066acf2da21\",\"673babc3ee7cdcdc03b1984e\",\"673babc4ee7cdcdc03b19852\",\"673babc4bf626fe16b8acac9\",\"67333356c48bba476d789343\",\"673babc5bf626fe16b8acacb\",\"673babc6bf626fe16b8acacd\",\"672bd689e78ce066acf2dcd0\",\"673babc6ee7cdcdc03b19856\",\"673babc7bf626fe16b8acacf\",\"673babc7ee7cdcdc03b19858\",\"673babc7bf626fe16b8acad1\",\"673babc7ee7cdcdc03b1985a\",\"673babc7bf626fe16b8acad2\",\"672bd668e78ce066acf2daec\",\"673babc8ee7cdcdc03b1985d\",\"673babc9ee7cdcdc03b1985e\",\"673babc9bf626fe16b8acad8\",\"673babc9bf626fe16b8acadb\",\"673babcaee7cdcdc03b19864\",\"673babcaee7cdcdc03b19865\",\"673babcabf626fe16b8acade\",\"673babcbbf626fe16b8acae3\",\"672bd68ae78ce066acf2dcdc\",\"673babcbbf626fe16b8acae4\",\"673babcbbf626fe16b8acae5\",\"673babccee7cdcdc03b1986c\",\"673babccbf626fe16b8acae9\",\"673babccee7cdcdc03b1986d\",\"673babcdee7cdcdc03b19870\",\"673babcebf626fe16b8acaeb\",\"673babcebf626fe16b8acaee\",\"673babceee7cdcdc03b19875\",\"6734b42f93ee437496011b24\",\"673babcfbf626fe16b8acaf3\",\"673babcfbf626fe16b8acaf4\",\"672bd682e78ce066acf2dc65\",\"673babcfee7cdcdc03b19877\",\"673babd0bf626fe16b8acaf7\",\"673babd0ee7cdcdc03b19879\",\"673babd0bf626fe16b8acaf8\",\"673babd1bf626fe16b8acafb\",\"673baba7ee7cdcdc03b197ed\",\"673babd2bf626fe16b8acafd\",\"673babd2ee7cdcdc03b19887\",\"673babd2ee7cdcdc03b1988a\",\"673babd2bf626fe16b8acaff\",\"673babd3bf626fe16b8acb01\",\"673babd3bf626fe16b8acb02\",\"673babd4ee7cdcdc03b19892\",\"673babd4bf626fe16b8acb05\",\"673babd5ee7cdcdc03b19897\",\"673babd5bf626fe16b8acb07\",\"673babd6ee7cdcdc03b1989a\",\"673babd7bf626fe16b8acb13\",\"672bcf44986a1370676de862\",\"673babd7ee7cdcdc03b1989f\",\"673babd7ee7cdcdc03b198a0\",\"673babd8ee7cdcdc03b198a3\",\"673babd8bf626fe16b8acb15\",\"673babc0ee7cdcdc03b19846\",\"673babd9bf626fe16b8acb1c\",\"673babd9ee7cdcdc03b198a9\",\"673babd8ee7cdcdc03b198a4\"],\"followingUsers\":[],\"created_at\":\"2024-08-01T20:14:12.461Z\",\"voted_paper_groups\":[],\"followerCount\":0,\"preferences\":{\"communities_order\":{\"communities\":[],\"global_community_index\":0},\"model\":\"gemini-2.0-flash\",\"folders\":[{\"folder_id\":\"67ad610dd4568bf90d84a61e\",\"opened\":false},{\"folder_id\":\"67ad610dd4568bf90d84a61f\",\"opened\":false},{\"folder_id\":\"67ad610dd4568bf90d84a620\",\"opened\":false},{\"folder_id\":\"67ad610dd4568bf90d84a621\",\"opened\":false}],\"show_my_communities_in_sidebar\":true,\"enable_dark_mode\":false,\"current_community_slug\":\"global\",\"topic_preferences\":[]},\"following_orgs\":[],\"following_topics\":[]}],\"authors\":[{\"_id\":\"672bce19986a1370676dd2f2\",\"full_name\":\"Florent Krzakala\",\"affiliation\":null,\"orcid\":null,\"semantic_scholarid\":null,\"user_id\":null},{\"_id\":\"672bce19986a1370676dd2fa\",\"full_name\":\"Lenka Zdeborová\",\"affiliation\":null,\"orcid\":null,\"semantic_scholarid\":null,\"user_id\":null},{\"_id\":\"672bd17c986a1370676e1825\",\"full_name\":\"Maria Refinetti\",\"affiliation\":null,\"orcid\":null,\"semantic_scholarid\":null,\"user_id\":null},{\"_id\":\"672bd17d986a1370676e183b\",\"full_name\":\"Sebastian Goldt\",\"affiliation\":null,\"orcid\":null,\"semantic_scholarid\":null,\"user_id\":null}],\"pdf_info\":{\"fetcher_url\":\"https://fetcher.alphaxiv.org/v2/pdf/2102.11742v2\"}}},\"dataUpdateCount\":1,\"dataUpdatedAt\":1744228196924,\"error\":null,\"errorUpdateCount\":0,\"errorUpdatedAt\":0,\"fetchFailureCount\":0,\"fetchFailureReason\":null,\"fetchMeta\":null,\"isInvalidated\":false,\"status\":\"success\",\"fetchStatus\":\"idle\"},\"queryKey\":[\"paper\",\"2102.11742\",\"metadata\"],\"queryHash\":\"[\\\"paper\\\",\\\"2102.11742\\\",\\\"metadata\\\"]\"},{\"state\":{\"data\":{\"data\":[]},\"dataUpdateCount\":1,\"dataUpdatedAt\":1744228196923,\"error\":null,\"errorUpdateCount\":0,\"errorUpdatedAt\":0,\"fetchFailureCount\":0,\"fetchFailureReason\":null,\"fetchMeta\":null,\"isInvalidated\":false,\"status\":\"success\",\"fetchStatus\":\"idle\"},\"queryKey\":[\"paper\",\"2102.11742\",\"comments\"],\"queryHash\":\"[\\\"paper\\\",\\\"2102.11742\\\",\\\"comments\\\"]\"},{\"state\":{\"data\":{\"data\":{\"paper_version\":{\"_id\":\"6751ea88d05836936a6fb655\",\"paper_group_id\":\"6751ea88d05836936a6fb654\",\"version_label\":\"v2\",\"version_order\":2,\"title\":\"Dimensionality Reduction for Wasserstein Barycenter\",\"abstract\":\"$4e\",\"author_ids\":[\"6734162429b032f35709a7fa\",\"673230decd1e32a6e7f0b9fe\",\"673228f3cd1e32a6e7f04168\"],\"publication_date\":\"2021-10-19T01:10:17.000Z\",\"license\":\"http://creativecommons.org/licenses/by/4.0/\",\"created_at\":\"2024-12-05T18:01:44.495Z\",\"updated_at\":\"2024-12-05T18:01:44.495Z\",\"is_deleted\":false,\"is_hidden\":false,\"universal_paper_id\":\"2110.08991\",\"imageURL\":\"image/2110.08991v2.png\"},\"paper_group\":{\"_id\":\"6751ea88d05836936a6fb654\",\"universal_paper_id\":\"2110.08991\",\"source\":{\"name\":\"arXiv\",\"url\":\"https://arXiv.org/paper/2110.08991\"},\"title\":\"Dimensionality Reduction for Wasserstein Barycenter\",\"created_at\":\"2024-12-05T18:01:33.198Z\",\"updated_at\":\"2025-03-03T19:39:15.676Z\",\"categories\":[\"Computer Science\",\"Mathematics\"],\"subcategories\":[\"cs.DS\",\"cs.LG\",\"math.PR\"],\"custom_categories\":null,\"author_user_ids\":[\"678892ea3f2bbf6b859e9091\"],\"metrics\":{\"activity_rank\":0,\"questions_count\":0,\"responses_count\":0,\"upvotes_count\":null,\"downvotes_count\":null,\"total_votes\":0,\"visits_count\":{\"last24Hours\":0,\"last7Days\":2,\"last30Days\":4,\"last90Days\":14,\"all\":58},\"weighted_visits\":{\"last24Hours\":0,\"last7Days\":0.002242283119243206,\"last30Days\":0.8196858349310829,\"last90Days\":8.253861591115824,\"hot\":0.002242283119243206},\"public_total_votes\":1,\"timeline\":[{\"date\":\"2025-04-02T23:48:41.217Z\",\"views\":3},{\"date\":\"2025-03-30T11:48:41.217Z\",\"views\":3},{\"date\":\"2025-03-26T23:48:41.217Z\",\"views\":5},{\"date\":\"2025-03-23T11:48:41.217Z\",\"views\":0},{\"date\":\"2025-03-19T23:48:41.217Z\",\"views\":1},{\"date\":\"2025-03-16T11:48:41.217Z\",\"views\":1},{\"date\":\"2025-03-12T23:48:41.217Z\",\"views\":5},{\"date\":\"2025-03-09T11:48:41.217Z\",\"views\":0},{\"date\":\"2025-03-05T23:48:41.217Z\",\"views\":4},{\"date\":\"2025-03-02T11:48:41.217Z\",\"views\":1},{\"date\":\"2025-02-26T23:48:41.217Z\",\"views\":0},{\"date\":\"2025-02-23T11:48:41.217Z\",\"views\":1},{\"date\":\"2025-02-19T23:48:41.242Z\",\"views\":0},{\"date\":\"2025-02-16T11:48:41.262Z\",\"views\":2},{\"date\":\"2025-02-12T23:48:41.286Z\",\"views\":0},{\"date\":\"2025-02-09T11:48:41.309Z\",\"views\":5},{\"date\":\"2025-02-05T23:48:41.320Z\",\"views\":2},{\"date\":\"2025-02-02T11:48:41.333Z\",\"views\":2},{\"date\":\"2025-01-29T23:48:41.355Z\",\"views\":4},{\"date\":\"2025-01-26T11:48:41.377Z\",\"views\":0},{\"date\":\"2025-01-22T23:48:41.410Z\",\"views\":0},{\"date\":\"2025-01-19T11:48:41.435Z\",\"views\":2},{\"date\":\"2025-01-15T23:48:41.464Z\",\"views\":7},{\"date\":\"2025-01-12T11:48:41.486Z\",\"views\":4},{\"date\":\"2025-01-08T23:48:41.513Z\",\"views\":0},{\"date\":\"2025-01-05T11:48:41.529Z\",\"views\":13},{\"date\":\"2025-01-01T23:48:41.559Z\",\"views\":3},{\"date\":\"2024-12-29T11:48:41.583Z\",\"views\":5},{\"date\":\"2024-12-25T23:48:41.617Z\",\"views\":2},{\"date\":\"2024-12-22T11:48:41.641Z\",\"views\":1},{\"date\":\"2024-12-18T23:48:41.685Z\",\"views\":1},{\"date\":\"2024-12-15T11:48:41.708Z\",\"views\":1},{\"date\":\"2024-12-11T23:48:41.808Z\",\"views\":1},{\"date\":\"2024-12-08T11:48:41.874Z\",\"views\":4},{\"date\":\"2024-12-04T23:48:41.899Z\",\"views\":8}]},\"ranking\":{\"current_rank\":0,\"previous_rank\":0,\"activity_score\":0,\"paper_score\":0},\"is_hidden\":false,\"first_publication_date\":\"2024-12-05T18:01:44.146Z\",\"claimed_at\":\"2025-01-16T10:45:48.212Z\",\"resources\":{\"github\":{\"url\":\"https://github.com/decarpentierg/dr4wb\",\"description\":\"Dimensionality Reduction for Wasserstein Barycenter\",\"language\":\"Jupyter Notebook\",\"stars\":0}},\"organizations\":[\"67be6376aa92218ccd8b0f8e\",\"67be637aaa92218ccd8b1158\",\"67be6376aa92218ccd8b0f81\"],\"citation\":{\"bibtex\":\"@misc{zhou2021dimensionalityreductionwasserstein,\\n title={Dimensionality Reduction for Wasserstein Barycenter}, \\n author={Samson Zhou and Sandeep Silwal and Zachary Izzo},\\n year={2021},\\n eprint={2110.08991},\\n archivePrefix={arXiv},\\n primaryClass={cs.DS},\\n url={https://arxiv.org/abs/2110.08991}, \\n}\"},\"paperVersions\":{\"_id\":\"6751ea88d05836936a6fb655\",\"paper_group_id\":\"6751ea88d05836936a6fb654\",\"version_label\":\"v2\",\"version_order\":2,\"title\":\"Dimensionality Reduction for Wasserstein Barycenter\",\"abstract\":\"$4f\",\"author_ids\":[\"6734162429b032f35709a7fa\",\"673230decd1e32a6e7f0b9fe\",\"673228f3cd1e32a6e7f04168\"],\"publication_date\":\"2021-10-19T01:10:17.000Z\",\"license\":\"http://creativecommons.org/licenses/by/4.0/\",\"created_at\":\"2024-12-05T18:01:44.495Z\",\"updated_at\":\"2024-12-05T18:01:44.495Z\",\"is_deleted\":false,\"is_hidden\":false,\"universal_paper_id\":\"2110.08991\",\"imageURL\":\"image/2110.08991v2.png\"},\"verifiedAuthors\":[{\"_id\":\"678892ea3f2bbf6b859e9091\",\"useremail\":\"zle.izzo@gmail.com\",\"username\":\"Zach Izzo\",\"realname\":\"Zach Izzo\",\"slug\":\"zach-izzo\",\"totalupvotes\":0,\"numquestions\":0,\"numresponses\":0,\"followerCount\":0,\"followingUsers\":[],\"followingPapers\":[],\"following_paper_groups\":[\"6751ea88d05836936a6fb654\",\"6788e35a259e6494acd4b0d1\",\"6788e35a259e6494acd4b0d0\",\"6788e35c259e6494acd4b0d8\",\"6788e35d259e6494acd4b0dd\",\"6788e35e259e6494acd4b0e1\"],\"votedPapers\":[],\"email_settings\":{\"direct_notifications\":true,\"relevant_activity\":true},\"claimed_paper_groups\":[\"673cf13bbdf5ad128bc1686c\",\"6734162329b032f35709a7f9\",\"6751ea88d05836936a6fb654\",\"6788e35a259e6494acd4b0d1\",\"6788e35b259e6494acd4b0d3\",\"6788e35a259e6494acd4b0d0\",\"6788e35b259e6494acd4b0d2\",\"6788e35c259e6494acd4b0d8\",\"6788e35c259e6494acd4b0d6\",\"6788e35d259e6494acd4b0dd\",\"6788e35e259e6494acd4b0e1\"],\"voted_paper_groups\":[],\"biography\":\"\",\"daysActive\":0,\"reputation\":15,\"weeklyReputation\":0,\"usernameChanged\":false,\"firstLogin\":true,\"subscribedPotw\":false,\"orcid_id\":\"\",\"gscholar_id\":\"K9XheYUAAAAJ\",\"role\":\"user\",\"institution\":null,\"interests\":{\"categories\":[],\"subcategories\":[{\"name\":\"cs.LG\",\"score\":484},{\"name\":\"cs.CV\",\"score\":44},{\"name\":\"stat.ML\",\"score\":43},{\"name\":\"cs.CL\",\"score\":33},{\"name\":\"cs.AI\",\"score\":31},{\"name\":\"stat.ME\",\"score\":25},{\"name\":\"cs.NE\",\"score\":6},{\"name\":\"cs.CR\",\"score\":5},{\"name\":\"math.OC\",\"score\":2},{\"name\":\"cs.GR\",\"score\":2},{\"name\":\"cs.SI\",\"score\":1},{\"name\":\"cs.DS\",\"score\":1},{\"name\":\"math.PR\",\"score\":1},{\"name\":\"math.ST\",\"score\":1},{\"name\":\"math.NA\",\"score\":1}],\"custom_categories\":[{\"name\":\"optimization-methods\",\"score\":430},{\"name\":\"statistical-learning\",\"score\":425},{\"name\":\"representation-learning\",\"score\":424},{\"name\":\"generative-models\",\"score\":399},{\"name\":\"transformers\",\"score\":37},{\"name\":\"reinforcement-learning\",\"score\":34},{\"name\":\"reasoning\",\"score\":32},{\"name\":\"fine-tuning\",\"score\":32},{\"name\":\"knowledge-distillation\",\"score\":32},{\"name\":\"parameter-efficient-training\",\"score\":32},{\"name\":\"agents\",\"score\":32},{\"name\":\"unsupervised-learning\",\"score\":27},{\"name\":\"geometric-deep-learning\",\"score\":25},{\"name\":\"adversarial-robustness\",\"score\":13},{\"name\":\"adversarial-attacks\",\"score\":12},{\"name\":\"model-interpretation\",\"score\":10},{\"name\":\"transfer-learning\",\"score\":9},{\"name\":\"deep-reinforcement-learning\",\"score\":5},{\"name\":\"computer-vision-security\",\"score\":4},{\"name\":\"image-generation\",\"score\":4},{\"name\":\"attention-mechanisms\",\"score\":4},{\"name\":\"image-recognition\",\"score\":4},{\"name\":\"computer-vision\",\"score\":4},{\"name\":\"online-learning\",\"score\":3},{\"name\":\"privacy-preserving-ml\",\"score\":2},{\"name\":\"domain-adaptation\",\"score\":2},{\"name\":\"explainable-ai\",\"score\":2},{\"name\":\"uncertainty-estimation\",\"score\":2},{\"name\":\"neural-architecture-search\",\"score\":2},{\"name\":\"continual-learning\",\"score\":2},{\"name\":\"multi-view-learning\",\"score\":2},{\"name\":\"self-supervised-learning\",\"score\":2},{\"name\":\"natural-language-processing\",\"score\":1},{\"name\":\"large-language-models\",\"score\":1},{\"name\":\"machine-learning-applications\",\"score\":1},{\"name\":\"text-classification\",\"score\":1},{\"name\":\"machine-learning\",\"score\":1},{\"name\":\"efficient-training\",\"score\":1},{\"name\":\"model-compression\",\"score\":1},{\"name\":\"clustering-algorithms\",\"score\":1},{\"name\":\"causal-inference\",\"score\":1},{\"name\":\"differential-privacy\",\"score\":1},{\"name\":\"ai-for-health\",\"score\":1},{\"name\":\"bayesian-optimization\",\"score\":1},{\"name\":\"semi-supervised-learning\",\"score\":1},{\"name\":\"weak-supervision\",\"score\":1}]},\"created_at\":\"2025-01-16T05:02:34.081Z\",\"semantic_scholar\":{\"id\":\"1502231522\"},\"preferences\":{\"communities_order\":{\"communities\":[],\"global_community_index\":0},\"model\":\"gemini-2.0-flash\",\"folders\":[{\"folder_id\":\"67ad611bd4568bf90d8551f6\",\"opened\":false},{\"folder_id\":\"67ad611bd4568bf90d8551f7\",\"opened\":false},{\"folder_id\":\"67ad611bd4568bf90d8551f8\",\"opened\":false},{\"folder_id\":\"67ad611bd4568bf90d8551f9\",\"opened\":false}],\"show_my_communities_in_sidebar\":true,\"enable_dark_mode\":false,\"current_community_slug\":\"global\",\"topic_preferences\":[],\"paper_right_sidebar_tab\":\"comments\"},\"following_orgs\":[],\"following_topics\":[]}],\"authors\":[{\"_id\":\"673228f3cd1e32a6e7f04168\",\"full_name\":\"Samson Zhou\",\"affiliation\":null,\"orcid\":null,\"semantic_scholarid\":null,\"user_id\":null},{\"_id\":\"673230decd1e32a6e7f0b9fe\",\"full_name\":\"Sandeep Silwal\",\"affiliation\":null,\"orcid\":null,\"semantic_scholarid\":null,\"user_id\":null},{\"_id\":\"6734162429b032f35709a7fa\",\"full_name\":\"Zachary Izzo\",\"affiliation\":null,\"orcid\":null,\"semantic_scholarid\":null,\"user_id\":null}]},\"max_version_order\":2,\"verified_authors\":[{\"_id\":\"678892ea3f2bbf6b859e9091\",\"useremail\":\"zle.izzo@gmail.com\",\"username\":\"Zach Izzo\",\"realname\":\"Zach Izzo\",\"slug\":\"zach-izzo\",\"totalupvotes\":0,\"numquestions\":0,\"numresponses\":0,\"followerCount\":0,\"followingUsers\":[],\"followingPapers\":[],\"following_paper_groups\":[\"6751ea88d05836936a6fb654\",\"6788e35a259e6494acd4b0d1\",\"6788e35a259e6494acd4b0d0\",\"6788e35c259e6494acd4b0d8\",\"6788e35d259e6494acd4b0dd\",\"6788e35e259e6494acd4b0e1\"],\"votedPapers\":[],\"email_settings\":{\"direct_notifications\":true,\"relevant_activity\":true},\"claimed_paper_groups\":[\"673cf13bbdf5ad128bc1686c\",\"6734162329b032f35709a7f9\",\"6751ea88d05836936a6fb654\",\"6788e35a259e6494acd4b0d1\",\"6788e35b259e6494acd4b0d3\",\"6788e35a259e6494acd4b0d0\",\"6788e35b259e6494acd4b0d2\",\"6788e35c259e6494acd4b0d8\",\"6788e35c259e6494acd4b0d6\",\"6788e35d259e6494acd4b0dd\",\"6788e35e259e6494acd4b0e1\"],\"voted_paper_groups\":[],\"biography\":\"\",\"daysActive\":0,\"reputation\":15,\"weeklyReputation\":0,\"usernameChanged\":false,\"firstLogin\":true,\"subscribedPotw\":false,\"orcid_id\":\"\",\"gscholar_id\":\"K9XheYUAAAAJ\",\"role\":\"user\",\"institution\":null,\"interests\":{\"categories\":[],\"subcategories\":[{\"name\":\"cs.LG\",\"score\":484},{\"name\":\"cs.CV\",\"score\":44},{\"name\":\"stat.ML\",\"score\":43},{\"name\":\"cs.CL\",\"score\":33},{\"name\":\"cs.AI\",\"score\":31},{\"name\":\"stat.ME\",\"score\":25},{\"name\":\"cs.NE\",\"score\":6},{\"name\":\"cs.CR\",\"score\":5},{\"name\":\"math.OC\",\"score\":2},{\"name\":\"cs.GR\",\"score\":2},{\"name\":\"cs.SI\",\"score\":1},{\"name\":\"cs.DS\",\"score\":1},{\"name\":\"math.PR\",\"score\":1},{\"name\":\"math.ST\",\"score\":1},{\"name\":\"math.NA\",\"score\":1}],\"custom_categories\":[{\"name\":\"optimization-methods\",\"score\":430},{\"name\":\"statistical-learning\",\"score\":425},{\"name\":\"representation-learning\",\"score\":424},{\"name\":\"generative-models\",\"score\":399},{\"name\":\"transformers\",\"score\":37},{\"name\":\"reinforcement-learning\",\"score\":34},{\"name\":\"reasoning\",\"score\":32},{\"name\":\"fine-tuning\",\"score\":32},{\"name\":\"knowledge-distillation\",\"score\":32},{\"name\":\"parameter-efficient-training\",\"score\":32},{\"name\":\"agents\",\"score\":32},{\"name\":\"unsupervised-learning\",\"score\":27},{\"name\":\"geometric-deep-learning\",\"score\":25},{\"name\":\"adversarial-robustness\",\"score\":13},{\"name\":\"adversarial-attacks\",\"score\":12},{\"name\":\"model-interpretation\",\"score\":10},{\"name\":\"transfer-learning\",\"score\":9},{\"name\":\"deep-reinforcement-learning\",\"score\":5},{\"name\":\"computer-vision-security\",\"score\":4},{\"name\":\"image-generation\",\"score\":4},{\"name\":\"attention-mechanisms\",\"score\":4},{\"name\":\"image-recognition\",\"score\":4},{\"name\":\"computer-vision\",\"score\":4},{\"name\":\"online-learning\",\"score\":3},{\"name\":\"privacy-preserving-ml\",\"score\":2},{\"name\":\"domain-adaptation\",\"score\":2},{\"name\":\"explainable-ai\",\"score\":2},{\"name\":\"uncertainty-estimation\",\"score\":2},{\"name\":\"neural-architecture-search\",\"score\":2},{\"name\":\"continual-learning\",\"score\":2},{\"name\":\"multi-view-learning\",\"score\":2},{\"name\":\"self-supervised-learning\",\"score\":2},{\"name\":\"natural-language-processing\",\"score\":1},{\"name\":\"large-language-models\",\"score\":1},{\"name\":\"machine-learning-applications\",\"score\":1},{\"name\":\"text-classification\",\"score\":1},{\"name\":\"machine-learning\",\"score\":1},{\"name\":\"efficient-training\",\"score\":1},{\"name\":\"model-compression\",\"score\":1},{\"name\":\"clustering-algorithms\",\"score\":1},{\"name\":\"causal-inference\",\"score\":1},{\"name\":\"differential-privacy\",\"score\":1},{\"name\":\"ai-for-health\",\"score\":1},{\"name\":\"bayesian-optimization\",\"score\":1},{\"name\":\"semi-supervised-learning\",\"score\":1},{\"name\":\"weak-supervision\",\"score\":1}]},\"created_at\":\"2025-01-16T05:02:34.081Z\",\"semantic_scholar\":{\"id\":\"1502231522\"},\"preferences\":{\"communities_order\":{\"communities\":[],\"global_community_index\":0},\"model\":\"gemini-2.0-flash\",\"folders\":[{\"folder_id\":\"67ad611bd4568bf90d8551f6\",\"opened\":false},{\"folder_id\":\"67ad611bd4568bf90d8551f7\",\"opened\":false},{\"folder_id\":\"67ad611bd4568bf90d8551f8\",\"opened\":false},{\"folder_id\":\"67ad611bd4568bf90d8551f9\",\"opened\":false}],\"show_my_communities_in_sidebar\":true,\"enable_dark_mode\":false,\"current_community_slug\":\"global\",\"topic_preferences\":[],\"paper_right_sidebar_tab\":\"comments\"},\"following_orgs\":[],\"following_topics\":[]}],\"authors\":[{\"_id\":\"673228f3cd1e32a6e7f04168\",\"full_name\":\"Samson Zhou\",\"affiliation\":null,\"orcid\":null,\"semantic_scholarid\":null,\"user_id\":null},{\"_id\":\"673230decd1e32a6e7f0b9fe\",\"full_name\":\"Sandeep Silwal\",\"affiliation\":null,\"orcid\":null,\"semantic_scholarid\":null,\"user_id\":null},{\"_id\":\"6734162429b032f35709a7fa\",\"full_name\":\"Zachary Izzo\",\"affiliation\":null,\"orcid\":null,\"semantic_scholarid\":null,\"user_id\":null}],\"pdf_info\":{\"fetcher_url\":\"https://fetcher.alphaxiv.org/v2/pdf/2110.08991v2\"}}},\"dataUpdateCount\":1,\"dataUpdatedAt\":1744228197107,\"error\":null,\"errorUpdateCount\":0,\"errorUpdatedAt\":0,\"fetchFailureCount\":0,\"fetchFailureReason\":null,\"fetchMeta\":null,\"isInvalidated\":false,\"status\":\"success\",\"fetchStatus\":\"idle\"},\"queryKey\":[\"paper\",\"2110.08991\",\"metadata\"],\"queryHash\":\"[\\\"paper\\\",\\\"2110.08991\\\",\\\"metadata\\\"]\"},{\"state\":{\"data\":{\"data\":[]},\"dataUpdateCount\":1,\"dataUpdatedAt\":1744228197107,\"error\":null,\"errorUpdateCount\":0,\"errorUpdatedAt\":0,\"fetchFailureCount\":0,\"fetchFailureReason\":null,\"fetchMeta\":null,\"isInvalidated\":false,\"status\":\"success\",\"fetchStatus\":\"idle\"},\"queryKey\":[\"paper\",\"2110.08991\",\"comments\"],\"queryHash\":\"[\\\"paper\\\",\\\"2110.08991\\\",\\\"comments\\\"]\"},{\"state\":{\"data\":{\"data\":{\"paper_version\":{\"_id\":\"676f67ca90f035bff48797f3\",\"paper_group_id\":\"676f67c890f035bff48797ef\",\"version_label\":\"v1\",\"version_order\":1,\"title\":\"Global optimization in the discrete and variable-dimension conformational space: The case of crystal with the strongest atomic cohesion\",\"abstract\":\"We introduce a computational method to optimize target physical properties in the full configuration space regarding atomic composition, chemical stoichiometry, and crystal structure. The approach combines the universal potential of the crystal graph neural network and Bayesian optimization. The proposed approach effectively obtains the crystal structure with the strongest atomic cohesion from all possible crystals. Several new crystals with high atomic cohesion are identified and confirmed by density functional theory for thermodynamic and dynamic stability. Our method introduces a novel approach to inverse materials design with additional functional properties for practical applications.\",\"author_ids\":[\"676f67c990f035bff48797f1\",\"6749045d9c393cb8c0590b2b\",\"675597fab33ed443d9b17c6d\"],\"publication_date\":\"2023-02-27T06:26:09.000Z\",\"license\":\"http://creativecommons.org/licenses/by/4.0/\",\"created_at\":\"2024-12-28T02:51:54.300Z\",\"updated_at\":\"2024-12-28T02:51:54.300Z\",\"is_deleted\":false,\"is_hidden\":false,\"universal_paper_id\":\"2302.13537\",\"imageURL\":\"image/2302.13537v1.png\"},\"paper_group\":{\"_id\":\"676f67c890f035bff48797ef\",\"universal_paper_id\":\"2302.13537\",\"title\":\"Global optimization in the discrete and variable-dimension conformational space: The case of crystal with the strongest atomic cohesion\",\"created_at\":\"2024-12-28T02:51:52.932Z\",\"updated_at\":\"2025-03-03T20:19:58.703Z\",\"categories\":[\"Physics\",\"Computer Science\"],\"subcategories\":[\"cond-mat.mtrl-sci\",\"cs.LG\"],\"custom_categories\":[\"bayesian-optimization\",\"graph-neural-networks\",\"optimization-methods\"],\"author_user_ids\":[],\"source\":{\"name\":\"alphaXiv\",\"url\":\"https://arxiv.org/paper/2302.13537\"},\"metrics\":{\"activity_rank\":0,\"questions_count\":0,\"responses_count\":0,\"upvotes_count\":0,\"downvotes_count\":0,\"total_votes\":0,\"visits_count\":{\"last24Hours\":0,\"last7Days\":0,\"last30Days\":2,\"last90Days\":2,\"all\":4},\"weighted_visits\":{\"last24Hours\":0,\"last7Days\":0,\"last30Days\":0.0000728698011521694,\"last90Days\":0.06630340904363942,\"hot\":0},\"public_total_votes\":0,\"timeline\":[{\"date\":\"2025-04-03T02:01:29.082Z\",\"views\":1},{\"date\":\"2025-03-30T14:01:29.082Z\",\"views\":0},{\"date\":\"2025-03-27T02:01:29.082Z\",\"views\":5},{\"date\":\"2025-03-23T14:01:29.082Z\",\"views\":1},{\"date\":\"2025-03-20T02:01:29.082Z\",\"views\":1},{\"date\":\"2025-03-16T14:01:29.082Z\",\"views\":5},{\"date\":\"2025-03-13T02:01:29.082Z\",\"views\":0},{\"date\":\"2025-03-09T14:01:29.082Z\",\"views\":1},{\"date\":\"2025-03-06T02:01:29.082Z\",\"views\":2},{\"date\":\"2025-03-02T14:01:29.082Z\",\"views\":1},{\"date\":\"2025-02-27T02:01:29.082Z\",\"views\":1},{\"date\":\"2025-02-23T14:01:29.082Z\",\"views\":2},{\"date\":\"2025-02-20T02:01:29.109Z\",\"views\":1},{\"date\":\"2025-02-16T14:01:29.159Z\",\"views\":1},{\"date\":\"2025-02-13T02:01:29.213Z\",\"views\":0},{\"date\":\"2025-02-09T14:01:29.282Z\",\"views\":0},{\"date\":\"2025-02-06T02:01:29.329Z\",\"views\":2},{\"date\":\"2025-02-02T14:01:29.380Z\",\"views\":1},{\"date\":\"2025-01-30T02:01:29.444Z\",\"views\":1},{\"date\":\"2025-01-26T14:01:29.550Z\",\"views\":2},{\"date\":\"2025-01-23T02:01:29.601Z\",\"views\":0},{\"date\":\"2025-01-19T14:01:29.695Z\",\"views\":1},{\"date\":\"2025-01-16T02:01:29.759Z\",\"views\":2},{\"date\":\"2025-01-12T14:01:29.806Z\",\"views\":0},{\"date\":\"2025-01-09T02:01:29.866Z\",\"views\":1},{\"date\":\"2025-01-05T14:01:29.920Z\",\"views\":0},{\"date\":\"2025-01-02T02:01:29.971Z\",\"views\":3},{\"date\":\"2024-12-29T14:01:30.023Z\",\"views\":2},{\"date\":\"2024-12-26T02:01:30.085Z\",\"views\":4},{\"date\":\"2024-12-22T14:01:30.142Z\",\"views\":2},{\"date\":\"2024-12-19T02:01:30.193Z\",\"views\":1},{\"date\":\"2024-12-15T14:01:30.266Z\",\"views\":0},{\"date\":\"2024-12-12T02:01:30.296Z\",\"views\":2},{\"date\":\"2024-12-08T14:01:30.334Z\",\"views\":1},{\"date\":\"2024-12-05T02:01:30.402Z\",\"views\":1},{\"date\":\"2024-12-01T14:01:30.447Z\",\"views\":2},{\"date\":\"2024-11-28T02:01:30.497Z\",\"views\":1},{\"date\":\"2024-11-24T14:01:30.538Z\",\"views\":0},{\"date\":\"2024-11-21T02:01:30.583Z\",\"views\":1},{\"date\":\"2024-11-17T14:01:30.634Z\",\"views\":0},{\"date\":\"2024-11-14T02:01:30.680Z\",\"views\":0},{\"date\":\"2024-11-10T14:01:30.728Z\",\"views\":2},{\"date\":\"2024-11-07T02:01:30.803Z\",\"views\":0},{\"date\":\"2024-11-03T14:01:30.842Z\",\"views\":0},{\"date\":\"2024-10-31T01:01:30.901Z\",\"views\":0},{\"date\":\"2024-10-27T13:01:30.949Z\",\"views\":1},{\"date\":\"2024-10-24T01:01:30.988Z\",\"views\":2},{\"date\":\"2024-10-20T13:01:31.030Z\",\"views\":2},{\"date\":\"2024-10-17T01:01:31.088Z\",\"views\":1},{\"date\":\"2024-10-13T13:01:31.174Z\",\"views\":2},{\"date\":\"2024-10-10T01:01:31.232Z\",\"views\":2},{\"date\":\"2024-10-06T13:01:31.285Z\",\"views\":2},{\"date\":\"2024-10-03T01:01:31.359Z\",\"views\":0},{\"date\":\"2024-09-29T13:01:31.407Z\",\"views\":0},{\"date\":\"2024-09-26T01:01:31.485Z\",\"views\":0},{\"date\":\"2024-09-22T13:01:31.549Z\",\"views\":1},{\"date\":\"2024-09-19T01:01:31.622Z\",\"views\":2},{\"date\":\"2024-09-15T13:01:31.644Z\",\"views\":0},{\"date\":\"2024-09-12T01:01:31.686Z\",\"views\":0},{\"date\":\"2024-09-08T13:01:31.790Z\",\"views\":2},{\"date\":\"2024-09-05T01:01:31.857Z\",\"views\":1},{\"date\":\"2024-09-01T13:01:31.907Z\",\"views\":0},{\"date\":\"2024-08-29T01:01:31.944Z\",\"views\":1}]},\"is_hidden\":false,\"first_publication_date\":\"2023-02-27T06:26:09.000Z\",\"paperVersions\":{\"_id\":\"676f67ca90f035bff48797f3\",\"paper_group_id\":\"676f67c890f035bff48797ef\",\"version_label\":\"v1\",\"version_order\":1,\"title\":\"Global optimization in the discrete and variable-dimension conformational space: The case of crystal with the strongest atomic cohesion\",\"abstract\":\"We introduce a computational method to optimize target physical properties in the full configuration space regarding atomic composition, chemical stoichiometry, and crystal structure. The approach combines the universal potential of the crystal graph neural network and Bayesian optimization. The proposed approach effectively obtains the crystal structure with the strongest atomic cohesion from all possible crystals. Several new crystals with high atomic cohesion are identified and confirmed by density functional theory for thermodynamic and dynamic stability. Our method introduces a novel approach to inverse materials design with additional functional properties for practical applications.\",\"author_ids\":[\"676f67c990f035bff48797f1\",\"6749045d9c393cb8c0590b2b\",\"675597fab33ed443d9b17c6d\"],\"publication_date\":\"2023-02-27T06:26:09.000Z\",\"license\":\"http://creativecommons.org/licenses/by/4.0/\",\"created_at\":\"2024-12-28T02:51:54.300Z\",\"updated_at\":\"2024-12-28T02:51:54.300Z\",\"is_deleted\":false,\"is_hidden\":false,\"universal_paper_id\":\"2302.13537\",\"imageURL\":\"image/2302.13537v1.png\"},\"verifiedAuthors\":[],\"authors\":[{\"_id\":\"6749045d9c393cb8c0590b2b\",\"full_name\":\"Xin-Gao Gong\",\"affiliation\":null,\"orcid\":null,\"semantic_scholarid\":null,\"user_id\":null},{\"_id\":\"675597fab33ed443d9b17c6d\",\"full_name\":\"Wan-Jian Yin\",\"affiliation\":null,\"orcid\":null,\"semantic_scholarid\":null,\"user_id\":null},{\"_id\":\"676f67c990f035bff48797f1\",\"full_name\":\"Guanjian Cheng\",\"affiliation\":null,\"orcid\":null,\"semantic_scholarid\":null,\"user_id\":null}]},\"max_version_order\":1,\"verified_authors\":[],\"authors\":[{\"_id\":\"6749045d9c393cb8c0590b2b\",\"full_name\":\"Xin-Gao Gong\",\"affiliation\":null,\"orcid\":null,\"semantic_scholarid\":null,\"user_id\":null},{\"_id\":\"675597fab33ed443d9b17c6d\",\"full_name\":\"Wan-Jian Yin\",\"affiliation\":null,\"orcid\":null,\"semantic_scholarid\":null,\"user_id\":null},{\"_id\":\"676f67c990f035bff48797f1\",\"full_name\":\"Guanjian Cheng\",\"affiliation\":null,\"orcid\":null,\"semantic_scholarid\":null,\"user_id\":null}],\"pdf_info\":{\"fetcher_url\":\"https://fetcher.alphaxiv.org/v2/pdf/2302.13537v1\"}}},\"dataUpdateCount\":1,\"dataUpdatedAt\":1744228197208,\"error\":null,\"errorUpdateCount\":0,\"errorUpdatedAt\":0,\"fetchFailureCount\":0,\"fetchFailureReason\":null,\"fetchMeta\":null,\"isInvalidated\":false,\"status\":\"success\",\"fetchStatus\":\"idle\"},\"queryKey\":[\"paper\",\"2302.13537\",\"metadata\"],\"queryHash\":\"[\\\"paper\\\",\\\"2302.13537\\\",\\\"metadata\\\"]\"},{\"state\":{\"data\":{\"data\":[]},\"dataUpdateCount\":1,\"dataUpdatedAt\":1744228197208,\"error\":null,\"errorUpdateCount\":0,\"errorUpdatedAt\":0,\"fetchFailureCount\":0,\"fetchFailureReason\":null,\"fetchMeta\":null,\"isInvalidated\":false,\"status\":\"success\",\"fetchStatus\":\"idle\"},\"queryKey\":[\"paper\",\"2302.13537\",\"comments\"],\"queryHash\":\"[\\\"paper\\\",\\\"2302.13537\\\",\\\"comments\\\"]\"},{\"state\":{\"data\":{\"data\":{\"paper_version\":{\"_id\":\"6773a8cb1f7590a207a510ed\",\"paper_group_id\":\"6773a8cb1f7590a207a510ec\",\"version_label\":\"v1\",\"version_order\":1,\"title\":\"Distributionally Robust Optimization via Iterative Algorithms in Continuous Probability Spaces\",\"abstract\":\"$50\",\"author_ids\":[\"673b8112bf626fe16b8a9453\",\"672bd160986a1370676e15b3\"],\"publication_date\":\"2024-12-29T19:31:23.000Z\",\"license\":\"http://arxiv.org/licenses/nonexclusive-distrib/1.0/\",\"created_at\":\"2024-12-31T08:18:19.367Z\",\"updated_at\":\"2024-12-31T08:18:19.367Z\",\"is_deleted\":false,\"is_hidden\":false,\"universal_paper_id\":\"2412.20556\",\"imageURL\":\"image/2412.20556v1.png\"},\"paper_group\":{\"_id\":\"6773a8cb1f7590a207a510ec\",\"universal_paper_id\":\"2412.20556\",\"title\":\"Distributionally Robust Optimization via Iterative Algorithms in Continuous Probability Spaces\",\"created_at\":\"2024-12-31T08:18:19.085Z\",\"updated_at\":\"2025-03-03T19:38:07.467Z\",\"categories\":[\"Statistics\",\"Computer Science\",\"Mathematics\"],\"subcategories\":[\"stat.ML\",\"cs.LG\",\"math.OC\"],\"custom_categories\":[\"optimization-methods\",\"statistical-learning\",\"uncertainty-estimation\",\"deep-reinforcement-learning\",\"probabilistic-programming\"],\"author_user_ids\":[],\"source\":{\"name\":\"alphaXiv\",\"url\":\"https://arxiv.org/paper/2412.20556\"},\"metrics\":{\"activity_rank\":0,\"questions_count\":0,\"responses_count\":0,\"upvotes_count\":0,\"downvotes_count\":0,\"total_votes\":0,\"visits_count\":{\"last24Hours\":0,\"last7Days\":3,\"last30Days\":4,\"last90Days\":4,\"all\":34},\"weighted_visits\":{\"last24Hours\":0,\"last7Days\":0.013309391223041724,\"last30Days\":1.1298876884651041,\"last90Days\":2.624531279112944,\"hot\":0.013309391223041724},\"public_total_votes\":1,\"timeline\":[{\"date\":\"2025-04-02T23:43:13.427Z\",\"views\":10},{\"date\":\"2025-03-30T11:43:13.427Z\",\"views\":0},{\"date\":\"2025-03-26T23:43:13.427Z\",\"views\":5},{\"date\":\"2025-03-23T11:43:13.427Z\",\"views\":2},{\"date\":\"2025-03-19T23:43:13.427Z\",\"views\":1},{\"date\":\"2025-03-16T11:43:13.427Z\",\"views\":0},{\"date\":\"2025-03-12T23:43:13.427Z\",\"views\":2},{\"date\":\"2025-03-09T11:43:13.427Z\",\"views\":0},{\"date\":\"2025-03-05T23:43:13.427Z\",\"views\":1},{\"date\":\"2025-03-02T11:43:13.427Z\",\"views\":2},{\"date\":\"2025-02-26T23:43:13.427Z\",\"views\":2},{\"date\":\"2025-02-23T11:43:13.427Z\",\"views\":2},{\"date\":\"2025-02-19T23:43:13.461Z\",\"views\":2},{\"date\":\"2025-02-16T11:43:13.491Z\",\"views\":0},{\"date\":\"2025-02-12T23:43:13.526Z\",\"views\":2},{\"date\":\"2025-02-09T11:43:13.558Z\",\"views\":1},{\"date\":\"2025-02-05T23:43:13.584Z\",\"views\":2},{\"date\":\"2025-02-02T11:43:13.614Z\",\"views\":0},{\"date\":\"2025-01-29T23:43:13.668Z\",\"views\":1},{\"date\":\"2025-01-26T11:43:13.712Z\",\"views\":2},{\"date\":\"2025-01-22T23:43:13.750Z\",\"views\":2},{\"date\":\"2025-01-19T11:43:13.780Z\",\"views\":2},{\"date\":\"2025-01-15T23:43:13.812Z\",\"views\":2},{\"date\":\"2025-01-12T11:43:13.848Z\",\"views\":0},{\"date\":\"2025-01-08T23:43:13.877Z\",\"views\":0},{\"date\":\"2025-01-05T11:43:13.908Z\",\"views\":1},{\"date\":\"2025-01-01T23:43:13.945Z\",\"views\":4},{\"date\":\"2024-12-29T11:43:13.967Z\",\"views\":20}]},\"is_hidden\":false,\"first_publication_date\":\"2024-12-29T19:31:23.000Z\",\"resources\":{\"github\":{\"url\":\"https://github.com/sayantann11/all-classification-templetes-for-ML\",\"description\":\"$51\",\"language\":\"Python\",\"stars\":226}},\"organizations\":[\"67be6377aa92218ccd8b101c\"],\"citation\":{\"bibtex\":\"@misc{xie2024distributionallyrobustoptimization,\\n title={Distributionally Robust Optimization via Iterative Algorithms in Continuous Probability Spaces}, \\n author={Yao Xie and Linglingzhi Zhu},\\n year={2024},\\n eprint={2412.20556},\\n archivePrefix={arXiv},\\n primaryClass={stat.ML},\\n url={https://arxiv.org/abs/2412.20556}, \\n}\"},\"paperVersions\":{\"_id\":\"6773a8cb1f7590a207a510ed\",\"paper_group_id\":\"6773a8cb1f7590a207a510ec\",\"version_label\":\"v1\",\"version_order\":1,\"title\":\"Distributionally Robust Optimization via Iterative Algorithms in Continuous Probability Spaces\",\"abstract\":\"$52\",\"author_ids\":[\"673b8112bf626fe16b8a9453\",\"672bd160986a1370676e15b3\"],\"publication_date\":\"2024-12-29T19:31:23.000Z\",\"license\":\"http://arxiv.org/licenses/nonexclusive-distrib/1.0/\",\"created_at\":\"2024-12-31T08:18:19.367Z\",\"updated_at\":\"2024-12-31T08:18:19.367Z\",\"is_deleted\":false,\"is_hidden\":false,\"universal_paper_id\":\"2412.20556\",\"imageURL\":\"image/2412.20556v1.png\"},\"verifiedAuthors\":[],\"authors\":[{\"_id\":\"672bd160986a1370676e15b3\",\"full_name\":\"Yao Xie\",\"affiliation\":null,\"orcid\":null,\"semantic_scholarid\":null,\"user_id\":null},{\"_id\":\"673b8112bf626fe16b8a9453\",\"full_name\":\"Linglingzhi Zhu\",\"affiliation\":null,\"orcid\":null,\"semantic_scholarid\":null,\"user_id\":null}]},\"max_version_order\":1,\"verified_authors\":[],\"authors\":[{\"_id\":\"672bd160986a1370676e15b3\",\"full_name\":\"Yao Xie\",\"affiliation\":null,\"orcid\":null,\"semantic_scholarid\":null,\"user_id\":null},{\"_id\":\"673b8112bf626fe16b8a9453\",\"full_name\":\"Linglingzhi Zhu\",\"affiliation\":null,\"orcid\":null,\"semantic_scholarid\":null,\"user_id\":null}],\"pdf_info\":{\"fetcher_url\":\"https://fetcher.alphaxiv.org/v2/pdf/2412.20556v1\"}}},\"dataUpdateCount\":1,\"dataUpdatedAt\":1744228198028,\"error\":null,\"errorUpdateCount\":0,\"errorUpdatedAt\":0,\"fetchFailureCount\":0,\"fetchFailureReason\":null,\"fetchMeta\":null,\"isInvalidated\":false,\"status\":\"success\",\"fetchStatus\":\"idle\"},\"queryKey\":[\"paper\",\"2412.20556\",\"metadata\"],\"queryHash\":\"[\\\"paper\\\",\\\"2412.20556\\\",\\\"metadata\\\"]\"},{\"state\":{\"data\":{\"data\":[]},\"dataUpdateCount\":1,\"dataUpdatedAt\":1744228198028,\"error\":null,\"errorUpdateCount\":0,\"errorUpdatedAt\":0,\"fetchFailureCount\":0,\"fetchFailureReason\":null,\"fetchMeta\":null,\"isInvalidated\":false,\"status\":\"success\",\"fetchStatus\":\"idle\"},\"queryKey\":[\"paper\",\"2412.20556\",\"comments\"],\"queryHash\":\"[\\\"paper\\\",\\\"2412.20556\\\",\\\"comments\\\"]\"},{\"state\":{\"data\":{\"data\":{\"paper_version\":{\"_id\":\"6734ad1493ee437496011447\",\"paper_group_id\":\"6734ad1193ee437496011446\",\"version_label\":\"v1\",\"version_order\":1,\"title\":\"Multilingual Large Language Model: A Survey of Resources, Taxonomy and Frontiers\",\"abstract\":\"$53\",\"author_ids\":[\"672bd0a3986a1370676e0693\",\"672bd0a2986a1370676e068c\",\"672bd0f8986a1370676e0d0b\",\"672bd08f986a1370676e04ed\",\"672bcb5a986a1370676da349\",\"672bc9c7986a1370676d8d9f\",\"672bd109986a1370676e0e5a\",\"672bce42986a1370676dd58f\",\"672bbe1f986a1370676d5653\"],\"publication_date\":\"2024-04-07T11:52:44.000Z\",\"license\":\"http://arxiv.org/licenses/nonexclusive-distrib/1.0/\",\"created_at\":\"2024-11-13T13:43:48.170Z\",\"updated_at\":\"2024-11-13T13:43:48.170Z\",\"is_deleted\":false,\"is_hidden\":false,\"imageURL\":\"image/2404.04925v1.png\",\"universal_paper_id\":\"2404.04925\"},\"paper_group\":{\"_id\":\"6734ad1193ee437496011446\",\"universal_paper_id\":\"2404.04925\",\"source\":{\"name\":\"alphaXiv\",\"url\":\"https://alphaxiv.org/paper/2404.04925\"},\"title\":\"Multilingual Large Language Model: A Survey of Resources, Taxonomy and Frontiers\",\"created_at\":\"1970-01-01T00:00:00.000Z\",\"updated_at\":\"2025-03-03T19:56:15.848Z\",\"categories\":[\"Computer Science\"],\"subcategories\":[\"cs.CL\"],\"metrics\":{\"activity_rank\":0,\"questions_count\":0,\"responses_count\":0,\"upvotes_count\":null,\"downvotes_count\":0,\"total_votes\":0,\"visits_count\":{\"last24Hours\":2,\"last7Days\":19,\"last30Days\":35,\"last90Days\":62,\"all\":309},\"weighted_visits\":{\"last24Hours\":3.564486377094541e-63,\"last7Days\":2.063507369925358e-8,\"last30Days\":0.2834220335722513,\"last90Days\":12.450311326192281,\"hot\":2.063507369925358e-8},\"public_total_votes\":6,\"timeline\":[{\"date\":\"2025-04-02T01:57:17.640Z\",\"views\":34},{\"date\":\"2025-03-29T13:57:17.640Z\",\"views\":16},{\"date\":\"2025-03-26T01:57:17.640Z\",\"views\":11},{\"date\":\"2025-03-22T13:57:17.640Z\",\"views\":8},{\"date\":\"2025-03-19T01:57:17.640Z\",\"views\":11},{\"date\":\"2025-03-15T13:57:17.640Z\",\"views\":8},{\"date\":\"2025-03-12T01:57:17.640Z\",\"views\":4},{\"date\":\"2025-03-08T13:57:17.640Z\",\"views\":13},{\"date\":\"2025-03-05T01:57:17.640Z\",\"views\":14},{\"date\":\"2025-03-01T13:57:17.640Z\",\"views\":4},{\"date\":\"2025-02-26T01:57:17.640Z\",\"views\":12},{\"date\":\"2025-02-22T13:57:17.640Z\",\"views\":14},{\"date\":\"2025-02-19T01:57:17.657Z\",\"views\":10},{\"date\":\"2025-02-15T13:57:17.677Z\",\"views\":8},{\"date\":\"2025-02-12T01:57:17.697Z\",\"views\":1},{\"date\":\"2025-02-08T13:57:17.710Z\",\"views\":3},{\"date\":\"2025-02-05T01:57:17.724Z\",\"views\":12},{\"date\":\"2025-02-01T13:57:17.740Z\",\"views\":8},{\"date\":\"2025-01-29T01:57:17.757Z\",\"views\":5},{\"date\":\"2025-01-25T13:57:17.774Z\",\"views\":0},{\"date\":\"2025-01-22T01:57:17.794Z\",\"views\":1},{\"date\":\"2025-01-18T13:57:17.810Z\",\"views\":7},{\"date\":\"2025-01-15T01:57:17.826Z\",\"views\":1},{\"date\":\"2025-01-11T13:57:17.843Z\",\"views\":1},{\"date\":\"2025-01-08T01:57:17.860Z\",\"views\":0},{\"date\":\"2025-01-04T13:57:17.880Z\",\"views\":1},{\"date\":\"2025-01-01T01:57:17.899Z\",\"views\":9},{\"date\":\"2024-12-28T13:57:17.915Z\",\"views\":5},{\"date\":\"2024-12-25T01:57:17.929Z\",\"views\":7},{\"date\":\"2024-12-21T13:57:17.951Z\",\"views\":0},{\"date\":\"2024-12-18T01:57:17.982Z\",\"views\":2},{\"date\":\"2024-12-14T13:57:17.996Z\",\"views\":7},{\"date\":\"2024-12-11T01:57:18.011Z\",\"views\":4},{\"date\":\"2024-12-07T13:57:18.027Z\",\"views\":1},{\"date\":\"2024-12-04T01:57:18.045Z\",\"views\":4},{\"date\":\"2024-11-30T13:57:18.060Z\",\"views\":0},{\"date\":\"2024-11-27T01:57:18.082Z\",\"views\":5},{\"date\":\"2024-11-23T13:57:18.099Z\",\"views\":9},{\"date\":\"2024-11-20T01:57:18.113Z\",\"views\":11},{\"date\":\"2024-11-16T13:57:18.136Z\",\"views\":14},{\"date\":\"2024-11-13T01:57:18.153Z\",\"views\":6},{\"date\":\"2024-11-09T13:57:18.169Z\",\"views\":16},{\"date\":\"2024-11-06T01:57:18.186Z\",\"views\":3},{\"date\":\"2024-11-02T12:57:18.202Z\",\"views\":5},{\"date\":\"2024-10-30T00:57:18.217Z\",\"views\":15},{\"date\":\"2024-10-26T12:57:18.233Z\",\"views\":7},{\"date\":\"2024-10-23T00:57:18.261Z\",\"views\":1},{\"date\":\"2024-10-19T12:57:18.277Z\",\"views\":7},{\"date\":\"2024-10-16T00:57:18.294Z\",\"views\":0},{\"date\":\"2024-10-12T12:57:18.313Z\",\"views\":7},{\"date\":\"2024-10-09T00:57:18.329Z\",\"views\":2},{\"date\":\"2024-10-05T12:57:18.347Z\",\"views\":2},{\"date\":\"2024-10-02T00:57:18.366Z\",\"views\":0},{\"date\":\"2024-09-28T12:57:18.384Z\",\"views\":1},{\"date\":\"2024-09-25T00:57:18.400Z\",\"views\":1},{\"date\":\"2024-09-21T12:57:18.420Z\",\"views\":2},{\"date\":\"2024-09-18T00:57:18.436Z\",\"views\":2},{\"date\":\"2024-09-14T12:57:18.455Z\",\"views\":0},{\"date\":\"2024-09-11T00:57:18.473Z\",\"views\":1},{\"date\":\"2024-09-07T12:57:18.492Z\",\"views\":2},{\"date\":\"2024-09-04T00:57:18.508Z\",\"views\":1},{\"date\":\"2024-08-31T12:57:18.524Z\",\"views\":0},{\"date\":\"2024-08-28T00:57:18.535Z\",\"views\":2}]},\"ranking\":{\"current_rank\":2604,\"previous_rank\":7114,\"activity_score\":0,\"paper_score\":0.9729550745276566},\"is_hidden\":false,\"custom_categories\":[\"machine-translation\",\"transformers\",\"multi-task-learning\",\"transfer-learning\",\"language-models\"],\"first_publication_date\":\"2024-04-07T11:52:44.000Z\",\"author_user_ids\":[],\"organizations\":[\"67be6377aa92218ccd8b0fe4\",\"67be6377aa92218ccd8b0fe3\",\"67be6377aa92218ccd8b1019\",\"67be6376aa92218ccd8b0f6f\",\"67be6378aa92218ccd8b10ad\",\"67c0fab39fdf15298df1e58b\"],\"citation\":{\"bibtex\":\"@misc{yu2024multilinguallargelanguage,\\n title={Multilingual Large Language Model: A Survey of Resources, Taxonomy and Frontiers}, \\n author={Philip S. Yu and Lizi Liao and Yinghui Li and Wanxiang Che and Zhi Chen and Qiguang Chen and Libo Qin and Yuhang Zhou and Min Li},\\n year={2024},\\n eprint={2404.04925},\\n archivePrefix={arXiv},\\n primaryClass={cs.CL},\\n url={https://arxiv.org/abs/2404.04925}, \\n}\"},\"paperVersions\":{\"_id\":\"6734ad1493ee437496011447\",\"paper_group_id\":\"6734ad1193ee437496011446\",\"version_label\":\"v1\",\"version_order\":1,\"title\":\"Multilingual Large Language Model: A Survey of Resources, Taxonomy and Frontiers\",\"abstract\":\"$54\",\"author_ids\":[\"672bd0a3986a1370676e0693\",\"672bd0a2986a1370676e068c\",\"672bd0f8986a1370676e0d0b\",\"672bd08f986a1370676e04ed\",\"672bcb5a986a1370676da349\",\"672bc9c7986a1370676d8d9f\",\"672bd109986a1370676e0e5a\",\"672bce42986a1370676dd58f\",\"672bbe1f986a1370676d5653\"],\"publication_date\":\"2024-04-07T11:52:44.000Z\",\"license\":\"http://arxiv.org/licenses/nonexclusive-distrib/1.0/\",\"created_at\":\"2024-11-13T13:43:48.170Z\",\"updated_at\":\"2024-11-13T13:43:48.170Z\",\"is_deleted\":false,\"is_hidden\":false,\"imageURL\":\"image/2404.04925v1.png\",\"universal_paper_id\":\"2404.04925\"},\"verifiedAuthors\":[],\"authors\":[{\"_id\":\"672bbe1f986a1370676d5653\",\"full_name\":\"Philip S. Yu\",\"affiliation\":null,\"orcid\":null,\"semantic_scholarid\":null,\"user_id\":null},{\"_id\":\"672bc9c7986a1370676d8d9f\",\"full_name\":\"Lizi Liao\",\"affiliation\":null,\"orcid\":null,\"semantic_scholarid\":null,\"user_id\":null},{\"_id\":\"672bcb5a986a1370676da349\",\"full_name\":\"Yinghui Li\",\"affiliation\":null,\"orcid\":null,\"semantic_scholarid\":null,\"user_id\":null},{\"_id\":\"672bce42986a1370676dd58f\",\"full_name\":\"Wanxiang Che\",\"affiliation\":null,\"orcid\":null,\"semantic_scholarid\":null,\"user_id\":null},{\"_id\":\"672bd08f986a1370676e04ed\",\"full_name\":\"Zhi Chen\",\"affiliation\":null,\"orcid\":null,\"semantic_scholarid\":null,\"user_id\":null},{\"_id\":\"672bd0a2986a1370676e068c\",\"full_name\":\"Qiguang Chen\",\"affiliation\":null,\"orcid\":null,\"semantic_scholarid\":null,\"user_id\":null},{\"_id\":\"672bd0a3986a1370676e0693\",\"full_name\":\"Libo Qin\",\"affiliation\":null,\"orcid\":null,\"semantic_scholarid\":null,\"user_id\":null},{\"_id\":\"672bd0f8986a1370676e0d0b\",\"full_name\":\"Yuhang Zhou\",\"affiliation\":null,\"orcid\":null,\"semantic_scholarid\":null,\"user_id\":null},{\"_id\":\"672bd109986a1370676e0e5a\",\"full_name\":\"Min Li\",\"affiliation\":null,\"orcid\":null,\"semantic_scholarid\":null,\"user_id\":null}]},\"max_version_order\":1,\"verified_authors\":[],\"authors\":[{\"_id\":\"672bbe1f986a1370676d5653\",\"full_name\":\"Philip S. Yu\",\"affiliation\":null,\"orcid\":null,\"semantic_scholarid\":null,\"user_id\":null},{\"_id\":\"672bc9c7986a1370676d8d9f\",\"full_name\":\"Lizi Liao\",\"affiliation\":null,\"orcid\":null,\"semantic_scholarid\":null,\"user_id\":null},{\"_id\":\"672bcb5a986a1370676da349\",\"full_name\":\"Yinghui Li\",\"affiliation\":null,\"orcid\":null,\"semantic_scholarid\":null,\"user_id\":null},{\"_id\":\"672bce42986a1370676dd58f\",\"full_name\":\"Wanxiang Che\",\"affiliation\":null,\"orcid\":null,\"semantic_scholarid\":null,\"user_id\":null},{\"_id\":\"672bd08f986a1370676e04ed\",\"full_name\":\"Zhi Chen\",\"affiliation\":null,\"orcid\":null,\"semantic_scholarid\":null,\"user_id\":null},{\"_id\":\"672bd0a2986a1370676e068c\",\"full_name\":\"Qiguang Chen\",\"affiliation\":null,\"orcid\":null,\"semantic_scholarid\":null,\"user_id\":null},{\"_id\":\"672bd0a3986a1370676e0693\",\"full_name\":\"Libo Qin\",\"affiliation\":null,\"orcid\":null,\"semantic_scholarid\":null,\"user_id\":null},{\"_id\":\"672bd0f8986a1370676e0d0b\",\"full_name\":\"Yuhang Zhou\",\"affiliation\":null,\"orcid\":null,\"semantic_scholarid\":null,\"user_id\":null},{\"_id\":\"672bd109986a1370676e0e5a\",\"full_name\":\"Min Li\",\"affiliation\":null,\"orcid\":null,\"semantic_scholarid\":null,\"user_id\":null}],\"pdf_info\":{\"fetcher_url\":\"https://fetcher.alphaxiv.org/v2/pdf/2404.04925v1\"}}},\"dataUpdateCount\":1,\"dataUpdatedAt\":1744228202480,\"error\":null,\"errorUpdateCount\":0,\"errorUpdatedAt\":0,\"fetchFailureCount\":0,\"fetchFailureReason\":null,\"fetchMeta\":null,\"isInvalidated\":false,\"status\":\"success\",\"fetchStatus\":\"idle\"},\"queryKey\":[\"paper\",\"2404.04925\",\"metadata\"],\"queryHash\":\"[\\\"paper\\\",\\\"2404.04925\\\",\\\"metadata\\\"]\"},{\"state\":{\"data\":{\"data\":[]},\"dataUpdateCount\":1,\"dataUpdatedAt\":1744228202480,\"error\":null,\"errorUpdateCount\":0,\"errorUpdatedAt\":0,\"fetchFailureCount\":0,\"fetchFailureReason\":null,\"fetchMeta\":null,\"isInvalidated\":false,\"status\":\"success\",\"fetchStatus\":\"idle\"},\"queryKey\":[\"paper\",\"2404.04925\",\"comments\"],\"queryHash\":\"[\\\"paper\\\",\\\"2404.04925\\\",\\\"comments\\\"]\"},{\"state\":{\"data\":{\"data\":{\"paper_version\":{\"_id\":\"67347ef593ee43749600ea9b\",\"paper_group_id\":\"67347ef393ee43749600ea98\",\"version_label\":\"v1\",\"version_order\":1,\"title\":\"Root data in character varieties\",\"abstract\":\"Given $G$ an algebraic reductive group over an algebraically closed field of\\ncharacteristic zero and $\\\\Gamma$ a finitely generated group, we provide a\\nstratification of the $G$-character variety of $\\\\Gamma$ in terms of conjugacy\\nclasses of parabolic subgroups of $G$. Each stratum has the structure of a\\npseudo-quotient, which is a relaxed GIT notion capturing the topology of the\\nquotient and, therefore, behaving well for motivic computations of invariants\\nof the character varieties. These stratifications are constructed by analyzing\\nthe root datum of $G$ to encode parabolic classes. Finally, detailed and\\nexplicit motivic formulae are provided for cases with Dynkin diagram of types\\n$A$, $B$, $C$ and $D$.\",\"author_ids\":[\"67347ef493ee43749600ea99\",\"67347ef593ee43749600ea9a\"],\"publication_date\":\"2024-08-06T11:38:43.000Z\",\"license\":\"http://arxiv.org/licenses/nonexclusive-distrib/1.0/\",\"created_at\":\"2024-11-13T10:27:01.265Z\",\"updated_at\":\"2024-11-13T10:27:01.265Z\",\"is_deleted\":false,\"is_hidden\":false,\"universal_paper_id\":\"2408.03111\",\"imageURL\":\"image/2408.03111v1.png\"},\"paper_group\":{\"_id\":\"67347ef393ee43749600ea98\",\"universal_paper_id\":\"2408.03111\",\"source\":{\"name\":\"alphaXiv\",\"url\":\"https://alphaxiv.org/paper/2408.03111\"},\"title\":\"Root data in character varieties\",\"created_at\":\"1970-01-01T00:00:00.000Z\",\"updated_at\":\"2025-03-03T19:47:45.807Z\",\"categories\":[\"Mathematics\"],\"subcategories\":[\"math.AG\",\"math.RT\"],\"metrics\":{\"activity_rank\":0,\"questions_count\":0,\"responses_count\":0,\"upvotes_count\":null,\"downvotes_count\":0,\"total_votes\":0,\"visits_count\":{\"last24Hours\":0,\"last7Days\":1,\"last30Days\":2,\"last90Days\":5,\"all\":19},\"weighted_visits\":{\"last24Hours\":0,\"last7Days\":0.0000010943607764501223,\"last30Days\":0.08131439780552113,\"last90Days\":1.7192900737546486,\"hot\":0.0000010943607764501223},\"public_total_votes\":0,\"timeline\":[{\"date\":\"2025-04-03T00:33:31.723Z\",\"views\":1},{\"date\":\"2025-03-30T12:33:31.723Z\",\"views\":3},{\"date\":\"2025-03-27T00:33:31.723Z\",\"views\":4},{\"date\":\"2025-03-23T12:33:31.723Z\",\"views\":2},{\"date\":\"2025-03-20T00:33:31.723Z\",\"views\":1},{\"date\":\"2025-03-16T12:33:31.723Z\",\"views\":0},{\"date\":\"2025-03-13T00:33:31.723Z\",\"views\":1},{\"date\":\"2025-03-09T12:33:31.723Z\",\"views\":2},{\"date\":\"2025-03-06T00:33:31.723Z\",\"views\":0},{\"date\":\"2025-03-02T12:33:31.723Z\",\"views\":0},{\"date\":\"2025-02-27T00:33:31.723Z\",\"views\":0},{\"date\":\"2025-02-23T12:33:31.723Z\",\"views\":2},{\"date\":\"2025-02-20T00:33:31.741Z\",\"views\":1},{\"date\":\"2025-02-16T12:33:31.759Z\",\"views\":8},{\"date\":\"2025-02-13T00:33:31.774Z\",\"views\":0},{\"date\":\"2025-02-09T12:33:31.800Z\",\"views\":1},{\"date\":\"2025-02-06T00:33:31.819Z\",\"views\":2},{\"date\":\"2025-02-02T12:33:31.874Z\",\"views\":0},{\"date\":\"2025-01-30T00:33:31.892Z\",\"views\":2},{\"date\":\"2025-01-26T12:33:31.910Z\",\"views\":2},{\"date\":\"2025-01-23T00:33:31.929Z\",\"views\":2},{\"date\":\"2025-01-19T12:33:31.948Z\",\"views\":1},{\"date\":\"2025-01-16T00:33:31.976Z\",\"views\":3},{\"date\":\"2025-01-12T12:33:31.994Z\",\"views\":1},{\"date\":\"2025-01-09T00:33:32.010Z\",\"views\":0},{\"date\":\"2025-01-05T12:33:32.026Z\",\"views\":4},{\"date\":\"2025-01-02T00:33:32.042Z\",\"views\":0},{\"date\":\"2024-12-29T12:33:32.061Z\",\"views\":1},{\"date\":\"2024-12-26T00:33:32.087Z\",\"views\":2},{\"date\":\"2024-12-22T12:33:32.105Z\",\"views\":0},{\"date\":\"2024-12-19T00:33:32.120Z\",\"views\":2},{\"date\":\"2024-12-15T12:33:32.141Z\",\"views\":1},{\"date\":\"2024-12-12T00:33:32.162Z\",\"views\":1},{\"date\":\"2024-12-08T12:33:32.183Z\",\"views\":2},{\"date\":\"2024-12-05T00:33:32.198Z\",\"views\":1},{\"date\":\"2024-12-01T12:33:32.213Z\",\"views\":1},{\"date\":\"2024-11-28T00:33:32.230Z\",\"views\":0},{\"date\":\"2024-11-24T12:33:32.250Z\",\"views\":2},{\"date\":\"2024-11-21T00:33:32.265Z\",\"views\":1},{\"date\":\"2024-11-17T12:33:32.281Z\",\"views\":0},{\"date\":\"2024-11-14T00:33:32.304Z\",\"views\":0},{\"date\":\"2024-11-10T12:33:32.324Z\",\"views\":0},{\"date\":\"2024-11-07T00:33:32.342Z\",\"views\":1},{\"date\":\"2024-11-03T12:33:32.357Z\",\"views\":0},{\"date\":\"2024-10-30T23:33:32.376Z\",\"views\":0},{\"date\":\"2024-10-27T11:33:32.394Z\",\"views\":1},{\"date\":\"2024-10-23T23:33:32.407Z\",\"views\":0},{\"date\":\"2024-10-20T11:33:32.422Z\",\"views\":0},{\"date\":\"2024-10-16T23:33:32.440Z\",\"views\":0},{\"date\":\"2024-10-13T11:33:32.459Z\",\"views\":0},{\"date\":\"2024-10-09T23:33:32.477Z\",\"views\":1},{\"date\":\"2024-10-06T11:33:32.497Z\",\"views\":2},{\"date\":\"2024-10-02T23:33:32.514Z\",\"views\":0},{\"date\":\"2024-09-29T11:33:32.536Z\",\"views\":0},{\"date\":\"2024-09-25T23:33:32.551Z\",\"views\":1},{\"date\":\"2024-09-22T11:33:32.566Z\",\"views\":1},{\"date\":\"2024-09-18T23:33:32.580Z\",\"views\":2},{\"date\":\"2024-09-15T11:33:32.601Z\",\"views\":2},{\"date\":\"2024-09-11T23:33:32.616Z\",\"views\":0},{\"date\":\"2024-09-08T11:33:32.637Z\",\"views\":0},{\"date\":\"2024-09-04T23:33:32.663Z\",\"views\":1},{\"date\":\"2024-09-01T11:33:32.685Z\",\"views\":2},{\"date\":\"2024-08-28T23:33:32.696Z\",\"views\":1}]},\"ranking\":{\"current_rank\":61924,\"previous_rank\":64025,\"activity_score\":0,\"paper_score\":0},\"is_hidden\":false,\"custom_categories\":null,\"first_publication_date\":\"2024-08-06T11:38:43.000Z\",\"author_user_ids\":[],\"organizations\":[\"67be6388aa92218ccd8b1553\"],\"citation\":{\"bibtex\":\"@misc{gonzález-prieto2024rootdatacharacter,\\n title={Root data in character varieties}, \\n author={Ángel González-Prieto and Alfonso Zamora},\\n year={2024},\\n eprint={2408.03111},\\n archivePrefix={arXiv},\\n primaryClass={math.AG},\\n url={https://arxiv.org/abs/2408.03111}, \\n}\"},\"paperVersions\":{\"_id\":\"67347ef593ee43749600ea9b\",\"paper_group_id\":\"67347ef393ee43749600ea98\",\"version_label\":\"v1\",\"version_order\":1,\"title\":\"Root data in character varieties\",\"abstract\":\"Given $G$ an algebraic reductive group over an algebraically closed field of\\ncharacteristic zero and $\\\\Gamma$ a finitely generated group, we provide a\\nstratification of the $G$-character variety of $\\\\Gamma$ in terms of conjugacy\\nclasses of parabolic subgroups of $G$. Each stratum has the structure of a\\npseudo-quotient, which is a relaxed GIT notion capturing the topology of the\\nquotient and, therefore, behaving well for motivic computations of invariants\\nof the character varieties. These stratifications are constructed by analyzing\\nthe root datum of $G$ to encode parabolic classes. Finally, detailed and\\nexplicit motivic formulae are provided for cases with Dynkin diagram of types\\n$A$, $B$, $C$ and $D$.\",\"author_ids\":[\"67347ef493ee43749600ea99\",\"67347ef593ee43749600ea9a\"],\"publication_date\":\"2024-08-06T11:38:43.000Z\",\"license\":\"http://arxiv.org/licenses/nonexclusive-distrib/1.0/\",\"created_at\":\"2024-11-13T10:27:01.265Z\",\"updated_at\":\"2024-11-13T10:27:01.265Z\",\"is_deleted\":false,\"is_hidden\":false,\"universal_paper_id\":\"2408.03111\",\"imageURL\":\"image/2408.03111v1.png\"},\"verifiedAuthors\":[],\"authors\":[{\"_id\":\"67347ef493ee43749600ea99\",\"full_name\":\"Ángel González-Prieto\",\"affiliation\":null,\"orcid\":null,\"semantic_scholarid\":null,\"user_id\":null},{\"_id\":\"67347ef593ee43749600ea9a\",\"full_name\":\"Alfonso Zamora\",\"affiliation\":null,\"orcid\":null,\"semantic_scholarid\":null,\"user_id\":null}]},\"max_version_order\":1,\"verified_authors\":[],\"authors\":[{\"_id\":\"67347ef493ee43749600ea99\",\"full_name\":\"Ángel González-Prieto\",\"affiliation\":null,\"orcid\":null,\"semantic_scholarid\":null,\"user_id\":null},{\"_id\":\"67347ef593ee43749600ea9a\",\"full_name\":\"Alfonso Zamora\",\"affiliation\":null,\"orcid\":null,\"semantic_scholarid\":null,\"user_id\":null}],\"pdf_info\":{\"fetcher_url\":\"https://fetcher.alphaxiv.org/v2/pdf/2408.03111v1\"}}},\"dataUpdateCount\":1,\"dataUpdatedAt\":1744228202908,\"error\":null,\"errorUpdateCount\":0,\"errorUpdatedAt\":0,\"fetchFailureCount\":0,\"fetchFailureReason\":null,\"fetchMeta\":null,\"isInvalidated\":false,\"status\":\"success\",\"fetchStatus\":\"idle\"},\"queryKey\":[\"paper\",\"2408.03111\",\"metadata\"],\"queryHash\":\"[\\\"paper\\\",\\\"2408.03111\\\",\\\"metadata\\\"]\"},{\"state\":{\"data\":{\"data\":[]},\"dataUpdateCount\":1,\"dataUpdatedAt\":1744228202908,\"error\":null,\"errorUpdateCount\":0,\"errorUpdatedAt\":0,\"fetchFailureCount\":0,\"fetchFailureReason\":null,\"fetchMeta\":null,\"isInvalidated\":false,\"status\":\"success\",\"fetchStatus\":\"idle\"},\"queryKey\":[\"paper\",\"2408.03111\",\"comments\"],\"queryHash\":\"[\\\"paper\\\",\\\"2408.03111\\\",\\\"comments\\\"]\"},{\"state\":{\"data\":{\"data\":{\"paper_version\":{\"_id\":\"677d97db6205d487655f021f\",\"paper_group_id\":\"677d97db6205d487655f021e\",\"version_label\":\"v1\",\"version_order\":1,\"title\":\"*-Hopf algebroids\",\"abstract\":\"$55\",\"author_ids\":[\"6758ff2f5be1bc98394284b2\",\"672bcf23986a1370676de5dd\",\"67405711474cb623c036dce7\"],\"publication_date\":\"2024-12-30T17:11:02.000Z\",\"license\":\"http://arxiv.org/licenses/nonexclusive-distrib/1.0/\",\"created_at\":\"2025-01-07T21:08:43.918Z\",\"updated_at\":\"2025-01-07T21:08:43.918Z\",\"is_deleted\":false,\"is_hidden\":false,\"universal_paper_id\":\"2412.21089\",\"imageURL\":\"image/2412.21089v1.png\"},\"paper_group\":{\"_id\":\"677d97db6205d487655f021e\",\"universal_paper_id\":\"2412.21089\",\"title\":\"*-Hopf algebroids\",\"created_at\":\"2025-01-07T21:08:43.428Z\",\"updated_at\":\"2025-03-03T19:38:04.985Z\",\"categories\":[\"Mathematics\"],\"subcategories\":[\"math.QA\"],\"custom_categories\":null,\"author_user_ids\":[],\"source\":{\"name\":\"alphaXiv\",\"url\":\"https://arxiv.org/paper/2412.21089\"},\"metrics\":{\"activity_rank\":0,\"questions_count\":0,\"responses_count\":0,\"upvotes_count\":0,\"downvotes_count\":0,\"total_votes\":0,\"visits_count\":{\"last24Hours\":0,\"last7Days\":3,\"last30Days\":10,\"last90Days\":21,\"all\":63},\"weighted_visits\":{\"last24Hours\":0,\"last7Days\":0.01401403180407947,\"last30Days\":2.85892720238502,\"last90Days\":13.834187590507831,\"hot\":0.01401403180407947},\"public_total_votes\":4,\"timeline\":[{\"date\":\"2025-04-02T23:43:04.187Z\",\"views\":11},{\"date\":\"2025-03-30T11:43:04.187Z\",\"views\":1},{\"date\":\"2025-03-26T23:43:04.187Z\",\"views\":4},{\"date\":\"2025-03-23T11:43:04.187Z\",\"views\":4},{\"date\":\"2025-03-19T23:43:04.187Z\",\"views\":3},{\"date\":\"2025-03-16T11:43:04.187Z\",\"views\":0},{\"date\":\"2025-03-12T23:43:04.187Z\",\"views\":14},{\"date\":\"2025-03-09T11:43:04.187Z\",\"views\":0},{\"date\":\"2025-03-05T23:43:04.187Z\",\"views\":1},{\"date\":\"2025-03-02T11:43:04.187Z\",\"views\":1},{\"date\":\"2025-02-26T23:43:04.187Z\",\"views\":6},{\"date\":\"2025-02-23T11:43:04.187Z\",\"views\":0},{\"date\":\"2025-02-19T23:43:04.220Z\",\"views\":6},{\"date\":\"2025-02-16T11:43:04.245Z\",\"views\":2},{\"date\":\"2025-02-12T23:43:04.264Z\",\"views\":1},{\"date\":\"2025-02-09T11:43:04.291Z\",\"views\":0},{\"date\":\"2025-02-05T23:43:04.328Z\",\"views\":1},{\"date\":\"2025-02-02T11:43:04.355Z\",\"views\":2},{\"date\":\"2025-01-29T23:43:04.390Z\",\"views\":8},{\"date\":\"2025-01-26T11:43:04.421Z\",\"views\":2},{\"date\":\"2025-01-22T23:43:04.458Z\",\"views\":13},{\"date\":\"2025-01-19T11:43:04.488Z\",\"views\":2},{\"date\":\"2025-01-15T23:43:04.531Z\",\"views\":2},{\"date\":\"2025-01-12T11:43:04.563Z\",\"views\":0},{\"date\":\"2025-01-08T23:43:04.595Z\",\"views\":0},{\"date\":\"2025-01-05T11:43:04.632Z\",\"views\":5},{\"date\":\"2025-01-01T23:43:04.666Z\",\"views\":0},{\"date\":\"2024-12-29T11:43:04.698Z\",\"views\":2}]},\"is_hidden\":false,\"first_publication_date\":\"2024-12-30T17:11:02.000Z\",\"organizations\":[],\"citation\":{\"bibtex\":\"@misc{han2024hopfalgebroids,\\n title={*-Hopf algebroids}, \\n author={Xiao Han and Shahn Majid and Edwin Beggs},\\n year={2024},\\n eprint={2412.21089},\\n archivePrefix={arXiv},\\n primaryClass={math.QA},\\n url={https://arxiv.org/abs/2412.21089}, \\n}\"},\"paperVersions\":{\"_id\":\"677d97db6205d487655f021f\",\"paper_group_id\":\"677d97db6205d487655f021e\",\"version_label\":\"v1\",\"version_order\":1,\"title\":\"*-Hopf algebroids\",\"abstract\":\"$56\",\"author_ids\":[\"6758ff2f5be1bc98394284b2\",\"672bcf23986a1370676de5dd\",\"67405711474cb623c036dce7\"],\"publication_date\":\"2024-12-30T17:11:02.000Z\",\"license\":\"http://arxiv.org/licenses/nonexclusive-distrib/1.0/\",\"created_at\":\"2025-01-07T21:08:43.918Z\",\"updated_at\":\"2025-01-07T21:08:43.918Z\",\"is_deleted\":false,\"is_hidden\":false,\"universal_paper_id\":\"2412.21089\",\"imageURL\":\"image/2412.21089v1.png\"},\"verifiedAuthors\":[],\"authors\":[{\"_id\":\"672bcf23986a1370676de5dd\",\"full_name\":\"Xiao Han\",\"affiliation\":null,\"orcid\":null,\"semantic_scholarid\":null,\"user_id\":null},{\"_id\":\"67405711474cb623c036dce7\",\"full_name\":\"Shahn Majid\",\"affiliation\":null,\"orcid\":null,\"semantic_scholarid\":null,\"user_id\":null},{\"_id\":\"6758ff2f5be1bc98394284b2\",\"full_name\":\"Edwin Beggs\",\"affiliation\":null,\"orcid\":null,\"semantic_scholarid\":null,\"user_id\":null}]},\"max_version_order\":1,\"verified_authors\":[],\"authors\":[{\"_id\":\"672bcf23986a1370676de5dd\",\"full_name\":\"Xiao Han\",\"affiliation\":null,\"orcid\":null,\"semantic_scholarid\":null,\"user_id\":null},{\"_id\":\"67405711474cb623c036dce7\",\"full_name\":\"Shahn Majid\",\"affiliation\":null,\"orcid\":null,\"semantic_scholarid\":null,\"user_id\":null},{\"_id\":\"6758ff2f5be1bc98394284b2\",\"full_name\":\"Edwin Beggs\",\"affiliation\":null,\"orcid\":null,\"semantic_scholarid\":null,\"user_id\":null}],\"pdf_info\":{\"fetcher_url\":\"https://fetcher.alphaxiv.org/v2/pdf/2412.21089v1\"}}},\"dataUpdateCount\":1,\"dataUpdatedAt\":1744228203002,\"error\":null,\"errorUpdateCount\":0,\"errorUpdatedAt\":0,\"fetchFailureCount\":0,\"fetchFailureReason\":null,\"fetchMeta\":null,\"isInvalidated\":false,\"status\":\"success\",\"fetchStatus\":\"idle\"},\"queryKey\":[\"paper\",\"2412.21089\",\"metadata\"],\"queryHash\":\"[\\\"paper\\\",\\\"2412.21089\\\",\\\"metadata\\\"]\"},{\"state\":{\"data\":{\"data\":[]},\"dataUpdateCount\":1,\"dataUpdatedAt\":1744228203002,\"error\":null,\"errorUpdateCount\":0,\"errorUpdatedAt\":0,\"fetchFailureCount\":0,\"fetchFailureReason\":null,\"fetchMeta\":null,\"isInvalidated\":false,\"status\":\"success\",\"fetchStatus\":\"idle\"},\"queryKey\":[\"paper\",\"2412.21089\",\"comments\"],\"queryHash\":\"[\\\"paper\\\",\\\"2412.21089\\\",\\\"comments\\\"]\"},{\"state\":{\"data\":{\"data\":{\"paper_version\":{\"_id\":\"673d9aec181e8ac859338a57\",\"paper_group_id\":\"673d9aec181e8ac859338a56\",\"version_label\":\"v1\",\"version_order\":1,\"title\":\"The Uniqueness of the Gauss Image Measure\",\"abstract\":\"We show that if the Gauss Image Measure of submeasure $\\\\lambda$ via convex body $K$ agrees with the Gauss Image Measure of $\\\\lambda$ via convex body $L$, then the radial Gauss Image maps of their duals, are equal to each other almost everywhere as multivalued maps with respect to $\\\\lambda$. As an application of this result, we establish that, in this case, dual bodies, $K^*$ and $L^*$, are equal up to a dilation on each rectifiable path connected component of the support of $\\\\lambda$. Additionally, we provide many previously unknown properties of the radial Gauss Image map, most notably its variational Lipschitz behavior, establish some measure theory concepts for multivalued maps and, as a supplement, show how the main uniqueness statement neatly follows from the Hopf Theorem under additional smooth assumptions on $K$ and $L$.\",\"author_ids\":[\"673c7ed27d2b7ed9dd515a94\"],\"publication_date\":\"2023-05-02T20:51:30.000Z\",\"license\":\"http://arxiv.org/licenses/nonexclusive-distrib/1.0/\",\"created_at\":\"2024-11-20T08:16:44.629Z\",\"updated_at\":\"2024-11-20T08:16:44.629Z\",\"is_deleted\":false,\"is_hidden\":false,\"universal_paper_id\":\"2305.01779\",\"imageURL\":\"image/2305.01779v1.png\"},\"paper_group\":{\"_id\":\"673d9aec181e8ac859338a56\",\"universal_paper_id\":\"2305.01779\",\"source\":{\"name\":\"arXiv\",\"url\":\"https://arXiv.org/paper/2305.01779\"},\"title\":\"The Uniqueness of the Gauss Image Measure\",\"created_at\":\"2024-11-12T02:52:06.393Z\",\"updated_at\":\"2025-03-03T20:16:44.645Z\",\"categories\":[\"Mathematics\"],\"subcategories\":[\"math.MG\",\"math.DG\",\"math.FA\"],\"metrics\":{\"activity_rank\":0,\"questions_count\":0,\"responses_count\":0,\"upvotes_count\":null,\"downvotes_count\":null,\"total_votes\":0,\"visits_count\":{\"last24Hours\":0,\"last7Days\":0,\"last30Days\":1,\"last90Days\":2,\"all\":4},\"weighted_visits\":{\"last24Hours\":0,\"last7Days\":0,\"last30Days\":0.00008622535346249535,\"last90Days\":0.0883571413977444,\"hot\":0},\"public_total_votes\":0,\"timeline\":[{\"date\":\"2025-04-03T01:53:48.561Z\",\"views\":0},{\"date\":\"2025-03-30T13:53:48.561Z\",\"views\":1},{\"date\":\"2025-03-27T01:53:48.561Z\",\"views\":2},{\"date\":\"2025-03-23T13:53:48.561Z\",\"views\":2},{\"date\":\"2025-03-20T01:53:48.561Z\",\"views\":1},{\"date\":\"2025-03-16T13:53:48.561Z\",\"views\":4},{\"date\":\"2025-03-13T01:53:48.561Z\",\"views\":2},{\"date\":\"2025-03-09T13:53:48.561Z\",\"views\":1},{\"date\":\"2025-03-06T01:53:48.561Z\",\"views\":2},{\"date\":\"2025-03-02T13:53:48.561Z\",\"views\":0},{\"date\":\"2025-02-27T01:53:48.561Z\",\"views\":0},{\"date\":\"2025-02-23T13:53:48.561Z\",\"views\":0},{\"date\":\"2025-02-20T01:53:48.590Z\",\"views\":0},{\"date\":\"2025-02-16T13:53:48.614Z\",\"views\":2},{\"date\":\"2025-02-13T01:53:48.634Z\",\"views\":1},{\"date\":\"2025-02-09T13:53:48.656Z\",\"views\":2},{\"date\":\"2025-02-06T01:53:48.678Z\",\"views\":2},{\"date\":\"2025-02-02T13:53:48.699Z\",\"views\":1},{\"date\":\"2025-01-30T01:53:48.717Z\",\"views\":3},{\"date\":\"2025-01-26T13:53:48.740Z\",\"views\":0},{\"date\":\"2025-01-23T01:53:48.763Z\",\"views\":2},{\"date\":\"2025-01-19T13:53:48.784Z\",\"views\":1},{\"date\":\"2025-01-16T01:53:48.808Z\",\"views\":2},{\"date\":\"2025-01-12T13:53:48.828Z\",\"views\":0},{\"date\":\"2025-01-09T01:53:48.851Z\",\"views\":2},{\"date\":\"2025-01-05T13:53:48.875Z\",\"views\":2},{\"date\":\"2025-01-02T01:53:48.897Z\",\"views\":4},{\"date\":\"2024-12-29T13:53:48.918Z\",\"views\":1},{\"date\":\"2024-12-26T01:53:48.938Z\",\"views\":2},{\"date\":\"2024-12-22T13:53:48.958Z\",\"views\":0},{\"date\":\"2024-12-19T01:53:48.979Z\",\"views\":2},{\"date\":\"2024-12-15T13:53:49.005Z\",\"views\":2},{\"date\":\"2024-12-12T01:53:49.031Z\",\"views\":1},{\"date\":\"2024-12-08T13:53:49.054Z\",\"views\":0},{\"date\":\"2024-12-05T01:53:49.082Z\",\"views\":1},{\"date\":\"2024-12-01T13:53:49.129Z\",\"views\":1},{\"date\":\"2024-11-28T01:53:49.149Z\",\"views\":0},{\"date\":\"2024-11-24T13:53:49.181Z\",\"views\":2},{\"date\":\"2024-11-21T01:53:49.211Z\",\"views\":0},{\"date\":\"2024-11-17T13:53:49.236Z\",\"views\":2},{\"date\":\"2024-11-14T01:53:49.264Z\",\"views\":2},{\"date\":\"2024-11-10T13:53:49.290Z\",\"views\":3},{\"date\":\"2024-11-07T01:53:49.311Z\",\"views\":0},{\"date\":\"2024-11-03T13:53:49.337Z\",\"views\":0},{\"date\":\"2024-10-31T00:53:49.359Z\",\"views\":2},{\"date\":\"2024-10-27T12:53:49.380Z\",\"views\":2},{\"date\":\"2024-10-24T00:53:49.408Z\",\"views\":2},{\"date\":\"2024-10-20T12:53:49.441Z\",\"views\":2},{\"date\":\"2024-10-17T00:53:49.485Z\",\"views\":2},{\"date\":\"2024-10-13T12:53:49.516Z\",\"views\":0},{\"date\":\"2024-10-10T00:53:49.546Z\",\"views\":2},{\"date\":\"2024-10-06T12:53:49.597Z\",\"views\":1},{\"date\":\"2024-10-03T00:53:49.626Z\",\"views\":2},{\"date\":\"2024-09-29T12:53:49.647Z\",\"views\":1},{\"date\":\"2024-09-26T00:53:49.670Z\",\"views\":2},{\"date\":\"2024-09-22T12:53:49.692Z\",\"views\":0},{\"date\":\"2024-09-19T00:53:49.715Z\",\"views\":1},{\"date\":\"2024-09-15T12:53:49.738Z\",\"views\":0},{\"date\":\"2024-09-12T00:53:49.759Z\",\"views\":0},{\"date\":\"2024-09-08T12:53:49.782Z\",\"views\":0},{\"date\":\"2024-09-05T00:53:49.804Z\",\"views\":2},{\"date\":\"2024-09-01T12:53:49.828Z\",\"views\":1},{\"date\":\"2024-08-29T00:53:49.850Z\",\"views\":0}]},\"ranking\":{\"current_rank\":145101,\"previous_rank\":143989,\"activity_score\":0,\"paper_score\":0},\"is_hidden\":false,\"custom_categories\":null,\"first_publication_date\":\"2023-05-02T20:51:30.000Z\",\"author_user_ids\":[],\"organizations\":[],\"citation\":{\"bibtex\":\"@misc{semenov2023uniquenessgaussimage,\\n title={The Uniqueness of the Gauss Image Measure}, \\n author={Vadim Semenov},\\n year={2023},\\n eprint={2305.01779},\\n archivePrefix={arXiv},\\n primaryClass={math.MG},\\n url={https://arxiv.org/abs/2305.01779}, \\n}\"},\"paperVersions\":{\"_id\":\"673d9aec181e8ac859338a57\",\"paper_group_id\":\"673d9aec181e8ac859338a56\",\"version_label\":\"v1\",\"version_order\":1,\"title\":\"The Uniqueness of the Gauss Image Measure\",\"abstract\":\"We show that if the Gauss Image Measure of submeasure $\\\\lambda$ via convex body $K$ agrees with the Gauss Image Measure of $\\\\lambda$ via convex body $L$, then the radial Gauss Image maps of their duals, are equal to each other almost everywhere as multivalued maps with respect to $\\\\lambda$. As an application of this result, we establish that, in this case, dual bodies, $K^*$ and $L^*$, are equal up to a dilation on each rectifiable path connected component of the support of $\\\\lambda$. Additionally, we provide many previously unknown properties of the radial Gauss Image map, most notably its variational Lipschitz behavior, establish some measure theory concepts for multivalued maps and, as a supplement, show how the main uniqueness statement neatly follows from the Hopf Theorem under additional smooth assumptions on $K$ and $L$.\",\"author_ids\":[\"673c7ed27d2b7ed9dd515a94\"],\"publication_date\":\"2023-05-02T20:51:30.000Z\",\"license\":\"http://arxiv.org/licenses/nonexclusive-distrib/1.0/\",\"created_at\":\"2024-11-20T08:16:44.629Z\",\"updated_at\":\"2024-11-20T08:16:44.629Z\",\"is_deleted\":false,\"is_hidden\":false,\"universal_paper_id\":\"2305.01779\",\"imageURL\":\"image/2305.01779v1.png\"},\"verifiedAuthors\":[],\"authors\":[{\"_id\":\"673c7ed27d2b7ed9dd515a94\",\"full_name\":\"Vadim Semenov\",\"affiliation\":null,\"orcid\":null,\"semantic_scholarid\":null,\"user_id\":null}]},\"max_version_order\":1,\"verified_authors\":[],\"authors\":[{\"_id\":\"673c7ed27d2b7ed9dd515a94\",\"full_name\":\"Vadim Semenov\",\"affiliation\":null,\"orcid\":null,\"semantic_scholarid\":null,\"user_id\":null}],\"pdf_info\":{\"fetcher_url\":\"https://fetcher.alphaxiv.org/v2/pdf/2305.01779v1\"}}},\"dataUpdateCount\":1,\"dataUpdatedAt\":1744228204672,\"error\":null,\"errorUpdateCount\":0,\"errorUpdatedAt\":0,\"fetchFailureCount\":0,\"fetchFailureReason\":null,\"fetchMeta\":null,\"isInvalidated\":false,\"status\":\"success\",\"fetchStatus\":\"idle\"},\"queryKey\":[\"paper\",\"2305.01779\",\"metadata\"],\"queryHash\":\"[\\\"paper\\\",\\\"2305.01779\\\",\\\"metadata\\\"]\"},{\"state\":{\"data\":{\"data\":[]},\"dataUpdateCount\":1,\"dataUpdatedAt\":1744228204672,\"error\":null,\"errorUpdateCount\":0,\"errorUpdatedAt\":0,\"fetchFailureCount\":0,\"fetchFailureReason\":null,\"fetchMeta\":null,\"isInvalidated\":false,\"status\":\"success\",\"fetchStatus\":\"idle\"},\"queryKey\":[\"paper\",\"2305.01779\",\"comments\"],\"queryHash\":\"[\\\"paper\\\",\\\"2305.01779\\\",\\\"comments\\\"]\"},{\"state\":{\"data\":{\"data\":{\"paper_version\":{\"_id\":\"67b6e4244f849806b8a808ed\",\"paper_group_id\":\"67b6e4234f849806b8a808eb\",\"version_label\":\"v1\",\"version_order\":1,\"title\":\"Beyond One-Size-Fits-All: Tailored Benchmarks for Efficient Evaluation\",\"abstract\":\"$57\",\"author_ids\":[\"673226c6cd1e32a6e7f01a2e\",\"673bac24ee7cdcdc03b1993b\",\"673226c7cd1e32a6e7f01a36\",\"672bd110986a1370676e0ef5\",\"673226c8cd1e32a6e7f01a45\",\"675aaad628731fef5f4cf9c4\",\"673bac24ee7cdcdc03b1993c\",\"673226c7cd1e32a6e7f01a3e\",\"672bccc1986a1370676dbd0f\",\"673226c8cd1e32a6e7f01a56\"],\"publication_date\":\"2025-02-19T09:31:50.000Z\",\"license\":\"http://creativecommons.org/licenses/by/4.0/\",\"created_at\":\"2025-02-20T08:13:24.264Z\",\"updated_at\":\"2025-02-20T08:13:24.264Z\",\"is_deleted\":false,\"is_hidden\":false,\"universal_paper_id\":\"2502.13576\",\"imageURL\":\"image/2502.13576v1.png\"},\"paper_group\":{\"_id\":\"67b6e4234f849806b8a808eb\",\"universal_paper_id\":\"2502.13576\",\"title\":\"Beyond One-Size-Fits-All: Tailored Benchmarks for Efficient Evaluation\",\"created_at\":\"2025-02-20T08:13:23.010Z\",\"updated_at\":\"2025-03-03T19:35:58.481Z\",\"categories\":[\"Computer Science\"],\"subcategories\":[\"cs.LG\",\"cs.AI\"],\"custom_categories\":[\"clustering-algorithms\",\"ml-systems\",\"efficient-transformers\"],\"author_user_ids\":[],\"source\":{\"name\":\"alphaXiv\",\"url\":\"https://arxiv.org/abs/2502.13576\"},\"metrics\":{\"activity_rank\":0,\"questions_count\":0,\"responses_count\":0,\"upvotes_count\":0,\"downvotes_count\":0,\"total_votes\":0,\"public_total_votes\":0,\"visits_count\":{\"last24Hours\":0,\"last7Days\":3,\"last30Days\":4,\"last90Days\":7,\"all\":21},\"weighted_visits\":{\"last24Hours\":0,\"last7Days\":0.25400839617514837,\"last30Days\":2.248344806327002,\"last90Days\":7,\"hot\":0.25400839617514837},\"timeline\":[{\"date\":\"2025-04-02T01:04:00.942Z\",\"views\":11},{\"date\":\"2025-03-29T13:04:00.942Z\",\"views\":1},{\"date\":\"2025-03-26T01:04:00.942Z\",\"views\":5},{\"date\":\"2025-03-22T13:04:00.942Z\",\"views\":0},{\"date\":\"2025-03-19T01:04:00.942Z\",\"views\":0},{\"date\":\"2025-03-15T13:04:00.942Z\",\"views\":0},{\"date\":\"2025-03-12T01:04:00.942Z\",\"views\":2},{\"date\":\"2025-03-08T13:04:00.942Z\",\"views\":2},{\"date\":\"2025-03-05T01:04:00.942Z\",\"views\":2},{\"date\":\"2025-03-01T13:04:00.942Z\",\"views\":1},{\"date\":\"2025-02-26T01:04:00.942Z\",\"views\":8},{\"date\":\"2025-02-22T13:04:00.942Z\",\"views\":1},{\"date\":\"2025-02-19T01:04:00.956Z\",\"views\":3}]},\"is_hidden\":false,\"first_publication_date\":\"2025-02-19T09:31:50.000Z\",\"organizations\":[\"67be6376aa92218ccd8b0f74\",\"67be6379aa92218ccd8b10d0\"],\"citation\":{\"bibtex\":\"@misc{hu2025beyondonesizefitsalltailored,\\n title={Beyond One-Size-Fits-All: Tailored Benchmarks for Efficient Evaluation}, \\n author={Yao Hu and Yiwei Li and Peiwen Yuan and Shaoxiong Feng and Boyuan Pan and Xinglin Wang and Kan Li and Yueqi Zhang and Chuyi Tan and Jiayi Shi},\\n year={2025},\\n eprint={2502.13576},\\n archivePrefix={arXiv},\\n primaryClass={cs.LG},\\n url={https://arxiv.org/abs/2502.13576}, \\n}\"},\"paperVersions\":{\"_id\":\"67b6e4244f849806b8a808ed\",\"paper_group_id\":\"67b6e4234f849806b8a808eb\",\"version_label\":\"v1\",\"version_order\":1,\"title\":\"Beyond One-Size-Fits-All: Tailored Benchmarks for Efficient Evaluation\",\"abstract\":\"$58\",\"author_ids\":[\"673226c6cd1e32a6e7f01a2e\",\"673bac24ee7cdcdc03b1993b\",\"673226c7cd1e32a6e7f01a36\",\"672bd110986a1370676e0ef5\",\"673226c8cd1e32a6e7f01a45\",\"675aaad628731fef5f4cf9c4\",\"673bac24ee7cdcdc03b1993c\",\"673226c7cd1e32a6e7f01a3e\",\"672bccc1986a1370676dbd0f\",\"673226c8cd1e32a6e7f01a56\"],\"publication_date\":\"2025-02-19T09:31:50.000Z\",\"license\":\"http://creativecommons.org/licenses/by/4.0/\",\"created_at\":\"2025-02-20T08:13:24.264Z\",\"updated_at\":\"2025-02-20T08:13:24.264Z\",\"is_deleted\":false,\"is_hidden\":false,\"universal_paper_id\":\"2502.13576\",\"imageURL\":\"image/2502.13576v1.png\"},\"verifiedAuthors\":[],\"authors\":[{\"_id\":\"672bccc1986a1370676dbd0f\",\"full_name\":\"Yao Hu\",\"affiliation\":null,\"orcid\":null,\"semantic_scholarid\":null,\"user_id\":null},{\"_id\":\"672bd110986a1370676e0ef5\",\"full_name\":\"Yiwei Li\",\"affiliation\":null,\"orcid\":null,\"semantic_scholarid\":null,\"user_id\":null},{\"_id\":\"673226c6cd1e32a6e7f01a2e\",\"full_name\":\"Peiwen Yuan\",\"affiliation\":null,\"orcid\":null,\"semantic_scholarid\":null,\"user_id\":null},{\"_id\":\"673226c7cd1e32a6e7f01a36\",\"full_name\":\"Shaoxiong Feng\",\"affiliation\":null,\"orcid\":null,\"semantic_scholarid\":null,\"user_id\":null},{\"_id\":\"673226c7cd1e32a6e7f01a3e\",\"full_name\":\"Boyuan Pan\",\"affiliation\":null,\"orcid\":null,\"semantic_scholarid\":null,\"user_id\":null},{\"_id\":\"673226c8cd1e32a6e7f01a45\",\"full_name\":\"Xinglin Wang\",\"affiliation\":null,\"orcid\":null,\"semantic_scholarid\":null,\"user_id\":null},{\"_id\":\"673226c8cd1e32a6e7f01a56\",\"full_name\":\"Kan Li\",\"affiliation\":null,\"orcid\":null,\"semantic_scholarid\":null,\"user_id\":null},{\"_id\":\"673bac24ee7cdcdc03b1993b\",\"full_name\":\"Yueqi Zhang\",\"affiliation\":null,\"orcid\":null,\"semantic_scholarid\":null,\"user_id\":null},{\"_id\":\"673bac24ee7cdcdc03b1993c\",\"full_name\":\"Chuyi Tan\",\"affiliation\":null,\"orcid\":null,\"semantic_scholarid\":null,\"user_id\":null},{\"_id\":\"675aaad628731fef5f4cf9c4\",\"full_name\":\"Jiayi Shi\",\"affiliation\":null,\"orcid\":null,\"semantic_scholarid\":null,\"user_id\":null}]},\"max_version_order\":1,\"verified_authors\":[],\"authors\":[{\"_id\":\"672bccc1986a1370676dbd0f\",\"full_name\":\"Yao Hu\",\"affiliation\":null,\"orcid\":null,\"semantic_scholarid\":null,\"user_id\":null},{\"_id\":\"672bd110986a1370676e0ef5\",\"full_name\":\"Yiwei Li\",\"affiliation\":null,\"orcid\":null,\"semantic_scholarid\":null,\"user_id\":null},{\"_id\":\"673226c6cd1e32a6e7f01a2e\",\"full_name\":\"Peiwen Yuan\",\"affiliation\":null,\"orcid\":null,\"semantic_scholarid\":null,\"user_id\":null},{\"_id\":\"673226c7cd1e32a6e7f01a36\",\"full_name\":\"Shaoxiong Feng\",\"affiliation\":null,\"orcid\":null,\"semantic_scholarid\":null,\"user_id\":null},{\"_id\":\"673226c7cd1e32a6e7f01a3e\",\"full_name\":\"Boyuan Pan\",\"affiliation\":null,\"orcid\":null,\"semantic_scholarid\":null,\"user_id\":null},{\"_id\":\"673226c8cd1e32a6e7f01a45\",\"full_name\":\"Xinglin Wang\",\"affiliation\":null,\"orcid\":null,\"semantic_scholarid\":null,\"user_id\":null},{\"_id\":\"673226c8cd1e32a6e7f01a56\",\"full_name\":\"Kan Li\",\"affiliation\":null,\"orcid\":null,\"semantic_scholarid\":null,\"user_id\":null},{\"_id\":\"673bac24ee7cdcdc03b1993b\",\"full_name\":\"Yueqi Zhang\",\"affiliation\":null,\"orcid\":null,\"semantic_scholarid\":null,\"user_id\":null},{\"_id\":\"673bac24ee7cdcdc03b1993c\",\"full_name\":\"Chuyi Tan\",\"affiliation\":null,\"orcid\":null,\"semantic_scholarid\":null,\"user_id\":null},{\"_id\":\"675aaad628731fef5f4cf9c4\",\"full_name\":\"Jiayi Shi\",\"affiliation\":null,\"orcid\":null,\"semantic_scholarid\":null,\"user_id\":null}],\"pdf_info\":{\"fetcher_url\":\"https://fetcher.alphaxiv.org/v2/pdf/2502.13576v1\"}}},\"dataUpdateCount\":1,\"dataUpdatedAt\":1744228206103,\"error\":null,\"errorUpdateCount\":0,\"errorUpdatedAt\":0,\"fetchFailureCount\":0,\"fetchFailureReason\":null,\"fetchMeta\":null,\"isInvalidated\":false,\"status\":\"success\",\"fetchStatus\":\"idle\"},\"queryKey\":[\"paper\",\"2502.13576\",\"metadata\"],\"queryHash\":\"[\\\"paper\\\",\\\"2502.13576\\\",\\\"metadata\\\"]\"},{\"state\":{\"data\":{\"data\":[]},\"dataUpdateCount\":1,\"dataUpdatedAt\":1744228206103,\"error\":null,\"errorUpdateCount\":0,\"errorUpdatedAt\":0,\"fetchFailureCount\":0,\"fetchFailureReason\":null,\"fetchMeta\":null,\"isInvalidated\":false,\"status\":\"success\",\"fetchStatus\":\"idle\"},\"queryKey\":[\"paper\",\"2502.13576\",\"comments\"],\"queryHash\":\"[\\\"paper\\\",\\\"2502.13576\\\",\\\"comments\\\"]\"},{\"state\":{\"data\":{\"data\":{\"paper_version\":{\"_id\":\"675165c8d05836936a6fa173\",\"paper_group_id\":\"675165c7d05836936a6fa172\",\"version_label\":\"v2\",\"version_order\":2,\"title\":\"511 keV galactic line from first-order phase transitions and primordial black holes\",\"abstract\":\"Hawking evaporation of primordial black hole (PBH), with mass $3\\\\times 10^{-17} \\\\lesssim M_{\\\\rm PBH}/M_\\\\odot \\\\lesssim 7\\\\times 10^{-17}$ and fractional abundance $0.01 \\\\lesssim f_{\\\\rm PBH} \\\\lesssim 0.5$, well reproduces 511 keV gamma-ray excess from galaxy center. In this work, we investigated the production mechanism of PBHs base on the first-order phase transition induced by quartic effective thermal potential of a scalar field in dark sector. We found the phase transition with vacuum energy, $\\\\mathcal{O}(1)\\\\lesssim B^{1/4}/{\\\\rm MeV} \\\\lesssim \\\\mathcal{O}(100)$, produces the desired PBH mass and abundance fraction. Correlated signatures of gravitational wave and extragalactic gamma-ray from phase transition and black hole evaporation, respectively, are within $\\\\mu$Ares and AMEGO/e-ASTROGAM projected sensitivities.\",\"author_ids\":[\"673dea77181e8ac85933ad01\",\"673e0575181e8ac85933c627\"],\"publication_date\":\"2023-08-16T17:30:10.000Z\",\"license\":\"http://creativecommons.org/licenses/by/4.0/\",\"created_at\":\"2024-12-05T08:35:20.467Z\",\"updated_at\":\"2024-12-05T08:35:20.467Z\",\"is_deleted\":false,\"is_hidden\":false,\"universal_paper_id\":\"2209.01552\",\"imageURL\":\"image/2209.01552v2.png\"},\"paper_group\":{\"_id\":\"675165c7d05836936a6fa172\",\"universal_paper_id\":\"2209.01552\",\"source\":{\"name\":\"arXiv\",\"url\":\"https://arXiv.org/paper/2209.01552\"},\"title\":\"511 keV galactic line from first-order phase transitions and primordial black holes\",\"created_at\":\"2024-12-05T08:35:17.549Z\",\"updated_at\":\"2025-03-03T19:39:24.684Z\",\"categories\":[\"Physics\"],\"subcategories\":[\"hep-ph\"],\"custom_categories\":null,\"author_user_ids\":[],\"metrics\":{\"activity_rank\":0,\"questions_count\":0,\"responses_count\":0,\"upvotes_count\":null,\"downvotes_count\":null,\"total_votes\":0,\"visits_count\":{\"last24Hours\":0,\"last7Days\":2,\"last30Days\":4,\"last90Days\":12,\"all\":39},\"weighted_visits\":{\"last24Hours\":0,\"last7Days\":0.002192327352625938,\"last30Days\":0.815387882109184,\"last90Days\":7.062351568609361,\"hot\":0.002192327352625938},\"public_total_votes\":0,\"timeline\":[{\"date\":\"2025-04-02T23:49:20.871Z\",\"views\":3},{\"date\":\"2025-03-30T11:49:20.871Z\",\"views\":4},{\"date\":\"2025-03-26T23:49:20.871Z\",\"views\":5},{\"date\":\"2025-03-23T11:49:20.871Z\",\"views\":1},{\"date\":\"2025-03-19T23:49:20.871Z\",\"views\":2},{\"date\":\"2025-03-16T11:49:20.871Z\",\"views\":2},{\"date\":\"2025-03-12T23:49:20.871Z\",\"views\":5},{\"date\":\"2025-03-09T11:49:20.871Z\",\"views\":0},{\"date\":\"2025-03-05T23:49:20.871Z\",\"views\":0},{\"date\":\"2025-03-02T11:49:20.871Z\",\"views\":2},{\"date\":\"2025-02-26T23:49:20.871Z\",\"views\":2},{\"date\":\"2025-02-23T11:49:20.871Z\",\"views\":8},{\"date\":\"2025-02-19T23:49:20.903Z\",\"views\":2},{\"date\":\"2025-02-16T11:49:20.926Z\",\"views\":4},{\"date\":\"2025-02-12T23:49:20.961Z\",\"views\":0},{\"date\":\"2025-02-09T11:49:20.986Z\",\"views\":0},{\"date\":\"2025-02-05T23:49:21.003Z\",\"views\":0},{\"date\":\"2025-02-02T11:49:21.039Z\",\"views\":5},{\"date\":\"2025-01-29T23:49:21.058Z\",\"views\":0},{\"date\":\"2025-01-26T11:49:21.088Z\",\"views\":1},{\"date\":\"2025-01-22T23:49:21.110Z\",\"views\":4},{\"date\":\"2025-01-19T11:49:21.130Z\",\"views\":1},{\"date\":\"2025-01-15T23:49:21.208Z\",\"views\":0},{\"date\":\"2025-01-12T11:49:21.249Z\",\"views\":1},{\"date\":\"2025-01-08T23:49:21.278Z\",\"views\":2},{\"date\":\"2025-01-05T11:49:21.294Z\",\"views\":10},{\"date\":\"2025-01-01T23:49:21.323Z\",\"views\":0},{\"date\":\"2024-12-29T11:49:21.353Z\",\"views\":2},{\"date\":\"2024-12-25T23:49:21.380Z\",\"views\":0},{\"date\":\"2024-12-22T11:49:21.400Z\",\"views\":1},{\"date\":\"2024-12-18T23:49:21.426Z\",\"views\":0},{\"date\":\"2024-12-15T11:49:21.453Z\",\"views\":1},{\"date\":\"2024-12-11T23:49:21.471Z\",\"views\":1},{\"date\":\"2024-12-08T11:49:21.494Z\",\"views\":2},{\"date\":\"2024-12-04T23:49:21.515Z\",\"views\":5}]},\"ranking\":{\"current_rank\":0,\"previous_rank\":0,\"activity_score\":0,\"paper_score\":0},\"is_hidden\":false,\"first_publication_date\":\"2024-12-05T08:35:19.934Z\",\"organizations\":[\"67be637caa92218ccd8b11ff\"],\"paperVersions\":{\"_id\":\"675165c8d05836936a6fa173\",\"paper_group_id\":\"675165c7d05836936a6fa172\",\"version_label\":\"v2\",\"version_order\":2,\"title\":\"511 keV galactic line from first-order phase transitions and primordial black holes\",\"abstract\":\"Hawking evaporation of primordial black hole (PBH), with mass $3\\\\times 10^{-17} \\\\lesssim M_{\\\\rm PBH}/M_\\\\odot \\\\lesssim 7\\\\times 10^{-17}$ and fractional abundance $0.01 \\\\lesssim f_{\\\\rm PBH} \\\\lesssim 0.5$, well reproduces 511 keV gamma-ray excess from galaxy center. In this work, we investigated the production mechanism of PBHs base on the first-order phase transition induced by quartic effective thermal potential of a scalar field in dark sector. We found the phase transition with vacuum energy, $\\\\mathcal{O}(1)\\\\lesssim B^{1/4}/{\\\\rm MeV} \\\\lesssim \\\\mathcal{O}(100)$, produces the desired PBH mass and abundance fraction. Correlated signatures of gravitational wave and extragalactic gamma-ray from phase transition and black hole evaporation, respectively, are within $\\\\mu$Ares and AMEGO/e-ASTROGAM projected sensitivities.\",\"author_ids\":[\"673dea77181e8ac85933ad01\",\"673e0575181e8ac85933c627\"],\"publication_date\":\"2023-08-16T17:30:10.000Z\",\"license\":\"http://creativecommons.org/licenses/by/4.0/\",\"created_at\":\"2024-12-05T08:35:20.467Z\",\"updated_at\":\"2024-12-05T08:35:20.467Z\",\"is_deleted\":false,\"is_hidden\":false,\"universal_paper_id\":\"2209.01552\",\"imageURL\":\"image/2209.01552v2.png\"},\"verifiedAuthors\":[],\"authors\":[{\"_id\":\"673dea77181e8ac85933ad01\",\"full_name\":\"Po-Yan Tseng\",\"affiliation\":null,\"orcid\":null,\"semantic_scholarid\":null,\"user_id\":null},{\"_id\":\"673e0575181e8ac85933c627\",\"full_name\":\"Yu-Min Yeh\",\"affiliation\":null,\"orcid\":null,\"semantic_scholarid\":null,\"user_id\":null}]},\"max_version_order\":2,\"verified_authors\":[],\"authors\":[{\"_id\":\"673dea77181e8ac85933ad01\",\"full_name\":\"Po-Yan Tseng\",\"affiliation\":null,\"orcid\":null,\"semantic_scholarid\":null,\"user_id\":null},{\"_id\":\"673e0575181e8ac85933c627\",\"full_name\":\"Yu-Min Yeh\",\"affiliation\":null,\"orcid\":null,\"semantic_scholarid\":null,\"user_id\":null}],\"pdf_info\":{\"fetcher_url\":\"https://fetcher.alphaxiv.org/v2/pdf/2209.01552v2\"}}},\"dataUpdateCount\":1,\"dataUpdatedAt\":1744228207231,\"error\":null,\"errorUpdateCount\":0,\"errorUpdatedAt\":0,\"fetchFailureCount\":0,\"fetchFailureReason\":null,\"fetchMeta\":null,\"isInvalidated\":false,\"status\":\"success\",\"fetchStatus\":\"idle\"},\"queryKey\":[\"paper\",\"2209.01552\",\"metadata\"],\"queryHash\":\"[\\\"paper\\\",\\\"2209.01552\\\",\\\"metadata\\\"]\"},{\"state\":{\"data\":{\"data\":[]},\"dataUpdateCount\":1,\"dataUpdatedAt\":1744228207231,\"error\":null,\"errorUpdateCount\":0,\"errorUpdatedAt\":0,\"fetchFailureCount\":0,\"fetchFailureReason\":null,\"fetchMeta\":null,\"isInvalidated\":false,\"status\":\"success\",\"fetchStatus\":\"idle\"},\"queryKey\":[\"paper\",\"2209.01552\",\"comments\"],\"queryHash\":\"[\\\"paper\\\",\\\"2209.01552\\\",\\\"comments\\\"]\"},{\"state\":{\"data\":{\"data\":{\"paper_version\":{\"_id\":\"6756d71156f478e9f97b8384\",\"paper_group_id\":\"6756d71056f478e9f97b8383\",\"version_label\":\"v2\",\"version_order\":2,\"title\":\"Langevin Diffusion Variational Inference\",\"abstract\":\"$59\",\"author_ids\":[\"6733ce4429b032f357096d61\",\"673d0853615941b897fbbf9e\"],\"publication_date\":\"2023-03-23T13:54:52.000Z\",\"license\":\"http://arxiv.org/licenses/nonexclusive-distrib/1.0/\",\"created_at\":\"2024-12-09T11:40:01.600Z\",\"updated_at\":\"2024-12-09T11:40:01.600Z\",\"is_deleted\":false,\"is_hidden\":false,\"universal_paper_id\":\"2208.07743\",\"imageURL\":\"image/2208.07743v2.png\"},\"paper_group\":{\"_id\":\"6756d71056f478e9f97b8383\",\"universal_paper_id\":\"2208.07743\",\"title\":\"Langevin Diffusion Variational Inference\",\"created_at\":\"2024-12-09T11:40:00.683Z\",\"updated_at\":\"2025-03-03T20:18:39.567Z\",\"categories\":[\"Computer Science\"],\"subcategories\":[\"cs.LG\"],\"custom_categories\":[\"bayesian-deep-learning\",\"uncertainty-estimation\",\"probabilistic-programming\",\"statistical-learning\",\"optimization-methods\"],\"author_user_ids\":[],\"source\":{\"name\":\"alphaXiv\",\"url\":\"https://arxiv.org/paper/2208.07743\"},\"metrics\":{\"activity_rank\":0,\"questions_count\":0,\"responses_count\":0,\"upvotes_count\":0,\"downvotes_count\":0,\"total_votes\":0,\"visits_count\":{\"last24Hours\":0,\"last7Days\":0,\"last30Days\":9,\"last90Days\":12,\"all\":39},\"weighted_visits\":{\"last24Hours\":0,\"last7Days\":0,\"last30Days\":0.00045347611174892063,\"last90Days\":0.4432191817525215,\"hot\":0},\"public_total_votes\":0,\"timeline\":[{\"date\":\"2025-04-03T01:57:38.334Z\",\"views\":1},{\"date\":\"2025-03-30T13:57:38.334Z\",\"views\":2},{\"date\":\"2025-03-27T01:57:38.334Z\",\"views\":12},{\"date\":\"2025-03-23T13:57:38.334Z\",\"views\":0},{\"date\":\"2025-03-20T01:57:38.334Z\",\"views\":2},{\"date\":\"2025-03-16T13:57:38.334Z\",\"views\":8},{\"date\":\"2025-03-13T01:57:38.334Z\",\"views\":1},{\"date\":\"2025-03-09T13:57:38.334Z\",\"views\":0},{\"date\":\"2025-03-06T01:57:38.334Z\",\"views\":11},{\"date\":\"2025-03-02T13:57:38.334Z\",\"views\":0},{\"date\":\"2025-02-27T01:57:38.334Z\",\"views\":0},{\"date\":\"2025-02-23T13:57:38.334Z\",\"views\":3},{\"date\":\"2025-02-20T01:57:38.371Z\",\"views\":2},{\"date\":\"2025-02-16T13:57:38.402Z\",\"views\":4},{\"date\":\"2025-02-13T01:57:38.424Z\",\"views\":1},{\"date\":\"2025-02-09T13:57:38.445Z\",\"views\":0},{\"date\":\"2025-02-06T01:57:38.464Z\",\"views\":1},{\"date\":\"2025-02-02T13:57:38.485Z\",\"views\":1},{\"date\":\"2025-01-30T01:57:38.511Z\",\"views\":1},{\"date\":\"2025-01-26T13:57:38.531Z\",\"views\":1},{\"date\":\"2025-01-23T01:57:38.552Z\",\"views\":2},{\"date\":\"2025-01-19T13:57:38.572Z\",\"views\":3},{\"date\":\"2025-01-16T01:57:38.592Z\",\"views\":0},{\"date\":\"2025-01-12T13:57:38.616Z\",\"views\":0},{\"date\":\"2025-01-09T01:57:38.640Z\",\"views\":2},{\"date\":\"2025-01-05T13:57:38.657Z\",\"views\":2},{\"date\":\"2025-01-02T01:57:38.691Z\",\"views\":0},{\"date\":\"2024-12-29T13:57:38.711Z\",\"views\":0},{\"date\":\"2024-12-26T01:57:38.731Z\",\"views\":2},{\"date\":\"2024-12-22T13:57:38.762Z\",\"views\":1},{\"date\":\"2024-12-19T01:57:38.789Z\",\"views\":0},{\"date\":\"2024-12-15T13:57:38.810Z\",\"views\":1},{\"date\":\"2024-12-12T01:57:38.843Z\",\"views\":2},{\"date\":\"2024-12-08T13:57:38.865Z\",\"views\":5},{\"date\":\"2024-12-05T01:57:38.887Z\",\"views\":2},{\"date\":\"2024-12-01T13:57:38.909Z\",\"views\":1},{\"date\":\"2024-11-28T01:57:38.949Z\",\"views\":0},{\"date\":\"2024-11-24T13:57:38.972Z\",\"views\":1},{\"date\":\"2024-11-21T01:57:38.993Z\",\"views\":0},{\"date\":\"2024-11-17T13:57:39.023Z\",\"views\":2},{\"date\":\"2024-11-14T01:57:39.047Z\",\"views\":1},{\"date\":\"2024-11-10T13:57:39.068Z\",\"views\":1},{\"date\":\"2024-11-07T01:57:39.091Z\",\"views\":2},{\"date\":\"2024-11-03T13:57:39.114Z\",\"views\":0},{\"date\":\"2024-10-31T00:57:39.135Z\",\"views\":2},{\"date\":\"2024-10-27T12:57:39.159Z\",\"views\":0},{\"date\":\"2024-10-24T00:57:39.179Z\",\"views\":1},{\"date\":\"2024-10-20T12:57:39.203Z\",\"views\":0},{\"date\":\"2024-10-17T00:57:39.227Z\",\"views\":2},{\"date\":\"2024-10-13T12:57:39.246Z\",\"views\":0},{\"date\":\"2024-10-10T00:57:39.268Z\",\"views\":0},{\"date\":\"2024-10-06T12:57:39.289Z\",\"views\":2},{\"date\":\"2024-10-03T00:57:39.314Z\",\"views\":0},{\"date\":\"2024-09-29T12:57:39.344Z\",\"views\":1},{\"date\":\"2024-09-26T00:57:39.369Z\",\"views\":0},{\"date\":\"2024-09-22T12:57:39.409Z\",\"views\":0},{\"date\":\"2024-09-19T00:57:39.431Z\",\"views\":1},{\"date\":\"2024-09-15T12:57:39.450Z\",\"views\":2},{\"date\":\"2024-09-12T00:57:39.479Z\",\"views\":1},{\"date\":\"2024-09-08T12:57:39.525Z\",\"views\":2},{\"date\":\"2024-09-05T00:57:39.545Z\",\"views\":0},{\"date\":\"2024-09-01T12:57:39.555Z\",\"views\":1},{\"date\":\"2024-08-29T00:57:39.565Z\",\"views\":1}]},\"is_hidden\":false,\"first_publication_date\":\"2023-03-23T13:54:52.000Z\",\"resources\":{\"github\":{\"url\":\"https://github.com/citiususc/voila\",\"description\":\"Variational Inference for Langevin Equations\",\"language\":\"R\",\"stars\":21}},\"paperVersions\":{\"_id\":\"6756d71156f478e9f97b8384\",\"paper_group_id\":\"6756d71056f478e9f97b8383\",\"version_label\":\"v2\",\"version_order\":2,\"title\":\"Langevin Diffusion Variational Inference\",\"abstract\":\"$5a\",\"author_ids\":[\"6733ce4429b032f357096d61\",\"673d0853615941b897fbbf9e\"],\"publication_date\":\"2023-03-23T13:54:52.000Z\",\"license\":\"http://arxiv.org/licenses/nonexclusive-distrib/1.0/\",\"created_at\":\"2024-12-09T11:40:01.600Z\",\"updated_at\":\"2024-12-09T11:40:01.600Z\",\"is_deleted\":false,\"is_hidden\":false,\"universal_paper_id\":\"2208.07743\",\"imageURL\":\"image/2208.07743v2.png\"},\"verifiedAuthors\":[],\"authors\":[{\"_id\":\"6733ce4429b032f357096d61\",\"full_name\":\"Tomas Geffner\",\"affiliation\":null,\"orcid\":null,\"semantic_scholarid\":null,\"user_id\":null},{\"_id\":\"673d0853615941b897fbbf9e\",\"full_name\":\"Justin Domke\",\"affiliation\":null,\"orcid\":null,\"semantic_scholarid\":null,\"user_id\":null}]},\"max_version_order\":2,\"verified_authors\":[],\"authors\":[{\"_id\":\"6733ce4429b032f357096d61\",\"full_name\":\"Tomas Geffner\",\"affiliation\":null,\"orcid\":null,\"semantic_scholarid\":null,\"user_id\":null},{\"_id\":\"673d0853615941b897fbbf9e\",\"full_name\":\"Justin Domke\",\"affiliation\":null,\"orcid\":null,\"semantic_scholarid\":null,\"user_id\":null}],\"pdf_info\":{\"fetcher_url\":\"https://fetcher.alphaxiv.org/v2/pdf/2208.07743v2\"}}},\"dataUpdateCount\":1,\"dataUpdatedAt\":1744228207659,\"error\":null,\"errorUpdateCount\":0,\"errorUpdatedAt\":0,\"fetchFailureCount\":0,\"fetchFailureReason\":null,\"fetchMeta\":null,\"isInvalidated\":false,\"status\":\"success\",\"fetchStatus\":\"idle\"},\"queryKey\":[\"paper\",\"2208.07743\",\"metadata\"],\"queryHash\":\"[\\\"paper\\\",\\\"2208.07743\\\",\\\"metadata\\\"]\"},{\"state\":{\"data\":{\"data\":[]},\"dataUpdateCount\":1,\"dataUpdatedAt\":1744228207659,\"error\":null,\"errorUpdateCount\":0,\"errorUpdatedAt\":0,\"fetchFailureCount\":0,\"fetchFailureReason\":null,\"fetchMeta\":null,\"isInvalidated\":false,\"status\":\"success\",\"fetchStatus\":\"idle\"},\"queryKey\":[\"paper\",\"2208.07743\",\"comments\"],\"queryHash\":\"[\\\"paper\\\",\\\"2208.07743\\\",\\\"comments\\\"]\"},{\"state\":{\"data\":{\"data\":{\"paper_version\":{\"_id\":\"6788ebac9541b9148ef2392c\",\"paper_group_id\":\"6788eba99d9f04dc61a9b06c\",\"version_label\":\"v1\",\"version_order\":1,\"title\":\"A Case Study on AI Engineering Practices: Developing an Autonomous Stock Trading System\",\"abstract\":\"$5b\",\"author_ids\":[\"6788ebac9541b9148ef2392b\",\"67322b1dcd1e32a6e7f067bc\"],\"publication_date\":\"2023-03-23T12:27:27.000Z\",\"license\":\"http://arxiv.org/licenses/nonexclusive-distrib/1.0/\",\"created_at\":\"2025-01-16T11:21:16.343Z\",\"updated_at\":\"2025-01-16T11:21:16.343Z\",\"is_deleted\":false,\"is_hidden\":false,\"universal_paper_id\":\"2303.13216\",\"imageURL\":\"image/2303.13216v1.png\"},\"paper_group\":{\"_id\":\"6788eba99d9f04dc61a9b06c\",\"universal_paper_id\":\"2303.13216\",\"title\":\"A Case Study on AI Engineering Practices: Developing an Autonomous Stock Trading System\",\"created_at\":\"2025-01-16T11:21:13.960Z\",\"updated_at\":\"2025-03-03T20:18:39.605Z\",\"categories\":[\"Computer Science\"],\"subcategories\":[\"cs.SE\",\"cs.AI\"],\"custom_categories\":[\"reinforcement-learning\",\"autonomous-vehicles\",\"multi-agent-learning\",\"industrial-automation\",\"explainable-ai\"],\"author_user_ids\":[],\"source\":{\"name\":\"alphaXiv\",\"url\":\"https://arxiv.org/paper/2303.13216\"},\"metrics\":{\"activity_rank\":0,\"questions_count\":0,\"responses_count\":0,\"upvotes_count\":0,\"downvotes_count\":0,\"total_votes\":0,\"public_total_votes\":0,\"visits_count\":{\"last24Hours\":0,\"last7Days\":6,\"last30Days\":7,\"last90Days\":12,\"all\":36},\"weighted_visits\":{\"last24Hours\":0,\"last7Days\":2.2802438895544455e-18,\"last30Days\":0.00035241824576289323,\"last90Days\":0.44309960314709096,\"hot\":2.2802438895544455e-18},\"timeline\":[{\"date\":\"2025-04-03T01:57:41.792Z\",\"views\":18},{\"date\":\"2025-03-30T13:57:41.792Z\",\"views\":0},{\"date\":\"2025-03-27T01:57:41.792Z\",\"views\":1},{\"date\":\"2025-03-23T13:57:41.792Z\",\"views\":1},{\"date\":\"2025-03-20T01:57:41.792Z\",\"views\":1},{\"date\":\"2025-03-16T13:57:41.792Z\",\"views\":1},{\"date\":\"2025-03-13T01:57:41.792Z\",\"views\":3},{\"date\":\"2025-03-09T13:57:41.792Z\",\"views\":0},{\"date\":\"2025-03-06T01:57:41.792Z\",\"views\":0},{\"date\":\"2025-03-02T13:57:41.792Z\",\"views\":0},{\"date\":\"2025-02-27T01:57:41.792Z\",\"views\":2},{\"date\":\"2025-02-23T13:57:41.792Z\",\"views\":1},{\"date\":\"2025-02-20T01:57:41.815Z\",\"views\":0},{\"date\":\"2025-02-16T13:57:41.837Z\",\"views\":1},{\"date\":\"2025-02-13T01:57:41.856Z\",\"views\":1},{\"date\":\"2025-02-09T13:57:41.876Z\",\"views\":6},{\"date\":\"2025-02-06T01:57:41.899Z\",\"views\":2},{\"date\":\"2025-02-02T13:57:41.925Z\",\"views\":2},{\"date\":\"2025-01-30T01:57:41.951Z\",\"views\":4},{\"date\":\"2025-01-26T13:57:41.977Z\",\"views\":2},{\"date\":\"2025-01-23T01:57:42.000Z\",\"views\":2},{\"date\":\"2025-01-19T13:57:42.019Z\",\"views\":1},{\"date\":\"2025-01-16T01:57:42.042Z\",\"views\":8},{\"date\":\"2025-01-12T13:57:42.065Z\",\"views\":0},{\"date\":\"2025-01-09T01:57:42.105Z\",\"views\":1},{\"date\":\"2025-01-05T13:57:42.126Z\",\"views\":2},{\"date\":\"2025-01-02T01:57:42.149Z\",\"views\":1},{\"date\":\"2024-12-29T13:57:42.173Z\",\"views\":2},{\"date\":\"2024-12-26T01:57:42.200Z\",\"views\":2},{\"date\":\"2024-12-22T13:57:42.220Z\",\"views\":2},{\"date\":\"2024-12-19T01:57:42.243Z\",\"views\":1},{\"date\":\"2024-12-15T13:57:42.267Z\",\"views\":2},{\"date\":\"2024-12-12T01:57:42.289Z\",\"views\":1},{\"date\":\"2024-12-08T13:57:42.313Z\",\"views\":0},{\"date\":\"2024-12-05T01:57:42.335Z\",\"views\":2},{\"date\":\"2024-12-01T13:57:42.358Z\",\"views\":1},{\"date\":\"2024-11-28T01:57:42.379Z\",\"views\":1},{\"date\":\"2024-11-24T13:57:42.402Z\",\"views\":1},{\"date\":\"2024-11-21T01:57:42.421Z\",\"views\":0},{\"date\":\"2024-11-17T13:57:42.446Z\",\"views\":1},{\"date\":\"2024-11-14T01:57:42.467Z\",\"views\":2},{\"date\":\"2024-11-10T13:57:42.490Z\",\"views\":0},{\"date\":\"2024-11-07T01:57:42.512Z\",\"views\":0},{\"date\":\"2024-11-03T13:57:42.535Z\",\"views\":0},{\"date\":\"2024-10-31T00:57:42.558Z\",\"views\":0},{\"date\":\"2024-10-27T12:57:42.589Z\",\"views\":2},{\"date\":\"2024-10-24T00:57:42.628Z\",\"views\":2},{\"date\":\"2024-10-20T12:57:42.649Z\",\"views\":0},{\"date\":\"2024-10-17T00:57:42.675Z\",\"views\":0},{\"date\":\"2024-10-13T12:57:42.709Z\",\"views\":1},{\"date\":\"2024-10-10T00:57:42.741Z\",\"views\":1},{\"date\":\"2024-10-06T12:57:42.771Z\",\"views\":1},{\"date\":\"2024-10-03T00:57:42.795Z\",\"views\":1},{\"date\":\"2024-09-29T12:57:42.817Z\",\"views\":2},{\"date\":\"2024-09-26T00:57:42.839Z\",\"views\":0},{\"date\":\"2024-09-22T12:57:42.861Z\",\"views\":0},{\"date\":\"2024-09-19T00:57:42.887Z\",\"views\":0},{\"date\":\"2024-09-15T12:57:42.908Z\",\"views\":1},{\"date\":\"2024-09-12T00:57:42.927Z\",\"views\":2},{\"date\":\"2024-09-08T12:57:42.949Z\",\"views\":0},{\"date\":\"2024-09-05T00:57:42.969Z\",\"views\":0},{\"date\":\"2024-09-01T12:57:42.991Z\",\"views\":2},{\"date\":\"2024-08-29T00:57:43.013Z\",\"views\":2}]},\"is_hidden\":false,\"first_publication_date\":\"2023-03-23T12:27:27.000Z\",\"organizations\":[\"67be6376aa92218ccd8b0fb8\"],\"paperVersions\":{\"_id\":\"6788ebac9541b9148ef2392c\",\"paper_group_id\":\"6788eba99d9f04dc61a9b06c\",\"version_label\":\"v1\",\"version_order\":1,\"title\":\"A Case Study on AI Engineering Practices: Developing an Autonomous Stock Trading System\",\"abstract\":\"$5c\",\"author_ids\":[\"6788ebac9541b9148ef2392b\",\"67322b1dcd1e32a6e7f067bc\"],\"publication_date\":\"2023-03-23T12:27:27.000Z\",\"license\":\"http://arxiv.org/licenses/nonexclusive-distrib/1.0/\",\"created_at\":\"2025-01-16T11:21:16.343Z\",\"updated_at\":\"2025-01-16T11:21:16.343Z\",\"is_deleted\":false,\"is_hidden\":false,\"universal_paper_id\":\"2303.13216\",\"imageURL\":\"image/2303.13216v1.png\"},\"verifiedAuthors\":[],\"authors\":[{\"_id\":\"67322b1dcd1e32a6e7f067bc\",\"full_name\":\"Justus Bogner\",\"affiliation\":null,\"orcid\":null,\"semantic_scholarid\":null,\"user_id\":null},{\"_id\":\"6788ebac9541b9148ef2392b\",\"full_name\":\"Marcel Grote\",\"affiliation\":null,\"orcid\":null,\"semantic_scholarid\":null,\"user_id\":null}]},\"max_version_order\":1,\"verified_authors\":[],\"authors\":[{\"_id\":\"67322b1dcd1e32a6e7f067bc\",\"full_name\":\"Justus Bogner\",\"affiliation\":null,\"orcid\":null,\"semantic_scholarid\":null,\"user_id\":null},{\"_id\":\"6788ebac9541b9148ef2392b\",\"full_name\":\"Marcel Grote\",\"affiliation\":null,\"orcid\":null,\"semantic_scholarid\":null,\"user_id\":null}],\"pdf_info\":{\"fetcher_url\":\"https://fetcher.alphaxiv.org/v2/pdf/2303.13216v1\"}}},\"dataUpdateCount\":1,\"dataUpdatedAt\":1744228207690,\"error\":null,\"errorUpdateCount\":0,\"errorUpdatedAt\":0,\"fetchFailureCount\":0,\"fetchFailureReason\":null,\"fetchMeta\":null,\"isInvalidated\":false,\"status\":\"success\",\"fetchStatus\":\"idle\"},\"queryKey\":[\"paper\",\"2303.13216\",\"metadata\"],\"queryHash\":\"[\\\"paper\\\",\\\"2303.13216\\\",\\\"metadata\\\"]\"},{\"state\":{\"data\":{\"data\":[]},\"dataUpdateCount\":1,\"dataUpdatedAt\":1744228207690,\"error\":null,\"errorUpdateCount\":0,\"errorUpdatedAt\":0,\"fetchFailureCount\":0,\"fetchFailureReason\":null,\"fetchMeta\":null,\"isInvalidated\":false,\"status\":\"success\",\"fetchStatus\":\"idle\"},\"queryKey\":[\"paper\",\"2303.13216\",\"comments\"],\"queryHash\":\"[\\\"paper\\\",\\\"2303.13216\\\",\\\"comments\\\"]\"},{\"state\":{\"data\":{\"data\":{\"paper_version\":{\"_id\":\"67322376cd1e32a6e7efe4d5\",\"paper_group_id\":\"67322375cd1e32a6e7efe4bf\",\"version_label\":\"v2\",\"version_order\":2,\"title\":\"DepGraph: Towards Any Structural Pruning\",\"abstract\":\"$5d\",\"author_ids\":[\"672bbf7f986a1370676d5efc\",\"672bbf7f986a1370676d5efa\",\"672bd0c4986a1370676e0920\",\"672bd09d986a1370676e061d\",\"672bbf7f986a1370676d5efe\"],\"publication_date\":\"2023-03-23T12:55:02.000Z\",\"license\":\"http://arxiv.org/licenses/nonexclusive-distrib/1.0/\",\"created_at\":\"2024-11-11T15:32:06.303Z\",\"updated_at\":\"2024-11-11T15:32:06.303Z\",\"is_deleted\":false,\"is_hidden\":false,\"imageURL\":\"image/2301.12900v2.png\",\"universal_paper_id\":\"2301.12900\"},\"paper_group\":{\"_id\":\"67322375cd1e32a6e7efe4bf\",\"universal_paper_id\":\"2301.12900\",\"source\":{\"name\":\"alphaXiv\",\"url\":\"https://alphaxiv.org/paper/2301.12900\"},\"title\":\"DepGraph: Towards Any Structural Pruning\",\"created_at\":\"2024-09-24T17:32:43.909Z\",\"updated_at\":\"2025-03-03T20:18:39.604Z\",\"categories\":[\"Computer Science\"],\"subcategories\":[\"cs.AI\",\"cs.CV\"],\"metrics\":{\"activity_rank\":0,\"questions_count\":0,\"responses_count\":0,\"upvotes_count\":null,\"downvotes_count\":0,\"total_votes\":0,\"visits_count\":{\"last24Hours\":1,\"last7Days\":6,\"last30Days\":34,\"last90Days\":91,\"all\":382},\"weighted_visits\":{\"last24Hours\":1.1538204007131747e-129,\"last7Days\":2.2827413692724213e-18,\"last30Days\":0.001712183039940506,\"last90Days\":3.3604580909350474,\"hot\":2.2827413692724213e-18},\"public_total_votes\":8,\"timeline\":[{\"date\":\"2025-04-03T01:57:41.792Z\",\"views\":9},{\"date\":\"2025-03-30T13:57:41.792Z\",\"views\":6},{\"date\":\"2025-03-27T01:57:41.792Z\",\"views\":6},{\"date\":\"2025-03-23T13:57:41.792Z\",\"views\":14},{\"date\":\"2025-03-20T01:57:41.792Z\",\"views\":28},{\"date\":\"2025-03-16T13:57:41.792Z\",\"views\":12},{\"date\":\"2025-03-13T01:57:41.792Z\",\"views\":3},{\"date\":\"2025-03-09T13:57:41.792Z\",\"views\":26},{\"date\":\"2025-03-06T01:57:41.792Z\",\"views\":3},{\"date\":\"2025-03-02T13:57:41.792Z\",\"views\":5},{\"date\":\"2025-02-27T01:57:41.792Z\",\"views\":1},{\"date\":\"2025-02-23T13:57:41.792Z\",\"views\":1},{\"date\":\"2025-02-20T01:57:41.806Z\",\"views\":6},{\"date\":\"2025-02-16T13:57:41.828Z\",\"views\":17},{\"date\":\"2025-02-13T01:57:41.849Z\",\"views\":21},{\"date\":\"2025-02-09T13:57:41.871Z\",\"views\":16},{\"date\":\"2025-02-06T01:57:41.895Z\",\"views\":25},{\"date\":\"2025-02-02T13:57:41.917Z\",\"views\":5},{\"date\":\"2025-01-30T01:57:41.946Z\",\"views\":1},{\"date\":\"2025-01-26T13:57:41.973Z\",\"views\":6},{\"date\":\"2025-01-23T01:57:41.999Z\",\"views\":19},{\"date\":\"2025-01-19T13:57:42.019Z\",\"views\":18},{\"date\":\"2025-01-16T01:57:42.042Z\",\"views\":13},{\"date\":\"2025-01-12T13:57:42.068Z\",\"views\":9},{\"date\":\"2025-01-09T01:57:42.106Z\",\"views\":20},{\"date\":\"2025-01-05T13:57:42.128Z\",\"views\":2},{\"date\":\"2025-01-02T01:57:42.151Z\",\"views\":4},{\"date\":\"2024-12-29T13:57:42.175Z\",\"views\":2},{\"date\":\"2024-12-26T01:57:42.201Z\",\"views\":13},{\"date\":\"2024-12-22T13:57:42.224Z\",\"views\":2},{\"date\":\"2024-12-19T01:57:42.254Z\",\"views\":1},{\"date\":\"2024-12-15T13:57:42.282Z\",\"views\":1},{\"date\":\"2024-12-12T01:57:42.306Z\",\"views\":12},{\"date\":\"2024-12-08T13:57:42.330Z\",\"views\":3},{\"date\":\"2024-12-05T01:57:42.349Z\",\"views\":6},{\"date\":\"2024-12-01T13:57:42.375Z\",\"views\":2},{\"date\":\"2024-11-28T01:57:42.399Z\",\"views\":2},{\"date\":\"2024-11-24T13:57:42.420Z\",\"views\":13},{\"date\":\"2024-11-21T01:57:42.447Z\",\"views\":1},{\"date\":\"2024-11-17T13:57:42.470Z\",\"views\":6},{\"date\":\"2024-11-14T01:57:42.493Z\",\"views\":1},{\"date\":\"2024-11-10T13:57:42.512Z\",\"views\":4},{\"date\":\"2024-11-07T01:57:42.533Z\",\"views\":2},{\"date\":\"2024-11-03T13:57:42.554Z\",\"views\":11},{\"date\":\"2024-10-31T00:57:42.577Z\",\"views\":28},{\"date\":\"2024-10-27T12:57:42.605Z\",\"views\":14},{\"date\":\"2024-10-24T00:57:42.638Z\",\"views\":2},{\"date\":\"2024-10-20T12:57:42.662Z\",\"views\":5},{\"date\":\"2024-10-17T00:57:42.687Z\",\"views\":1},{\"date\":\"2024-10-13T12:57:42.754Z\",\"views\":1},{\"date\":\"2024-10-10T00:57:42.777Z\",\"views\":2},{\"date\":\"2024-10-06T12:57:42.799Z\",\"views\":2},{\"date\":\"2024-10-03T00:57:42.826Z\",\"views\":1},{\"date\":\"2024-09-29T12:57:42.847Z\",\"views\":1},{\"date\":\"2024-09-26T00:57:42.874Z\",\"views\":0},{\"date\":\"2024-09-22T12:57:42.903Z\",\"views\":1},{\"date\":\"2024-09-19T00:57:42.924Z\",\"views\":2},{\"date\":\"2024-09-15T12:57:42.948Z\",\"views\":0},{\"date\":\"2024-09-12T00:57:42.970Z\",\"views\":0},{\"date\":\"2024-09-08T12:57:42.992Z\",\"views\":2},{\"date\":\"2024-09-05T00:57:43.016Z\",\"views\":2},{\"date\":\"2024-09-01T12:57:43.037Z\",\"views\":0},{\"date\":\"2024-08-29T00:57:43.060Z\",\"views\":2}]},\"ranking\":{\"current_rank\":788,\"previous_rank\":787,\"activity_score\":0,\"paper_score\":1.2824746787307684},\"is_hidden\":false,\"custom_categories\":[\"model-compression\",\"lightweight-models\",\"neural-architecture-search\",\"parameter-efficient-training\",\"efficient-transformers\"],\"first_publication_date\":\"2023-03-23T12:55:02.000Z\",\"author_user_ids\":[],\"citation\":{\"bibtex\":\"@Article{Fang2023DepGraphTA,\\n author = {Gongfan Fang and Xinyin Ma and Mingli Song and M. B. Mi and Xinchao Wang},\\n booktitle = {Computer Vision and Pattern Recognition},\\n journal = {2023 IEEE/CVF Conference on Computer Vision and Pattern Recognition (CVPR)},\\n pages = {16091-16101},\\n title = {DepGraph: Towards Any Structural Pruning},\\n year = {2023}\\n}\\n\"},\"resources\":{\"github\":{\"url\":\"https://github.com/VainF/Torch-Pruning\",\"description\":\"[CVPR 2023] DepGraph: Towards Any Structural Pruning\",\"language\":\"Python\",\"stars\":2874}},\"paperVersions\":{\"_id\":\"67322376cd1e32a6e7efe4d5\",\"paper_group_id\":\"67322375cd1e32a6e7efe4bf\",\"version_label\":\"v2\",\"version_order\":2,\"title\":\"DepGraph: Towards Any Structural Pruning\",\"abstract\":\"$5e\",\"author_ids\":[\"672bbf7f986a1370676d5efc\",\"672bbf7f986a1370676d5efa\",\"672bd0c4986a1370676e0920\",\"672bd09d986a1370676e061d\",\"672bbf7f986a1370676d5efe\"],\"publication_date\":\"2023-03-23T12:55:02.000Z\",\"license\":\"http://arxiv.org/licenses/nonexclusive-distrib/1.0/\",\"created_at\":\"2024-11-11T15:32:06.303Z\",\"updated_at\":\"2024-11-11T15:32:06.303Z\",\"is_deleted\":false,\"is_hidden\":false,\"imageURL\":\"image/2301.12900v2.png\",\"universal_paper_id\":\"2301.12900\"},\"verifiedAuthors\":[],\"authors\":[{\"_id\":\"672bbf7f986a1370676d5efa\",\"full_name\":\"Xinyin Ma\",\"affiliation\":null,\"orcid\":null,\"semantic_scholarid\":null,\"user_id\":null},{\"_id\":\"672bbf7f986a1370676d5efc\",\"full_name\":\"Gongfan Fang\",\"affiliation\":null,\"orcid\":null,\"semantic_scholarid\":null,\"user_id\":null},{\"_id\":\"672bbf7f986a1370676d5efe\",\"full_name\":\"Xinchao Wang\",\"affiliation\":null,\"orcid\":null,\"semantic_scholarid\":null,\"user_id\":null},{\"_id\":\"672bd09d986a1370676e061d\",\"full_name\":\"Michael Bi Mi\",\"affiliation\":null,\"orcid\":null,\"semantic_scholarid\":null,\"user_id\":null},{\"_id\":\"672bd0c4986a1370676e0920\",\"full_name\":\"Mingli Song\",\"affiliation\":null,\"orcid\":null,\"semantic_scholarid\":null,\"user_id\":null}]},\"max_version_order\":2,\"verified_authors\":[],\"authors\":[{\"_id\":\"672bbf7f986a1370676d5efa\",\"full_name\":\"Xinyin Ma\",\"affiliation\":null,\"orcid\":null,\"semantic_scholarid\":null,\"user_id\":null},{\"_id\":\"672bbf7f986a1370676d5efc\",\"full_name\":\"Gongfan Fang\",\"affiliation\":null,\"orcid\":null,\"semantic_scholarid\":null,\"user_id\":null},{\"_id\":\"672bbf7f986a1370676d5efe\",\"full_name\":\"Xinchao Wang\",\"affiliation\":null,\"orcid\":null,\"semantic_scholarid\":null,\"user_id\":null},{\"_id\":\"672bd09d986a1370676e061d\",\"full_name\":\"Michael Bi Mi\",\"affiliation\":null,\"orcid\":null,\"semantic_scholarid\":null,\"user_id\":null},{\"_id\":\"672bd0c4986a1370676e0920\",\"full_name\":\"Mingli Song\",\"affiliation\":null,\"orcid\":null,\"semantic_scholarid\":null,\"user_id\":null}],\"pdf_info\":{\"fetcher_url\":\"https://fetcher.alphaxiv.org/v2/pdf/2301.12900v2\"}}},\"dataUpdateCount\":1,\"dataUpdatedAt\":1744228207987,\"error\":null,\"errorUpdateCount\":0,\"errorUpdatedAt\":0,\"fetchFailureCount\":0,\"fetchFailureReason\":null,\"fetchMeta\":null,\"isInvalidated\":false,\"status\":\"success\",\"fetchStatus\":\"idle\"},\"queryKey\":[\"paper\",\"2301.12900\",\"metadata\"],\"queryHash\":\"[\\\"paper\\\",\\\"2301.12900\\\",\\\"metadata\\\"]\"},{\"state\":{\"data\":{\"data\":[]},\"dataUpdateCount\":1,\"dataUpdatedAt\":1744228207987,\"error\":null,\"errorUpdateCount\":0,\"errorUpdatedAt\":0,\"fetchFailureCount\":0,\"fetchFailureReason\":null,\"fetchMeta\":null,\"isInvalidated\":false,\"status\":\"success\",\"fetchStatus\":\"idle\"},\"queryKey\":[\"paper\",\"2301.12900\",\"comments\"],\"queryHash\":\"[\\\"paper\\\",\\\"2301.12900\\\",\\\"comments\\\"]\"},{\"state\":{\"data\":{\"data\":{\"paper_version\":{\"_id\":\"672bcf50986a1370676de970\",\"paper_group_id\":\"672bcf4e986a1370676de93e\",\"version_label\":\"v2\",\"version_order\":2,\"title\":\"Sydr-Fuzz: Continuous Hybrid Fuzzing and Dynamic Analysis for Security\\n Development Lifecycle\",\"abstract\":\"$5f\",\"author_ids\":[\"672bcf4e986a1370676de944\",\"672bcf4e986a1370676de948\",\"672bcf4f986a1370676de94f\",\"672bcf4f986a1370676de957\",\"672bcf4f986a1370676de95d\",\"672bcf4f986a1370676de963\",\"672bcf50986a1370676de96b\"],\"publication_date\":\"2023-03-23T12:53:23.000Z\",\"license\":\"http://creativecommons.org/licenses/by-nc-nd/4.0/\",\"created_at\":\"2024-11-06T20:19:28.433Z\",\"updated_at\":\"2024-11-06T20:19:28.433Z\",\"is_deleted\":false,\"is_hidden\":false,\"imageURL\":\"image/2211.11595v2.png\",\"universal_paper_id\":\"2211.11595\"},\"paper_group\":{\"_id\":\"672bcf4e986a1370676de93e\",\"universal_paper_id\":\"2211.11595\",\"source\":{\"name\":\"alphaXiv\",\"url\":\"https://alphaxiv.org/paper/2211.11595\"},\"title\":\"Sydr-Fuzz: Continuous Hybrid Fuzzing and Dynamic Analysis for Security\\n Development Lifecycle\",\"created_at\":\"1970-01-01T00:00:00.000Z\",\"updated_at\":\"2025-03-03T20:18:39.604Z\",\"categories\":[\"Computer Science\"],\"subcategories\":[\"cs.CR\",\"cs.SE\"],\"metrics\":{\"activity_rank\":0,\"questions_count\":0,\"responses_count\":0,\"upvotes_count\":null,\"downvotes_count\":0,\"total_votes\":0,\"visits_count\":{\"last24Hours\":0,\"last7Days\":4,\"last30Days\":18,\"last90Days\":21,\"all\":67},\"weighted_visits\":{\"last24Hours\":0,\"last7Days\":1.5217279312530693e-18,\"last30Days\":0.0009064359951218224,\"last90Days\":0.7754863791119408,\"hot\":1.5217279312530693e-18},\"public_total_votes\":0,\"timeline\":[{\"date\":\"2025-04-03T01:57:41.792Z\",\"views\":2},{\"date\":\"2025-03-30T13:57:41.792Z\",\"views\":14},{\"date\":\"2025-03-27T01:57:41.792Z\",\"views\":2},{\"date\":\"2025-03-23T13:57:41.792Z\",\"views\":42},{\"date\":\"2025-03-20T01:57:41.792Z\",\"views\":2},{\"date\":\"2025-03-16T13:57:41.792Z\",\"views\":1},{\"date\":\"2025-03-13T01:57:41.792Z\",\"views\":2},{\"date\":\"2025-03-09T13:57:41.792Z\",\"views\":2},{\"date\":\"2025-03-06T01:57:41.792Z\",\"views\":2},{\"date\":\"2025-03-02T13:57:41.792Z\",\"views\":0},{\"date\":\"2025-02-27T01:57:41.792Z\",\"views\":2},{\"date\":\"2025-02-23T13:57:41.792Z\",\"views\":2},{\"date\":\"2025-02-20T01:57:41.807Z\",\"views\":0},{\"date\":\"2025-02-16T13:57:41.827Z\",\"views\":1},{\"date\":\"2025-02-13T01:57:41.846Z\",\"views\":2},{\"date\":\"2025-02-09T13:57:41.868Z\",\"views\":0},{\"date\":\"2025-02-06T01:57:41.891Z\",\"views\":1},{\"date\":\"2025-02-02T13:57:41.911Z\",\"views\":4},{\"date\":\"2025-01-30T01:57:41.938Z\",\"views\":0},{\"date\":\"2025-01-26T13:57:41.962Z\",\"views\":1},{\"date\":\"2025-01-23T01:57:41.990Z\",\"views\":3},{\"date\":\"2025-01-19T13:57:42.010Z\",\"views\":1},{\"date\":\"2025-01-16T01:57:42.031Z\",\"views\":1},{\"date\":\"2025-01-12T13:57:42.054Z\",\"views\":1},{\"date\":\"2025-01-09T01:57:42.082Z\",\"views\":4},{\"date\":\"2025-01-05T13:57:42.114Z\",\"views\":2},{\"date\":\"2025-01-02T01:57:42.135Z\",\"views\":0},{\"date\":\"2024-12-29T13:57:42.160Z\",\"views\":0},{\"date\":\"2024-12-26T01:57:42.183Z\",\"views\":1},{\"date\":\"2024-12-22T13:57:42.204Z\",\"views\":0},{\"date\":\"2024-12-19T01:57:42.232Z\",\"views\":2},{\"date\":\"2024-12-15T13:57:42.253Z\",\"views\":0},{\"date\":\"2024-12-12T01:57:42.278Z\",\"views\":2},{\"date\":\"2024-12-08T13:57:42.299Z\",\"views\":1},{\"date\":\"2024-12-05T01:57:42.320Z\",\"views\":0},{\"date\":\"2024-12-01T13:57:42.342Z\",\"views\":2},{\"date\":\"2024-11-28T01:57:42.364Z\",\"views\":0},{\"date\":\"2024-11-24T13:57:42.389Z\",\"views\":0},{\"date\":\"2024-11-21T01:57:42.410Z\",\"views\":1},{\"date\":\"2024-11-17T13:57:42.436Z\",\"views\":1},{\"date\":\"2024-11-14T01:57:42.457Z\",\"views\":2},{\"date\":\"2024-11-10T13:57:42.478Z\",\"views\":1},{\"date\":\"2024-11-07T01:57:42.503Z\",\"views\":1},{\"date\":\"2024-11-03T13:57:42.523Z\",\"views\":2},{\"date\":\"2024-10-31T00:57:42.547Z\",\"views\":0},{\"date\":\"2024-10-27T12:57:42.573Z\",\"views\":5},{\"date\":\"2024-10-24T00:57:42.606Z\",\"views\":1},{\"date\":\"2024-10-20T12:57:42.638Z\",\"views\":1},{\"date\":\"2024-10-17T00:57:42.661Z\",\"views\":2},{\"date\":\"2024-10-13T12:57:42.685Z\",\"views\":2},{\"date\":\"2024-10-10T00:57:42.710Z\",\"views\":2},{\"date\":\"2024-10-06T12:57:42.751Z\",\"views\":1},{\"date\":\"2024-10-03T00:57:42.777Z\",\"views\":2},{\"date\":\"2024-09-29T12:57:42.799Z\",\"views\":1},{\"date\":\"2024-09-26T00:57:42.819Z\",\"views\":2},{\"date\":\"2024-09-22T12:57:42.841Z\",\"views\":1},{\"date\":\"2024-09-19T00:57:42.863Z\",\"views\":1},{\"date\":\"2024-09-15T12:57:42.888Z\",\"views\":1},{\"date\":\"2024-09-12T00:57:42.911Z\",\"views\":1},{\"date\":\"2024-09-08T12:57:42.936Z\",\"views\":2},{\"date\":\"2024-09-05T00:57:42.956Z\",\"views\":1},{\"date\":\"2024-09-01T12:57:42.979Z\",\"views\":2},{\"date\":\"2024-08-29T00:57:43.004Z\",\"views\":1}]},\"ranking\":{\"current_rank\":21836,\"previous_rank\":21832,\"activity_score\":0,\"paper_score\":0.34657359027997264},\"is_hidden\":false,\"custom_categories\":null,\"first_publication_date\":\"2023-03-23T12:53:23.000Z\",\"author_user_ids\":[],\"citation\":{\"bibtex\":\"@Article{Vishnyakov2022SydrFuzzCH,\\n author = {A. Vishnyakov and D. Kuts and V. Logunova and Darya Parygina and Eli Kobrin and Georgy Savidov and A. Fedotov},\\n booktitle = {2022 Ivannikov Ispras Open Conference (ISPRAS)},\\n journal = {2022 Ivannikov Ispras Open Conference (ISPRAS)},\\n pages = {111-123},\\n title = {Sydr-Fuzz: Continuous Hybrid Fuzzing and Dynamic Analysis for Security Development Lifecycle},\\n year = {2022}\\n}\\n\"},\"paperVersions\":{\"_id\":\"672bcf50986a1370676de970\",\"paper_group_id\":\"672bcf4e986a1370676de93e\",\"version_label\":\"v2\",\"version_order\":2,\"title\":\"Sydr-Fuzz: Continuous Hybrid Fuzzing and Dynamic Analysis for Security\\n Development Lifecycle\",\"abstract\":\"$60\",\"author_ids\":[\"672bcf4e986a1370676de944\",\"672bcf4e986a1370676de948\",\"672bcf4f986a1370676de94f\",\"672bcf4f986a1370676de957\",\"672bcf4f986a1370676de95d\",\"672bcf4f986a1370676de963\",\"672bcf50986a1370676de96b\"],\"publication_date\":\"2023-03-23T12:53:23.000Z\",\"license\":\"http://creativecommons.org/licenses/by-nc-nd/4.0/\",\"created_at\":\"2024-11-06T20:19:28.433Z\",\"updated_at\":\"2024-11-06T20:19:28.433Z\",\"is_deleted\":false,\"is_hidden\":false,\"imageURL\":\"image/2211.11595v2.png\",\"universal_paper_id\":\"2211.11595\"},\"verifiedAuthors\":[],\"authors\":[{\"_id\":\"672bcf4e986a1370676de944\",\"full_name\":\"Alexey Vishnyakov\",\"affiliation\":null,\"orcid\":null,\"semantic_scholarid\":null,\"user_id\":null},{\"_id\":\"672bcf4e986a1370676de948\",\"full_name\":\"Daniil Kuts\",\"affiliation\":null,\"orcid\":null,\"semantic_scholarid\":null,\"user_id\":null},{\"_id\":\"672bcf4f986a1370676de94f\",\"full_name\":\"Vlada Logunova\",\"affiliation\":null,\"orcid\":null,\"semantic_scholarid\":null,\"user_id\":null},{\"_id\":\"672bcf4f986a1370676de957\",\"full_name\":\"Darya Parygina\",\"affiliation\":null,\"orcid\":null,\"semantic_scholarid\":null,\"user_id\":null},{\"_id\":\"672bcf4f986a1370676de95d\",\"full_name\":\"Eli Kobrin\",\"affiliation\":null,\"orcid\":null,\"semantic_scholarid\":null,\"user_id\":null},{\"_id\":\"672bcf4f986a1370676de963\",\"full_name\":\"Georgy Savidov\",\"affiliation\":null,\"orcid\":null,\"semantic_scholarid\":null,\"user_id\":null},{\"_id\":\"672bcf50986a1370676de96b\",\"full_name\":\"Andrey Fedotov\",\"affiliation\":null,\"orcid\":null,\"semantic_scholarid\":null,\"user_id\":null}]},\"max_version_order\":2,\"verified_authors\":[],\"authors\":[{\"_id\":\"672bcf4e986a1370676de944\",\"full_name\":\"Alexey Vishnyakov\",\"affiliation\":null,\"orcid\":null,\"semantic_scholarid\":null,\"user_id\":null},{\"_id\":\"672bcf4e986a1370676de948\",\"full_name\":\"Daniil Kuts\",\"affiliation\":null,\"orcid\":null,\"semantic_scholarid\":null,\"user_id\":null},{\"_id\":\"672bcf4f986a1370676de94f\",\"full_name\":\"Vlada Logunova\",\"affiliation\":null,\"orcid\":null,\"semantic_scholarid\":null,\"user_id\":null},{\"_id\":\"672bcf4f986a1370676de957\",\"full_name\":\"Darya Parygina\",\"affiliation\":null,\"orcid\":null,\"semantic_scholarid\":null,\"user_id\":null},{\"_id\":\"672bcf4f986a1370676de95d\",\"full_name\":\"Eli Kobrin\",\"affiliation\":null,\"orcid\":null,\"semantic_scholarid\":null,\"user_id\":null},{\"_id\":\"672bcf4f986a1370676de963\",\"full_name\":\"Georgy Savidov\",\"affiliation\":null,\"orcid\":null,\"semantic_scholarid\":null,\"user_id\":null},{\"_id\":\"672bcf50986a1370676de96b\",\"full_name\":\"Andrey Fedotov\",\"affiliation\":null,\"orcid\":null,\"semantic_scholarid\":null,\"user_id\":null}],\"pdf_info\":{\"fetcher_url\":\"https://fetcher.alphaxiv.org/v2/pdf/2211.11595v2\"}}},\"dataUpdateCount\":1,\"dataUpdatedAt\":1744228208328,\"error\":null,\"errorUpdateCount\":0,\"errorUpdatedAt\":0,\"fetchFailureCount\":0,\"fetchFailureReason\":null,\"fetchMeta\":null,\"isInvalidated\":false,\"status\":\"success\",\"fetchStatus\":\"idle\"},\"queryKey\":[\"paper\",\"2211.11595\",\"metadata\"],\"queryHash\":\"[\\\"paper\\\",\\\"2211.11595\\\",\\\"metadata\\\"]\"},{\"state\":{\"data\":{\"data\":[]},\"dataUpdateCount\":1,\"dataUpdatedAt\":1744228208328,\"error\":null,\"errorUpdateCount\":0,\"errorUpdatedAt\":0,\"fetchFailureCount\":0,\"fetchFailureReason\":null,\"fetchMeta\":null,\"isInvalidated\":false,\"status\":\"success\",\"fetchStatus\":\"idle\"},\"queryKey\":[\"paper\",\"2211.11595\",\"comments\"],\"queryHash\":\"[\\\"paper\\\",\\\"2211.11595\\\",\\\"comments\\\"]\"},{\"state\":{\"data\":{\"data\":{\"paper_version\":{\"_id\":\"67447c3cd2555cdbdff92475\",\"paper_group_id\":\"67447c3bd2555cdbdff92474\",\"version_label\":\"v1\",\"version_order\":1,\"title\":\"TAPS3D: Text-Guided 3D Textured Shape Generation from Pseudo Supervision\",\"abstract\":\"$61\",\"author_ids\":[\"673cf74f615941b897fb700d\",\"672bbca9986a1370676d5032\",\"672bc60c986a1370676d68a0\",\"672bcc37986a1370676db243\",\"673cad798a52218f8bc901f8\"],\"publication_date\":\"2023-03-23T13:53:16.000Z\",\"license\":\"http://creativecommons.org/licenses/by-nc-sa/4.0/\",\"created_at\":\"2024-11-25T13:31:40.209Z\",\"updated_at\":\"2024-11-25T13:31:40.209Z\",\"is_deleted\":false,\"is_hidden\":false,\"universal_paper_id\":\"2303.13273\",\"imageURL\":\"image/2303.13273v1.png\"},\"paper_group\":{\"_id\":\"67447c3bd2555cdbdff92474\",\"universal_paper_id\":\"2303.13273\",\"source\":{\"name\":\"arXiv\",\"url\":\"https://arXiv.org/paper/2303.13273\"},\"title\":\"TAPS3D: Text-Guided 3D Textured Shape Generation from Pseudo Supervision\",\"created_at\":\"2024-11-25T13:12:05.031Z\",\"updated_at\":\"2025-03-03T20:18:39.599Z\",\"categories\":[\"Computer Science\"],\"subcategories\":[\"cs.CV\"],\"metrics\":{\"activity_rank\":0,\"questions_count\":0,\"responses_count\":0,\"upvotes_count\":null,\"downvotes_count\":null,\"total_votes\":0,\"visits_count\":{\"last24Hours\":0,\"last7Days\":0,\"last30Days\":2,\"last90Days\":10,\"all\":42},\"weighted_visits\":{\"last24Hours\":0,\"last7Days\":0,\"last30Days\":0.00010077097558557207,\"last90Days\":0.369347493234387,\"hot\":0},\"public_total_votes\":0,\"timeline\":[{\"date\":\"2025-04-03T01:57:38.334Z\",\"views\":1},{\"date\":\"2025-03-30T13:57:38.334Z\",\"views\":1},{\"date\":\"2025-03-27T01:57:38.334Z\",\"views\":2},{\"date\":\"2025-03-23T13:57:38.334Z\",\"views\":2},{\"date\":\"2025-03-20T01:57:38.334Z\",\"views\":2},{\"date\":\"2025-03-16T13:57:38.334Z\",\"views\":6},{\"date\":\"2025-03-13T01:57:38.334Z\",\"views\":0},{\"date\":\"2025-03-09T13:57:38.334Z\",\"views\":0},{\"date\":\"2025-03-06T01:57:38.334Z\",\"views\":1},{\"date\":\"2025-03-02T13:57:38.334Z\",\"views\":2},{\"date\":\"2025-02-27T01:57:38.334Z\",\"views\":2},{\"date\":\"2025-02-23T13:57:38.334Z\",\"views\":0},{\"date\":\"2025-02-20T01:57:38.370Z\",\"views\":10},{\"date\":\"2025-02-16T13:57:38.394Z\",\"views\":2},{\"date\":\"2025-02-13T01:57:38.414Z\",\"views\":2},{\"date\":\"2025-02-09T13:57:38.437Z\",\"views\":2},{\"date\":\"2025-02-06T01:57:38.459Z\",\"views\":2},{\"date\":\"2025-02-02T13:57:38.481Z\",\"views\":0},{\"date\":\"2025-01-30T01:57:38.505Z\",\"views\":0},{\"date\":\"2025-01-26T13:57:38.529Z\",\"views\":0},{\"date\":\"2025-01-23T01:57:38.550Z\",\"views\":1},{\"date\":\"2025-01-19T13:57:38.569Z\",\"views\":1},{\"date\":\"2025-01-16T01:57:38.589Z\",\"views\":1},{\"date\":\"2025-01-12T13:57:38.610Z\",\"views\":2},{\"date\":\"2025-01-09T01:57:38.630Z\",\"views\":15},{\"date\":\"2025-01-05T13:57:38.649Z\",\"views\":2},{\"date\":\"2025-01-02T01:57:38.682Z\",\"views\":5},{\"date\":\"2024-12-29T13:57:38.702Z\",\"views\":1},{\"date\":\"2024-12-26T01:57:38.722Z\",\"views\":5},{\"date\":\"2024-12-22T13:57:38.760Z\",\"views\":2},{\"date\":\"2024-12-19T01:57:38.783Z\",\"views\":1},{\"date\":\"2024-12-15T13:57:38.803Z\",\"views\":1},{\"date\":\"2024-12-12T01:57:38.821Z\",\"views\":1},{\"date\":\"2024-12-08T13:57:38.855Z\",\"views\":2},{\"date\":\"2024-12-05T01:57:38.876Z\",\"views\":0},{\"date\":\"2024-12-01T13:57:38.898Z\",\"views\":1},{\"date\":\"2024-11-28T01:57:38.918Z\",\"views\":0},{\"date\":\"2024-11-24T13:57:38.954Z\",\"views\":6},{\"date\":\"2024-11-21T01:57:38.983Z\",\"views\":2},{\"date\":\"2024-11-17T13:57:39.006Z\",\"views\":2},{\"date\":\"2024-11-14T01:57:39.028Z\",\"views\":0},{\"date\":\"2024-11-10T13:57:39.052Z\",\"views\":2},{\"date\":\"2024-11-07T01:57:39.073Z\",\"views\":2},{\"date\":\"2024-11-03T13:57:39.094Z\",\"views\":0},{\"date\":\"2024-10-31T00:57:39.118Z\",\"views\":1},{\"date\":\"2024-10-27T12:57:39.140Z\",\"views\":1},{\"date\":\"2024-10-24T00:57:39.164Z\",\"views\":1},{\"date\":\"2024-10-20T12:57:39.189Z\",\"views\":1},{\"date\":\"2024-10-17T00:57:39.212Z\",\"views\":2},{\"date\":\"2024-10-13T12:57:39.244Z\",\"views\":2},{\"date\":\"2024-10-10T00:57:39.270Z\",\"views\":1},{\"date\":\"2024-10-06T12:57:39.290Z\",\"views\":0},{\"date\":\"2024-10-03T00:57:39.317Z\",\"views\":2},{\"date\":\"2024-09-29T12:57:39.344Z\",\"views\":1},{\"date\":\"2024-09-26T00:57:39.371Z\",\"views\":1},{\"date\":\"2024-09-22T12:57:39.410Z\",\"views\":1},{\"date\":\"2024-09-19T00:57:39.431Z\",\"views\":0},{\"date\":\"2024-09-15T12:57:39.455Z\",\"views\":1},{\"date\":\"2024-09-12T00:57:39.481Z\",\"views\":2},{\"date\":\"2024-09-08T12:57:39.508Z\",\"views\":2},{\"date\":\"2024-09-05T00:57:39.531Z\",\"views\":0},{\"date\":\"2024-09-01T12:57:39.544Z\",\"views\":1},{\"date\":\"2024-08-29T00:57:39.553Z\",\"views\":2}]},\"ranking\":{\"current_rank\":0,\"previous_rank\":0,\"activity_score\":0,\"paper_score\":0},\"is_hidden\":false,\"custom_categories\":[\"text-generation\",\"generative-models\",\"vision-language-models\",\"self-supervised-learning\",\"image-generation\"],\"first_publication_date\":\"2023-03-23T13:53:16.000Z\",\"author_user_ids\":[],\"resources\":{\"github\":{\"url\":\"https://github.com/plusmultiply/TAPS3D\",\"description\":\"Official code repository for the paper: \\\"TAPS3D: Text-Guided 3D Textured Shape Generation from Pseudo Supervision\\\"\",\"language\":\"Python\",\"stars\":40}},\"organizations\":[\"67be6379aa92218ccd8b10c5\",\"67be6377aa92218ccd8b0fe7\"],\"paperVersions\":{\"_id\":\"67447c3cd2555cdbdff92475\",\"paper_group_id\":\"67447c3bd2555cdbdff92474\",\"version_label\":\"v1\",\"version_order\":1,\"title\":\"TAPS3D: Text-Guided 3D Textured Shape Generation from Pseudo Supervision\",\"abstract\":\"$62\",\"author_ids\":[\"673cf74f615941b897fb700d\",\"672bbca9986a1370676d5032\",\"672bc60c986a1370676d68a0\",\"672bcc37986a1370676db243\",\"673cad798a52218f8bc901f8\"],\"publication_date\":\"2023-03-23T13:53:16.000Z\",\"license\":\"http://creativecommons.org/licenses/by-nc-sa/4.0/\",\"created_at\":\"2024-11-25T13:31:40.209Z\",\"updated_at\":\"2024-11-25T13:31:40.209Z\",\"is_deleted\":false,\"is_hidden\":false,\"universal_paper_id\":\"2303.13273\",\"imageURL\":\"image/2303.13273v1.png\"},\"verifiedAuthors\":[],\"authors\":[{\"_id\":\"672bbca9986a1370676d5032\",\"full_name\":\"Hao Wang\",\"affiliation\":null,\"orcid\":null,\"semantic_scholarid\":null,\"user_id\":null},{\"_id\":\"672bc60c986a1370676d68a0\",\"full_name\":\"Jiashi Feng\",\"affiliation\":null,\"orcid\":null,\"semantic_scholarid\":null,\"user_id\":null},{\"_id\":\"672bcc37986a1370676db243\",\"full_name\":\"Guosheng Lin\",\"affiliation\":null,\"orcid\":null,\"semantic_scholarid\":null,\"user_id\":null},{\"_id\":\"673cad798a52218f8bc901f8\",\"full_name\":\"Kim-Hui Yap\",\"affiliation\":null,\"orcid\":null,\"semantic_scholarid\":null,\"user_id\":null},{\"_id\":\"673cf74f615941b897fb700d\",\"full_name\":\"Jiacheng Wei\",\"affiliation\":null,\"orcid\":null,\"semantic_scholarid\":null,\"user_id\":null}]},\"max_version_order\":1,\"verified_authors\":[],\"authors\":[{\"_id\":\"672bbca9986a1370676d5032\",\"full_name\":\"Hao Wang\",\"affiliation\":null,\"orcid\":null,\"semantic_scholarid\":null,\"user_id\":null},{\"_id\":\"672bc60c986a1370676d68a0\",\"full_name\":\"Jiashi Feng\",\"affiliation\":null,\"orcid\":null,\"semantic_scholarid\":null,\"user_id\":null},{\"_id\":\"672bcc37986a1370676db243\",\"full_name\":\"Guosheng Lin\",\"affiliation\":null,\"orcid\":null,\"semantic_scholarid\":null,\"user_id\":null},{\"_id\":\"673cad798a52218f8bc901f8\",\"full_name\":\"Kim-Hui Yap\",\"affiliation\":null,\"orcid\":null,\"semantic_scholarid\":null,\"user_id\":null},{\"_id\":\"673cf74f615941b897fb700d\",\"full_name\":\"Jiacheng Wei\",\"affiliation\":null,\"orcid\":null,\"semantic_scholarid\":null,\"user_id\":null}],\"pdf_info\":{\"fetcher_url\":\"https://fetcher.alphaxiv.org/v2/pdf/2303.13273v1\"}}},\"dataUpdateCount\":1,\"dataUpdatedAt\":1744228208504,\"error\":null,\"errorUpdateCount\":0,\"errorUpdatedAt\":0,\"fetchFailureCount\":0,\"fetchFailureReason\":null,\"fetchMeta\":null,\"isInvalidated\":false,\"status\":\"success\",\"fetchStatus\":\"idle\"},\"queryKey\":[\"paper\",\"2303.13273\",\"metadata\"],\"queryHash\":\"[\\\"paper\\\",\\\"2303.13273\\\",\\\"metadata\\\"]\"},{\"state\":{\"data\":{\"data\":[]},\"dataUpdateCount\":1,\"dataUpdatedAt\":1744228208504,\"error\":null,\"errorUpdateCount\":0,\"errorUpdatedAt\":0,\"fetchFailureCount\":0,\"fetchFailureReason\":null,\"fetchMeta\":null,\"isInvalidated\":false,\"status\":\"success\",\"fetchStatus\":\"idle\"},\"queryKey\":[\"paper\",\"2303.13273\",\"comments\"],\"queryHash\":\"[\\\"paper\\\",\\\"2303.13273\\\",\\\"comments\\\"]\"},{\"state\":{\"data\":{\"data\":{\"paper_version\":{\"_id\":\"673dd40d181e8ac859339b7e\",\"paper_group_id\":\"673dd40c181e8ac859339b77\",\"version_label\":\"v1\",\"version_order\":1,\"title\":\"Avast-CTU Public CAPE Dataset\",\"abstract\":\"$63\",\"author_ids\":[\"673dd40c181e8ac859339b78\",\"673dd40c181e8ac859339b79\",\"673dd40d181e8ac859339b7a\",\"673dd40d181e8ac859339b7b\",\"6732281ecd1e32a6e7f03284\",\"673dd40d181e8ac859339b7c\",\"673dd40d181e8ac859339b7d\"],\"publication_date\":\"2022-09-06T13:22:27.000Z\",\"license\":\"http://arxiv.org/licenses/nonexclusive-distrib/1.0/\",\"created_at\":\"2024-11-20T12:20:29.830Z\",\"updated_at\":\"2024-11-20T12:20:29.830Z\",\"is_deleted\":false,\"is_hidden\":false,\"universal_paper_id\":\"2209.03188\",\"imageURL\":\"image/2209.03188v1.png\"},\"paper_group\":{\"_id\":\"673dd40c181e8ac859339b77\",\"universal_paper_id\":\"2209.03188\",\"source\":{\"name\":\"arXiv\",\"url\":\"https://arXiv.org/paper/2209.03188\"},\"title\":\"Avast-CTU Public CAPE Dataset\",\"created_at\":\"2024-11-12T11:29:47.210Z\",\"updated_at\":\"2025-03-03T20:27:34.515Z\",\"categories\":[\"Computer Science\"],\"subcategories\":[\"cs.CR\",\"cs.AI\",\"cs.LG\"],\"metrics\":{\"activity_rank\":0,\"questions_count\":0,\"responses_count\":0,\"upvotes_count\":null,\"downvotes_count\":null,\"total_votes\":0,\"visits_count\":{\"last24Hours\":0,\"last7Days\":3,\"last30Days\":11,\"last90Days\":20,\"all\":79},\"weighted_visits\":{\"last24Hours\":0,\"last7Days\":1.3923359441399366e-23,\"last30Days\":0.00003953101819355885,\"last90Days\":0.3063456849678029,\"hot\":1.3923359441399366e-23},\"public_total_votes\":1,\"timeline\":[{\"date\":\"2025-04-02T02:53:34.893Z\",\"views\":10},{\"date\":\"2025-03-29T14:53:34.893Z\",\"views\":5},{\"date\":\"2025-03-26T02:53:34.893Z\",\"views\":2},{\"date\":\"2025-03-22T14:53:34.893Z\",\"views\":11},{\"date\":\"2025-03-19T02:53:34.893Z\",\"views\":14},{\"date\":\"2025-03-15T14:53:34.893Z\",\"views\":0},{\"date\":\"2025-03-12T02:53:34.893Z\",\"views\":2},{\"date\":\"2025-03-08T14:53:34.893Z\",\"views\":0},{\"date\":\"2025-03-05T02:53:34.893Z\",\"views\":2},{\"date\":\"2025-03-01T14:53:34.893Z\",\"views\":2},{\"date\":\"2025-02-26T02:53:34.893Z\",\"views\":2},{\"date\":\"2025-02-22T14:53:34.893Z\",\"views\":1},{\"date\":\"2025-02-19T02:53:34.909Z\",\"views\":0},{\"date\":\"2025-02-15T14:53:34.922Z\",\"views\":9},{\"date\":\"2025-02-12T02:53:34.941Z\",\"views\":2},{\"date\":\"2025-02-08T14:53:34.961Z\",\"views\":2},{\"date\":\"2025-02-05T02:53:34.974Z\",\"views\":2},{\"date\":\"2025-02-01T14:53:34.990Z\",\"views\":1},{\"date\":\"2025-01-29T02:53:35.014Z\",\"views\":0},{\"date\":\"2025-01-25T14:53:35.032Z\",\"views\":2},{\"date\":\"2025-01-22T02:53:35.049Z\",\"views\":13},{\"date\":\"2025-01-18T14:53:35.065Z\",\"views\":6},{\"date\":\"2025-01-15T02:53:35.082Z\",\"views\":1},{\"date\":\"2025-01-11T14:53:35.098Z\",\"views\":0},{\"date\":\"2025-01-08T02:53:35.117Z\",\"views\":1},{\"date\":\"2025-01-04T14:53:35.132Z\",\"views\":1},{\"date\":\"2025-01-01T02:53:35.154Z\",\"views\":2},{\"date\":\"2024-12-28T14:53:35.171Z\",\"views\":3},{\"date\":\"2024-12-25T02:53:35.196Z\",\"views\":1},{\"date\":\"2024-12-21T14:53:35.216Z\",\"views\":2},{\"date\":\"2024-12-18T02:53:35.231Z\",\"views\":1},{\"date\":\"2024-12-14T14:53:35.248Z\",\"views\":2},{\"date\":\"2024-12-11T02:53:35.266Z\",\"views\":2},{\"date\":\"2024-12-07T14:53:35.286Z\",\"views\":3},{\"date\":\"2024-12-04T02:53:35.302Z\",\"views\":2},{\"date\":\"2024-11-30T14:53:35.319Z\",\"views\":1},{\"date\":\"2024-11-27T02:53:35.335Z\",\"views\":3},{\"date\":\"2024-11-23T14:53:35.353Z\",\"views\":4},{\"date\":\"2024-11-20T02:53:35.371Z\",\"views\":1},{\"date\":\"2024-11-16T14:53:35.387Z\",\"views\":1},{\"date\":\"2024-11-13T02:53:35.408Z\",\"views\":2},{\"date\":\"2024-11-09T14:53:35.423Z\",\"views\":7},{\"date\":\"2024-11-06T02:53:35.440Z\",\"views\":1},{\"date\":\"2024-11-02T13:53:35.461Z\",\"views\":0},{\"date\":\"2024-10-30T01:53:35.477Z\",\"views\":2},{\"date\":\"2024-10-26T13:53:35.495Z\",\"views\":2},{\"date\":\"2024-10-23T01:53:35.513Z\",\"views\":2},{\"date\":\"2024-10-19T13:53:35.530Z\",\"views\":0},{\"date\":\"2024-10-16T01:53:35.544Z\",\"views\":0},{\"date\":\"2024-10-12T13:53:35.562Z\",\"views\":1},{\"date\":\"2024-10-09T01:53:35.578Z\",\"views\":2},{\"date\":\"2024-10-05T13:53:35.608Z\",\"views\":1},{\"date\":\"2024-10-02T01:53:35.626Z\",\"views\":0},{\"date\":\"2024-09-28T13:53:35.641Z\",\"views\":0},{\"date\":\"2024-09-25T01:53:35.656Z\",\"views\":1},{\"date\":\"2024-09-21T13:53:35.671Z\",\"views\":1},{\"date\":\"2024-09-18T01:53:35.688Z\",\"views\":0},{\"date\":\"2024-09-14T13:53:35.707Z\",\"views\":2},{\"date\":\"2024-09-11T01:53:35.726Z\",\"views\":2},{\"date\":\"2024-09-07T13:53:35.758Z\",\"views\":0},{\"date\":\"2024-09-04T01:53:35.775Z\",\"views\":0},{\"date\":\"2024-08-31T13:53:35.785Z\",\"views\":1},{\"date\":\"2024-08-28T01:53:35.799Z\",\"views\":1}]},\"ranking\":{\"current_rank\":18250,\"previous_rank\":16730,\"activity_score\":0,\"paper_score\":0.5493061443340548},\"is_hidden\":false,\"custom_categories\":[\"information-extraction\",\"security\",\"machine-learning\"],\"first_publication_date\":\"2022-09-06T13:22:27.000Z\",\"author_user_ids\":[],\"organizations\":[\"67c39bd96238d4c4ef214203\",\"67c522797a0238cd90171091\"],\"citation\":{\"bibtex\":\"@misc{lisy2022avastctupubliccape,\\n title={Avast-CTU Public CAPE Dataset}, \\n author={Viliam Lisy and Branislav Bosansky and Dominik Kouba and Ondrej Manhal and Thorsten Sick and Jakub Kroustek and Petr Somol},\\n year={2022},\\n eprint={2209.03188},\\n archivePrefix={arXiv},\\n primaryClass={cs.CR},\\n url={https://arxiv.org/abs/2209.03188}, \\n}\"},\"paperVersions\":{\"_id\":\"673dd40d181e8ac859339b7e\",\"paper_group_id\":\"673dd40c181e8ac859339b77\",\"version_label\":\"v1\",\"version_order\":1,\"title\":\"Avast-CTU Public CAPE Dataset\",\"abstract\":\"$64\",\"author_ids\":[\"673dd40c181e8ac859339b78\",\"673dd40c181e8ac859339b79\",\"673dd40d181e8ac859339b7a\",\"673dd40d181e8ac859339b7b\",\"6732281ecd1e32a6e7f03284\",\"673dd40d181e8ac859339b7c\",\"673dd40d181e8ac859339b7d\"],\"publication_date\":\"2022-09-06T13:22:27.000Z\",\"license\":\"http://arxiv.org/licenses/nonexclusive-distrib/1.0/\",\"created_at\":\"2024-11-20T12:20:29.830Z\",\"updated_at\":\"2024-11-20T12:20:29.830Z\",\"is_deleted\":false,\"is_hidden\":false,\"universal_paper_id\":\"2209.03188\",\"imageURL\":\"image/2209.03188v1.png\"},\"verifiedAuthors\":[],\"authors\":[{\"_id\":\"6732281ecd1e32a6e7f03284\",\"full_name\":\"Viliam Lisy\",\"affiliation\":null,\"orcid\":null,\"semantic_scholarid\":null,\"user_id\":null},{\"_id\":\"673dd40c181e8ac859339b78\",\"full_name\":\"Branislav Bosansky\",\"affiliation\":null,\"orcid\":null,\"semantic_scholarid\":null,\"user_id\":null},{\"_id\":\"673dd40c181e8ac859339b79\",\"full_name\":\"Dominik Kouba\",\"affiliation\":null,\"orcid\":null,\"semantic_scholarid\":null,\"user_id\":null},{\"_id\":\"673dd40d181e8ac859339b7a\",\"full_name\":\"Ondrej Manhal\",\"affiliation\":null,\"orcid\":null,\"semantic_scholarid\":null,\"user_id\":null},{\"_id\":\"673dd40d181e8ac859339b7b\",\"full_name\":\"Thorsten Sick\",\"affiliation\":null,\"orcid\":null,\"semantic_scholarid\":null,\"user_id\":null},{\"_id\":\"673dd40d181e8ac859339b7c\",\"full_name\":\"Jakub Kroustek\",\"affiliation\":null,\"orcid\":null,\"semantic_scholarid\":null,\"user_id\":null},{\"_id\":\"673dd40d181e8ac859339b7d\",\"full_name\":\"Petr Somol\",\"affiliation\":null,\"orcid\":null,\"semantic_scholarid\":null,\"user_id\":null}]},\"max_version_order\":1,\"verified_authors\":[],\"authors\":[{\"_id\":\"6732281ecd1e32a6e7f03284\",\"full_name\":\"Viliam Lisy\",\"affiliation\":null,\"orcid\":null,\"semantic_scholarid\":null,\"user_id\":null},{\"_id\":\"673dd40c181e8ac859339b78\",\"full_name\":\"Branislav Bosansky\",\"affiliation\":null,\"orcid\":null,\"semantic_scholarid\":null,\"user_id\":null},{\"_id\":\"673dd40c181e8ac859339b79\",\"full_name\":\"Dominik Kouba\",\"affiliation\":null,\"orcid\":null,\"semantic_scholarid\":null,\"user_id\":null},{\"_id\":\"673dd40d181e8ac859339b7a\",\"full_name\":\"Ondrej Manhal\",\"affiliation\":null,\"orcid\":null,\"semantic_scholarid\":null,\"user_id\":null},{\"_id\":\"673dd40d181e8ac859339b7b\",\"full_name\":\"Thorsten Sick\",\"affiliation\":null,\"orcid\":null,\"semantic_scholarid\":null,\"user_id\":null},{\"_id\":\"673dd40d181e8ac859339b7c\",\"full_name\":\"Jakub Kroustek\",\"affiliation\":null,\"orcid\":null,\"semantic_scholarid\":null,\"user_id\":null},{\"_id\":\"673dd40d181e8ac859339b7d\",\"full_name\":\"Petr Somol\",\"affiliation\":null,\"orcid\":null,\"semantic_scholarid\":null,\"user_id\":null}],\"pdf_info\":{\"fetcher_url\":\"https://fetcher.alphaxiv.org/v2/pdf/2209.03188v1\"}}},\"dataUpdateCount\":1,\"dataUpdatedAt\":1744228208850,\"error\":null,\"errorUpdateCount\":0,\"errorUpdatedAt\":0,\"fetchFailureCount\":0,\"fetchFailureReason\":null,\"fetchMeta\":null,\"isInvalidated\":false,\"status\":\"success\",\"fetchStatus\":\"idle\"},\"queryKey\":[\"paper\",\"2209.03188\",\"metadata\"],\"queryHash\":\"[\\\"paper\\\",\\\"2209.03188\\\",\\\"metadata\\\"]\"},{\"state\":{\"data\":{\"data\":[]},\"dataUpdateCount\":1,\"dataUpdatedAt\":1744228208850,\"error\":null,\"errorUpdateCount\":0,\"errorUpdatedAt\":0,\"fetchFailureCount\":0,\"fetchFailureReason\":null,\"fetchMeta\":null,\"isInvalidated\":false,\"status\":\"success\",\"fetchStatus\":\"idle\"},\"queryKey\":[\"paper\",\"2209.03188\",\"comments\"],\"queryHash\":\"[\\\"paper\\\",\\\"2209.03188\\\",\\\"comments\\\"]\"},{\"state\":{\"data\":{\"data\":{\"paper_version\":{\"_id\":\"67dbd2c00a8b4fda22dd66b3\",\"paper_group_id\":\"67dbd2be0a8b4fda22dd66ad\",\"version_label\":\"v1\",\"version_order\":1,\"title\":\"Enhancement of theColor Image Compression Using a New Algorithm based on Discrete Hermite Wavelet Transform\",\"abstract\":\"$65\",\"author_ids\":[\"67dbd2be0a8b4fda22dd66ae\",\"67dbd2bf0a8b4fda22dd66af\",\"67dbd2bf0a8b4fda22dd66b0\",\"67dbd2c00a8b4fda22dd66b1\",\"67dbd2c00a8b4fda22dd66b2\"],\"publication_date\":\"2023-03-23T11:11:41.000Z\",\"license\":\"http://creativecommons.org/licenses/by/4.0/\",\"created_at\":\"2025-03-20T08:33:04.830Z\",\"updated_at\":\"2025-03-20T08:33:04.830Z\",\"is_deleted\":false,\"is_hidden\":false,\"universal_paper_id\":\"2303.13175\",\"imageURL\":\"image/2303.13175v1.png\"},\"paper_group\":{\"_id\":\"67dbd2be0a8b4fda22dd66ad\",\"universal_paper_id\":\"2303.13175\",\"title\":\"Enhancement of theColor Image Compression Using a New Algorithm based on Discrete Hermite Wavelet Transform\",\"created_at\":\"2025-03-20T08:33:02.286Z\",\"updated_at\":\"2025-03-20T08:33:02.286Z\",\"categories\":[\"Computer Science\",\"Electrical Engineering and Systems Science\"],\"subcategories\":[\"cs.CV\",\"eess.IV\"],\"custom_categories\":[\"image-segmentation\",\"image-generation\",\"transformers\",\"representation-learning\",\"efficient-transformers\"],\"author_user_ids\":[],\"source\":{\"name\":\"alphaXiv\",\"url\":\"https://arxiv.org/abs/2303.13175\"},\"metrics\":{\"activity_rank\":0,\"questions_count\":0,\"responses_count\":0,\"upvotes_count\":0,\"downvotes_count\":0,\"total_votes\":0,\"public_total_votes\":4,\"visits_count\":{\"last24Hours\":0,\"last7Days\":0,\"last30Days\":2,\"last90Days\":2,\"all\":2},\"timeline\":[{\"date\":\"2025-03-31T02:52:22.984Z\",\"views\":2},{\"date\":\"2025-03-27T14:52:22.984Z\",\"views\":0},{\"date\":\"2025-03-24T02:52:22.984Z\",\"views\":1},{\"date\":\"2025-03-20T14:52:22.984Z\",\"views\":1},{\"date\":\"2025-03-17T02:52:22.984Z\",\"views\":7},{\"date\":\"2025-03-13T14:52:23.007Z\",\"views\":0},{\"date\":\"2025-03-10T02:52:23.030Z\",\"views\":0},{\"date\":\"2025-03-06T14:52:23.053Z\",\"views\":0},{\"date\":\"2025-03-03T02:52:23.076Z\",\"views\":1},{\"date\":\"2025-02-27T14:52:23.099Z\",\"views\":1},{\"date\":\"2025-02-24T02:52:23.122Z\",\"views\":2},{\"date\":\"2025-02-20T14:52:23.144Z\",\"views\":1},{\"date\":\"2025-02-17T02:52:23.168Z\",\"views\":1},{\"date\":\"2025-02-13T14:52:23.192Z\",\"views\":0},{\"date\":\"2025-02-10T02:52:23.214Z\",\"views\":0},{\"date\":\"2025-02-06T14:52:23.237Z\",\"views\":1},{\"date\":\"2025-02-03T02:52:23.260Z\",\"views\":2},{\"date\":\"2025-01-30T14:52:23.282Z\",\"views\":0},{\"date\":\"2025-01-27T02:52:23.305Z\",\"views\":0},{\"date\":\"2025-01-23T14:52:23.328Z\",\"views\":2},{\"date\":\"2025-01-20T02:52:23.351Z\",\"views\":0},{\"date\":\"2025-01-16T14:52:23.373Z\",\"views\":0},{\"date\":\"2025-01-13T02:52:23.396Z\",\"views\":0},{\"date\":\"2025-01-09T14:52:23.419Z\",\"views\":0},{\"date\":\"2025-01-06T02:52:23.446Z\",\"views\":2},{\"date\":\"2025-01-02T14:52:23.470Z\",\"views\":1},{\"date\":\"2024-12-30T02:52:23.493Z\",\"views\":2},{\"date\":\"2024-12-26T14:52:23.515Z\",\"views\":0},{\"date\":\"2024-12-23T02:52:23.539Z\",\"views\":2},{\"date\":\"2024-12-19T14:52:23.561Z\",\"views\":2},{\"date\":\"2024-12-16T02:52:23.584Z\",\"views\":0},{\"date\":\"2024-12-12T14:52:23.607Z\",\"views\":0},{\"date\":\"2024-12-09T02:52:23.630Z\",\"views\":2},{\"date\":\"2024-12-05T14:52:23.653Z\",\"views\":0},{\"date\":\"2024-12-02T02:52:23.676Z\",\"views\":2},{\"date\":\"2024-11-28T14:52:23.698Z\",\"views\":0},{\"date\":\"2024-11-25T02:52:23.721Z\",\"views\":1},{\"date\":\"2024-11-21T14:52:23.744Z\",\"views\":0},{\"date\":\"2024-11-18T02:52:23.767Z\",\"views\":0},{\"date\":\"2024-11-14T14:52:23.790Z\",\"views\":0},{\"date\":\"2024-11-11T02:52:23.813Z\",\"views\":1},{\"date\":\"2024-11-07T14:52:23.836Z\",\"views\":1},{\"date\":\"2024-11-04T02:52:23.858Z\",\"views\":2},{\"date\":\"2024-10-31T14:52:23.881Z\",\"views\":2},{\"date\":\"2024-10-28T02:52:23.903Z\",\"views\":1},{\"date\":\"2024-10-24T14:52:23.926Z\",\"views\":1},{\"date\":\"2024-10-21T02:52:23.949Z\",\"views\":0},{\"date\":\"2024-10-17T14:52:23.974Z\",\"views\":0},{\"date\":\"2024-10-14T02:52:23.996Z\",\"views\":1},{\"date\":\"2024-10-10T14:52:24.019Z\",\"views\":2},{\"date\":\"2024-10-07T02:52:24.041Z\",\"views\":2},{\"date\":\"2024-10-03T14:52:24.063Z\",\"views\":1},{\"date\":\"2024-09-30T02:52:24.086Z\",\"views\":2},{\"date\":\"2024-09-26T14:52:24.108Z\",\"views\":1},{\"date\":\"2024-09-23T02:52:24.131Z\",\"views\":0},{\"date\":\"2024-09-19T14:52:24.155Z\",\"views\":1}],\"weighted_visits\":{\"last24Hours\":0,\"last7Days\":0,\"last30Days\":0.0001006202962801813,\"last90Days\":0.07383266212383302,\"hot\":0}},\"is_hidden\":false,\"first_publication_date\":\"2023-03-23T11:11:41.000Z\",\"organizations\":[\"67dbd2c3affd2123a36f4a44\",\"67be6417aa92218ccd8b2ed8\",\"67d125796b64b3dda16d5873\"],\"paperVersions\":{\"_id\":\"67dbd2c00a8b4fda22dd66b3\",\"paper_group_id\":\"67dbd2be0a8b4fda22dd66ad\",\"version_label\":\"v1\",\"version_order\":1,\"title\":\"Enhancement of theColor Image Compression Using a New Algorithm based on Discrete Hermite Wavelet Transform\",\"abstract\":\"$66\",\"author_ids\":[\"67dbd2be0a8b4fda22dd66ae\",\"67dbd2bf0a8b4fda22dd66af\",\"67dbd2bf0a8b4fda22dd66b0\",\"67dbd2c00a8b4fda22dd66b1\",\"67dbd2c00a8b4fda22dd66b2\"],\"publication_date\":\"2023-03-23T11:11:41.000Z\",\"license\":\"http://creativecommons.org/licenses/by/4.0/\",\"created_at\":\"2025-03-20T08:33:04.830Z\",\"updated_at\":\"2025-03-20T08:33:04.830Z\",\"is_deleted\":false,\"is_hidden\":false,\"universal_paper_id\":\"2303.13175\",\"imageURL\":\"image/2303.13175v1.png\"},\"verifiedAuthors\":[],\"authors\":[{\"_id\":\"67dbd2be0a8b4fda22dd66ae\",\"full_name\":\"Hassan Mohamed Muhi-Aldeen\",\"affiliation\":null,\"orcid\":null,\"semantic_scholarid\":null,\"user_id\":null},{\"_id\":\"67dbd2bf0a8b4fda22dd66af\",\"full_name\":\"Asma A. Abdulrahman\",\"affiliation\":null,\"orcid\":null,\"semantic_scholarid\":null,\"user_id\":null},{\"_id\":\"67dbd2bf0a8b4fda22dd66b0\",\"full_name\":\"Jabbar Abed Eleiwy\",\"affiliation\":null,\"orcid\":null,\"semantic_scholarid\":null,\"user_id\":null},{\"_id\":\"67dbd2c00a8b4fda22dd66b1\",\"full_name\":\"Fouad S. Tahir\",\"affiliation\":null,\"orcid\":null,\"semantic_scholarid\":null,\"user_id\":null},{\"_id\":\"67dbd2c00a8b4fda22dd66b2\",\"full_name\":\"Yurii Khlaponin\",\"affiliation\":null,\"orcid\":null,\"semantic_scholarid\":null,\"user_id\":null}]},\"max_version_order\":1,\"verified_authors\":[],\"authors\":[{\"_id\":\"67dbd2be0a8b4fda22dd66ae\",\"full_name\":\"Hassan Mohamed Muhi-Aldeen\",\"affiliation\":null,\"orcid\":null,\"semantic_scholarid\":null,\"user_id\":null},{\"_id\":\"67dbd2bf0a8b4fda22dd66af\",\"full_name\":\"Asma A. Abdulrahman\",\"affiliation\":null,\"orcid\":null,\"semantic_scholarid\":null,\"user_id\":null},{\"_id\":\"67dbd2bf0a8b4fda22dd66b0\",\"full_name\":\"Jabbar Abed Eleiwy\",\"affiliation\":null,\"orcid\":null,\"semantic_scholarid\":null,\"user_id\":null},{\"_id\":\"67dbd2c00a8b4fda22dd66b1\",\"full_name\":\"Fouad S. Tahir\",\"affiliation\":null,\"orcid\":null,\"semantic_scholarid\":null,\"user_id\":null},{\"_id\":\"67dbd2c00a8b4fda22dd66b2\",\"full_name\":\"Yurii Khlaponin\",\"affiliation\":null,\"orcid\":null,\"semantic_scholarid\":null,\"user_id\":null}],\"pdf_info\":{\"fetcher_url\":\"https://fetcher.alphaxiv.org/v2/pdf/2303.13175v1\"}}},\"dataUpdateCount\":1,\"dataUpdatedAt\":1744228214360,\"error\":null,\"errorUpdateCount\":0,\"errorUpdatedAt\":0,\"fetchFailureCount\":0,\"fetchFailureReason\":null,\"fetchMeta\":null,\"isInvalidated\":false,\"status\":\"success\",\"fetchStatus\":\"idle\"},\"queryKey\":[\"paper\",\"2303.13175\",\"metadata\"],\"queryHash\":\"[\\\"paper\\\",\\\"2303.13175\\\",\\\"metadata\\\"]\"},{\"state\":{\"data\":{\"data\":[]},\"dataUpdateCount\":1,\"dataUpdatedAt\":1744228214360,\"error\":null,\"errorUpdateCount\":0,\"errorUpdatedAt\":0,\"fetchFailureCount\":0,\"fetchFailureReason\":null,\"fetchMeta\":null,\"isInvalidated\":false,\"status\":\"success\",\"fetchStatus\":\"idle\"},\"queryKey\":[\"paper\",\"2303.13175\",\"comments\"],\"queryHash\":\"[\\\"paper\\\",\\\"2303.13175\\\",\\\"comments\\\"]\"},{\"state\":{\"data\":{\"data\":{\"paper_version\":{\"_id\":\"6771011890f035bff487a63c\",\"paper_group_id\":\"6771011890f035bff487a63b\",\"version_label\":\"v2\",\"version_order\":2,\"title\":\"Gluing AdS/CFT\",\"abstract\":\"In this paper, we investigate gluing together two Anti-de Sitter (AdS) geometries along a timelike brane, which corresponds to coupling two brane field theories (BFTs) through gravitational interactions in the dual holographic perspective. By exploring the general conditions for this gluing process, we show that the energy stress tensors of the BFTs backreact on the dynamical metric in a manner reminiscent of the TTbar deformation. In particular, we present explicit solutions for the three-dimensional case with chiral excitations and further construct perturbative solutions with non-chiral excitations.\",\"author_ids\":[\"672bd10f986a1370676e0ed8\",\"672bd10f986a1370676e0edf\",\"672bd051986a1370676dff68\"],\"publication_date\":\"2023-03-23T10:03:58.000Z\",\"license\":\"http://creativecommons.org/licenses/by/4.0/\",\"created_at\":\"2024-12-29T07:58:16.479Z\",\"updated_at\":\"2024-12-29T07:58:16.479Z\",\"is_deleted\":false,\"is_hidden\":false,\"universal_paper_id\":\"2303.01247\",\"imageURL\":\"image/2303.01247v2.png\"},\"paper_group\":{\"_id\":\"6771011890f035bff487a63b\",\"universal_paper_id\":\"2303.01247\",\"title\":\"Gluing AdS/CFT\",\"created_at\":\"2024-12-29T07:58:16.259Z\",\"updated_at\":\"2025-03-03T20:18:39.664Z\",\"categories\":[\"Physics\"],\"subcategories\":[\"hep-th\",\"gr-qc\"],\"custom_categories\":null,\"author_user_ids\":[],\"source\":{\"name\":\"alphaXiv\",\"url\":\"https://arxiv.org/paper/2303.01247\"},\"metrics\":{\"activity_rank\":0,\"questions_count\":0,\"responses_count\":0,\"upvotes_count\":0,\"downvotes_count\":0,\"total_votes\":0,\"visits_count\":{\"last24Hours\":0,\"last7Days\":0,\"last30Days\":1,\"last90Days\":4,\"all\":21},\"weighted_visits\":{\"last24Hours\":0,\"last7Days\":0,\"last30Days\":0.000050278609265818334,\"last90Days\":0.14763446121247958,\"hot\":0},\"public_total_votes\":0,\"timeline\":[{\"date\":\"2025-04-03T01:57:44.452Z\",\"views\":0},{\"date\":\"2025-03-30T13:57:44.452Z\",\"views\":1},{\"date\":\"2025-03-27T01:57:44.452Z\",\"views\":0},{\"date\":\"2025-03-23T13:57:44.452Z\",\"views\":2},{\"date\":\"2025-03-20T01:57:44.452Z\",\"views\":2},{\"date\":\"2025-03-16T13:57:44.452Z\",\"views\":5},{\"date\":\"2025-03-13T01:57:44.452Z\",\"views\":0},{\"date\":\"2025-03-09T13:57:44.452Z\",\"views\":2},{\"date\":\"2025-03-06T01:57:44.452Z\",\"views\":1},{\"date\":\"2025-03-02T13:57:44.452Z\",\"views\":1},{\"date\":\"2025-02-27T01:57:44.452Z\",\"views\":1},{\"date\":\"2025-02-23T13:57:44.452Z\",\"views\":2},{\"date\":\"2025-02-20T01:57:44.477Z\",\"views\":0},{\"date\":\"2025-02-16T13:57:44.490Z\",\"views\":2},{\"date\":\"2025-02-13T01:57:44.503Z\",\"views\":2},{\"date\":\"2025-02-09T13:57:44.515Z\",\"views\":2},{\"date\":\"2025-02-06T01:57:44.530Z\",\"views\":0},{\"date\":\"2025-02-02T13:57:44.550Z\",\"views\":0},{\"date\":\"2025-01-30T01:57:44.572Z\",\"views\":1},{\"date\":\"2025-01-26T13:57:44.595Z\",\"views\":1},{\"date\":\"2025-01-23T01:57:44.617Z\",\"views\":3},{\"date\":\"2025-01-19T13:57:44.639Z\",\"views\":6},{\"date\":\"2025-01-16T01:57:44.667Z\",\"views\":1},{\"date\":\"2025-01-12T13:57:44.689Z\",\"views\":0},{\"date\":\"2025-01-09T01:57:44.716Z\",\"views\":1},{\"date\":\"2025-01-05T13:57:44.740Z\",\"views\":7},{\"date\":\"2025-01-02T01:57:44.763Z\",\"views\":0},{\"date\":\"2024-12-29T13:57:44.785Z\",\"views\":0},{\"date\":\"2024-12-26T01:57:44.822Z\",\"views\":4},{\"date\":\"2024-12-22T13:57:44.845Z\",\"views\":0},{\"date\":\"2024-12-19T01:57:44.871Z\",\"views\":1},{\"date\":\"2024-12-15T13:57:44.896Z\",\"views\":1},{\"date\":\"2024-12-12T01:57:44.921Z\",\"views\":1},{\"date\":\"2024-12-08T13:57:44.946Z\",\"views\":1},{\"date\":\"2024-12-05T01:57:44.969Z\",\"views\":1},{\"date\":\"2024-12-01T13:57:44.993Z\",\"views\":2},{\"date\":\"2024-11-28T01:57:45.014Z\",\"views\":2},{\"date\":\"2024-11-24T13:57:45.033Z\",\"views\":0},{\"date\":\"2024-11-21T01:57:45.058Z\",\"views\":2},{\"date\":\"2024-11-17T13:57:45.080Z\",\"views\":2},{\"date\":\"2024-11-14T01:57:45.101Z\",\"views\":1},{\"date\":\"2024-11-10T13:57:45.129Z\",\"views\":1},{\"date\":\"2024-11-07T01:57:45.154Z\",\"views\":2},{\"date\":\"2024-11-03T13:57:45.179Z\",\"views\":1},{\"date\":\"2024-10-31T00:57:45.202Z\",\"views\":1},{\"date\":\"2024-10-27T12:57:45.224Z\",\"views\":2},{\"date\":\"2024-10-24T00:57:45.254Z\",\"views\":2},{\"date\":\"2024-10-20T12:57:45.282Z\",\"views\":2},{\"date\":\"2024-10-17T00:57:45.302Z\",\"views\":1},{\"date\":\"2024-10-13T12:57:45.322Z\",\"views\":2},{\"date\":\"2024-10-10T00:57:45.346Z\",\"views\":0},{\"date\":\"2024-10-06T12:57:45.367Z\",\"views\":1},{\"date\":\"2024-10-03T00:57:45.388Z\",\"views\":0},{\"date\":\"2024-09-29T12:57:45.409Z\",\"views\":2},{\"date\":\"2024-09-26T00:57:45.429Z\",\"views\":1},{\"date\":\"2024-09-22T12:57:45.463Z\",\"views\":2},{\"date\":\"2024-09-19T00:57:45.486Z\",\"views\":1},{\"date\":\"2024-09-15T12:57:45.509Z\",\"views\":0},{\"date\":\"2024-09-12T00:57:45.530Z\",\"views\":1},{\"date\":\"2024-09-08T12:57:45.554Z\",\"views\":1},{\"date\":\"2024-09-05T00:57:45.574Z\",\"views\":1},{\"date\":\"2024-09-01T12:57:45.598Z\",\"views\":1},{\"date\":\"2024-08-29T00:57:45.617Z\",\"views\":0}]},\"is_hidden\":false,\"first_publication_date\":\"2023-03-23T10:03:58.000Z\",\"paperVersions\":{\"_id\":\"6771011890f035bff487a63c\",\"paper_group_id\":\"6771011890f035bff487a63b\",\"version_label\":\"v2\",\"version_order\":2,\"title\":\"Gluing AdS/CFT\",\"abstract\":\"In this paper, we investigate gluing together two Anti-de Sitter (AdS) geometries along a timelike brane, which corresponds to coupling two brane field theories (BFTs) through gravitational interactions in the dual holographic perspective. By exploring the general conditions for this gluing process, we show that the energy stress tensors of the BFTs backreact on the dynamical metric in a manner reminiscent of the TTbar deformation. In particular, we present explicit solutions for the three-dimensional case with chiral excitations and further construct perturbative solutions with non-chiral excitations.\",\"author_ids\":[\"672bd10f986a1370676e0ed8\",\"672bd10f986a1370676e0edf\",\"672bd051986a1370676dff68\"],\"publication_date\":\"2023-03-23T10:03:58.000Z\",\"license\":\"http://creativecommons.org/licenses/by/4.0/\",\"created_at\":\"2024-12-29T07:58:16.479Z\",\"updated_at\":\"2024-12-29T07:58:16.479Z\",\"is_deleted\":false,\"is_hidden\":false,\"universal_paper_id\":\"2303.01247\",\"imageURL\":\"image/2303.01247v2.png\"},\"verifiedAuthors\":[],\"authors\":[{\"_id\":\"672bd051986a1370676dff68\",\"full_name\":\"Tadashi Takayanagi\",\"affiliation\":null,\"orcid\":null,\"semantic_scholarid\":null,\"user_id\":null},{\"_id\":\"672bd10f986a1370676e0ed8\",\"full_name\":\"Taishi Kawamoto\",\"affiliation\":null,\"orcid\":null,\"semantic_scholarid\":null,\"user_id\":null},{\"_id\":\"672bd10f986a1370676e0edf\",\"full_name\":\"Shan-Ming Ruan\",\"affiliation\":null,\"orcid\":null,\"semantic_scholarid\":null,\"user_id\":null}]},\"max_version_order\":2,\"verified_authors\":[],\"authors\":[{\"_id\":\"672bd051986a1370676dff68\",\"full_name\":\"Tadashi Takayanagi\",\"affiliation\":null,\"orcid\":null,\"semantic_scholarid\":null,\"user_id\":null},{\"_id\":\"672bd10f986a1370676e0ed8\",\"full_name\":\"Taishi Kawamoto\",\"affiliation\":null,\"orcid\":null,\"semantic_scholarid\":null,\"user_id\":null},{\"_id\":\"672bd10f986a1370676e0edf\",\"full_name\":\"Shan-Ming Ruan\",\"affiliation\":null,\"orcid\":null,\"semantic_scholarid\":null,\"user_id\":null}],\"pdf_info\":{\"fetcher_url\":\"https://fetcher.alphaxiv.org/v2/pdf/2303.01247v2\"}}},\"dataUpdateCount\":1,\"dataUpdatedAt\":1744228229065,\"error\":null,\"errorUpdateCount\":0,\"errorUpdatedAt\":0,\"fetchFailureCount\":0,\"fetchFailureReason\":null,\"fetchMeta\":null,\"isInvalidated\":false,\"status\":\"success\",\"fetchStatus\":\"idle\"},\"queryKey\":[\"paper\",\"2303.01247\",\"metadata\"],\"queryHash\":\"[\\\"paper\\\",\\\"2303.01247\\\",\\\"metadata\\\"]\"},{\"state\":{\"data\":{\"data\":[]},\"dataUpdateCount\":1,\"dataUpdatedAt\":1744228229065,\"error\":null,\"errorUpdateCount\":0,\"errorUpdatedAt\":0,\"fetchFailureCount\":0,\"fetchFailureReason\":null,\"fetchMeta\":null,\"isInvalidated\":false,\"status\":\"success\",\"fetchStatus\":\"idle\"},\"queryKey\":[\"paper\",\"2303.01247\",\"comments\"],\"queryHash\":\"[\\\"paper\\\",\\\"2303.01247\\\",\\\"comments\\\"]\"},{\"state\":{\"data\":{\"data\":{\"paper_version\":{\"_id\":\"673cccd37d2b7ed9dd51d777\",\"paper_group_id\":\"673cccd27d2b7ed9dd51d772\",\"version_label\":\"v1\",\"version_order\":1,\"title\":\"MMFormer: Multimodal Transformer Using Multiscale Self-Attention for Remote Sensing Image Classification\",\"abstract\":\"$67\",\"author_ids\":[\"672bcaa2986a1370676d986d\",\"673cccd27d2b7ed9dd51d773\",\"67322794cd1e32a6e7f0291a\",\"673cccd37d2b7ed9dd51d775\",\"672bcacf986a1370676d9b20\",\"673cccd37d2b7ed9dd51d776\"],\"publication_date\":\"2023-03-23T08:34:24.000Z\",\"license\":\"http://creativecommons.org/licenses/by-nc-sa/4.0/\",\"created_at\":\"2024-11-19T17:37:23.654Z\",\"updated_at\":\"2024-11-19T17:37:23.654Z\",\"is_deleted\":false,\"is_hidden\":false,\"universal_paper_id\":\"2303.13101\",\"imageURL\":\"image/2303.13101v1.png\"},\"paper_group\":{\"_id\":\"673cccd27d2b7ed9dd51d772\",\"universal_paper_id\":\"2303.13101\",\"source\":{\"name\":\"arXiv\",\"url\":\"https://arXiv.org/paper/2303.13101\"},\"title\":\"MMFormer: Multimodal Transformer Using Multiscale Self-Attention for Remote Sensing Image Classification\",\"created_at\":\"2024-10-21T21:17:16.164Z\",\"updated_at\":\"2025-03-03T20:18:39.677Z\",\"categories\":[\"Computer Science\"],\"subcategories\":[\"cs.CV\"],\"metrics\":{\"activity_rank\":0,\"questions_count\":0,\"responses_count\":0,\"upvotes_count\":null,\"downvotes_count\":null,\"total_votes\":0,\"visits_count\":{\"last24Hours\":0,\"last7Days\":2,\"last30Days\":4,\"last90Days\":5,\"all\":22},\"weighted_visits\":{\"last24Hours\":0,\"last7Days\":7.530832207201265e-19,\"last30Days\":0.00020094769039892638,\"last90Days\":0.18449206003748153,\"hot\":7.530832207201265e-19},\"public_total_votes\":0,\"timeline\":[{\"date\":\"2025-04-03T01:57:44.521Z\",\"views\":6},{\"date\":\"2025-03-30T13:57:44.521Z\",\"views\":2},{\"date\":\"2025-03-27T01:57:44.521Z\",\"views\":1},{\"date\":\"2025-03-23T13:57:44.521Z\",\"views\":2},{\"date\":\"2025-03-20T01:57:44.521Z\",\"views\":2},{\"date\":\"2025-03-16T13:57:44.521Z\",\"views\":0},{\"date\":\"2025-03-13T01:57:44.521Z\",\"views\":8},{\"date\":\"2025-03-09T13:57:44.521Z\",\"views\":1},{\"date\":\"2025-03-06T01:57:44.521Z\",\"views\":0},{\"date\":\"2025-03-02T13:57:44.521Z\",\"views\":2},{\"date\":\"2025-02-27T01:57:44.521Z\",\"views\":2},{\"date\":\"2025-02-23T13:57:44.521Z\",\"views\":2},{\"date\":\"2025-02-20T01:57:44.541Z\",\"views\":2},{\"date\":\"2025-02-16T13:57:44.563Z\",\"views\":0},{\"date\":\"2025-02-13T01:57:44.584Z\",\"views\":1},{\"date\":\"2025-02-09T13:57:44.605Z\",\"views\":0},{\"date\":\"2025-02-06T01:57:44.626Z\",\"views\":1},{\"date\":\"2025-02-02T13:57:44.647Z\",\"views\":2},{\"date\":\"2025-01-30T01:57:44.678Z\",\"views\":2},{\"date\":\"2025-01-26T13:57:44.699Z\",\"views\":1},{\"date\":\"2025-01-23T01:57:44.717Z\",\"views\":1},{\"date\":\"2025-01-19T13:57:44.740Z\",\"views\":0},{\"date\":\"2025-01-16T01:57:44.762Z\",\"views\":3},{\"date\":\"2025-01-12T13:57:44.785Z\",\"views\":0},{\"date\":\"2025-01-09T01:57:44.820Z\",\"views\":2},{\"date\":\"2025-01-05T13:57:44.844Z\",\"views\":2},{\"date\":\"2025-01-02T01:57:44.868Z\",\"views\":0},{\"date\":\"2024-12-29T13:57:44.888Z\",\"views\":0},{\"date\":\"2024-12-26T01:57:44.910Z\",\"views\":0},{\"date\":\"2024-12-22T13:57:44.935Z\",\"views\":1},{\"date\":\"2024-12-19T01:57:44.958Z\",\"views\":2},{\"date\":\"2024-12-15T13:57:44.981Z\",\"views\":2},{\"date\":\"2024-12-12T01:57:45.003Z\",\"views\":2},{\"date\":\"2024-12-08T13:57:45.023Z\",\"views\":1},{\"date\":\"2024-12-05T01:57:45.044Z\",\"views\":0},{\"date\":\"2024-12-01T13:57:45.069Z\",\"views\":0},{\"date\":\"2024-11-28T01:57:45.093Z\",\"views\":0},{\"date\":\"2024-11-24T13:57:45.113Z\",\"views\":1},{\"date\":\"2024-11-21T01:57:45.138Z\",\"views\":2},{\"date\":\"2024-11-17T13:57:45.159Z\",\"views\":0},{\"date\":\"2024-11-14T01:57:45.181Z\",\"views\":2},{\"date\":\"2024-11-10T13:57:45.205Z\",\"views\":1},{\"date\":\"2024-11-07T01:57:45.227Z\",\"views\":0},{\"date\":\"2024-11-03T13:57:45.265Z\",\"views\":1},{\"date\":\"2024-10-31T00:57:45.284Z\",\"views\":2},{\"date\":\"2024-10-27T12:57:45.308Z\",\"views\":1},{\"date\":\"2024-10-24T00:57:45.331Z\",\"views\":0},{\"date\":\"2024-10-20T12:57:45.351Z\",\"views\":5},{\"date\":\"2024-10-17T00:57:45.372Z\",\"views\":4},{\"date\":\"2024-10-13T12:57:45.393Z\",\"views\":2},{\"date\":\"2024-10-10T00:57:45.417Z\",\"views\":2},{\"date\":\"2024-10-06T12:57:45.452Z\",\"views\":0},{\"date\":\"2024-10-03T00:57:45.472Z\",\"views\":1},{\"date\":\"2024-09-29T12:57:45.494Z\",\"views\":1},{\"date\":\"2024-09-26T00:57:45.516Z\",\"views\":2},{\"date\":\"2024-09-22T12:57:45.539Z\",\"views\":1},{\"date\":\"2024-09-19T00:57:45.562Z\",\"views\":1},{\"date\":\"2024-09-15T12:57:45.583Z\",\"views\":0},{\"date\":\"2024-09-12T00:57:45.607Z\",\"views\":0},{\"date\":\"2024-09-08T12:57:45.626Z\",\"views\":2},{\"date\":\"2024-09-05T00:57:45.712Z\",\"views\":0},{\"date\":\"2024-09-01T12:57:45.732Z\",\"views\":2},{\"date\":\"2024-08-29T00:57:45.755Z\",\"views\":1}]},\"ranking\":{\"current_rank\":91769,\"previous_rank\":91428,\"activity_score\":0,\"paper_score\":0},\"is_hidden\":false,\"custom_categories\":[\"multi-modal-learning\",\"attention-mechanisms\",\"transformers\",\"image-classification\",\"representation-learning\"],\"first_publication_date\":\"2023-03-23T08:34:24.000Z\",\"author_user_ids\":[],\"organizations\":[\"67be6377aa92218ccd8b0ffe\",\"67be6388aa92218ccd8b156f\",\"67be6377aa92218ccd8b0ffd\"],\"paperVersions\":{\"_id\":\"673cccd37d2b7ed9dd51d777\",\"paper_group_id\":\"673cccd27d2b7ed9dd51d772\",\"version_label\":\"v1\",\"version_order\":1,\"title\":\"MMFormer: Multimodal Transformer Using Multiscale Self-Attention for Remote Sensing Image Classification\",\"abstract\":\"$68\",\"author_ids\":[\"672bcaa2986a1370676d986d\",\"673cccd27d2b7ed9dd51d773\",\"67322794cd1e32a6e7f0291a\",\"673cccd37d2b7ed9dd51d775\",\"672bcacf986a1370676d9b20\",\"673cccd37d2b7ed9dd51d776\"],\"publication_date\":\"2023-03-23T08:34:24.000Z\",\"license\":\"http://creativecommons.org/licenses/by-nc-sa/4.0/\",\"created_at\":\"2024-11-19T17:37:23.654Z\",\"updated_at\":\"2024-11-19T17:37:23.654Z\",\"is_deleted\":false,\"is_hidden\":false,\"universal_paper_id\":\"2303.13101\",\"imageURL\":\"image/2303.13101v1.png\"},\"verifiedAuthors\":[],\"authors\":[{\"_id\":\"672bcaa2986a1370676d986d\",\"full_name\":\"Bo Zhang\",\"affiliation\":null,\"orcid\":null,\"semantic_scholarid\":null,\"user_id\":null},{\"_id\":\"672bcacf986a1370676d9b20\",\"full_name\":\"Liang He\",\"affiliation\":null,\"orcid\":null,\"semantic_scholarid\":null,\"user_id\":null},{\"_id\":\"67322794cd1e32a6e7f0291a\",\"full_name\":\"Wei Feng\",\"affiliation\":null,\"orcid\":null,\"semantic_scholarid\":null,\"user_id\":null},{\"_id\":\"673cccd27d2b7ed9dd51d773\",\"full_name\":\"Zuheng Ming\",\"affiliation\":null,\"orcid\":null,\"semantic_scholarid\":null,\"user_id\":null},{\"_id\":\"673cccd37d2b7ed9dd51d775\",\"full_name\":\"Yaqian Liu\",\"affiliation\":null,\"orcid\":null,\"semantic_scholarid\":null,\"user_id\":null},{\"_id\":\"673cccd37d2b7ed9dd51d776\",\"full_name\":\"Kaixing Zhao\",\"affiliation\":null,\"orcid\":null,\"semantic_scholarid\":null,\"user_id\":null}]},\"max_version_order\":1,\"verified_authors\":[],\"authors\":[{\"_id\":\"672bcaa2986a1370676d986d\",\"full_name\":\"Bo Zhang\",\"affiliation\":null,\"orcid\":null,\"semantic_scholarid\":null,\"user_id\":null},{\"_id\":\"672bcacf986a1370676d9b20\",\"full_name\":\"Liang He\",\"affiliation\":null,\"orcid\":null,\"semantic_scholarid\":null,\"user_id\":null},{\"_id\":\"67322794cd1e32a6e7f0291a\",\"full_name\":\"Wei Feng\",\"affiliation\":null,\"orcid\":null,\"semantic_scholarid\":null,\"user_id\":null},{\"_id\":\"673cccd27d2b7ed9dd51d773\",\"full_name\":\"Zuheng Ming\",\"affiliation\":null,\"orcid\":null,\"semantic_scholarid\":null,\"user_id\":null},{\"_id\":\"673cccd37d2b7ed9dd51d775\",\"full_name\":\"Yaqian Liu\",\"affiliation\":null,\"orcid\":null,\"semantic_scholarid\":null,\"user_id\":null},{\"_id\":\"673cccd37d2b7ed9dd51d776\",\"full_name\":\"Kaixing Zhao\",\"affiliation\":null,\"orcid\":null,\"semantic_scholarid\":null,\"user_id\":null}],\"pdf_info\":{\"fetcher_url\":\"https://fetcher.alphaxiv.org/v2/pdf/2303.13101v1\"}}},\"dataUpdateCount\":1,\"dataUpdatedAt\":1744228229385,\"error\":null,\"errorUpdateCount\":0,\"errorUpdatedAt\":0,\"fetchFailureCount\":0,\"fetchFailureReason\":null,\"fetchMeta\":null,\"isInvalidated\":false,\"status\":\"success\",\"fetchStatus\":\"idle\"},\"queryKey\":[\"paper\",\"2303.13101\",\"metadata\"],\"queryHash\":\"[\\\"paper\\\",\\\"2303.13101\\\",\\\"metadata\\\"]\"},{\"state\":{\"data\":{\"data\":[]},\"dataUpdateCount\":1,\"dataUpdatedAt\":1744228229385,\"error\":null,\"errorUpdateCount\":0,\"errorUpdatedAt\":0,\"fetchFailureCount\":0,\"fetchFailureReason\":null,\"fetchMeta\":null,\"isInvalidated\":false,\"status\":\"success\",\"fetchStatus\":\"idle\"},\"queryKey\":[\"paper\",\"2303.13101\",\"comments\"],\"queryHash\":\"[\\\"paper\\\",\\\"2303.13101\\\",\\\"comments\\\"]\"},{\"state\":{\"data\":{\"data\":{\"paper_version\":{\"_id\":\"673cf4f3615941b897fb6537\",\"paper_group_id\":\"673cf4f2615941b897fb6531\",\"version_label\":\"v1\",\"version_order\":1,\"title\":\"RLOR: A Flexible Framework of Deep Reinforcement Learning for Operation Research\",\"abstract\":\"$69\",\"author_ids\":[\"673cf4f3615941b897fb6533\",\"673cf4f3615941b897fb6535\",\"673cf4f3615941b897fb6536\"],\"publication_date\":\"2023-03-23T09:07:30.000Z\",\"license\":\"http://creativecommons.org/licenses/by/4.0/\",\"created_at\":\"2024-11-19T20:28:35.514Z\",\"updated_at\":\"2024-11-19T20:28:35.514Z\",\"is_deleted\":false,\"is_hidden\":false,\"universal_paper_id\":\"2303.13117\",\"imageURL\":\"image/2303.13117v1.png\"},\"paper_group\":{\"_id\":\"673cf4f2615941b897fb6531\",\"universal_paper_id\":\"2303.13117\",\"source\":{\"name\":\"arXiv\",\"url\":\"https://arXiv.org/paper/2303.13117\"},\"title\":\"RLOR: A Flexible Framework of Deep Reinforcement Learning for Operation Research\",\"created_at\":\"2024-10-27T05:42:42.059Z\",\"updated_at\":\"2025-03-03T20:18:39.677Z\",\"categories\":[\"Mathematics\",\"Computer Science\"],\"subcategories\":[\"math.OC\",\"cs.LG\",\"cs.NE\"],\"metrics\":{\"activity_rank\":0,\"questions_count\":0,\"responses_count\":0,\"upvotes_count\":null,\"downvotes_count\":null,\"total_votes\":0,\"visits_count\":{\"last24Hours\":0,\"last7Days\":1,\"last30Days\":2,\"last90Days\":5,\"all\":40},\"weighted_visits\":{\"last24Hours\":0,\"last7Days\":3.7703660551626335e-19,\"last30Days\":0.00010050464862710327,\"last90Days\":0.18451091206523315,\"hot\":3.7703660551626335e-19},\"public_total_votes\":0,\"timeline\":[{\"date\":\"2025-04-03T01:57:44.508Z\",\"views\":4},{\"date\":\"2025-03-30T13:57:44.508Z\",\"views\":0},{\"date\":\"2025-03-27T01:57:44.508Z\",\"views\":1},{\"date\":\"2025-03-23T13:57:44.508Z\",\"views\":0},{\"date\":\"2025-03-20T01:57:44.508Z\",\"views\":1},{\"date\":\"2025-03-16T13:57:44.508Z\",\"views\":1},{\"date\":\"2025-03-13T01:57:44.508Z\",\"views\":0},{\"date\":\"2025-03-09T13:57:44.508Z\",\"views\":5},{\"date\":\"2025-03-06T01:57:44.508Z\",\"views\":5},{\"date\":\"2025-03-02T13:57:44.508Z\",\"views\":5},{\"date\":\"2025-02-27T01:57:44.508Z\",\"views\":0},{\"date\":\"2025-02-23T13:57:44.508Z\",\"views\":0},{\"date\":\"2025-02-20T01:57:44.529Z\",\"views\":0},{\"date\":\"2025-02-16T13:57:44.545Z\",\"views\":2},{\"date\":\"2025-02-13T01:57:44.569Z\",\"views\":1},{\"date\":\"2025-02-09T13:57:44.589Z\",\"views\":1},{\"date\":\"2025-02-06T01:57:44.613Z\",\"views\":0},{\"date\":\"2025-02-02T13:57:44.634Z\",\"views\":2},{\"date\":\"2025-01-30T01:57:44.665Z\",\"views\":0},{\"date\":\"2025-01-26T13:57:44.690Z\",\"views\":0},{\"date\":\"2025-01-23T01:57:44.712Z\",\"views\":4},{\"date\":\"2025-01-19T13:57:44.735Z\",\"views\":1},{\"date\":\"2025-01-16T01:57:44.763Z\",\"views\":0},{\"date\":\"2025-01-12T13:57:44.785Z\",\"views\":1},{\"date\":\"2025-01-09T01:57:44.821Z\",\"views\":2},{\"date\":\"2025-01-05T13:57:44.844Z\",\"views\":0},{\"date\":\"2025-01-02T01:57:44.865Z\",\"views\":2},{\"date\":\"2024-12-29T13:57:44.888Z\",\"views\":2},{\"date\":\"2024-12-26T01:57:44.908Z\",\"views\":4},{\"date\":\"2024-12-22T13:57:44.933Z\",\"views\":1},{\"date\":\"2024-12-19T01:57:44.957Z\",\"views\":7},{\"date\":\"2024-12-15T13:57:44.980Z\",\"views\":0},{\"date\":\"2024-12-12T01:57:45.000Z\",\"views\":2},{\"date\":\"2024-12-08T13:57:45.020Z\",\"views\":5},{\"date\":\"2024-12-05T01:57:45.041Z\",\"views\":2},{\"date\":\"2024-12-01T13:57:45.061Z\",\"views\":8},{\"date\":\"2024-11-28T01:57:45.082Z\",\"views\":0},{\"date\":\"2024-11-24T13:57:45.102Z\",\"views\":1},{\"date\":\"2024-11-21T01:57:45.126Z\",\"views\":0},{\"date\":\"2024-11-17T13:57:45.152Z\",\"views\":0},{\"date\":\"2024-11-14T01:57:45.173Z\",\"views\":1},{\"date\":\"2024-11-10T13:57:45.191Z\",\"views\":2},{\"date\":\"2024-11-07T01:57:45.214Z\",\"views\":0},{\"date\":\"2024-11-03T13:57:45.243Z\",\"views\":1},{\"date\":\"2024-10-31T00:57:45.273Z\",\"views\":3},{\"date\":\"2024-10-27T12:57:45.301Z\",\"views\":1},{\"date\":\"2024-10-24T00:57:45.322Z\",\"views\":5},{\"date\":\"2024-10-20T12:57:45.342Z\",\"views\":0},{\"date\":\"2024-10-17T00:57:45.368Z\",\"views\":0},{\"date\":\"2024-10-13T12:57:45.388Z\",\"views\":0},{\"date\":\"2024-10-10T00:57:45.410Z\",\"views\":2},{\"date\":\"2024-10-06T12:57:45.432Z\",\"views\":2},{\"date\":\"2024-10-03T00:57:45.464Z\",\"views\":2},{\"date\":\"2024-09-29T12:57:45.484Z\",\"views\":0},{\"date\":\"2024-09-26T00:57:45.505Z\",\"views\":2},{\"date\":\"2024-09-22T12:57:45.527Z\",\"views\":0},{\"date\":\"2024-09-19T00:57:45.551Z\",\"views\":0},{\"date\":\"2024-09-15T12:57:45.571Z\",\"views\":1},{\"date\":\"2024-09-12T00:57:45.591Z\",\"views\":0},{\"date\":\"2024-09-08T12:57:45.614Z\",\"views\":2},{\"date\":\"2024-09-05T00:57:45.634Z\",\"views\":1},{\"date\":\"2024-09-01T12:57:45.716Z\",\"views\":1},{\"date\":\"2024-08-29T00:57:45.739Z\",\"views\":0}]},\"ranking\":{\"current_rank\":105850,\"previous_rank\":105485,\"activity_score\":0,\"paper_score\":0},\"is_hidden\":false,\"custom_categories\":[\"deep-reinforcement-learning\",\"optimization-methods\",\"attention-mechanisms\"],\"first_publication_date\":\"2023-03-23T09:07:30.000Z\",\"author_user_ids\":[],\"organizations\":[\"67c531672538b5438c35666e\"],\"paperVersions\":{\"_id\":\"673cf4f3615941b897fb6537\",\"paper_group_id\":\"673cf4f2615941b897fb6531\",\"version_label\":\"v1\",\"version_order\":1,\"title\":\"RLOR: A Flexible Framework of Deep Reinforcement Learning for Operation Research\",\"abstract\":\"$6a\",\"author_ids\":[\"673cf4f3615941b897fb6533\",\"673cf4f3615941b897fb6535\",\"673cf4f3615941b897fb6536\"],\"publication_date\":\"2023-03-23T09:07:30.000Z\",\"license\":\"http://creativecommons.org/licenses/by/4.0/\",\"created_at\":\"2024-11-19T20:28:35.514Z\",\"updated_at\":\"2024-11-19T20:28:35.514Z\",\"is_deleted\":false,\"is_hidden\":false,\"universal_paper_id\":\"2303.13117\",\"imageURL\":\"image/2303.13117v1.png\"},\"verifiedAuthors\":[],\"authors\":[{\"_id\":\"673cf4f3615941b897fb6533\",\"full_name\":\"Ching Pui Wan\",\"affiliation\":null,\"orcid\":null,\"semantic_scholarid\":null,\"user_id\":null},{\"_id\":\"673cf4f3615941b897fb6535\",\"full_name\":\"Tung Li\",\"affiliation\":null,\"orcid\":null,\"semantic_scholarid\":null,\"user_id\":null},{\"_id\":\"673cf4f3615941b897fb6536\",\"full_name\":\"Jason Min Wang\",\"affiliation\":null,\"orcid\":null,\"semantic_scholarid\":null,\"user_id\":null}]},\"max_version_order\":1,\"verified_authors\":[],\"authors\":[{\"_id\":\"673cf4f3615941b897fb6533\",\"full_name\":\"Ching Pui Wan\",\"affiliation\":null,\"orcid\":null,\"semantic_scholarid\":null,\"user_id\":null},{\"_id\":\"673cf4f3615941b897fb6535\",\"full_name\":\"Tung Li\",\"affiliation\":null,\"orcid\":null,\"semantic_scholarid\":null,\"user_id\":null},{\"_id\":\"673cf4f3615941b897fb6536\",\"full_name\":\"Jason Min Wang\",\"affiliation\":null,\"orcid\":null,\"semantic_scholarid\":null,\"user_id\":null}],\"pdf_info\":{\"fetcher_url\":\"https://fetcher.alphaxiv.org/v2/pdf/2303.13117v1\"}}},\"dataUpdateCount\":1,\"dataUpdatedAt\":1744228229751,\"error\":null,\"errorUpdateCount\":0,\"errorUpdatedAt\":0,\"fetchFailureCount\":0,\"fetchFailureReason\":null,\"fetchMeta\":null,\"isInvalidated\":false,\"status\":\"success\",\"fetchStatus\":\"idle\"},\"queryKey\":[\"paper\",\"2303.13117\",\"metadata\"],\"queryHash\":\"[\\\"paper\\\",\\\"2303.13117\\\",\\\"metadata\\\"]\"},{\"state\":{\"data\":{\"data\":[]},\"dataUpdateCount\":1,\"dataUpdatedAt\":1744228229751,\"error\":null,\"errorUpdateCount\":0,\"errorUpdatedAt\":0,\"fetchFailureCount\":0,\"fetchFailureReason\":null,\"fetchMeta\":null,\"isInvalidated\":false,\"status\":\"success\",\"fetchStatus\":\"idle\"},\"queryKey\":[\"paper\",\"2303.13117\",\"comments\"],\"queryHash\":\"[\\\"paper\\\",\\\"2303.13117\\\",\\\"comments\\\"]\"},{\"state\":{\"data\":{\"data\":{\"paper_version\":{\"_id\":\"673d1c982025a7c320108b28\",\"paper_group_id\":\"673d1c972025a7c320108b26\",\"version_label\":\"v2\",\"version_order\":2,\"title\":\"Can Large Language Models Automatically Score Proficiency of Written Essays?\",\"abstract\":\"$6b\",\"author_ids\":[\"673cf6d2615941b897fb6d6a\",\"673b8e32bf626fe16b8aabac\",\"673b8e32bf626fe16b8aabad\",\"673418a529b032f35709ab4a\"],\"publication_date\":\"2024-04-16T00:24:55.000Z\",\"license\":\"http://creativecommons.org/licenses/by-nc-sa/4.0/\",\"created_at\":\"2024-11-19T23:17:44.099Z\",\"updated_at\":\"2024-11-19T23:17:44.099Z\",\"is_deleted\":false,\"is_hidden\":false,\"universal_paper_id\":\"2403.06149\",\"imageURL\":\"image/2403.06149v2.png\"},\"paper_group\":{\"_id\":\"673d1c972025a7c320108b26\",\"universal_paper_id\":\"2403.06149\",\"source\":{\"name\":\"arXiv\",\"url\":\"https://arXiv.org/paper/2403.06149\"},\"title\":\"Can Large Language Models Automatically Score Proficiency of Written Essays?\",\"created_at\":\"2024-11-04T12:55:58.057Z\",\"updated_at\":\"2025-03-03T19:55:43.659Z\",\"categories\":[\"Computer Science\"],\"subcategories\":[\"cs.CL\",\"cs.AI\"],\"metrics\":{\"activity_rank\":0,\"questions_count\":0,\"responses_count\":0,\"upvotes_count\":null,\"downvotes_count\":null,\"total_votes\":0,\"visits_count\":{\"last24Hours\":1,\"last7Days\":6,\"last30Days\":12,\"last90Days\":15,\"all\":66},\"weighted_visits\":{\"last24Hours\":5.3920944526034465e-62,\"last7Days\":1.0605842603910773e-8,\"last30Days\":0.10886956702963012,\"last90Days\":3.1284773060522375,\"hot\":1.0605842603910773e-8},\"public_total_votes\":2,\"timeline\":[{\"date\":\"2025-04-02T01:55:57.137Z\",\"views\":7},{\"date\":\"2025-03-29T13:55:57.137Z\",\"views\":9},{\"date\":\"2025-03-26T01:55:57.137Z\",\"views\":12},{\"date\":\"2025-03-22T13:55:57.137Z\",\"views\":6},{\"date\":\"2025-03-19T01:55:57.137Z\",\"views\":0},{\"date\":\"2025-03-15T13:55:57.137Z\",\"views\":1},{\"date\":\"2025-03-12T01:55:57.137Z\",\"views\":1},{\"date\":\"2025-03-08T13:55:57.137Z\",\"views\":2},{\"date\":\"2025-03-05T01:55:57.137Z\",\"views\":2},{\"date\":\"2025-03-01T13:55:57.137Z\",\"views\":0},{\"date\":\"2025-02-26T01:55:57.137Z\",\"views\":2},{\"date\":\"2025-02-22T13:55:57.137Z\",\"views\":1},{\"date\":\"2025-02-19T01:55:57.146Z\",\"views\":4},{\"date\":\"2025-02-15T13:55:57.163Z\",\"views\":1},{\"date\":\"2025-02-12T01:55:57.178Z\",\"views\":0},{\"date\":\"2025-02-08T13:55:57.196Z\",\"views\":2},{\"date\":\"2025-02-05T01:55:57.212Z\",\"views\":2},{\"date\":\"2025-02-01T13:55:57.230Z\",\"views\":1},{\"date\":\"2025-01-29T01:55:57.251Z\",\"views\":1},{\"date\":\"2025-01-25T13:55:57.269Z\",\"views\":1},{\"date\":\"2025-01-22T01:55:57.287Z\",\"views\":2},{\"date\":\"2025-01-18T13:55:57.310Z\",\"views\":2},{\"date\":\"2025-01-15T01:55:57.325Z\",\"views\":0},{\"date\":\"2025-01-11T13:55:57.341Z\",\"views\":1},{\"date\":\"2025-01-08T01:55:57.360Z\",\"views\":3},{\"date\":\"2025-01-04T13:55:57.377Z\",\"views\":5},{\"date\":\"2025-01-01T01:55:57.396Z\",\"views\":1},{\"date\":\"2024-12-28T13:55:57.414Z\",\"views\":2},{\"date\":\"2024-12-25T01:55:57.429Z\",\"views\":4},{\"date\":\"2024-12-21T13:55:57.447Z\",\"views\":3},{\"date\":\"2024-12-18T01:55:57.464Z\",\"views\":1},{\"date\":\"2024-12-14T13:55:57.479Z\",\"views\":2},{\"date\":\"2024-12-11T01:55:57.497Z\",\"views\":2},{\"date\":\"2024-12-07T13:55:57.511Z\",\"views\":1},{\"date\":\"2024-12-04T01:55:57.528Z\",\"views\":1},{\"date\":\"2024-11-30T13:55:57.543Z\",\"views\":0},{\"date\":\"2024-11-27T01:55:57.556Z\",\"views\":1},{\"date\":\"2024-11-23T13:55:57.572Z\",\"views\":0},{\"date\":\"2024-11-20T01:55:57.594Z\",\"views\":0},{\"date\":\"2024-11-16T13:55:57.612Z\",\"views\":1},{\"date\":\"2024-11-13T01:55:57.627Z\",\"views\":2},{\"date\":\"2024-11-09T13:55:57.725Z\",\"views\":2},{\"date\":\"2024-11-06T01:55:57.742Z\",\"views\":1},{\"date\":\"2024-11-02T12:55:57.758Z\",\"views\":16},{\"date\":\"2024-10-30T00:55:57.776Z\",\"views\":2},{\"date\":\"2024-10-26T12:55:57.794Z\",\"views\":1},{\"date\":\"2024-10-23T00:55:57.813Z\",\"views\":0},{\"date\":\"2024-10-19T12:55:57.830Z\",\"views\":1},{\"date\":\"2024-10-16T00:55:57.846Z\",\"views\":0},{\"date\":\"2024-10-12T12:55:57.863Z\",\"views\":1},{\"date\":\"2024-10-09T00:55:57.977Z\",\"views\":1},{\"date\":\"2024-10-05T12:55:57.998Z\",\"views\":2},{\"date\":\"2024-10-02T00:55:58.013Z\",\"views\":0},{\"date\":\"2024-09-28T12:55:58.043Z\",\"views\":0},{\"date\":\"2024-09-25T00:55:58.062Z\",\"views\":1},{\"date\":\"2024-09-21T12:55:58.079Z\",\"views\":2},{\"date\":\"2024-09-18T00:55:58.095Z\",\"views\":2},{\"date\":\"2024-09-14T12:55:58.115Z\",\"views\":0},{\"date\":\"2024-09-11T00:55:58.134Z\",\"views\":0},{\"date\":\"2024-09-07T12:55:58.164Z\",\"views\":2},{\"date\":\"2024-09-04T00:55:58.204Z\",\"views\":1},{\"date\":\"2024-08-31T12:55:58.213Z\",\"views\":2},{\"date\":\"2024-08-28T00:55:58.223Z\",\"views\":2}]},\"ranking\":{\"current_rank\":126103,\"previous_rank\":125718,\"activity_score\":0,\"paper_score\":0},\"is_hidden\":false,\"custom_categories\":null,\"first_publication_date\":\"2024-04-16T00:24:55.000Z\",\"author_user_ids\":[],\"resources\":{\"github\":{\"url\":\"https://github.com/Watheq9/AES-with-LLMs\",\"description\":\"This repo contains the data resulted from our paper titled \\\"Can Large Language Models Automatically Score Proficiency of Written Essays?\\\"\",\"language\":null,\"stars\":0}},\"paperVersions\":{\"_id\":\"673d1c982025a7c320108b28\",\"paper_group_id\":\"673d1c972025a7c320108b26\",\"version_label\":\"v2\",\"version_order\":2,\"title\":\"Can Large Language Models Automatically Score Proficiency of Written Essays?\",\"abstract\":\"$6c\",\"author_ids\":[\"673cf6d2615941b897fb6d6a\",\"673b8e32bf626fe16b8aabac\",\"673b8e32bf626fe16b8aabad\",\"673418a529b032f35709ab4a\"],\"publication_date\":\"2024-04-16T00:24:55.000Z\",\"license\":\"http://creativecommons.org/licenses/by-nc-sa/4.0/\",\"created_at\":\"2024-11-19T23:17:44.099Z\",\"updated_at\":\"2024-11-19T23:17:44.099Z\",\"is_deleted\":false,\"is_hidden\":false,\"universal_paper_id\":\"2403.06149\",\"imageURL\":\"image/2403.06149v2.png\"},\"verifiedAuthors\":[],\"authors\":[{\"_id\":\"673418a529b032f35709ab4a\",\"full_name\":\"Tamer Elsayed\",\"affiliation\":null,\"orcid\":null,\"semantic_scholarid\":null,\"user_id\":null},{\"_id\":\"673b8e32bf626fe16b8aabac\",\"full_name\":\"Salam Albatarni\",\"affiliation\":null,\"orcid\":null,\"semantic_scholarid\":null,\"user_id\":null},{\"_id\":\"673b8e32bf626fe16b8aabad\",\"full_name\":\"Sohaila Eltanbouly\",\"affiliation\":null,\"orcid\":null,\"semantic_scholarid\":null,\"user_id\":null},{\"_id\":\"673cf6d2615941b897fb6d6a\",\"full_name\":\"Watheq Mansour\",\"affiliation\":null,\"orcid\":null,\"semantic_scholarid\":null,\"user_id\":null}]},\"max_version_order\":2,\"verified_authors\":[],\"authors\":[{\"_id\":\"673418a529b032f35709ab4a\",\"full_name\":\"Tamer Elsayed\",\"affiliation\":null,\"orcid\":null,\"semantic_scholarid\":null,\"user_id\":null},{\"_id\":\"673b8e32bf626fe16b8aabac\",\"full_name\":\"Salam Albatarni\",\"affiliation\":null,\"orcid\":null,\"semantic_scholarid\":null,\"user_id\":null},{\"_id\":\"673b8e32bf626fe16b8aabad\",\"full_name\":\"Sohaila Eltanbouly\",\"affiliation\":null,\"orcid\":null,\"semantic_scholarid\":null,\"user_id\":null},{\"_id\":\"673cf6d2615941b897fb6d6a\",\"full_name\":\"Watheq Mansour\",\"affiliation\":null,\"orcid\":null,\"semantic_scholarid\":null,\"user_id\":null}],\"pdf_info\":{\"fetcher_url\":\"https://fetcher.alphaxiv.org/v2/pdf/2403.06149v2\"}}},\"dataUpdateCount\":1,\"dataUpdatedAt\":1744228232644,\"error\":null,\"errorUpdateCount\":0,\"errorUpdatedAt\":0,\"fetchFailureCount\":0,\"fetchFailureReason\":null,\"fetchMeta\":null,\"isInvalidated\":false,\"status\":\"success\",\"fetchStatus\":\"idle\"},\"queryKey\":[\"paper\",\"2403.06149\",\"metadata\"],\"queryHash\":\"[\\\"paper\\\",\\\"2403.06149\\\",\\\"metadata\\\"]\"},{\"state\":{\"data\":{\"data\":[]},\"dataUpdateCount\":1,\"dataUpdatedAt\":1744228232644,\"error\":null,\"errorUpdateCount\":0,\"errorUpdatedAt\":0,\"fetchFailureCount\":0,\"fetchFailureReason\":null,\"fetchMeta\":null,\"isInvalidated\":false,\"status\":\"success\",\"fetchStatus\":\"idle\"},\"queryKey\":[\"paper\",\"2403.06149\",\"comments\"],\"queryHash\":\"[\\\"paper\\\",\\\"2403.06149\\\",\\\"comments\\\"]\"},{\"state\":{\"data\":{\"data\":{\"paper_version\":{\"_id\":\"677e56f5a79894af4b88af30\",\"paper_group_id\":\"677e56f4a79894af4b88af2f\",\"version_label\":\"v2\",\"version_order\":2,\"title\":\"Higher topological complexity of a map\",\"abstract\":\"$6d\",\"author_ids\":[\"677d4bdbbc1fc17816a2a3a9\",\"673cd9058a52218f8bc998de\"],\"publication_date\":\"2023-03-23T08:00:31.000Z\",\"license\":\"http://arxiv.org/licenses/nonexclusive-distrib/1.0/\",\"created_at\":\"2025-01-08T10:44:05.328Z\",\"updated_at\":\"2025-01-08T10:44:05.328Z\",\"is_deleted\":false,\"is_hidden\":false,\"universal_paper_id\":\"2212.03441\",\"imageURL\":\"image/2212.03441v2.png\"},\"paper_group\":{\"_id\":\"677e56f4a79894af4b88af2f\",\"universal_paper_id\":\"2212.03441\",\"title\":\"Higher topological complexity of a map\",\"created_at\":\"2025-01-08T10:44:04.866Z\",\"updated_at\":\"2025-03-03T20:18:39.689Z\",\"categories\":[\"Mathematics\"],\"subcategories\":[\"math.AT\"],\"custom_categories\":null,\"author_user_ids\":[],\"source\":{\"name\":\"alphaXiv\",\"url\":\"https://arxiv.org/paper/2212.03441\"},\"metrics\":{\"activity_rank\":0,\"questions_count\":0,\"responses_count\":0,\"upvotes_count\":0,\"downvotes_count\":0,\"total_votes\":0,\"visits_count\":{\"last24Hours\":0,\"last7Days\":6,\"last30Days\":6,\"last90Days\":8,\"all\":24},\"weighted_visits\":{\"last24Hours\":0,\"last7Days\":2.2562137018747826e-18,\"last30Days\":0.00030132697575228597,\"last90Days\":0.2951564248034132,\"hot\":2.2562137018747826e-18},\"public_total_votes\":0,\"timeline\":[{\"date\":\"2025-04-03T01:57:44.631Z\",\"views\":18},{\"date\":\"2025-03-30T13:57:44.631Z\",\"views\":1},{\"date\":\"2025-03-27T01:57:44.631Z\",\"views\":2},{\"date\":\"2025-03-23T13:57:44.631Z\",\"views\":2},{\"date\":\"2025-03-20T01:57:44.631Z\",\"views\":1},{\"date\":\"2025-03-16T13:57:44.631Z\",\"views\":2},{\"date\":\"2025-03-13T01:57:44.631Z\",\"views\":2},{\"date\":\"2025-03-09T13:57:44.631Z\",\"views\":2},{\"date\":\"2025-03-06T01:57:44.631Z\",\"views\":1},{\"date\":\"2025-03-02T13:57:44.631Z\",\"views\":1},{\"date\":\"2025-02-27T01:57:44.631Z\",\"views\":3},{\"date\":\"2025-02-23T13:57:44.631Z\",\"views\":0},{\"date\":\"2025-02-20T01:57:44.665Z\",\"views\":2},{\"date\":\"2025-02-16T13:57:44.688Z\",\"views\":2},{\"date\":\"2025-02-13T01:57:44.711Z\",\"views\":0},{\"date\":\"2025-02-09T13:57:44.734Z\",\"views\":0},{\"date\":\"2025-02-06T01:57:44.762Z\",\"views\":0},{\"date\":\"2025-02-02T13:57:44.785Z\",\"views\":1},{\"date\":\"2025-01-30T01:57:44.821Z\",\"views\":2},{\"date\":\"2025-01-26T13:57:44.846Z\",\"views\":1},{\"date\":\"2025-01-23T01:57:44.871Z\",\"views\":1},{\"date\":\"2025-01-19T13:57:44.894Z\",\"views\":0},{\"date\":\"2025-01-16T01:57:44.918Z\",\"views\":2},{\"date\":\"2025-01-12T13:57:44.948Z\",\"views\":2},{\"date\":\"2025-01-09T01:57:44.974Z\",\"views\":2},{\"date\":\"2025-01-05T13:57:44.998Z\",\"views\":5},{\"date\":\"2025-01-02T01:57:45.019Z\",\"views\":1},{\"date\":\"2024-12-29T13:57:45.039Z\",\"views\":2},{\"date\":\"2024-12-26T01:57:45.060Z\",\"views\":2},{\"date\":\"2024-12-22T13:57:45.086Z\",\"views\":0},{\"date\":\"2024-12-19T01:57:45.112Z\",\"views\":2},{\"date\":\"2024-12-15T13:57:45.140Z\",\"views\":1},{\"date\":\"2024-12-12T01:57:45.165Z\",\"views\":0},{\"date\":\"2024-12-08T13:57:45.190Z\",\"views\":1},{\"date\":\"2024-12-05T01:57:45.211Z\",\"views\":0},{\"date\":\"2024-12-01T13:57:45.231Z\",\"views\":0},{\"date\":\"2024-11-28T01:57:45.272Z\",\"views\":2},{\"date\":\"2024-11-24T13:57:45.293Z\",\"views\":2},{\"date\":\"2024-11-21T01:57:45.314Z\",\"views\":1},{\"date\":\"2024-11-17T13:57:45.334Z\",\"views\":0},{\"date\":\"2024-11-14T01:57:45.357Z\",\"views\":1},{\"date\":\"2024-11-10T13:57:45.378Z\",\"views\":0},{\"date\":\"2024-11-07T01:57:45.399Z\",\"views\":1},{\"date\":\"2024-11-03T13:57:45.420Z\",\"views\":0},{\"date\":\"2024-10-31T00:57:45.452Z\",\"views\":1},{\"date\":\"2024-10-27T12:57:45.476Z\",\"views\":0},{\"date\":\"2024-10-24T00:57:45.502Z\",\"views\":0},{\"date\":\"2024-10-20T12:57:45.523Z\",\"views\":1},{\"date\":\"2024-10-17T00:57:45.544Z\",\"views\":2},{\"date\":\"2024-10-13T12:57:45.564Z\",\"views\":2},{\"date\":\"2024-10-10T00:57:45.587Z\",\"views\":1},{\"date\":\"2024-10-06T12:57:45.610Z\",\"views\":2},{\"date\":\"2024-10-03T00:57:45.632Z\",\"views\":0},{\"date\":\"2024-09-29T12:57:45.714Z\",\"views\":0},{\"date\":\"2024-09-26T00:57:45.732Z\",\"views\":0},{\"date\":\"2024-09-22T12:57:45.756Z\",\"views\":1},{\"date\":\"2024-09-19T00:57:45.774Z\",\"views\":0},{\"date\":\"2024-09-15T12:57:45.788Z\",\"views\":2},{\"date\":\"2024-09-12T00:57:45.808Z\",\"views\":0},{\"date\":\"2024-09-08T12:57:45.819Z\",\"views\":2},{\"date\":\"2024-09-05T00:57:45.837Z\",\"views\":2},{\"date\":\"2024-09-01T12:57:45.898Z\",\"views\":1},{\"date\":\"2024-08-29T00:57:45.923Z\",\"views\":1}]},\"is_hidden\":false,\"first_publication_date\":\"2023-03-23T08:00:31.000Z\",\"paperVersions\":{\"_id\":\"677e56f5a79894af4b88af30\",\"paper_group_id\":\"677e56f4a79894af4b88af2f\",\"version_label\":\"v2\",\"version_order\":2,\"title\":\"Higher topological complexity of a map\",\"abstract\":\"$6e\",\"author_ids\":[\"677d4bdbbc1fc17816a2a3a9\",\"673cd9058a52218f8bc998de\"],\"publication_date\":\"2023-03-23T08:00:31.000Z\",\"license\":\"http://arxiv.org/licenses/nonexclusive-distrib/1.0/\",\"created_at\":\"2025-01-08T10:44:05.328Z\",\"updated_at\":\"2025-01-08T10:44:05.328Z\",\"is_deleted\":false,\"is_hidden\":false,\"universal_paper_id\":\"2212.03441\",\"imageURL\":\"image/2212.03441v2.png\"},\"verifiedAuthors\":[],\"authors\":[{\"_id\":\"673cd9058a52218f8bc998de\",\"full_name\":\"Jesús González\",\"affiliation\":null,\"orcid\":null,\"semantic_scholarid\":null,\"user_id\":null},{\"_id\":\"677d4bdbbc1fc17816a2a3a9\",\"full_name\":\"Cesar A. Ipanaque Zapata\",\"affiliation\":null,\"orcid\":null,\"semantic_scholarid\":null,\"user_id\":null}]},\"max_version_order\":2,\"verified_authors\":[],\"authors\":[{\"_id\":\"673cd9058a52218f8bc998de\",\"full_name\":\"Jesús González\",\"affiliation\":null,\"orcid\":null,\"semantic_scholarid\":null,\"user_id\":null},{\"_id\":\"677d4bdbbc1fc17816a2a3a9\",\"full_name\":\"Cesar A. Ipanaque Zapata\",\"affiliation\":null,\"orcid\":null,\"semantic_scholarid\":null,\"user_id\":null}],\"pdf_info\":{\"fetcher_url\":\"https://fetcher.alphaxiv.org/v2/pdf/2212.03441v2\"}}},\"dataUpdateCount\":1,\"dataUpdatedAt\":1744228232734,\"error\":null,\"errorUpdateCount\":0,\"errorUpdatedAt\":0,\"fetchFailureCount\":0,\"fetchFailureReason\":null,\"fetchMeta\":null,\"isInvalidated\":false,\"status\":\"success\",\"fetchStatus\":\"idle\"},\"queryKey\":[\"paper\",\"2212.03441\",\"metadata\"],\"queryHash\":\"[\\\"paper\\\",\\\"2212.03441\\\",\\\"metadata\\\"]\"},{\"state\":{\"data\":{\"data\":[]},\"dataUpdateCount\":1,\"dataUpdatedAt\":1744228232734,\"error\":null,\"errorUpdateCount\":0,\"errorUpdatedAt\":0,\"fetchFailureCount\":0,\"fetchFailureReason\":null,\"fetchMeta\":null,\"isInvalidated\":false,\"status\":\"success\",\"fetchStatus\":\"idle\"},\"queryKey\":[\"paper\",\"2212.03441\",\"comments\"],\"queryHash\":\"[\\\"paper\\\",\\\"2212.03441\\\",\\\"comments\\\"]\"},{\"state\":{\"data\":{\"data\":{\"paper_version\":{\"_id\":\"67ac58a9d2eff6b9cfae247b\",\"paper_group_id\":\"67ac58a8d2eff6b9cfae247a\",\"version_label\":\"v1\",\"version_order\":1,\"title\":\"LEMDA: A Novel Feature Engineering Method for Intrusion Detection in IoT Systems\",\"abstract\":\"$6f\",\"author_ids\":[\"672bcba8986a1370676da847\",\"672bcba7986a1370676da841\",\"673b73f4ee7cdcdc03b13c06\",\"672bcba8986a1370676da84d\"],\"publication_date\":\"2024-04-20T11:11:47.000Z\",\"license\":\"http://arxiv.org/licenses/nonexclusive-distrib/1.0/\",\"created_at\":\"2025-02-12T08:15:37.489Z\",\"updated_at\":\"2025-02-12T08:15:37.489Z\",\"is_deleted\":false,\"is_hidden\":false,\"universal_paper_id\":\"2404.16870\",\"imageURL\":\"image/2404.16870v1.png\"},\"paper_group\":{\"_id\":\"67ac58a8d2eff6b9cfae247a\",\"universal_paper_id\":\"2404.16870\",\"title\":\"LEMDA: A Novel Feature Engineering Method for Intrusion Detection in IoT Systems\",\"created_at\":\"2025-02-12T08:15:36.900Z\",\"updated_at\":\"2025-03-03T19:55:24.978Z\",\"categories\":[\"Computer Science\"],\"subcategories\":[\"cs.CR\",\"cs.AI\",\"cs.LG\"],\"custom_categories\":[\"cybersecurity\",\"ai-for-cybersecurity\",\"network-security\",\"efficient-transformers\"],\"author_user_ids\":[],\"source\":{\"name\":\"alphaXiv\",\"url\":\"https://arxiv.org/abs/2404.16870\"},\"metrics\":{\"activity_rank\":0,\"questions_count\":0,\"responses_count\":0,\"upvotes_count\":0,\"downvotes_count\":0,\"total_votes\":0,\"public_total_votes\":0,\"visits_count\":{\"last24Hours\":0,\"last7Days\":3,\"last30Days\":4,\"last90Days\":7,\"all\":22},\"weighted_visits\":{\"last24Hours\":0,\"last7Days\":6.8384055506908554e-9,\"last30Days\":0.0385083133936847,\"last90Days\":1.489119470362468,\"hot\":6.8384055506908554e-9},\"timeline\":[{\"date\":\"2025-04-02T01:55:17.535Z\",\"views\":4},{\"date\":\"2025-03-29T13:55:17.535Z\",\"views\":7},{\"date\":\"2025-03-26T01:55:17.535Z\",\"views\":1},{\"date\":\"2025-03-22T13:55:17.535Z\",\"views\":4},{\"date\":\"2025-03-19T01:55:17.535Z\",\"views\":0},{\"date\":\"2025-03-15T13:55:17.535Z\",\"views\":2},{\"date\":\"2025-03-12T01:55:17.535Z\",\"views\":1},{\"date\":\"2025-03-08T13:55:17.535Z\",\"views\":0},{\"date\":\"2025-03-05T01:55:17.535Z\",\"views\":2},{\"date\":\"2025-03-01T13:55:17.535Z\",\"views\":0},{\"date\":\"2025-02-26T01:55:17.535Z\",\"views\":4},{\"date\":\"2025-02-22T13:55:17.535Z\",\"views\":3},{\"date\":\"2025-02-19T01:55:17.557Z\",\"views\":2},{\"date\":\"2025-02-15T13:55:17.572Z\",\"views\":1},{\"date\":\"2025-02-12T01:55:17.588Z\",\"views\":5},{\"date\":\"2025-02-08T13:55:17.607Z\",\"views\":1},{\"date\":\"2025-02-05T01:55:17.623Z\",\"views\":1},{\"date\":\"2025-02-01T13:55:17.641Z\",\"views\":2},{\"date\":\"2025-01-29T01:55:17.662Z\",\"views\":0},{\"date\":\"2025-01-25T13:55:17.677Z\",\"views\":0},{\"date\":\"2025-01-22T01:55:17.692Z\",\"views\":0},{\"date\":\"2025-01-18T13:55:17.712Z\",\"views\":1},{\"date\":\"2025-01-15T01:55:17.728Z\",\"views\":0},{\"date\":\"2025-01-11T13:55:17.746Z\",\"views\":0},{\"date\":\"2025-01-08T01:55:17.763Z\",\"views\":0},{\"date\":\"2025-01-04T13:55:17.778Z\",\"views\":1},{\"date\":\"2025-01-01T01:55:17.796Z\",\"views\":2},{\"date\":\"2024-12-28T13:55:17.813Z\",\"views\":0},{\"date\":\"2024-12-25T01:55:17.829Z\",\"views\":1},{\"date\":\"2024-12-21T13:55:17.846Z\",\"views\":2},{\"date\":\"2024-12-18T01:55:17.870Z\",\"views\":0},{\"date\":\"2024-12-14T13:55:17.889Z\",\"views\":2},{\"date\":\"2024-12-11T01:55:17.905Z\",\"views\":0},{\"date\":\"2024-12-07T13:55:17.920Z\",\"views\":2},{\"date\":\"2024-12-04T01:55:17.936Z\",\"views\":2},{\"date\":\"2024-11-30T13:55:17.954Z\",\"views\":2},{\"date\":\"2024-11-27T01:55:17.974Z\",\"views\":0},{\"date\":\"2024-11-23T13:55:17.991Z\",\"views\":1},{\"date\":\"2024-11-20T01:55:18.007Z\",\"views\":0},{\"date\":\"2024-11-16T13:55:18.033Z\",\"views\":1},{\"date\":\"2024-11-13T01:55:18.047Z\",\"views\":2},{\"date\":\"2024-11-09T13:55:18.067Z\",\"views\":2},{\"date\":\"2024-11-06T01:55:18.084Z\",\"views\":0},{\"date\":\"2024-11-02T12:55:18.100Z\",\"views\":1},{\"date\":\"2024-10-30T00:55:18.114Z\",\"views\":0},{\"date\":\"2024-10-26T12:55:18.129Z\",\"views\":0},{\"date\":\"2024-10-23T00:55:18.183Z\",\"views\":0},{\"date\":\"2024-10-19T12:55:18.202Z\",\"views\":0},{\"date\":\"2024-10-16T00:55:18.216Z\",\"views\":2},{\"date\":\"2024-10-12T12:55:18.231Z\",\"views\":1},{\"date\":\"2024-10-09T00:55:18.247Z\",\"views\":1},{\"date\":\"2024-10-05T12:55:18.274Z\",\"views\":0},{\"date\":\"2024-10-02T00:55:18.291Z\",\"views\":1},{\"date\":\"2024-09-28T12:55:18.305Z\",\"views\":1},{\"date\":\"2024-09-25T00:55:18.320Z\",\"views\":0},{\"date\":\"2024-09-21T12:55:18.351Z\",\"views\":0},{\"date\":\"2024-09-18T00:55:18.370Z\",\"views\":1},{\"date\":\"2024-09-14T12:55:18.386Z\",\"views\":1},{\"date\":\"2024-09-11T00:55:18.401Z\",\"views\":0},{\"date\":\"2024-09-07T12:55:18.417Z\",\"views\":2},{\"date\":\"2024-09-04T00:55:18.433Z\",\"views\":1},{\"date\":\"2024-08-31T12:55:18.448Z\",\"views\":0},{\"date\":\"2024-08-28T00:55:18.465Z\",\"views\":0}]},\"is_hidden\":false,\"first_publication_date\":\"2024-04-20T11:11:47.000Z\",\"organizations\":[\"67be6491aa92218ccd8b3d32\",\"67be6389aa92218ccd8b15c4\"],\"paperVersions\":{\"_id\":\"67ac58a9d2eff6b9cfae247b\",\"paper_group_id\":\"67ac58a8d2eff6b9cfae247a\",\"version_label\":\"v1\",\"version_order\":1,\"title\":\"LEMDA: A Novel Feature Engineering Method for Intrusion Detection in IoT Systems\",\"abstract\":\"$70\",\"author_ids\":[\"672bcba8986a1370676da847\",\"672bcba7986a1370676da841\",\"673b73f4ee7cdcdc03b13c06\",\"672bcba8986a1370676da84d\"],\"publication_date\":\"2024-04-20T11:11:47.000Z\",\"license\":\"http://arxiv.org/licenses/nonexclusive-distrib/1.0/\",\"created_at\":\"2025-02-12T08:15:37.489Z\",\"updated_at\":\"2025-02-12T08:15:37.489Z\",\"is_deleted\":false,\"is_hidden\":false,\"universal_paper_id\":\"2404.16870\",\"imageURL\":\"image/2404.16870v1.png\"},\"verifiedAuthors\":[],\"authors\":[{\"_id\":\"672bcba7986a1370676da841\",\"full_name\":\"Zebo Yang\",\"affiliation\":null,\"orcid\":null,\"semantic_scholarid\":null,\"user_id\":null},{\"_id\":\"672bcba8986a1370676da847\",\"full_name\":\"Ali Ghubaish\",\"affiliation\":null,\"orcid\":null,\"semantic_scholarid\":null,\"user_id\":null},{\"_id\":\"672bcba8986a1370676da84d\",\"full_name\":\"Raj Jain\",\"affiliation\":null,\"orcid\":null,\"semantic_scholarid\":null,\"user_id\":null},{\"_id\":\"673b73f4ee7cdcdc03b13c06\",\"full_name\":\"Aiman Erbad\",\"affiliation\":null,\"orcid\":null,\"semantic_scholarid\":null,\"user_id\":null}]},\"max_version_order\":1,\"verified_authors\":[],\"authors\":[{\"_id\":\"672bcba7986a1370676da841\",\"full_name\":\"Zebo Yang\",\"affiliation\":null,\"orcid\":null,\"semantic_scholarid\":null,\"user_id\":null},{\"_id\":\"672bcba8986a1370676da847\",\"full_name\":\"Ali Ghubaish\",\"affiliation\":null,\"orcid\":null,\"semantic_scholarid\":null,\"user_id\":null},{\"_id\":\"672bcba8986a1370676da84d\",\"full_name\":\"Raj Jain\",\"affiliation\":null,\"orcid\":null,\"semantic_scholarid\":null,\"user_id\":null},{\"_id\":\"673b73f4ee7cdcdc03b13c06\",\"full_name\":\"Aiman Erbad\",\"affiliation\":null,\"orcid\":null,\"semantic_scholarid\":null,\"user_id\":null}],\"pdf_info\":{\"fetcher_url\":\"https://fetcher.alphaxiv.org/v2/pdf/2404.16870v1\"}}},\"dataUpdateCount\":1,\"dataUpdatedAt\":1744228233543,\"error\":null,\"errorUpdateCount\":0,\"errorUpdatedAt\":0,\"fetchFailureCount\":0,\"fetchFailureReason\":null,\"fetchMeta\":null,\"isInvalidated\":false,\"status\":\"success\",\"fetchStatus\":\"idle\"},\"queryKey\":[\"paper\",\"2404.16870\",\"metadata\"],\"queryHash\":\"[\\\"paper\\\",\\\"2404.16870\\\",\\\"metadata\\\"]\"},{\"state\":{\"data\":{\"data\":[]},\"dataUpdateCount\":1,\"dataUpdatedAt\":1744228233543,\"error\":null,\"errorUpdateCount\":0,\"errorUpdatedAt\":0,\"fetchFailureCount\":0,\"fetchFailureReason\":null,\"fetchMeta\":null,\"isInvalidated\":false,\"status\":\"success\",\"fetchStatus\":\"idle\"},\"queryKey\":[\"paper\",\"2404.16870\",\"comments\"],\"queryHash\":\"[\\\"paper\\\",\\\"2404.16870\\\",\\\"comments\\\"]\"},{\"state\":{\"data\":{\"data\":{\"paper_version\":{\"_id\":\"67745d20890de0f04d436073\",\"paper_group_id\":\"67745d1f890de0f04d436072\",\"version_label\":\"v1\",\"version_order\":1,\"title\":\"Disentangling Preference Representation and Text Generation for Efficient Individual Preference Alignment\",\"abstract\":\"Aligning Large Language Models (LLMs) with general human preferences has been proved crucial in improving the interaction quality between LLMs and human. However, human values are inherently diverse among different individuals, making it insufficient to align LLMs solely with general preferences. To address this, personalizing LLMs according to individual feedback emerges as a promising solution. Nonetheless, this approach presents challenges in terms of the efficiency of alignment algorithms. In this work, we introduce a flexible paradigm for individual preference alignment. Our method fundamentally improves efficiency by disentangling preference representation from text generation in LLMs. We validate our approach across multiple text generation tasks and demonstrate that it can produce aligned quality as well as or better than PEFT-based methods, while reducing additional training time for each new individual preference by $80\\\\%$ to $90\\\\%$ in comparison with them.\",\"author_ids\":[\"673cd23b8a52218f8bc9797a\",\"6732286ecd1e32a6e7f037fa\",\"672bbf6e986a1370676d5e65\",\"67348c2893ee43749600f862\",\"673b7610ee7cdcdc03b14657\",\"672bcbf7986a1370676dad54\",\"67325d412aa08508fa766ba8\"],\"publication_date\":\"2024-12-30T09:58:31.000Z\",\"license\":\"http://creativecommons.org/licenses/by/4.0/\",\"created_at\":\"2024-12-31T21:07:44.566Z\",\"updated_at\":\"2024-12-31T21:07:44.566Z\",\"is_deleted\":false,\"is_hidden\":false,\"universal_paper_id\":\"2412.20834\",\"imageURL\":\"image/2412.20834v1.png\"},\"paper_group\":{\"_id\":\"67745d1f890de0f04d436072\",\"universal_paper_id\":\"2412.20834\",\"title\":\"Disentangling Preference Representation and Text Generation for Efficient Individual Preference Alignment\",\"created_at\":\"2024-12-31T21:07:43.541Z\",\"updated_at\":\"2025-03-03T19:38:06.161Z\",\"categories\":[\"Computer Science\"],\"subcategories\":[\"cs.CL\",\"cs.AI\"],\"custom_categories\":[\"parameter-efficient-training\",\"text-generation\",\"human-ai-interaction\"],\"author_user_ids\":[],\"source\":{\"name\":\"alphaXiv\",\"url\":\"https://arxiv.org/paper/2412.20834\"},\"metrics\":{\"activity_rank\":0,\"questions_count\":0,\"responses_count\":0,\"upvotes_count\":0,\"downvotes_count\":0,\"total_votes\":0,\"visits_count\":{\"last24Hours\":0,\"last7Days\":4,\"last30Days\":6,\"last90Days\":7,\"all\":33},\"weighted_visits\":{\"last24Hours\":0,\"last7Days\":0.018367265372279944,\"last30Days\":1.708497322751855,\"last90Days\":4.6052412996817,\"hot\":0.018367265372279944},\"public_total_votes\":0,\"timeline\":[{\"date\":\"2025-04-02T23:43:08.252Z\",\"views\":10},{\"date\":\"2025-03-30T11:43:08.252Z\",\"views\":5},{\"date\":\"2025-03-26T23:43:08.252Z\",\"views\":3},{\"date\":\"2025-03-23T11:43:08.252Z\",\"views\":0},{\"date\":\"2025-03-19T23:43:08.252Z\",\"views\":0},{\"date\":\"2025-03-16T11:43:08.252Z\",\"views\":0},{\"date\":\"2025-03-12T23:43:08.252Z\",\"views\":1},{\"date\":\"2025-03-09T11:43:08.252Z\",\"views\":3},{\"date\":\"2025-03-05T23:43:08.252Z\",\"views\":0},{\"date\":\"2025-03-02T11:43:08.252Z\",\"views\":0},{\"date\":\"2025-02-26T23:43:08.252Z\",\"views\":2},{\"date\":\"2025-02-23T11:43:08.252Z\",\"views\":2},{\"date\":\"2025-02-19T23:43:08.281Z\",\"views\":1},{\"date\":\"2025-02-16T11:43:08.306Z\",\"views\":0},{\"date\":\"2025-02-12T23:43:08.327Z\",\"views\":2},{\"date\":\"2025-02-09T11:43:08.369Z\",\"views\":2},{\"date\":\"2025-02-05T23:43:08.488Z\",\"views\":2},{\"date\":\"2025-02-02T11:43:08.517Z\",\"views\":0},{\"date\":\"2025-01-29T23:43:08.550Z\",\"views\":2},{\"date\":\"2025-01-26T11:43:08.575Z\",\"views\":0},{\"date\":\"2025-01-22T23:43:08.598Z\",\"views\":1},{\"date\":\"2025-01-19T11:43:08.630Z\",\"views\":1},{\"date\":\"2025-01-15T23:43:08.663Z\",\"views\":2},{\"date\":\"2025-01-12T11:43:08.712Z\",\"views\":1},{\"date\":\"2025-01-08T23:43:08.746Z\",\"views\":3},{\"date\":\"2025-01-05T11:43:08.779Z\",\"views\":1},{\"date\":\"2025-01-01T23:43:08.859Z\",\"views\":2},{\"date\":\"2024-12-29T11:43:08.894Z\",\"views\":12}]},\"is_hidden\":false,\"first_publication_date\":\"2024-12-30T09:58:31.000Z\",\"organizations\":[\"67be6378aa92218ccd8b1080\",\"67be63aaaa92218ccd8b1d5b\",\"67be6399aa92218ccd8b19bc\",\"67be6383aa92218ccd8b1400\",\"67be6378aa92218ccd8b107b\"],\"citation\":{\"bibtex\":\"@misc{li2024disentanglingpreferencerepresentation,\\n title={Disentangling Preference Representation and Text Generation for Efficient Individual Preference Alignment}, \\n author={Bei Li and Chenghua Lin and Jun Bai and Wenge Rong and Yanmeng Wang and Rumei Li and Jianfei Zhang},\\n year={2024},\\n eprint={2412.20834},\\n archivePrefix={arXiv},\\n primaryClass={cs.CL},\\n url={https://arxiv.org/abs/2412.20834}, \\n}\"},\"paperVersions\":{\"_id\":\"67745d20890de0f04d436073\",\"paper_group_id\":\"67745d1f890de0f04d436072\",\"version_label\":\"v1\",\"version_order\":1,\"title\":\"Disentangling Preference Representation and Text Generation for Efficient Individual Preference Alignment\",\"abstract\":\"Aligning Large Language Models (LLMs) with general human preferences has been proved crucial in improving the interaction quality between LLMs and human. However, human values are inherently diverse among different individuals, making it insufficient to align LLMs solely with general preferences. To address this, personalizing LLMs according to individual feedback emerges as a promising solution. Nonetheless, this approach presents challenges in terms of the efficiency of alignment algorithms. In this work, we introduce a flexible paradigm for individual preference alignment. Our method fundamentally improves efficiency by disentangling preference representation from text generation in LLMs. We validate our approach across multiple text generation tasks and demonstrate that it can produce aligned quality as well as or better than PEFT-based methods, while reducing additional training time for each new individual preference by $80\\\\%$ to $90\\\\%$ in comparison with them.\",\"author_ids\":[\"673cd23b8a52218f8bc9797a\",\"6732286ecd1e32a6e7f037fa\",\"672bbf6e986a1370676d5e65\",\"67348c2893ee43749600f862\",\"673b7610ee7cdcdc03b14657\",\"672bcbf7986a1370676dad54\",\"67325d412aa08508fa766ba8\"],\"publication_date\":\"2024-12-30T09:58:31.000Z\",\"license\":\"http://creativecommons.org/licenses/by/4.0/\",\"created_at\":\"2024-12-31T21:07:44.566Z\",\"updated_at\":\"2024-12-31T21:07:44.566Z\",\"is_deleted\":false,\"is_hidden\":false,\"universal_paper_id\":\"2412.20834\",\"imageURL\":\"image/2412.20834v1.png\"},\"verifiedAuthors\":[],\"authors\":[{\"_id\":\"672bbf6e986a1370676d5e65\",\"full_name\":\"Bei Li\",\"affiliation\":null,\"orcid\":null,\"semantic_scholarid\":null,\"user_id\":null},{\"_id\":\"672bcbf7986a1370676dad54\",\"full_name\":\"Chenghua Lin\",\"affiliation\":null,\"orcid\":null,\"semantic_scholarid\":null,\"user_id\":null},{\"_id\":\"6732286ecd1e32a6e7f037fa\",\"full_name\":\"Jun Bai\",\"affiliation\":null,\"orcid\":null,\"semantic_scholarid\":null,\"user_id\":null},{\"_id\":\"67325d412aa08508fa766ba8\",\"full_name\":\"Wenge Rong\",\"affiliation\":null,\"orcid\":null,\"semantic_scholarid\":null,\"user_id\":null},{\"_id\":\"67348c2893ee43749600f862\",\"full_name\":\"Yanmeng Wang\",\"affiliation\":null,\"orcid\":null,\"semantic_scholarid\":null,\"user_id\":null},{\"_id\":\"673b7610ee7cdcdc03b14657\",\"full_name\":\"Rumei Li\",\"affiliation\":null,\"orcid\":null,\"semantic_scholarid\":null,\"user_id\":null},{\"_id\":\"673cd23b8a52218f8bc9797a\",\"full_name\":\"Jianfei Zhang\",\"affiliation\":null,\"orcid\":null,\"semantic_scholarid\":null,\"user_id\":null}]},\"max_version_order\":1,\"verified_authors\":[],\"authors\":[{\"_id\":\"672bbf6e986a1370676d5e65\",\"full_name\":\"Bei Li\",\"affiliation\":null,\"orcid\":null,\"semantic_scholarid\":null,\"user_id\":null},{\"_id\":\"672bcbf7986a1370676dad54\",\"full_name\":\"Chenghua Lin\",\"affiliation\":null,\"orcid\":null,\"semantic_scholarid\":null,\"user_id\":null},{\"_id\":\"6732286ecd1e32a6e7f037fa\",\"full_name\":\"Jun Bai\",\"affiliation\":null,\"orcid\":null,\"semantic_scholarid\":null,\"user_id\":null},{\"_id\":\"67325d412aa08508fa766ba8\",\"full_name\":\"Wenge Rong\",\"affiliation\":null,\"orcid\":null,\"semantic_scholarid\":null,\"user_id\":null},{\"_id\":\"67348c2893ee43749600f862\",\"full_name\":\"Yanmeng Wang\",\"affiliation\":null,\"orcid\":null,\"semantic_scholarid\":null,\"user_id\":null},{\"_id\":\"673b7610ee7cdcdc03b14657\",\"full_name\":\"Rumei Li\",\"affiliation\":null,\"orcid\":null,\"semantic_scholarid\":null,\"user_id\":null},{\"_id\":\"673cd23b8a52218f8bc9797a\",\"full_name\":\"Jianfei Zhang\",\"affiliation\":null,\"orcid\":null,\"semantic_scholarid\":null,\"user_id\":null}],\"pdf_info\":{\"fetcher_url\":\"https://fetcher.alphaxiv.org/v2/pdf/2412.20834v1\"}}},\"dataUpdateCount\":1,\"dataUpdatedAt\":1744228233544,\"error\":null,\"errorUpdateCount\":0,\"errorUpdatedAt\":0,\"fetchFailureCount\":0,\"fetchFailureReason\":null,\"fetchMeta\":null,\"isInvalidated\":false,\"status\":\"success\",\"fetchStatus\":\"idle\"},\"queryKey\":[\"paper\",\"2412.20834\",\"metadata\"],\"queryHash\":\"[\\\"paper\\\",\\\"2412.20834\\\",\\\"metadata\\\"]\"},{\"state\":{\"data\":{\"data\":[]},\"dataUpdateCount\":1,\"dataUpdatedAt\":1744228233544,\"error\":null,\"errorUpdateCount\":0,\"errorUpdatedAt\":0,\"fetchFailureCount\":0,\"fetchFailureReason\":null,\"fetchMeta\":null,\"isInvalidated\":false,\"status\":\"success\",\"fetchStatus\":\"idle\"},\"queryKey\":[\"paper\",\"2412.20834\",\"comments\"],\"queryHash\":\"[\\\"paper\\\",\\\"2412.20834\\\",\\\"comments\\\"]\"},{\"state\":{\"data\":{\"data\":{\"paper_version\":{\"_id\":\"67a4de1ad2c41194fa0de460\",\"paper_group_id\":\"67a4de19d2c41194fa0de45e\",\"version_label\":\"v1\",\"version_order\":1,\"title\":\"End-to-end Sinkhorn Autoencoder with Noise Generator\",\"abstract\":\"$71\",\"author_ids\":[\"673223ebcd1e32a6e7efec30\",\"673b75efbf626fe16b8a7a6f\",\"67a4de1ad2c41194fa0de45f\",\"673b7f9fee7cdcdc03b15f8c\",\"6732279ecd1e32a6e7f029d8\"],\"publication_date\":\"2020-06-11T18:04:10.000Z\",\"license\":\"http://arxiv.org/licenses/nonexclusive-distrib/1.0/\",\"created_at\":\"2025-02-06T16:06:50.299Z\",\"updated_at\":\"2025-02-06T16:06:50.299Z\",\"is_deleted\":false,\"is_hidden\":false,\"universal_paper_id\":\"2006.06704\",\"imageURL\":\"image/2006.06704v1.png\"},\"paper_group\":{\"_id\":\"67a4de19d2c41194fa0de45e\",\"universal_paper_id\":\"2006.06704\",\"title\":\"End-to-end Sinkhorn Autoencoder with Noise Generator\",\"created_at\":\"2025-02-06T16:06:49.182Z\",\"updated_at\":\"2025-03-03T20:55:28.600Z\",\"categories\":[\"Computer Science\",\"Statistics\"],\"subcategories\":[\"cs.LG\",\"stat.ML\"],\"custom_categories\":[\"generative-models\",\"unsupervised-learning\",\"representation-learning\",\"synthetic-data\"],\"author_user_ids\":[],\"source\":{\"name\":\"alphaXiv\",\"url\":\"https://arxiv.org/abs/2006.06704\"},\"metrics\":{\"activity_rank\":0,\"questions_count\":0,\"responses_count\":0,\"upvotes_count\":0,\"downvotes_count\":0,\"total_votes\":0,\"public_total_votes\":0,\"visits_count\":{\"last24Hours\":0,\"last7Days\":0,\"last30Days\":2,\"last90Days\":5,\"all\":5},\"weighted_visits\":{\"last24Hours\":0,\"last7Days\":0,\"last30Days\":1.3381490576177018e-10,\"last90Days\":0.002029838583111892,\"hot\":0},\"timeline\":[{\"date\":\"2025-04-03T03:13:27.149Z\",\"views\":0},{\"date\":\"2025-03-30T15:13:27.149Z\",\"views\":0},{\"date\":\"2025-03-27T03:13:27.149Z\",\"views\":3},{\"date\":\"2025-03-23T15:13:27.149Z\",\"views\":2},{\"date\":\"2025-03-20T03:13:27.149Z\",\"views\":2},{\"date\":\"2025-03-16T15:13:27.149Z\",\"views\":0},{\"date\":\"2025-03-13T03:13:27.149Z\",\"views\":3},{\"date\":\"2025-03-09T15:13:27.149Z\",\"views\":1},{\"date\":\"2025-03-06T03:13:27.149Z\",\"views\":2},{\"date\":\"2025-03-02T15:13:27.149Z\",\"views\":2},{\"date\":\"2025-02-27T03:13:27.149Z\",\"views\":0},{\"date\":\"2025-02-23T15:13:27.149Z\",\"views\":0},{\"date\":\"2025-02-20T03:13:27.159Z\",\"views\":2},{\"date\":\"2025-02-16T15:13:27.171Z\",\"views\":0},{\"date\":\"2025-02-13T03:13:27.185Z\",\"views\":2},{\"date\":\"2025-02-09T15:13:27.217Z\",\"views\":0},{\"date\":\"2025-02-06T03:13:27.239Z\",\"views\":10},{\"date\":\"2025-02-02T15:13:27.265Z\",\"views\":2},{\"date\":\"2025-01-30T03:13:27.288Z\",\"views\":2},{\"date\":\"2025-01-26T15:13:27.383Z\",\"views\":1},{\"date\":\"2025-01-23T03:13:27.420Z\",\"views\":0},{\"date\":\"2025-01-19T15:13:27.454Z\",\"views\":2},{\"date\":\"2025-01-16T03:13:27.491Z\",\"views\":2},{\"date\":\"2025-01-12T15:13:27.514Z\",\"views\":0},{\"date\":\"2025-01-09T03:13:27.540Z\",\"views\":1},{\"date\":\"2025-01-05T15:13:27.560Z\",\"views\":1},{\"date\":\"2025-01-02T03:13:27.584Z\",\"views\":2},{\"date\":\"2024-12-29T15:13:27.604Z\",\"views\":0},{\"date\":\"2024-12-26T03:13:27.637Z\",\"views\":2},{\"date\":\"2024-12-22T15:13:27.662Z\",\"views\":1},{\"date\":\"2024-12-19T03:13:27.686Z\",\"views\":0},{\"date\":\"2024-12-15T15:13:27.738Z\",\"views\":2},{\"date\":\"2024-12-12T03:13:27.759Z\",\"views\":0},{\"date\":\"2024-12-08T15:13:27.781Z\",\"views\":2},{\"date\":\"2024-12-05T03:13:27.808Z\",\"views\":1},{\"date\":\"2024-12-01T15:13:27.830Z\",\"views\":1},{\"date\":\"2024-11-28T03:13:27.851Z\",\"views\":2},{\"date\":\"2024-11-24T15:13:27.878Z\",\"views\":2},{\"date\":\"2024-11-21T03:13:27.899Z\",\"views\":0},{\"date\":\"2024-11-17T15:13:27.922Z\",\"views\":1},{\"date\":\"2024-11-14T03:13:27.944Z\",\"views\":2},{\"date\":\"2024-11-10T15:13:27.967Z\",\"views\":0},{\"date\":\"2024-11-07T03:13:27.989Z\",\"views\":0},{\"date\":\"2024-11-03T15:13:28.027Z\",\"views\":2},{\"date\":\"2024-10-31T02:13:28.052Z\",\"views\":0},{\"date\":\"2024-10-27T14:13:28.074Z\",\"views\":2},{\"date\":\"2024-10-24T02:13:28.096Z\",\"views\":2},{\"date\":\"2024-10-20T14:13:28.127Z\",\"views\":2},{\"date\":\"2024-10-17T02:13:28.151Z\",\"views\":2},{\"date\":\"2024-10-13T14:13:28.174Z\",\"views\":2},{\"date\":\"2024-10-10T02:13:28.195Z\",\"views\":0},{\"date\":\"2024-10-06T14:13:28.234Z\",\"views\":0},{\"date\":\"2024-10-03T02:13:28.269Z\",\"views\":0},{\"date\":\"2024-09-29T14:13:28.296Z\",\"views\":1},{\"date\":\"2024-09-26T02:13:28.323Z\",\"views\":1},{\"date\":\"2024-09-22T14:13:28.345Z\",\"views\":2},{\"date\":\"2024-09-19T02:13:28.365Z\",\"views\":2},{\"date\":\"2024-09-15T14:13:28.393Z\",\"views\":1},{\"date\":\"2024-09-12T02:13:28.414Z\",\"views\":0},{\"date\":\"2024-09-08T14:13:28.434Z\",\"views\":1},{\"date\":\"2024-09-05T02:13:28.455Z\",\"views\":0},{\"date\":\"2024-09-01T14:13:28.466Z\",\"views\":0},{\"date\":\"2024-08-29T02:13:28.483Z\",\"views\":0}]},\"is_hidden\":false,\"first_publication_date\":\"2020-06-11T18:04:10.000Z\",\"paperVersions\":{\"_id\":\"67a4de1ad2c41194fa0de460\",\"paper_group_id\":\"67a4de19d2c41194fa0de45e\",\"version_label\":\"v1\",\"version_order\":1,\"title\":\"End-to-end Sinkhorn Autoencoder with Noise Generator\",\"abstract\":\"$72\",\"author_ids\":[\"673223ebcd1e32a6e7efec30\",\"673b75efbf626fe16b8a7a6f\",\"67a4de1ad2c41194fa0de45f\",\"673b7f9fee7cdcdc03b15f8c\",\"6732279ecd1e32a6e7f029d8\"],\"publication_date\":\"2020-06-11T18:04:10.000Z\",\"license\":\"http://arxiv.org/licenses/nonexclusive-distrib/1.0/\",\"created_at\":\"2025-02-06T16:06:50.299Z\",\"updated_at\":\"2025-02-06T16:06:50.299Z\",\"is_deleted\":false,\"is_hidden\":false,\"universal_paper_id\":\"2006.06704\",\"imageURL\":\"image/2006.06704v1.png\"},\"verifiedAuthors\":[],\"authors\":[{\"_id\":\"673223ebcd1e32a6e7efec30\",\"full_name\":\"Kamil Deja\",\"affiliation\":null,\"orcid\":null,\"semantic_scholarid\":null,\"user_id\":null},{\"_id\":\"6732279ecd1e32a6e7f029d8\",\"full_name\":\"Tomasz Trzciński\",\"affiliation\":null,\"orcid\":null,\"semantic_scholarid\":null,\"user_id\":null},{\"_id\":\"673b75efbf626fe16b8a7a6f\",\"full_name\":\"Jan Dubiński\",\"affiliation\":null,\"orcid\":null,\"semantic_scholarid\":null,\"user_id\":null},{\"_id\":\"673b7f9fee7cdcdc03b15f8c\",\"full_name\":\"Sandro Wenzel\",\"affiliation\":null,\"orcid\":null,\"semantic_scholarid\":null,\"user_id\":null},{\"_id\":\"67a4de1ad2c41194fa0de45f\",\"full_name\":\"Piotr Nowak\",\"affiliation\":null,\"orcid\":null,\"semantic_scholarid\":null,\"user_id\":null}]},\"max_version_order\":1,\"verified_authors\":[],\"authors\":[{\"_id\":\"673223ebcd1e32a6e7efec30\",\"full_name\":\"Kamil Deja\",\"affiliation\":null,\"orcid\":null,\"semantic_scholarid\":null,\"user_id\":null},{\"_id\":\"6732279ecd1e32a6e7f029d8\",\"full_name\":\"Tomasz Trzciński\",\"affiliation\":null,\"orcid\":null,\"semantic_scholarid\":null,\"user_id\":null},{\"_id\":\"673b75efbf626fe16b8a7a6f\",\"full_name\":\"Jan Dubiński\",\"affiliation\":null,\"orcid\":null,\"semantic_scholarid\":null,\"user_id\":null},{\"_id\":\"673b7f9fee7cdcdc03b15f8c\",\"full_name\":\"Sandro Wenzel\",\"affiliation\":null,\"orcid\":null,\"semantic_scholarid\":null,\"user_id\":null},{\"_id\":\"67a4de1ad2c41194fa0de45f\",\"full_name\":\"Piotr Nowak\",\"affiliation\":null,\"orcid\":null,\"semantic_scholarid\":null,\"user_id\":null}],\"pdf_info\":{\"fetcher_url\":\"https://fetcher.alphaxiv.org/v2/pdf/2006.06704v1\"}}},\"dataUpdateCount\":1,\"dataUpdatedAt\":1744228233545,\"error\":null,\"errorUpdateCount\":0,\"errorUpdatedAt\":0,\"fetchFailureCount\":0,\"fetchFailureReason\":null,\"fetchMeta\":null,\"isInvalidated\":false,\"status\":\"success\",\"fetchStatus\":\"idle\"},\"queryKey\":[\"paper\",\"2006.06704\",\"metadata\"],\"queryHash\":\"[\\\"paper\\\",\\\"2006.06704\\\",\\\"metadata\\\"]\"},{\"state\":{\"data\":{\"data\":[]},\"dataUpdateCount\":1,\"dataUpdatedAt\":1744228233545,\"error\":null,\"errorUpdateCount\":0,\"errorUpdatedAt\":0,\"fetchFailureCount\":0,\"fetchFailureReason\":null,\"fetchMeta\":null,\"isInvalidated\":false,\"status\":\"success\",\"fetchStatus\":\"idle\"},\"queryKey\":[\"paper\",\"2006.06704\",\"comments\"],\"queryHash\":\"[\\\"paper\\\",\\\"2006.06704\\\",\\\"comments\\\"]\"},{\"state\":{\"data\":{\"data\":{\"paper_version\":{\"_id\":\"673cfbaa615941b897fb848d\",\"paper_group_id\":\"673cfba9615941b897fb848a\",\"version_label\":\"v1\",\"version_order\":1,\"title\":\"CORA: Adapting CLIP for Open-Vocabulary Detection with Region Prompting and Anchor Pre-Matching\",\"abstract\":\"$73\",\"author_ids\":[\"672bced6986a1370676ddfd0\",\"6732261fcd1e32a6e7f00ea6\",\"672bd0ec986a1370676e0c0d\",\"672bbe7c986a1370676d5797\"],\"publication_date\":\"2023-03-23T07:13:57.000Z\",\"license\":\"http://creativecommons.org/licenses/by/4.0/\",\"created_at\":\"2024-11-19T20:57:14.131Z\",\"updated_at\":\"2024-11-19T20:57:14.131Z\",\"is_deleted\":false,\"is_hidden\":false,\"universal_paper_id\":\"2303.13076\",\"imageURL\":\"image/2303.13076v1.png\"},\"paper_group\":{\"_id\":\"673cfba9615941b897fb848a\",\"universal_paper_id\":\"2303.13076\",\"source\":{\"name\":\"arXiv\",\"url\":\"https://arXiv.org/paper/2303.13076\"},\"title\":\"CORA: Adapting CLIP for Open-Vocabulary Detection with Region Prompting and Anchor Pre-Matching\",\"created_at\":\"2024-10-29T01:21:10.267Z\",\"updated_at\":\"2025-03-03T20:18:39.702Z\",\"categories\":[\"Computer Science\"],\"subcategories\":[\"cs.CV\",\"cs.AI\"],\"metrics\":{\"activity_rank\":0,\"questions_count\":0,\"responses_count\":0,\"upvotes_count\":null,\"downvotes_count\":null,\"total_votes\":0,\"visits_count\":{\"last24Hours\":0,\"last7Days\":0,\"last30Days\":7,\"last90Days\":57,\"all\":186},\"weighted_visits\":{\"last24Hours\":0,\"last7Days\":0,\"last30Days\":0.0003513965921202095,\"last90Days\":2.1026872959496576,\"hot\":0},\"public_total_votes\":9,\"timeline\":[{\"date\":\"2025-04-03T01:57:45.769Z\",\"views\":0},{\"date\":\"2025-03-30T13:57:45.769Z\",\"views\":2},{\"date\":\"2025-03-27T01:57:45.769Z\",\"views\":1},{\"date\":\"2025-03-23T13:57:45.769Z\",\"views\":4},{\"date\":\"2025-03-20T01:57:45.769Z\",\"views\":6},{\"date\":\"2025-03-16T13:57:45.769Z\",\"views\":2},{\"date\":\"2025-03-13T01:57:45.769Z\",\"views\":1},{\"date\":\"2025-03-09T13:57:45.769Z\",\"views\":14},{\"date\":\"2025-03-06T01:57:45.769Z\",\"views\":0},{\"date\":\"2025-03-02T13:57:45.769Z\",\"views\":1},{\"date\":\"2025-02-27T01:57:45.769Z\",\"views\":0},{\"date\":\"2025-02-23T13:57:45.769Z\",\"views\":2},{\"date\":\"2025-02-20T01:57:45.786Z\",\"views\":1},{\"date\":\"2025-02-16T13:57:45.801Z\",\"views\":1},{\"date\":\"2025-02-13T01:57:45.817Z\",\"views\":0},{\"date\":\"2025-02-09T13:57:45.835Z\",\"views\":5},{\"date\":\"2025-02-06T01:57:45.853Z\",\"views\":11},{\"date\":\"2025-02-02T13:57:45.887Z\",\"views\":35},{\"date\":\"2025-01-30T01:57:45.912Z\",\"views\":26},{\"date\":\"2025-01-26T13:57:45.932Z\",\"views\":26},{\"date\":\"2025-01-23T01:57:45.955Z\",\"views\":18},{\"date\":\"2025-01-19T13:57:45.976Z\",\"views\":32},{\"date\":\"2025-01-16T01:57:46.002Z\",\"views\":8},{\"date\":\"2025-01-12T13:57:46.031Z\",\"views\":0},{\"date\":\"2025-01-09T01:57:46.051Z\",\"views\":5},{\"date\":\"2025-01-05T13:57:46.075Z\",\"views\":1},{\"date\":\"2025-01-02T01:57:46.095Z\",\"views\":2},{\"date\":\"2024-12-29T13:57:46.117Z\",\"views\":2},{\"date\":\"2024-12-26T01:57:46.137Z\",\"views\":1},{\"date\":\"2024-12-22T13:57:46.163Z\",\"views\":0},{\"date\":\"2024-12-19T01:57:46.193Z\",\"views\":2},{\"date\":\"2024-12-15T13:57:46.219Z\",\"views\":4},{\"date\":\"2024-12-12T01:57:46.249Z\",\"views\":1},{\"date\":\"2024-12-08T13:57:46.272Z\",\"views\":0},{\"date\":\"2024-12-05T01:57:46.298Z\",\"views\":2},{\"date\":\"2024-12-01T13:57:46.320Z\",\"views\":1},{\"date\":\"2024-11-28T01:57:46.343Z\",\"views\":4},{\"date\":\"2024-11-24T13:57:46.371Z\",\"views\":0},{\"date\":\"2024-11-21T01:57:46.403Z\",\"views\":1},{\"date\":\"2024-11-17T13:57:46.424Z\",\"views\":4},{\"date\":\"2024-11-14T01:57:46.447Z\",\"views\":1},{\"date\":\"2024-11-10T13:57:46.467Z\",\"views\":0},{\"date\":\"2024-11-07T01:57:46.486Z\",\"views\":1},{\"date\":\"2024-11-03T13:57:46.508Z\",\"views\":2},{\"date\":\"2024-10-31T00:57:46.531Z\",\"views\":2},{\"date\":\"2024-10-27T12:57:46.563Z\",\"views\":6},{\"date\":\"2024-10-24T00:57:46.586Z\",\"views\":1},{\"date\":\"2024-10-20T12:57:46.608Z\",\"views\":2},{\"date\":\"2024-10-17T00:57:46.629Z\",\"views\":2},{\"date\":\"2024-10-13T12:57:46.688Z\",\"views\":0},{\"date\":\"2024-10-10T00:57:46.712Z\",\"views\":0},{\"date\":\"2024-10-06T12:57:46.733Z\",\"views\":0},{\"date\":\"2024-10-03T00:57:46.758Z\",\"views\":1},{\"date\":\"2024-09-29T12:57:46.810Z\",\"views\":1},{\"date\":\"2024-09-26T00:57:46.833Z\",\"views\":1},{\"date\":\"2024-09-22T12:57:46.863Z\",\"views\":0},{\"date\":\"2024-09-19T00:57:46.890Z\",\"views\":2},{\"date\":\"2024-09-15T12:57:46.915Z\",\"views\":1},{\"date\":\"2024-09-12T00:57:46.940Z\",\"views\":0},{\"date\":\"2024-09-08T12:57:46.964Z\",\"views\":2},{\"date\":\"2024-09-05T00:57:46.989Z\",\"views\":1},{\"date\":\"2024-09-01T12:57:47.016Z\",\"views\":1},{\"date\":\"2024-08-29T00:57:47.041Z\",\"views\":1}]},\"ranking\":{\"current_rank\":109974,\"previous_rank\":109613,\"activity_score\":0,\"paper_score\":0},\"is_hidden\":false,\"custom_categories\":null,\"first_publication_date\":\"2023-03-23T07:13:57.000Z\",\"author_user_ids\":[],\"organizations\":[\"67be6376aa92218ccd8b0f71\",\"67be637aaa92218ccd8b115c\",\"67be661eaa92218ccd8b614a\",\"67c50afbb0cebe70c2cdeee0\"],\"paperVersions\":{\"_id\":\"673cfbaa615941b897fb848d\",\"paper_group_id\":\"673cfba9615941b897fb848a\",\"version_label\":\"v1\",\"version_order\":1,\"title\":\"CORA: Adapting CLIP for Open-Vocabulary Detection with Region Prompting and Anchor Pre-Matching\",\"abstract\":\"$74\",\"author_ids\":[\"672bced6986a1370676ddfd0\",\"6732261fcd1e32a6e7f00ea6\",\"672bd0ec986a1370676e0c0d\",\"672bbe7c986a1370676d5797\"],\"publication_date\":\"2023-03-23T07:13:57.000Z\",\"license\":\"http://creativecommons.org/licenses/by/4.0/\",\"created_at\":\"2024-11-19T20:57:14.131Z\",\"updated_at\":\"2024-11-19T20:57:14.131Z\",\"is_deleted\":false,\"is_hidden\":false,\"universal_paper_id\":\"2303.13076\",\"imageURL\":\"image/2303.13076v1.png\"},\"verifiedAuthors\":[],\"authors\":[{\"_id\":\"672bbe7c986a1370676d5797\",\"full_name\":\"Hongsheng Li\",\"affiliation\":null,\"orcid\":null,\"semantic_scholarid\":null,\"user_id\":null},{\"_id\":\"672bced6986a1370676ddfd0\",\"full_name\":\"Xiaoshi Wu\",\"affiliation\":null,\"orcid\":null,\"semantic_scholarid\":null,\"user_id\":null},{\"_id\":\"672bd0ec986a1370676e0c0d\",\"full_name\":\"Rui Zhao\",\"affiliation\":null,\"orcid\":null,\"semantic_scholarid\":null,\"user_id\":null},{\"_id\":\"6732261fcd1e32a6e7f00ea6\",\"full_name\":\"Feng Zhu\",\"affiliation\":null,\"orcid\":null,\"semantic_scholarid\":null,\"user_id\":null}]},\"max_version_order\":1,\"verified_authors\":[],\"authors\":[{\"_id\":\"672bbe7c986a1370676d5797\",\"full_name\":\"Hongsheng Li\",\"affiliation\":null,\"orcid\":null,\"semantic_scholarid\":null,\"user_id\":null},{\"_id\":\"672bced6986a1370676ddfd0\",\"full_name\":\"Xiaoshi Wu\",\"affiliation\":null,\"orcid\":null,\"semantic_scholarid\":null,\"user_id\":null},{\"_id\":\"672bd0ec986a1370676e0c0d\",\"full_name\":\"Rui Zhao\",\"affiliation\":null,\"orcid\":null,\"semantic_scholarid\":null,\"user_id\":null},{\"_id\":\"6732261fcd1e32a6e7f00ea6\",\"full_name\":\"Feng Zhu\",\"affiliation\":null,\"orcid\":null,\"semantic_scholarid\":null,\"user_id\":null}],\"pdf_info\":{\"fetcher_url\":\"https://fetcher.alphaxiv.org/v2/pdf/2303.13076v1\"}}},\"dataUpdateCount\":1,\"dataUpdatedAt\":1744228233562,\"error\":null,\"errorUpdateCount\":0,\"errorUpdatedAt\":0,\"fetchFailureCount\":0,\"fetchFailureReason\":null,\"fetchMeta\":null,\"isInvalidated\":false,\"status\":\"success\",\"fetchStatus\":\"idle\"},\"queryKey\":[\"paper\",\"2303.13076\",\"metadata\"],\"queryHash\":\"[\\\"paper\\\",\\\"2303.13076\\\",\\\"metadata\\\"]\"},{\"state\":{\"data\":{\"data\":[]},\"dataUpdateCount\":1,\"dataUpdatedAt\":1744228233562,\"error\":null,\"errorUpdateCount\":0,\"errorUpdatedAt\":0,\"fetchFailureCount\":0,\"fetchFailureReason\":null,\"fetchMeta\":null,\"isInvalidated\":false,\"status\":\"success\",\"fetchStatus\":\"idle\"},\"queryKey\":[\"paper\",\"2303.13076\",\"comments\"],\"queryHash\":\"[\\\"paper\\\",\\\"2303.13076\\\",\\\"comments\\\"]\"},{\"state\":{\"data\":{\"data\":{\"paper_version\":{\"_id\":\"677bbeb192731567736e6e1e\",\"paper_group_id\":\"677bbeb092731567736e6e1d\",\"version_label\":\"v1\",\"version_order\":1,\"title\":\"Overcoming Beam Squint in Dual-Wideband mmWave MIMO Channel Estimation: A Bayesian Multi-Band Sparsity Approach\",\"abstract\":\"$75\",\"author_ids\":[\"672bce02986a1370676dd15f\",\"673223e3cd1e32a6e7efeba4\",\"672bcd10986a1370676dc19b\",\"673cbd918a52218f8bc93923\",\"672bc876986a1370676d7a95\"],\"publication_date\":\"2023-06-19T20:10:40.000Z\",\"license\":\"http://creativecommons.org/licenses/by-nc-sa/4.0/\",\"created_at\":\"2025-01-06T11:29:53.083Z\",\"updated_at\":\"2025-01-06T11:29:53.083Z\",\"is_deleted\":false,\"is_hidden\":false,\"universal_paper_id\":\"2306.11149\",\"imageURL\":\"image/2306.11149v1.png\"},\"paper_group\":{\"_id\":\"677bbeb092731567736e6e1d\",\"universal_paper_id\":\"2306.11149\",\"title\":\"Overcoming Beam Squint in Dual-Wideband mmWave MIMO Channel Estimation: A Bayesian Multi-Band Sparsity Approach\",\"created_at\":\"2025-01-06T11:29:52.815Z\",\"updated_at\":\"2025-03-03T20:13:54.603Z\",\"categories\":[\"Electrical Engineering and Systems Science\"],\"subcategories\":[\"eess.SP\"],\"custom_categories\":null,\"author_user_ids\":[],\"source\":{\"name\":\"alphaXiv\",\"url\":\"https://arxiv.org/paper/2306.11149\"},\"metrics\":{\"activity_rank\":0,\"questions_count\":0,\"responses_count\":0,\"upvotes_count\":0,\"downvotes_count\":0,\"total_votes\":0,\"visits_count\":{\"last24Hours\":0,\"last7Days\":0,\"last30Days\":0,\"last90Days\":0,\"all\":2},\"weighted_visits\":{\"last24Hours\":0,\"last7Days\":0,\"last30Days\":0,\"last90Days\":0,\"hot\":0},\"public_total_votes\":0,\"timeline\":[{\"date\":\"2025-04-02T02:31:22.900Z\",\"views\":2},{\"date\":\"2025-03-29T14:31:22.900Z\",\"views\":2},{\"date\":\"2025-03-26T02:31:22.900Z\",\"views\":0},{\"date\":\"2025-03-22T14:31:22.900Z\",\"views\":1},{\"date\":\"2025-03-19T02:31:22.900Z\",\"views\":1},{\"date\":\"2025-03-15T14:31:22.900Z\",\"views\":0},{\"date\":\"2025-03-12T02:31:22.900Z\",\"views\":2},{\"date\":\"2025-03-08T14:31:22.900Z\",\"views\":0},{\"date\":\"2025-03-05T02:31:22.900Z\",\"views\":0},{\"date\":\"2025-03-01T14:31:22.900Z\",\"views\":0},{\"date\":\"2025-02-26T02:31:22.900Z\",\"views\":1},{\"date\":\"2025-02-22T14:31:22.900Z\",\"views\":1},{\"date\":\"2025-02-19T02:31:22.923Z\",\"views\":1},{\"date\":\"2025-02-15T14:31:22.941Z\",\"views\":0},{\"date\":\"2025-02-12T02:31:22.970Z\",\"views\":2},{\"date\":\"2025-02-08T14:31:22.985Z\",\"views\":0},{\"date\":\"2025-02-05T02:31:23.001Z\",\"views\":1},{\"date\":\"2025-02-01T14:31:23.018Z\",\"views\":2},{\"date\":\"2025-01-29T02:31:23.036Z\",\"views\":0},{\"date\":\"2025-01-25T14:31:23.052Z\",\"views\":2},{\"date\":\"2025-01-22T02:31:23.068Z\",\"views\":1},{\"date\":\"2025-01-18T14:31:23.085Z\",\"views\":2},{\"date\":\"2025-01-15T02:31:23.102Z\",\"views\":2},{\"date\":\"2025-01-11T14:31:23.126Z\",\"views\":0},{\"date\":\"2025-01-08T02:31:23.150Z\",\"views\":0},{\"date\":\"2025-01-04T14:31:23.167Z\",\"views\":8},{\"date\":\"2025-01-01T02:31:23.183Z\",\"views\":1},{\"date\":\"2024-12-28T14:31:23.204Z\",\"views\":2},{\"date\":\"2024-12-25T02:31:23.219Z\",\"views\":2},{\"date\":\"2024-12-21T14:31:23.233Z\",\"views\":1},{\"date\":\"2024-12-18T02:31:23.251Z\",\"views\":1},{\"date\":\"2024-12-14T14:31:23.267Z\",\"views\":2},{\"date\":\"2024-12-11T02:31:23.285Z\",\"views\":1},{\"date\":\"2024-12-07T14:31:23.301Z\",\"views\":2},{\"date\":\"2024-12-04T02:31:23.316Z\",\"views\":0},{\"date\":\"2024-11-30T14:31:23.332Z\",\"views\":2},{\"date\":\"2024-11-27T02:31:23.350Z\",\"views\":2},{\"date\":\"2024-11-23T14:31:23.367Z\",\"views\":2},{\"date\":\"2024-11-20T02:31:23.382Z\",\"views\":2},{\"date\":\"2024-11-16T14:31:23.397Z\",\"views\":0},{\"date\":\"2024-11-13T02:31:23.412Z\",\"views\":2},{\"date\":\"2024-11-09T14:31:23.437Z\",\"views\":1},{\"date\":\"2024-11-06T02:31:23.455Z\",\"views\":2},{\"date\":\"2024-11-02T13:31:23.513Z\",\"views\":1},{\"date\":\"2024-10-30T01:31:23.532Z\",\"views\":0},{\"date\":\"2024-10-26T13:31:23.553Z\",\"views\":1},{\"date\":\"2024-10-23T01:31:23.567Z\",\"views\":2},{\"date\":\"2024-10-19T13:31:23.581Z\",\"views\":0},{\"date\":\"2024-10-16T01:31:23.600Z\",\"views\":0},{\"date\":\"2024-10-12T13:31:23.615Z\",\"views\":1},{\"date\":\"2024-10-09T01:31:23.630Z\",\"views\":0},{\"date\":\"2024-10-05T13:31:23.646Z\",\"views\":2},{\"date\":\"2024-10-02T01:31:23.665Z\",\"views\":0},{\"date\":\"2024-09-28T13:31:23.683Z\",\"views\":1},{\"date\":\"2024-09-25T01:31:23.700Z\",\"views\":0},{\"date\":\"2024-09-21T13:31:23.719Z\",\"views\":2},{\"date\":\"2024-09-18T01:31:23.740Z\",\"views\":1},{\"date\":\"2024-09-14T13:31:23.761Z\",\"views\":2},{\"date\":\"2024-09-11T01:31:23.778Z\",\"views\":2},{\"date\":\"2024-09-07T13:31:23.797Z\",\"views\":1},{\"date\":\"2024-09-04T01:31:23.813Z\",\"views\":2},{\"date\":\"2024-08-31T13:31:23.843Z\",\"views\":2},{\"date\":\"2024-08-28T01:31:23.873Z\",\"views\":0}]},\"is_hidden\":false,\"first_publication_date\":\"2023-06-19T20:10:40.000Z\",\"organizations\":[\"67be6379aa92218ccd8b10fe\",\"67be6376aa92218ccd8b0fa4\",\"67be6379aa92218ccd8b10c6\"],\"citation\":{\"bibtex\":\"@misc{poor2023overcomingbeamsquint,\\n title={Overcoming Beam Squint in Dual-Wideband mmWave MIMO Channel Estimation: A Bayesian Multi-Band Sparsity Approach}, \\n author={H. Vincent Poor and Ngai Wong and Le Xu and Lei Cheng and Yik-Chung Wu},\\n year={2023},\\n eprint={2306.11149},\\n archivePrefix={arXiv},\\n primaryClass={eess.SP},\\n url={https://arxiv.org/abs/2306.11149}, \\n}\"},\"paperVersions\":{\"_id\":\"677bbeb192731567736e6e1e\",\"paper_group_id\":\"677bbeb092731567736e6e1d\",\"version_label\":\"v1\",\"version_order\":1,\"title\":\"Overcoming Beam Squint in Dual-Wideband mmWave MIMO Channel Estimation: A Bayesian Multi-Band Sparsity Approach\",\"abstract\":\"$76\",\"author_ids\":[\"672bce02986a1370676dd15f\",\"673223e3cd1e32a6e7efeba4\",\"672bcd10986a1370676dc19b\",\"673cbd918a52218f8bc93923\",\"672bc876986a1370676d7a95\"],\"publication_date\":\"2023-06-19T20:10:40.000Z\",\"license\":\"http://creativecommons.org/licenses/by-nc-sa/4.0/\",\"created_at\":\"2025-01-06T11:29:53.083Z\",\"updated_at\":\"2025-01-06T11:29:53.083Z\",\"is_deleted\":false,\"is_hidden\":false,\"universal_paper_id\":\"2306.11149\",\"imageURL\":\"image/2306.11149v1.png\"},\"verifiedAuthors\":[],\"authors\":[{\"_id\":\"672bc876986a1370676d7a95\",\"full_name\":\"H. Vincent Poor\",\"affiliation\":null,\"orcid\":null,\"semantic_scholarid\":null,\"user_id\":null},{\"_id\":\"672bcd10986a1370676dc19b\",\"full_name\":\"Ngai Wong\",\"affiliation\":null,\"orcid\":null,\"semantic_scholarid\":null,\"user_id\":null},{\"_id\":\"672bce02986a1370676dd15f\",\"full_name\":\"Le Xu\",\"affiliation\":null,\"orcid\":null,\"semantic_scholarid\":null,\"user_id\":null},{\"_id\":\"673223e3cd1e32a6e7efeba4\",\"full_name\":\"Lei Cheng\",\"affiliation\":null,\"orcid\":null,\"semantic_scholarid\":null,\"user_id\":null},{\"_id\":\"673cbd918a52218f8bc93923\",\"full_name\":\"Yik-Chung Wu\",\"affiliation\":null,\"orcid\":null,\"semantic_scholarid\":null,\"user_id\":null}]},\"max_version_order\":1,\"verified_authors\":[],\"authors\":[{\"_id\":\"672bc876986a1370676d7a95\",\"full_name\":\"H. Vincent Poor\",\"affiliation\":null,\"orcid\":null,\"semantic_scholarid\":null,\"user_id\":null},{\"_id\":\"672bcd10986a1370676dc19b\",\"full_name\":\"Ngai Wong\",\"affiliation\":null,\"orcid\":null,\"semantic_scholarid\":null,\"user_id\":null},{\"_id\":\"672bce02986a1370676dd15f\",\"full_name\":\"Le Xu\",\"affiliation\":null,\"orcid\":null,\"semantic_scholarid\":null,\"user_id\":null},{\"_id\":\"673223e3cd1e32a6e7efeba4\",\"full_name\":\"Lei Cheng\",\"affiliation\":null,\"orcid\":null,\"semantic_scholarid\":null,\"user_id\":null},{\"_id\":\"673cbd918a52218f8bc93923\",\"full_name\":\"Yik-Chung Wu\",\"affiliation\":null,\"orcid\":null,\"semantic_scholarid\":null,\"user_id\":null}],\"pdf_info\":{\"fetcher_url\":\"https://fetcher.alphaxiv.org/v2/pdf/2306.11149v1\"}}},\"dataUpdateCount\":1,\"dataUpdatedAt\":1744228234047,\"error\":null,\"errorUpdateCount\":0,\"errorUpdatedAt\":0,\"fetchFailureCount\":0,\"fetchFailureReason\":null,\"fetchMeta\":null,\"isInvalidated\":false,\"status\":\"success\",\"fetchStatus\":\"idle\"},\"queryKey\":[\"paper\",\"2306.11149\",\"metadata\"],\"queryHash\":\"[\\\"paper\\\",\\\"2306.11149\\\",\\\"metadata\\\"]\"},{\"state\":{\"data\":{\"data\":[]},\"dataUpdateCount\":1,\"dataUpdatedAt\":1744228234047,\"error\":null,\"errorUpdateCount\":0,\"errorUpdatedAt\":0,\"fetchFailureCount\":0,\"fetchFailureReason\":null,\"fetchMeta\":null,\"isInvalidated\":false,\"status\":\"success\",\"fetchStatus\":\"idle\"},\"queryKey\":[\"paper\",\"2306.11149\",\"comments\"],\"queryHash\":\"[\\\"paper\\\",\\\"2306.11149\\\",\\\"comments\\\"]\"},{\"state\":{\"data\":{\"data\":{\"paper_version\":{\"_id\":\"67b82f0d70017eeec4c81c52\",\"paper_group_id\":\"67b82f0d70017eeec4c81c51\",\"version_label\":\"v3\",\"version_order\":3,\"title\":\"SPeC: A Soft Prompt-Based Calibration on Performance Variability of Large Language Model in Clinical Notes Summarization\",\"abstract\":\"$77\",\"author_ids\":[\"672bcf65986a1370676deb3b\",\"672bc87d986a1370676d7aff\",\"672bc87d986a1370676d7b02\",\"672bc87e986a1370676d7b04\"],\"publication_date\":\"2023-08-04T07:49:26.000Z\",\"license\":\"http://arxiv.org/licenses/nonexclusive-distrib/1.0/\",\"created_at\":\"2025-02-21T07:45:17.872Z\",\"updated_at\":\"2025-02-21T07:45:17.872Z\",\"is_deleted\":false,\"is_hidden\":false,\"universal_paper_id\":\"2303.13035\",\"imageURL\":\"image/2303.13035v3.png\"},\"paper_group\":{\"_id\":\"67b82f0d70017eeec4c81c51\",\"universal_paper_id\":\"2303.13035\",\"title\":\"SPeC: A Soft Prompt-Based Calibration on Performance Variability of Large Language Model in Clinical Notes Summarization\",\"created_at\":\"2025-02-21T07:45:17.521Z\",\"updated_at\":\"2025-03-03T20:18:39.711Z\",\"categories\":[\"Computer Science\"],\"subcategories\":[\"cs.CL\",\"cs.AI\",\"cs.LG\"],\"custom_categories\":[\"ai-for-health\",\"text-generation\",\"fine-tuning\",\"model-interpretation\"],\"author_user_ids\":[],\"source\":{\"name\":\"alphaXiv\",\"url\":\"https://arxiv.org/abs/2303.13035\"},\"metrics\":{\"activity_rank\":0,\"questions_count\":0,\"responses_count\":0,\"upvotes_count\":0,\"downvotes_count\":0,\"total_votes\":0,\"public_total_votes\":2,\"visits_count\":{\"last24Hours\":1,\"last7Days\":1,\"last30Days\":1,\"last90Days\":2,\"all\":2},\"weighted_visits\":{\"last24Hours\":1.0077403313141498e-129,\"last7Days\":3.7317019422449435e-19,\"last30Days\":0.00005013160641330362,\"last90Days\":0.07374521896001561,\"hot\":3.7317019422449435e-19},\"timeline\":[{\"date\":\"2025-04-03T01:57:45.809Z\",\"views\":4},{\"date\":\"2025-03-30T13:57:45.809Z\",\"views\":2},{\"date\":\"2025-03-27T01:57:45.809Z\",\"views\":2},{\"date\":\"2025-03-23T13:57:45.809Z\",\"views\":0},{\"date\":\"2025-03-20T01:57:45.809Z\",\"views\":0},{\"date\":\"2025-03-16T13:57:45.809Z\",\"views\":0},{\"date\":\"2025-03-13T01:57:45.809Z\",\"views\":1},{\"date\":\"2025-03-09T13:57:45.809Z\",\"views\":2},{\"date\":\"2025-03-06T01:57:45.809Z\",\"views\":2},{\"date\":\"2025-03-02T13:57:45.809Z\",\"views\":2},{\"date\":\"2025-02-27T01:57:45.809Z\",\"views\":2},{\"date\":\"2025-02-23T13:57:45.809Z\",\"views\":0},{\"date\":\"2025-02-20T01:57:45.822Z\",\"views\":5},{\"date\":\"2025-02-16T13:57:45.838Z\",\"views\":2},{\"date\":\"2025-02-13T01:57:45.859Z\",\"views\":1},{\"date\":\"2025-02-09T13:57:45.895Z\",\"views\":2},{\"date\":\"2025-02-06T01:57:45.920Z\",\"views\":0},{\"date\":\"2025-02-02T13:57:45.945Z\",\"views\":2},{\"date\":\"2025-01-30T01:57:45.971Z\",\"views\":2},{\"date\":\"2025-01-26T13:57:46.000Z\",\"views\":0},{\"date\":\"2025-01-23T01:57:46.023Z\",\"views\":0},{\"date\":\"2025-01-19T13:57:46.042Z\",\"views\":1},{\"date\":\"2025-01-16T01:57:46.065Z\",\"views\":1},{\"date\":\"2025-01-12T13:57:46.090Z\",\"views\":0},{\"date\":\"2025-01-09T01:57:46.109Z\",\"views\":0},{\"date\":\"2025-01-05T13:57:46.129Z\",\"views\":0},{\"date\":\"2025-01-02T01:57:46.150Z\",\"views\":0},{\"date\":\"2024-12-29T13:57:46.175Z\",\"views\":2},{\"date\":\"2024-12-26T01:57:46.202Z\",\"views\":2},{\"date\":\"2024-12-22T13:57:46.232Z\",\"views\":1},{\"date\":\"2024-12-19T01:57:46.260Z\",\"views\":1},{\"date\":\"2024-12-15T13:57:46.279Z\",\"views\":0},{\"date\":\"2024-12-12T01:57:46.301Z\",\"views\":1},{\"date\":\"2024-12-08T13:57:46.323Z\",\"views\":2},{\"date\":\"2024-12-05T01:57:46.349Z\",\"views\":0},{\"date\":\"2024-12-01T13:57:46.392Z\",\"views\":2},{\"date\":\"2024-11-28T01:57:46.414Z\",\"views\":0},{\"date\":\"2024-11-24T13:57:46.435Z\",\"views\":0},{\"date\":\"2024-11-21T01:57:46.455Z\",\"views\":1},{\"date\":\"2024-11-17T13:57:46.477Z\",\"views\":0},{\"date\":\"2024-11-14T01:57:46.497Z\",\"views\":1},{\"date\":\"2024-11-10T13:57:46.519Z\",\"views\":1},{\"date\":\"2024-11-07T01:57:46.543Z\",\"views\":2},{\"date\":\"2024-11-03T13:57:46.576Z\",\"views\":1},{\"date\":\"2024-10-31T00:57:46.600Z\",\"views\":2},{\"date\":\"2024-10-27T12:57:46.622Z\",\"views\":2},{\"date\":\"2024-10-24T00:57:46.681Z\",\"views\":2},{\"date\":\"2024-10-20T12:57:46.709Z\",\"views\":2},{\"date\":\"2024-10-17T00:57:46.730Z\",\"views\":2},{\"date\":\"2024-10-13T12:57:46.758Z\",\"views\":2},{\"date\":\"2024-10-10T00:57:46.792Z\",\"views\":1},{\"date\":\"2024-10-06T12:57:46.818Z\",\"views\":2},{\"date\":\"2024-10-03T00:57:46.873Z\",\"views\":2},{\"date\":\"2024-09-29T12:57:46.893Z\",\"views\":1},{\"date\":\"2024-09-26T00:57:46.914Z\",\"views\":1},{\"date\":\"2024-09-22T12:57:46.939Z\",\"views\":1},{\"date\":\"2024-09-19T00:57:46.963Z\",\"views\":1},{\"date\":\"2024-09-15T12:57:46.986Z\",\"views\":1},{\"date\":\"2024-09-12T00:57:47.010Z\",\"views\":0},{\"date\":\"2024-09-08T12:57:47.034Z\",\"views\":1},{\"date\":\"2024-09-05T00:57:47.054Z\",\"views\":1},{\"date\":\"2024-09-01T12:57:47.073Z\",\"views\":1},{\"date\":\"2024-08-29T00:57:47.088Z\",\"views\":0}]},\"is_hidden\":false,\"first_publication_date\":\"2023-03-23T04:47:46.000Z\",\"organizations\":[\"67be637caa92218ccd8b11f6\",\"67be6509aa92218ccd8b48f2\"],\"paperVersions\":{\"_id\":\"67b82f0d70017eeec4c81c52\",\"paper_group_id\":\"67b82f0d70017eeec4c81c51\",\"version_label\":\"v3\",\"version_order\":3,\"title\":\"SPeC: A Soft Prompt-Based Calibration on Performance Variability of Large Language Model in Clinical Notes Summarization\",\"abstract\":\"$78\",\"author_ids\":[\"672bcf65986a1370676deb3b\",\"672bc87d986a1370676d7aff\",\"672bc87d986a1370676d7b02\",\"672bc87e986a1370676d7b04\"],\"publication_date\":\"2023-08-04T07:49:26.000Z\",\"license\":\"http://arxiv.org/licenses/nonexclusive-distrib/1.0/\",\"created_at\":\"2025-02-21T07:45:17.872Z\",\"updated_at\":\"2025-02-21T07:45:17.872Z\",\"is_deleted\":false,\"is_hidden\":false,\"universal_paper_id\":\"2303.13035\",\"imageURL\":\"image/2303.13035v3.png\"},\"verifiedAuthors\":[],\"authors\":[{\"_id\":\"672bc87d986a1370676d7aff\",\"full_name\":\"Ruixiang Tang\",\"affiliation\":null,\"orcid\":null,\"semantic_scholarid\":null,\"user_id\":null},{\"_id\":\"672bc87d986a1370676d7b02\",\"full_name\":\"Xiaoqian Jiang\",\"affiliation\":null,\"orcid\":null,\"semantic_scholarid\":null,\"user_id\":null},{\"_id\":\"672bc87e986a1370676d7b04\",\"full_name\":\"Xia Hu\",\"affiliation\":null,\"orcid\":null,\"semantic_scholarid\":null,\"user_id\":null},{\"_id\":\"672bcf65986a1370676deb3b\",\"full_name\":\"Yu-Neng Chuang\",\"affiliation\":null,\"orcid\":null,\"semantic_scholarid\":null,\"user_id\":null}]},\"max_version_order\":3,\"verified_authors\":[],\"authors\":[{\"_id\":\"672bc87d986a1370676d7aff\",\"full_name\":\"Ruixiang Tang\",\"affiliation\":null,\"orcid\":null,\"semantic_scholarid\":null,\"user_id\":null},{\"_id\":\"672bc87d986a1370676d7b02\",\"full_name\":\"Xiaoqian Jiang\",\"affiliation\":null,\"orcid\":null,\"semantic_scholarid\":null,\"user_id\":null},{\"_id\":\"672bc87e986a1370676d7b04\",\"full_name\":\"Xia Hu\",\"affiliation\":null,\"orcid\":null,\"semantic_scholarid\":null,\"user_id\":null},{\"_id\":\"672bcf65986a1370676deb3b\",\"full_name\":\"Yu-Neng Chuang\",\"affiliation\":null,\"orcid\":null,\"semantic_scholarid\":null,\"user_id\":null}],\"pdf_info\":{\"fetcher_url\":\"https://fetcher.alphaxiv.org/v2/pdf/2303.13035v3\"}}},\"dataUpdateCount\":1,\"dataUpdatedAt\":1744228234512,\"error\":null,\"errorUpdateCount\":0,\"errorUpdatedAt\":0,\"fetchFailureCount\":0,\"fetchFailureReason\":null,\"fetchMeta\":null,\"isInvalidated\":false,\"status\":\"success\",\"fetchStatus\":\"idle\"},\"queryKey\":[\"paper\",\"2303.13035\",\"metadata\"],\"queryHash\":\"[\\\"paper\\\",\\\"2303.13035\\\",\\\"metadata\\\"]\"},{\"state\":{\"data\":{\"data\":[]},\"dataUpdateCount\":1,\"dataUpdatedAt\":1744228234512,\"error\":null,\"errorUpdateCount\":0,\"errorUpdatedAt\":0,\"fetchFailureCount\":0,\"fetchFailureReason\":null,\"fetchMeta\":null,\"isInvalidated\":false,\"status\":\"success\",\"fetchStatus\":\"idle\"},\"queryKey\":[\"paper\",\"2303.13035\",\"comments\"],\"queryHash\":\"[\\\"paper\\\",\\\"2303.13035\\\",\\\"comments\\\"]\"},{\"state\":{\"data\":{\"data\":{\"paper_version\":{\"_id\":\"674deccbe57dd4be770d8e7f\",\"paper_group_id\":\"674deccae57dd4be770d8e7c\",\"version_label\":\"v1\",\"version_order\":1,\"title\":\"Explaining Exchange Rate Forecasts with Macroeconomic Fundamentals Using Interpretive Machine Learning\",\"abstract\":\"$79\",\"author_ids\":[\"674deccae57dd4be770d8e7d\",\"673bac6eee7cdcdc03b19b22\",\"674deccbe57dd4be770d8e7e\"],\"publication_date\":\"2023-03-23T04:40:23.000Z\",\"license\":\"http://arxiv.org/licenses/nonexclusive-distrib/1.0/\",\"created_at\":\"2024-12-02T17:22:19.158Z\",\"updated_at\":\"2024-12-02T17:22:19.158Z\",\"is_deleted\":false,\"is_hidden\":false,\"universal_paper_id\":\"2303.16149\",\"imageURL\":\"image/2303.16149v1.png\"},\"paper_group\":{\"_id\":\"674deccae57dd4be770d8e7c\",\"universal_paper_id\":\"2303.16149\",\"source\":{\"name\":\"arXiv\",\"url\":\"https://arXiv.org/paper/2303.16149\"},\"title\":\"Explaining Exchange Rate Forecasts with Macroeconomic Fundamentals Using Interpretive Machine Learning\",\"created_at\":\"2024-12-02T10:55:53.706Z\",\"updated_at\":\"2025-03-03T20:18:39.712Z\",\"categories\":[\"Quantitative Finance\",\"Computer Science\"],\"subcategories\":[\"q-fin.ST\",\"cs.LG\"],\"custom_categories\":null,\"metrics\":{\"activity_rank\":0,\"questions_count\":0,\"responses_count\":0,\"upvotes_count\":null,\"downvotes_count\":null,\"total_votes\":0,\"visits_count\":{\"last24Hours\":0,\"last7Days\":11,\"last30Days\":24,\"last90Days\":25,\"all\":78},\"weighted_visits\":{\"last24Hours\":0,\"last7Days\":4.103669020589887e-18,\"last30Days\":0.0012030762621739388,\"last90Days\":0.9217942202317269,\"hot\":4.103669020589887e-18},\"public_total_votes\":0,\"timeline\":[{\"date\":\"2025-04-03T01:57:45.823Z\",\"views\":35},{\"date\":\"2025-03-30T13:57:45.823Z\",\"views\":1},{\"date\":\"2025-03-27T01:57:45.823Z\",\"views\":1},{\"date\":\"2025-03-23T13:57:45.823Z\",\"views\":4},{\"date\":\"2025-03-20T01:57:45.823Z\",\"views\":13},{\"date\":\"2025-03-16T13:57:45.823Z\",\"views\":7},{\"date\":\"2025-03-13T01:57:45.823Z\",\"views\":18},{\"date\":\"2025-03-09T13:57:45.823Z\",\"views\":1},{\"date\":\"2025-03-06T01:57:45.823Z\",\"views\":1},{\"date\":\"2025-03-02T13:57:45.823Z\",\"views\":2},{\"date\":\"2025-02-27T01:57:45.823Z\",\"views\":1},{\"date\":\"2025-02-23T13:57:45.823Z\",\"views\":0},{\"date\":\"2025-02-20T01:57:45.841Z\",\"views\":0},{\"date\":\"2025-02-16T13:57:45.877Z\",\"views\":1},{\"date\":\"2025-02-13T01:57:45.906Z\",\"views\":2},{\"date\":\"2025-02-09T13:57:45.932Z\",\"views\":0},{\"date\":\"2025-02-06T01:57:45.955Z\",\"views\":0},{\"date\":\"2025-02-02T13:57:45.980Z\",\"views\":1},{\"date\":\"2025-01-30T01:57:46.002Z\",\"views\":1},{\"date\":\"2025-01-26T13:57:46.032Z\",\"views\":1},{\"date\":\"2025-01-23T01:57:46.054Z\",\"views\":2},{\"date\":\"2025-01-19T13:57:46.078Z\",\"views\":0},{\"date\":\"2025-01-16T01:57:46.096Z\",\"views\":2},{\"date\":\"2025-01-12T13:57:46.116Z\",\"views\":1},{\"date\":\"2025-01-09T01:57:46.136Z\",\"views\":2},{\"date\":\"2025-01-05T13:57:46.163Z\",\"views\":5},{\"date\":\"2025-01-02T01:57:46.184Z\",\"views\":1},{\"date\":\"2024-12-29T13:57:46.203Z\",\"views\":0},{\"date\":\"2024-12-26T01:57:46.233Z\",\"views\":2},{\"date\":\"2024-12-22T13:57:46.260Z\",\"views\":0},{\"date\":\"2024-12-19T01:57:46.282Z\",\"views\":0},{\"date\":\"2024-12-15T13:57:46.302Z\",\"views\":0},{\"date\":\"2024-12-12T01:57:46.320Z\",\"views\":1},{\"date\":\"2024-12-08T13:57:46.344Z\",\"views\":2},{\"date\":\"2024-12-05T01:57:46.373Z\",\"views\":1},{\"date\":\"2024-12-01T13:57:46.407Z\",\"views\":5},{\"date\":\"2024-11-28T01:57:46.433Z\",\"views\":1},{\"date\":\"2024-11-24T13:57:46.453Z\",\"views\":1},{\"date\":\"2024-11-21T01:57:46.474Z\",\"views\":2},{\"date\":\"2024-11-17T13:57:46.498Z\",\"views\":1},{\"date\":\"2024-11-14T01:57:46.520Z\",\"views\":0},{\"date\":\"2024-11-10T13:57:46.543Z\",\"views\":0},{\"date\":\"2024-11-07T01:57:46.574Z\",\"views\":0},{\"date\":\"2024-11-03T13:57:46.597Z\",\"views\":0},{\"date\":\"2024-10-31T00:57:46.619Z\",\"views\":2},{\"date\":\"2024-10-27T12:57:46.639Z\",\"views\":2},{\"date\":\"2024-10-24T00:57:46.698Z\",\"views\":2},{\"date\":\"2024-10-20T12:57:46.719Z\",\"views\":1},{\"date\":\"2024-10-17T00:57:46.741Z\",\"views\":0},{\"date\":\"2024-10-13T12:57:46.761Z\",\"views\":0},{\"date\":\"2024-10-10T00:57:46.795Z\",\"views\":0},{\"date\":\"2024-10-06T12:57:46.822Z\",\"views\":1},{\"date\":\"2024-10-03T00:57:46.877Z\",\"views\":0},{\"date\":\"2024-09-29T12:57:46.901Z\",\"views\":1},{\"date\":\"2024-09-26T00:57:46.925Z\",\"views\":1},{\"date\":\"2024-09-22T12:57:46.949Z\",\"views\":1},{\"date\":\"2024-09-19T00:57:46.971Z\",\"views\":0},{\"date\":\"2024-09-15T12:57:46.996Z\",\"views\":1},{\"date\":\"2024-09-12T00:57:47.018Z\",\"views\":0},{\"date\":\"2024-09-08T12:57:47.041Z\",\"views\":1},{\"date\":\"2024-09-05T00:57:47.062Z\",\"views\":0},{\"date\":\"2024-09-01T12:57:47.075Z\",\"views\":0},{\"date\":\"2024-08-29T00:57:47.089Z\",\"views\":1}]},\"ranking\":{\"current_rank\":0,\"previous_rank\":0,\"activity_score\":0,\"paper_score\":0},\"is_hidden\":false,\"first_publication_date\":\"2023-03-23T04:40:23.000Z\",\"author_user_ids\":[],\"organizations\":[\"67be6385aa92218ccd8b147f\"],\"overview\":{\"created_at\":\"2025-03-15T06:31:00.548Z\",\"text\":\"$7a\"},\"paperVersions\":{\"_id\":\"674deccbe57dd4be770d8e7f\",\"paper_group_id\":\"674deccae57dd4be770d8e7c\",\"version_label\":\"v1\",\"version_order\":1,\"title\":\"Explaining Exchange Rate Forecasts with Macroeconomic Fundamentals Using Interpretive Machine Learning\",\"abstract\":\"$7b\",\"author_ids\":[\"674deccae57dd4be770d8e7d\",\"673bac6eee7cdcdc03b19b22\",\"674deccbe57dd4be770d8e7e\"],\"publication_date\":\"2023-03-23T04:40:23.000Z\",\"license\":\"http://arxiv.org/licenses/nonexclusive-distrib/1.0/\",\"created_at\":\"2024-12-02T17:22:19.158Z\",\"updated_at\":\"2024-12-02T17:22:19.158Z\",\"is_deleted\":false,\"is_hidden\":false,\"universal_paper_id\":\"2303.16149\",\"imageURL\":\"image/2303.16149v1.png\"},\"verifiedAuthors\":[],\"authors\":[{\"_id\":\"673bac6eee7cdcdc03b19b22\",\"full_name\":\"Mucahit Cevik\",\"affiliation\":null,\"orcid\":null,\"semantic_scholarid\":null,\"user_id\":null},{\"_id\":\"674deccae57dd4be770d8e7d\",\"full_name\":\"Davood Pirayesh Neghab\",\"affiliation\":null,\"orcid\":null,\"semantic_scholarid\":null,\"user_id\":null},{\"_id\":\"674deccbe57dd4be770d8e7e\",\"full_name\":\"M. I. M. Wahab\",\"affiliation\":null,\"orcid\":null,\"semantic_scholarid\":null,\"user_id\":null}]},\"max_version_order\":1,\"verified_authors\":[],\"authors\":[{\"_id\":\"673bac6eee7cdcdc03b19b22\",\"full_name\":\"Mucahit Cevik\",\"affiliation\":null,\"orcid\":null,\"semantic_scholarid\":null,\"user_id\":null},{\"_id\":\"674deccae57dd4be770d8e7d\",\"full_name\":\"Davood Pirayesh Neghab\",\"affiliation\":null,\"orcid\":null,\"semantic_scholarid\":null,\"user_id\":null},{\"_id\":\"674deccbe57dd4be770d8e7e\",\"full_name\":\"M. I. M. Wahab\",\"affiliation\":null,\"orcid\":null,\"semantic_scholarid\":null,\"user_id\":null}],\"pdf_info\":{\"fetcher_url\":\"https://fetcher.alphaxiv.org/v2/pdf/2303.16149v1\"}}},\"dataUpdateCount\":1,\"dataUpdatedAt\":1744228234776,\"error\":null,\"errorUpdateCount\":0,\"errorUpdatedAt\":0,\"fetchFailureCount\":0,\"fetchFailureReason\":null,\"fetchMeta\":null,\"isInvalidated\":false,\"status\":\"success\",\"fetchStatus\":\"idle\"},\"queryKey\":[\"paper\",\"2303.16149\",\"metadata\"],\"queryHash\":\"[\\\"paper\\\",\\\"2303.16149\\\",\\\"metadata\\\"]\"},{\"state\":{\"data\":{\"data\":[]},\"dataUpdateCount\":1,\"dataUpdatedAt\":1744228234776,\"error\":null,\"errorUpdateCount\":0,\"errorUpdatedAt\":0,\"fetchFailureCount\":0,\"fetchFailureReason\":null,\"fetchMeta\":null,\"isInvalidated\":false,\"status\":\"success\",\"fetchStatus\":\"idle\"},\"queryKey\":[\"paper\",\"2303.16149\",\"comments\"],\"queryHash\":\"[\\\"paper\\\",\\\"2303.16149\\\",\\\"comments\\\"]\"},{\"state\":{\"data\":{\"data\":{\"paper_version\":{\"_id\":\"67d2a199428acbf208416f76\",\"paper_group_id\":\"67d2a198428acbf208416f75\",\"version_label\":\"v1\",\"version_order\":1,\"title\":\"Enabling Design Space Exploration of DRAM Caches in Emerging Memory Systems\",\"abstract\":\"The increasing growth of applications' memory capacity and performance\\ndemands has led the CPU vendors to deploy heterogeneous memory systems either\\nwithin a single system or via disaggregation. For instance, systems like\\nIntel's Knights Landing and Sapphire Rapids can be configured to use high\\nbandwidth memory as a cache to main memory. While there is significant research\\ninvestigating the designs of DRAM caches, there has been little research\\ninvestigating DRAM caches from a full system point of view, because there is\\nnot a suitable model available to the community to accurately study largescale\\nsystems with DRAM caches at a cycle-level. In this work we describe a new\\ncycle-level DRAM cache model in the gem5 simulator which can be used for\\nheterogeneous and disaggregated systems. We believe this model enables the\\ncommunity to perform a design space exploration for future generation of memory\\nsystems supporting DRAM caches.\",\"author_ids\":[\"67bf8ca4b81c9ef9023daa46\",\"673457e193ee43749600c203\",\"673457e093ee43749600c201\"],\"publication_date\":\"2023-03-23T04:29:32.000Z\",\"license\":\"http://creativecommons.org/licenses/by/4.0/\",\"created_at\":\"2025-03-13T09:12:57.392Z\",\"updated_at\":\"2025-03-13T09:12:57.392Z\",\"is_deleted\":false,\"is_hidden\":false,\"universal_paper_id\":\"2303.13029\",\"imageURL\":\"image/2303.13029v1.png\"},\"paper_group\":{\"_id\":\"67d2a198428acbf208416f75\",\"universal_paper_id\":\"2303.13029\",\"title\":\"Enabling Design Space Exploration of DRAM Caches in Emerging Memory Systems\",\"created_at\":\"2025-03-13T09:12:56.743Z\",\"updated_at\":\"2025-03-13T09:12:56.743Z\",\"categories\":[\"Computer Science\"],\"subcategories\":[\"cs.AR\",\"cs.PF\"],\"custom_categories\":null,\"author_user_ids\":[],\"source\":{\"name\":\"alphaXiv\",\"url\":\"https://arxiv.org/abs/2303.13029\"},\"metrics\":{\"activity_rank\":0,\"questions_count\":0,\"responses_count\":0,\"upvotes_count\":0,\"downvotes_count\":0,\"total_votes\":0,\"public_total_votes\":0,\"visits_count\":{\"last24Hours\":0,\"last7Days\":1,\"last30Days\":5,\"last90Days\":5,\"all\":5},\"timeline\":[{\"date\":\"2025-03-31T02:45:27.364Z\",\"views\":3},{\"date\":\"2025-03-27T14:45:27.364Z\",\"views\":0},{\"date\":\"2025-03-24T02:45:27.364Z\",\"views\":2},{\"date\":\"2025-03-20T14:45:27.364Z\",\"views\":0},{\"date\":\"2025-03-17T02:45:27.364Z\",\"views\":4},{\"date\":\"2025-03-13T14:45:27.364Z\",\"views\":6},{\"date\":\"2025-03-10T02:45:27.364Z\",\"views\":5},{\"date\":\"2025-03-06T14:45:27.391Z\",\"views\":1},{\"date\":\"2025-03-03T02:45:27.418Z\",\"views\":2},{\"date\":\"2025-02-27T14:45:27.453Z\",\"views\":1},{\"date\":\"2025-02-24T02:45:27.482Z\",\"views\":0},{\"date\":\"2025-02-20T14:45:27.508Z\",\"views\":2},{\"date\":\"2025-02-17T02:45:27.554Z\",\"views\":2},{\"date\":\"2025-02-13T14:45:27.582Z\",\"views\":0},{\"date\":\"2025-02-10T02:45:27.714Z\",\"views\":0},{\"date\":\"2025-02-06T14:45:27.738Z\",\"views\":0},{\"date\":\"2025-02-03T02:45:27.844Z\",\"views\":1},{\"date\":\"2025-01-30T14:45:27.869Z\",\"views\":0},{\"date\":\"2025-01-27T02:45:27.960Z\",\"views\":1},{\"date\":\"2025-01-23T14:45:27.987Z\",\"views\":2},{\"date\":\"2025-01-20T02:45:28.014Z\",\"views\":1},{\"date\":\"2025-01-16T14:45:28.041Z\",\"views\":0},{\"date\":\"2025-01-13T02:45:28.068Z\",\"views\":0},{\"date\":\"2025-01-09T14:45:28.101Z\",\"views\":2},{\"date\":\"2025-01-06T02:45:28.127Z\",\"views\":0},{\"date\":\"2025-01-02T14:45:28.188Z\",\"views\":1},{\"date\":\"2024-12-30T02:45:28.214Z\",\"views\":0},{\"date\":\"2024-12-26T14:45:28.266Z\",\"views\":0},{\"date\":\"2024-12-23T02:45:28.293Z\",\"views\":0},{\"date\":\"2024-12-19T14:45:28.358Z\",\"views\":2},{\"date\":\"2024-12-16T02:45:28.423Z\",\"views\":2},{\"date\":\"2024-12-12T14:45:28.448Z\",\"views\":2},{\"date\":\"2024-12-09T02:45:28.474Z\",\"views\":0},{\"date\":\"2024-12-05T14:45:28.503Z\",\"views\":1},{\"date\":\"2024-12-02T02:45:28.526Z\",\"views\":0},{\"date\":\"2024-11-28T14:45:28.572Z\",\"views\":1},{\"date\":\"2024-11-25T02:45:28.598Z\",\"views\":0},{\"date\":\"2024-11-21T14:45:28.622Z\",\"views\":1},{\"date\":\"2024-11-18T02:45:28.646Z\",\"views\":0},{\"date\":\"2024-11-14T14:45:28.692Z\",\"views\":0},{\"date\":\"2024-11-11T02:45:28.722Z\",\"views\":0},{\"date\":\"2024-11-07T14:45:28.753Z\",\"views\":1},{\"date\":\"2024-11-04T02:45:28.780Z\",\"views\":0},{\"date\":\"2024-10-31T14:45:28.808Z\",\"views\":2},{\"date\":\"2024-10-28T02:45:28.834Z\",\"views\":1},{\"date\":\"2024-10-24T14:45:28.860Z\",\"views\":1},{\"date\":\"2024-10-21T02:45:28.887Z\",\"views\":1},{\"date\":\"2024-10-17T14:45:28.934Z\",\"views\":0},{\"date\":\"2024-10-14T02:45:28.960Z\",\"views\":2},{\"date\":\"2024-10-10T14:45:28.986Z\",\"views\":2},{\"date\":\"2024-10-07T02:45:29.014Z\",\"views\":1},{\"date\":\"2024-10-03T14:45:29.041Z\",\"views\":1},{\"date\":\"2024-09-30T02:45:29.121Z\",\"views\":1},{\"date\":\"2024-09-26T14:45:29.158Z\",\"views\":0},{\"date\":\"2024-09-23T02:45:29.205Z\",\"views\":1},{\"date\":\"2024-09-19T14:45:29.282Z\",\"views\":1},{\"date\":\"2024-09-16T02:45:29.309Z\",\"views\":2},{\"date\":\"2024-09-12T14:45:29.334Z\",\"views\":1}],\"weighted_visits\":{\"last24Hours\":0,\"last7Days\":3.729002460163097e-19,\"last30Days\":0.000250615711411969,\"last90Days\":0.18435267097346567,\"hot\":3.729002460163097e-19}},\"is_hidden\":false,\"first_publication_date\":\"2023-03-23T04:29:32.000Z\",\"organizations\":[\"67be637caa92218ccd8b11ec\"],\"paperVersions\":{\"_id\":\"67d2a199428acbf208416f76\",\"paper_group_id\":\"67d2a198428acbf208416f75\",\"version_label\":\"v1\",\"version_order\":1,\"title\":\"Enabling Design Space Exploration of DRAM Caches in Emerging Memory Systems\",\"abstract\":\"The increasing growth of applications' memory capacity and performance\\ndemands has led the CPU vendors to deploy heterogeneous memory systems either\\nwithin a single system or via disaggregation. For instance, systems like\\nIntel's Knights Landing and Sapphire Rapids can be configured to use high\\nbandwidth memory as a cache to main memory. While there is significant research\\ninvestigating the designs of DRAM caches, there has been little research\\ninvestigating DRAM caches from a full system point of view, because there is\\nnot a suitable model available to the community to accurately study largescale\\nsystems with DRAM caches at a cycle-level. In this work we describe a new\\ncycle-level DRAM cache model in the gem5 simulator which can be used for\\nheterogeneous and disaggregated systems. We believe this model enables the\\ncommunity to perform a design space exploration for future generation of memory\\nsystems supporting DRAM caches.\",\"author_ids\":[\"67bf8ca4b81c9ef9023daa46\",\"673457e193ee43749600c203\",\"673457e093ee43749600c201\"],\"publication_date\":\"2023-03-23T04:29:32.000Z\",\"license\":\"http://creativecommons.org/licenses/by/4.0/\",\"created_at\":\"2025-03-13T09:12:57.392Z\",\"updated_at\":\"2025-03-13T09:12:57.392Z\",\"is_deleted\":false,\"is_hidden\":false,\"universal_paper_id\":\"2303.13029\",\"imageURL\":\"image/2303.13029v1.png\"},\"verifiedAuthors\":[],\"authors\":[{\"_id\":\"673457e093ee43749600c201\",\"full_name\":\"Jason Lowe-Power\",\"affiliation\":null,\"orcid\":null,\"semantic_scholarid\":null,\"user_id\":null},{\"_id\":\"673457e193ee43749600c203\",\"full_name\":\"Ayaz Akram\",\"affiliation\":null,\"orcid\":null,\"semantic_scholarid\":null,\"user_id\":null},{\"_id\":\"67bf8ca4b81c9ef9023daa46\",\"full_name\":\"Maryam Babaie\",\"affiliation\":null,\"orcid\":null,\"semantic_scholarid\":null,\"user_id\":null}]},\"max_version_order\":1,\"verified_authors\":[],\"authors\":[{\"_id\":\"673457e093ee43749600c201\",\"full_name\":\"Jason Lowe-Power\",\"affiliation\":null,\"orcid\":null,\"semantic_scholarid\":null,\"user_id\":null},{\"_id\":\"673457e193ee43749600c203\",\"full_name\":\"Ayaz Akram\",\"affiliation\":null,\"orcid\":null,\"semantic_scholarid\":null,\"user_id\":null},{\"_id\":\"67bf8ca4b81c9ef9023daa46\",\"full_name\":\"Maryam Babaie\",\"affiliation\":null,\"orcid\":null,\"semantic_scholarid\":null,\"user_id\":null}],\"pdf_info\":{\"fetcher_url\":\"https://fetcher.alphaxiv.org/v2/pdf/2303.13029v1\"}}},\"dataUpdateCount\":1,\"dataUpdatedAt\":1744228235604,\"error\":null,\"errorUpdateCount\":0,\"errorUpdatedAt\":0,\"fetchFailureCount\":0,\"fetchFailureReason\":null,\"fetchMeta\":null,\"isInvalidated\":false,\"status\":\"success\",\"fetchStatus\":\"idle\"},\"queryKey\":[\"paper\",\"2303.13029\",\"metadata\"],\"queryHash\":\"[\\\"paper\\\",\\\"2303.13029\\\",\\\"metadata\\\"]\"},{\"state\":{\"data\":{\"data\":[]},\"dataUpdateCount\":1,\"dataUpdatedAt\":1744228235604,\"error\":null,\"errorUpdateCount\":0,\"errorUpdatedAt\":0,\"fetchFailureCount\":0,\"fetchFailureReason\":null,\"fetchMeta\":null,\"isInvalidated\":false,\"status\":\"success\",\"fetchStatus\":\"idle\"},\"queryKey\":[\"paper\",\"2303.13029\",\"comments\"],\"queryHash\":\"[\\\"paper\\\",\\\"2303.13029\\\",\\\"comments\\\"]\"},{\"state\":{\"data\":{\"data\":{\"paper_version\":{\"_id\":\"6733f69f29b032f3570994c4\",\"paper_group_id\":\"6733f69e29b032f3570994c1\",\"version_label\":\"v2\",\"version_order\":2,\"title\":\"A negative imaginary approach to hybrid integrator-gain system control\",\"abstract\":\"In this paper, we show that a hybrid integrator-gain system (HIGS) is a\\nnonlinear negative imaginary (NNI) system. We prove that the positive feedback\\ninterconnection of a linear negative imaginary (NI) system and a HIGS is\\nasymptotically stable. We apply the HIGS to a MEMS nanopositioner, as an\\nexample of a linear NI system, in a single-input single-output framework. We\\nanalyze the stability and the performance of the closed-loop interconnection in\\nboth time and frequency domains through simulations and demonstrate the\\napplicability of HIGS as an NNI controller to a linear NI system.\",\"author_ids\":[\"67322578cd1e32a6e7f0031d\",\"6733f69e29b032f3570994c2\",\"67322578cd1e32a6e7f00324\",\"6733f69f29b032f3570994c3\"],\"publication_date\":\"2023-03-23T04:24:51.000Z\",\"license\":null,\"created_at\":\"2024-11-13T00:45:19.904Z\",\"updated_at\":\"2024-11-13T00:45:19.904Z\",\"is_deleted\":false,\"is_hidden\":false,\"universal_paper_id\":\"2209.01759\",\"imageURL\":\"image/2209.01759v2.png\"},\"paper_group\":{\"_id\":\"6733f69e29b032f3570994c1\",\"universal_paper_id\":\"2209.01759\",\"source\":{\"name\":\"alphaXiv\",\"url\":\"https://alphaxiv.org/paper/2209.01759\"},\"title\":\"A negative imaginary approach to hybrid integrator-gain system control\",\"created_at\":\"1970-01-01T00:00:00.000Z\",\"updated_at\":\"2025-03-03T20:18:39.712Z\",\"categories\":[\"Electrical Engineering and Systems Science\",\"Mathematics\"],\"subcategories\":[\"eess.SY\",\"math.OC\"],\"metrics\":{\"activity_rank\":0,\"questions_count\":0,\"responses_count\":0,\"upvotes_count\":null,\"downvotes_count\":0,\"total_votes\":0,\"visits_count\":{\"last24Hours\":0,\"last7Days\":2,\"last30Days\":2,\"last90Days\":4,\"all\":4},\"weighted_visits\":{\"last24Hours\":0,\"last7Days\":7.456619561822522e-19,\"last30Days\":0.00010024193930740085,\"last90Days\":0.14748000583660809,\"hot\":7.456619561822522e-19},\"public_total_votes\":0,\"timeline\":[{\"date\":\"2025-04-03T01:57:45.827Z\",\"views\":8},{\"date\":\"2025-03-30T13:57:45.827Z\",\"views\":2},{\"date\":\"2025-03-27T01:57:45.827Z\",\"views\":0},{\"date\":\"2025-03-23T13:57:45.827Z\",\"views\":0},{\"date\":\"2025-03-20T01:57:45.827Z\",\"views\":1},{\"date\":\"2025-03-16T13:57:45.827Z\",\"views\":1},{\"date\":\"2025-03-13T01:57:45.827Z\",\"views\":1},{\"date\":\"2025-03-09T13:57:45.827Z\",\"views\":1},{\"date\":\"2025-03-06T01:57:45.827Z\",\"views\":2},{\"date\":\"2025-03-02T13:57:45.827Z\",\"views\":0},{\"date\":\"2025-02-27T01:57:45.827Z\",\"views\":1},{\"date\":\"2025-02-23T13:57:45.827Z\",\"views\":0},{\"date\":\"2025-02-20T01:57:45.844Z\",\"views\":1},{\"date\":\"2025-02-16T13:57:45.869Z\",\"views\":1},{\"date\":\"2025-02-13T01:57:45.895Z\",\"views\":1},{\"date\":\"2025-02-09T13:57:45.922Z\",\"views\":0},{\"date\":\"2025-02-06T01:57:45.945Z\",\"views\":2},{\"date\":\"2025-02-02T13:57:45.967Z\",\"views\":0},{\"date\":\"2025-01-30T01:57:45.985Z\",\"views\":2},{\"date\":\"2025-01-26T13:57:46.013Z\",\"views\":2},{\"date\":\"2025-01-23T01:57:46.035Z\",\"views\":2},{\"date\":\"2025-01-19T13:57:46.057Z\",\"views\":5},{\"date\":\"2025-01-16T01:57:46.081Z\",\"views\":3},{\"date\":\"2025-01-12T13:57:46.101Z\",\"views\":1},{\"date\":\"2025-01-09T01:57:46.120Z\",\"views\":2},{\"date\":\"2025-01-05T13:57:46.141Z\",\"views\":0},{\"date\":\"2025-01-02T01:57:46.163Z\",\"views\":2},{\"date\":\"2024-12-29T13:57:46.190Z\",\"views\":0},{\"date\":\"2024-12-26T01:57:46.218Z\",\"views\":2},{\"date\":\"2024-12-22T13:57:46.238Z\",\"views\":1},{\"date\":\"2024-12-19T01:57:46.261Z\",\"views\":0},{\"date\":\"2024-12-15T13:57:46.285Z\",\"views\":1},{\"date\":\"2024-12-12T01:57:46.306Z\",\"views\":1},{\"date\":\"2024-12-08T13:57:46.327Z\",\"views\":1},{\"date\":\"2024-12-05T01:57:46.349Z\",\"views\":0},{\"date\":\"2024-12-01T13:57:46.393Z\",\"views\":2},{\"date\":\"2024-11-28T01:57:46.423Z\",\"views\":0},{\"date\":\"2024-11-24T13:57:46.443Z\",\"views\":1},{\"date\":\"2024-11-21T01:57:46.466Z\",\"views\":0},{\"date\":\"2024-11-17T13:57:46.487Z\",\"views\":1},{\"date\":\"2024-11-14T01:57:46.509Z\",\"views\":0},{\"date\":\"2024-11-10T13:57:46.531Z\",\"views\":2},{\"date\":\"2024-11-07T01:57:46.560Z\",\"views\":0},{\"date\":\"2024-11-03T13:57:46.586Z\",\"views\":1},{\"date\":\"2024-10-31T00:57:46.609Z\",\"views\":1},{\"date\":\"2024-10-27T12:57:46.629Z\",\"views\":1},{\"date\":\"2024-10-24T00:57:46.687Z\",\"views\":1},{\"date\":\"2024-10-20T12:57:46.708Z\",\"views\":0},{\"date\":\"2024-10-17T00:57:46.730Z\",\"views\":0},{\"date\":\"2024-10-13T12:57:46.750Z\",\"views\":1},{\"date\":\"2024-10-10T00:57:46.771Z\",\"views\":0},{\"date\":\"2024-10-06T12:57:46.803Z\",\"views\":0},{\"date\":\"2024-10-03T00:57:46.826Z\",\"views\":2},{\"date\":\"2024-09-29T12:57:46.852Z\",\"views\":0},{\"date\":\"2024-09-26T00:57:46.882Z\",\"views\":0},{\"date\":\"2024-09-22T12:57:46.905Z\",\"views\":1},{\"date\":\"2024-09-19T00:57:46.931Z\",\"views\":0},{\"date\":\"2024-09-15T12:57:46.955Z\",\"views\":0},{\"date\":\"2024-09-12T00:57:46.976Z\",\"views\":0},{\"date\":\"2024-09-08T12:57:46.999Z\",\"views\":2},{\"date\":\"2024-09-05T00:57:47.020Z\",\"views\":1},{\"date\":\"2024-09-01T12:57:47.042Z\",\"views\":2},{\"date\":\"2024-08-29T00:57:47.064Z\",\"views\":2}]},\"ranking\":{\"current_rank\":58684,\"previous_rank\":61407,\"activity_score\":0,\"paper_score\":0},\"is_hidden\":false,\"custom_categories\":null,\"first_publication_date\":\"2023-03-23T04:24:51.000Z\",\"author_user_ids\":[],\"citation\":{\"bibtex\":\"@Article{Shi2022ANI,\\n author = {Kanghong Shi and N. Nikooienejad and I. Petersen and S. Moheimani},\\n booktitle = {IEEE Conference on Decision and Control},\\n journal = {2022 IEEE 61st Conference on Decision and Control (CDC)},\\n pages = {1968-1973},\\n title = {A negative imaginary approach to hybrid integrator-gain system control},\\n year = {2022}\\n}\\n\"},\"paperVersions\":{\"_id\":\"6733f69f29b032f3570994c4\",\"paper_group_id\":\"6733f69e29b032f3570994c1\",\"version_label\":\"v2\",\"version_order\":2,\"title\":\"A negative imaginary approach to hybrid integrator-gain system control\",\"abstract\":\"In this paper, we show that a hybrid integrator-gain system (HIGS) is a\\nnonlinear negative imaginary (NNI) system. We prove that the positive feedback\\ninterconnection of a linear negative imaginary (NI) system and a HIGS is\\nasymptotically stable. We apply the HIGS to a MEMS nanopositioner, as an\\nexample of a linear NI system, in a single-input single-output framework. We\\nanalyze the stability and the performance of the closed-loop interconnection in\\nboth time and frequency domains through simulations and demonstrate the\\napplicability of HIGS as an NNI controller to a linear NI system.\",\"author_ids\":[\"67322578cd1e32a6e7f0031d\",\"6733f69e29b032f3570994c2\",\"67322578cd1e32a6e7f00324\",\"6733f69f29b032f3570994c3\"],\"publication_date\":\"2023-03-23T04:24:51.000Z\",\"license\":null,\"created_at\":\"2024-11-13T00:45:19.904Z\",\"updated_at\":\"2024-11-13T00:45:19.904Z\",\"is_deleted\":false,\"is_hidden\":false,\"universal_paper_id\":\"2209.01759\",\"imageURL\":\"image/2209.01759v2.png\"},\"verifiedAuthors\":[],\"authors\":[{\"_id\":\"67322578cd1e32a6e7f0031d\",\"full_name\":\"Kanghong Shi\",\"affiliation\":null,\"orcid\":null,\"semantic_scholarid\":null,\"user_id\":null},{\"_id\":\"67322578cd1e32a6e7f00324\",\"full_name\":\"Ian R. Petersen\",\"affiliation\":null,\"orcid\":null,\"semantic_scholarid\":null,\"user_id\":null},{\"_id\":\"6733f69e29b032f3570994c2\",\"full_name\":\"Nastaran Nikooienejad\",\"affiliation\":null,\"orcid\":null,\"semantic_scholarid\":null,\"user_id\":null},{\"_id\":\"6733f69f29b032f3570994c3\",\"full_name\":\"S. O. Reza Moheimani\",\"affiliation\":null,\"orcid\":null,\"semantic_scholarid\":null,\"user_id\":null}]},\"max_version_order\":2,\"verified_authors\":[],\"authors\":[{\"_id\":\"67322578cd1e32a6e7f0031d\",\"full_name\":\"Kanghong Shi\",\"affiliation\":null,\"orcid\":null,\"semantic_scholarid\":null,\"user_id\":null},{\"_id\":\"67322578cd1e32a6e7f00324\",\"full_name\":\"Ian R. Petersen\",\"affiliation\":null,\"orcid\":null,\"semantic_scholarid\":null,\"user_id\":null},{\"_id\":\"6733f69e29b032f3570994c2\",\"full_name\":\"Nastaran Nikooienejad\",\"affiliation\":null,\"orcid\":null,\"semantic_scholarid\":null,\"user_id\":null},{\"_id\":\"6733f69f29b032f3570994c3\",\"full_name\":\"S. O. Reza Moheimani\",\"affiliation\":null,\"orcid\":null,\"semantic_scholarid\":null,\"user_id\":null}],\"pdf_info\":{\"fetcher_url\":\"https://fetcher.alphaxiv.org/v2/pdf/2209.01759v2\"}}},\"dataUpdateCount\":1,\"dataUpdatedAt\":1744228235605,\"error\":null,\"errorUpdateCount\":0,\"errorUpdatedAt\":0,\"fetchFailureCount\":0,\"fetchFailureReason\":null,\"fetchMeta\":null,\"isInvalidated\":false,\"status\":\"success\",\"fetchStatus\":\"idle\"},\"queryKey\":[\"paper\",\"2209.01759\",\"metadata\"],\"queryHash\":\"[\\\"paper\\\",\\\"2209.01759\\\",\\\"metadata\\\"]\"},{\"state\":{\"data\":{\"data\":[]},\"dataUpdateCount\":1,\"dataUpdatedAt\":1744228235605,\"error\":null,\"errorUpdateCount\":0,\"errorUpdatedAt\":0,\"fetchFailureCount\":0,\"fetchFailureReason\":null,\"fetchMeta\":null,\"isInvalidated\":false,\"status\":\"success\",\"fetchStatus\":\"idle\"},\"queryKey\":[\"paper\",\"2209.01759\",\"comments\"],\"queryHash\":\"[\\\"paper\\\",\\\"2209.01759\\\",\\\"comments\\\"]\"},{\"state\":{\"data\":{\"data\":{\"paper_version\":{\"_id\":\"67f278a1c104c77576da2239\",\"paper_group_id\":\"67f278a0c104c77576da2238\",\"version_label\":\"v1\",\"version_order\":1,\"title\":\"Weighted Pressure and Mode Matching for Sound Field Reproduction: Theoretical and Experimental Comparisons\",\"abstract\":\"Two sound field reproduction methods, weighted pressure matching and weighted\\nmode matching, are theoretically and experimentally compared. The weighted\\npressure and mode matching are a generalization of conventional pressure and\\nmode matching, respectively. Both methods are derived by introducing a\\nweighting matrix in the pressure and mode matching. The weighting matrix in the\\nweighted pressure matching is defined on the basis of the kernel interpolation\\nof the sound field from pressure at a discrete set of control points. In the\\nweighted mode matching, the weighting matrix is defined by a regional\\nintegration of spherical wavefunctions. It is theoretically shown that the\\nweighted pressure matching is a special case of the weighted mode matching by\\ninfinite-dimensional harmonic analysis for estimating expansion coefficients\\nfrom pressure observations. The difference between the two methods are\\ndiscussed through experiments.\",\"author_ids\":[\"67530b77c54492edf5824d44\",\"67530b78c54492edf5824d46\",\"67530b79c54492edf5824d48\"],\"publication_date\":\"2023-03-23T04:26:06.000Z\",\"license\":\"http://creativecommons.org/licenses/by/4.0/\",\"created_at\":\"2025-04-06T12:50:41.284Z\",\"updated_at\":\"2025-04-06T12:50:41.284Z\",\"is_deleted\":false,\"is_hidden\":false,\"universal_paper_id\":\"2303.13027\",\"imageURL\":\"image/2303.13027v1.png\"},\"paper_group\":{\"_id\":\"67f278a0c104c77576da2238\",\"universal_paper_id\":\"2303.13027\",\"title\":\"Weighted Pressure and Mode Matching for Sound Field Reproduction: Theoretical and Experimental Comparisons\",\"created_at\":\"2025-04-06T12:50:40.588Z\",\"updated_at\":\"2025-04-06T12:50:40.588Z\",\"categories\":[\"Electrical Engineering and Systems Science\",\"Computer Science\"],\"subcategories\":[\"eess.AS\",\"cs.SD\"],\"custom_categories\":null,\"author_user_ids\":[],\"source\":{\"name\":\"alphaXiv\",\"url\":\"https://arxiv.org/abs/2303.13027\"},\"metrics\":{\"activity_rank\":0,\"questions_count\":0,\"responses_count\":0,\"upvotes_count\":0,\"downvotes_count\":0,\"total_votes\":0,\"public_total_votes\":2,\"visits_count\":{\"last24Hours\":0,\"last7Days\":1,\"last30Days\":1,\"last90Days\":1,\"all\":1},\"timeline\":[{\"date\":\"2025-04-03T06:20:31.887Z\",\"views\":3},{\"date\":\"2025-03-30T18:20:31.888Z\",\"views\":1},{\"date\":\"2025-03-27T06:20:31.889Z\",\"views\":0},{\"date\":\"2025-03-23T18:20:31.889Z\",\"views\":1},{\"date\":\"2025-03-20T06:20:31.890Z\",\"views\":0},{\"date\":\"2025-03-16T18:20:31.890Z\",\"views\":0},{\"date\":\"2025-03-13T06:20:31.891Z\",\"views\":1},{\"date\":\"2025-03-09T18:20:31.891Z\",\"views\":1},{\"date\":\"2025-03-06T06:20:31.892Z\",\"views\":1},{\"date\":\"2025-03-02T18:20:31.892Z\",\"views\":2},{\"date\":\"2025-02-27T06:20:31.893Z\",\"views\":2},{\"date\":\"2025-02-23T18:20:31.893Z\",\"views\":1},{\"date\":\"2025-02-20T06:20:31.894Z\",\"views\":2},{\"date\":\"2025-02-16T18:20:31.894Z\",\"views\":2},{\"date\":\"2025-02-13T06:20:31.895Z\",\"views\":1},{\"date\":\"2025-02-09T18:20:31.895Z\",\"views\":0},{\"date\":\"2025-02-06T06:20:31.896Z\",\"views\":1},{\"date\":\"2025-02-02T18:20:31.896Z\",\"views\":1},{\"date\":\"2025-01-30T06:20:31.897Z\",\"views\":1},{\"date\":\"2025-01-26T18:20:31.898Z\",\"views\":2},{\"date\":\"2025-01-23T06:20:31.898Z\",\"views\":2},{\"date\":\"2025-01-19T18:20:31.899Z\",\"views\":0},{\"date\":\"2025-01-16T06:20:31.899Z\",\"views\":0},{\"date\":\"2025-01-12T18:20:31.900Z\",\"views\":1},{\"date\":\"2025-01-09T06:20:31.901Z\",\"views\":2},{\"date\":\"2025-01-05T18:20:31.901Z\",\"views\":0},{\"date\":\"2025-01-02T06:20:31.902Z\",\"views\":0},{\"date\":\"2024-12-29T18:20:31.902Z\",\"views\":1},{\"date\":\"2024-12-26T06:20:31.903Z\",\"views\":1},{\"date\":\"2024-12-22T18:20:31.903Z\",\"views\":2},{\"date\":\"2024-12-19T06:20:31.904Z\",\"views\":0},{\"date\":\"2024-12-15T18:20:31.905Z\",\"views\":2},{\"date\":\"2024-12-12T06:20:31.905Z\",\"views\":2},{\"date\":\"2024-12-08T18:20:31.906Z\",\"views\":0},{\"date\":\"2024-12-05T06:20:31.906Z\",\"views\":1},{\"date\":\"2024-12-01T18:20:31.907Z\",\"views\":2},{\"date\":\"2024-11-28T06:20:31.907Z\",\"views\":2},{\"date\":\"2024-11-24T18:20:31.908Z\",\"views\":2},{\"date\":\"2024-11-21T06:20:31.908Z\",\"views\":1},{\"date\":\"2024-11-17T18:20:31.909Z\",\"views\":2},{\"date\":\"2024-11-14T06:20:31.909Z\",\"views\":2},{\"date\":\"2024-11-10T18:20:31.910Z\",\"views\":1},{\"date\":\"2024-11-07T06:20:31.910Z\",\"views\":2},{\"date\":\"2024-11-03T18:20:31.911Z\",\"views\":2},{\"date\":\"2024-10-31T06:20:31.911Z\",\"views\":1},{\"date\":\"2024-10-27T18:20:31.912Z\",\"views\":0},{\"date\":\"2024-10-24T06:20:31.912Z\",\"views\":0},{\"date\":\"2024-10-20T18:20:31.913Z\",\"views\":1},{\"date\":\"2024-10-17T06:20:31.913Z\",\"views\":2},{\"date\":\"2024-10-13T18:20:31.914Z\",\"views\":1},{\"date\":\"2024-10-10T06:20:31.914Z\",\"views\":1},{\"date\":\"2024-10-06T18:20:31.915Z\",\"views\":2}],\"weighted_visits\":{\"last24Hours\":0,\"last7Days\":3.171160169434499e-19,\"last30Days\":0.000048263362390826096,\"last90Days\":0.03640875750090294,\"hot\":3.171160169434499e-19}},\"is_hidden\":false,\"first_publication_date\":\"2023-03-23T04:26:06.000Z\",\"organizations\":[\"67be6376aa92218ccd8b0fb7\",\"67be639aaa92218ccd8b19ef\"],\"paperVersions\":{\"_id\":\"67f278a1c104c77576da2239\",\"paper_group_id\":\"67f278a0c104c77576da2238\",\"version_label\":\"v1\",\"version_order\":1,\"title\":\"Weighted Pressure and Mode Matching for Sound Field Reproduction: Theoretical and Experimental Comparisons\",\"abstract\":\"Two sound field reproduction methods, weighted pressure matching and weighted\\nmode matching, are theoretically and experimentally compared. The weighted\\npressure and mode matching are a generalization of conventional pressure and\\nmode matching, respectively. Both methods are derived by introducing a\\nweighting matrix in the pressure and mode matching. The weighting matrix in the\\nweighted pressure matching is defined on the basis of the kernel interpolation\\nof the sound field from pressure at a discrete set of control points. In the\\nweighted mode matching, the weighting matrix is defined by a regional\\nintegration of spherical wavefunctions. It is theoretically shown that the\\nweighted pressure matching is a special case of the weighted mode matching by\\ninfinite-dimensional harmonic analysis for estimating expansion coefficients\\nfrom pressure observations. The difference between the two methods are\\ndiscussed through experiments.\",\"author_ids\":[\"67530b77c54492edf5824d44\",\"67530b78c54492edf5824d46\",\"67530b79c54492edf5824d48\"],\"publication_date\":\"2023-03-23T04:26:06.000Z\",\"license\":\"http://creativecommons.org/licenses/by/4.0/\",\"created_at\":\"2025-04-06T12:50:41.284Z\",\"updated_at\":\"2025-04-06T12:50:41.284Z\",\"is_deleted\":false,\"is_hidden\":false,\"universal_paper_id\":\"2303.13027\",\"imageURL\":\"image/2303.13027v1.png\"},\"verifiedAuthors\":[],\"authors\":[{\"_id\":\"67530b77c54492edf5824d44\",\"full_name\":\"Shoichi Koyama\",\"affiliation\":null,\"orcid\":null,\"semantic_scholarid\":null,\"user_id\":null},{\"_id\":\"67530b78c54492edf5824d46\",\"full_name\":\"Keisuke Kimura\",\"affiliation\":null,\"orcid\":null,\"semantic_scholarid\":null,\"user_id\":null},{\"_id\":\"67530b79c54492edf5824d48\",\"full_name\":\"Natsuki Ueno\",\"affiliation\":null,\"orcid\":null,\"semantic_scholarid\":null,\"user_id\":null}]},\"max_version_order\":1,\"verified_authors\":[],\"authors\":[{\"_id\":\"67530b77c54492edf5824d44\",\"full_name\":\"Shoichi Koyama\",\"affiliation\":null,\"orcid\":null,\"semantic_scholarid\":null,\"user_id\":null},{\"_id\":\"67530b78c54492edf5824d46\",\"full_name\":\"Keisuke Kimura\",\"affiliation\":null,\"orcid\":null,\"semantic_scholarid\":null,\"user_id\":null},{\"_id\":\"67530b79c54492edf5824d48\",\"full_name\":\"Natsuki Ueno\",\"affiliation\":null,\"orcid\":null,\"semantic_scholarid\":null,\"user_id\":null}],\"pdf_info\":{\"fetcher_url\":\"https://fetcher.alphaxiv.org/v2/pdf/2303.13027v1\"}}},\"dataUpdateCount\":1,\"dataUpdatedAt\":1744228235606,\"error\":null,\"errorUpdateCount\":0,\"errorUpdatedAt\":0,\"fetchFailureCount\":0,\"fetchFailureReason\":null,\"fetchMeta\":null,\"isInvalidated\":false,\"status\":\"success\",\"fetchStatus\":\"idle\"},\"queryKey\":[\"paper\",\"2303.13027\",\"metadata\"],\"queryHash\":\"[\\\"paper\\\",\\\"2303.13027\\\",\\\"metadata\\\"]\"},{\"state\":{\"data\":{\"data\":[]},\"dataUpdateCount\":1,\"dataUpdatedAt\":1744228235606,\"error\":null,\"errorUpdateCount\":0,\"errorUpdatedAt\":0,\"fetchFailureCount\":0,\"fetchFailureReason\":null,\"fetchMeta\":null,\"isInvalidated\":false,\"status\":\"success\",\"fetchStatus\":\"idle\"},\"queryKey\":[\"paper\",\"2303.13027\",\"comments\"],\"queryHash\":\"[\\\"paper\\\",\\\"2303.13027\\\",\\\"comments\\\"]\"},{\"state\":{\"data\":{\"data\":{\"paper_version\":{\"_id\":\"67dbcb0bc8de43b5b39db98f\",\"paper_group_id\":\"67dbcb0ac8de43b5b39db98e\",\"version_label\":\"v1\",\"version_order\":1,\"title\":\"A Cycle-level Unified DRAM Cache Controller Model for 3DXPoint Memory Systems in gem5\",\"abstract\":\"$7c\",\"author_ids\":[\"67bf8ca4b81c9ef9023daa46\",\"673457e193ee43749600c203\",\"673457e093ee43749600c201\"],\"publication_date\":\"2023-03-23T04:24:30.000Z\",\"license\":\"http://creativecommons.org/licenses/by/4.0/\",\"created_at\":\"2025-03-20T08:00:11.317Z\",\"updated_at\":\"2025-03-20T08:00:11.317Z\",\"is_deleted\":false,\"is_hidden\":false,\"universal_paper_id\":\"2303.13026\",\"imageURL\":\"image/2303.13026v1.png\"},\"paper_group\":{\"_id\":\"67dbcb0ac8de43b5b39db98e\",\"universal_paper_id\":\"2303.13026\",\"title\":\"A Cycle-level Unified DRAM Cache Controller Model for 3DXPoint Memory Systems in gem5\",\"created_at\":\"2025-03-20T08:00:10.545Z\",\"updated_at\":\"2025-03-20T08:00:10.545Z\",\"categories\":[\"Computer Science\"],\"subcategories\":[\"cs.AR\",\"cs.PF\"],\"custom_categories\":null,\"author_user_ids\":[],\"source\":{\"name\":\"alphaXiv\",\"url\":\"https://arxiv.org/abs/2303.13026\"},\"metrics\":{\"activity_rank\":0,\"questions_count\":0,\"responses_count\":0,\"upvotes_count\":0,\"downvotes_count\":0,\"total_votes\":0,\"public_total_votes\":48,\"visits_count\":{\"last24Hours\":0,\"last7Days\":2,\"last30Days\":617,\"last90Days\":617,\"all\":1852},\"timeline\":[{\"date\":\"2025-03-30T20:52:27.607Z\",\"views\":5},{\"date\":\"2025-03-27T08:52:27.607Z\",\"views\":0},{\"date\":\"2025-03-23T20:52:27.607Z\",\"views\":11},{\"date\":\"2025-03-20T08:52:27.607Z\",\"views\":1465},{\"date\":\"2025-03-16T20:52:27.607Z\",\"views\":374},{\"date\":\"2025-03-13T08:52:27.631Z\",\"views\":0},{\"date\":\"2025-03-09T20:52:27.676Z\",\"views\":1},{\"date\":\"2025-03-06T08:52:27.702Z\",\"views\":2},{\"date\":\"2025-03-02T20:52:27.730Z\",\"views\":1},{\"date\":\"2025-02-27T08:52:27.754Z\",\"views\":0},{\"date\":\"2025-02-23T20:52:27.778Z\",\"views\":0},{\"date\":\"2025-02-20T08:52:27.802Z\",\"views\":2},{\"date\":\"2025-02-16T20:52:27.825Z\",\"views\":0},{\"date\":\"2025-02-13T08:52:27.850Z\",\"views\":2},{\"date\":\"2025-02-09T20:52:27.874Z\",\"views\":0},{\"date\":\"2025-02-06T08:52:27.900Z\",\"views\":0},{\"date\":\"2025-02-02T20:52:27.954Z\",\"views\":2},{\"date\":\"2025-01-30T08:52:27.978Z\",\"views\":0},{\"date\":\"2025-01-26T20:52:28.002Z\",\"views\":0},{\"date\":\"2025-01-23T08:52:28.026Z\",\"views\":0},{\"date\":\"2025-01-19T20:52:28.056Z\",\"views\":1},{\"date\":\"2025-01-16T08:52:28.079Z\",\"views\":0},{\"date\":\"2025-01-12T20:52:28.107Z\",\"views\":1},{\"date\":\"2025-01-09T08:52:28.239Z\",\"views\":1},{\"date\":\"2025-01-05T20:52:28.262Z\",\"views\":1},{\"date\":\"2025-01-02T08:52:28.286Z\",\"views\":1},{\"date\":\"2024-12-29T20:52:28.311Z\",\"views\":2},{\"date\":\"2024-12-26T08:52:28.338Z\",\"views\":2},{\"date\":\"2024-12-22T20:52:28.369Z\",\"views\":0},{\"date\":\"2024-12-19T08:52:28.391Z\",\"views\":0},{\"date\":\"2024-12-15T20:52:28.415Z\",\"views\":0},{\"date\":\"2024-12-12T08:52:28.459Z\",\"views\":1},{\"date\":\"2024-12-08T20:52:28.482Z\",\"views\":0},{\"date\":\"2024-12-05T08:52:28.506Z\",\"views\":0},{\"date\":\"2024-12-01T20:52:28.530Z\",\"views\":1},{\"date\":\"2024-11-28T08:52:28.554Z\",\"views\":1},{\"date\":\"2024-11-24T20:52:28.577Z\",\"views\":1},{\"date\":\"2024-11-21T08:52:28.601Z\",\"views\":1},{\"date\":\"2024-11-17T20:52:28.624Z\",\"views\":1},{\"date\":\"2024-11-14T08:52:28.647Z\",\"views\":2},{\"date\":\"2024-11-10T20:52:28.671Z\",\"views\":1},{\"date\":\"2024-11-07T08:52:28.694Z\",\"views\":0},{\"date\":\"2024-11-03T20:52:28.718Z\",\"views\":2},{\"date\":\"2024-10-31T08:52:28.741Z\",\"views\":1},{\"date\":\"2024-10-27T20:52:28.764Z\",\"views\":1},{\"date\":\"2024-10-24T08:52:28.788Z\",\"views\":2},{\"date\":\"2024-10-20T20:52:28.813Z\",\"views\":0},{\"date\":\"2024-10-17T08:52:28.842Z\",\"views\":1},{\"date\":\"2024-10-13T20:52:28.870Z\",\"views\":2},{\"date\":\"2024-10-10T08:52:28.893Z\",\"views\":0},{\"date\":\"2024-10-06T20:52:28.918Z\",\"views\":0},{\"date\":\"2024-10-03T08:52:28.942Z\",\"views\":2},{\"date\":\"2024-09-29T20:52:28.967Z\",\"views\":1},{\"date\":\"2024-09-26T08:52:28.990Z\",\"views\":1},{\"date\":\"2024-09-22T20:52:29.044Z\",\"views\":0},{\"date\":\"2024-09-19T08:52:29.067Z\",\"views\":2}],\"weighted_visits\":{\"last24Hours\":0,\"last7Days\":7.456514553434756e-19,\"last30Days\":0.030924536659475043,\"last90Days\":22.748765983129147,\"hot\":7.456514553434756e-19}},\"is_hidden\":false,\"first_publication_date\":\"2023-03-23T04:24:30.000Z\",\"organizations\":[\"67be637caa92218ccd8b11ec\"],\"overview\":{\"created_at\":\"2025-03-20T08:01:22.315Z\",\"text\":\"$7d\"},\"paperVersions\":{\"_id\":\"67dbcb0bc8de43b5b39db98f\",\"paper_group_id\":\"67dbcb0ac8de43b5b39db98e\",\"version_label\":\"v1\",\"version_order\":1,\"title\":\"A Cycle-level Unified DRAM Cache Controller Model for 3DXPoint Memory Systems in gem5\",\"abstract\":\"$7e\",\"author_ids\":[\"67bf8ca4b81c9ef9023daa46\",\"673457e193ee43749600c203\",\"673457e093ee43749600c201\"],\"publication_date\":\"2023-03-23T04:24:30.000Z\",\"license\":\"http://creativecommons.org/licenses/by/4.0/\",\"created_at\":\"2025-03-20T08:00:11.317Z\",\"updated_at\":\"2025-03-20T08:00:11.317Z\",\"is_deleted\":false,\"is_hidden\":false,\"universal_paper_id\":\"2303.13026\",\"imageURL\":\"image/2303.13026v1.png\"},\"verifiedAuthors\":[],\"authors\":[{\"_id\":\"673457e093ee43749600c201\",\"full_name\":\"Jason Lowe-Power\",\"affiliation\":null,\"orcid\":null,\"semantic_scholarid\":null,\"user_id\":null},{\"_id\":\"673457e193ee43749600c203\",\"full_name\":\"Ayaz Akram\",\"affiliation\":null,\"orcid\":null,\"semantic_scholarid\":null,\"user_id\":null},{\"_id\":\"67bf8ca4b81c9ef9023daa46\",\"full_name\":\"Maryam Babaie\",\"affiliation\":null,\"orcid\":null,\"semantic_scholarid\":null,\"user_id\":null}]},\"max_version_order\":1,\"verified_authors\":[],\"authors\":[{\"_id\":\"673457e093ee43749600c201\",\"full_name\":\"Jason Lowe-Power\",\"affiliation\":null,\"orcid\":null,\"semantic_scholarid\":null,\"user_id\":null},{\"_id\":\"673457e193ee43749600c203\",\"full_name\":\"Ayaz Akram\",\"affiliation\":null,\"orcid\":null,\"semantic_scholarid\":null,\"user_id\":null},{\"_id\":\"67bf8ca4b81c9ef9023daa46\",\"full_name\":\"Maryam Babaie\",\"affiliation\":null,\"orcid\":null,\"semantic_scholarid\":null,\"user_id\":null}],\"pdf_info\":{\"fetcher_url\":\"https://fetcher.alphaxiv.org/v2/pdf/2303.13026v1\"}}},\"dataUpdateCount\":1,\"dataUpdatedAt\":1744228235624,\"error\":null,\"errorUpdateCount\":0,\"errorUpdatedAt\":0,\"fetchFailureCount\":0,\"fetchFailureReason\":null,\"fetchMeta\":null,\"isInvalidated\":false,\"status\":\"success\",\"fetchStatus\":\"idle\"},\"queryKey\":[\"paper\",\"2303.13026\",\"metadata\"],\"queryHash\":\"[\\\"paper\\\",\\\"2303.13026\\\",\\\"metadata\\\"]\"},{\"state\":{\"data\":{\"data\":[]},\"dataUpdateCount\":1,\"dataUpdatedAt\":1744228235624,\"error\":null,\"errorUpdateCount\":0,\"errorUpdatedAt\":0,\"fetchFailureCount\":0,\"fetchFailureReason\":null,\"fetchMeta\":null,\"isInvalidated\":false,\"status\":\"success\",\"fetchStatus\":\"idle\"},\"queryKey\":[\"paper\",\"2303.13026\",\"comments\"],\"queryHash\":\"[\\\"paper\\\",\\\"2303.13026\\\",\\\"comments\\\"]\"},{\"state\":{\"data\":{\"data\":{\"paper_version\":{\"_id\":\"67722dd4dc5b8f619c3fc640\",\"paper_group_id\":\"67722dd3dc5b8f619c3fc63f\",\"version_label\":\"v1\",\"version_order\":1,\"title\":\"Preference-Aware Constrained Multi-Objective Bayesian Optimization\",\"abstract\":\"$7f\",\"author_ids\":[\"675a536328731fef5f4cec71\",\"673ccbc38a52218f8bc95a10\",\"673cae408a52218f8bc90390\"],\"publication_date\":\"2023-03-23T04:46:49.000Z\",\"license\":\"http://arxiv.org/licenses/nonexclusive-distrib/1.0/\",\"created_at\":\"2024-12-30T05:21:24.182Z\",\"updated_at\":\"2024-12-30T05:21:24.182Z\",\"is_deleted\":false,\"is_hidden\":false,\"universal_paper_id\":\"2303.13034\",\"imageURL\":\"image/2303.13034v1.png\"},\"paper_group\":{\"_id\":\"67722dd3dc5b8f619c3fc63f\",\"universal_paper_id\":\"2303.13034\",\"title\":\"Preference-Aware Constrained Multi-Objective Bayesian Optimization\",\"created_at\":\"2024-12-30T05:21:23.544Z\",\"updated_at\":\"2025-03-03T20:18:39.711Z\",\"categories\":[\"Computer Science\",\"Mathematics\",\"Statistics\"],\"subcategories\":[\"cs.LG\",\"cs.AI\",\"math.OC\",\"stat.ML\"],\"custom_categories\":[\"bayesian-optimization\",\"industrial-automation\",\"optimization-methods\"],\"author_user_ids\":[],\"source\":{\"name\":\"alphaXiv\",\"url\":\"https://arxiv.org/paper/2303.13034\"},\"metrics\":{\"activity_rank\":0,\"questions_count\":0,\"responses_count\":0,\"upvotes_count\":0,\"downvotes_count\":0,\"total_votes\":0,\"visits_count\":{\"last24Hours\":0,\"last7Days\":0,\"last30Days\":0,\"last90Days\":2,\"all\":3},\"weighted_visits\":{\"last24Hours\":0,\"last7Days\":0,\"last30Days\":0,\"last90Days\":0.07374500207200385,\"hot\":0},\"public_total_votes\":0,\"timeline\":[{\"date\":\"2025-04-03T01:57:45.809Z\",\"views\":1},{\"date\":\"2025-03-30T13:57:45.809Z\",\"views\":1},{\"date\":\"2025-03-27T01:57:45.809Z\",\"views\":2},{\"date\":\"2025-03-23T13:57:45.809Z\",\"views\":1},{\"date\":\"2025-03-20T01:57:45.809Z\",\"views\":2},{\"date\":\"2025-03-16T13:57:45.809Z\",\"views\":1},{\"date\":\"2025-03-13T01:57:45.809Z\",\"views\":2},{\"date\":\"2025-03-09T13:57:45.809Z\",\"views\":1},{\"date\":\"2025-03-06T01:57:45.809Z\",\"views\":1},{\"date\":\"2025-03-02T13:57:45.809Z\",\"views\":1},{\"date\":\"2025-02-27T01:57:45.809Z\",\"views\":0},{\"date\":\"2025-02-23T13:57:45.809Z\",\"views\":2},{\"date\":\"2025-02-20T01:57:45.828Z\",\"views\":1},{\"date\":\"2025-02-16T13:57:45.851Z\",\"views\":1},{\"date\":\"2025-02-13T01:57:45.885Z\",\"views\":1},{\"date\":\"2025-02-09T13:57:45.905Z\",\"views\":2},{\"date\":\"2025-02-06T01:57:45.926Z\",\"views\":2},{\"date\":\"2025-02-02T13:57:45.953Z\",\"views\":1},{\"date\":\"2025-01-30T01:57:45.973Z\",\"views\":1},{\"date\":\"2025-01-26T13:57:45.994Z\",\"views\":2},{\"date\":\"2025-01-23T01:57:46.022Z\",\"views\":4},{\"date\":\"2025-01-19T13:57:46.043Z\",\"views\":5},{\"date\":\"2025-01-16T01:57:46.065Z\",\"views\":2},{\"date\":\"2025-01-12T13:57:46.086Z\",\"views\":2},{\"date\":\"2025-01-09T01:57:46.106Z\",\"views\":1},{\"date\":\"2025-01-05T13:57:46.126Z\",\"views\":1},{\"date\":\"2025-01-02T01:57:46.147Z\",\"views\":0},{\"date\":\"2024-12-29T13:57:46.174Z\",\"views\":4},{\"date\":\"2024-12-26T01:57:46.202Z\",\"views\":0},{\"date\":\"2024-12-22T13:57:46.229Z\",\"views\":2},{\"date\":\"2024-12-19T01:57:46.261Z\",\"views\":0},{\"date\":\"2024-12-15T13:57:46.283Z\",\"views\":0},{\"date\":\"2024-12-12T01:57:46.305Z\",\"views\":2},{\"date\":\"2024-12-08T13:57:46.326Z\",\"views\":1},{\"date\":\"2024-12-05T01:57:46.361Z\",\"views\":1},{\"date\":\"2024-12-01T13:57:46.393Z\",\"views\":1},{\"date\":\"2024-11-28T01:57:46.416Z\",\"views\":1},{\"date\":\"2024-11-24T13:57:46.437Z\",\"views\":2},{\"date\":\"2024-11-21T01:57:46.463Z\",\"views\":1},{\"date\":\"2024-11-17T13:57:46.486Z\",\"views\":0},{\"date\":\"2024-11-14T01:57:46.511Z\",\"views\":0},{\"date\":\"2024-11-10T13:57:46.531Z\",\"views\":2},{\"date\":\"2024-11-07T01:57:46.561Z\",\"views\":1},{\"date\":\"2024-11-03T13:57:46.581Z\",\"views\":2},{\"date\":\"2024-10-31T00:57:46.605Z\",\"views\":2},{\"date\":\"2024-10-27T12:57:46.629Z\",\"views\":0},{\"date\":\"2024-10-24T00:57:46.680Z\",\"views\":0},{\"date\":\"2024-10-20T12:57:46.706Z\",\"views\":0},{\"date\":\"2024-10-17T00:57:46.726Z\",\"views\":0},{\"date\":\"2024-10-13T12:57:46.747Z\",\"views\":2},{\"date\":\"2024-10-10T00:57:46.782Z\",\"views\":1},{\"date\":\"2024-10-06T12:57:46.811Z\",\"views\":1},{\"date\":\"2024-10-03T00:57:46.838Z\",\"views\":1},{\"date\":\"2024-09-29T12:57:46.873Z\",\"views\":0},{\"date\":\"2024-09-26T00:57:46.894Z\",\"views\":1},{\"date\":\"2024-09-22T12:57:46.924Z\",\"views\":2},{\"date\":\"2024-09-19T00:57:46.945Z\",\"views\":2},{\"date\":\"2024-09-15T12:57:46.968Z\",\"views\":0},{\"date\":\"2024-09-12T00:57:46.994Z\",\"views\":1},{\"date\":\"2024-09-08T12:57:47.022Z\",\"views\":2},{\"date\":\"2024-09-05T00:57:47.042Z\",\"views\":2},{\"date\":\"2024-09-01T12:57:47.065Z\",\"views\":0},{\"date\":\"2024-08-29T00:57:47.085Z\",\"views\":2}]},\"is_hidden\":false,\"first_publication_date\":\"2023-03-23T04:46:49.000Z\",\"resources\":{\"github\":{\"url\":\"https://github.com/Alaleh/PAC-MOO\",\"description\":\"Preference-Aware Constrained Multi-Objective Bayesian Optimization\",\"language\":\"Python\",\"stars\":7}},\"organizations\":[\"67be639eaa92218ccd8b1ac0\"],\"paperVersions\":{\"_id\":\"67722dd4dc5b8f619c3fc640\",\"paper_group_id\":\"67722dd3dc5b8f619c3fc63f\",\"version_label\":\"v1\",\"version_order\":1,\"title\":\"Preference-Aware Constrained Multi-Objective Bayesian Optimization\",\"abstract\":\"$80\",\"author_ids\":[\"675a536328731fef5f4cec71\",\"673ccbc38a52218f8bc95a10\",\"673cae408a52218f8bc90390\"],\"publication_date\":\"2023-03-23T04:46:49.000Z\",\"license\":\"http://arxiv.org/licenses/nonexclusive-distrib/1.0/\",\"created_at\":\"2024-12-30T05:21:24.182Z\",\"updated_at\":\"2024-12-30T05:21:24.182Z\",\"is_deleted\":false,\"is_hidden\":false,\"universal_paper_id\":\"2303.13034\",\"imageURL\":\"image/2303.13034v1.png\"},\"verifiedAuthors\":[],\"authors\":[{\"_id\":\"673cae408a52218f8bc90390\",\"full_name\":\"Janardhan Rao Doppa\",\"affiliation\":null,\"orcid\":null,\"semantic_scholarid\":null,\"user_id\":null},{\"_id\":\"673ccbc38a52218f8bc95a10\",\"full_name\":\"Syrine Belakaria\",\"affiliation\":null,\"orcid\":null,\"semantic_scholarid\":null,\"user_id\":null},{\"_id\":\"675a536328731fef5f4cec71\",\"full_name\":\"Alaleh Ahmadianshalchi\",\"affiliation\":null,\"orcid\":null,\"semantic_scholarid\":null,\"user_id\":null}]},\"max_version_order\":1,\"verified_authors\":[],\"authors\":[{\"_id\":\"673cae408a52218f8bc90390\",\"full_name\":\"Janardhan Rao Doppa\",\"affiliation\":null,\"orcid\":null,\"semantic_scholarid\":null,\"user_id\":null},{\"_id\":\"673ccbc38a52218f8bc95a10\",\"full_name\":\"Syrine Belakaria\",\"affiliation\":null,\"orcid\":null,\"semantic_scholarid\":null,\"user_id\":null},{\"_id\":\"675a536328731fef5f4cec71\",\"full_name\":\"Alaleh Ahmadianshalchi\",\"affiliation\":null,\"orcid\":null,\"semantic_scholarid\":null,\"user_id\":null}],\"pdf_info\":{\"fetcher_url\":\"https://fetcher.alphaxiv.org/v2/pdf/2303.13034v1\"}}},\"dataUpdateCount\":1,\"dataUpdatedAt\":1744228236209,\"error\":null,\"errorUpdateCount\":0,\"errorUpdatedAt\":0,\"fetchFailureCount\":0,\"fetchFailureReason\":null,\"fetchMeta\":null,\"isInvalidated\":false,\"status\":\"success\",\"fetchStatus\":\"idle\"},\"queryKey\":[\"paper\",\"2303.13034\",\"metadata\"],\"queryHash\":\"[\\\"paper\\\",\\\"2303.13034\\\",\\\"metadata\\\"]\"},{\"state\":{\"data\":{\"data\":[]},\"dataUpdateCount\":1,\"dataUpdatedAt\":1744228236209,\"error\":null,\"errorUpdateCount\":0,\"errorUpdatedAt\":0,\"fetchFailureCount\":0,\"fetchFailureReason\":null,\"fetchMeta\":null,\"isInvalidated\":false,\"status\":\"success\",\"fetchStatus\":\"idle\"},\"queryKey\":[\"paper\",\"2303.13034\",\"comments\"],\"queryHash\":\"[\\\"paper\\\",\\\"2303.13034\\\",\\\"comments\\\"]\"},{\"state\":{\"data\":{\"data\":{\"paper_version\":{\"_id\":\"67b6c5564a381def1559b915\",\"paper_group_id\":\"67b6c5554a381def1559b914\",\"version_label\":\"v1\",\"version_order\":1,\"title\":\"The RR Lyrae distribution in the Galactic Bulge\",\"abstract\":\"$81\",\"author_ids\":[\"675f9e4671a7a0255e97859d\"],\"publication_date\":\"2025-02-19T11:56:11.000Z\",\"license\":\"http://creativecommons.org/licenses/by/4.0/\",\"created_at\":\"2025-02-20T06:01:58.081Z\",\"updated_at\":\"2025-02-20T06:01:58.081Z\",\"is_deleted\":false,\"is_hidden\":false,\"universal_paper_id\":\"2502.13650\",\"imageURL\":\"image/2502.13650v1.png\"},\"paper_group\":{\"_id\":\"67b6c5554a381def1559b914\",\"universal_paper_id\":\"2502.13650\",\"title\":\"The RR Lyrae distribution in the Galactic Bulge\",\"created_at\":\"2025-02-20T06:01:57.792Z\",\"updated_at\":\"2025-03-03T19:35:58.269Z\",\"categories\":[\"Physics\"],\"subcategories\":[\"astro-ph.GA\"],\"custom_categories\":null,\"author_user_ids\":[],\"source\":{\"name\":\"alphaXiv\",\"url\":\"https://arxiv.org/abs/2502.13650\"},\"metrics\":{\"activity_rank\":0,\"questions_count\":0,\"responses_count\":0,\"upvotes_count\":0,\"downvotes_count\":0,\"total_votes\":0,\"public_total_votes\":0,\"visits_count\":{\"last24Hours\":0,\"last7Days\":2,\"last30Days\":3,\"last90Days\":5,\"all\":5},\"weighted_visits\":{\"last24Hours\":0,\"last7Days\":0.17031225058281968,\"last30Days\":1.6885151549557529,\"last90Days\":5,\"hot\":0.17031225058281968},\"timeline\":[{\"date\":\"2025-04-02T01:01:37.188Z\",\"views\":6},{\"date\":\"2025-03-29T13:01:37.188Z\",\"views\":2},{\"date\":\"2025-03-26T01:01:37.188Z\",\"views\":5},{\"date\":\"2025-03-22T13:01:37.188Z\",\"views\":1},{\"date\":\"2025-03-19T01:01:37.188Z\",\"views\":1},{\"date\":\"2025-03-15T13:01:37.188Z\",\"views\":1},{\"date\":\"2025-03-12T01:01:37.188Z\",\"views\":1},{\"date\":\"2025-03-08T13:01:37.188Z\",\"views\":2},{\"date\":\"2025-03-05T01:01:37.188Z\",\"views\":0},{\"date\":\"2025-03-01T13:01:37.188Z\",\"views\":2},{\"date\":\"2025-02-26T01:01:37.188Z\",\"views\":3},{\"date\":\"2025-02-22T13:01:37.188Z\",\"views\":0},{\"date\":\"2025-02-19T01:01:37.220Z\",\"views\":5},{\"date\":\"2025-02-15T13:01:37.231Z\",\"views\":0},{\"date\":\"2025-02-12T01:01:37.243Z\",\"views\":1},{\"date\":\"2025-02-08T13:01:37.256Z\",\"views\":2},{\"date\":\"2025-02-05T01:01:37.266Z\",\"views\":1},{\"date\":\"2025-02-01T13:01:37.275Z\",\"views\":1},{\"date\":\"2025-01-29T01:01:37.287Z\",\"views\":0},{\"date\":\"2025-01-25T13:01:37.303Z\",\"views\":2},{\"date\":\"2025-01-22T01:01:37.312Z\",\"views\":0},{\"date\":\"2025-01-18T13:01:37.327Z\",\"views\":0},{\"date\":\"2025-01-15T01:01:37.337Z\",\"views\":1},{\"date\":\"2025-01-11T13:01:37.347Z\",\"views\":0},{\"date\":\"2025-01-08T01:01:37.361Z\",\"views\":2},{\"date\":\"2025-01-04T13:01:37.372Z\",\"views\":0},{\"date\":\"2025-01-01T01:01:37.384Z\",\"views\":2},{\"date\":\"2024-12-28T13:01:37.396Z\",\"views\":1},{\"date\":\"2024-12-25T01:01:37.409Z\",\"views\":0},{\"date\":\"2024-12-21T13:01:37.418Z\",\"views\":0},{\"date\":\"2024-12-18T01:01:37.431Z\",\"views\":1},{\"date\":\"2024-12-14T13:01:37.443Z\",\"views\":2},{\"date\":\"2024-12-11T01:01:37.453Z\",\"views\":2},{\"date\":\"2024-12-07T13:01:37.466Z\",\"views\":1},{\"date\":\"2024-12-04T01:01:37.481Z\",\"views\":1},{\"date\":\"2024-11-30T13:01:37.495Z\",\"views\":0},{\"date\":\"2024-11-27T01:01:37.513Z\",\"views\":1},{\"date\":\"2024-11-23T13:01:37.526Z\",\"views\":1},{\"date\":\"2024-11-20T01:01:37.535Z\",\"views\":0},{\"date\":\"2024-11-16T13:01:37.548Z\",\"views\":2},{\"date\":\"2024-11-13T01:01:37.569Z\",\"views\":1},{\"date\":\"2024-11-09T13:01:37.583Z\",\"views\":2},{\"date\":\"2024-11-06T01:01:37.644Z\",\"views\":0},{\"date\":\"2024-11-02T12:01:37.653Z\",\"views\":1},{\"date\":\"2024-10-30T00:01:37.662Z\",\"views\":1},{\"date\":\"2024-10-26T12:01:37.675Z\",\"views\":2},{\"date\":\"2024-10-23T00:01:37.695Z\",\"views\":1},{\"date\":\"2024-10-19T12:01:37.705Z\",\"views\":0},{\"date\":\"2024-10-16T00:01:37.717Z\",\"views\":1},{\"date\":\"2024-10-12T12:01:37.727Z\",\"views\":0},{\"date\":\"2024-10-09T00:01:37.739Z\",\"views\":1},{\"date\":\"2024-10-05T12:01:37.749Z\",\"views\":0},{\"date\":\"2024-10-02T00:01:37.841Z\",\"views\":1},{\"date\":\"2024-09-28T12:01:37.856Z\",\"views\":0},{\"date\":\"2024-09-25T00:01:37.868Z\",\"views\":0},{\"date\":\"2024-09-21T12:01:37.881Z\",\"views\":1},{\"date\":\"2024-09-18T00:01:37.893Z\",\"views\":0},{\"date\":\"2024-09-14T12:01:37.905Z\",\"views\":1},{\"date\":\"2024-09-11T00:01:37.963Z\",\"views\":2},{\"date\":\"2024-09-07T12:01:37.977Z\",\"views\":2},{\"date\":\"2024-09-04T00:01:38.028Z\",\"views\":1},{\"date\":\"2024-08-31T12:01:38.038Z\",\"views\":0},{\"date\":\"2024-08-28T00:01:38.050Z\",\"views\":2}]},\"is_hidden\":false,\"first_publication_date\":\"2025-02-19T11:56:11.000Z\",\"organizations\":[\"67be659aaa92218ccd8b54d4\"],\"citation\":{\"bibtex\":\"@misc{capuzzo-dolcetta2025rrlyraedistribution,\\n title={The RR Lyrae distribution in the Galactic Bulge}, \\n author={Roberto Capuzzo-Dolcetta},\\n year={2025},\\n eprint={2502.13650},\\n archivePrefix={arXiv},\\n primaryClass={astro-ph.GA},\\n url={https://arxiv.org/abs/2502.13650}, \\n}\"},\"paperVersions\":{\"_id\":\"67b6c5564a381def1559b915\",\"paper_group_id\":\"67b6c5554a381def1559b914\",\"version_label\":\"v1\",\"version_order\":1,\"title\":\"The RR Lyrae distribution in the Galactic Bulge\",\"abstract\":\"$82\",\"author_ids\":[\"675f9e4671a7a0255e97859d\"],\"publication_date\":\"2025-02-19T11:56:11.000Z\",\"license\":\"http://creativecommons.org/licenses/by/4.0/\",\"created_at\":\"2025-02-20T06:01:58.081Z\",\"updated_at\":\"2025-02-20T06:01:58.081Z\",\"is_deleted\":false,\"is_hidden\":false,\"universal_paper_id\":\"2502.13650\",\"imageURL\":\"image/2502.13650v1.png\"},\"verifiedAuthors\":[],\"authors\":[{\"_id\":\"675f9e4671a7a0255e97859d\",\"full_name\":\"Roberto Capuzzo-Dolcetta\",\"affiliation\":null,\"orcid\":null,\"semantic_scholarid\":null,\"user_id\":null}]},\"max_version_order\":1,\"verified_authors\":[],\"authors\":[{\"_id\":\"675f9e4671a7a0255e97859d\",\"full_name\":\"Roberto Capuzzo-Dolcetta\",\"affiliation\":null,\"orcid\":null,\"semantic_scholarid\":null,\"user_id\":null}],\"pdf_info\":{\"fetcher_url\":\"https://fetcher.alphaxiv.org/v2/pdf/2502.13650v1\"}}},\"dataUpdateCount\":2,\"dataUpdatedAt\":1744228238452,\"error\":null,\"errorUpdateCount\":0,\"errorUpdatedAt\":0,\"fetchFailureCount\":0,\"fetchFailureReason\":null,\"fetchMeta\":null,\"isInvalidated\":false,\"status\":\"success\",\"fetchStatus\":\"idle\"},\"queryKey\":[\"paper\",\"2502.13650\",\"metadata\"],\"queryHash\":\"[\\\"paper\\\",\\\"2502.13650\\\",\\\"metadata\\\"]\"},{\"state\":{\"data\":{\"data\":[]},\"dataUpdateCount\":2,\"dataUpdatedAt\":1744228238452,\"error\":null,\"errorUpdateCount\":0,\"errorUpdatedAt\":0,\"fetchFailureCount\":0,\"fetchFailureReason\":null,\"fetchMeta\":null,\"isInvalidated\":false,\"status\":\"success\",\"fetchStatus\":\"idle\"},\"queryKey\":[\"paper\",\"2502.13650\",\"comments\"],\"queryHash\":\"[\\\"paper\\\",\\\"2502.13650\\\",\\\"comments\\\"]\"},{\"state\":{\"data\":{\"data\":{\"paper_version\":{\"_id\":\"67a94ca66d2566004e768c01\",\"paper_group_id\":\"67a94ca56d2566004e768bff\",\"version_label\":\"v1\",\"version_order\":1,\"title\":\"The Evolution of Emojis for Sharing Emotions: A Systematic Review of the HCI Literature\",\"abstract\":\"With the prevalence of instant messaging and social media platforms, emojis\\nhave become important artifacts for expressing emotions and feelings in our\\ndaily lives. We ask how HCI researchers have examined the role and evolution of\\nemojis in sharing emotions over the past 10 years. We conducted a systematic\\nliterature review of papers addressing emojis employed for emotion\\ncommunication between users. After screening more than 1,000 articles, we\\nidentified 42 articles of studies analyzing ways and systems that enable users\\nto share emotions with emojis. Two main themes described how these papers have\\n(1) improved how users select the right emoji from an increasing emoji lexicon,\\nand (2) employed emojis in new ways and digital materials to enhance\\ncommunication. We also discovered an increasingly broad scope of functionality\\nacross appearance, medium, and affordance. We discuss and offer insights into\\npotential opportunities and challenges emojis will bring for HCI research.\",\"author_ids\":[\"67a94ca66d2566004e768c00\",\"673e337fa9573df1a3236f19\"],\"publication_date\":\"2024-09-25T20:02:58.000Z\",\"license\":\"http://creativecommons.org/licenses/by/4.0/\",\"created_at\":\"2025-02-10T00:47:34.455Z\",\"updated_at\":\"2025-02-10T00:47:34.455Z\",\"is_deleted\":false,\"is_hidden\":false,\"universal_paper_id\":\"2409.17322\",\"imageURL\":\"image/2409.17322v1.png\"},\"paper_group\":{\"_id\":\"67a94ca56d2566004e768bff\",\"universal_paper_id\":\"2409.17322\",\"title\":\"The Evolution of Emojis for Sharing Emotions: A Systematic Review of the HCI Literature\",\"created_at\":\"2025-02-10T00:47:33.826Z\",\"updated_at\":\"2025-03-03T19:44:46.875Z\",\"categories\":[\"Computer Science\"],\"subcategories\":[\"cs.HC\"],\"custom_categories\":null,\"author_user_ids\":[],\"source\":{\"name\":\"alphaXiv\",\"url\":\"https://arxiv.org/abs/2409.17322\"},\"metrics\":{\"activity_rank\":0,\"questions_count\":0,\"responses_count\":0,\"upvotes_count\":0,\"downvotes_count\":0,\"total_votes\":0,\"public_total_votes\":2,\"visits_count\":{\"last24Hours\":0,\"last7Days\":3,\"last30Days\":11,\"last90Days\":12,\"all\":36},\"weighted_visits\":{\"last24Hours\":0,\"last7Days\":0.00005836193158363171,\"last30Days\":0.8753088356352161,\"last90Days\":5.161438874280165,\"hot\":0.00005836193158363171},\"timeline\":[{\"date\":\"2025-04-02T01:27:21.088Z\",\"views\":3},{\"date\":\"2025-03-29T13:27:21.088Z\",\"views\":10},{\"date\":\"2025-03-26T01:27:21.088Z\",\"views\":7},{\"date\":\"2025-03-22T13:27:21.088Z\",\"views\":1},{\"date\":\"2025-03-19T01:27:21.088Z\",\"views\":2},{\"date\":\"2025-03-15T13:27:21.088Z\",\"views\":13},{\"date\":\"2025-03-12T01:27:21.088Z\",\"views\":5},{\"date\":\"2025-03-08T13:27:21.088Z\",\"views\":1},{\"date\":\"2025-03-05T01:27:21.088Z\",\"views\":1},{\"date\":\"2025-03-01T13:27:21.088Z\",\"views\":2},{\"date\":\"2025-02-26T01:27:21.088Z\",\"views\":1},{\"date\":\"2025-02-22T13:27:21.088Z\",\"views\":2},{\"date\":\"2025-02-19T01:27:21.097Z\",\"views\":2},{\"date\":\"2025-02-15T13:27:21.147Z\",\"views\":1},{\"date\":\"2025-02-12T01:27:21.164Z\",\"views\":1},{\"date\":\"2025-02-08T13:27:21.203Z\",\"views\":4},{\"date\":\"2025-02-05T01:27:21.222Z\",\"views\":0},{\"date\":\"2025-02-01T13:27:21.240Z\",\"views\":1},{\"date\":\"2025-01-29T01:27:21.260Z\",\"views\":1},{\"date\":\"2025-01-25T13:27:21.280Z\",\"views\":2},{\"date\":\"2025-01-22T01:27:21.299Z\",\"views\":0},{\"date\":\"2025-01-18T13:27:21.313Z\",\"views\":2},{\"date\":\"2025-01-15T01:27:21.328Z\",\"views\":1},{\"date\":\"2025-01-11T13:27:21.344Z\",\"views\":2},{\"date\":\"2025-01-08T01:27:21.362Z\",\"views\":2},{\"date\":\"2025-01-04T13:27:21.387Z\",\"views\":0},{\"date\":\"2025-01-01T01:27:21.409Z\",\"views\":0},{\"date\":\"2024-12-28T13:27:21.431Z\",\"views\":0},{\"date\":\"2024-12-25T01:27:21.447Z\",\"views\":1},{\"date\":\"2024-12-21T13:27:21.468Z\",\"views\":1},{\"date\":\"2024-12-18T01:27:21.486Z\",\"views\":1},{\"date\":\"2024-12-14T13:27:21.502Z\",\"views\":2},{\"date\":\"2024-12-11T01:27:21.524Z\",\"views\":2},{\"date\":\"2024-12-07T13:27:21.540Z\",\"views\":2},{\"date\":\"2024-12-04T01:27:21.557Z\",\"views\":1},{\"date\":\"2024-11-30T13:27:21.572Z\",\"views\":1},{\"date\":\"2024-11-27T01:27:21.589Z\",\"views\":1},{\"date\":\"2024-11-23T13:27:21.608Z\",\"views\":0},{\"date\":\"2024-11-20T01:27:21.622Z\",\"views\":0},{\"date\":\"2024-11-16T13:27:21.640Z\",\"views\":0},{\"date\":\"2024-11-13T01:27:21.657Z\",\"views\":1},{\"date\":\"2024-11-09T13:27:21.675Z\",\"views\":2},{\"date\":\"2024-11-06T01:27:21.690Z\",\"views\":0},{\"date\":\"2024-11-02T12:27:21.703Z\",\"views\":2},{\"date\":\"2024-10-30T00:27:21.721Z\",\"views\":1},{\"date\":\"2024-10-26T12:27:21.738Z\",\"views\":0},{\"date\":\"2024-10-23T00:27:21.760Z\",\"views\":2},{\"date\":\"2024-10-19T12:27:21.777Z\",\"views\":0},{\"date\":\"2024-10-16T00:27:21.801Z\",\"views\":0},{\"date\":\"2024-10-12T12:27:21.823Z\",\"views\":2},{\"date\":\"2024-10-09T00:27:21.840Z\",\"views\":1},{\"date\":\"2024-10-05T12:27:21.863Z\",\"views\":2},{\"date\":\"2024-10-02T00:27:21.878Z\",\"views\":0},{\"date\":\"2024-09-28T12:27:21.899Z\",\"views\":2},{\"date\":\"2024-09-25T00:27:21.916Z\",\"views\":2}]},\"is_hidden\":false,\"first_publication_date\":\"2024-09-25T20:02:58.000Z\",\"organizations\":[\"67be6378aa92218ccd8b1049\"],\"paperVersions\":{\"_id\":\"67a94ca66d2566004e768c01\",\"paper_group_id\":\"67a94ca56d2566004e768bff\",\"version_label\":\"v1\",\"version_order\":1,\"title\":\"The Evolution of Emojis for Sharing Emotions: A Systematic Review of the HCI Literature\",\"abstract\":\"With the prevalence of instant messaging and social media platforms, emojis\\nhave become important artifacts for expressing emotions and feelings in our\\ndaily lives. We ask how HCI researchers have examined the role and evolution of\\nemojis in sharing emotions over the past 10 years. We conducted a systematic\\nliterature review of papers addressing emojis employed for emotion\\ncommunication between users. After screening more than 1,000 articles, we\\nidentified 42 articles of studies analyzing ways and systems that enable users\\nto share emotions with emojis. Two main themes described how these papers have\\n(1) improved how users select the right emoji from an increasing emoji lexicon,\\nand (2) employed emojis in new ways and digital materials to enhance\\ncommunication. We also discovered an increasingly broad scope of functionality\\nacross appearance, medium, and affordance. We discuss and offer insights into\\npotential opportunities and challenges emojis will bring for HCI research.\",\"author_ids\":[\"67a94ca66d2566004e768c00\",\"673e337fa9573df1a3236f19\"],\"publication_date\":\"2024-09-25T20:02:58.000Z\",\"license\":\"http://creativecommons.org/licenses/by/4.0/\",\"created_at\":\"2025-02-10T00:47:34.455Z\",\"updated_at\":\"2025-02-10T00:47:34.455Z\",\"is_deleted\":false,\"is_hidden\":false,\"universal_paper_id\":\"2409.17322\",\"imageURL\":\"image/2409.17322v1.png\"},\"verifiedAuthors\":[],\"authors\":[{\"_id\":\"673e337fa9573df1a3236f19\",\"full_name\":\"Diego Gomez-Zara\",\"affiliation\":null,\"orcid\":null,\"semantic_scholarid\":null,\"user_id\":null},{\"_id\":\"67a94ca66d2566004e768c00\",\"full_name\":\"Charles Chiang\",\"affiliation\":null,\"orcid\":null,\"semantic_scholarid\":null,\"user_id\":null}]},\"max_version_order\":1,\"verified_authors\":[],\"authors\":[{\"_id\":\"673e337fa9573df1a3236f19\",\"full_name\":\"Diego Gomez-Zara\",\"affiliation\":null,\"orcid\":null,\"semantic_scholarid\":null,\"user_id\":null},{\"_id\":\"67a94ca66d2566004e768c00\",\"full_name\":\"Charles Chiang\",\"affiliation\":null,\"orcid\":null,\"semantic_scholarid\":null,\"user_id\":null}],\"pdf_info\":{\"fetcher_url\":\"https://fetcher.alphaxiv.org/v2/pdf/2409.17322v1\"}}},\"dataUpdateCount\":2,\"dataUpdatedAt\":1744228238570,\"error\":null,\"errorUpdateCount\":0,\"errorUpdatedAt\":0,\"fetchFailureCount\":0,\"fetchFailureReason\":null,\"fetchMeta\":null,\"isInvalidated\":false,\"status\":\"success\",\"fetchStatus\":\"idle\"},\"queryKey\":[\"paper\",\"2409.17322\",\"metadata\"],\"queryHash\":\"[\\\"paper\\\",\\\"2409.17322\\\",\\\"metadata\\\"]\"},{\"state\":{\"data\":{\"data\":[]},\"dataUpdateCount\":2,\"dataUpdatedAt\":1744228238570,\"error\":null,\"errorUpdateCount\":0,\"errorUpdatedAt\":0,\"fetchFailureCount\":0,\"fetchFailureReason\":null,\"fetchMeta\":null,\"isInvalidated\":false,\"status\":\"success\",\"fetchStatus\":\"idle\"},\"queryKey\":[\"paper\",\"2409.17322\",\"comments\"],\"queryHash\":\"[\\\"paper\\\",\\\"2409.17322\\\",\\\"comments\\\"]\"},{\"state\":{\"data\":{\"data\":{\"paper_version\":{\"_id\":\"673d87191e502f9ec7d25bda\",\"paper_group_id\":\"673d87181e502f9ec7d25bd5\",\"version_label\":\"v1\",\"version_order\":1,\"title\":\"Securing Vehicle to Vehicle Communications using Blockchain through Visible Light and Acoustic Side-Channels\",\"abstract\":\"$83\",\"author_ids\":[\"673d87191e502f9ec7d25bd6\",\"673d87191e502f9ec7d25bd7\",\"6733d59f29b032f3570974ff\",\"673d87191e502f9ec7d25bd8\",\"673d87191e502f9ec7d25bd9\"],\"publication_date\":\"2017-04-09T01:48:57.000Z\",\"license\":\"http://arxiv.org/licenses/nonexclusive-distrib/1.0/\",\"created_at\":\"2024-11-20T06:52:09.707Z\",\"updated_at\":\"2024-11-20T06:52:09.707Z\",\"is_deleted\":false,\"is_hidden\":false,\"universal_paper_id\":\"1704.02553\",\"imageURL\":\"image/1704.02553v1.png\"},\"paper_group\":{\"_id\":\"673d87181e502f9ec7d25bd5\",\"universal_paper_id\":\"1704.02553\",\"source\":{\"name\":\"arXiv\",\"url\":\"https://arXiv.org/paper/1704.02553\"},\"title\":\"Securing Vehicle to Vehicle Communications using Blockchain through Visible Light and Acoustic Side-Channels\",\"created_at\":\"2024-11-10T13:43:07.385Z\",\"updated_at\":\"2025-03-03T21:16:46.267Z\",\"categories\":[\"Computer Science\"],\"subcategories\":[\"cs.CR\"],\"metrics\":{\"activity_rank\":0,\"questions_count\":0,\"responses_count\":0,\"upvotes_count\":null,\"downvotes_count\":null,\"total_votes\":0,\"visits_count\":{\"last24Hours\":0,\"last7Days\":0,\"last30Days\":1,\"last90Days\":1,\"all\":4},\"weighted_visits\":{\"last24Hours\":0,\"last7Days\":0,\"last30Days\":1.2884072798596656e-17,\"last90Days\":0.000002344324484108949,\"hot\":0},\"public_total_votes\":0,\"timeline\":[{\"date\":\"2025-03-30T21:22:15.054Z\",\"views\":2},{\"date\":\"2025-03-27T09:22:15.054Z\",\"views\":4},{\"date\":\"2025-03-23T21:22:15.054Z\",\"views\":2},{\"date\":\"2025-03-20T09:22:15.054Z\",\"views\":2},{\"date\":\"2025-03-16T21:22:15.054Z\",\"views\":1},{\"date\":\"2025-03-13T09:22:15.054Z\",\"views\":2},{\"date\":\"2025-03-09T21:22:15.054Z\",\"views\":2},{\"date\":\"2025-03-06T09:22:15.054Z\",\"views\":0},{\"date\":\"2025-03-02T21:22:15.054Z\",\"views\":1},{\"date\":\"2025-02-27T09:22:15.054Z\",\"views\":0},{\"date\":\"2025-02-23T21:22:15.054Z\",\"views\":2},{\"date\":\"2025-02-20T09:22:15.078Z\",\"views\":1},{\"date\":\"2025-02-16T21:22:15.098Z\",\"views\":0},{\"date\":\"2025-02-13T09:22:15.118Z\",\"views\":1},{\"date\":\"2025-02-09T21:22:15.139Z\",\"views\":2},{\"date\":\"2025-02-06T09:22:15.158Z\",\"views\":0},{\"date\":\"2025-02-02T21:22:15.177Z\",\"views\":2},{\"date\":\"2025-01-30T09:22:15.197Z\",\"views\":1},{\"date\":\"2025-01-26T21:22:15.217Z\",\"views\":0},{\"date\":\"2025-01-23T09:22:15.235Z\",\"views\":0},{\"date\":\"2025-01-19T21:22:15.258Z\",\"views\":1},{\"date\":\"2025-01-16T09:22:15.285Z\",\"views\":0},{\"date\":\"2025-01-12T21:22:15.310Z\",\"views\":2},{\"date\":\"2025-01-09T09:22:15.339Z\",\"views\":1},{\"date\":\"2025-01-05T21:22:15.359Z\",\"views\":1},{\"date\":\"2025-01-02T09:22:15.385Z\",\"views\":4},{\"date\":\"2024-12-29T21:22:15.405Z\",\"views\":1},{\"date\":\"2024-12-26T09:22:15.425Z\",\"views\":0},{\"date\":\"2024-12-22T21:22:15.444Z\",\"views\":3},{\"date\":\"2024-12-19T09:22:15.466Z\",\"views\":0},{\"date\":\"2024-12-15T21:22:15.486Z\",\"views\":0},{\"date\":\"2024-12-12T09:22:15.509Z\",\"views\":0},{\"date\":\"2024-12-08T21:22:15.531Z\",\"views\":2},{\"date\":\"2024-12-05T09:22:15.558Z\",\"views\":0},{\"date\":\"2024-12-01T21:22:15.604Z\",\"views\":0},{\"date\":\"2024-11-28T09:22:15.626Z\",\"views\":2},{\"date\":\"2024-11-24T21:22:15.646Z\",\"views\":0},{\"date\":\"2024-11-21T09:22:15.667Z\",\"views\":1},{\"date\":\"2024-11-17T21:22:15.688Z\",\"views\":1},{\"date\":\"2024-11-14T09:22:15.709Z\",\"views\":1},{\"date\":\"2024-11-10T21:22:15.731Z\",\"views\":2},{\"date\":\"2024-11-07T09:22:15.751Z\",\"views\":5},{\"date\":\"2024-11-03T21:22:15.768Z\",\"views\":2},{\"date\":\"2024-10-31T08:22:15.787Z\",\"views\":2},{\"date\":\"2024-10-27T20:22:15.812Z\",\"views\":2},{\"date\":\"2024-10-24T08:22:15.833Z\",\"views\":1},{\"date\":\"2024-10-20T20:22:15.858Z\",\"views\":2},{\"date\":\"2024-10-17T08:22:15.882Z\",\"views\":2},{\"date\":\"2024-10-13T20:22:15.906Z\",\"views\":2},{\"date\":\"2024-10-10T08:22:15.926Z\",\"views\":0},{\"date\":\"2024-10-06T20:22:15.949Z\",\"views\":1},{\"date\":\"2024-10-03T08:22:15.970Z\",\"views\":2},{\"date\":\"2024-09-29T20:22:15.992Z\",\"views\":2},{\"date\":\"2024-09-26T08:22:16.011Z\",\"views\":0},{\"date\":\"2024-09-22T20:22:16.033Z\",\"views\":2},{\"date\":\"2024-09-19T08:22:16.051Z\",\"views\":0},{\"date\":\"2024-09-15T20:22:16.070Z\",\"views\":2},{\"date\":\"2024-09-12T08:22:16.082Z\",\"views\":0},{\"date\":\"2024-09-08T20:22:16.094Z\",\"views\":2},{\"date\":\"2024-09-05T08:22:16.106Z\",\"views\":0},{\"date\":\"2024-09-01T20:22:16.115Z\",\"views\":2},{\"date\":\"2024-08-29T08:22:16.125Z\",\"views\":0}]},\"ranking\":{\"current_rank\":141922,\"previous_rank\":140739,\"activity_score\":0,\"paper_score\":0},\"is_hidden\":false,\"custom_categories\":null,\"first_publication_date\":\"2017-04-09T01:48:57.000Z\",\"author_user_ids\":[],\"citation\":{\"bibtex\":\"@misc{gerla2017securingvehiclevehicle,\\n title={Securing Vehicle to Vehicle Communications using Blockchain through Visible Light and Acoustic Side-Channels}, \\n author={Mario Gerla and Sean Rowan and Michael Clear and Meriel Huggard and Ciarán Mc Goldrick},\\n year={2017},\\n eprint={1704.02553},\\n archivePrefix={arXiv},\\n primaryClass={cs.CR},\\n url={https://arxiv.org/abs/1704.02553}, \\n}\"},\"paperVersions\":{\"_id\":\"673d87191e502f9ec7d25bda\",\"paper_group_id\":\"673d87181e502f9ec7d25bd5\",\"version_label\":\"v1\",\"version_order\":1,\"title\":\"Securing Vehicle to Vehicle Communications using Blockchain through Visible Light and Acoustic Side-Channels\",\"abstract\":\"$84\",\"author_ids\":[\"673d87191e502f9ec7d25bd6\",\"673d87191e502f9ec7d25bd7\",\"6733d59f29b032f3570974ff\",\"673d87191e502f9ec7d25bd8\",\"673d87191e502f9ec7d25bd9\"],\"publication_date\":\"2017-04-09T01:48:57.000Z\",\"license\":\"http://arxiv.org/licenses/nonexclusive-distrib/1.0/\",\"created_at\":\"2024-11-20T06:52:09.707Z\",\"updated_at\":\"2024-11-20T06:52:09.707Z\",\"is_deleted\":false,\"is_hidden\":false,\"universal_paper_id\":\"1704.02553\",\"imageURL\":\"image/1704.02553v1.png\"},\"verifiedAuthors\":[],\"authors\":[{\"_id\":\"6733d59f29b032f3570974ff\",\"full_name\":\"Mario Gerla\",\"affiliation\":null,\"orcid\":null,\"semantic_scholarid\":null,\"user_id\":null},{\"_id\":\"673d87191e502f9ec7d25bd6\",\"full_name\":\"Sean Rowan\",\"affiliation\":null,\"orcid\":null,\"semantic_scholarid\":null,\"user_id\":null},{\"_id\":\"673d87191e502f9ec7d25bd7\",\"full_name\":\"Michael Clear\",\"affiliation\":null,\"orcid\":null,\"semantic_scholarid\":null,\"user_id\":null},{\"_id\":\"673d87191e502f9ec7d25bd8\",\"full_name\":\"Meriel Huggard\",\"affiliation\":null,\"orcid\":null,\"semantic_scholarid\":null,\"user_id\":null},{\"_id\":\"673d87191e502f9ec7d25bd9\",\"full_name\":\"Ciarán Mc Goldrick\",\"affiliation\":null,\"orcid\":null,\"semantic_scholarid\":null,\"user_id\":null}]},\"max_version_order\":1,\"verified_authors\":[],\"authors\":[{\"_id\":\"6733d59f29b032f3570974ff\",\"full_name\":\"Mario Gerla\",\"affiliation\":null,\"orcid\":null,\"semantic_scholarid\":null,\"user_id\":null},{\"_id\":\"673d87191e502f9ec7d25bd6\",\"full_name\":\"Sean Rowan\",\"affiliation\":null,\"orcid\":null,\"semantic_scholarid\":null,\"user_id\":null},{\"_id\":\"673d87191e502f9ec7d25bd7\",\"full_name\":\"Michael Clear\",\"affiliation\":null,\"orcid\":null,\"semantic_scholarid\":null,\"user_id\":null},{\"_id\":\"673d87191e502f9ec7d25bd8\",\"full_name\":\"Meriel Huggard\",\"affiliation\":null,\"orcid\":null,\"semantic_scholarid\":null,\"user_id\":null},{\"_id\":\"673d87191e502f9ec7d25bd9\",\"full_name\":\"Ciarán Mc Goldrick\",\"affiliation\":null,\"orcid\":null,\"semantic_scholarid\":null,\"user_id\":null}],\"pdf_info\":{\"fetcher_url\":\"https://fetcher.alphaxiv.org/v2/pdf/1704.02553v1\"}}},\"dataUpdateCount\":1,\"dataUpdatedAt\":1744228239097,\"error\":null,\"errorUpdateCount\":0,\"errorUpdatedAt\":0,\"fetchFailureCount\":0,\"fetchFailureReason\":null,\"fetchMeta\":null,\"isInvalidated\":false,\"status\":\"success\",\"fetchStatus\":\"idle\"},\"queryKey\":[\"paper\",\"1704.02553\",\"metadata\"],\"queryHash\":\"[\\\"paper\\\",\\\"1704.02553\\\",\\\"metadata\\\"]\"},{\"state\":{\"data\":{\"data\":[]},\"dataUpdateCount\":1,\"dataUpdatedAt\":1744228239097,\"error\":null,\"errorUpdateCount\":0,\"errorUpdatedAt\":0,\"fetchFailureCount\":0,\"fetchFailureReason\":null,\"fetchMeta\":null,\"isInvalidated\":false,\"status\":\"success\",\"fetchStatus\":\"idle\"},\"queryKey\":[\"paper\",\"1704.02553\",\"comments\"],\"queryHash\":\"[\\\"paper\\\",\\\"1704.02553\\\",\\\"comments\\\"]\"},{\"state\":{\"data\":{\"data\":{\"paper_version\":{\"_id\":\"673b8b5aee7cdcdc03b175d4\",\"paper_group_id\":\"673b8b59ee7cdcdc03b175ce\",\"version_label\":\"v1\",\"version_order\":1,\"title\":\"Fixing the Loose Brake: Exponential-Tailed Stopping Time in Best Arm Identification\",\"abstract\":\"$85\",\"author_ids\":[\"673b8b59ee7cdcdc03b175d1\",\"673b8b5aee7cdcdc03b175d2\",\"672bc0b3986a1370676d6558\",\"67322f5dcd1e32a6e7f0a66d\"],\"publication_date\":\"2024-11-04T05:26:05.000Z\",\"license\":\"http://creativecommons.org/licenses/by-nc-sa/4.0/\",\"created_at\":\"2024-11-18T18:45:46.130Z\",\"updated_at\":\"2024-11-18T18:45:46.130Z\",\"is_deleted\":false,\"is_hidden\":false,\"universal_paper_id\":\"2411.01808\",\"imageURL\":\"image/2411.01808v1.png\"},\"paper_group\":{\"_id\":\"673b8b59ee7cdcdc03b175ce\",\"universal_paper_id\":\"2411.01808\",\"source\":{\"name\":\"arXiv\",\"url\":\"https://arXiv.org/paper/2411.01808\"},\"title\":\"Fixing the Loose Brake: Exponential-Tailed Stopping Time in Best Arm Identification\",\"created_at\":\"2024-11-05T04:32:41.908Z\",\"updated_at\":\"2025-03-03T19:41:43.872Z\",\"categories\":[\"Computer Science\",\"Statistics\"],\"subcategories\":[\"cs.LG\",\"stat.ML\"],\"metrics\":{\"activity_rank\":0,\"questions_count\":0,\"responses_count\":0,\"upvotes_count\":null,\"downvotes_count\":null,\"total_votes\":0,\"visits_count\":{\"last24Hours\":0,\"last7Days\":9,\"last30Days\":11,\"last90Days\":15,\"all\":55},\"weighted_visits\":{\"last24Hours\":0,\"last7Days\":0.0016641040297150885,\"last30Days\":1.4802834256533417,\"last90Days\":7.686736424126781,\"hot\":0.0016641040297150885},\"public_total_votes\":0,\"timeline\":[{\"date\":\"2025-04-02T01:18:35.366Z\",\"views\":24},{\"date\":\"2025-03-29T13:18:35.366Z\",\"views\":10},{\"date\":\"2025-03-26T01:18:35.366Z\",\"views\":1},{\"date\":\"2025-03-22T13:18:35.366Z\",\"views\":1},{\"date\":\"2025-03-19T01:18:35.366Z\",\"views\":2},{\"date\":\"2025-03-15T13:18:35.366Z\",\"views\":1},{\"date\":\"2025-03-12T01:18:35.366Z\",\"views\":2},{\"date\":\"2025-03-08T13:18:35.366Z\",\"views\":2},{\"date\":\"2025-03-05T01:18:35.366Z\",\"views\":0},{\"date\":\"2025-03-01T13:18:35.366Z\",\"views\":0},{\"date\":\"2025-02-26T01:18:35.366Z\",\"views\":0},{\"date\":\"2025-02-22T13:18:35.366Z\",\"views\":7},{\"date\":\"2025-02-19T01:18:35.385Z\",\"views\":0},{\"date\":\"2025-02-15T13:18:35.404Z\",\"views\":3},{\"date\":\"2025-02-12T01:18:35.418Z\",\"views\":0},{\"date\":\"2025-02-08T13:18:35.432Z\",\"views\":2},{\"date\":\"2025-02-05T01:18:35.445Z\",\"views\":2},{\"date\":\"2025-02-01T13:18:35.473Z\",\"views\":3},{\"date\":\"2025-01-29T01:18:35.489Z\",\"views\":1},{\"date\":\"2025-01-25T13:18:35.508Z\",\"views\":2},{\"date\":\"2025-01-22T01:18:35.525Z\",\"views\":1},{\"date\":\"2025-01-18T13:18:35.549Z\",\"views\":1},{\"date\":\"2025-01-15T01:18:35.567Z\",\"views\":1},{\"date\":\"2025-01-11T13:18:35.587Z\",\"views\":0},{\"date\":\"2025-01-08T01:18:35.606Z\",\"views\":0},{\"date\":\"2025-01-04T13:18:35.620Z\",\"views\":0},{\"date\":\"2025-01-01T01:18:35.635Z\",\"views\":1},{\"date\":\"2024-12-28T13:18:35.654Z\",\"views\":1},{\"date\":\"2024-12-25T01:18:35.673Z\",\"views\":1},{\"date\":\"2024-12-21T13:18:35.686Z\",\"views\":0},{\"date\":\"2024-12-18T01:18:35.705Z\",\"views\":2},{\"date\":\"2024-12-14T13:18:35.718Z\",\"views\":2},{\"date\":\"2024-12-11T01:18:35.737Z\",\"views\":1},{\"date\":\"2024-12-07T13:18:35.754Z\",\"views\":2},{\"date\":\"2024-12-04T01:18:35.772Z\",\"views\":2},{\"date\":\"2024-11-30T13:18:35.790Z\",\"views\":1},{\"date\":\"2024-11-27T01:18:35.807Z\",\"views\":0},{\"date\":\"2024-11-23T13:18:35.822Z\",\"views\":0},{\"date\":\"2024-11-20T01:18:35.838Z\",\"views\":2},{\"date\":\"2024-11-16T13:18:35.853Z\",\"views\":3},{\"date\":\"2024-11-13T01:18:35.872Z\",\"views\":4},{\"date\":\"2024-11-09T13:18:35.888Z\",\"views\":2},{\"date\":\"2024-11-06T01:18:35.900Z\",\"views\":2},{\"date\":\"2024-11-02T12:18:35.914Z\",\"views\":4}]},\"ranking\":{\"current_rank\":31374,\"previous_rank\":34987,\"activity_score\":0,\"paper_score\":0.34657359027997264},\"is_hidden\":false,\"custom_categories\":[\"active-learning\",\"adversarial-robustness\",\"statistical-learning\",\"optimization-methods\"],\"first_publication_date\":\"2024-11-04T05:26:05.000Z\",\"author_user_ids\":[],\"organizations\":[\"67be6378aa92218ccd8b10a3\"],\"paperVersions\":{\"_id\":\"673b8b5aee7cdcdc03b175d4\",\"paper_group_id\":\"673b8b59ee7cdcdc03b175ce\",\"version_label\":\"v1\",\"version_order\":1,\"title\":\"Fixing the Loose Brake: Exponential-Tailed Stopping Time in Best Arm Identification\",\"abstract\":\"$86\",\"author_ids\":[\"673b8b59ee7cdcdc03b175d1\",\"673b8b5aee7cdcdc03b175d2\",\"672bc0b3986a1370676d6558\",\"67322f5dcd1e32a6e7f0a66d\"],\"publication_date\":\"2024-11-04T05:26:05.000Z\",\"license\":\"http://creativecommons.org/licenses/by-nc-sa/4.0/\",\"created_at\":\"2024-11-18T18:45:46.130Z\",\"updated_at\":\"2024-11-18T18:45:46.130Z\",\"is_deleted\":false,\"is_hidden\":false,\"universal_paper_id\":\"2411.01808\",\"imageURL\":\"image/2411.01808v1.png\"},\"verifiedAuthors\":[],\"authors\":[{\"_id\":\"672bc0b3986a1370676d6558\",\"full_name\":\"Yao Zhao\",\"affiliation\":null,\"orcid\":null,\"semantic_scholarid\":null,\"user_id\":null},{\"_id\":\"67322f5dcd1e32a6e7f0a66d\",\"full_name\":\"Kwang-Sung Jun\",\"affiliation\":null,\"orcid\":null,\"semantic_scholarid\":null,\"user_id\":null},{\"_id\":\"673b8b59ee7cdcdc03b175d1\",\"full_name\":\"Kapilan Balagopalan\",\"affiliation\":null,\"orcid\":null,\"semantic_scholarid\":null,\"user_id\":null},{\"_id\":\"673b8b5aee7cdcdc03b175d2\",\"full_name\":\"Tuan Ngo Nguyen\",\"affiliation\":null,\"orcid\":null,\"semantic_scholarid\":null,\"user_id\":null}]},\"max_version_order\":1,\"verified_authors\":[],\"authors\":[{\"_id\":\"672bc0b3986a1370676d6558\",\"full_name\":\"Yao Zhao\",\"affiliation\":null,\"orcid\":null,\"semantic_scholarid\":null,\"user_id\":null},{\"_id\":\"67322f5dcd1e32a6e7f0a66d\",\"full_name\":\"Kwang-Sung Jun\",\"affiliation\":null,\"orcid\":null,\"semantic_scholarid\":null,\"user_id\":null},{\"_id\":\"673b8b59ee7cdcdc03b175d1\",\"full_name\":\"Kapilan Balagopalan\",\"affiliation\":null,\"orcid\":null,\"semantic_scholarid\":null,\"user_id\":null},{\"_id\":\"673b8b5aee7cdcdc03b175d2\",\"full_name\":\"Tuan Ngo Nguyen\",\"affiliation\":null,\"orcid\":null,\"semantic_scholarid\":null,\"user_id\":null}],\"pdf_info\":{\"fetcher_url\":\"https://fetcher.alphaxiv.org/v2/pdf/2411.01808v1\"}}},\"dataUpdateCount\":2,\"dataUpdatedAt\":1744228240242,\"error\":null,\"errorUpdateCount\":0,\"errorUpdatedAt\":0,\"fetchFailureCount\":0,\"fetchFailureReason\":null,\"fetchMeta\":null,\"isInvalidated\":false,\"status\":\"success\",\"fetchStatus\":\"idle\"},\"queryKey\":[\"paper\",\"2411.01808\",\"metadata\"],\"queryHash\":\"[\\\"paper\\\",\\\"2411.01808\\\",\\\"metadata\\\"]\"},{\"state\":{\"data\":{\"data\":[]},\"dataUpdateCount\":2,\"dataUpdatedAt\":1744228240242,\"error\":null,\"errorUpdateCount\":0,\"errorUpdatedAt\":0,\"fetchFailureCount\":0,\"fetchFailureReason\":null,\"fetchMeta\":null,\"isInvalidated\":false,\"status\":\"success\",\"fetchStatus\":\"idle\"},\"queryKey\":[\"paper\",\"2411.01808\",\"comments\"],\"queryHash\":\"[\\\"paper\\\",\\\"2411.01808\\\",\\\"comments\\\"]\"}]},\"data-sentry-element\":\"Hydrate\",\"data-sentry-component\":\"ServerAuthWrapper\",\"data-sentry-source-file\":\"ServerAuthWrapper.tsx\",\"children\":[\"$\",\"$L87\",null,{\"jwtFromServer\":null,\"data-sentry-element\":\"JwtHydrate\",\"data-sentry-source-file\":\"ServerAuthWrapper.tsx\",\"children\":[\"$\",\"$L88\",null,{\"data-sentry-element\":\"ClientLayout\",\"data-sentry-source-file\":\"layout.tsx\",\"children\":[\"$\",\"$L7\",null,{\"parallelRouterKey\":\"children\",\"segmentPath\":[\"children\"],\"error\":\"$89\",\"errorStyles\":[],\"errorScripts\":[],\"template\":[\"$\",\"$L8\",null,{}],\"templateStyles\":\"$undefined\",\"templateScripts\":\"$undefined\",\"notFound\":[[],[\"$\",\"div\",null,{\"className\":\"flex min-h-screen flex-col items-center justify-center bg-gray-100 px-8 dark:bg-gray-900\",\"data-sentry-component\":\"NotFound\",\"data-sentry-source-file\":\"not-found.tsx\",\"children\":[[\"$\",\"h1\",null,{\"className\":\"text-9xl font-medium text-customRed dark:text-red-400\",\"children\":\"404\"}],[\"$\",\"p\",null,{\"className\":\"max-w-md pb-12 pt-8 text-center text-lg text-gray-600 dark:text-gray-300\",\"children\":[\"We couldn't locate the page you're looking for.\",[\"$\",\"br\",null,{}],\"It's possible the link is outdated, or the page has been moved.\"]}],[\"$\",\"div\",null,{\"className\":\"space-x-4\",\"children\":[[\"$\",\"$L8a\",null,{\"href\":\"/\",\"data-sentry-element\":\"Link\",\"data-sentry-source-file\":\"not-found.tsx\",\"children\":[\"Go back home\"],\"className\":\"inline-flex items-center justify-center whitespace-nowrap rounded-md text-sm ring-offset-white transition-all duration-200 outline-none focus-visible:outline-none disabled:pointer-events-none disabled:opacity-50 dark:ring-offset-neutral-950 bg-customRed text-white hover:bg-customRed-hover enabled:active:ring-2 enabled:active:ring-customRed enabled:active:ring-opacity-50 enabled:active:ring-offset-2 h-10 py-1.5 px-4\",\"ref\":null,\"disabled\":\"$undefined\"}],[\"$\",\"$L8a\",null,{\"href\":\"mailto:contact@alphaxiv.org\",\"data-sentry-element\":\"Link\",\"data-sentry-source-file\":\"not-found.tsx\",\"children\":[\"Contact support\"],\"className\":\"inline-flex items-center justify-center whitespace-nowrap rounded-md text-sm ring-offset-white transition-all duration-200 outline-none focus-visible:outline-none disabled:pointer-events-none disabled:opacity-50 dark:ring-offset-neutral-950 bg-transparent text-customRed hover:bg-[#9a20360a] dark:hover:bg-customRed/25 enabled:active:ring-2 enabled:active:ring-customRed enabled:active:ring-opacity-25 enabled:active:ring-offset-2 h-10 py-1.5 px-4\",\"ref\":null,\"disabled\":\"$undefined\"}]]}]]}]],\"forbidden\":\"$undefined\",\"unauthorized\":\"$undefined\"}]}]}]}]\n"])</script><script>self.__next_f.push([1,"e:[[\"$\",\"meta\",\"0\",{\"charSet\":\"utf-8\"}],[\"$\",\"title\",\"1\",{\"children\":\"Transformers without Normalization | alphaXiv\"}],[\"$\",\"meta\",\"2\",{\"name\":\"description\",\"content\":\"View 2 comments: Should be α\"}],[\"$\",\"link\",\"3\",{\"rel\":\"manifest\",\"href\":\"/manifest.webmanifest\",\"crossOrigin\":\"$undefined\"}],[\"$\",\"meta\",\"4\",{\"name\":\"keywords\",\"content\":\"alphaxiv, arxiv, forum, discussion, explore, trending papers\"}],[\"$\",\"meta\",\"5\",{\"name\":\"robots\",\"content\":\"index, follow\"}],[\"$\",\"meta\",\"6\",{\"name\":\"googlebot\",\"content\":\"index, follow\"}],[\"$\",\"link\",\"7\",{\"rel\":\"canonical\",\"href\":\"https://www.alphaxiv.org/abs/2503.10622\"}],[\"$\",\"meta\",\"8\",{\"property\":\"og:title\",\"content\":\"Transformers without Normalization | alphaXiv\"}],[\"$\",\"meta\",\"9\",{\"property\":\"og:description\",\"content\":\"View 2 comments: Should be α\"}],[\"$\",\"meta\",\"10\",{\"property\":\"og:url\",\"content\":\"https://www.alphaxiv.org/abs/2503.10622\"}],[\"$\",\"meta\",\"11\",{\"property\":\"og:site_name\",\"content\":\"alphaXiv\"}],[\"$\",\"meta\",\"12\",{\"property\":\"og:locale\",\"content\":\"en_US\"}],[\"$\",\"meta\",\"13\",{\"property\":\"og:image\",\"content\":\"https://paper-assets.alphaxiv.org/image/2503.10622v1.png\"}],[\"$\",\"meta\",\"14\",{\"property\":\"og:image:width\",\"content\":\"816\"}],[\"$\",\"meta\",\"15\",{\"property\":\"og:image:height\",\"content\":\"1056\"}],[\"$\",\"meta\",\"16\",{\"property\":\"og:type\",\"content\":\"website\"}],[\"$\",\"meta\",\"17\",{\"name\":\"twitter:card\",\"content\":\"summary_large_image\"}],[\"$\",\"meta\",\"18\",{\"name\":\"twitter:creator\",\"content\":\"@askalphaxiv\"}],[\"$\",\"meta\",\"19\",{\"name\":\"twitter:title\",\"content\":\"Transformers without Normalization | alphaXiv\"}],[\"$\",\"meta\",\"20\",{\"name\":\"twitter:description\",\"content\":\"View 2 comments: Should be α\"}],[\"$\",\"meta\",\"21\",{\"name\":\"twitter:image\",\"content\":\"https://www.alphaxiv.org/nextapi/og?paperTitle=Transformers+without+Normalization\u0026authors=Kaiming+He%2C+Yann+LeCun%2C+Xinlei+Chen%2C+Zhuang+Liu%2C+Jiachen+Zhu\"}],[\"$\",\"meta\",\"22\",{\"name\":\"twitter:image:alt\",\"content\":\"Transformers without Normalization | alphaXiv\"}],[\"$\",\"link\",\"23\",{\"rel\":\"icon\",\"href\":\"/icon.ico?ba7039e153811708\",\"type\":\"image/x-icon\",\"sizes\":\"16x16\"}]]\n"])</script><script>self.__next_f.push([1,"c:null\n"])</script><script>self.__next_f.push([1,"97:I[44368,[\"3110\",\"static/chunks/1da0d171-1f9041fa20b0f780.js\",\"6906\",\"static/chunks/62420ecc-ba068cf8c61f9a07.js\",\"2029\",\"static/chunks/9d987bc4-d447aa4b86ffa8da.js\",\"7701\",\"static/chunks/c386c4a4-4ae2baf83c93de20.js\",\"6117\",\"static/chunks/6117-41689ef6ff9b033c.js\",\"1350\",\"static/chunks/1350-a1024eb8f8a6859e.js\",\"8951\",\"static/chunks/8951-bcdd0e91584e856e.js\",\"1447\",\"static/chunks/1447-9124dd5e537fe7a8.js\",\"666\",\"static/chunks/666-78ffa3c0d0a5a2a3.js\",\"932\",\"static/chunks/932-76dfd6658f5c91af.js\",\"5872\",\"static/chunks/5872-dd1cde135170beb6.js\",\"7299\",\"static/chunks/7299-64abce2685056cd4.js\",\"4765\",\"static/chunks/4765-88aa2d5b19cb25bc.js\",\"7362\",\"static/chunks/7362-50e5d1ac2abc44a0.js\",\"2068\",\"static/chunks/2068-7fbc56857b0cc3b1.js\",\"2755\",\"static/chunks/2755-54255117838ce4e4.js\",\"4505\",\"static/chunks/4505-4fe5d5f302c56050.js\",\"8273\",\"static/chunks/8273-4cb3558ea58359d7.js\",\"6681\",\"static/chunks/6681-13aed21c8bb47aa3.js\",\"4005\",\"static/chunks/4005-6fe3c26cb25644be.js\",\"4785\",\"static/chunks/4785-5dbc1af26cd46ec5.js\",\"6335\",\"static/chunks/6335-5d291246680ceb4d.js\",\"2642\",\"static/chunks/2642-b497e0f313459fb9.js\",\"5145\",\"static/chunks/5145-f10798defa0dde88.js\",\"8114\",\"static/chunks/8114-2172b7ef97f83184.js\",\"9392\",\"static/chunks/9392-5fab98d8656406c4.js\",\"9305\",\"static/chunks/app/(paper)/%5Bid%5D/layout-3e11c64ff66d737f.js\"],\"default\"]\n99:I[43268,[\"3110\",\"static/chunks/1da0d171-1f9041fa20b0f780.js\",\"6906\",\"static/chunks/62420ecc-ba068cf8c61f9a07.js\",\"2029\",\"static/chunks/9d987bc4-d447aa4b86ffa8da.js\",\"7701\",\"static/chunks/c386c4a4-4ae2baf83c93de20.js\",\"6117\",\"static/chunks/6117-41689ef6ff9b033c.js\",\"1350\",\"static/chunks/1350-a1024eb8f8a6859e.js\",\"8951\",\"static/chunks/8951-bcdd0e91584e856e.js\",\"1447\",\"static/chunks/1447-9124dd5e537fe7a8.js\",\"666\",\"static/chunks/666-78ffa3c0d0a5a2a3.js\",\"932\",\"static/chunks/932-76dfd6658f5c91af.js\",\"5872\",\"static/chunks/5872-dd1cde135170beb6.js\",\"7299\",\"static/chunks/7299-64abce2685056cd4.js\",\"4765\",\"static/chunks/4765-88aa2d5b19cb25bc.js\",\"7362\",\"static/chunks/7362-50e5d1ac2abc44a0.j"])</script><script>self.__next_f.push([1,"s\",\"2068\",\"static/chunks/2068-7fbc56857b0cc3b1.js\",\"2755\",\"static/chunks/2755-54255117838ce4e4.js\",\"4505\",\"static/chunks/4505-4fe5d5f302c56050.js\",\"8273\",\"static/chunks/8273-4cb3558ea58359d7.js\",\"6681\",\"static/chunks/6681-13aed21c8bb47aa3.js\",\"4005\",\"static/chunks/4005-6fe3c26cb25644be.js\",\"4785\",\"static/chunks/4785-5dbc1af26cd46ec5.js\",\"6335\",\"static/chunks/6335-5d291246680ceb4d.js\",\"2642\",\"static/chunks/2642-b497e0f313459fb9.js\",\"5145\",\"static/chunks/5145-f10798defa0dde88.js\",\"8114\",\"static/chunks/8114-2172b7ef97f83184.js\",\"9392\",\"static/chunks/9392-5fab98d8656406c4.js\",\"9305\",\"static/chunks/app/(paper)/%5Bid%5D/layout-3e11c64ff66d737f.js\"],\"default\"]\n9a:I[53145,[\"3110\",\"static/chunks/1da0d171-1f9041fa20b0f780.js\",\"6906\",\"static/chunks/62420ecc-ba068cf8c61f9a07.js\",\"2029\",\"static/chunks/9d987bc4-d447aa4b86ffa8da.js\",\"7701\",\"static/chunks/c386c4a4-4ae2baf83c93de20.js\",\"6117\",\"static/chunks/6117-41689ef6ff9b033c.js\",\"1350\",\"static/chunks/1350-a1024eb8f8a6859e.js\",\"8951\",\"static/chunks/8951-bcdd0e91584e856e.js\",\"1447\",\"static/chunks/1447-9124dd5e537fe7a8.js\",\"666\",\"static/chunks/666-78ffa3c0d0a5a2a3.js\",\"932\",\"static/chunks/932-76dfd6658f5c91af.js\",\"5872\",\"static/chunks/5872-dd1cde135170beb6.js\",\"7299\",\"static/chunks/7299-64abce2685056cd4.js\",\"4765\",\"static/chunks/4765-88aa2d5b19cb25bc.js\",\"7362\",\"static/chunks/7362-50e5d1ac2abc44a0.js\",\"2068\",\"static/chunks/2068-7fbc56857b0cc3b1.js\",\"2755\",\"static/chunks/2755-54255117838ce4e4.js\",\"4505\",\"static/chunks/4505-4fe5d5f302c56050.js\",\"8273\",\"static/chunks/8273-4cb3558ea58359d7.js\",\"6681\",\"static/chunks/6681-13aed21c8bb47aa3.js\",\"4005\",\"static/chunks/4005-6fe3c26cb25644be.js\",\"4785\",\"static/chunks/4785-5dbc1af26cd46ec5.js\",\"6335\",\"static/chunks/6335-5d291246680ceb4d.js\",\"2642\",\"static/chunks/2642-b497e0f313459fb9.js\",\"5145\",\"static/chunks/5145-f10798defa0dde88.js\",\"8114\",\"static/chunks/8114-2172b7ef97f83184.js\",\"9392\",\"static/chunks/9392-5fab98d8656406c4.js\",\"9305\",\"static/chunks/app/(paper)/%5Bid%5D/layout-3e11c64ff66d737f.js\"],\"default\"]\n8b:T440,Normalization layers ar"])</script><script>self.__next_f.push([1,"e ubiquitous in modern neural networks and have long\nbeen considered essential. This work demonstrates that Transformers without\nnormalization can achieve the same or better performance using a remarkably\nsimple technique. We introduce Dynamic Tanh (DyT), an element-wise operation\n$DyT($x$) = \\tanh(\\alpha $x$)$, as a drop-in replacement for normalization\nlayers in Transformers. DyT is inspired by the observation that layer\nnormalization in Transformers often produces tanh-like, $S$-shaped input-output\nmappings. By incorporating DyT, Transformers without normalization can match or\nexceed the performance of their normalized counterparts, mostly without\nhyperparameter tuning. We validate the effectiveness of Transformers with DyT\nacross diverse settings, ranging from recognition to generation, supervised to\nself-supervised learning, and computer vision to language models. These\nfindings challenge the conventional understanding that normalization layers are\nindispensable in modern neural networks, and offer new insights into their role\nin deep networks.8c:T1fad,"])</script><script>self.__next_f.push([1,"## Research Paper Analysis: Transformers without Normalization\n\n**1. Authors, Institution(s), and Research Group Context**\n\n* **Authors:** Jiachen Zhu, Xinlei Chen, Kaiming He, Yann LeCun, and Zhuang Liu.\n* **Institutions:**\n * FAIR, Meta (Zhu, Chen, Liu)\n * New York University (Zhu, LeCun)\n * MIT (He)\n * Princeton University (Liu)\n* **Research Group Context:** This research appears to stem from a collaboration across Meta's FAIR (Fundamental AI Research) lab, prominent academic institutions (NYU, MIT, Princeton). Kaiming He and Yann LeCun are exceptionally well-known figures in the deep learning community, with significant contributions to areas like residual networks, object recognition, and convolutional neural networks. Xinlei Chen and Zhuang Liu also have strong research backgrounds, evident from their presence at FAIR and affiliations with top universities.\n * The participation of FAIR, Meta implies access to substantial computational resources and a focus on cutting-edge research with potential for real-world applications.\n * The involvement of researchers from top academic institutions ensures theoretical rigor and connection to the broader scientific community.\n * The project lead, Zhuang Liu, and the corresponding author, Jiachen Zhu, would likely be responsible for driving the research forward, while senior researchers such as Kaiming He and Yann LeCun might provide high-level guidance and expertise.\n\n**2. How This Work Fits into the Broader Research Landscape**\n\n* **Normalization Layers in Deep Learning:** Normalization layers, particularly Batch Normalization (BN) and Layer Normalization (LN), have become a standard component in modern neural networks since the introduction of BN in 2015. They are primarily used to improve training stability, accelerate convergence, and enhance model performance.\n* **Transformers and Normalization:** LN has become the normalization layer of choice for Transformer architectures, which have revolutionized natural language processing and computer vision.\n* **Challenging the Status Quo:** This paper directly challenges the conventional wisdom that normalization layers are indispensable for training deep neural networks, specifically Transformers. This challenges recent architectures that almost always retain normalization layers.\n* **Prior Work on Removing Normalization:** Previous research has explored alternative initialization schemes, weight normalization techniques, or modifications to the network architecture to reduce the reliance on normalization layers. This work builds upon this research direction by providing a simpler alternative that doesn't involve architecture change.\n* **Significance:** If successful, this research could lead to more efficient neural networks, potentially reducing training and inference time and opening avenues for deployment on resource-constrained devices.\n* **Competition:** This paper compares the results to two popular initialization-based methods, Fixup and SkipInit, and weight-normalization-based method σReparam.\n\n**3. Key Objectives and Motivation**\n\n* **Objective:** To demonstrate that Transformers can achieve comparable or better performance without normalization layers.\n* **Motivation:**\n * To challenge the widely held belief that normalization layers are essential for training deep neural networks.\n * To develop a simpler and potentially more efficient alternative to normalization layers in Transformers.\n * To gain a better understanding of the role and mechanisms of normalization layers in deep learning.\n * The authors observed that Layer Normalization (LN) layers in trained Transformers exhibit tanh-like, S-shaped input-output mappings. This observation inspired them to explore a more direct way to achieve this effect.\n* **Goal:** Replace existing normalization layers with DyT, while still maintaining a stable model\n\n**4. Methodology and Approach**\n\n* **Dynamic Tanh (DyT):** The authors propose Dynamic Tanh (DyT), an element-wise operation defined as `DyT(x) = tanh(αx)`, where α is a learnable parameter. This operation is designed to emulate the behavior of LN by learning an appropriate scaling factor through α and squashing extreme values using the tanh function.\n* **Drop-in Replacement:** The approach involves directly replacing existing LN or RMSNorm layers with DyT layers in various Transformer architectures, including Vision Transformers, Diffusion Transformers, and language models.\n* **Empirical Validation:** The effectiveness of DyT is evaluated empirically across a diverse range of tasks and domains, including supervised learning, self-supervised learning, image generation, and language modeling.\n* **Experimental Setup:** The experiments use the same training protocols and hyperparameters as the original normalized models to highlight the simplicity of adapting DyT.\n* **Ablation Studies:** Ablation studies are conducted to analyze the role of the tanh function and the learnable scale α in DyT.\n* **Comparison with Other Methods:** DyT is compared against other methods for training Transformers without normalization, such as Fixup, SkipInit, and σReparam.\n* **Efficiency Benchmarking:** The computational efficiency of DyT is compared to that of RMSNorm by measuring the inference and training latency of LLaMA models.\n* **Analysis of α Values:** The behavior of the learnable parameter α is analyzed throughout training and in trained networks to understand its role in maintaining activations within a suitable range.\n\n**5. Main Findings and Results**\n\n* **Comparable or Better Performance:** Transformers with DyT match or exceed the performance of their normalized counterparts across a wide range of tasks and domains, including image classification, self-supervised learning, image generation, and language modeling.\n* **Training Stability:** Models with DyT train stably, often without the need for hyperparameter tuning.\n* **Computational Efficiency:** DyT significantly reduces computation time compared to RMSNorm, both in inference and training.\n* **Importance of Squashing Function:** The tanh function is crucial for stable training, as replacing it with the identity function leads to divergence.\n* **Role of Learnable Scale α:** The learnable parameter α is essential for overall model performance and functions partially as a normalization mechanism by learning values approximating 1/std of the input activations.\n* **Superior Performance Compared to Other Methods:** DyT consistently outperforms other methods for training Transformers without normalization, such as Fixup, SkipInit, and σReparam.\n* **Sensitivity of LLMs to α initialization:** LLMs showed more performance variability to alpha initialization than other models tested.\n\n**6. Significance and Potential Impact**\n\n* **Challenges Conventional Understanding:** The findings challenge the widely held belief that normalization layers are indispensable for training modern neural networks.\n* **Simpler and More Efficient Alternative:** DyT provides a simpler and potentially more efficient alternative to normalization layers in Transformers.\n* **Improved Training and Inference Speed:** DyT improves training and inference speed, making it a promising candidate for efficiency-oriented network design.\n* **Better Understanding of Normalization Layers:** The study contributes to a better understanding of the mechanisms of normalization layers.\n* **Future Directions:** This work could open up new avenues for research in deep learning, including:\n * Exploring other alternatives to normalization layers.\n * Investigating the theoretical properties of DyT.\n * Applying DyT to other types of neural networks and tasks.\n * Developing adaptive methods for setting the initial value of α.\n* **Limitations** DyT struggles to replace BN directly in classic networks like ResNets, so further studies are needed to determine how DyT can adapt to models with other types of normalization layers."])</script><script>self.__next_f.push([1,"8d:T3998,"])</script><script>self.__next_f.push([1,"# Transformers without Normalization: A Simple Alternative with Dynamic Tanh\n\n## Table of Contents\n- [Introduction](#introduction)\n- [Understanding Normalization Layers](#understanding-normalization-layers)\n- [The Dynamic Tanh Solution](#the-dynamic-tanh-solution)\n- [How DyT Works](#how-dyt-works)\n- [Experimental Evidence](#experimental-evidence)\n- [Tuning and Scalability](#tuning-and-scalability)\n- [Analysis of Alpha Parameter](#analysis-of-alpha-parameter)\n- [Comparing with Other Approaches](#comparing-with-other-approaches)\n- [Implications and Applications](#implications-and-applications)\n- [Conclusion](#conclusion)\n\n## Introduction\n\nNormalization layers have been considered essential components in modern neural networks, particularly in Transformer architectures that dominate natural language processing, computer vision, and other domains. Layer Normalization (LN) and its variants are ubiquitous in Transformers, believed to be crucial for stabilizing training and improving performance. However, a new paper by researchers from Meta AI, NYU, MIT, and Princeton University challenges this fundamental assumption by demonstrating that Transformers can achieve equivalent or better performance without traditional normalization layers.\n\n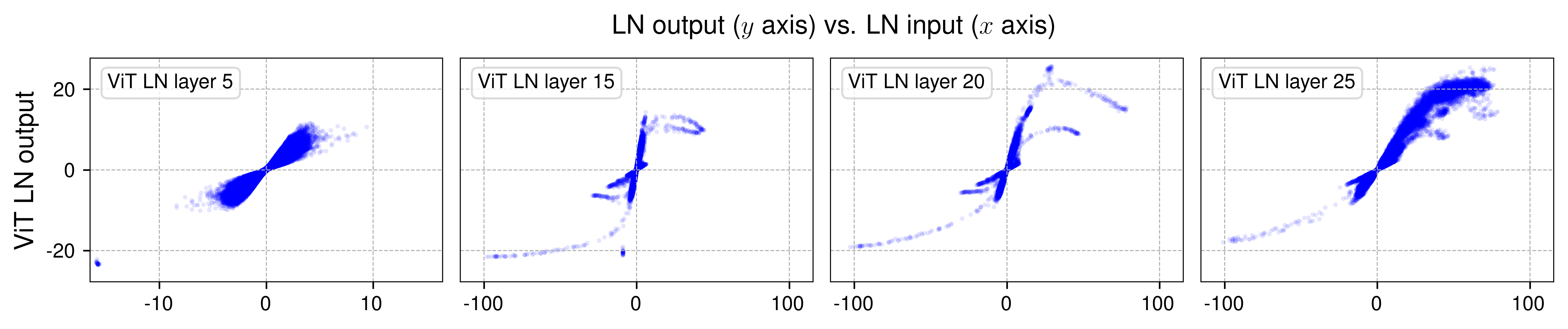\n*Figure 1: Visualization of Layer Normalization's input-output behavior in various ViT layers, showing S-shaped, tanh-like relationships.*\n\n## Understanding Normalization Layers\n\nNormalization techniques like Batch Normalization, Layer Normalization, and RMSNorm have become standard practice in deep learning. These methods typically normalize activations by computing statistics (mean and/or standard deviation) across specified dimensions, helping to stabilize training by controlling the distribution of network activations.\n\nIn Transformers specifically, Layer Normalization operates by computing the mean and standard deviation across the feature dimension for each token or position. This normalization process is computationally expensive as it requires calculating these statistics at each layer during both training and inference.\n\nThe authors observed that Layer Normalization often produces tanh-like, S-shaped input-output mappings, as shown in Figure 1. This observation led to their key insight: perhaps the beneficial effect of normalization could be achieved through a simpler mechanism that mimics this S-shaped behavior without computing activation statistics.\n\n## The Dynamic Tanh Solution\n\nThe researchers propose Dynamic Tanh (DyT) as a straightforward replacement for normalization layers. DyT is defined as:\n\n```\nDyT(x) = tanh(αx)\n```\n\nWhere α is a learnable parameter that controls the steepness of the tanh function. This simple formulation eliminates the need to compute activation statistics while preserving the S-shaped transformation that seems to be important for Transformer performance.\n\n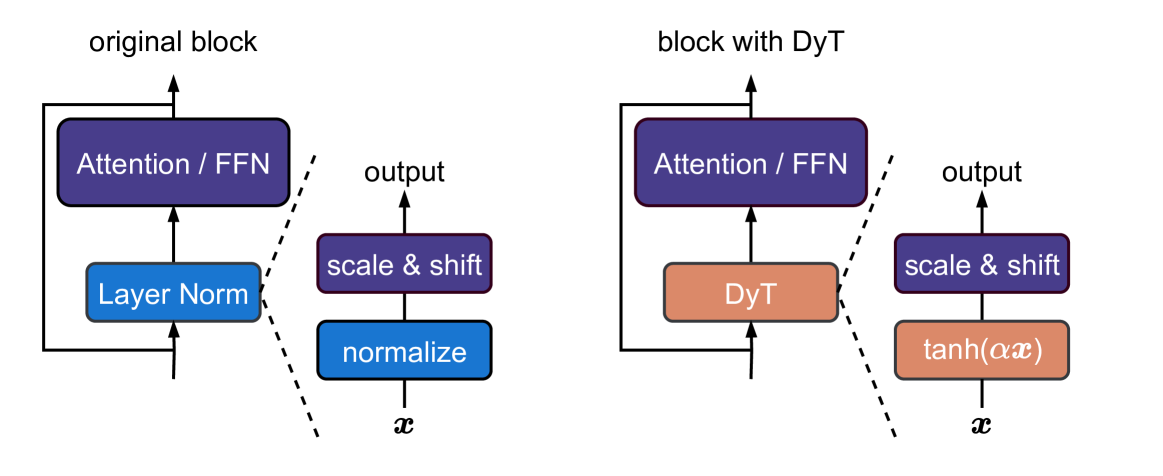\n*Figure 2: Left: Original Transformer block with Layer Normalization. Right: Proposed block with Dynamic Tanh (DyT) replacement.*\n\nThe beauty of this approach lies in its simplicity - replacing complex normalization operations with a single element-wise operation that has a learnable parameter. Figure 2 shows how the traditional Transformer block with Layer Normalization compares to the proposed block with DyT.\n\n## How DyT Works\n\nDynamic Tanh works through two key mechanisms:\n\n1. **Value Squashing**: The tanh function squashes extreme values, providing a form of implicit regularization similar to normalization layers. This prevents activations from growing too large during forward and backward passes.\n\n2. **Adaptive Scaling**: The learnable parameter α adjusts the steepness of the tanh function, allowing the network to control how aggressively values are squashed. This adaptivity is crucial for performance.\n\nThe hyperbolic tangent function (tanh) is bounded between -1 and 1, squashing any input value into this range. The steepness of this squashing is controlled by α:\n\n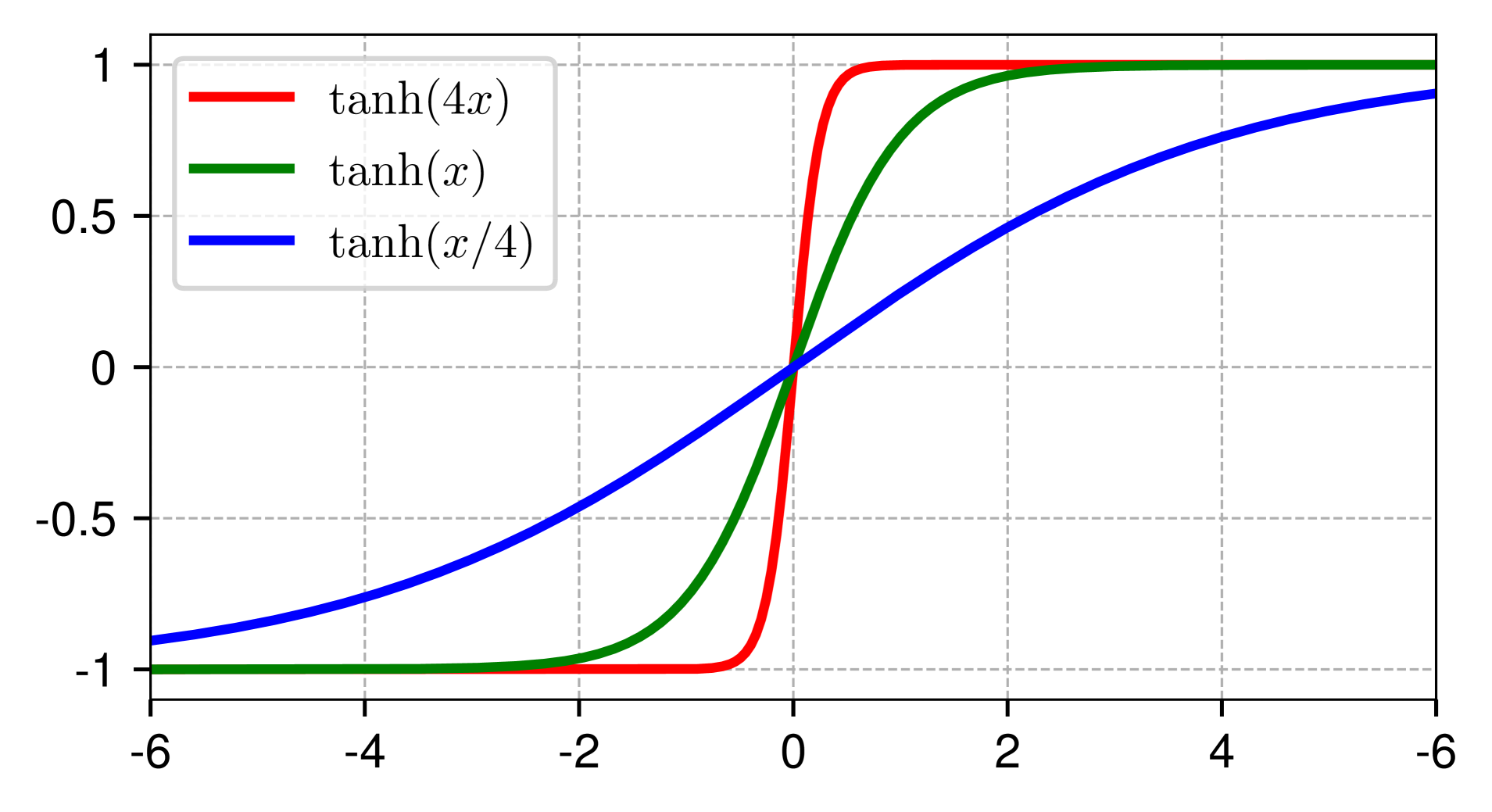\n*Figure 3: The tanh function with different α values, showing how larger α values create sharper transitions.*\n\nAs shown in Figure 3, a larger α value makes the transition from -1 to 1 sharper, while a smaller α makes it more gradual. This flexibility allows the network to adjust the degree of value squashing based on the task and layer depth.\n\n## Experimental Evidence\n\nThe researchers conducted extensive experiments across diverse tasks and domains to validate the effectiveness of DyT as a replacement for normalization layers. These experiments included:\n\n1. **Vision Tasks**:\n - ImageNet classification with Vision Transformers (ViT) and ConvNeXt\n - Self-supervised learning with MAE and DINO\n\n2. **Generative Models**:\n - Diffusion models for image generation (DiT)\n\n3. **Large Language Models**:\n - LLaMA pretraining at scales from 7B to 70B parameters\n\n4. **Other Domains**:\n - Speech processing with wav2vec 2.0\n - DNA sequence modeling with HyenaDNA and Caduceus\n\nThe results consistently showed that Transformers with DyT could match or exceed the performance of their normalized counterparts. For example, with Vision Transformers on ImageNet classification, the DyT variant achieved comparable accuracy to the LN version:\n\n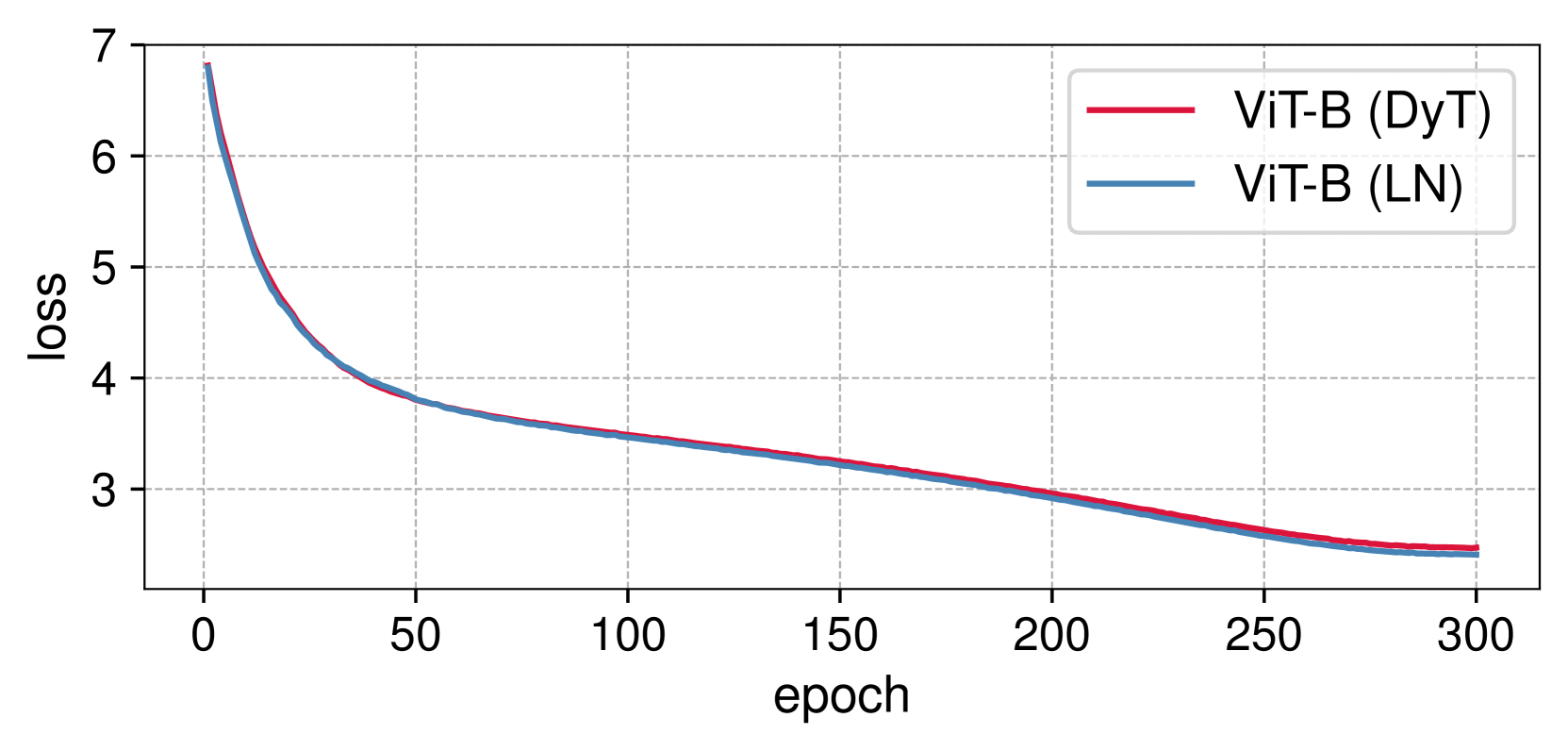\n*Figure 4: Training loss curves for ViT-B with Layer Normalization (LN) and Dynamic Tanh (DyT), showing nearly identical convergence.*\n\nSimilarly, for LLaMA models of various sizes (7B to 70B parameters), DyT variants achieved comparable or slightly better loss values compared to RMSNorm models:\n\n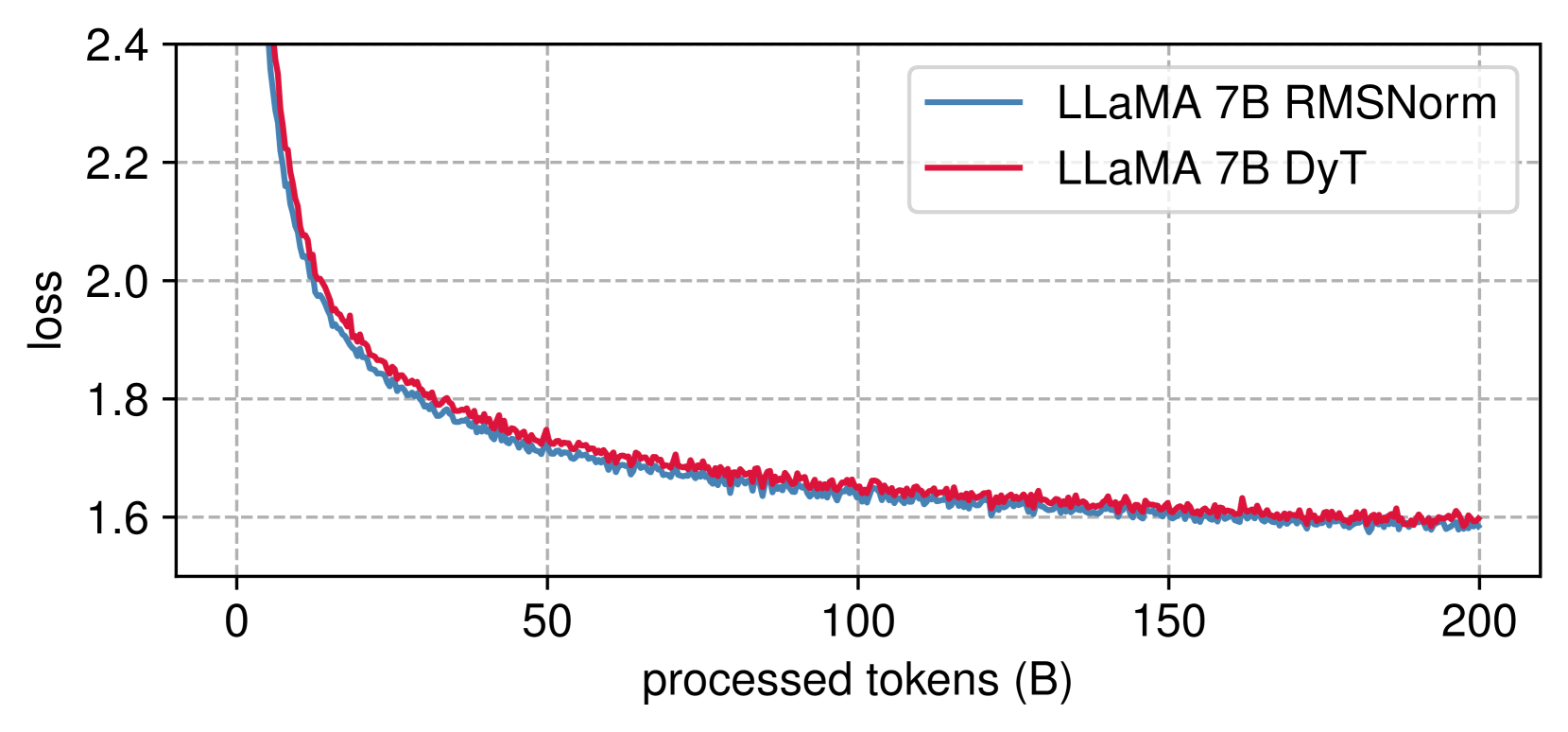\n*Figure 5: Training loss curves for LLaMA 7B with RMSNorm and DyT, showing comparable performance.*\n\n## Tuning and Scalability\n\nWhile DyT is generally robust and works well with minimal tuning, the researchers found that for larger models, particularly Large Language Models (LLMs), careful initialization of α is important. They conducted a thorough exploration of initialization values for the LLaMA architecture:\n\n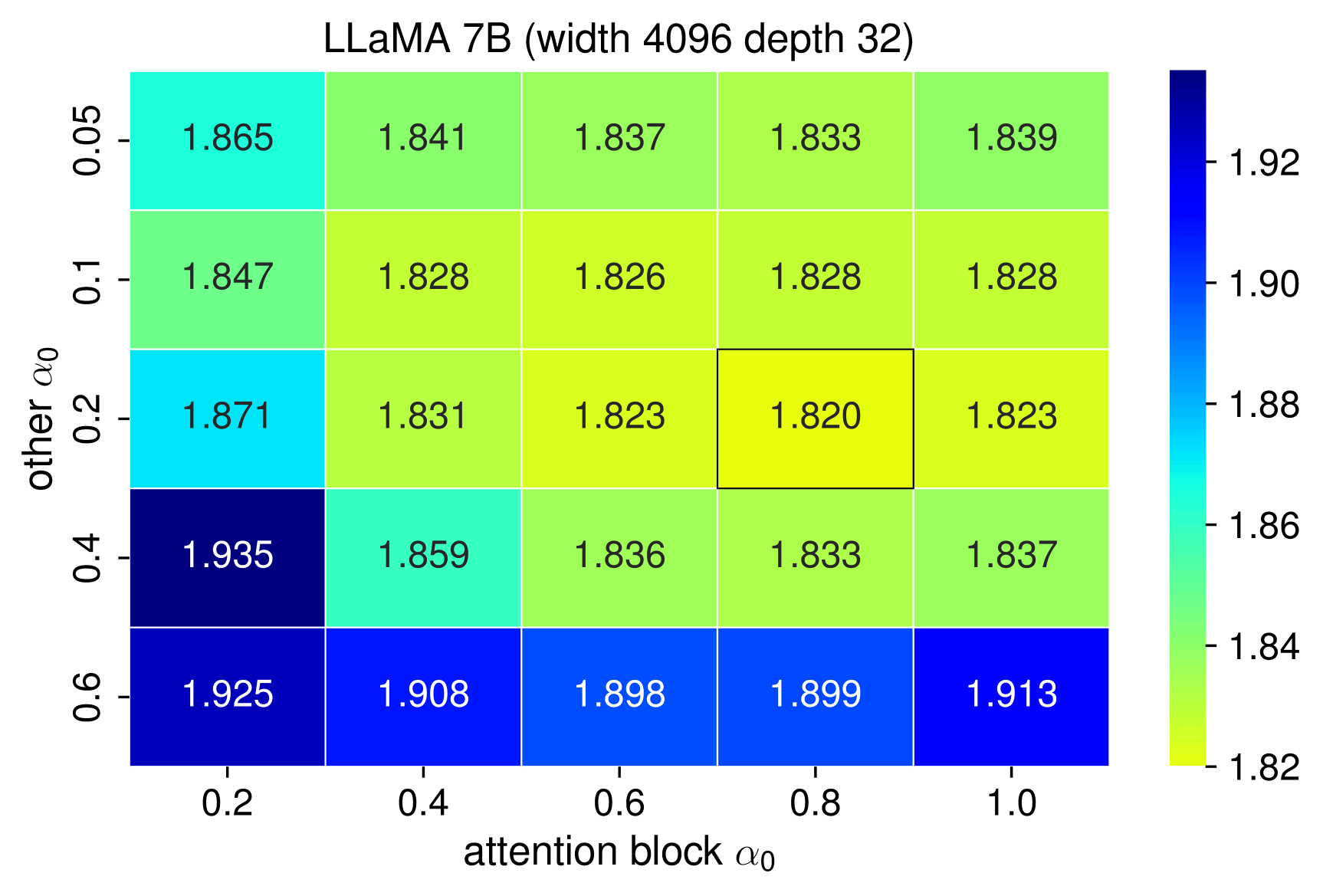\n*Figure 6: Heatmap showing LLaMA 7B performance with different α initialization values for attention and feedforward blocks.*\n\nFor LLaMA 7B, the optimal α initialization was found to be 0.2 for attention blocks and 0.2 for other blocks, while for LLaMA 13B, it was 0.6 for attention blocks and 0.15 for other blocks. This suggests that larger models may require more careful tuning of the α parameter.\n\nThe researchers also tested the scalability of their approach by training models of different depths and widths:\n\n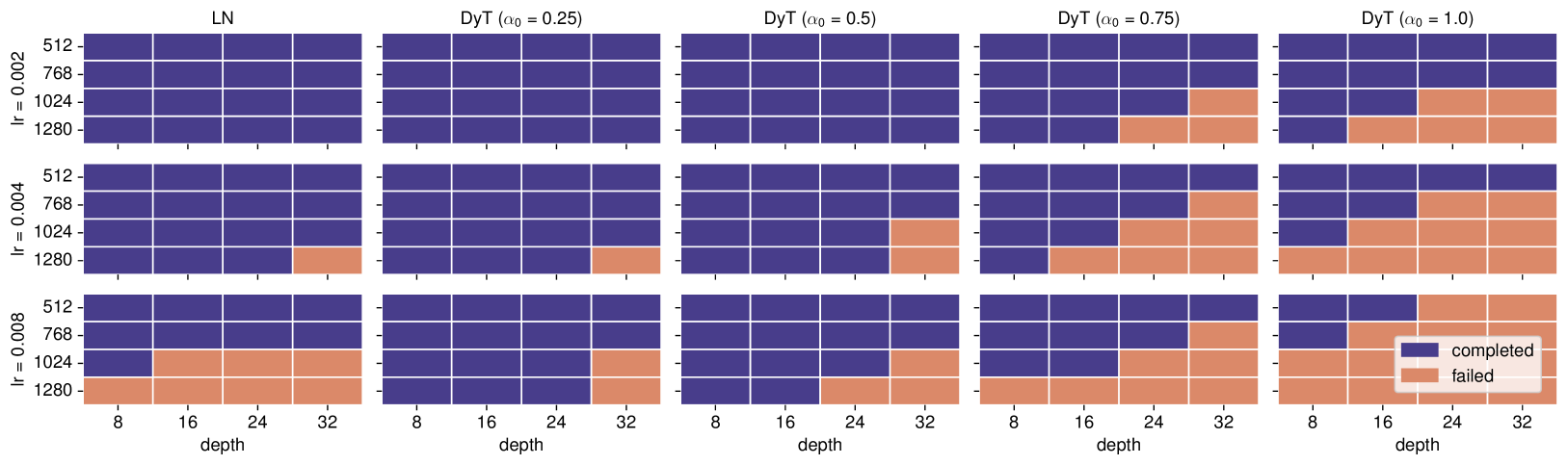\n*Figure 7: Training stability comparison between LN and DyT across different model depths and widths, with blue indicating successful training and orange indicating instability.*\n\nThe results showed that DyT models could scale comparably to LN models, though with some additional sensitivity to learning rate at larger scales.\n\n## Analysis of Alpha Parameter\n\nThe researchers analyzed how the α parameter in DyT relates to the statistical properties of activations. Interestingly, they found that α learns to approximate the inverse of the standard deviation of layer activations:\n\n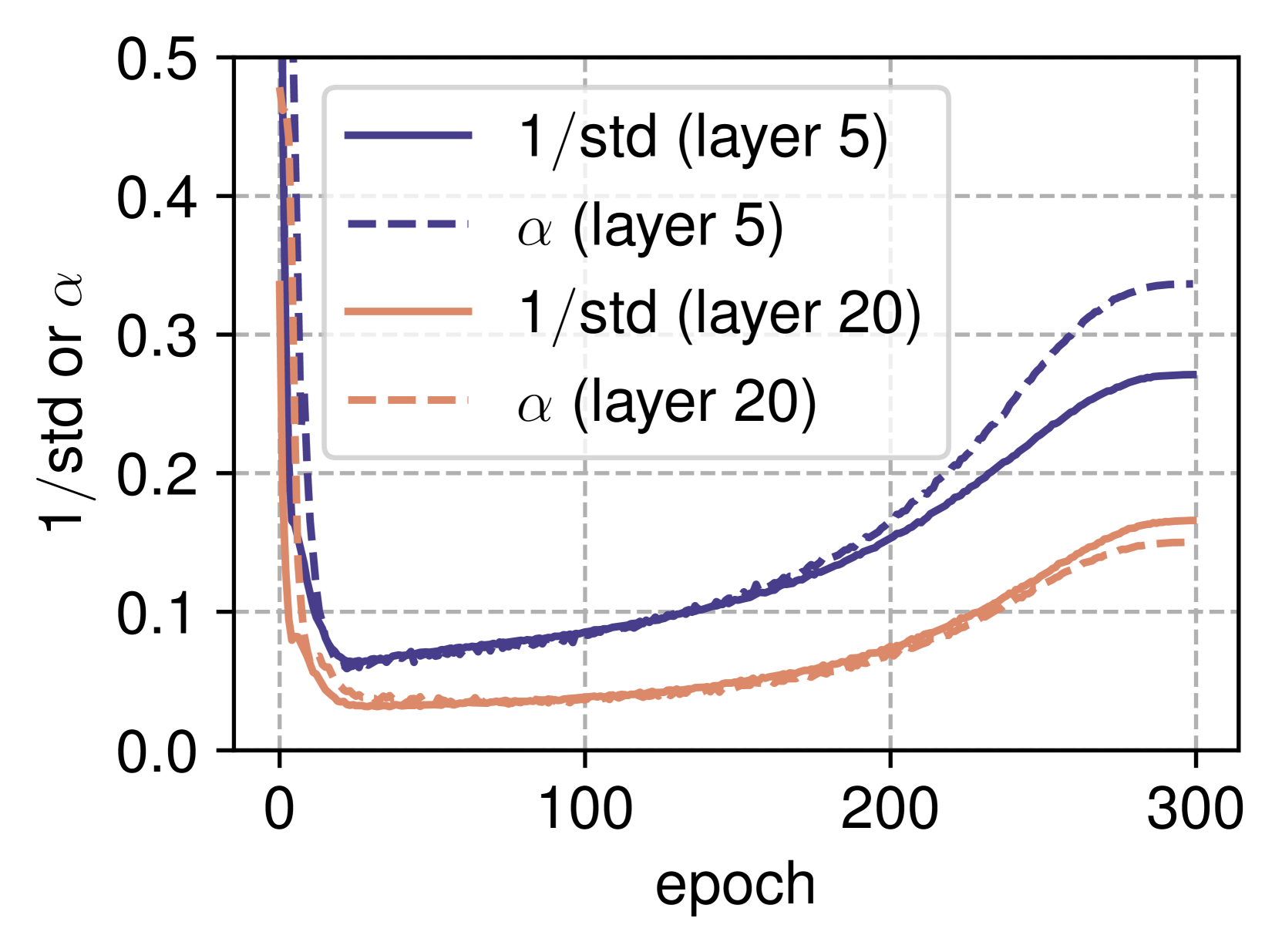\n*Figure 8: Comparison between the learned α values and the inverse of activation standard deviation (1/std) across training epochs, showing how α partially mimics normalization behavior.*\n\nThis finding suggests that DyT implicitly learns to perform a form of adaptive scaling similar to normalization layers, but without explicitly computing statistics. The α parameter tends to be inversely proportional to the standard deviation of activations, effectively scaling inputs such that their magnitude is appropriate for the tanh function.\n\nFurthermore, they observed a consistent correlation between the learned α values and the inverse standard deviation of activations across different layers and models:\n\n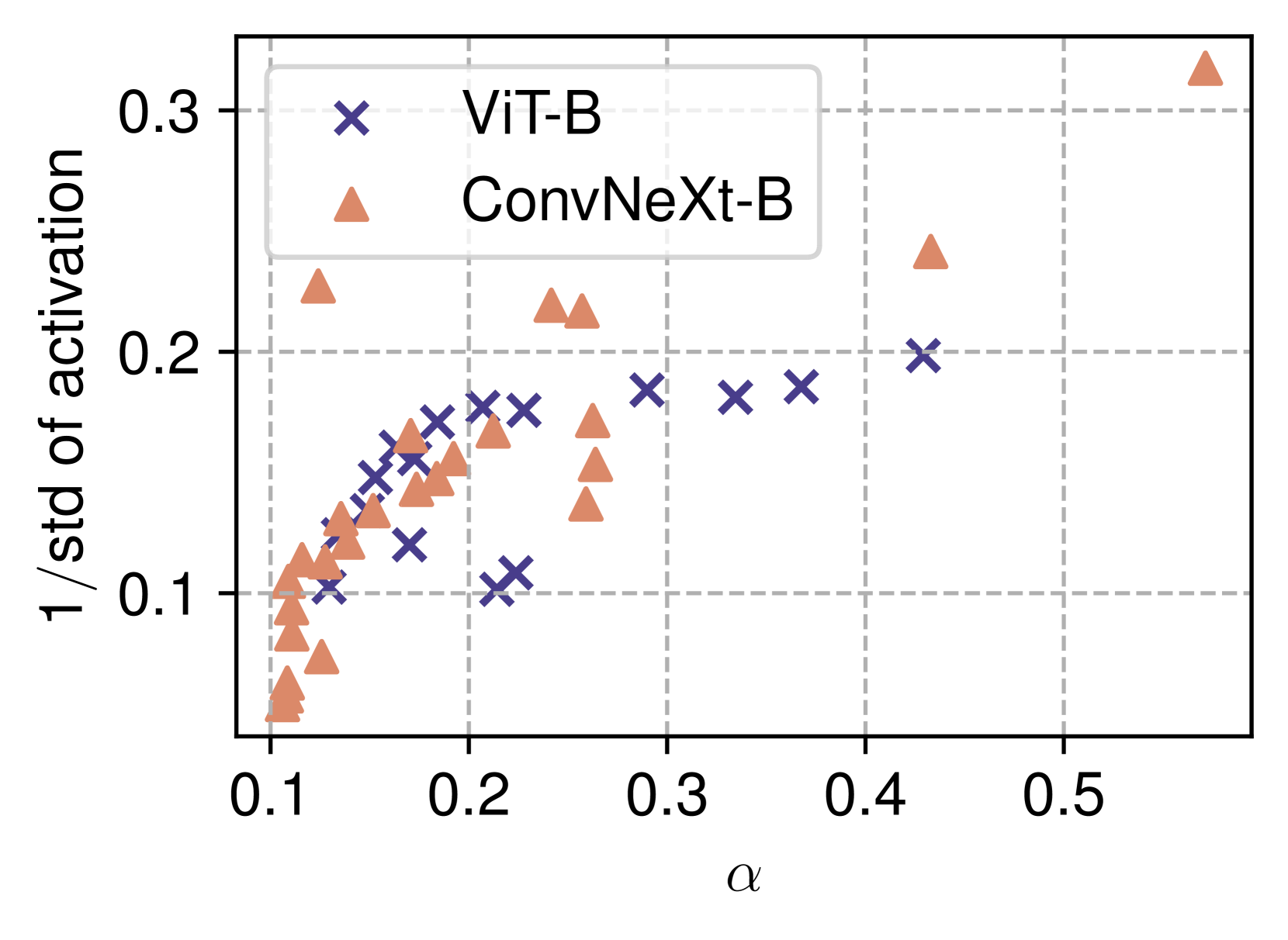\n*Figure 9: Scatter plot showing the relationship between learned α values and the inverse standard deviation of activations across different layers in ViT-B and ConvNeXt-B models.*\n\n## Comparing with Other Approaches\n\nThe researchers compared DyT with other methods proposed for training deep networks without normalization, including Fixup, SkipInit, and σReparam. Across various tasks and model architectures, DyT consistently outperformed these alternatives.\n\nThey also conducted ablation studies to validate the importance of both the tanh function and the learnable scale parameter α. These studies showed that:\n\n1. Replacing tanh with other functions like sigmoid or hardtanh led to reduced performance, highlighting the importance of tanh's specific properties.\n\n2. Using a fixed α instead of a learnable one significantly degraded performance, demonstrating the importance of adaptivity.\n\n3. Completely removing the non-linearity (using just a learnable scale) led to training instability, indicating that the bounded nature of tanh is crucial.\n\nThe impact of initial α values on model performance was also studied across different tasks:\n\n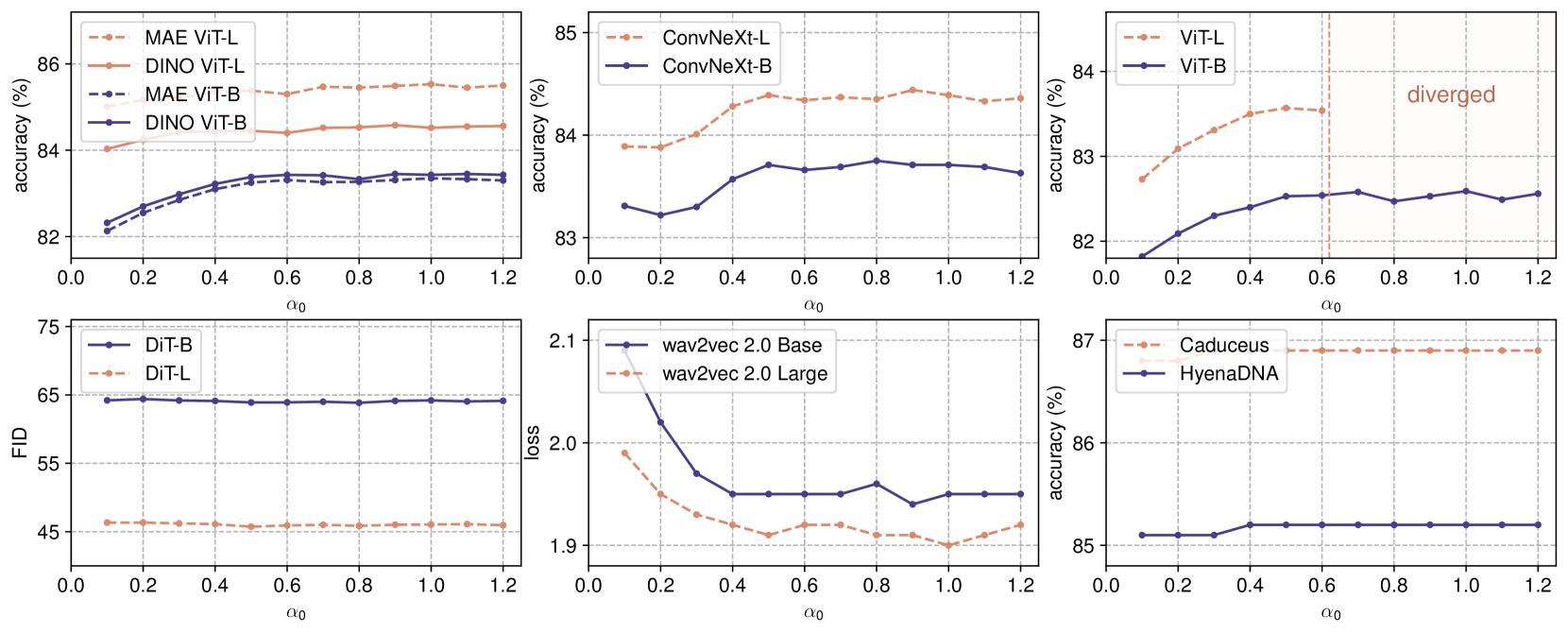\n*Figure 10: Performance of various models with different α initialization values (α₀), showing task-dependent sensitivity.*\n\n## Implications and Applications\n\nThe findings of this research have several important implications:\n\n1. **Architectural Simplification**: By replacing normalization layers with DyT, Transformer architectures can be simplified, potentially leading to more interpretable models.\n\n2. **Computational Efficiency**: Preliminary measurements suggest that DyT can improve training and inference speed compared to normalization layers, as it eliminates the need to compute statistics.\n\n3. **Theoretical Understanding**: The success of DyT provides insights into the fundamental role of normalization in deep learning, suggesting that the key benefit may be the S-shaped transformation rather than the normalization of statistics per se.\n\n4. **Cross-Domain Applicability**: The consistent success of DyT across diverse domains (vision, language, speech, biology) suggests it captures a fundamental principle of deep learning optimization.\n\nOne limitation noted by the authors is that DyT may not be directly applicable to classic CNN architectures that use batch normalization without further research. The focus of their work was primarily on Transformer architectures.\n\n## Conclusion\n\nThe paper \"Transformers without Normalization\" presents a significant contribution to deep learning architecture design by demonstrating that normalization layers in Transformers can be effectively replaced with a simple Dynamic Tanh (DyT) operation. This challenges the conventional wisdom that normalization layers are indispensable for training high-performance Transformers.\n\nThe proposed DyT approach offers a compelling alternative that is easy to implement, often requires minimal tuning, and can match or exceed the performance of normalized models across a wide range of tasks and domains. The finding that α in DyT learns to approximate the inverse of activation standard deviation provides insight into how this simple mechanism effectively mimics certain aspects of normalization.\n\nThis research opens new avenues for simplifying neural network architectures and may inspire further exploration of alternatives to traditional normalization techniques. As deep learning continues to evolve, such simplifications could contribute to more efficient and interpretable models.\n## Relevant Citations\n\n\n\nJimmy Lei Ba, Jamie Ryan Kiros, and Geoffrey E Hinton. [Layer normalization](https://alphaxiv.org/abs/1607.06450).arXiv preprint arXiv:1607.06450, 2016.\n\n * This paper introduces Layer Normalization (LN), a crucial component for stabilizing training in deep networks, especially Transformers. The paper analyzes LN's behavior and proposes Dynamic Tanh (DyT) as a replacement, making this citation highly relevant.\n\nAlexey Dosovitskiy, Lucas Beyer, Alexander Kolesnikov, Dirk Weissenborn, Xiaohua Zhai, Thomas Unterthiner, Mostafa Dehghani, Matthias Minderer, Georg Heigold, Sylvain Gelly, et al. An image is worth 16x16 words: Transformers for image recognition at scale.arXiv preprint arXiv:2010.11929, 2020.\n\n * This paper introduces the Vision Transformer (ViT), a prominent architecture used for benchmarking DyT's effectiveness in image classification tasks. The paper uses ViT as a core architecture to demonstrate that DyT can replace layer normalization.\n\nBiao Zhang and Rico Sennrich. [Root mean square layer normalization](https://alphaxiv.org/abs/1910.07467).NeurIPS, 2019.\n\n * This work introduces RMSNorm, an alternative to Layer Normalization, and is used as a baseline comparison for DyT, particularly in Large Language Model experiments. The paper explores DyT as a replacement for both LN and RMSNorm.\n\nHugo Touvron, Thibaut Lavril, Gautier Izacard, Xavier Martinet, Marie-Anne Lachaux, Timothée Lacroix, Baptiste Rozière, Naman Goyal, Eric Hambro, Faisal Azhar, et al. [Llama: Open and efficient foundation language models](https://alphaxiv.org/abs/2302.13971). arXiv preprint arXiv:2302.13971, 2023a.\n\n * This citation introduces the LLaMA language model, which serves as a key architecture for testing and evaluating DyT in the context of large language models. The paper uses LLaMA as an important architecture for verifying DyT's generalizability.\n\nAshish Vaswani, Noam Shazeer, Niki Parmar, Jakob Uszkoreit, Llion Jones, Aidan N Gomez, Łukasz Kaiser, and Illia Polosukhin. [Attention is all you need](https://alphaxiv.org/abs/1706.03762).NeurIPS, 2017.\n\n * This foundational paper introduces the Transformer architecture, which is the primary focus of the DyT study. The paper focuses on showing how DyT can improve Transformers.\n\n"])</script><script>self.__next_f.push([1,"8e:T42fc,"])</script><script>self.__next_f.push([1,"# Transformers sans Normalisation : Une Alternative Simple avec Tangente Hyperbolique Dynamique\n\n## Table des matières\n- [Introduction](#introduction)\n- [Comprendre les Couches de Normalisation](#comprendre-les-couches-de-normalisation)\n- [La Solution de la Tangente Hyperbolique Dynamique](#la-solution-de-la-tangente-hyperbolique-dynamique)\n- [Comment fonctionne DyT](#comment-fonctionne-dyt)\n- [Preuves Expérimentales](#preuves-experimentales)\n- [Réglage et Évolutivité](#reglage-et-evolutivite)\n- [Analyse du Paramètre Alpha](#analyse-du-parametre-alpha)\n- [Comparaison avec d'Autres Approches](#comparaison-avec-dautres-approches)\n- [Implications et Applications](#implications-et-applications)\n- [Conclusion](#conclusion)\n\n## Introduction\n\nLes couches de normalisation sont considérées comme des composants essentiels dans les réseaux de neurones modernes, particulièrement dans les architectures Transformer qui dominent le traitement du langage naturel, la vision par ordinateur et d'autres domaines. La normalisation des couches (LN) et ses variantes sont omniprésentes dans les Transformers, considérées comme cruciales pour stabiliser l'entraînement et améliorer les performances. Cependant, un nouvel article de chercheurs de Meta AI, NYU, MIT et de l'Université de Princeton remet en question cette hypothèse fondamentale en démontrant que les Transformers peuvent atteindre des performances équivalentes ou meilleures sans les couches de normalisation traditionnelles.\n\n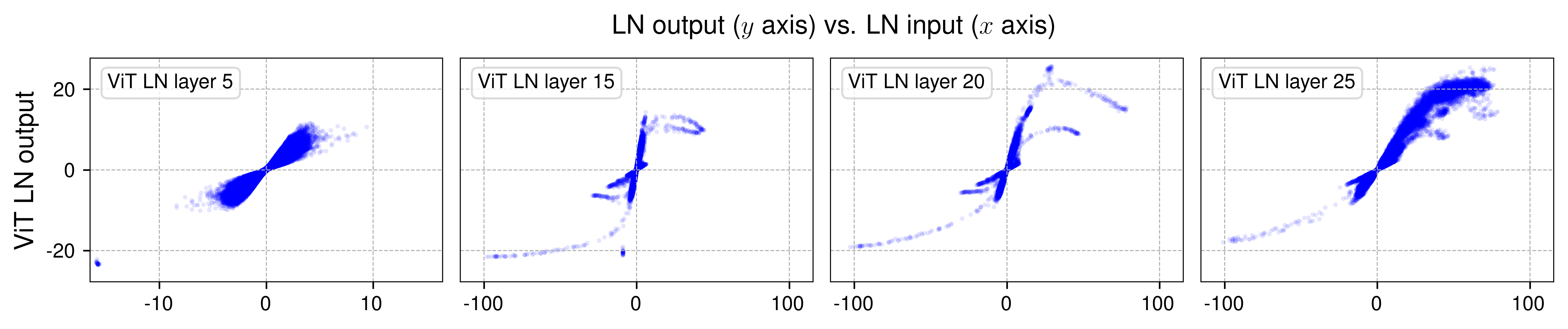\n*Figure 1 : Visualisation du comportement entrée-sortie de la normalisation des couches dans diverses couches ViT, montrant des relations en forme de S, similaires à tanh.*\n\n## Comprendre les Couches de Normalisation\n\nLes techniques de normalisation comme la normalisation par lots, la normalisation des couches et RMSNorm sont devenues une pratique standard en apprentissage profond. Ces méthodes normalisent généralement les activations en calculant des statistiques (moyenne et/ou écart-type) à travers des dimensions spécifiées, aidant à stabiliser l'entraînement en contrôlant la distribution des activations du réseau.\n\nDans les Transformers spécifiquement, la normalisation des couches fonctionne en calculant la moyenne et l'écart-type à travers la dimension des caractéristiques pour chaque token ou position. Ce processus de normalisation est coûteux en calcul car il nécessite de calculer ces statistiques à chaque couche pendant l'entraînement et l'inférence.\n\nLes auteurs ont observé que la normalisation des couches produit souvent des mappages entrée-sortie en forme de S similaires à tanh, comme montré dans la Figure 1. Cette observation a conduit à leur intuition clé : peut-être que l'effet bénéfique de la normalisation pourrait être obtenu par un mécanisme plus simple qui imite ce comportement en forme de S sans calculer de statistiques d'activation.\n\n## La Solution de la Tangente Hyperbolique Dynamique\n\nLes chercheurs proposent la Tangente Hyperbolique Dynamique (DyT) comme remplacement direct des couches de normalisation. DyT est définie comme :\n\n```\nDyT(x) = tanh(αx)\n```\n\nOù α est un paramètre apprenable qui contrôle la pente de la fonction tanh. Cette formulation simple élimine le besoin de calculer des statistiques d'activation tout en préservant la transformation en forme de S qui semble être importante pour la performance des Transformers.\n\n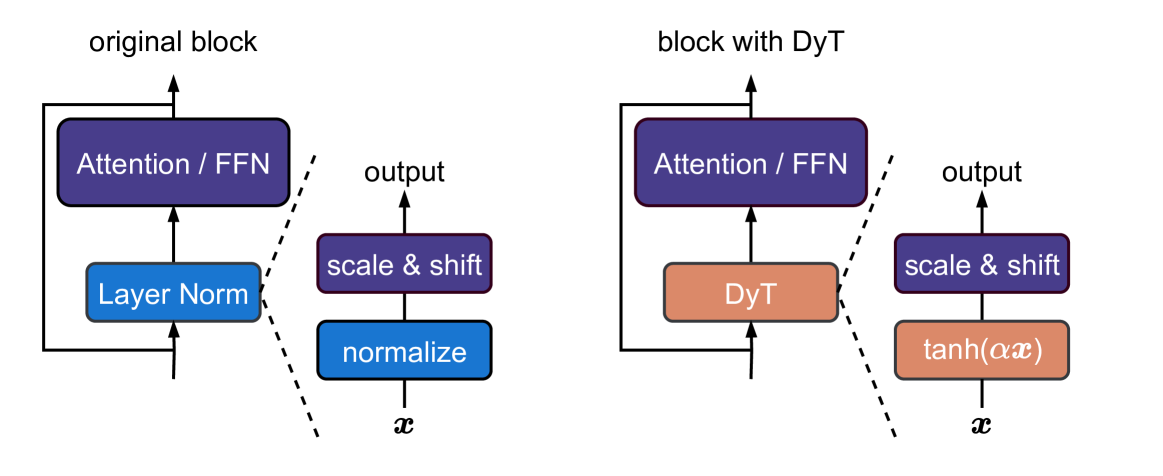\n*Figure 2 : À gauche : Bloc Transformer original avec normalisation des couches. À droite : Bloc proposé avec remplacement par Tangente Hyperbolique Dynamique (DyT).*\n\nLa beauté de cette approche réside dans sa simplicité - remplacer des opérations de normalisation complexes par une seule opération élément par élément avec un paramètre apprenable. La Figure 2 montre comment le bloc Transformer traditionnel avec normalisation des couches se compare au bloc proposé avec DyT.\n\n## Comment DyT Fonctionne\n\nLa Tangente Hyperbolique Dynamique fonctionne à travers deux mécanismes clés :\n\n1. **Compression des Valeurs** : La fonction tanh compresse les valeurs extrêmes, fournissant une forme de régularisation implicite similaire aux couches de normalisation. Cela empêche les activations de devenir trop grandes pendant les passes avant et arrière.\n\n2. **Mise à l'échelle adaptative** : Le paramètre apprenable α ajuste la pente de la fonction tanh, permettant au réseau de contrôler l'agressivité de l'écrasement des valeurs. Cette adaptabilité est cruciale pour la performance.\n\nLa fonction tangente hyperbolique (tanh) est bornée entre -1 et 1, écrasant toute valeur d'entrée dans cet intervalle. La pente de cet écrasement est contrôlée par α :\n\n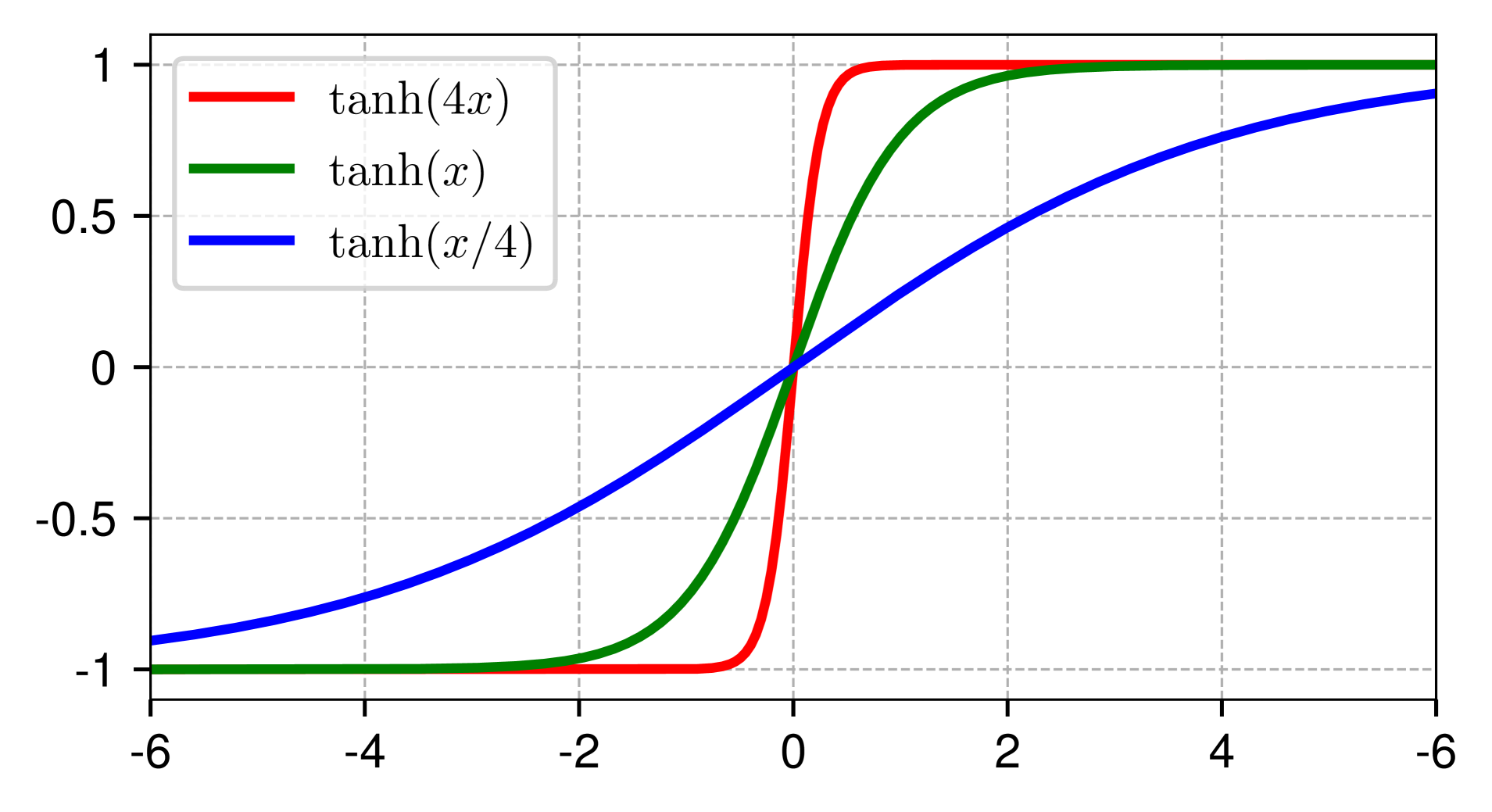\n*Figure 3 : La fonction tanh avec différentes valeurs de α, montrant comment des valeurs plus élevées de α créent des transitions plus nettes.*\n\nComme montré dans la Figure 3, une valeur α plus grande rend la transition de -1 à 1 plus nette, tandis qu'une valeur α plus petite la rend plus graduelle. Cette flexibilité permet au réseau d'ajuster le degré d'écrasement des valeurs en fonction de la tâche et de la profondeur de la couche.\n\n## Preuves expérimentales\n\nLes chercheurs ont mené des expériences approfondies sur diverses tâches et domaines pour valider l'efficacité de DyT comme remplacement des couches de normalisation. Ces expériences incluaient :\n\n1. **Tâches de vision** :\n - Classification ImageNet avec Vision Transformers (ViT) et ConvNeXt\n - Apprentissage auto-supervisé avec MAE et DINO\n\n2. **Modèles génératifs** :\n - Modèles de diffusion pour la génération d'images (DiT)\n\n3. **Grands modèles de langage** :\n - Pré-entraînement LLaMA à des échelles de 7B à 70B paramètres\n\n4. **Autres domaines** :\n - Traitement de la parole avec wav2vec 2.0\n - Modélisation de séquences ADN avec HyenaDNA et Caduceus\n\nLes résultats ont systématiquement montré que les Transformers avec DyT pouvaient égaler ou dépasser les performances de leurs homologues normalisés. Par exemple, avec les Vision Transformers sur la classification ImageNet, la variante DyT a atteint une précision comparable à la version LN :\n\n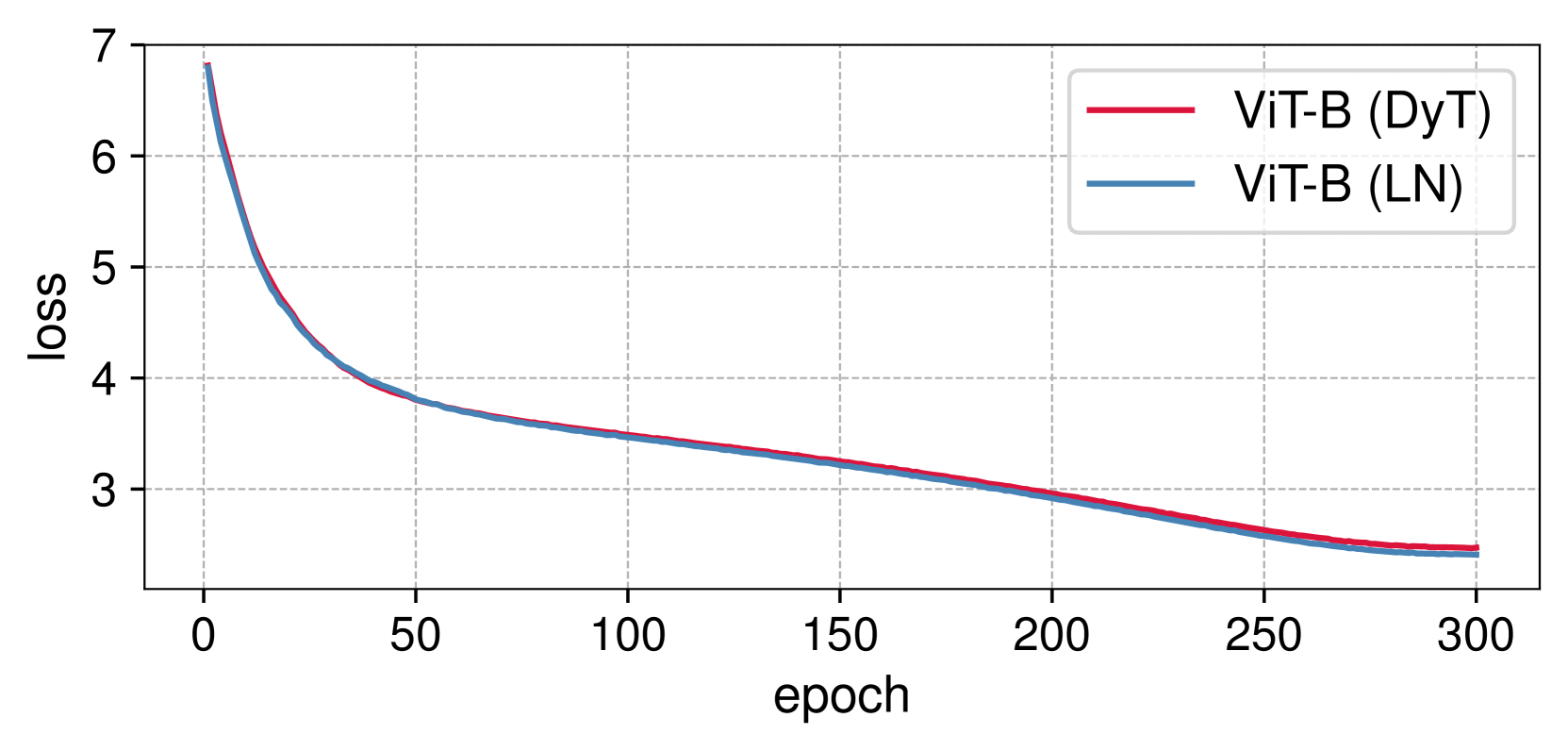\n*Figure 4 : Courbes de perte d'entraînement pour ViT-B avec Layer Normalization (LN) et Dynamic Tanh (DyT), montrant une convergence presque identique.*\n\nDe même, pour les modèles LLaMA de différentes tailles (7B à 70B paramètres), les variantes DyT ont atteint des valeurs de perte comparables ou légèrement meilleures par rapport aux modèles RMSNorm :\n\n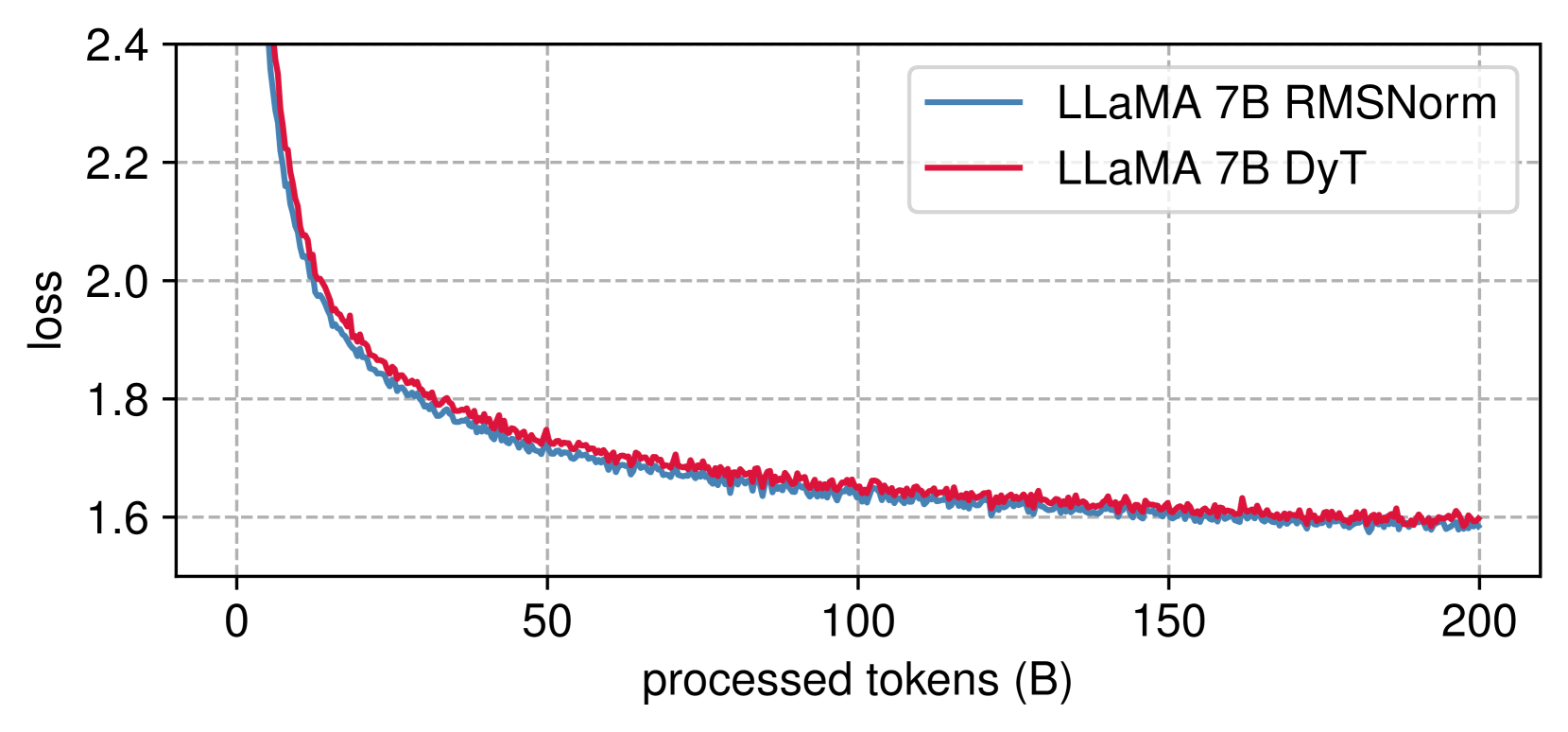\n*Figure 5 : Courbes de perte d'entraînement pour LLaMA 7B avec RMSNorm et DyT, montrant des performances comparables.*\n\n## Réglage et Extensibilité\n\nBien que DyT soit généralement robuste et fonctionne bien avec un réglage minimal, les chercheurs ont constaté que pour les modèles plus grands, particulièrement les Grands Modèles de Langage (LLMs), une initialisation soigneuse de α est importante. Ils ont mené une exploration approfondie des valeurs d'initialisation pour l'architecture LLaMA :\n\n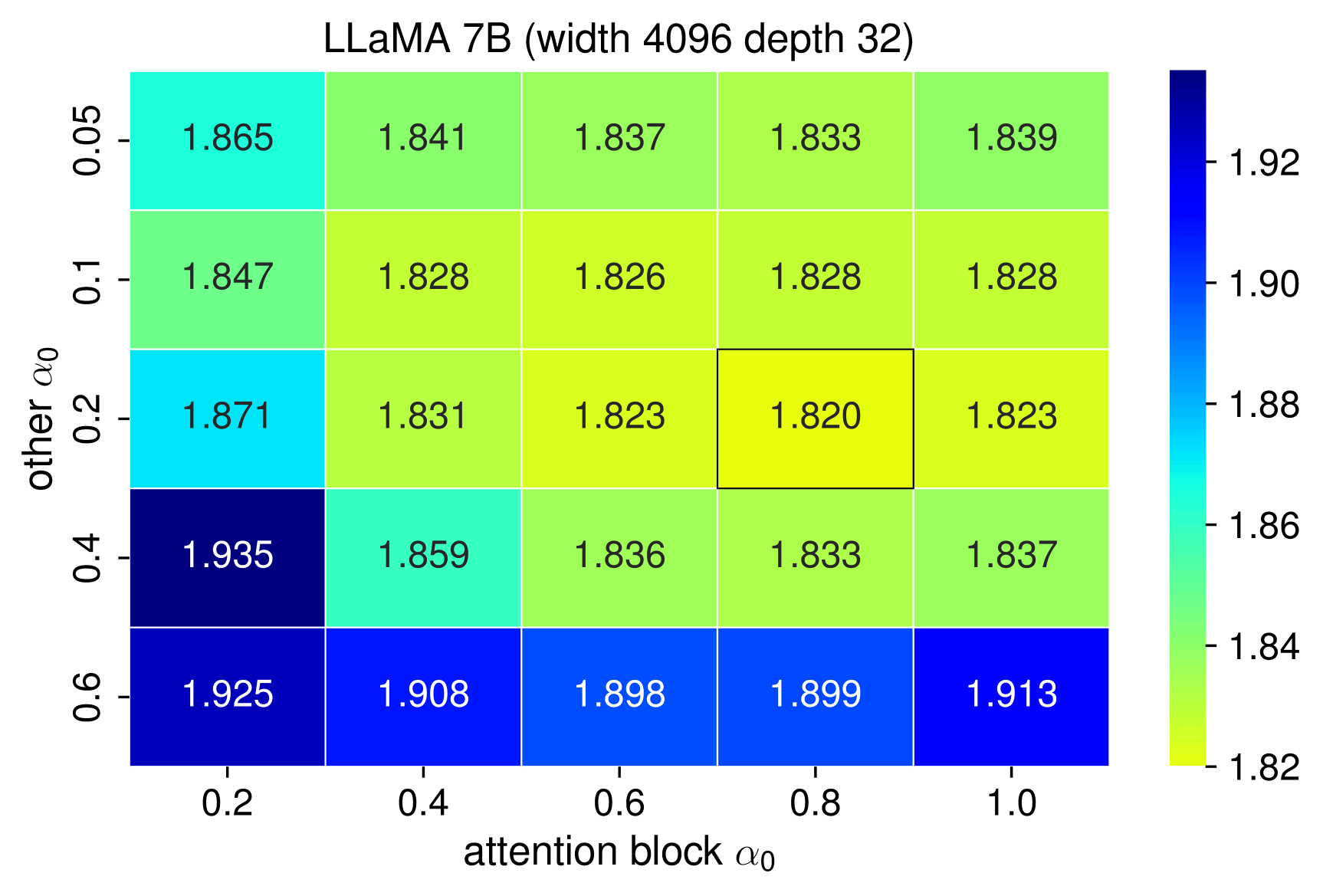\n*Figure 6 : Carte thermique montrant la performance de LLaMA 7B avec différentes valeurs d'initialisation de α pour les blocs d'attention et les blocs feed-forward.*\n\nPour LLaMA 7B, l'initialisation optimale de α s'est avérée être 0,2 pour les blocs d'attention et 0,2 pour les autres blocs, tandis que pour LLaMA 13B, elle était de 0,6 pour les blocs d'attention et 0,15 pour les autres blocs. Cela suggère que les modèles plus grands peuvent nécessiter un réglage plus minutieux du paramètre α.\n\nLes chercheurs ont également testé l'extensibilité de leur approche en entraînant des modèles de différentes profondeurs et largeurs :\n\n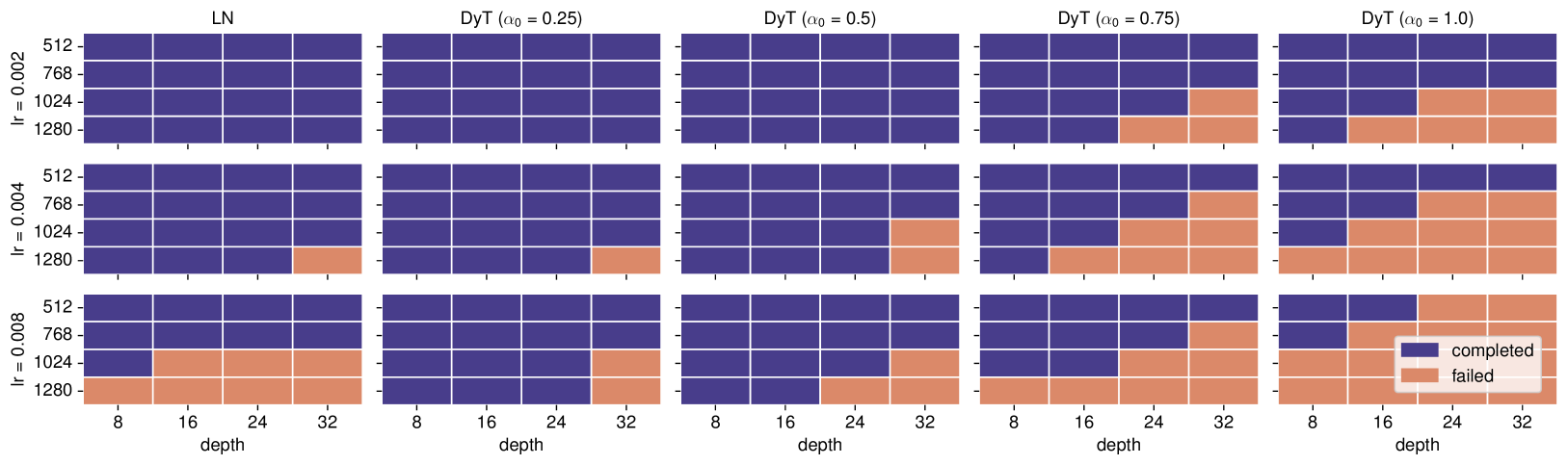\n*Figure 7 : Comparaison de la stabilité d'entraînement entre LN et DyT pour différentes profondeurs et largeurs de modèle, le bleu indiquant un entraînement réussi et l'orange indiquant une instabilité.*\n\nLes résultats ont montré que les modèles DyT pouvaient s'étendre de manière comparable aux modèles LN, bien qu'avec une sensibilité supplémentaire au taux d'apprentissage à plus grande échelle.\n\n## Analyse du Paramètre Alpha\n\nLes chercheurs ont analysé comment le paramètre α dans DyT est lié aux propriétés statistiques des activations. De façon intéressante, ils ont découvert que α apprend à approximer l'inverse de l'écart-type des activations de couche :\n\n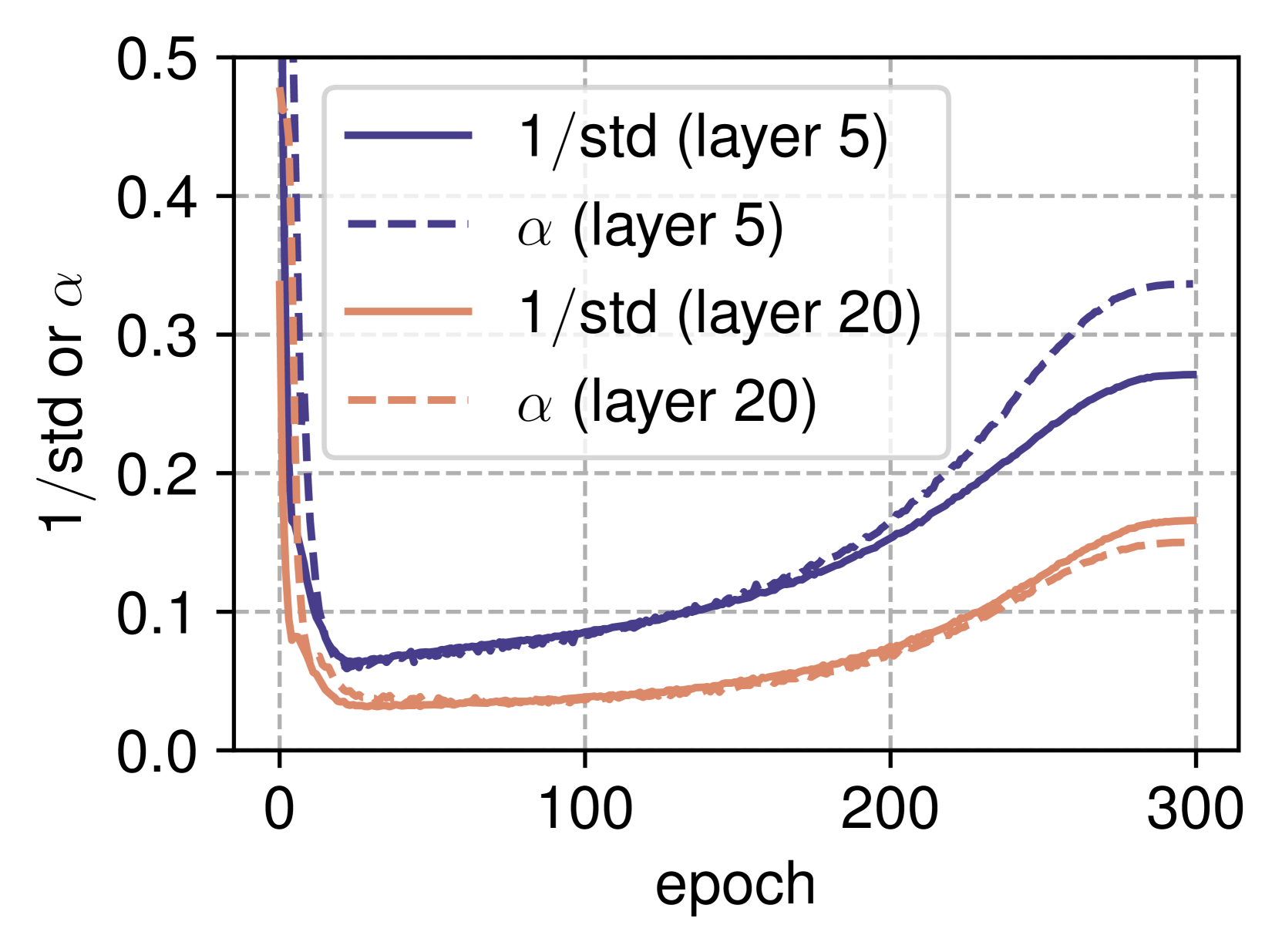\n*Figure 8 : Comparaison entre les valeurs α apprises et l'inverse de l'écart-type des activations (1/std) au cours des époques d'entraînement, montrant comment α imite partiellement le comportement de la normalisation.*\n\nCette découverte suggère que DyT apprend implicitement à effectuer une forme de mise à l'échelle adaptative similaire aux couches de normalisation, mais sans calculer explicitement de statistiques. Le paramètre α tend à être inversement proportionnel à l'écart-type des activations, mettant efficacement à l'échelle les entrées de manière à ce que leur magnitude soit appropriée pour la fonction tanh.\n\nDe plus, ils ont observé une corrélation constante entre les valeurs α apprises et l'inverse de l'écart-type des activations à travers différentes couches et modèles :\n\n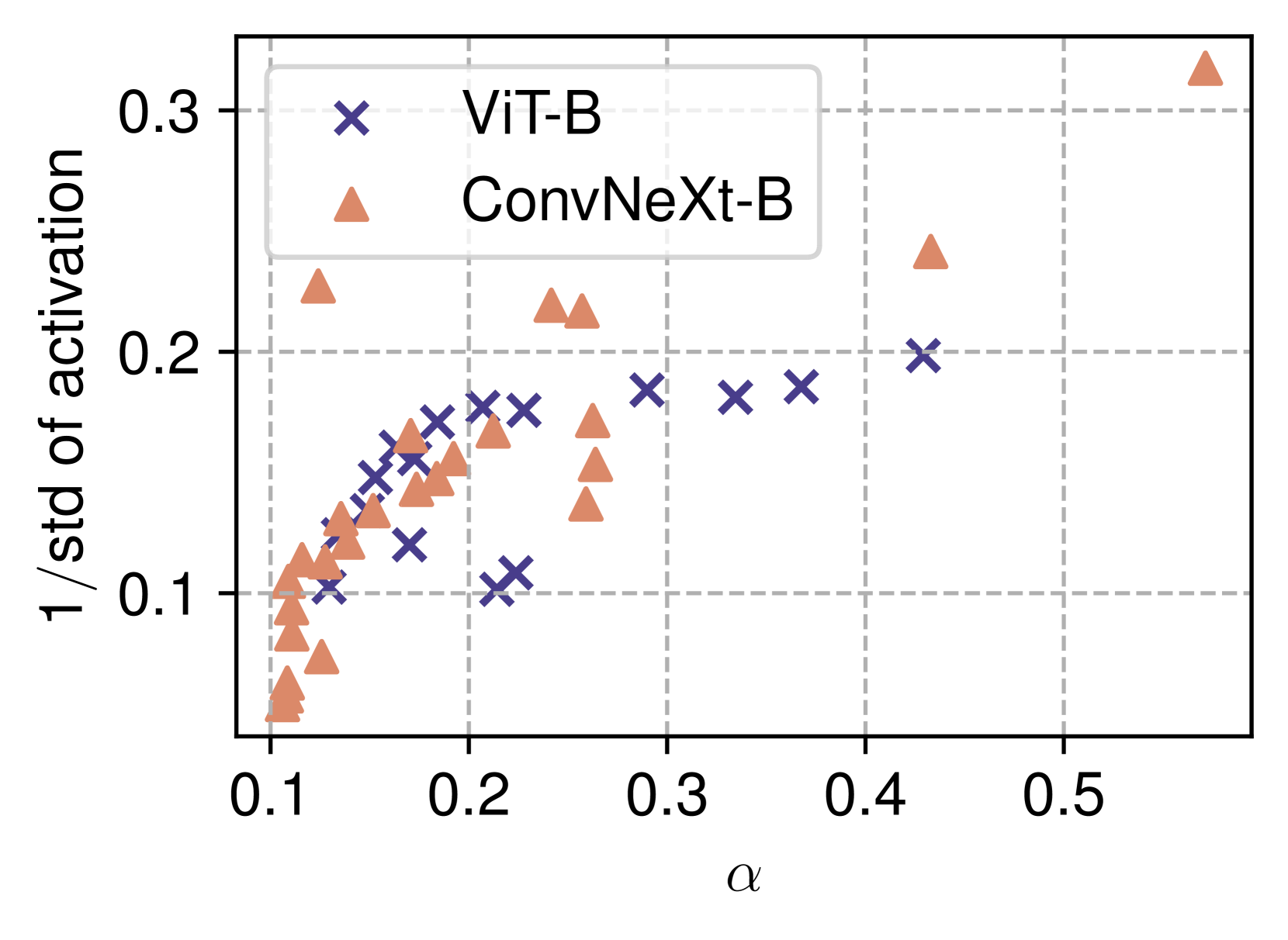\n*Figure 9 : Nuage de points montrant la relation entre les valeurs α apprises et l'inverse de l'écart-type des activations à travers différentes couches dans les modèles ViT-B et ConvNeXt-B.*\n\n## Comparaison avec d'autres approches\n\nLes chercheurs ont comparé DyT avec d'autres méthodes proposées pour entraîner des réseaux profonds sans normalisation, notamment Fixup, SkipInit et σReparam. À travers diverses tâches et architectures de modèles, DyT a systématiquement surpassé ces alternatives.\n\nIls ont également mené des études d'ablation pour valider l'importance à la fois de la fonction tanh et du paramètre d'échelle apprenable α. Ces études ont montré que :\n\n1. Remplacer tanh par d'autres fonctions comme sigmoid ou hardtanh a conduit à une réduction des performances, soulignant l'importance des propriétés spécifiques de tanh.\n\n2. Utiliser un α fixe au lieu d'un α apprenable a significativement dégradé les performances, démontrant l'importance de l'adaptabilité.\n\n3. Supprimer complètement la non-linéarité (en utilisant juste une échelle apprenable) a conduit à une instabilité de l'entraînement, indiquant que la nature bornée de tanh est cruciale.\n\nL'impact des valeurs initiales de α sur les performances du modèle a également été étudié à travers différentes tâches :\n\n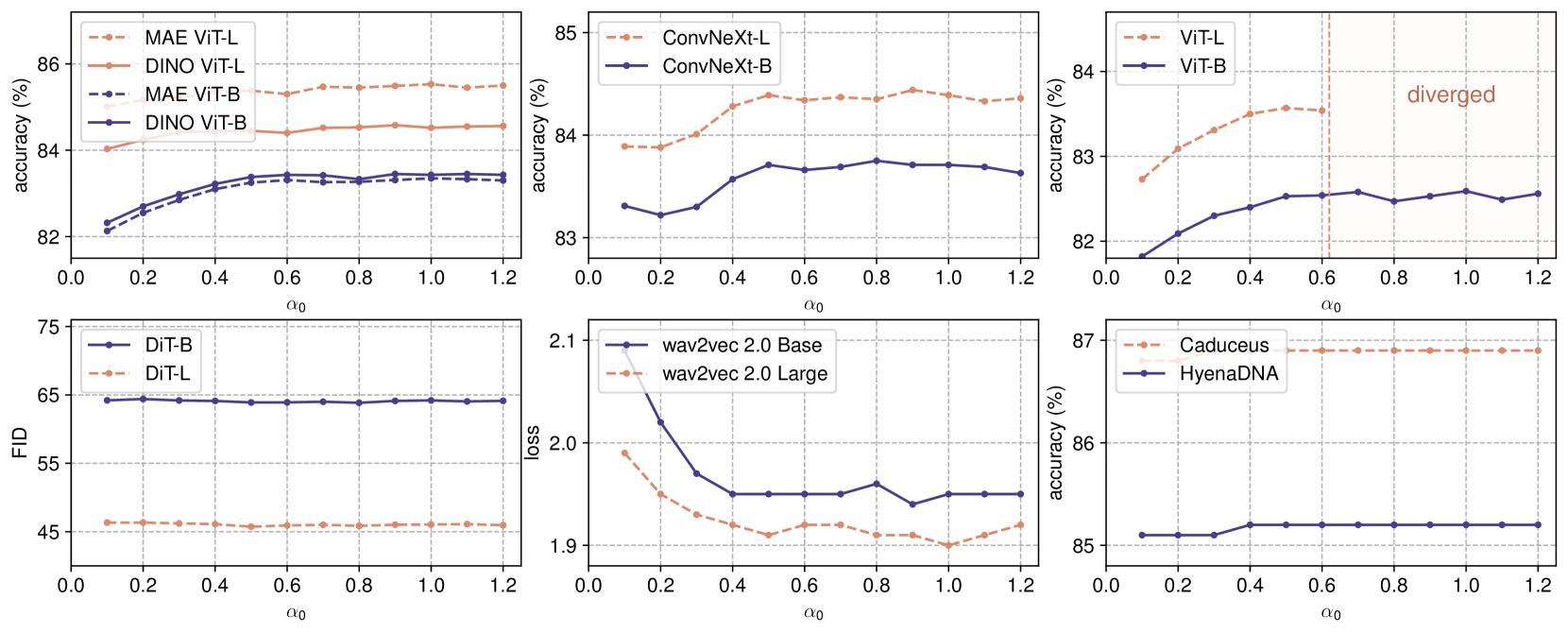\n*Figure 10 : Performance de divers modèles avec différentes valeurs d'initialisation de α (α₀), montrant une sensibilité dépendante de la tâche.*\n\n## Implications et applications\n\nLes résultats de cette recherche ont plusieurs implications importantes :\n\n1. **Simplification architecturale** : En remplaçant les couches de normalisation par DyT, les architectures Transformer peuvent être simplifiées, conduisant potentiellement à des modèles plus interprétables.\n\n2. **Efficacité computationnelle** : Les mesures préliminaires suggèrent que DyT peut améliorer la vitesse d'entraînement et d'inférence par rapport aux couches de normalisation, car il élimine le besoin de calculer des statistiques.\n\n3. **Compréhension théorique** : Le succès de DyT fournit des aperçus sur le rôle fondamental de la normalisation dans l'apprentissage profond, suggérant que l'avantage clé pourrait être la transformation en forme de S plutôt que la normalisation des statistiques en soi.\n\n4. **Applicabilité multi-domaines** : Le succès constant de DyT à travers divers domaines (vision, langage, parole, biologie) suggère qu'il capture un principe fondamental de l'optimisation de l'apprentissage profond.\n\nUne limitation notée par les auteurs est que DyT pourrait ne pas être directement applicable aux architectures CNN classiques qui utilisent la normalisation par lots sans recherche supplémentaire. Leur travail s'est principalement concentré sur les architectures Transformer.\n\n## Conclusion\n\nL'article \"Transformers without Normalization\" présente une contribution significative à la conception d'architecture d'apprentissage profond en démontrant que les couches de normalisation dans les Transformers peuvent être efficacement remplacées par une simple opération Dynamic Tanh (DyT). Cela remet en question la sagesse conventionnelle selon laquelle les couches de normalisation sont indispensables pour entraîner des Transformers haute performance.\n\nL'approche DyT proposée offre une alternative convaincante qui est facile à mettre en œuvre, nécessite souvent un réglage minimal et peut égaler ou dépasser les performances des modèles normalisés dans un large éventail de tâches et de domaines. La découverte que α dans DyT apprend à approximer l'inverse de l'écart-type d'activation fournit un aperçu de la façon dont ce mécanisme simple imite efficacement certains aspects de la normalisation.\n\nCette recherche ouvre de nouvelles voies pour simplifier les architectures de réseaux neuronaux et pourrait inspirer une exploration plus approfondie des alternatives aux techniques de normalisation traditionnelles. Alors que l'apprentissage profond continue d'évoluer, de telles simplifications pourraient contribuer à des modèles plus efficaces et interprétables.\n\n## Citations Pertinentes\n\nJimmy Lei Ba, Jamie Ryan Kiros, et Geoffrey E Hinton. [Layer normalization](https://alphaxiv.org/abs/1607.06450). arXiv preprint arXiv:1607.06450, 2016.\n\n * Cet article introduit la normalisation des couches (LN), un composant crucial pour stabiliser l'entraînement dans les réseaux profonds, en particulier les Transformers. L'article analyse le comportement de LN et propose Dynamic Tanh (DyT) comme remplacement, rendant cette citation hautement pertinente.\n\nAlexey Dosovitskiy, Lucas Beyer, Alexander Kolesnikov, Dirk Weissenborn, Xiaohua Zhai, Thomas Unterthiner, Mostafa Dehghani, Matthias Minderer, Georg Heigold, Sylvain Gelly, et al. An image is worth 16x16 words: Transformers for image recognition at scale. arXiv preprint arXiv:2010.11929, 2020.\n\n * Cet article introduit le Vision Transformer (ViT), une architecture majeure utilisée pour évaluer l'efficacité de DyT dans les tâches de classification d'images. L'article utilise ViT comme architecture principale pour démontrer que DyT peut remplacer la normalisation des couches.\n\nBiao Zhang et Rico Sennrich. [Root mean square layer normalization](https://alphaxiv.org/abs/1910.07467). NeurIPS, 2019.\n\n * Ce travail introduit RMSNorm, une alternative à la normalisation des couches, et est utilisé comme comparaison de référence pour DyT, particulièrement dans les expériences sur les grands modèles de langage. L'article explore DyT comme remplacement pour LN et RMSNorm.\n\nHugo Touvron, Thibaut Lavril, Gautier Izacard, Xavier Martinet, Marie-Anne Lachaux, Timothée Lacroix, Baptiste Rozière, Naman Goyal, Eric Hambro, Faisal Azhar, et al. [Llama: Open and efficient foundation language models](https://alphaxiv.org/abs/2302.13971). arXiv preprint arXiv:2302.13971, 2023a.\n\n * Cette citation introduit le modèle de langage LLaMA, qui sert d'architecture clé pour tester et évaluer DyT dans le contexte des grands modèles de langage. L'article utilise LLaMA comme architecture importante pour vérifier la généralisabilité de DyT.\n\nAshish Vaswani, Noam Shazeer, Niki Parmar, Jakob Uszkoreit, Llion Jones, Aidan N Gomez, Łukasz Kaiser, et Illia Polosukhin. [Attention is all you need](https://alphaxiv.org/abs/1706.03762). NeurIPS, 2017.\n\n * Cet article fondamental introduit l'architecture Transformer, qui est le focus principal de l'étude DyT. L'article se concentre sur la démonstration de la façon dont DyT peut améliorer les Transformers."])</script><script>self.__next_f.push([1,"8f:T435b,"])</script><script>self.__next_f.push([1,"# 正規化なしのTransformer:Dynamic Tanhによるシンプルな代替手法\n\n## 目次\n- [はじめに](#introduction)\n- [正規化層の理解](#understanding-normalization-layers)\n- [Dynamic Tanhソリューション](#the-dynamic-tanh-solution)\n- [DyTの仕組み](#how-dyt-works)\n- [実験的証拠](#experimental-evidence)\n- [チューニングとスケーラビリティ](#tuning-and-scalability)\n- [アルファパラメータの分析](#analysis-of-alpha-parameter)\n- [他のアプローチとの比較](#comparing-with-other-approaches)\n- [影響と応用](#implications-and-applications)\n- [結論](#conclusion)\n\n## はじめに\n\n正規化層は、現代のニューラルネットワーク、特に自然言語処理、コンピュータビジョン、その他の分野で支配的なTransformerアーキテクチャにおいて、不可欠な要素とされてきました。Layer Normalization(LN)とその変種は、Transformerにおいて遍在的であり、トレーニングの安定化とパフォーマンスの向上に不可欠だと考えられてきました。しかし、Meta AI、NYU、MIT、プリンストン大学の研究者による新しい論文は、従来の正規化層なしでもTransformerが同等以上のパフォーマンスを達成できることを実証し、この基本的な前提に挑戦しています。\n\n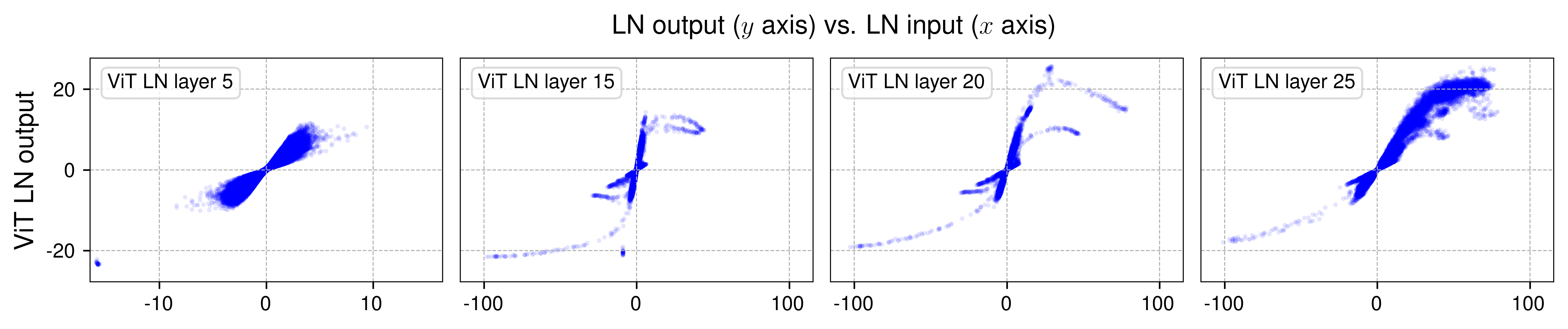\n*図1:様々なViT層におけるLayer Normalizationの入出力挙動の可視化。S字型のtanhに似た関係を示している。*\n\n## 正規化層の理解\n\nBatch Normalization、Layer Normalization、RMSNormなどの正規化手法は、ディープラーニングにおいて標準的な実践となっています。これらの手法は通常、指定された次元にわたって統計量(平均および/または標準偏差)を計算することで活性化を正規化し、ネットワーク活性化の分布を制御することでトレーニングを安定化させます。\n\n特にTransformerでは、Layer Normalizationは各トークンまたは位置の特徴次元にわたって平均と標準偏差を計算することで動作します。この正規化プロセスは、トレーニングと推論の両方で各層でこれらの統計量を計算する必要があるため、計算コストが高くなります。\n\n著者らは、図1に示すように、Layer Normalizationがしばしばtanhに似たS字型の入出力マッピングを生成することを観察しました。この観察から、正規化の有益な効果は、活性化統計量を計算することなく、このS字型の挙動を模倣するよりシンプルなメカニズムによって達成できるかもしれないという重要な洞察が得られました。\n\n## Dynamic Tanhソリューション\n\n研究者らは、正規化層の直接的な代替としてDynamic Tanh(DyT)を提案しています。DyTは以下のように定義されます:\n\n```\nDyT(x) = tanh(αx)\n```\n\nここでαは、tanh関数の傾きを制御する学習可能なパラメータです。この単純な定式化により、Transformerのパフォーマンスに重要と思われるS字型の変換を保持しながら、活性化統計量の計算の必要性を排除します。\n\n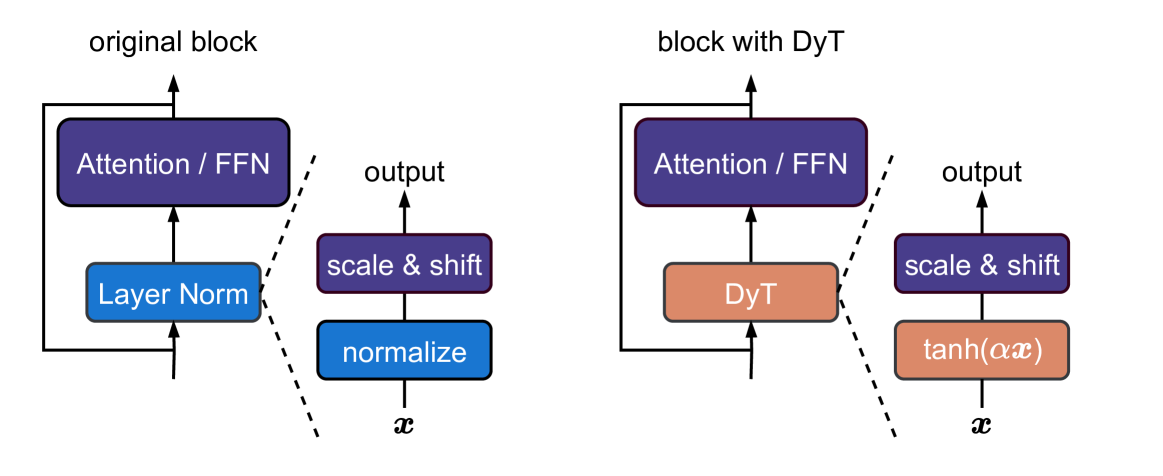\n*図2:左:Layer Normalizationを持つオリジナルのTransformerブロック。右:Dynamic Tanh(DyT)に置き換えられた提案ブロック。*\n\nこのアプローチの美しさは、複雑な正規化演算を、学習可能なパラメータを持つ単一の要素ごとの演算に置き換えるシンプルさにあります。図2は、従来のLayer Normalizationを持つTransformerブロックと、DyTを用いた提案ブロックの比較を示しています。\n\n## DyTの仕組み\n\nDynamic Tanhは2つの主要なメカニズムを通じて機能します:\n\n1. **値の圧縮**:tanh関数は極端な値を圧縮し、正規化層と同様の暗黙的な正則化を提供します。これにより、順伝播と逆伝播の過程で活性化が大きくなりすぎることを防ぎます。\n\n2. **適応的スケーリング**: 学習可能なパラメータαはtanh関数の急峻さを調整し、ネットワークが値の圧縮をどの程度積極的に行うかを制御できるようにします。この適応性は性能にとって重要です。\n\n双曲線正接関数(tanh)は-1から1の間に制限され、どのような入力値もこの範囲に圧縮します。この圧縮の急峻さはαによって制御されます:\n\n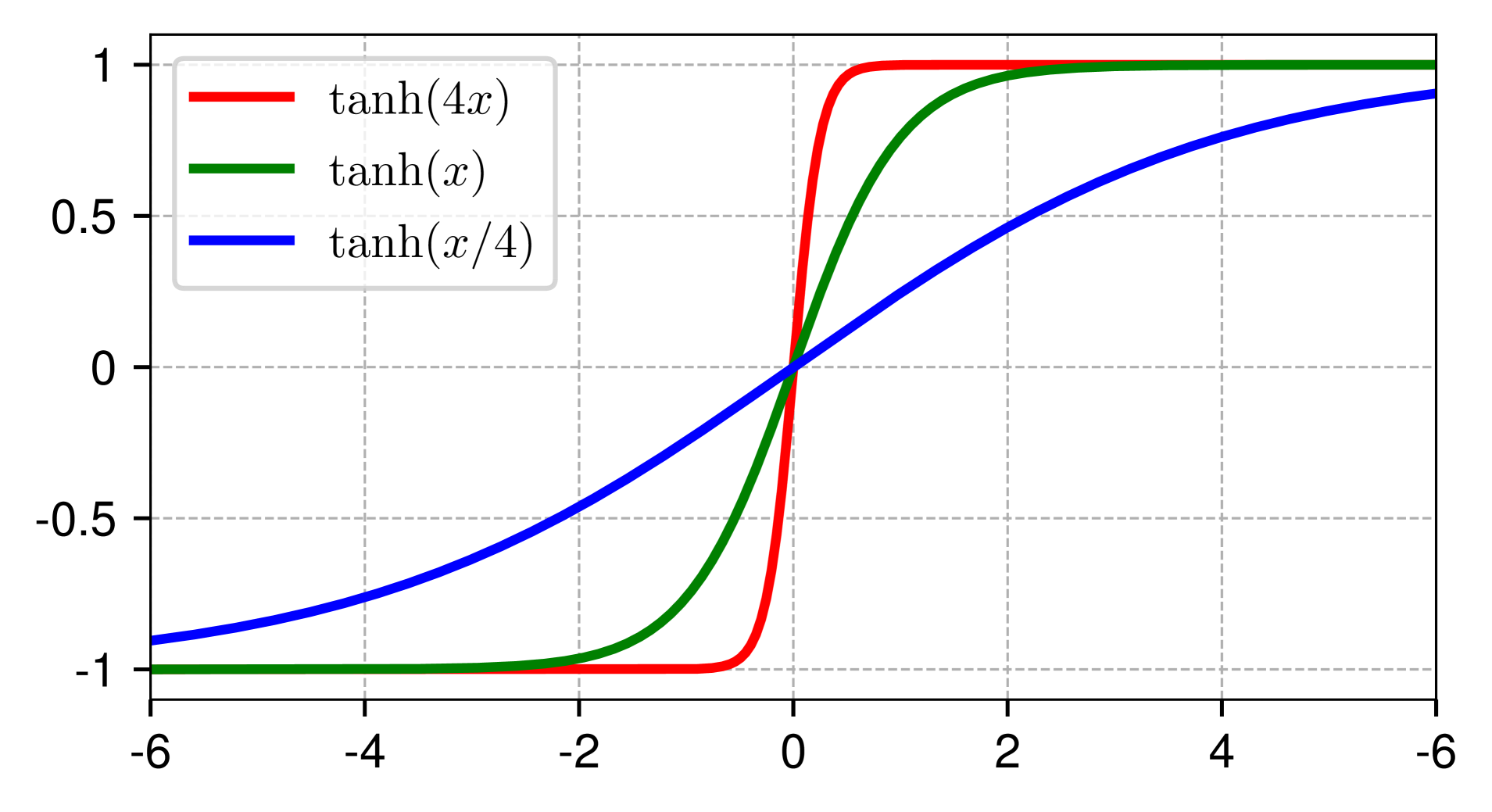\n*図3:異なるα値でのtanh関数。αの値が大きいほど、より鋭い遷移を示します。*\n\n図3に示すように、αの値が大きいほど-1から1への遷移が急峻になり、小さいほどより緩やかになります。この柔軟性により、ネットワークはタスクや層の深さに基づいて値の圧縮度を調整することができます。\n\n## 実験的証拠\n\n研究者たちは、DyTが正規化層の代替として有効であることを検証するため、様々なタスクとドメインにわたる広範な実験を行いました。これらの実験には以下が含まれます:\n\n1. **ビジョンタスク**:\n - Vision Transformer(ViT)とConvNeXtによるImageNet分類\n - MAEとDINOによる自己教師あり学習\n\n2. **生成モデル**:\n - 画像生成のための拡散モデル(DiT)\n\n3. **大規模言語モデル**:\n - 7Bから70BパラメータまでのスケールでのLLaMAの事前学習\n\n4. **その他のドメイン**:\n - wav2vec 2.0による音声処理\n - HyenaDNAとCaduceusによるDNA配列モデリング\n\n結果は一貫して、DyTを使用したTransformerが正規化されたモデルと同等かそれ以上の性能を示しました。例えば、ImageNet分類におけるVision Transformerでは、DyT変種はLNバージョンと同等の精度を達成しました:\n\n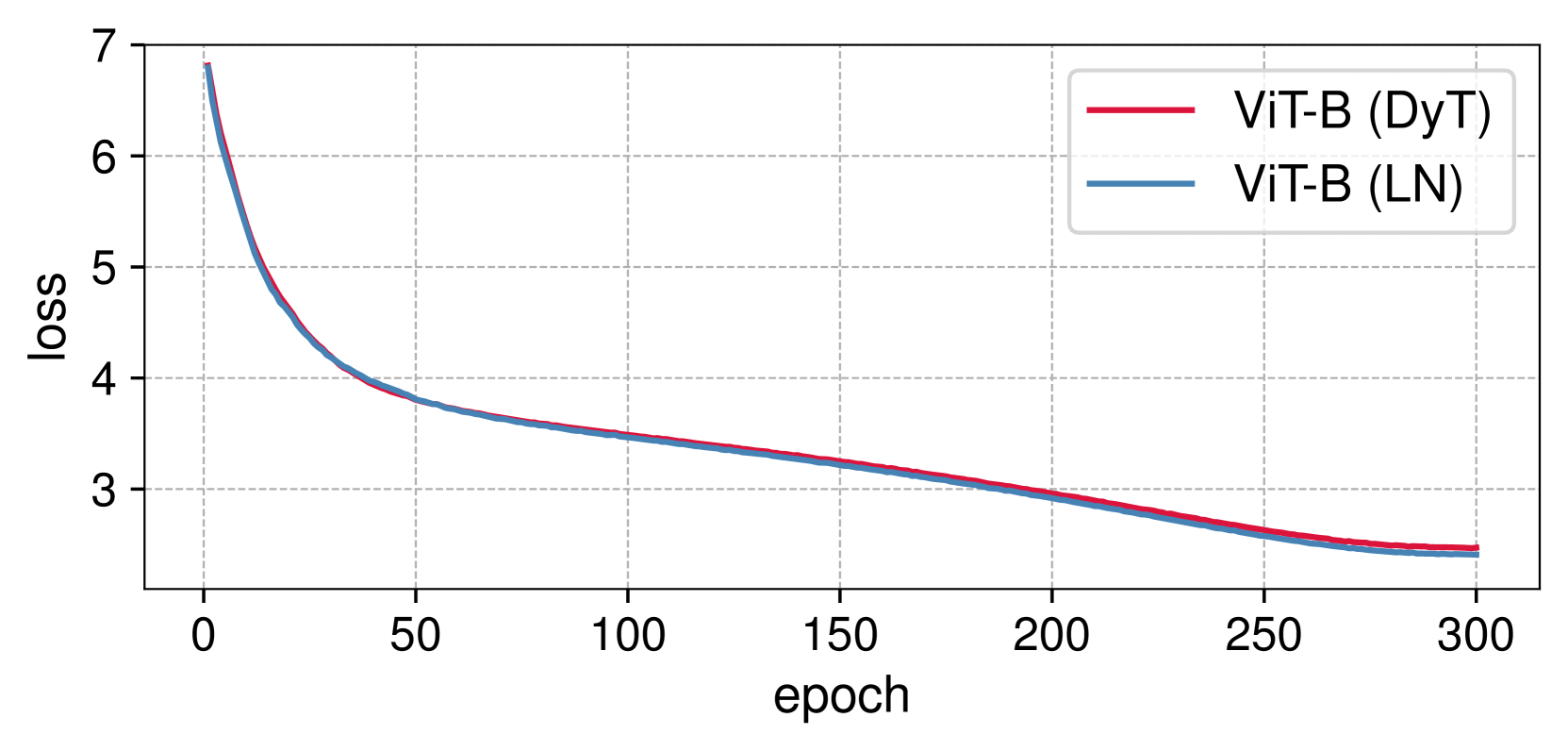\n*図4:Layer Normalization(LN)とDynamic Tanh(DyT)を使用したViT-Bのトレーニング損失曲線。ほぼ同一の収束を示しています。*\n\n同様に、様々なサイズ(7Bから70Bパラメータ)のLLaMaモデルにおいて、DyT変種はRMSNormモデルと比較して同等かやや良好な損失値を達成しました:\n\n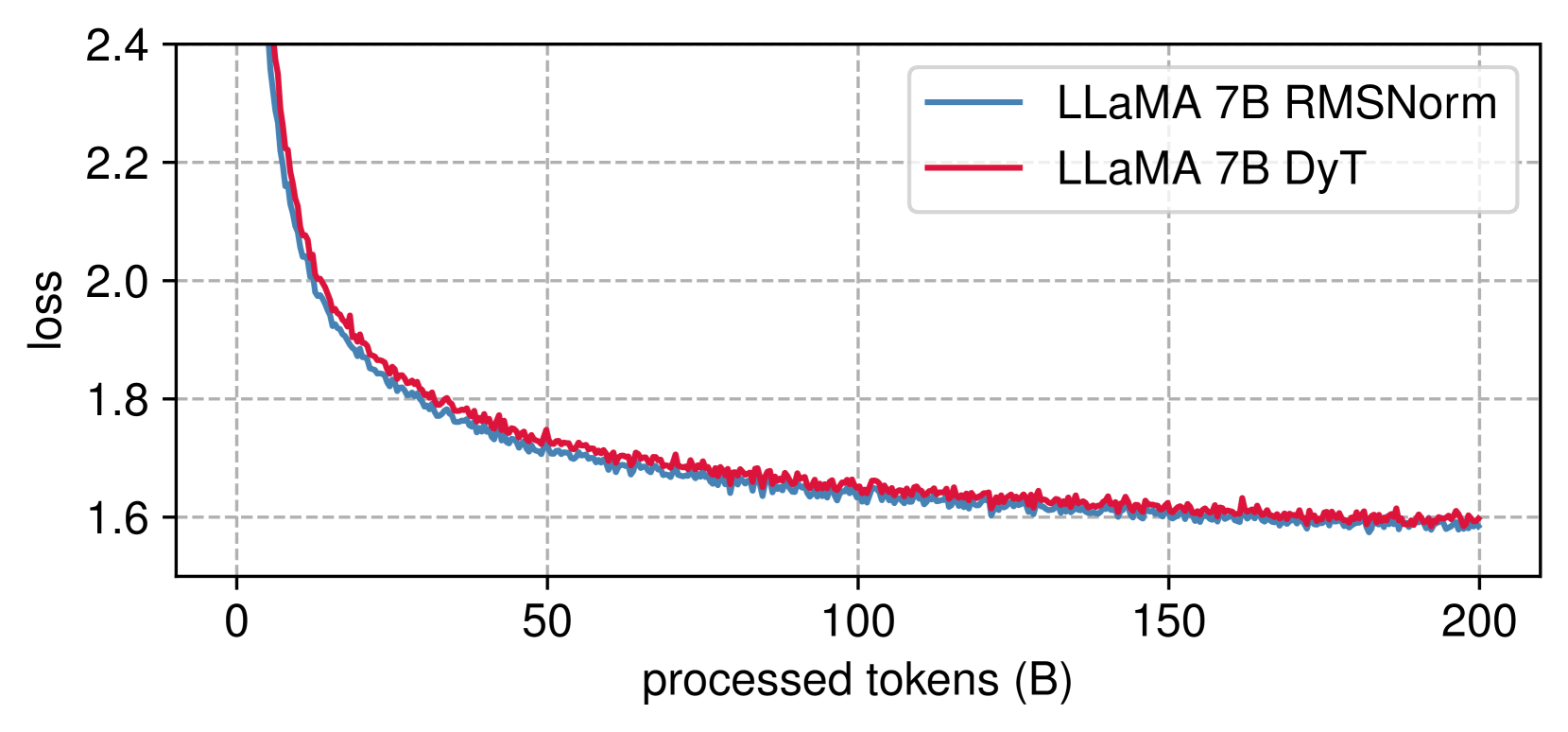\n*図5:RMSNormとDyTを使用したLLaMA 7Bのトレーニング損失曲線。同等の性能を示しています。*\n\n## チューニングとスケーラビリティ\n\nDyTは一般的に堅牢で最小限のチューニングで良好に機能しますが、研究者たちは、特に大規模言語モデル(LLM)などの大きなモデルでは、αの初期化が重要であることを発見しました。彼らはLLaMaアーキテクチャに対して詳細な初期化値の探索を行いました:\n\n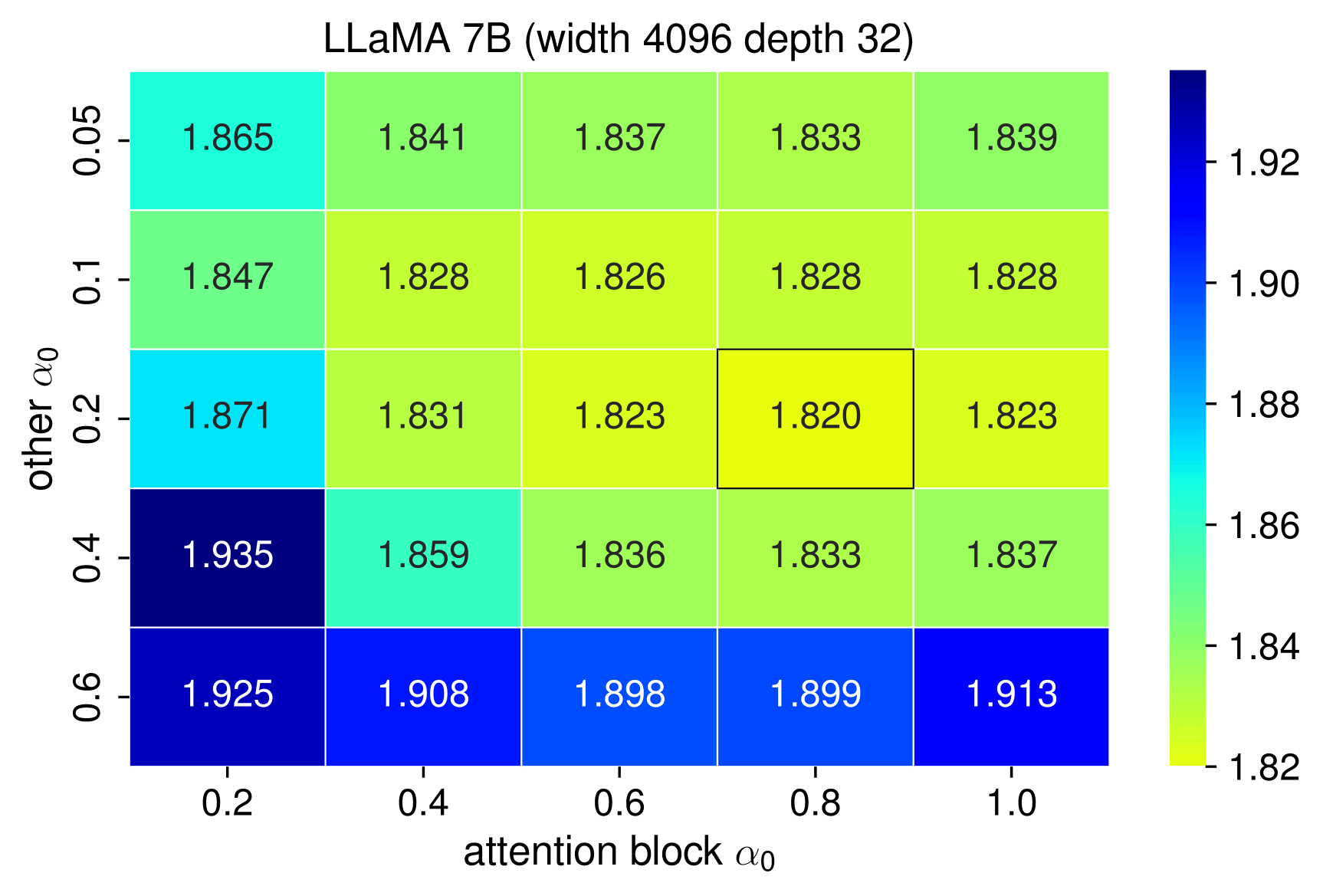\n*図6:注意ブロックとフィードフォワードブロックの異なるα初期化値におけるLLaMA 7Bの性能を示すヒートマップ。*\n\nLLaMA 7Bでは、注意ブロックで0.2、その他のブロックで0.2が最適なα初期化値であることが分かり、LLaMA 13Bでは、注意ブロックで0.6、その他のブロックで0.15が最適でした。これは、より大きなモデルではαパラメータのより慎重なチューニングが必要かもしれないことを示唆しています。\n\n研究者たちは、異なる深さと幅のモデルを訓練することで、彼らのアプローチのスケーラビリティもテストしました:\n\n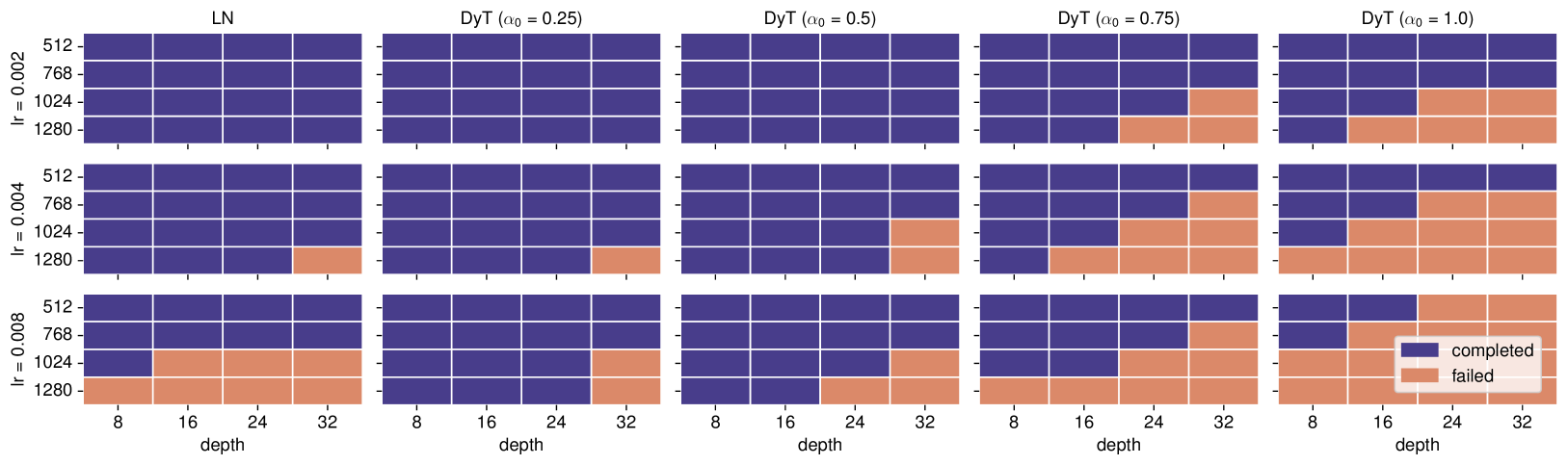\n*図7:異なるモデルの深さと幅におけるLNとDyTのトレーニング安定性の比較。青は成功したトレーニングを、オレンジは不安定性を示します。*\n\n結果は、DyTモデルがLNモデルと同等にスケールできることを示しましたが、より大きなスケールでは学習率に対してやや敏感になることも分かりました。\n\n## アルファパラメータの分析\n\n研究者たちは、DyTのαパラメータが活性化の統計的特性とどのように関連しているかを分析しました。興味深いことに、αは層の活性化の標準偏差の逆数を近似することを学習することが分かりました:\n\n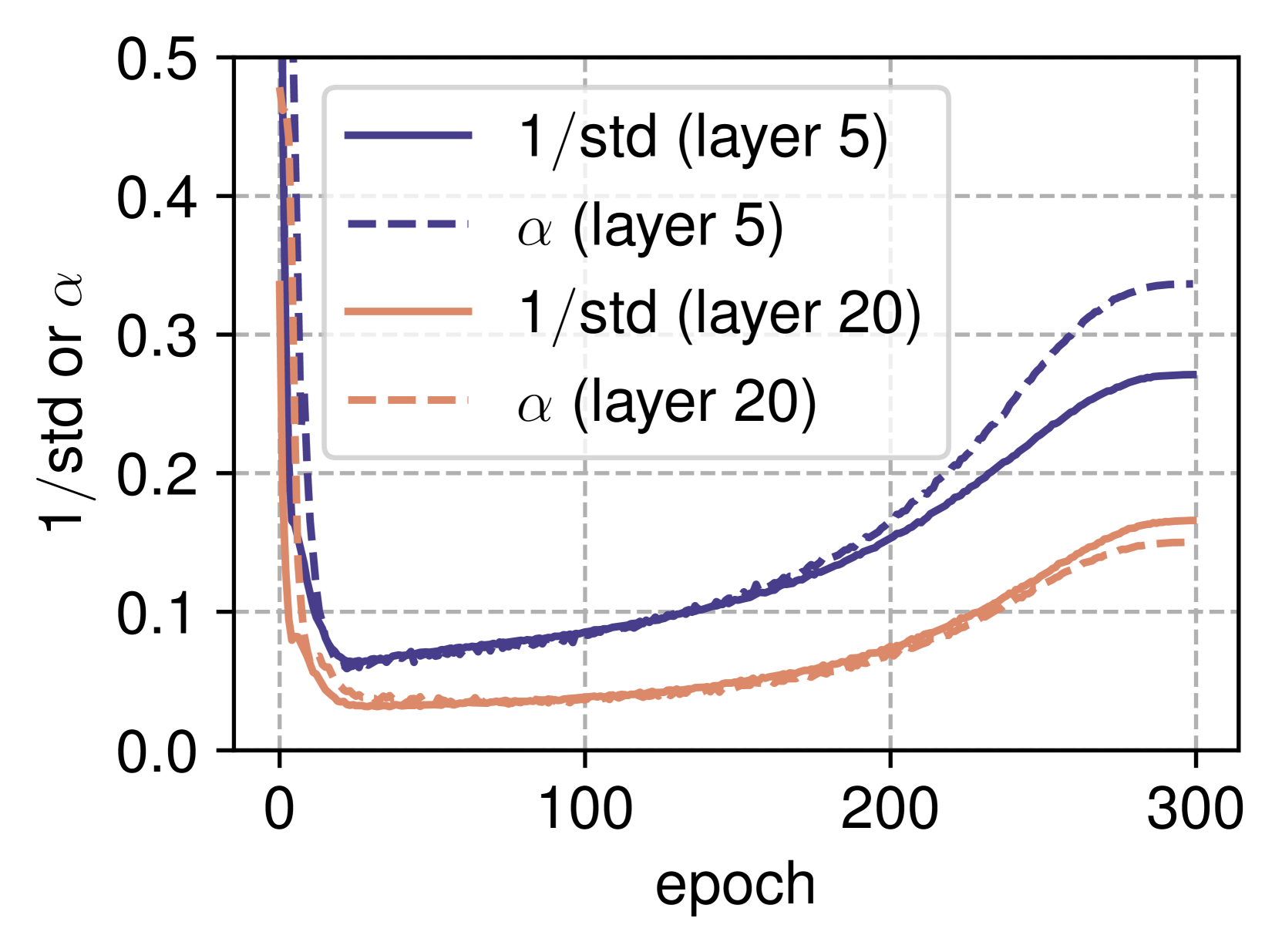\n*図8:学習されたα値と活性化の標準偏差の逆数(1/std)の訓練エポック全体での比較。αが部分的に正規化の挙動を模倣する様子を示している。*\n\nこの発見は、DyTが統計量を明示的に計算することなく、正規化層に似た適応的なスケーリングを暗黙的に学習していることを示唆しています。αパラメータは活性化の標準偏差に反比例する傾向があり、入力の大きさをtanh関数に適した値に効果的にスケーリングします。\n\nさらに、研究者たちは、異なる層やモデル間で、学習されたα値と活性化の標準偏差の逆数との間に一貫した相関関係があることを観察しました:\n\n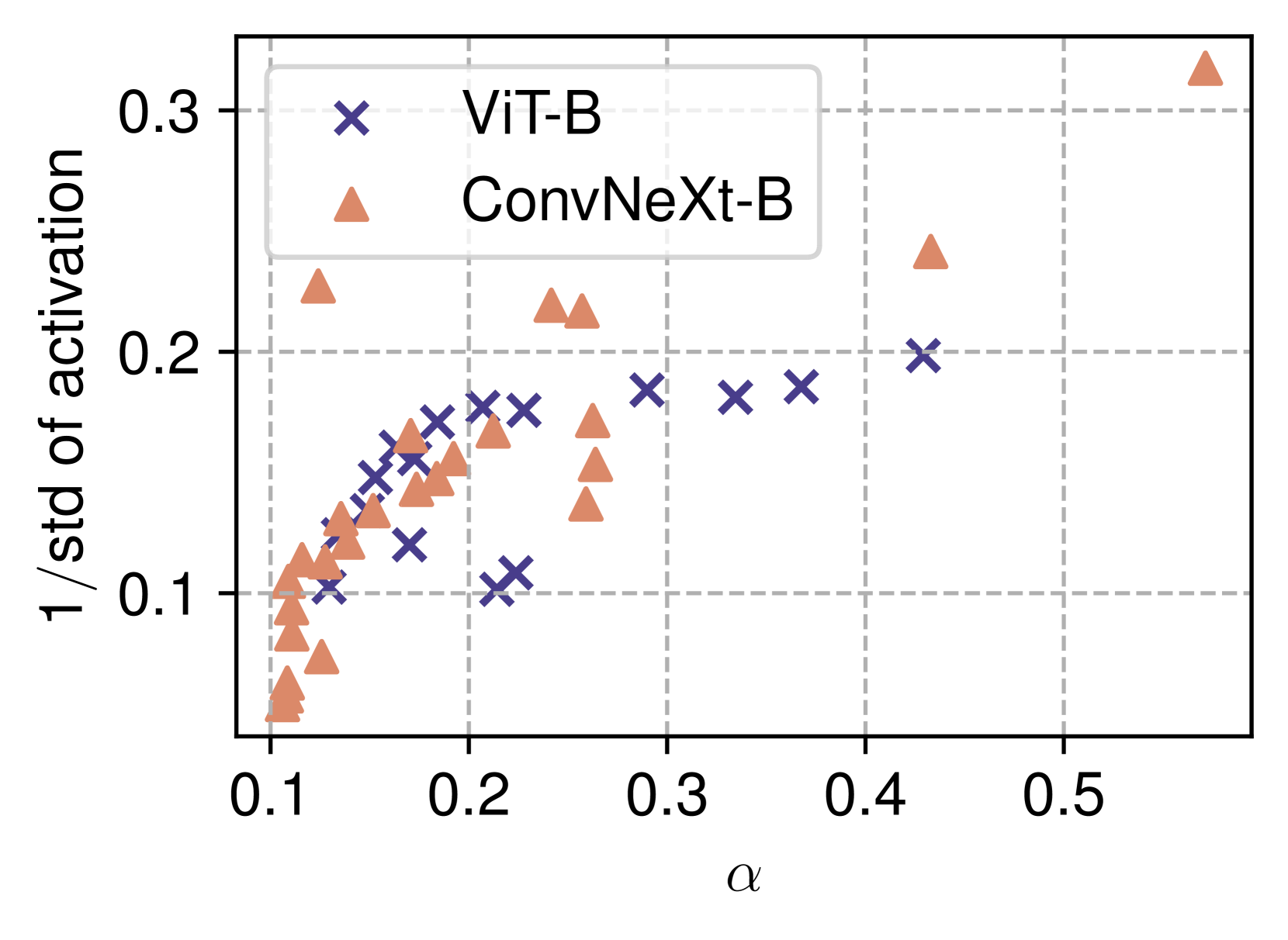\n*図9:ViT-BとConvNeXt-Bモデルの異なる層における、学習されたα値と活性化の標準偏差の逆数との関係を示す散布図。*\n\n## 他のアプローチとの比較\n\n研究者たちは、DyTを、Fixup、SkipInit、σReparamなど、正規化なしでディープネットワークを訓練するために提案された他の手法と比較しました。様々なタスクやモデルアーキテクチャにおいて、DyTは一貫してこれらの代替手法を上回る性能を示しました。\n\nまた、tanhの関数と学習可能なスケールパラメータαの両方の重要性を検証するためのアブレーション研究も実施しました。これらの研究により以下が示されました:\n\n1. tanhをsigmoidやhardtanhなどの他の関数に置き換えると性能が低下し、tanhの特性の重要性が浮き彫りになりました。\n\n2. 学習可能なαを固定αに変更すると性能が大幅に低下し、適応性の重要性が実証されました。\n\n3. 非線形性を完全に除去すると(学習可能なスケールのみを使用)、訓練が不安定になり、tanhの有界性が重要であることが示されました。\n\nまた、異なるタスクにおける初期α値のモデル性能への影響も研究されました:\n\n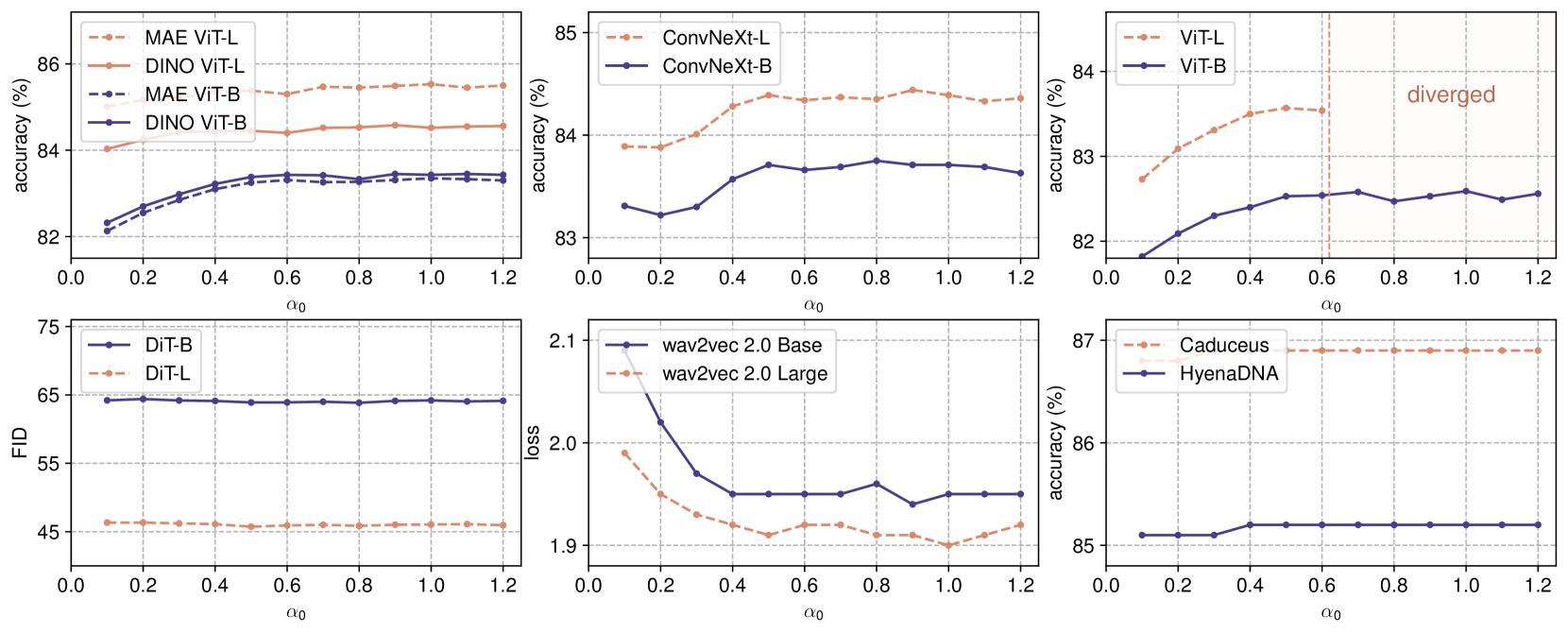\n*図10:異なるα初期化値(α₀)におけるさまざまなモデルの性能を示し、タスクに依存する感度を表している。*\n\n## 意義と応用\n\nこの研究の発見には、いくつかの重要な意義があります:\n\n1. **アーキテクチャの簡素化**:正規化層をDyTに置き換えることで、Transformerアーキテクチャを簡素化でき、より解釈しやすいモデルにつながる可能性があります。\n\n2. **計算効率**:予備的な測定によると、DyTは統計量の計算が不要になるため、正規化層と比較して訓練と推論の速度を改善できる可能性があります。\n\n3. **理論的理解**:DyTの成功は、ディープラーニングにおける正規化の基本的な役割について洞察を提供し、主要な利点は統計量の正規化ではなくS字型の変換にある可能性を示唆しています。\n\n4. **領域横断的な適用可能性**:DyTが多様な領域(視覚、言語、音声、生物学)で一貫して成功していることは、ディープラーニングの最適化における基本的な原理を捉えていることを示唆しています。\n\n著者らが指摘した制限の一つは、DyTがさらなる研究なしには、バッチ正規化を使用する古典的なCNNアーキテクチャに直接適用できない可能性があることです。彼らの研究は主にTransformerアーキテクチャに焦点を当てています。\n\n## 結論\n\n「Transformers without Normalization」論文は、Transformerの正規化層がシンプルなDynamic Tanh(DyT)操作で効果的に置き換えられることを示すことで、ディープラーニングのアーキテクチャ設計に重要な貢献をしています。これは、正規化層が高性能Transformerの訓練に不可欠であるという従来の常識に異議を唱えるものです。\n\n提案されたDyTアプローチは、実装が容易で、多くの場合最小限のチューニングしか必要とせず、幅広いタスクやドメインにおいて正規化されたモデルと同等もしくはそれ以上の性能を発揮できる魅力的な代替手法を提供します。DyTにおけるαが活性化の標準偏差の逆数を近似的に学習するという発見は、この単純なメカニズムがどのように正規化の特定の側面を効果的に模倣しているかについての洞察を提供しています。\n\nこの研究は、ニューラルネットワークアーキテクチャを単純化する新しい可能性を開き、従来の正規化技術に対する代替手法のさらなる探求を促すかもしれません。ディープラーニングが進化し続ける中で、このような単純化はより効率的で解釈可能なモデルの実現に貢献する可能性があります。\n\n## 関連文献\n\nJimmy Lei Ba、Jamie Ryan Kiros、Geoffrey E Hinton。[レイヤー正規化](https://alphaxiv.org/abs/1607.06450)。arXivプレプリントarXiv:1607.06450、2016年。\n\n * この論文は、特にTransformerにおいて訓練を安定化させる重要な要素であるレイヤー正規化(LN)を紹介しています。論文はLNの挙動を分析し、Dynamic Tanh(DyT)を代替として提案しており、この引用は非常に関連性が高いものです。\n\nAlexey Dosovitskiy、Lucas Beyer、Alexander Kolesnikov、Dirk Weissenborn、Xiaohua Zhai、Thomas Unterthiner、Mostafa Dehghani、Matthias Minderer、Georg Heigold、Sylvain Gelly他。画像は16x16の単語に値する:大規模な画像認識のためのTransformer。arXivプレプリントarXiv:2010.11929、2020年。\n\n * この論文は、画像分類タスクにおけるDyTの有効性のベンチマークに使用される主要なアーキテクチャであるVision Transformer(ViT)を紹介しています。論文ではDyTがレイヤー正規化の代替となることを実証するためのコアアーキテクチャとしてViTを使用しています。\n\nBiao Zhang、Rico Sennrich。[二乗平均平方根レイヤー正規化](https://alphaxiv.org/abs/1910.07467)。NeurIPS、2019年。\n\n * この研究は、レイヤー正規化の代替としてRMSNormを導入し、特に大規模言語モデル実験においてDyTのベースライン比較として使用されています。論文ではLNとRMSNormの両方の代替としてDyTを探求しています。\n\nHugo Touvron、Thibaut Lavril、Gautier Izacard、Xavier Martinet、Marie-Anne Lachaux、Timothée Lacroix、Baptiste Rozière、Naman Goyal、Eric Hambro、Faisal Azhar他。[Llama:オープンで効率的な基盤言語モデル](https://alphaxiv.org/abs/2302.13971)。arXivプレプリントarXiv:2302.13971、2023年a。\n\n * この引用は、大規模言語モデルの文脈でDyTのテストと評価のための重要なアーキテクチャとして機能するLLaMA言語モデルを紹介しています。論文ではDyTの一般化可能性を検証するための重要なアーキテクチャとしてLLaMAを使用しています。\n\nAshish Vaswani、Noam Shazeer、Niki Parmar、Jakob Uszkoreit、Llion Jones、Aidan N Gomez、Łukasz Kaiser、Illia Polosukhin。[注意機構がすべて](https://alphaxiv.org/abs/1706.03762)。NeurIPS、2017年。\n\n * この基礎的な論文は、DyT研究の主な焦点であるTransformerアーキテクチャを紹介しています。論文ではDyTがTransformerをどのように改善できるかを示すことに焦点を当てています。"])</script><script>self.__next_f.push([1,"90:T4056,"])</script><script>self.__next_f.push([1,"# Transformer ohne Normalisierung: Eine einfache Alternative mit dynamischem Tanh\n\n## Inhaltsverzeichnis\n- [Einführung](#einführung)\n- [Verständnis von Normalisierungsschichten](#verständnis-von-normalisierungsschichten)\n- [Die dynamische Tanh-Lösung](#die-dynamische-tanh-lösung)\n- [Wie DyT funktioniert](#wie-dyt-funktioniert)\n- [Experimentelle Beweise](#experimentelle-beweise)\n- [Abstimmung und Skalierbarkeit](#abstimmung-und-skalierbarkeit)\n- [Analyse des Alpha-Parameters](#analyse-des-alpha-parameters)\n- [Vergleich mit anderen Ansätzen](#vergleich-mit-anderen-ansätzen)\n- [Implikationen und Anwendungen](#implikationen-und-anwendungen)\n- [Fazit](#fazit)\n\n## Einführung\n\nNormalisierungsschichten gelten als wesentliche Komponenten in modernen neuronalen Netzen, insbesondere in Transformer-Architekturen, die die natürliche Sprachverarbeitung, Computer Vision und andere Bereiche dominieren. Layer Normalization (LN) und ihre Varianten sind in Transformern allgegenwärtig und werden als entscheidend für die Stabilisierung des Trainings und die Verbesserung der Leistung angesehen. Eine neue Arbeit von Forschern von Meta AI, NYU, MIT und der Princeton University stellt diese grundlegende Annahme jedoch in Frage, indem sie zeigt, dass Transformer ohne traditionelle Normalisierungsschichten gleichwertige oder bessere Leistungen erzielen können.\n\n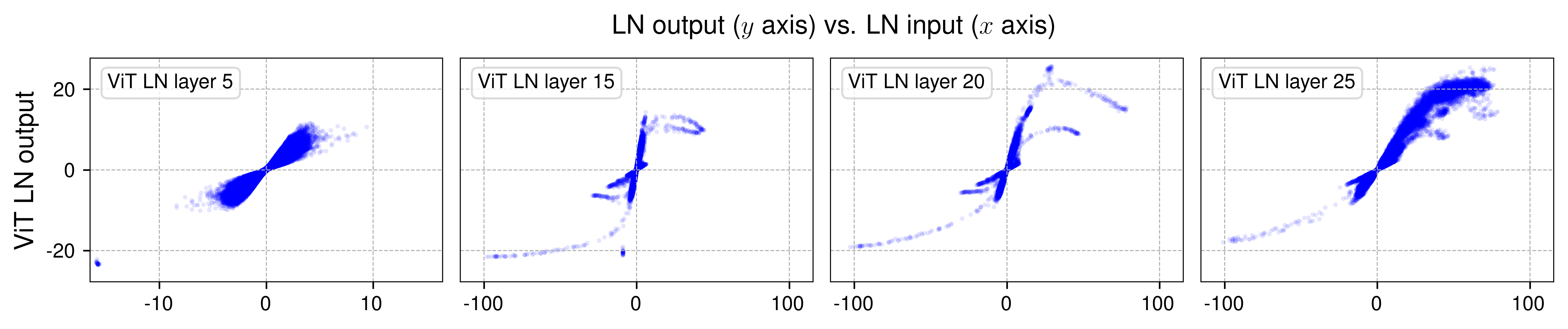\n*Abbildung 1: Visualisierung des Eingabe-Ausgabe-Verhaltens der Layer-Normalisierung in verschiedenen ViT-Schichten, die S-förmige, tanh-ähnliche Beziehungen zeigen.*\n\n## Verständnis von Normalisierungsschichten\n\nNormalisierungstechniken wie Batch Normalization, Layer Normalization und RMSNorm sind in der Deep Learning-Praxis zum Standard geworden. Diese Methoden normalisieren typischerweise Aktivierungen durch Berechnung von Statistiken (Mittelwert und/oder Standardabweichung) über bestimmte Dimensionen und helfen dabei, das Training zu stabilisieren, indem sie die Verteilung der Netzwerkaktivierungen kontrollieren.\n\nSpeziell bei Transformern arbeitet die Layer Normalization, indem sie den Mittelwert und die Standardabweichung über die Feature-Dimension für jeden Token oder jede Position berechnet. Dieser Normalisierungsprozess ist rechenintensiv, da diese Statistiken während des Trainings und der Inferenz in jeder Schicht berechnet werden müssen.\n\nDie Autoren beobachteten, dass Layer Normalization oft tanh-ähnliche, S-förmige Eingabe-Ausgabe-Abbildungen erzeugt, wie in Abbildung 1 gezeigt. Diese Beobachtung führte zu ihrer wichtigen Erkenntnis: Vielleicht könnte der positive Effekt der Normalisierung durch einen einfacheren Mechanismus erreicht werden, der dieses S-förmige Verhalten nachahmt, ohne Aktivierungsstatistiken zu berechnen.\n\n## Die dynamische Tanh-Lösung\n\nDie Forscher schlagen Dynamic Tanh (DyT) als unkomplizierten Ersatz für Normalisierungsschichten vor. DyT ist definiert als:\n\n```\nDyT(x) = tanh(αx)\n```\n\nWobei α ein lernbarer Parameter ist, der die Steilheit der Tanh-Funktion steuert. Diese einfache Formulierung eliminiert die Notwendigkeit, Aktivierungsstatistiken zu berechnen, während die S-förmige Transformation erhalten bleibt, die für die Transformer-Leistung wichtig zu sein scheint.\n\n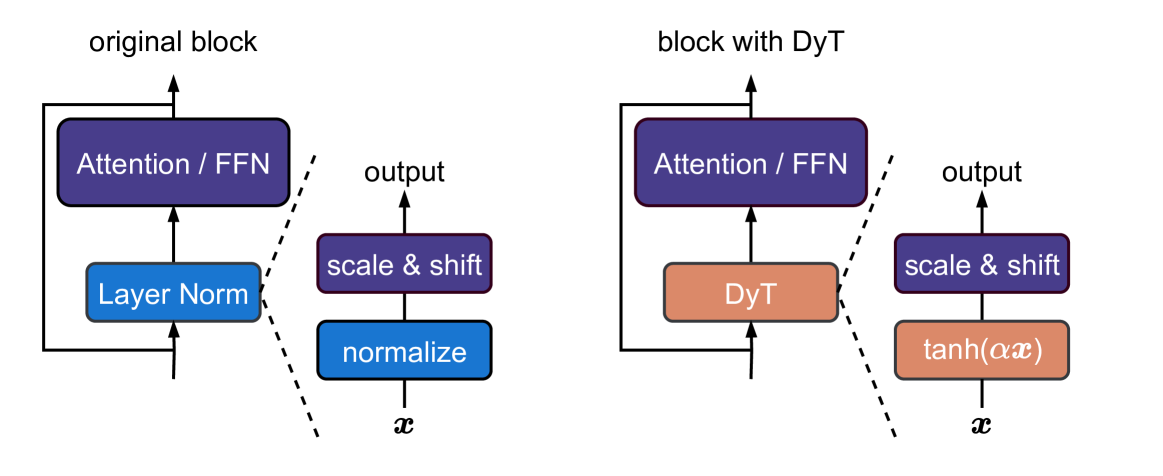\n*Abbildung 2: Links: Ursprünglicher Transformer-Block mit Layer Normalization. Rechts: Vorgeschlagener Block mit Dynamic Tanh (DyT) Ersatz.*\n\nDie Schönheit dieses Ansatzes liegt in seiner Einfachheit - komplexe Normalisierungsoperationen werden durch eine einzige elementweise Operation mit einem lernbaren Parameter ersetzt. Abbildung 2 zeigt, wie sich der traditionelle Transformer-Block mit Layer Normalization im Vergleich zum vorgeschlagenen Block mit DyT verhält.\n\n## Wie DyT funktioniert\n\nDynamic Tanh arbeitet durch zwei Schlüsselmechanismen:\n\n1. **Wertestauchung**: Die Tanh-Funktion staucht extreme Werte und bietet eine Form der impliziten Regularisierung ähnlich wie Normalisierungsschichten. Dies verhindert, dass Aktivierungen während der Vorwärts- und Rückwärtsdurchläufe zu groß werden.\n\n2. **Adaptive Skalierung**: Der lernbare Parameter α passt die Steilheit der tanh-Funktion an und ermöglicht dem Netzwerk zu kontrollieren, wie aggressiv Werte gestaucht werden. Diese Anpassungsfähigkeit ist entscheidend für die Leistung.\n\nDie hyperbolische Tangensfunktion (tanh) ist zwischen -1 und 1 begrenzt und staucht jeden Eingabewert in diesen Bereich. Die Steilheit dieser Stauchung wird durch α gesteuert:\n\n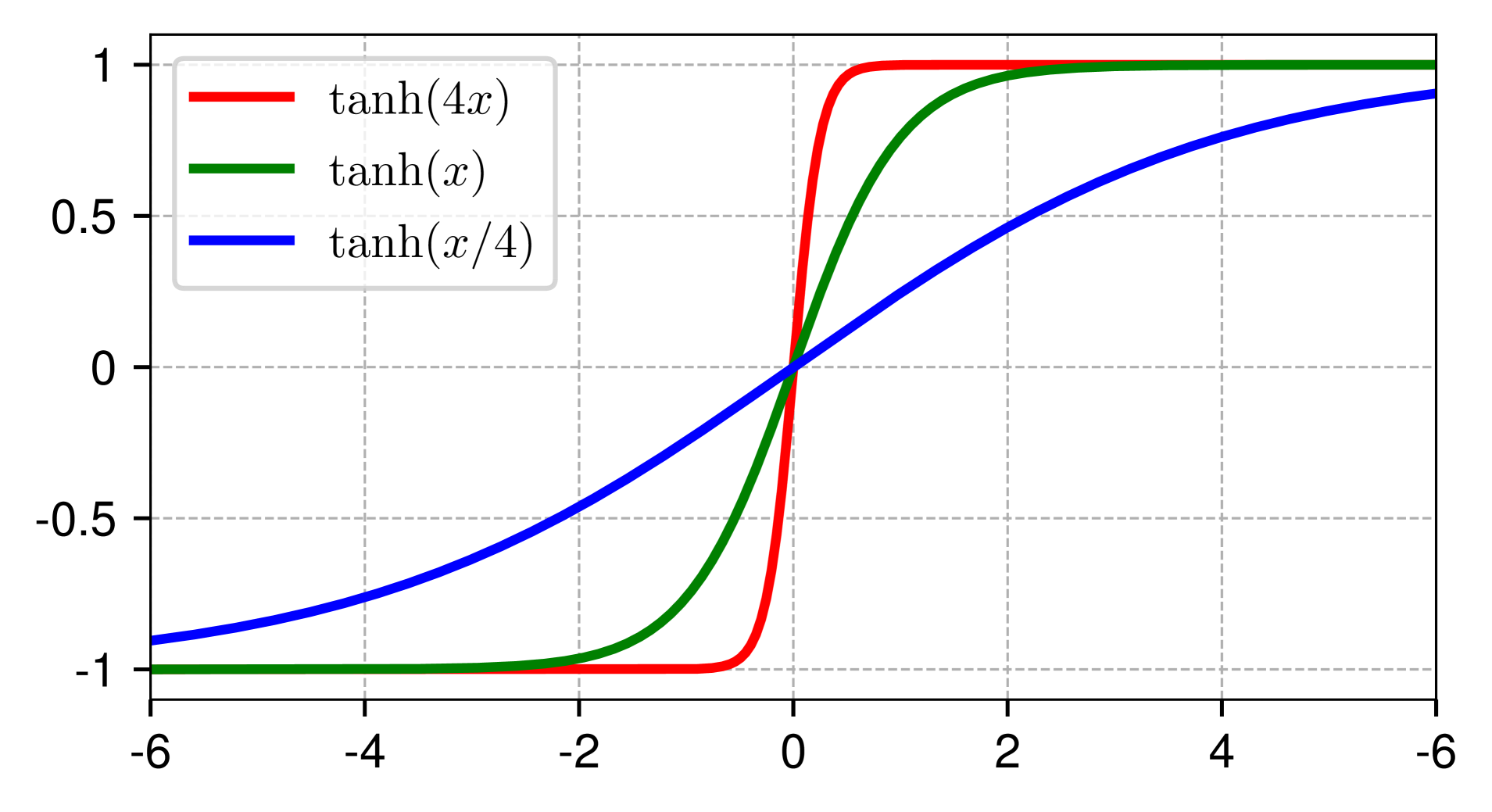\n*Abbildung 3: Die tanh-Funktion mit verschiedenen α-Werten zeigt, wie größere α-Werte schärfere Übergänge erzeugen.*\n\nWie in Abbildung 3 gezeigt, macht ein größerer α-Wert den Übergang von -1 zu 1 schärfer, während ein kleinerer α-Wert ihn allmählicher gestaltet. Diese Flexibilität ermöglicht es dem Netzwerk, den Grad der Wertestauchung basierend auf der Aufgabe und Schichttiefe anzupassen.\n\n## Experimentelle Beweise\n\nDie Forscher führten umfangreiche Experimente in verschiedenen Aufgaben und Bereichen durch, um die Wirksamkeit von DyT als Ersatz für Normalisierungsschichten zu validieren. Diese Experimente umfassten:\n\n1. **Visuelle Aufgaben**:\n - ImageNet-Klassifizierung mit Vision Transformers (ViT) und ConvNeXt\n - Selbstüberwachtes Lernen mit MAE und DINO\n\n2. **Generative Modelle**:\n - Diffusionsmodelle für Bilderzeugung (DiT)\n\n3. **Große Sprachmodelle**:\n - LLaMA-Vortraining in Größenordnungen von 7B bis 70B Parametern\n\n4. **Andere Bereiche**:\n - Sprachverarbeitung mit wav2vec 2.0\n - DNA-Sequenzmodellierung mit HyenaDNA und Caduceus\n\nDie Ergebnisse zeigten durchgängig, dass Transformer mit DyT die Leistung ihrer normalisierten Gegenstücke erreichen oder übertreffen konnten. Bei Vision Transformern zur ImageNet-Klassifizierung erreichte die DyT-Variante beispielsweise eine vergleichbare Genauigkeit zur LN-Version:\n\n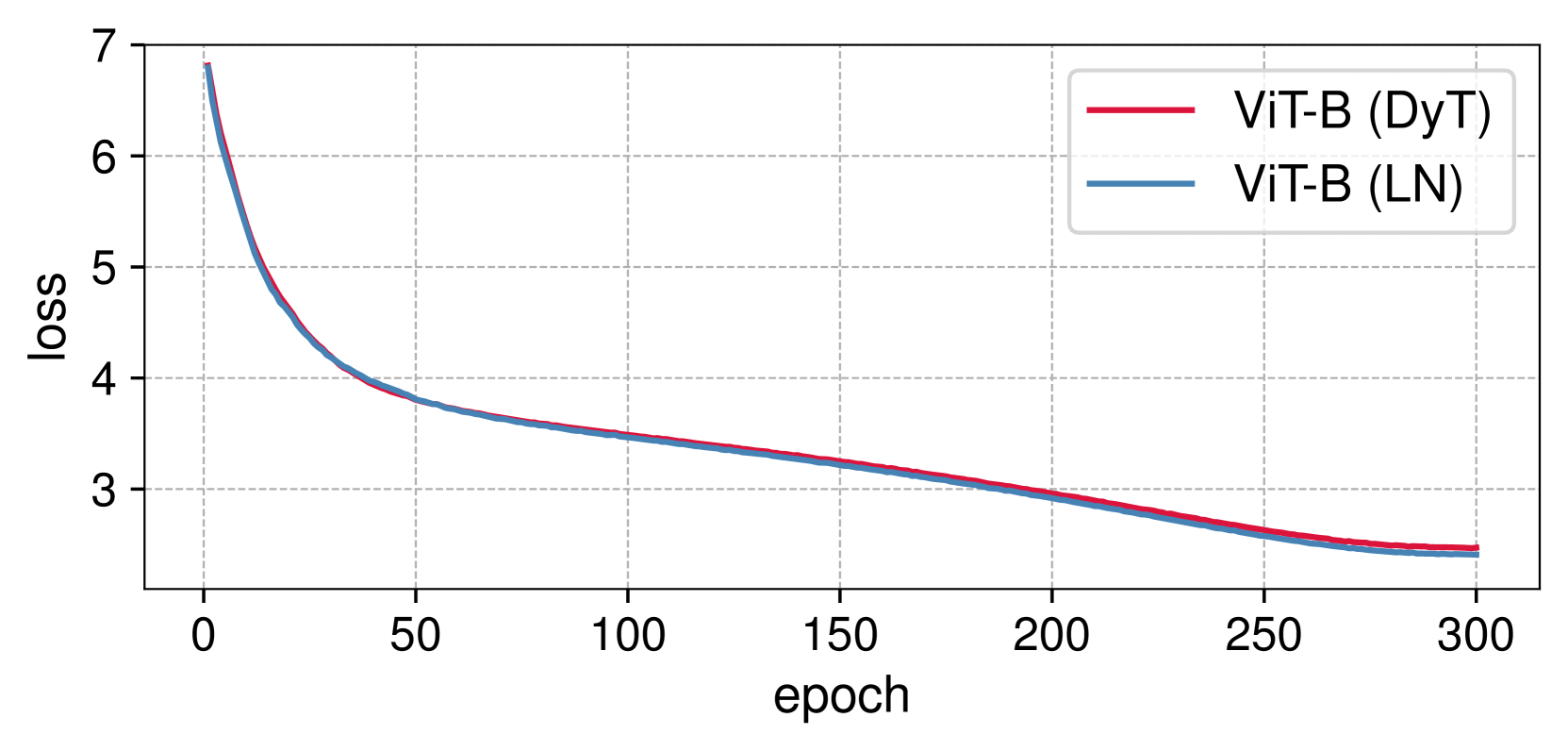\n*Abbildung 4: Trainingsverlust-Kurven für ViT-B mit Layer Normalization (LN) und Dynamic Tanh (DyT) zeigen nahezu identische Konvergenz.*\n\nÄhnlich erreichten DyT-Varianten bei LLaMA-Modellen verschiedener Größen (7B bis 70B Parameter) vergleichbare oder leicht bessere Verlustwerte im Vergleich zu RMSNorm-Modellen:\n\n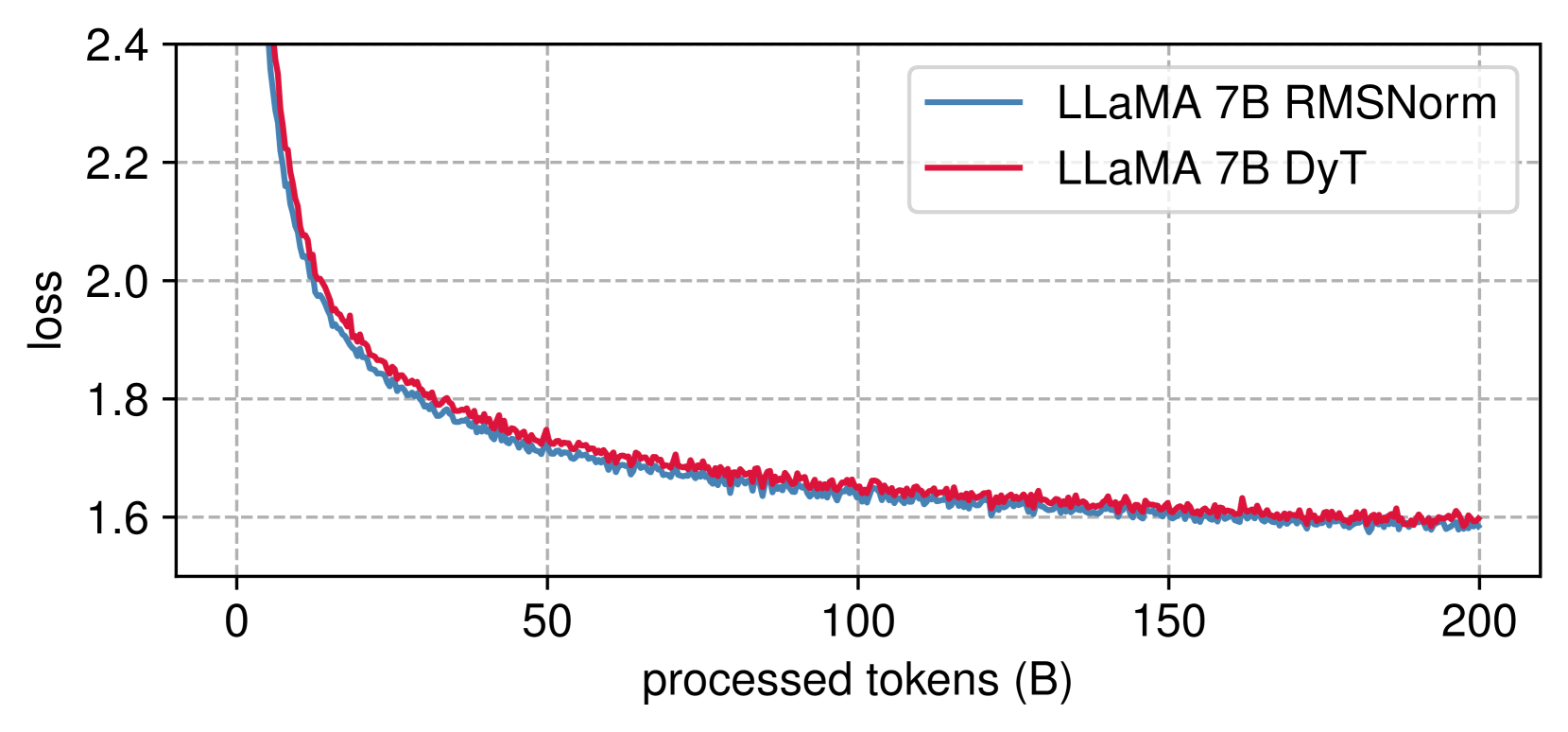\n*Abbildung 5: Trainingsverlust-Kurven für LLaMA 7B mit RMSNorm und DyT zeigen vergleichbare Leistung.*\n\n## Abstimmung und Skalierbarkeit\n\nWährend DyT im Allgemeinen robust ist und mit minimaler Abstimmung gut funktioniert, stellten die Forscher fest, dass bei größeren Modellen, insbesondere bei Large Language Models (LLMs), eine sorgfältige Initialisierung von α wichtig ist. Sie führten eine gründliche Untersuchung der Initialisierungswerte für die LLaMA-Architektur durch:\n\n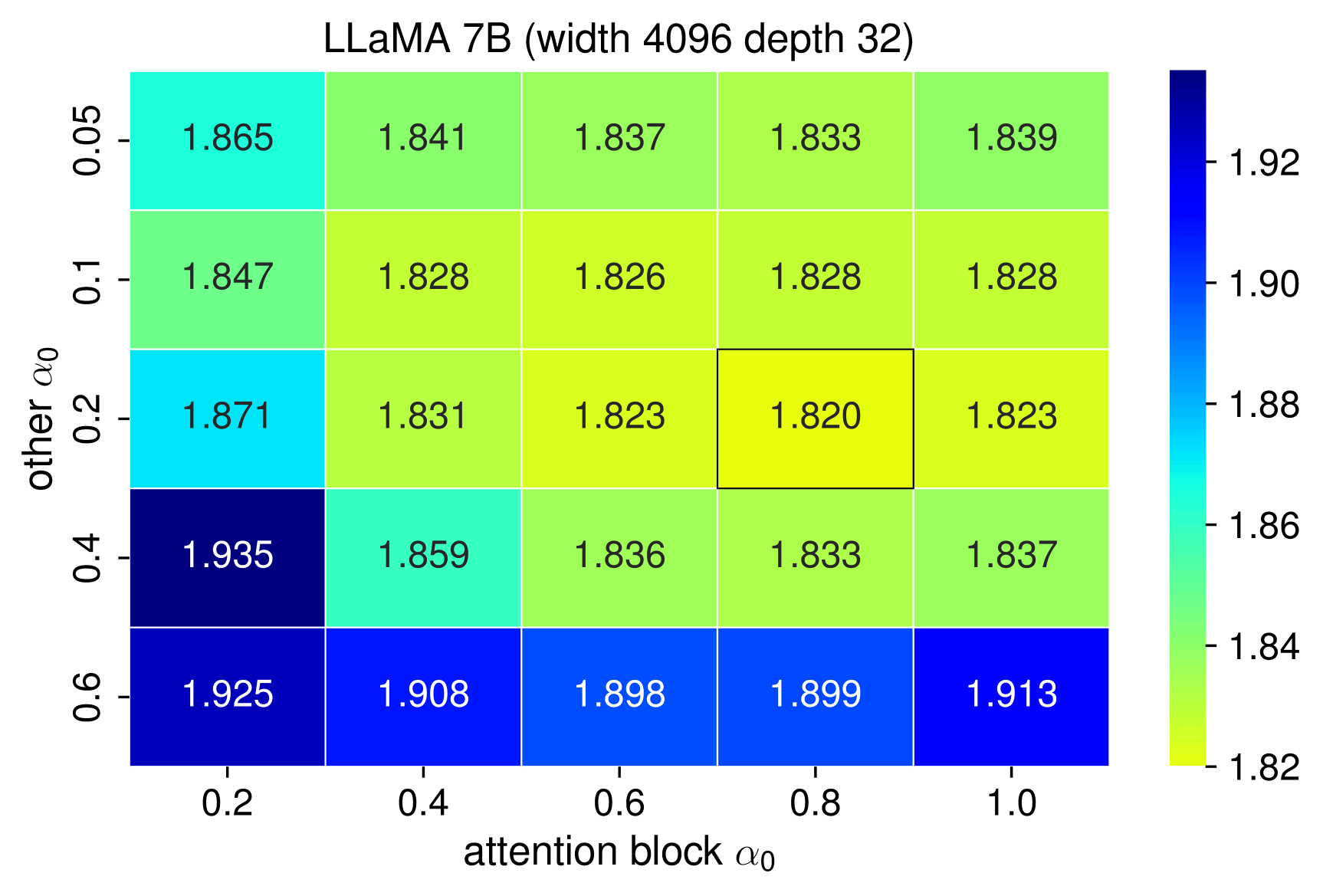\n*Abbildung 6: Heatmap zeigt LLaMA 7B-Leistung mit verschiedenen α-Initialisierungswerten für Attention- und Feedforward-Blöcke.*\n\nFür LLaMA 7B wurde die optimale α-Initialisierung mit 0,2 für Attention-Blöcke und 0,2 für andere Blöcke gefunden, während sie für LLaMA 13B bei 0,6 für Attention-Blöcke und 0,15 für andere Blöcke lag. Dies deutet darauf hin, dass größere Modelle eine sorgfältigere Abstimmung des α-Parameters erfordern könnten.\n\nDie Forscher testeten auch die Skalierbarkeit ihres Ansatzes durch das Training von Modellen unterschiedlicher Tiefen und Breiten:\n\n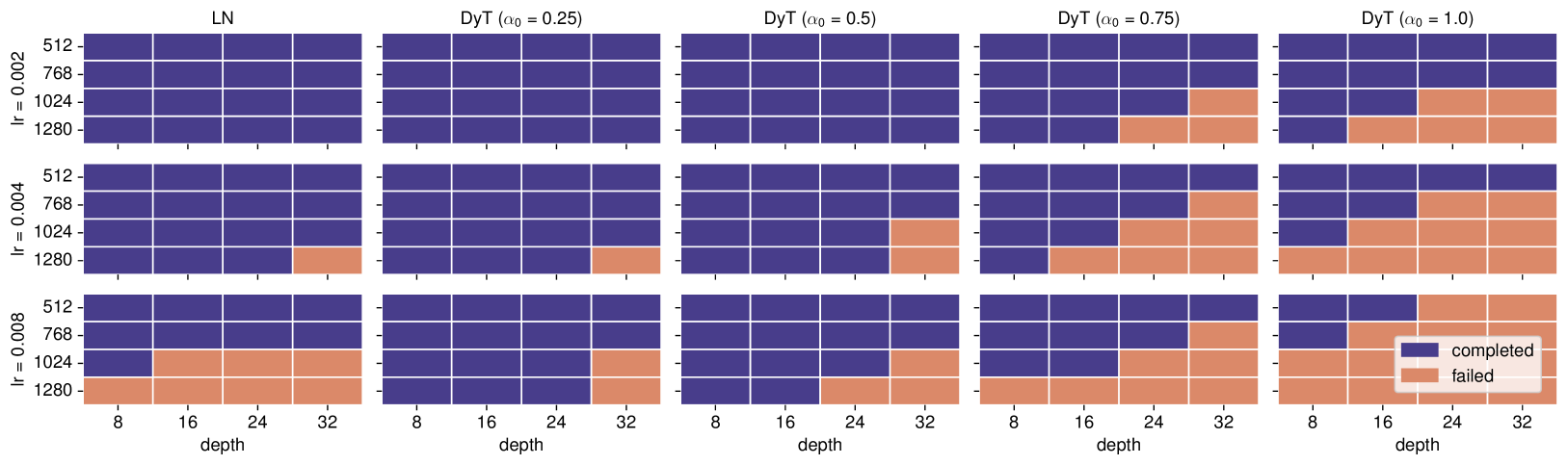\n*Abbildung 7: Vergleich der Trainingsstabilität zwischen LN und DyT über verschiedene Modelltiefen und -breiten, wobei Blau erfolgreiches Training und Orange Instabilität anzeigt.*\n\nDie Ergebnisse zeigten, dass DyT-Modelle vergleichbar zu LN-Modellen skalieren können, allerdings mit einer zusätzlichen Empfindlichkeit gegenüber der Lernrate bei größeren Skalen.\n\n## Analyse des Alpha-Parameters\n\nDie Forscher analysierten, wie der α-Parameter in DyT mit den statistischen Eigenschaften der Aktivierungen zusammenhängt. Interessanterweise fanden sie heraus, dass α lernt, den Kehrwert der Standardabweichung der Schichtaktivierungen anzunähern:\n\n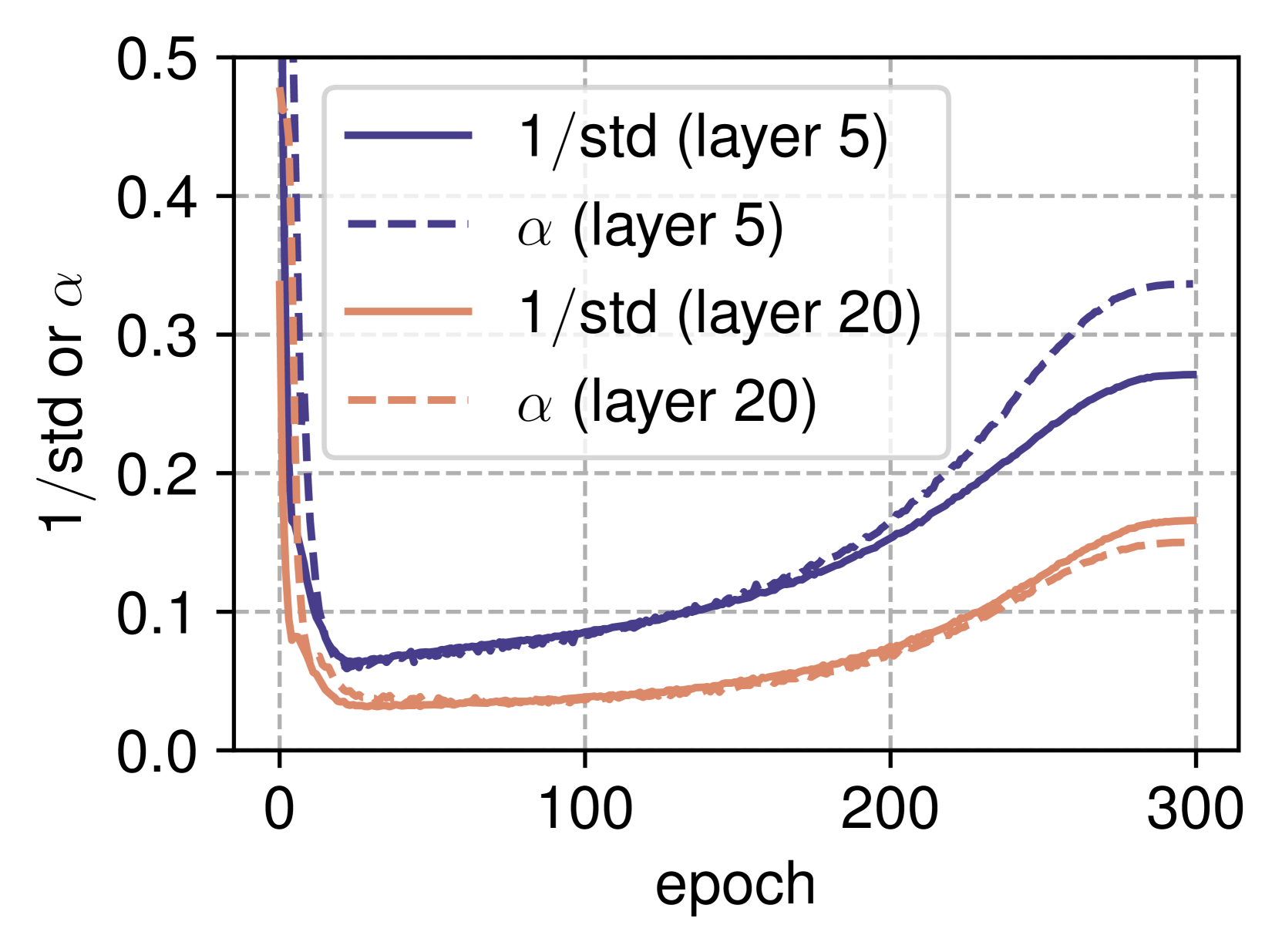\n*Abbildung 8: Vergleich zwischen den gelernten α-Werten und der inversen Aktivierungsstandardabweichung (1/std) über die Trainingsepochen hinweg, der zeigt, wie α teilweise das Normalisierungsverhalten nachahmt.*\n\nDiese Erkenntnis deutet darauf hin, dass DyT implizit lernt, eine Form der adaptiven Skalierung ähnlich wie Normalisierungsschichten durchzuführen, jedoch ohne explizite Berechnung von Statistiken. Der α-Parameter tendiert dazu, umgekehrt proportional zur Standardabweichung der Aktivierungen zu sein und skaliert die Eingaben effektiv so, dass ihre Größenordnung für die tanh-Funktion angemessen ist.\n\nDarüber hinaus beobachteten sie eine konsistente Korrelation zwischen den gelernten α-Werten und der inversen Standardabweichung der Aktivierungen über verschiedene Schichten und Modelle hinweg:\n\n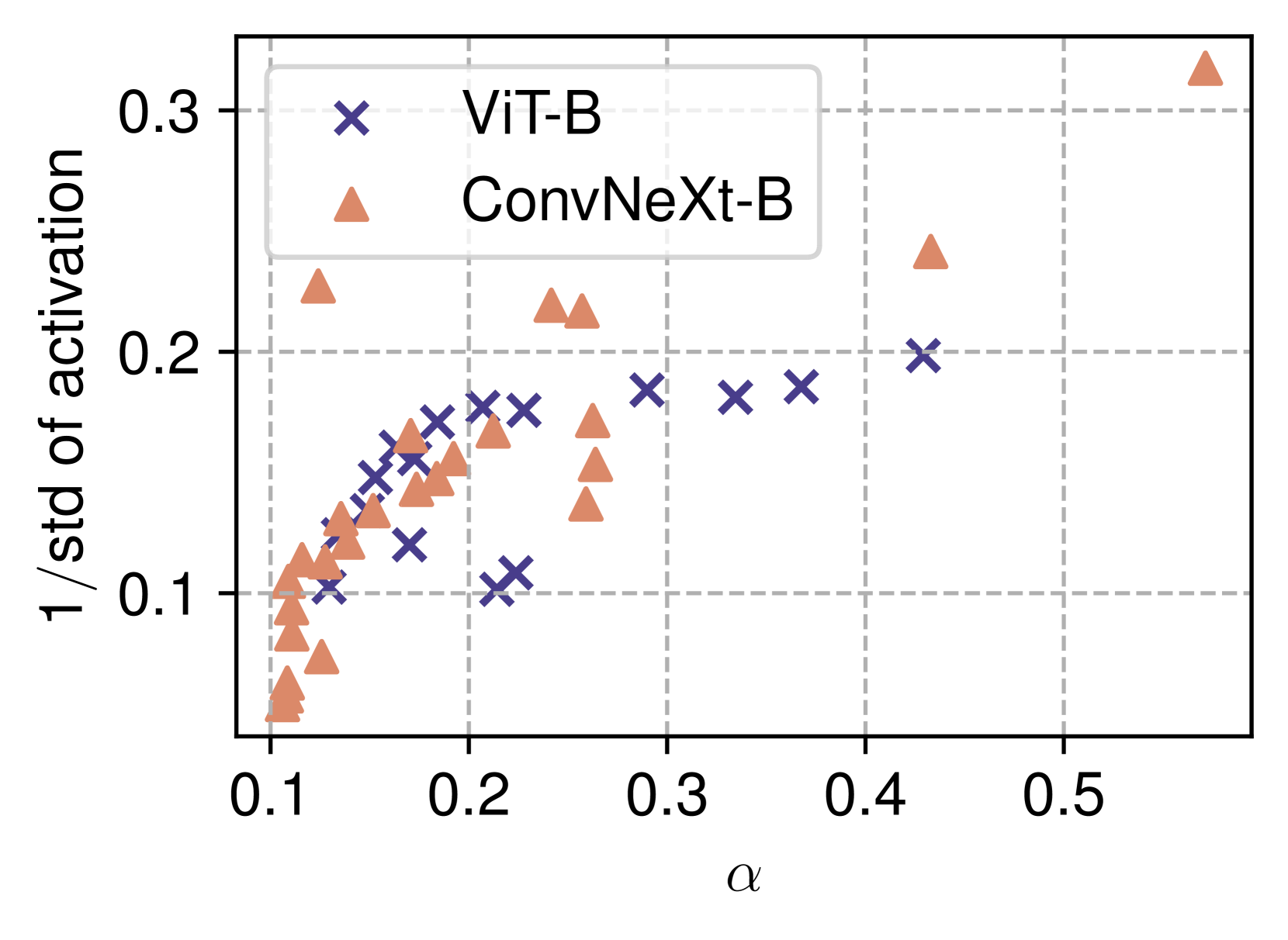\n*Abbildung 9: Streudiagramm, das die Beziehung zwischen gelernten α-Werten und der inversen Standardabweichung der Aktivierungen über verschiedene Schichten in ViT-B und ConvNeXt-B Modellen zeigt.*\n\n## Vergleich mit anderen Ansätzen\n\nDie Forscher verglichen DyT mit anderen Methoden, die für das Training tiefer Netzwerke ohne Normalisierung vorgeschlagen wurden, einschließlich Fixup, SkipInit und σReparam. Bei verschiedenen Aufgaben und Modellarchitekturen übertraf DyT diese Alternativen durchweg.\n\nSie führten auch Ablationsstudien durch, um die Bedeutung sowohl der tanh-Funktion als auch des lernbaren Skalenparameters α zu validieren. Diese Studien zeigten:\n\n1. Das Ersetzen von tanh durch andere Funktionen wie sigmoid oder hardtanh führte zu reduzierter Leistung, was die Bedeutung der spezifischen Eigenschaften von tanh hervorhebt.\n\n2. Die Verwendung eines festen α anstelle eines lernbaren führte zu deutlich schlechterer Leistung, was die Bedeutung der Adaptivität demonstriert.\n\n3. Das vollständige Entfernen der Nichtlinearität (nur mit lernbarer Skalierung) führte zu Trainingsinstabilität, was zeigt, dass die Beschränktheit von tanh entscheidend ist.\n\nDer Einfluss der initialen α-Werte auf die Modellleistung wurde auch über verschiedene Aufgaben hinweg untersucht:\n\n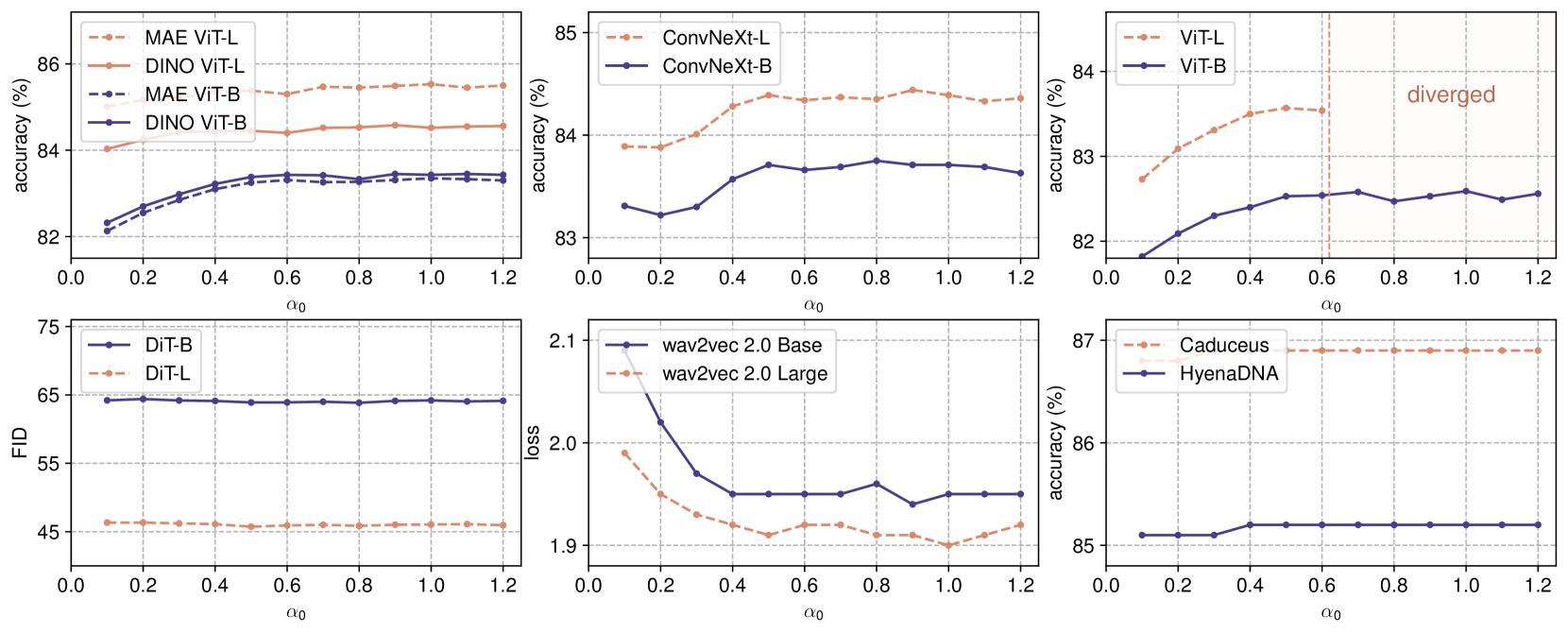\n*Abbildung 10: Leistung verschiedener Modelle mit unterschiedlichen α-Initialisierungswerten (α₀), die aufgabenabhängige Sensitivität zeigt.*\n\n## Implikationen und Anwendungen\n\nDie Ergebnisse dieser Forschung haben mehrere wichtige Implikationen:\n\n1. **Architektonische Vereinfachung**: Durch das Ersetzen von Normalisierungsschichten durch DyT können Transformer-Architekturen vereinfacht werden, was potenziell zu besser interpretierbaren Modellen führt.\n\n2. **Rechnerische Effizienz**: Vorläufige Messungen deuten darauf hin, dass DyT die Trainings- und Inferenzgeschwindigkeit im Vergleich zu Normalisierungsschichten verbessern kann, da die Berechnung von Statistiken entfällt.\n\n3. **Theoretisches Verständnis**: Der Erfolg von DyT liefert Einblicke in die fundamentale Rolle der Normalisierung im Deep Learning und deutet darauf hin, dass der Hauptvorteil möglicherweise die S-förmige Transformation und nicht die Normalisierung der Statistiken an sich ist.\n\n4. **Domänenübergreifende Anwendbarkeit**: Der konsistente Erfolg von DyT über verschiedene Domänen hinweg (Vision, Sprache, Sprache, Biologie) deutet darauf hin, dass es ein fundamentales Prinzip der Deep-Learning-Optimierung erfasst.\n\nEine von den Autoren festgestellte Einschränkung ist, dass DyT möglicherweise nicht direkt auf klassische CNN-Architekturen anwendbar ist, die Batch-Normalisierung verwenden, ohne weitere Forschung zu betreiben. Der Fokus ihrer Arbeit lag hauptsächlich auf Transformer-Architekturen.\n\n## Fazit\n\nDie Arbeit \"Transformers without Normalization\" leistet einen bedeutenden Beitrag zum Design von Deep-Learning-Architekturen, indem sie zeigt, dass Normalisierungsschichten in Transformern effektiv durch eine einfache Dynamic Tanh (DyT)-Operation ersetzt werden können. Dies stellt die konventionelle Weisheit in Frage, dass Normalisierungsschichten für das Training leistungsfähiger Transformer unerlässlich sind.\n\nDer vorgeschlagene DyT-Ansatz bietet eine überzeugende Alternative, die einfach zu implementieren ist, oft nur minimale Anpassungen erfordert und die Leistung normalisierter Modelle über ein breites Spektrum von Aufgaben und Domänen hinweg erreichen oder übertreffen kann. Die Erkenntnis, dass α in DyT lernt, den Kehrwert der Aktivierungsstandardabweichung anzunähern, gibt Einblick darin, wie dieser einfache Mechanismus bestimmte Aspekte der Normalisierung effektiv nachahmt.\n\nDiese Forschung eröffnet neue Wege zur Vereinfachung von neuronalen Netzwerkarchitekturen und könnte zu weiterer Erforschung von Alternativen zu traditionellen Normalisierungstechniken anregen. Mit der kontinuierlichen Weiterentwicklung des Deep Learning könnten solche Vereinfachungen zu effizienteren und interpretierbaren Modellen beitragen.\n\n## Relevante Zitierungen\n\nJimmy Lei Ba, Jamie Ryan Kiros, und Geoffrey E Hinton. [Layer normalization](https://alphaxiv.org/abs/1607.06450). arXiv preprint arXiv:1607.06450, 2016.\n\n * Diese Arbeit führt Layer Normalization (LN) ein, eine entscheidende Komponente zur Stabilisierung des Trainings in tiefen Netzwerken, insbesondere Transformern. Die Arbeit analysiert das Verhalten von LN und schlägt Dynamic Tanh (DyT) als Ersatz vor, was diese Zitierung hochrelevant macht.\n\nAlexey Dosovitskiy, Lucas Beyer, Alexander Kolesnikov, Dirk Weissenborn, Xiaohua Zhai, Thomas Unterthiner, Mostafa Dehghani, Matthias Minderer, Georg Heigold, Sylvain Gelly, et al. An image is worth 16x16 words: Transformers for image recognition at scale. arXiv preprint arXiv:2010.11929, 2020.\n\n * Diese Arbeit führt den Vision Transformer (ViT) ein, eine bedeutende Architektur, die für Benchmarking der Effektivität von DyT in Bildklassifizierungsaufgaben verwendet wird. Die Arbeit nutzt ViT als Kernarchitektur, um zu demonstrieren, dass DyT die Layer-Normalisierung ersetzen kann.\n\nBiao Zhang und Rico Sennrich. [Root mean square layer normalization](https://alphaxiv.org/abs/1910.07467). NeurIPS, 2019.\n\n * Diese Arbeit führt RMSNorm ein, eine Alternative zur Layer-Normalisierung, und wird als Baseline-Vergleich für DyT verwendet, insbesondere in Experimenten mit großen Sprachmodellen. Die Arbeit untersucht DyT als Ersatz sowohl für LN als auch für RMSNorm.\n\nHugo Touvron, Thibaut Lavril, Gautier Izacard, Xavier Martinet, Marie-Anne Lachaux, Timothée Lacroix, Baptiste Rozière, Naman Goyal, Eric Hambro, Faisal Azhar, et al. [Llama: Open and efficient foundation language models](https://alphaxiv.org/abs/2302.13971). arXiv preprint arXiv:2302.13971, 2023a.\n\n * Diese Zitierung stellt das LLaMA-Sprachmodell vor, das als Schlüsselarchitektur für das Testen und Evaluieren von DyT im Kontext großer Sprachmodelle dient. Die Arbeit verwendet LLaMA als wichtige Architektur zur Überprüfung der Generalisierbarkeit von DyT.\n\nAshish Vaswani, Noam Shazeer, Niki Parmar, Jakob Uszkoreit, Llion Jones, Aidan N Gomez, Łukasz Kaiser, und Illia Polosukhin. [Attention is all you need](https://alphaxiv.org/abs/1706.03762). NeurIPS, 2017.\n\n * Diese grundlegende Arbeit führt die Transformer-Architektur ein, die den Hauptfokus der DyT-Studie bildet. Die Arbeit konzentriert sich darauf zu zeigen, wie DyT Transformer verbessern kann."])</script><script>self.__next_f.push([1,"91:T662d,"])</script><script>self.__next_f.push([1,"# Трансформеры без нормализации: простая альтернатива с динамическим тангенсом\n\n## Содержание\n- [Введение](#введение)\n- [Понимание слоев нормализации](#понимание-слоев-нормализации)\n- [Решение с динамическим тангенсом](#решение-с-динамическим-тангенсом)\n- [Как работает DyT](#как-работает-dyt)\n- [Экспериментальные доказательства](#экспериментальные-доказательства)\n- [Настройка и масштабируемость](#настройка-и-масштабируемость)\n- [Анализ параметра альфа](#анализ-параметра-альфа)\n- [Сравнение с другими подходами](#сравнение-с-другими-подходами)\n- [Последствия и применения](#последствия-и-применения)\n- [Заключение](#заключение)\n\n## Введение\n\nСлои нормализации считались важнейшими компонентами в современных нейронных сетях, особенно в архитектурах Трансформеров, которые доминируют в обработке естественного языка, компьютерном зрении и других областях. Нормализация слоев (Layer Normalization, LN) и её варианты повсеместно используются в Трансформерах и считаются критически важными для стабилизации обучения и улучшения производительности. Однако новая статья исследователей из Meta AI, NYU, MIT и Принстонского университета оспаривает это фундаментальное предположение, демонстрируя, что Трансформеры могут достигать эквивалентной или лучшей производительности без традиционных слоев нормализации.\n\n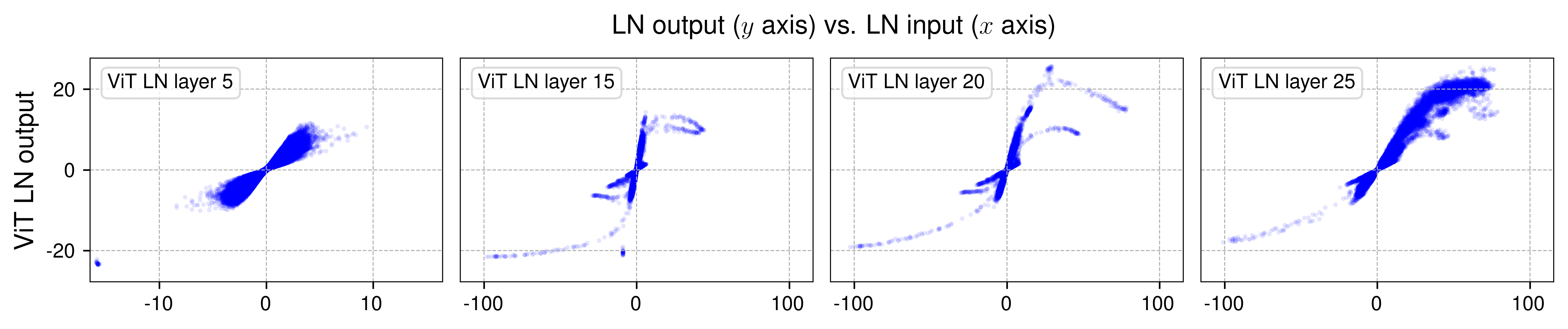\n*Рисунок 1: Визуализация поведения входа-выхода нормализации слоя в различных слоях ViT, показывающая S-образные, подобные тангенсу зависимости.*\n\n## Понимание слоев нормализации\n\nМетоды нормализации, такие как пакетная нормализация, нормализация слоев и RMSNorm, стали стандартной практикой в глубоком обучении. Эти методы обычно нормализуют активации путем вычисления статистик (среднего значения и/или стандартного отклонения) по заданным измерениям, помогая стабилизировать обучение путем контроля распределения активаций сети.\n\nВ Трансформерах, в частности, нормализация слоя работает путем вычисления среднего значения и стандартного отклонения по размерности признаков для каждого токена или позиции. Этот процесс нормализации требует значительных вычислительных ресурсов, так как требует вычисления этих статистик на каждом слое как во время обучения, так и во время вывода.\n\nАвторы заметили, что нормализация слоя часто создает подобные тангенсу, S-образные отображения входа-выхода, как показано на Рисунке 1. Это наблюдение привело к их ключевому пониманию: возможно, полезный эффект нормализации можно достичь через более простой механизм, который имитирует это S-образное поведение без вычисления статистик активации.\n\n## Решение с динамическим тангенсом\n\nИсследователи предлагают динамический тангенс (DyT) как простую замену слоям нормализации. DyT определяется как:\n\n```\nDyT(x) = tanh(αx)\n```\n\nГде α - обучаемый параметр, который контролирует крутизну функции тангенса. Эта простая формулировка устраняет необходимость вычисления статистик активации, сохраняя при этом S-образное преобразование, которое, по-видимому, важно для производительности Трансформера.\n\n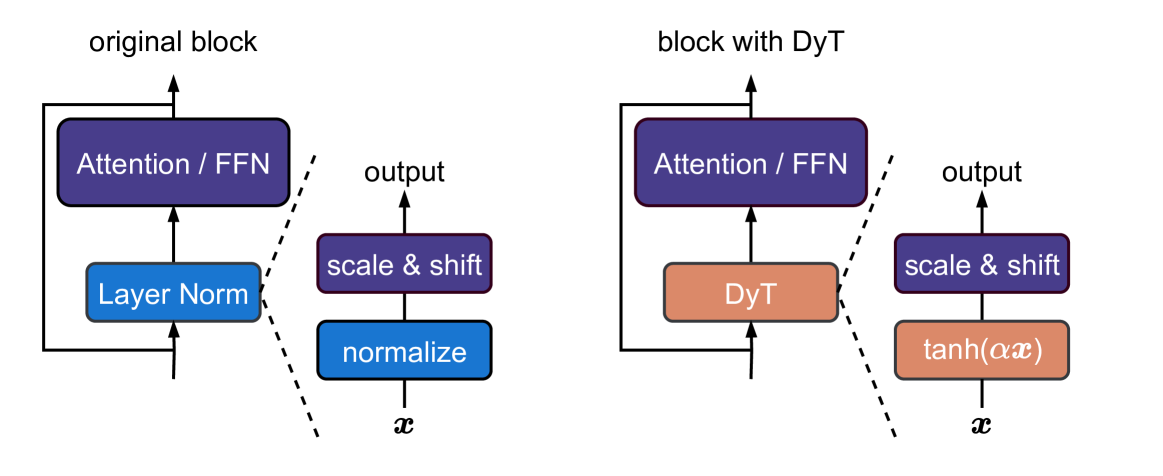\n*Рисунок 2: Слева: Оригинальный блок Трансформера с нормализацией слоя. Справа: Предложенный блок с заменой на динамический тангенс (DyT).*\n\nКрасота этого подхода заключается в его простоте - замена сложных операций нормализации на единственную поэлементную операцию с обучаемым параметром. Рисунок 2 показывает, как традиционный блок Трансформера с нормализацией слоя сравнивается с предложенным блоком с DyT.\n\n## Как работает DyT\n\nДинамический тангенс работает через два ключевых механизма:\n\n1. **Сжатие значений**: Функция тангенса сжимает экстремальные значения, обеспечивая форму неявной регуляризации, подобную слоям нормализации. Это предотвращает слишком большой рост активаций во время прямого и обратного проходов.\n\n2. **Адаптивное масштабирование**: Обучаемый параметр α регулирует крутизну функции tanh, позволяя сети контролировать, насколько агрессивно сжимаются значения. Эта адаптивность критически важна для производительности.\n\nГиперболический тангенс (tanh) ограничен значениями от -1 до 1, сжимая любое входное значение в этот диапазон. Крутизна этого сжатия контролируется параметром α:\n\n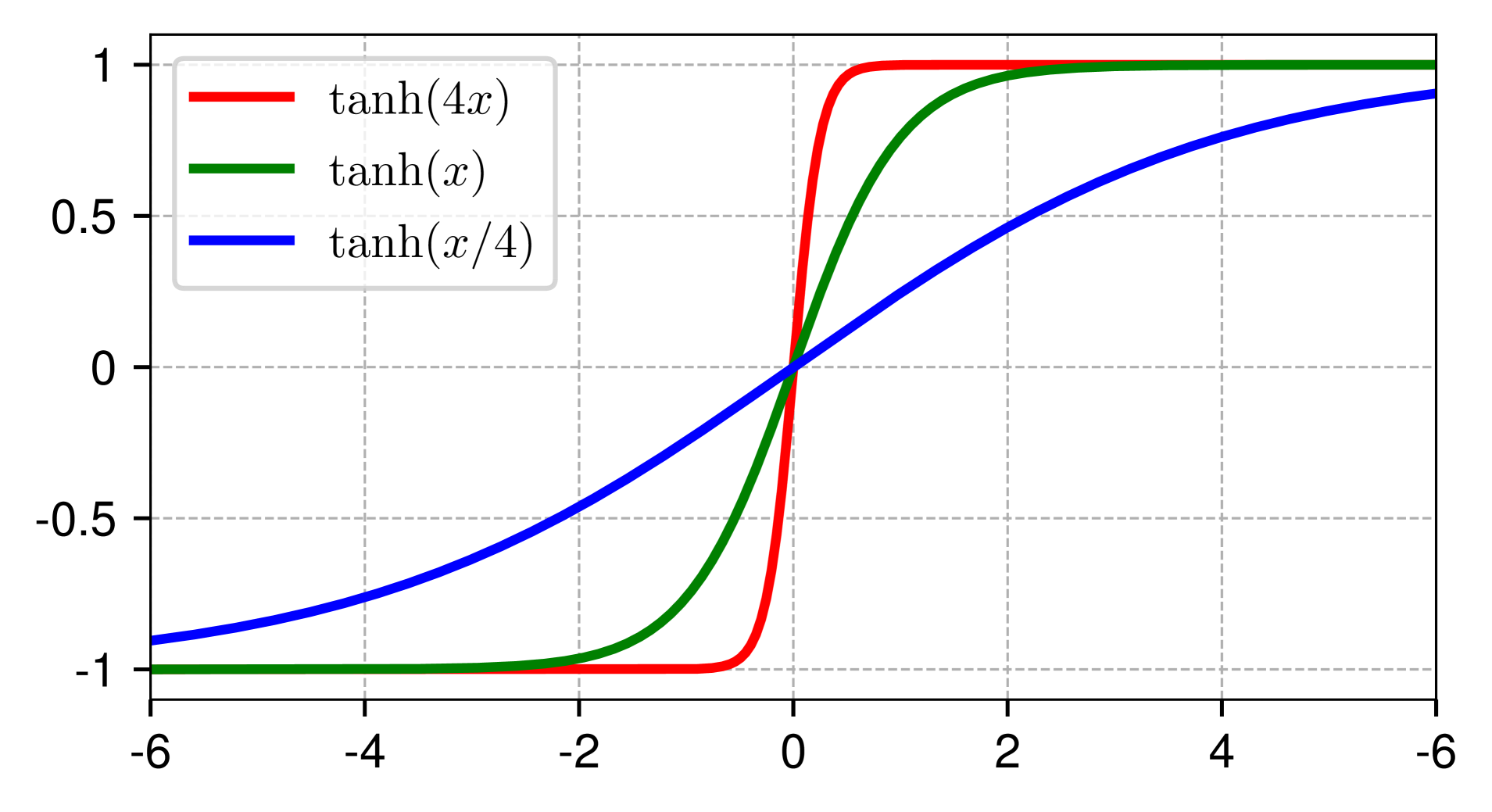\n*Рисунок 3: Функция tanh с разными значениями α, показывающая, как большие значения α создают более резкие переходы.*\n\nКак показано на Рисунке 3, большее значение α делает переход от -1 к 1 более резким, в то время как меньшее α делает его более плавным. Эта гибкость позволяет сети регулировать степень сжатия значений в зависимости от задачи и глубины слоя.\n\n## Экспериментальные доказательства\n\nИсследователи провели обширные эксперименты в различных задачах и областях, чтобы подтвердить эффективность DyT как замены слоев нормализации. Эти эксперименты включали:\n\n1. **Задачи компьютерного зрения**:\n - Классификация ImageNet с использованием Vision Transformers (ViT) и ConvNeXt\n - Самоконтролируемое обучение с MAE и DINO\n\n2. **Генеративные модели**:\n - Диффузионные модели для генерации изображений (DiT)\n\n3. **Большие языковые модели**:\n - Предварительное обучение LLaMA в масштабах от 7B до 70B параметров\n\n4. **Другие области**:\n - Обработка речи с wav2vec 2.0\n - Моделирование последовательностей ДНК с HyenaDNA и Caduceus\n\nРезультаты последовательно показывали, что Трансформеры с DyT могли соответствовать или превосходить производительность их нормализованных аналогов. Например, в классификации ImageNet с Vision Transformers, вариант с DyT достиг сопоставимой точности с версией LN:\n\n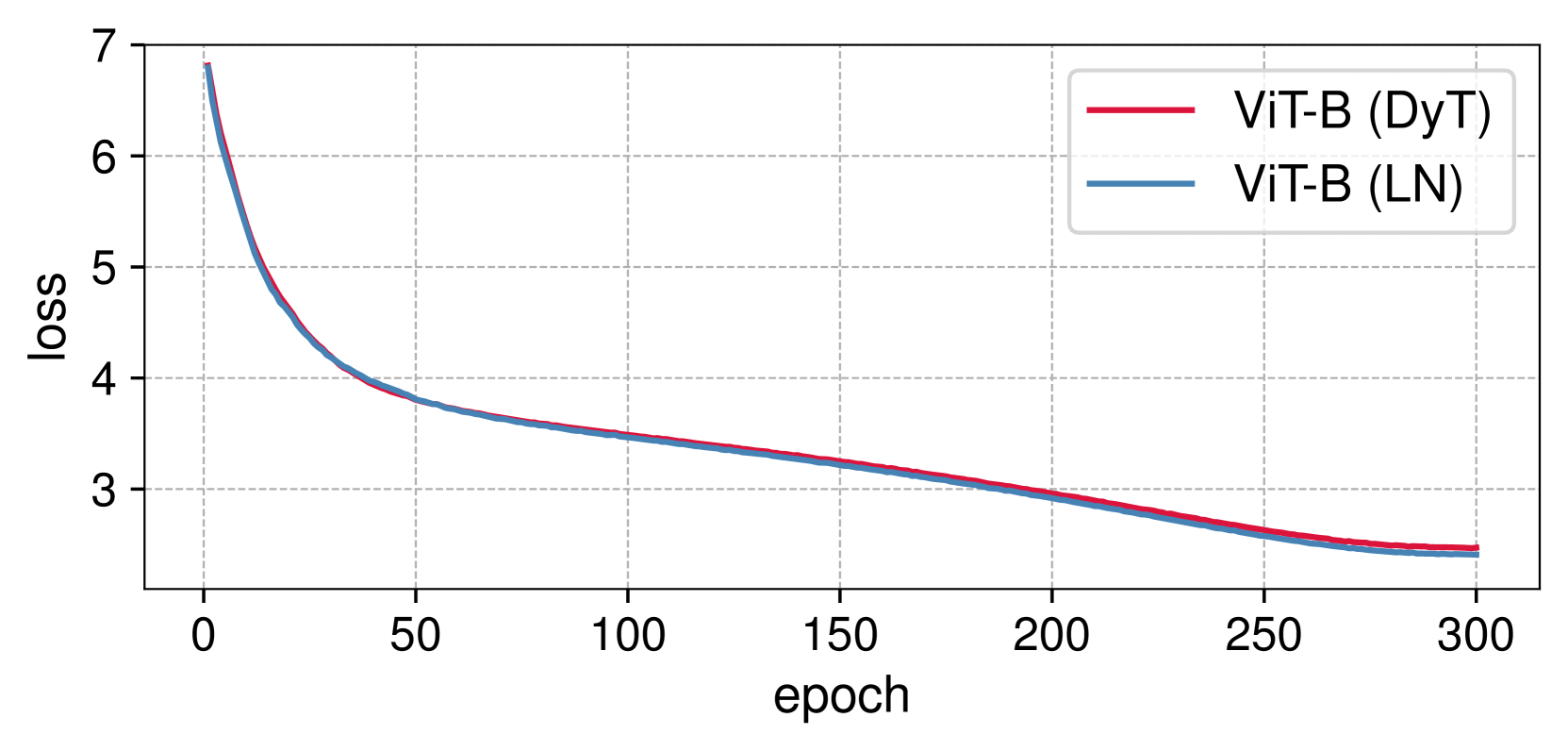\n*Рисунок 4: Кривые потерь при обучении для ViT-B с Layer Normalization (LN) и Dynamic Tanh (DyT), показывающие практически идентичную сходимость.*\n\nАналогично, для моделей LLaMA различных размеров (от 7B до 70B параметров), варианты с DyT достигли сопоставимых или немного лучших значений потерь по сравнению с моделями RMSNorm:\n\n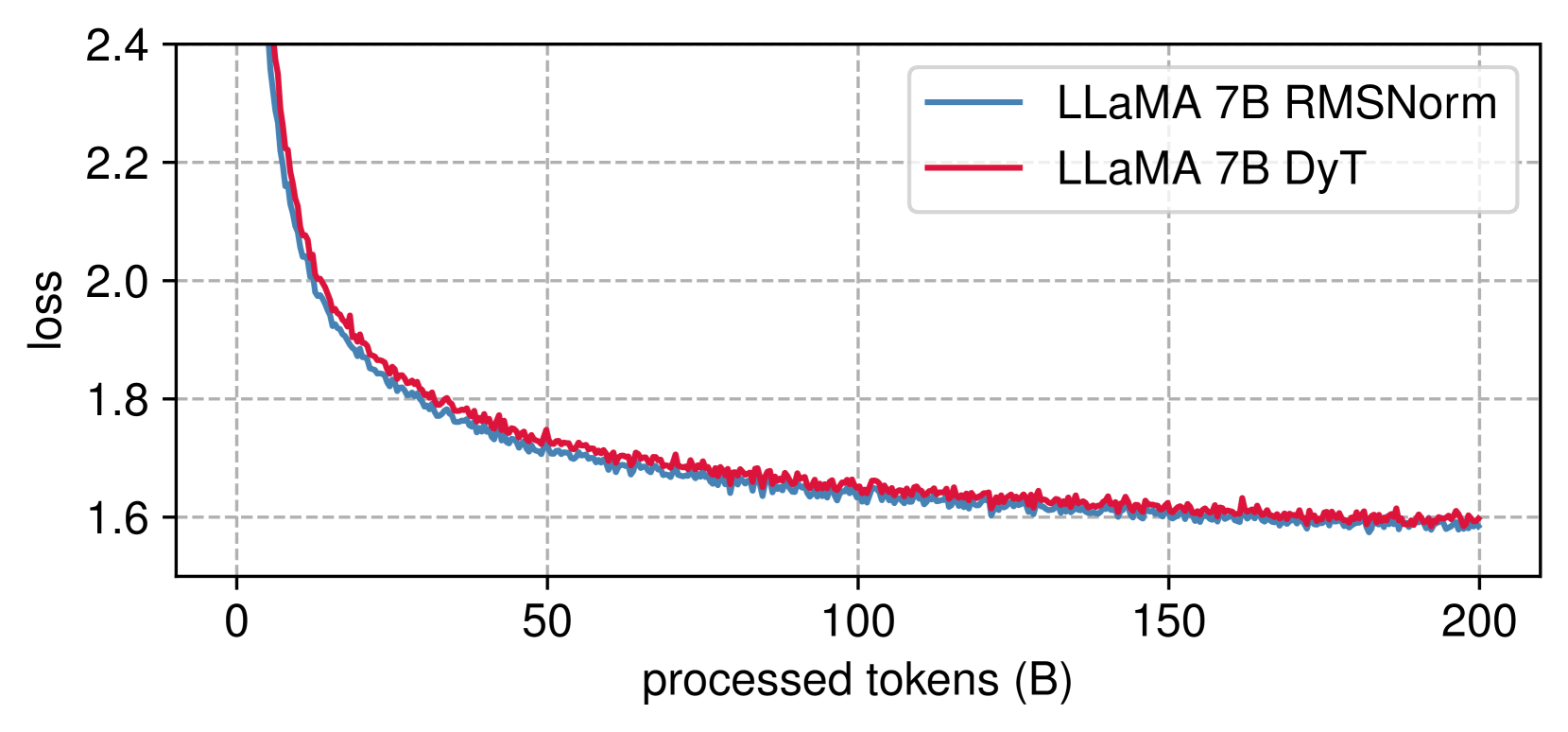\n*Рисунок 5: Кривые потерь при обучении для LLaMA 7B с RMSNorm и DyT, показывающие сопоставимую производительность.*\n\n## Настройка и масштабируемость\n\nХотя DyT в целом надежен и хорошо работает с минимальной настройкой, исследователи обнаружили, что для более крупных моделей, особенно больших языковых моделей (LLM), важна тщательная инициализация α. Они провели тщательное исследование значений инициализации для архитектуры LLaMA:\n\n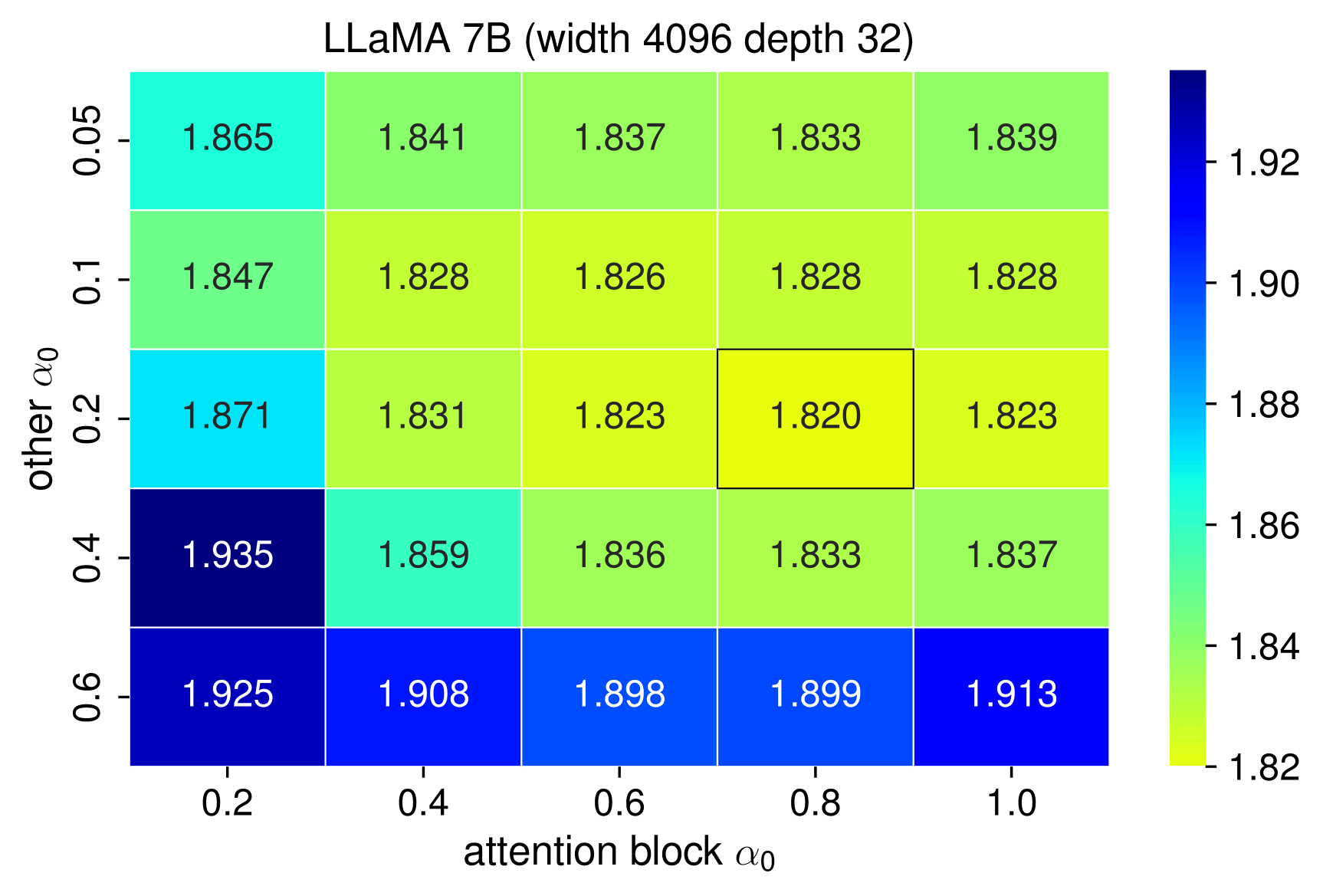\n*Рисунок 6: Тепловая карта, показывающая производительность LLaMA 7B с различными значениями инициализации α для блоков внимания и прямой связи.*\n\nДля LLaMA 7B оптимальная инициализация α оказалась равной 0.2 для блоков внимания и 0.2 для других блоков, в то время как для LLaMA 13B она составила 0.6 для блоков внимания и 0.15 для других блоков. Это предполагает, что более крупные модели могут требовать более тщательной настройки параметра α.\n\nИсследователи также проверили масштабируемость своего подхода, обучая модели различной глубины и ширины:\n\n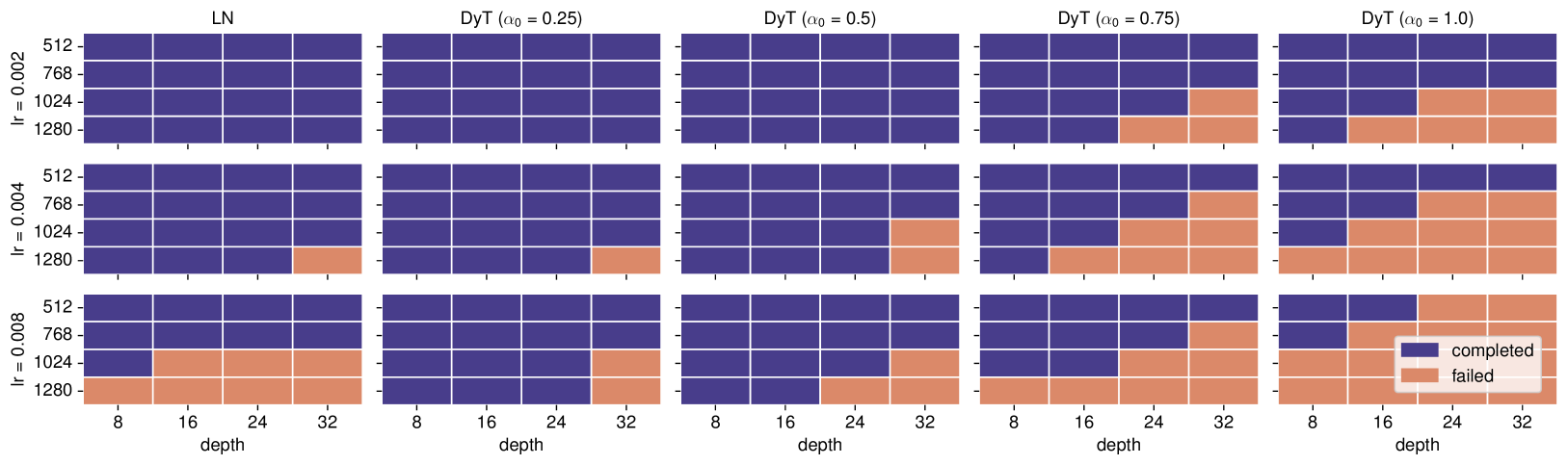\n*Рисунок 7: Сравнение стабильности обучения между LN и DyT при различных глубинах и ширинах модели, где синий цвет указывает на успешное обучение, а оранжевый - на нестабильность.*\n\nРезультаты показали, что модели DyT могут масштабироваться сопоставимо с моделями LN, хотя с некоторой дополнительной чувствительностью к скорости обучения при больших масштабах.\n\n## Анализ параметра Alpha\n\nИсследователи проанализировали, как параметр α в DyT связан со статистическими свойствами активаций. Интересно, что они обнаружили, что α учится аппроксимировать обратное значение стандартного отклонения активаций слоя:\n\n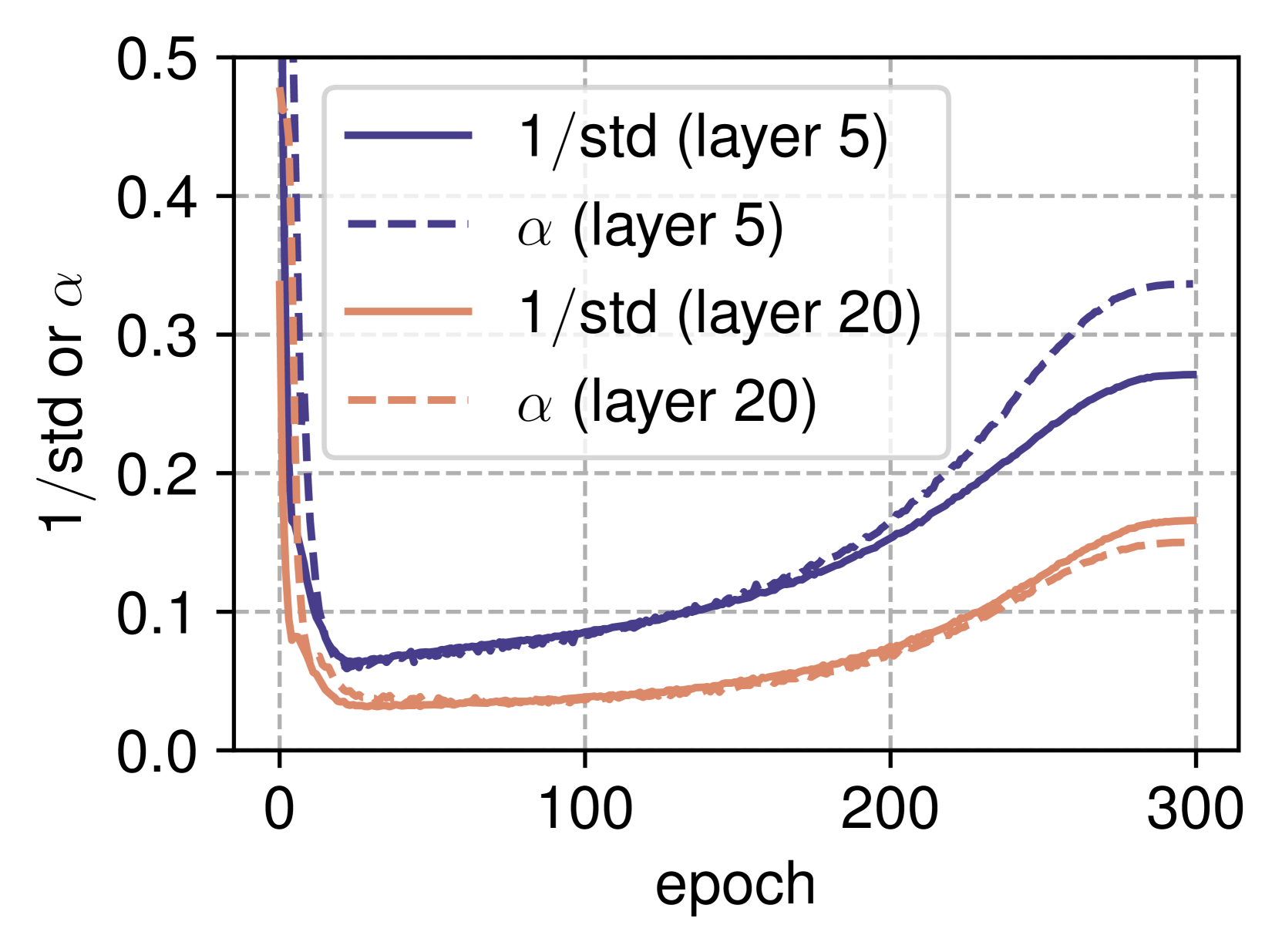\n*Рисунок 8: Сравнение между изученными значениями α и обратной величиной стандартного отклонения активации (1/std) в течение эпох обучения, показывающее, как α частично имитирует поведение нормализации.*\n\nЭто открытие предполагает, что DyT неявно учится выполнять форму адаптивного масштабирования, подобную нормализационным слоям, но без явного вычисления статистик. Параметр α, как правило, обратно пропорционален стандартному отклонению активаций, эффективно масштабируя входные данные так, чтобы их величина была подходящей для функции tanh.\n\nБолее того, они наблюдали устойчивую корреляцию между изученными значениями α и обратным стандартным отклонением активаций в разных слоях и моделях:\n\n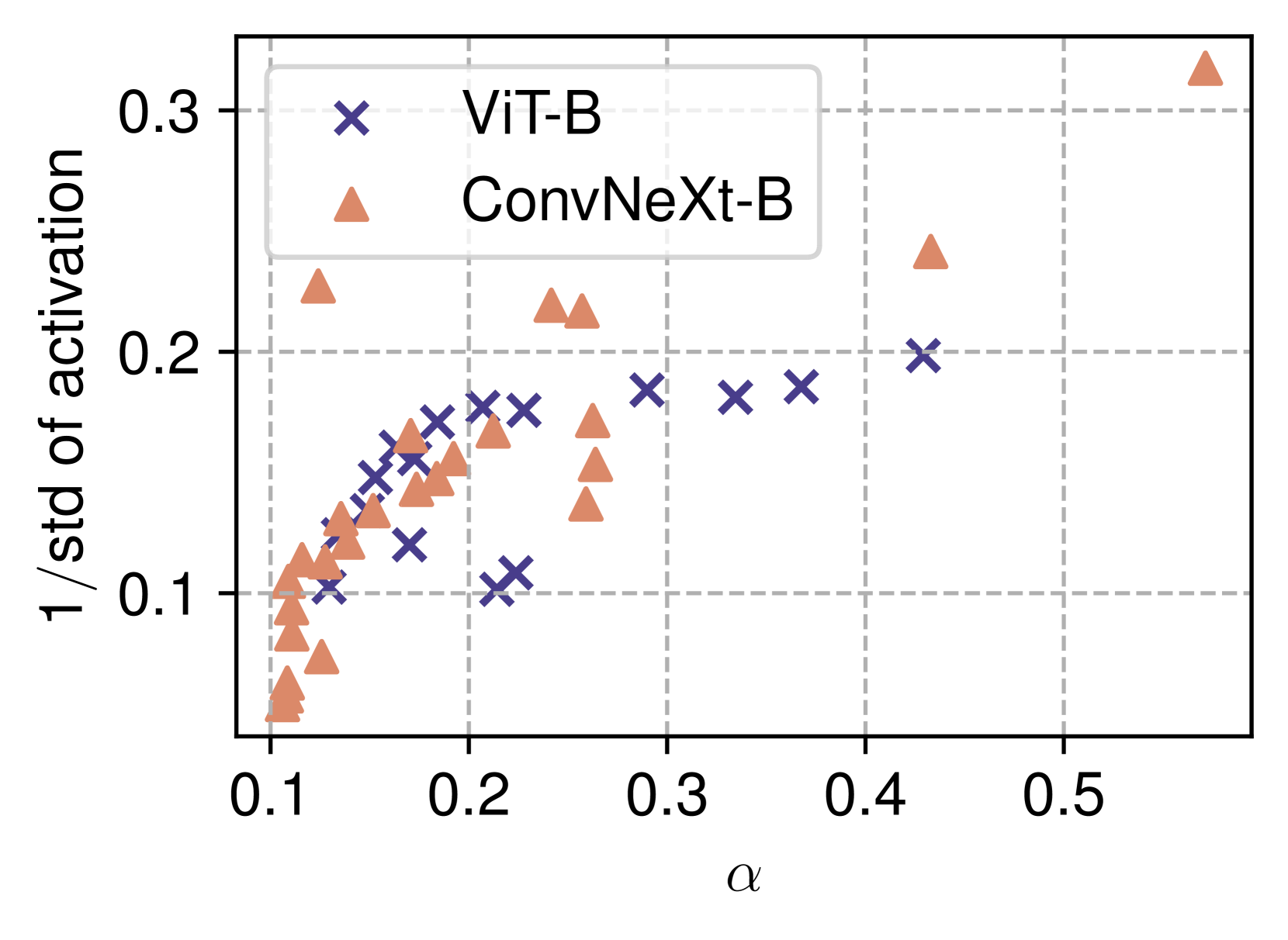\n*Рисунок 9: Диаграмма рассеяния, показывающая связь между изученными значениями α и обратным стандартным отклонением активаций в различных слоях моделей ViT-B и ConvNeXt-B.*\n\n## Сравнение с другими подходами\n\nИсследователи сравнили DyT с другими методами, предложенными для обучения глубоких сетей без нормализации, включая Fixup, SkipInit и σReparam. В различных задачах и архитектурах моделей DyT стабильно превосходил эти альтернативы.\n\nОни также провели абляционные исследования для проверки важности как функции tanh, так и обучаемого параметра масштаба α. Эти исследования показали, что:\n\n1. Замена tanh другими функциями, такими как sigmoid или hardtanh, приводила к снижению производительности, подчеркивая важность специфических свойств tanh.\n\n2. Использование фиксированного α вместо обучаемого значительно ухудшало производительность, демонстрируя важность адаптивности.\n\n3. Полное удаление нелинейности (использование только обучаемого масштаба) приводило к нестабильности обучения, указывая на то, что ограниченность tanh имеет решающее значение.\n\nТакже было изучено влияние начальных значений α на производительность модели для различных задач:\n\n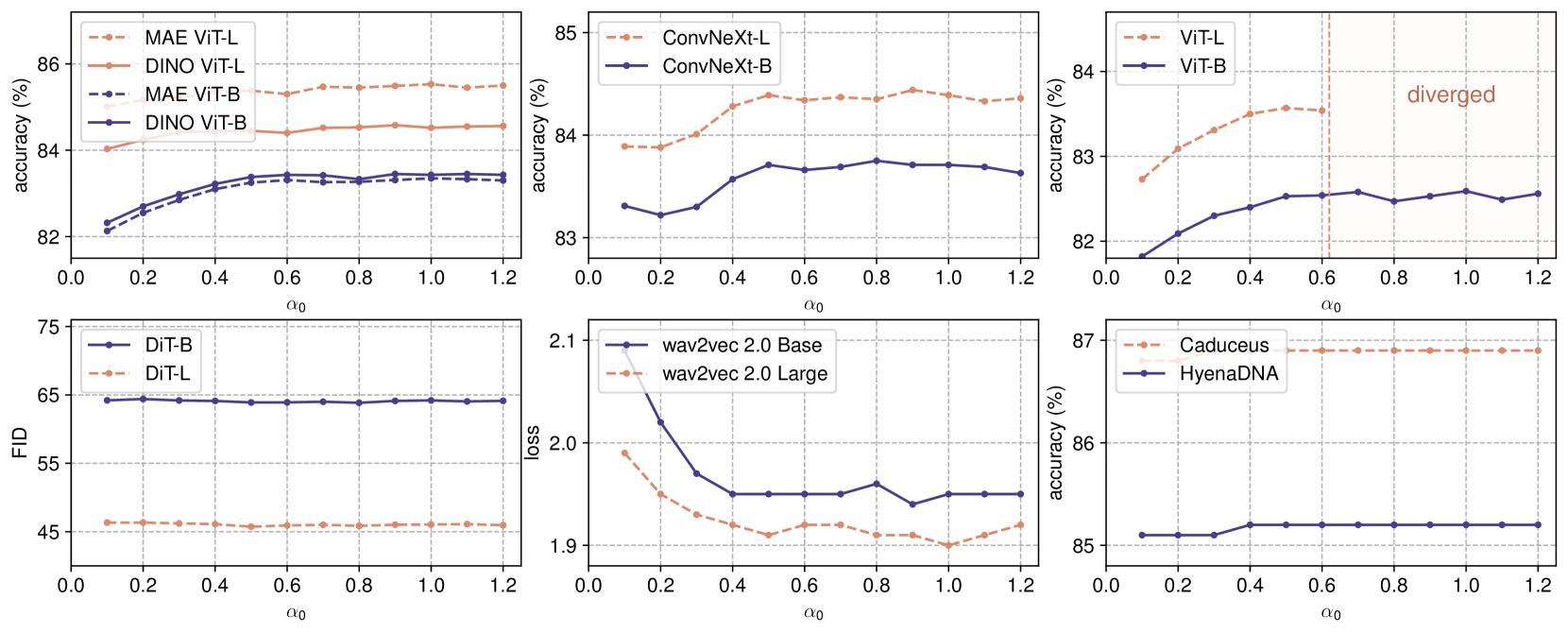\n*Рисунок 10: Производительность различных моделей с разными значениями инициализации α (α₀), показывающая зависимость чувствительности от задачи.*\n\n## Последствия и применения\n\nРезультаты этого исследования имеют несколько важных последствий:\n\n1. **Упрощение архитектуры**: Заменяя нормализационные слои на DyT, архитектуры трансформеров могут быть упрощены, потенциально приводя к более интерпретируемым моделям.\n\n2. **Вычислительная эффективность**: Предварительные измерения показывают, что DyT может улучшить скорость обучения и вывода по сравнению с нормализационными слоями, так как устраняет необходимость вычисления статистик.\n\n3. **Теоретическое понимание**: Успех DyT дает представление о фундаментальной роли нормализации в глубоком обучении, предполагая, что ключевым преимуществом может быть S-образное преобразование, а не нормализация статистик как таковая.\n\n4. **Применимость в разных областях**: Постоянный успех DyT в различных областях (зрение, язык, речь, биология) предполагает, что он охватывает фундаментальный принцип оптимизации глубокого обучения.\n\nОдним из ограничений, отмеченных авторами, является то, что DyT может быть не напрямую применим к классическим архитектурам CNN, использующим пакетную нормализацию, без дополнительных исследований. Основное внимание в их работе было сосредоточено на архитектурах трансформеров.\n\n## Заключение\n\nСтатья \"Трансформеры без нормализации\" представляет значительный вклад в проектирование архитектуры глубокого обучения, демонстрируя, что нормализационные слои в трансформерах могут быть эффективно заменены простой операцией Dynamic Tanh (DyT). Это ставит под сомнение общепринятое мнение о том, что нормализационные слои незаменимы для обучения высокопроизводительных трансформеров.\n\nПредложенный подход DyT представляет собой убедительную альтернативу, которую легко реализовать, часто требует минимальной настройки и может соответствовать или превосходить производительность нормализованных моделей в широком спектре задач и областей. Обнаружение того, что α в DyT учится аппроксимировать обратную величину стандартного отклонения активации, дает представление о том, как этот простой механизм эффективно имитирует определенные аспекты нормализации.\n\nЭто исследование открывает новые пути для упрощения архитектур нейронных сетей и может вдохновить на дальнейшее изучение альтернатив традиционным методам нормализации. По мере развития глубокого обучения такие упрощения могут способствовать созданию более эффективных и интерпретируемых моделей.\n\n## Соответствующие цитаты\n\nJimmy Lei Ba, Jamie Ryan Kiros и Geoffrey E Hinton. [Нормализация слоев](https://alphaxiv.org/abs/1607.06450). arXiv preprint arXiv:1607.06450, 2016.\n\n * Эта статья представляет Layer Normalization (LN), важнейший компонент для стабилизации обучения в глубоких сетях, особенно в Трансформерах. В статье анализируется поведение LN и предлагается Dynamic Tanh (DyT) в качестве замены, что делает эту цитату особенно актуальной.\n\nAlexey Dosovitskiy, Lucas Beyer, Alexander Kolesnikov, Dirk Weissenborn, Xiaohua Zhai, Thomas Unterthiner, Mostafa Dehghani, Matthias Minderer, Georg Heigold, Sylvain Gelly и др. Изображение стоит 16x16 слов: Трансформеры для распознавания изображений в масштабе. arXiv preprint arXiv:2010.11929, 2020.\n\n * Эта статья представляет Vision Transformer (ViT), известную архитектуру, используемую для оценки эффективности DyT в задачах классификации изображений. В статье используется ViT как основная архитектура для демонстрации того, что DyT может заменить нормализацию слоев.\n\nBiao Zhang и Rico Sennrich. [Нормализация слоя среднеквадратичного корня](https://alphaxiv.org/abs/1910.07467). NeurIPS, 2019.\n\n * Эта работа представляет RMSNorm, альтернативу Layer Normalization, и используется как базовое сравнение для DyT, особенно в экспериментах с большими языковыми моделями. В статье исследуется DyT как замена как LN, так и RMSNorm.\n\nHugo Touvron, Thibaut Lavril, Gautier Izacard, Xavier Martinet, Marie-Anne Lachaux, Timothée Lacroix, Baptiste Rozière, Naman Goyal, Eric Hambro, Faisal Azhar и др. [Llama: Открытые и эффективные базовые языковые модели](https://alphaxiv.org/abs/2302.13971). arXiv preprint arXiv:2302.13971, 2023a.\n\n * Эта цитата представляет языковую модель LLaMA, которая служит ключевой архитектурой для тестирования и оценки DyT в контексте больших языковых моделей. В статье используется LLaMA как важная архитектура для проверки обобщаемости DyT.\n\nAshish Vaswani, Noam Shazeer, Niki Parmar, Jakob Uszkoreit, Llion Jones, Aidan N Gomez, Łukasz Kaiser и Illia Polosukhin. [Внимание - это всё, что нужно](https://alphaxiv.org/abs/1706.03762). NeurIPS, 2017.\n\n * Эта фундаментальная статья представляет архитектуру Transformer, которая является основным фокусом исследования DyT. В статье основное внимание уделяется демонстрации того, как DyT может улучшить Трансформеры."])</script><script>self.__next_f.push([1,"92:T2f3a,"])</script><script>self.__next_f.push([1,"# 无归一化的Transformer:动态双曲正切函数的简单替代方案\n\n## 目录\n- [简介](#简介)\n- [理解归一化层](#理解归一化层)\n- [动态双曲正切函数解决方案](#动态双曲正切函数解决方案)\n- [DyT的工作原理](#dyt的工作原理)\n- [实验证据](#实验证据)\n- [调优和可扩展性](#调优和可扩展性)\n- [Alpha参数分析](#alpha参数分析)\n- [与其他方法的比较](#与其他方法的比较)\n- [影响和应用](#影响和应用)\n- [结论](#结论)\n\n## 简介\n\n归一化层一直被认为是现代神经网络中的重要组成部分,特别是在主导自然语言处理、计算机视觉和其他领域的Transformer架构中。层归一化(LN)及其变体在Transformer中无处不在,被认为对于稳定训练和提高性能至关重要。然而,来自Meta AI、纽约大学、麻省理工学院和普林斯顿大学的研究人员在一篇新论文中挑战了这一基本假设,他们证明Transformer无需传统归一化层也能达到同等或更好的性能。\n\n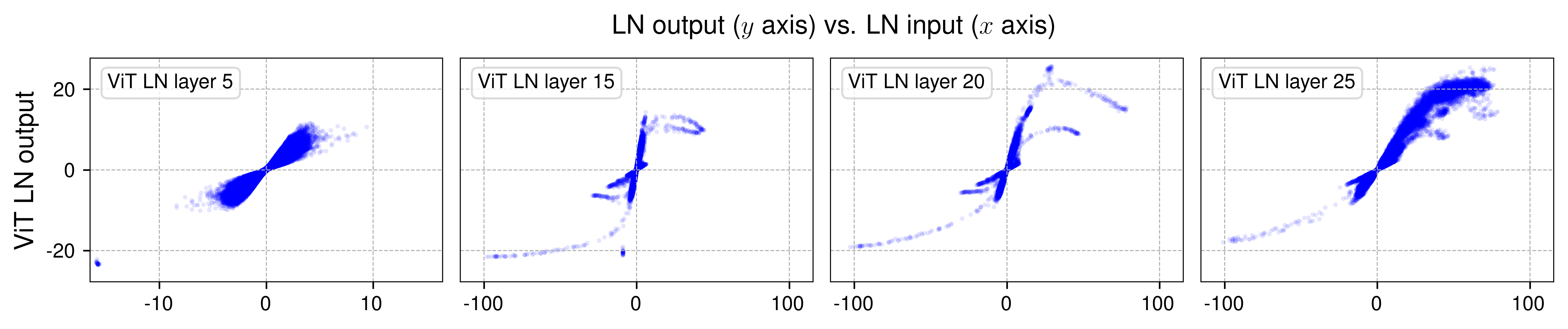\n*图1:各种ViT层中层归一化的输入输出行为可视化,显示出S形、类似双曲正切的关系。*\n\n## 理解归一化层\n\n批量归一化、层归一化和RMSNorm等归一化技术已成为深度学习中的标准实践。这些方法通常通过计算指定维度上的统计量(均值和/或标准差)来归一化激活值,通过控制网络激活值的分布来帮助稳定训练。\n\n特别是在Transformer中,层归一化通过计算每个标记或位置的特征维度上的均值和标准差来运作。这个归一化过程在计算上很昂贵,因为它需要在训练和推理期间在每一层计算这些统计量。\n\n作者观察到层归一化通常产生类似双曲正切的S形输入输出映射,如图1所示。这一观察导致了他们的关键洞见:也许归一化的有益效果可以通过一个更简单的机制来实现,该机制模仿这种S形行为而无需计算激活统计量。\n\n## 动态双曲正切函数解决方案\n\n研究人员提出动态双曲正切函数(DyT)作为归一化层的直接替代品。DyT定义为:\n\n```\nDyT(x) = tanh(αx)\n```\n\n其中α是一个可学习参数,用于控制双曲正切函数的陡度。这种简单的公式消除了计算激活统计量的需求,同时保留了对Transformer性能似乎很重要的S形变换。\n\n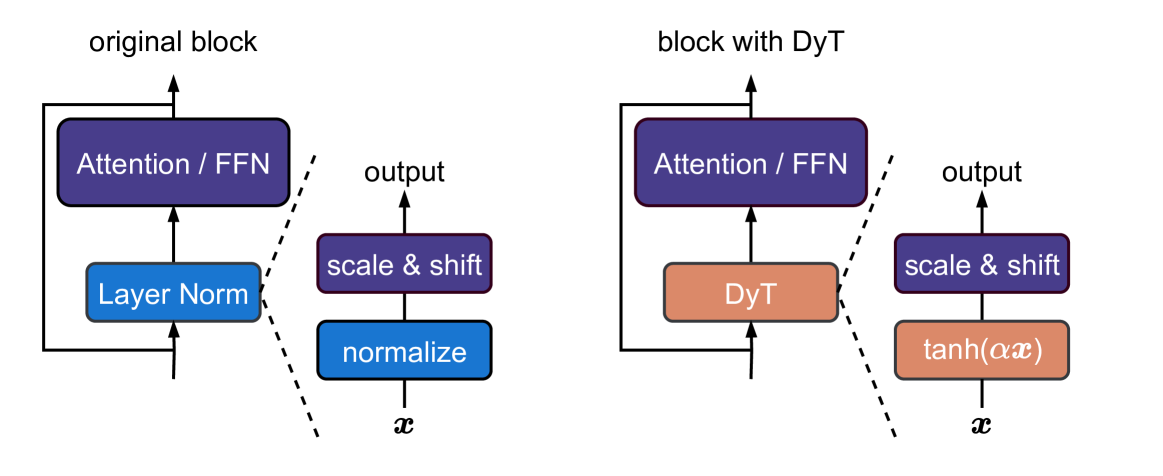\n*图2:左:带有层归一化的原始Transformer模块。右:提出的带有动态双曲正切函数(DyT)替代的模块。*\n\n这种方法的优美之处在于其简单性——用一个具有可学习参数的单一元素级操作替代复杂的归一化操作。图2显示了传统的带有层归一化的Transformer模块与提出的带有DyT的模块的比较。\n\n## DyT的工作原理\n\n动态双曲正切函数通过两个关键机制工作:\n\n1. **值压缩**:双曲正切函数压缩极端值,提供类似于归一化层的隐式正则化。这防止激活值在前向和后向传播过程中变得过大。\n\n2. **自适应缩放**:可学习参数α调整tanh函数的陡峭程度,使网络能够控制值被压缩的程度。这种适应性对性能至关重要。\n\n双曲正切函数(tanh)的取值范围在-1到1之间,将任何输入值压缩到这个范围内。压缩的陡峭程度由α控制:\n\n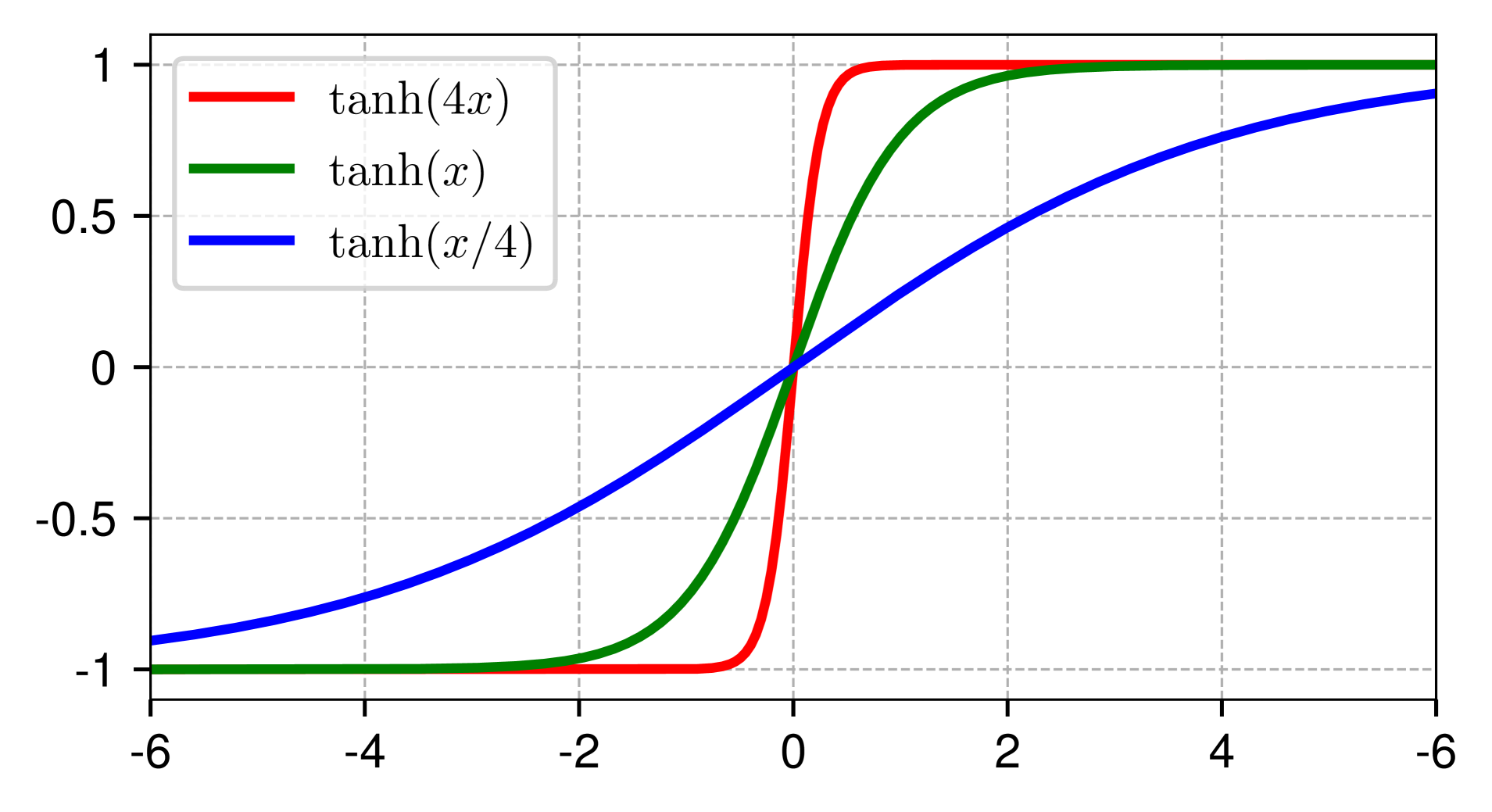\n*图3:具有不同α值的tanh函数,展示了较大的α值如何创造更陡峭的过渡。*\n\n如图3所示,较大的α值使从-1到1的过渡更加陡峭,而较小的α值则使其更加平缓。这种灵活性使网络能够根据任务和层深度调整值压缩的程度。\n\n## 实验证据\n\n研究人员在diverse各种任务和领域进行了广泛的实验,以验证DyT作为归一化层替代品的有效性。这些实验包括:\n\n1. **视觉任务**:\n - 使用Vision Transformers (ViT)和ConvNeXt进行ImageNet分类\n - 使用MAE和DINO进行自监督学习\n\n2. **生成模型**:\n - 用于图像生成的扩散模型(DiT)\n\n3. **大型语言模型**:\n - 从7B到70B参数规模的LLaMA预训练\n\n4. **其他领域**:\n - 使用wav2vec 2.0进行语音处理\n - 使用HyenaDNA和Caduceus进行DNA序列建模\n\n实验结果一致表明,使用DyT的Transformers可以达到或超过其归一化对应版本的性能。例如,在ImageNet分类任务中,DyT变体达到了与LN版本相当的准确率:\n\n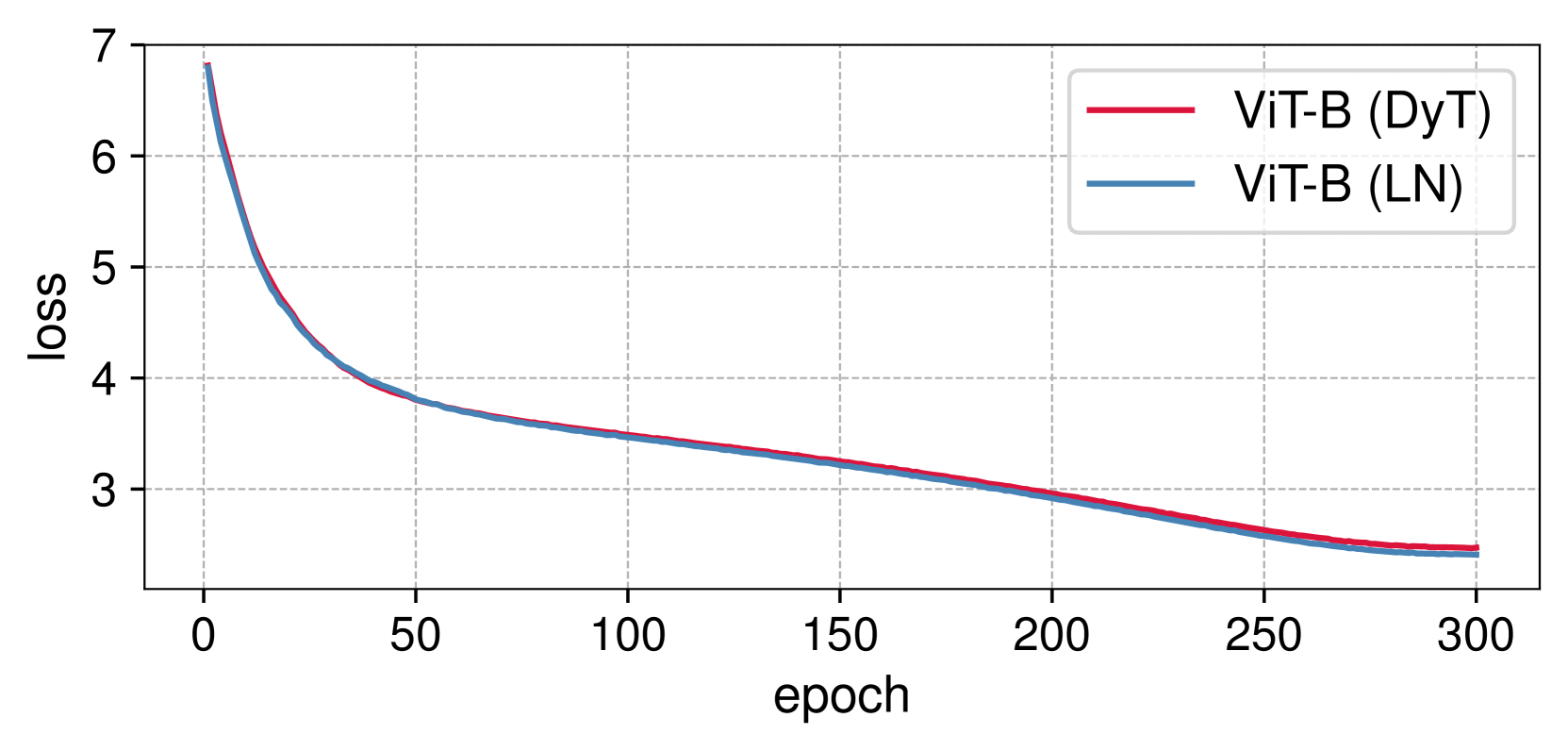\n*图4:ViT-B使用层归一化(LN)和动态Tanh(DyT)的训练损失曲线,显示几乎相同的收敛性。*\n\n同样,对于不同规模的LLaMA模型(7B到70B参数),DyT变体相比RMSNorm模型达到了相当或略好的损失值:\n\n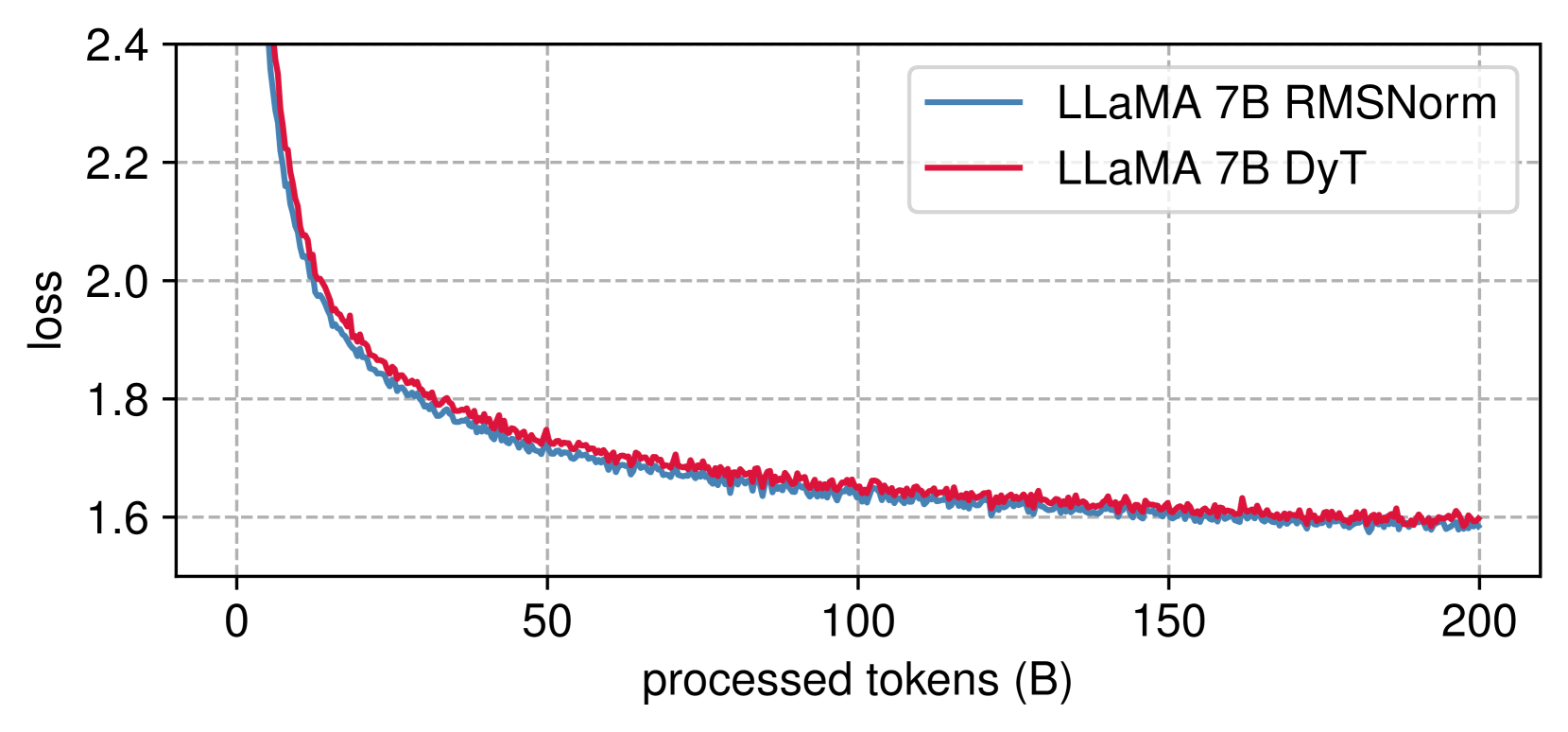\n*图5:LLaMA 7B使用RMSNorm和DyT的训练损失曲线,显示相当的性能。*\n\n## 调优和可扩展性\n\n虽然DyT通常很稳健,只需最少的调优就能工作良好,但研究人员发现对于较大的模型,特别是大型语言模型(LLM),α的初始化很重要。他们对LLaMA架构进行了详细的初始化值探索:\n\n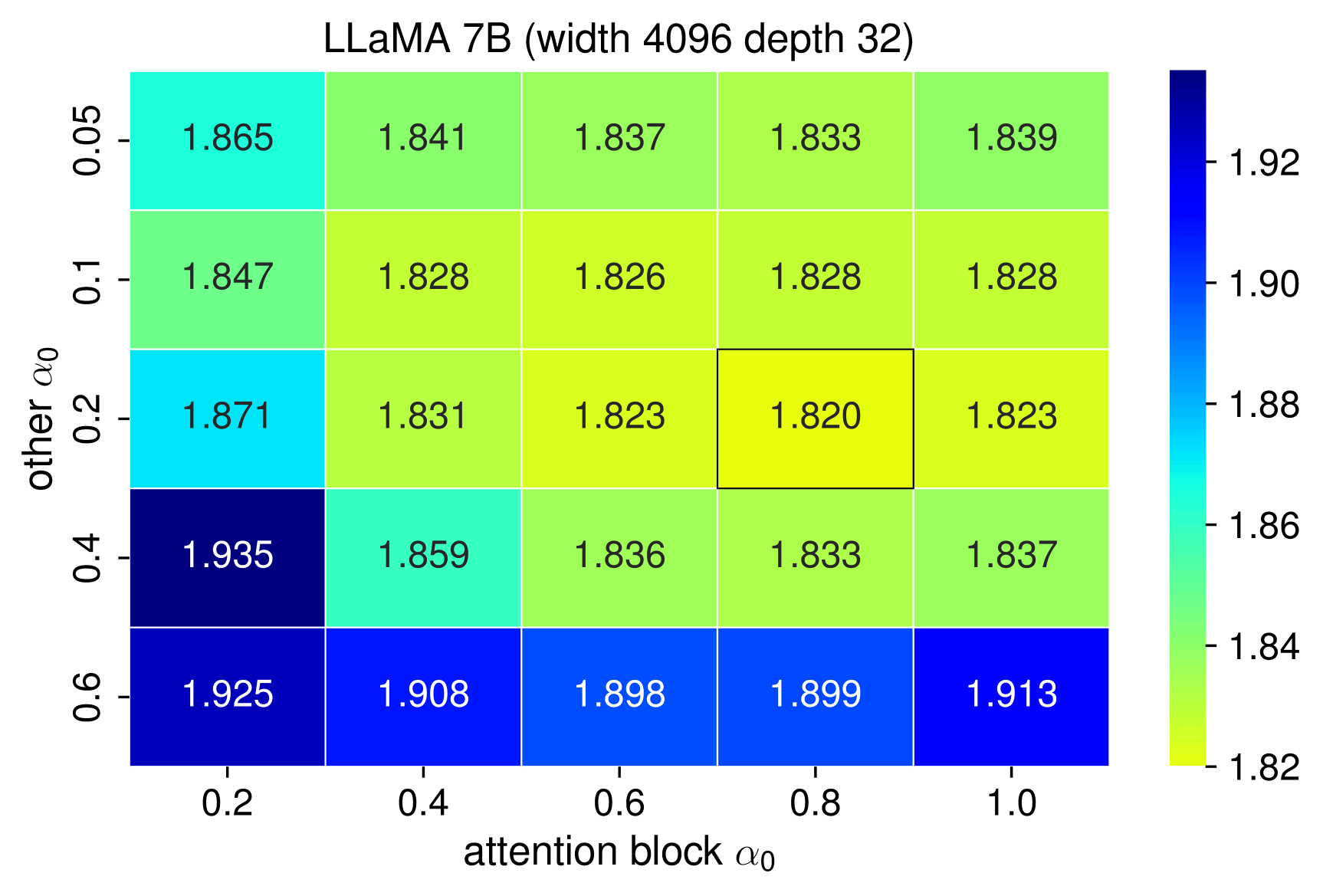\n*图6:热图显示了注意力块和前馈块使用不同α初始化值时LLaMA 7B的性能。*\n\n对于LLaMA 7B,最佳的α初始化值为注意力块0.2,其他块0.2,而对于LLaMA 13B,注意力块为0.6,其他块为0.15。这表明较大的模型可能需要更仔细地调整α参数。\n\n研究人员还通过训练不同深度和宽度的模型测试了他们方法的可扩展性:\n\n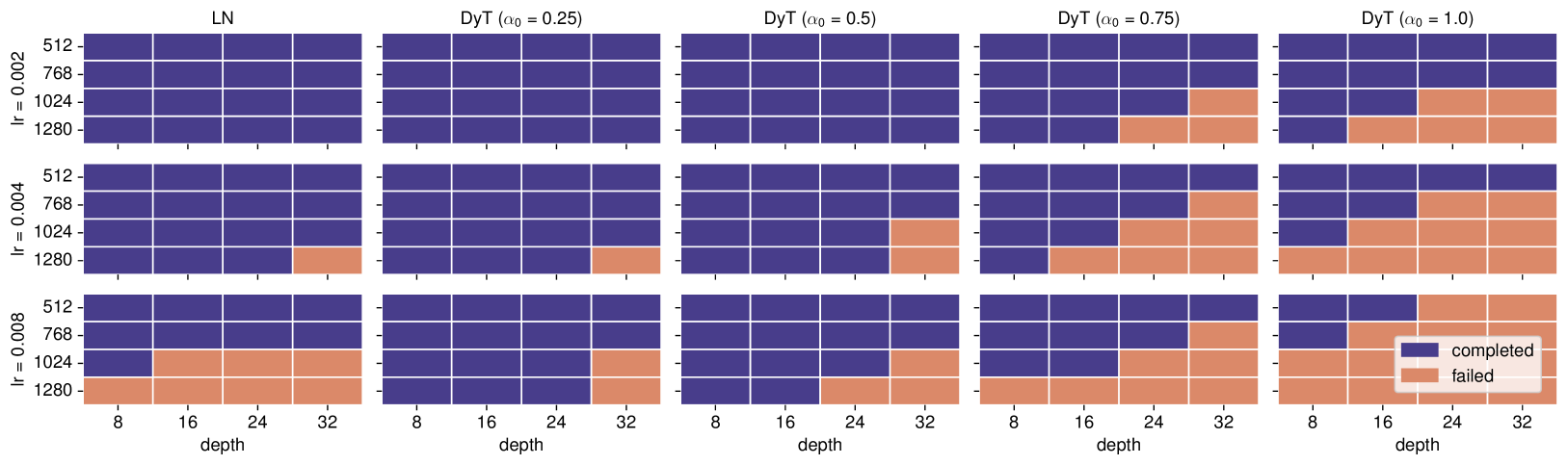\n*图7:在不同模型深度和宽度下LN和DyT的训练稳定性比较,蓝色表示训练成功,橙色表示不稳定。*\n\n结果表明,DyT模型可以与LN模型相当地扩展,但在更大规模时对学习率的敏感度有所增加。\n\n## Alpha参数分析\n\n研究人员分析了DyT中α参数与激活值统计特性的关系。有趣的是,他们发现α学会了近似层激活值标准差的倒数:\n\n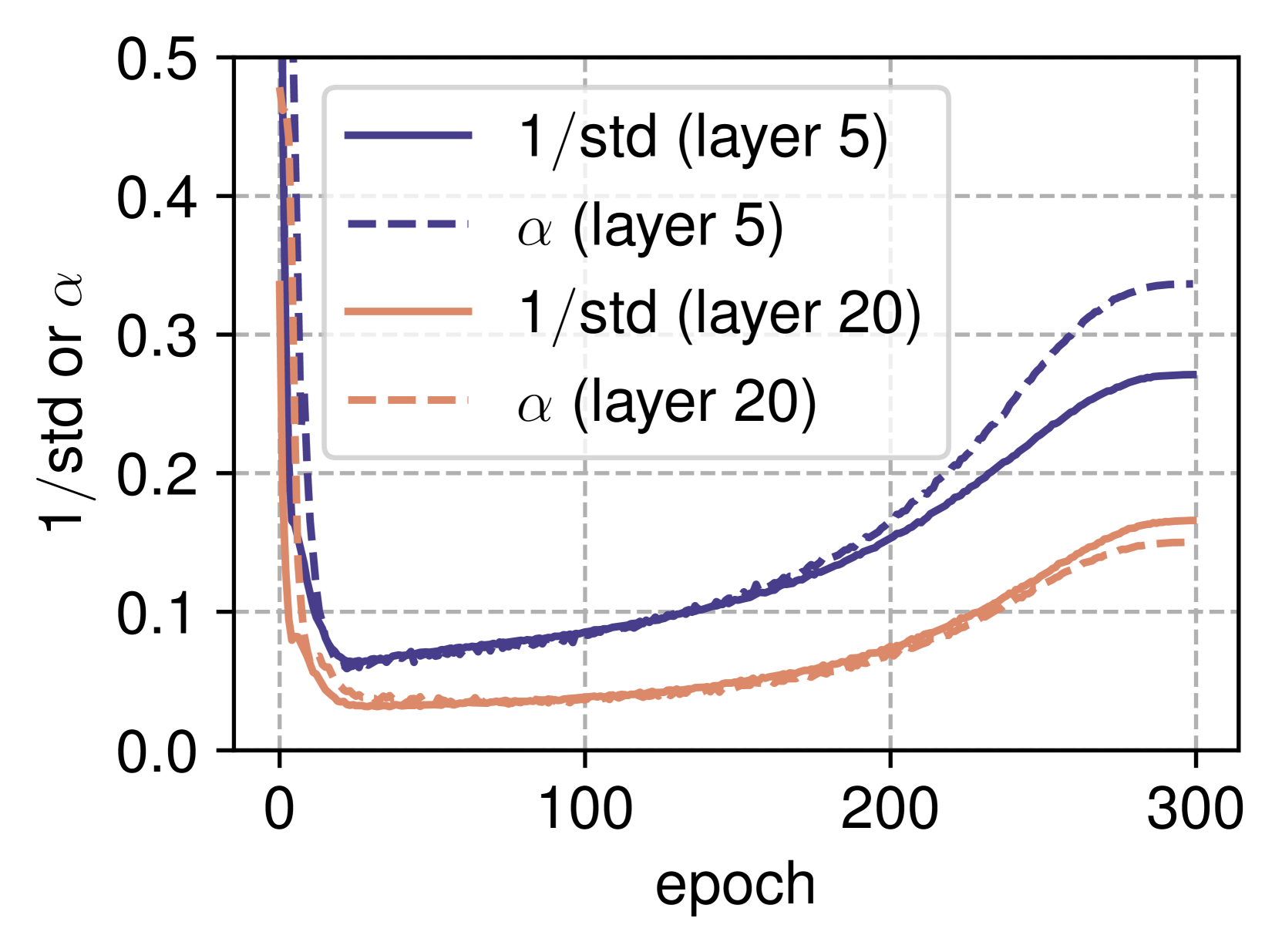\n*图8:训练过程中学习到的α值与激活标准差倒数(1/std)的对比,展示了α是如何部分模拟归一化行为的。*\n\n这一发现表明,DyT隐式地学习执行了一种类似于归一化层的自适应缩放,但无需显式计算统计量。α参数往往与激活值的标准差成反比,有效地缩放输入使其幅度适合tanh函数。\n\n此外,他们观察到在不同层和模型中,学习到的α值与激活标准差倒数之间存在一致的相关性:\n\n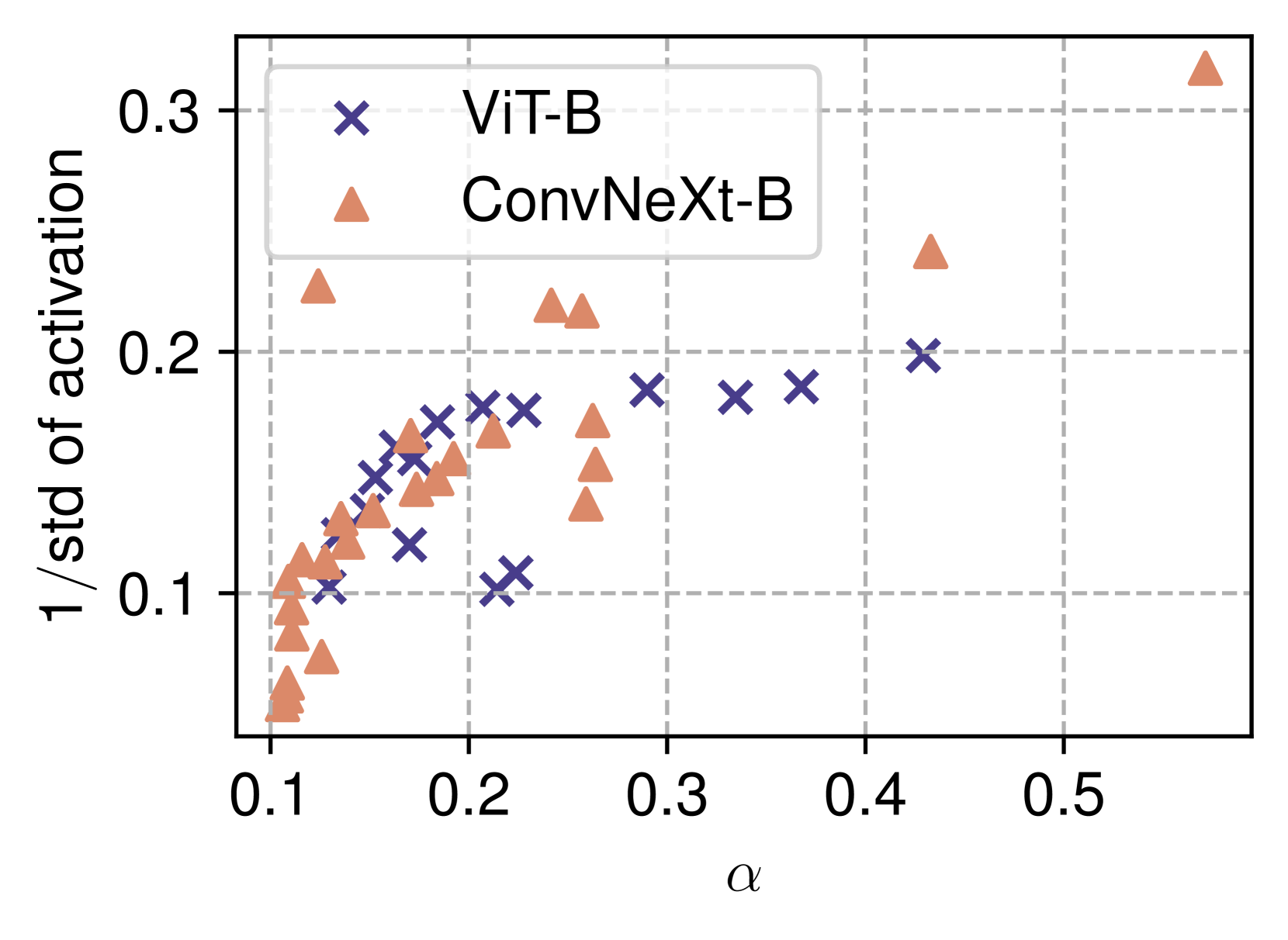\n*图9:散点图显示了ViT-B和ConvNeXt-B模型中不同层的学习α值与激活标准差倒数之间的关系。*\n\n## 与其他方法的比较\n\n研究人员将DyT与其他提出的无归一化深度网络训练方法进行了比较,包括Fixup、SkipInit和σReparam。在各种任务和模型架构中,DyT始终优于这些替代方案。\n\n他们还进行了消融研究,验证了tanh函数和可学习缩放参数α的重要性。这些研究表明:\n\n1. 用sigmoid或hardtanh等其他函数替换tanh会导致性能下降,突显了tanh特性的重要性。\n\n2. 使用固定α而不是可学习的α会显著降低性能,证明了自适应性的重要性。\n\n3. 完全移除非线性(仅使用可学习缩放)会导致训练不稳定,表明tanh的有界性是至关重要的。\n\n研究还探讨了初始α值对不同任务模型性能的影响:\n\n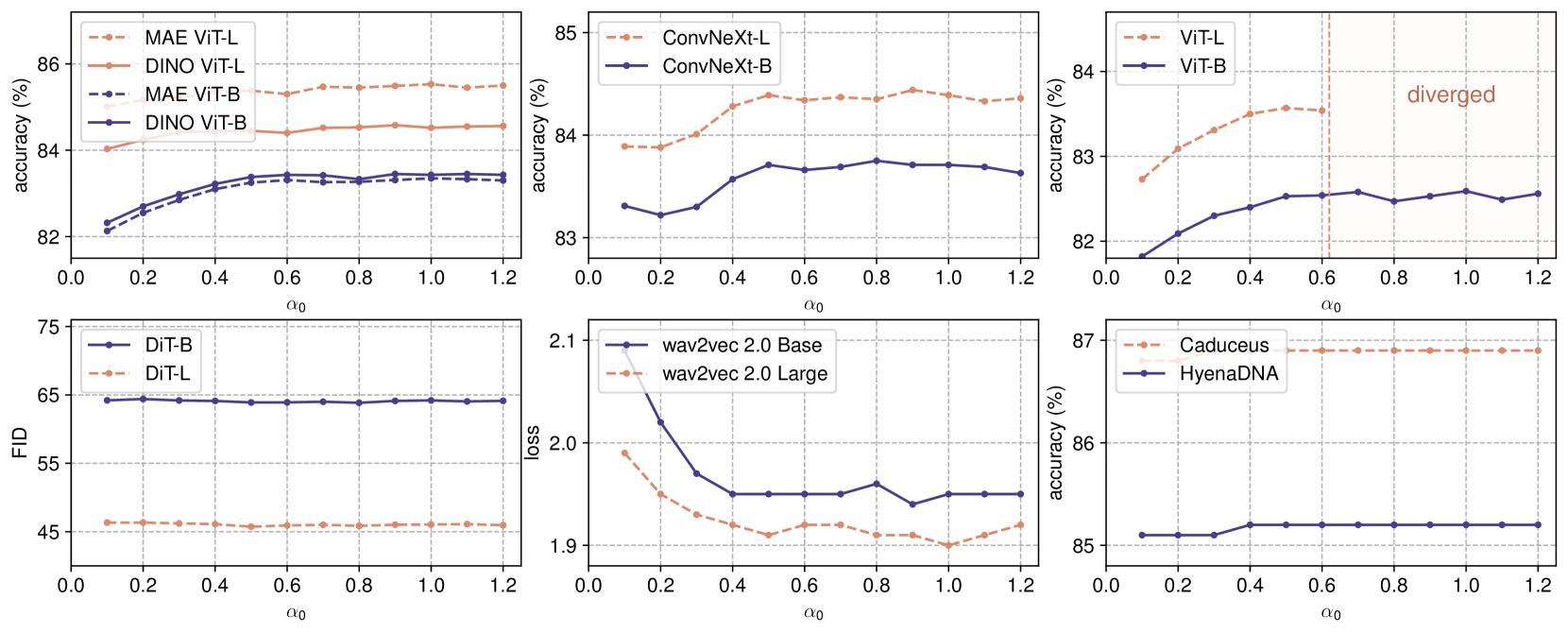\n*图10:不同α初始化值(α₀)下各种模型的性能表现,展示了任务相关的敏感度。*\n\n## 影响和应用\n\n这项研究的发现具有几个重要意义:\n\n1. **架构简化**:通过用DyT替换归一化层,Transformer架构可以得到简化,potentially导致更易解释的模型。\n\n2. **计算效率**:初步测量表明,与归一化层相比,DyT可以提高训练和推理速度,因为它消除了计算统计量的需求。\n\n3. **理论理解**:DyT的成功为深度学习中归一化的基本作用提供了见解,表明关键benefit可能是S形变换而不是统计量的归一化本身。\n\n4. **跨域适用性**:DyT在不同领域(视觉、语言、语音、生物)的一致成功表明它捕捉到了深度学习优化的基本原理。\n\n作者指出的一个限制是,在没有进一步研究的情况下,DyT可能不能直接应用于使用批量归一化的经典CNN架构。他们的工作主要集中在Transformer架构上。\n\n## 结论\n\n论文\"无归一化的Transformer\"对深度学习架构设计做出了重要贡献,证明了Transformer中的归一化层可以被简单的动态Tanh(DyT)操作有效替代。这挑战了归一化层对训练高性能Transformer不可或缺的传统观点。\n\n所提出的DyT方法提供了一个引人注目的替代方案,该方案易于实现,通常只需要最少的调整,并且可以在广泛的任务和领域中达到或超过归一化模型的性能。研究发现DyT中的α学会了近似激活标准差的倒数,这为这种简单机制如何有效模拟归一化的某些方面提供了见解。\n\n这项研究为简化神经网络架构开辟了新途径,并可能激发对传统归一化技术替代方案的进一步探索。随着深度学习的不断发展,这种简化可能有助于构建更高效和更易解释的模型。\n\n## 相关引用\n\nJimmy Lei Ba, Jamie Ryan Kiros, 和 Geoffrey E Hinton. [层归一化](https://alphaxiv.org/abs/1607.06450).arXiv预印本 arXiv:1607.06450, 2016.\n\n * 这篇论文介绍了层归一化(LN),这是稳定深度网络特别是Transformer训练的关键组件。论文分析了LN的行为并提出动态双曲正切(DyT)作为替代方案,使这个引用极其相关。\n\nAlexey Dosovitskiy, Lucas Beyer, Alexander Kolesnikov, Dirk Weissenborn, Xiaohua Zhai, Thomas Unterthiner, Mostafa Dehghani, Matthias Minderer, Georg Heigold, Sylvain Gelly等. 一张图像值16x16个词:大规模图像识别的Transformer.arXiv预印本 arXiv:2010.11929, 2020.\n\n * 这篇论文介绍了视觉Transformer(ViT),这是用于评估DyT在图像分类任务中有效性的重要架构。该论文使用ViT作为核心架构来证明DyT可以替代层归一化。\n\nBiao Zhang 和 Rico Sennrich. [均方根层归一化](https://alphaxiv.org/abs/1910.07467).NeurIPS, 2019.\n\n * 这项工作介绍了RMSNorm,一种层归一化的替代方案,并被用作DyT的基准比较,特别是在大型语言模型实验中。该论文探讨了DyT作为LN和RMSNorm的替代方案。\n\nHugo Touvron, Thibaut Lavril, Gautier Izacard, Xavier Martinet, Marie-Anne Lachaux, Timothée Lacroix, Baptiste Rozière, Naman Goyal, Eric Hambro, Faisal Azhar等. [Llama:开放和高效的基础语言模型](https://alphaxiv.org/abs/2302.13971). arXiv预印本 arXiv:2302.13971, 2023a.\n\n * 这个引用介绍了LLaMA语言模型,它作为测试和评估DyT在大型语言模型环境中的关键架构。该论文使用LLaMA作为验证DyT通用性的重要架构。\n\nAshish Vaswani, Noam Shazeer, Niki Parmar, Jakob Uszkoreit, Llion Jones, Aidan N Gomez, Łukasz Kaiser, 和 Illia Polosukhin. [注意力就是一切](https://alphaxiv.org/abs/1706.03762).NeurIPS, 2017.\n\n * 这篇基础性论文介绍了Transformer架构,这是DyT研究的主要焦点。该论文着重展示了DyT如何改进Transformer。"])</script><script>self.__next_f.push([1,"93:T3e28,"])</script><script>self.__next_f.push([1,"# 정규화 없는 트랜스포머: 동적 쌍곡탄젠트를 활용한 간단한 대안\n\n## 목차\n- [소개](#소개)\n- [정규화 레이어의 이해](#정규화-레이어의-이해)\n- [동적 쌍곡탄젠트 해결책](#동적-쌍곡탄젠트-해결책)\n- [DyT의 작동 방식](#dyt의-작동-방식)\n- [실험적 증거](#실험적-증거)\n- [튜닝과 확장성](#튜닝과-확장성)\n- [알파 매개변수 분석](#알파-매개변수-분석)\n- [다른 접근법과의 비교](#다른-접근법과의-비교)\n- [시사점과 응용](#시사점과-응용)\n- [결론](#결론)\n\n## 소개\n\n정규화 레이어는 현대 신경망, 특히 자연어 처리, 컴퓨터 비전 및 기타 영역을 지배하는 트랜스포머 아키텍처에서 필수적인 구성 요소로 여겨져 왔습니다. 레이어 정규화(LN)와 그 변형들은 트랜스포머에서 보편적으로 사용되며, 학습 안정화와 성능 향상에 중요한 것으로 알려져 있습니다. 하지만 Meta AI, NYU, MIT, Princeton University의 연구진이 발표한 새로운 논문은 전통적인 정규화 레이어 없이도 트랜스포머가 동등하거나 더 나은 성능을 달성할 수 있음을 보여주며 이러한 기본 가정에 도전합니다.\n\n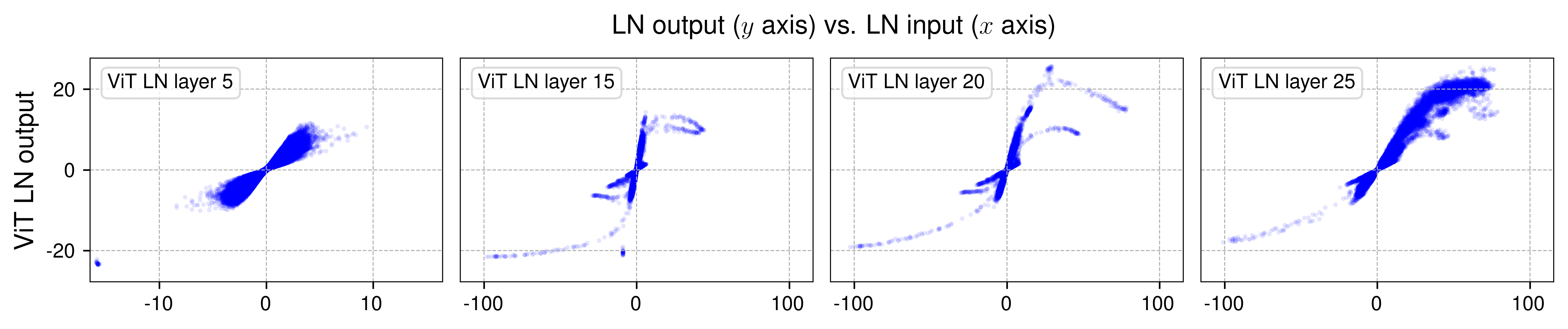\n*그림 1: 다양한 ViT 레이어에서 레이어 정규화의 입출력 동작을 시각화한 것으로, S자형, tanh와 유사한 관계를 보여줍니다.*\n\n## 정규화 레이어의 이해\n\n배치 정규화, 레이어 정규화, RMSNorm과 같은 정규화 기법들은 딥러닝에서 표준 관행이 되었습니다. 이러한 방법들은 일반적으로 지정된 차원에서 통계(평균 및/또는 표준편차)를 계산하여 활성화를 정규화하며, 네트워크 활성화의 분포를 제어함으로써 학습을 안정화하는 데 도움을 줍니다.\n\n특히 트랜스포머에서 레이어 정규화는 각 토큰이나 위치에 대해 특징 차원에서 평균과 표준편차를 계산하여 작동합니다. 이 정규화 과정은 학습과 추론 과정에서 각 레이어마다 이러한 통계를 계산해야 하므로 계산 비용이 많이 듭니다.\n\n저자들은 그림 1에서 보이듯이 레이어 정규화가 종종 tanh와 유사한 S자형 입출력 매핑을 생성한다는 것을 관찰했습니다. 이러한 관찰은 그들의 핵심 통찰로 이어졌습니다: 아마도 정규화의 유익한 효과는 활성화 통계를 계산하지 않고도 이러한 S자형 동작을 모방하는 더 간단한 메커니즘을 통해 달성할 수 있을 것입니다.\n\n## 동적 쌍곡탄젠트 해결책\n\n연구진은 정규화 레이어를 대체할 간단한 방법으로 동적 쌍곡탄젠트(DyT)를 제안합니다. DyT는 다음과 같이 정의됩니다:\n\n```\nDyT(x) = tanh(αx)\n```\n\n여기서 α는 tanh 함수의 기울기를 제어하는 학습 가능한 매개변수입니다. 이 간단한 공식은 활성화 통계를 계산할 필요가 없으면서도 트랜스포머 성능에 중요해 보이는 S자형 변환을 보존합니다.\n\n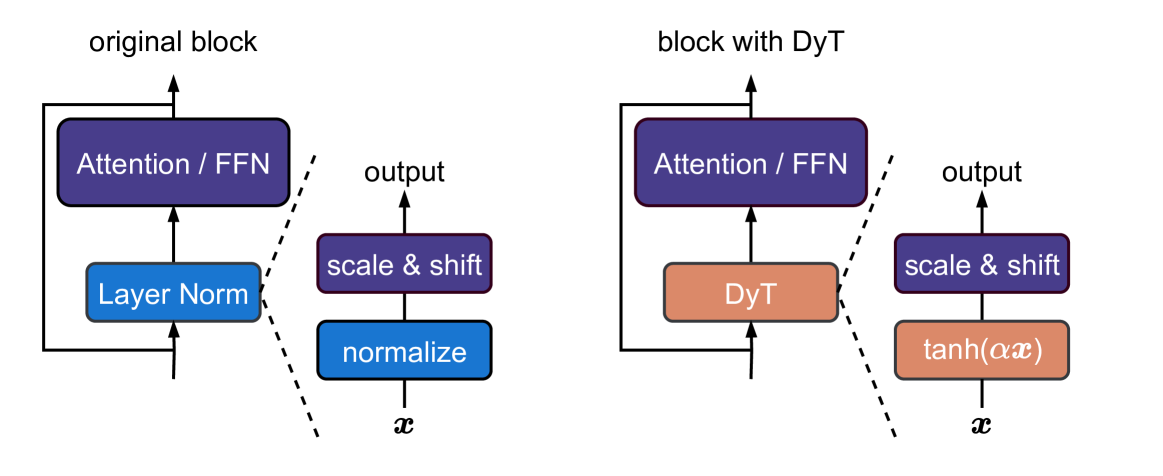\n*그림 2: 왼쪽: 레이어 정규화가 있는 기존 트랜스포머 블록. 오른쪽: 동적 쌍곡탄젠트(DyT)로 대체된 제안된 블록.*\n\n이 접근법의 장점은 복잡한 정규화 연산을 학습 가능한 매개변수가 있는 단일 원소별 연산으로 대체하는 단순성에 있습니다. 그림 2는 레이어 정규화가 있는 전통적인 트랜스포머 블록과 DyT가 있는 제안된 블록을 비교하여 보여줍니다.\n\n## DyT의 작동 방식\n\n동적 쌍곡탄젠트는 두 가지 주요 메커니즘을 통해 작동합니다:\n\n1. **값 압축**: tanh 함수는 극단적인 값들을 압축하여, 정규화 레이어와 유사한 암묵적 정규화를 제공합니다. 이는 순전파와 역전파 과정에서 활성화가 너무 커지는 것을 방지합니다.\n\n2. **적응형 스케일링**: 학습 가능한 매개변수 α는 tanh 함수의 기울기를 조절하여 네트워크가 값을 얼마나 적극적으로 압축할지 제어할 수 있게 합니다. 이러한 적응성은 성능에 매우 중요합니다.\n\n쌍곡선 탄젠트 함수(tanh)는 -1과 1 사이로 제한되어 있어 모든 입력값을 이 범위로 압축합니다. 이 압축의 기울기는 α에 의해 제어됩니다:\n\n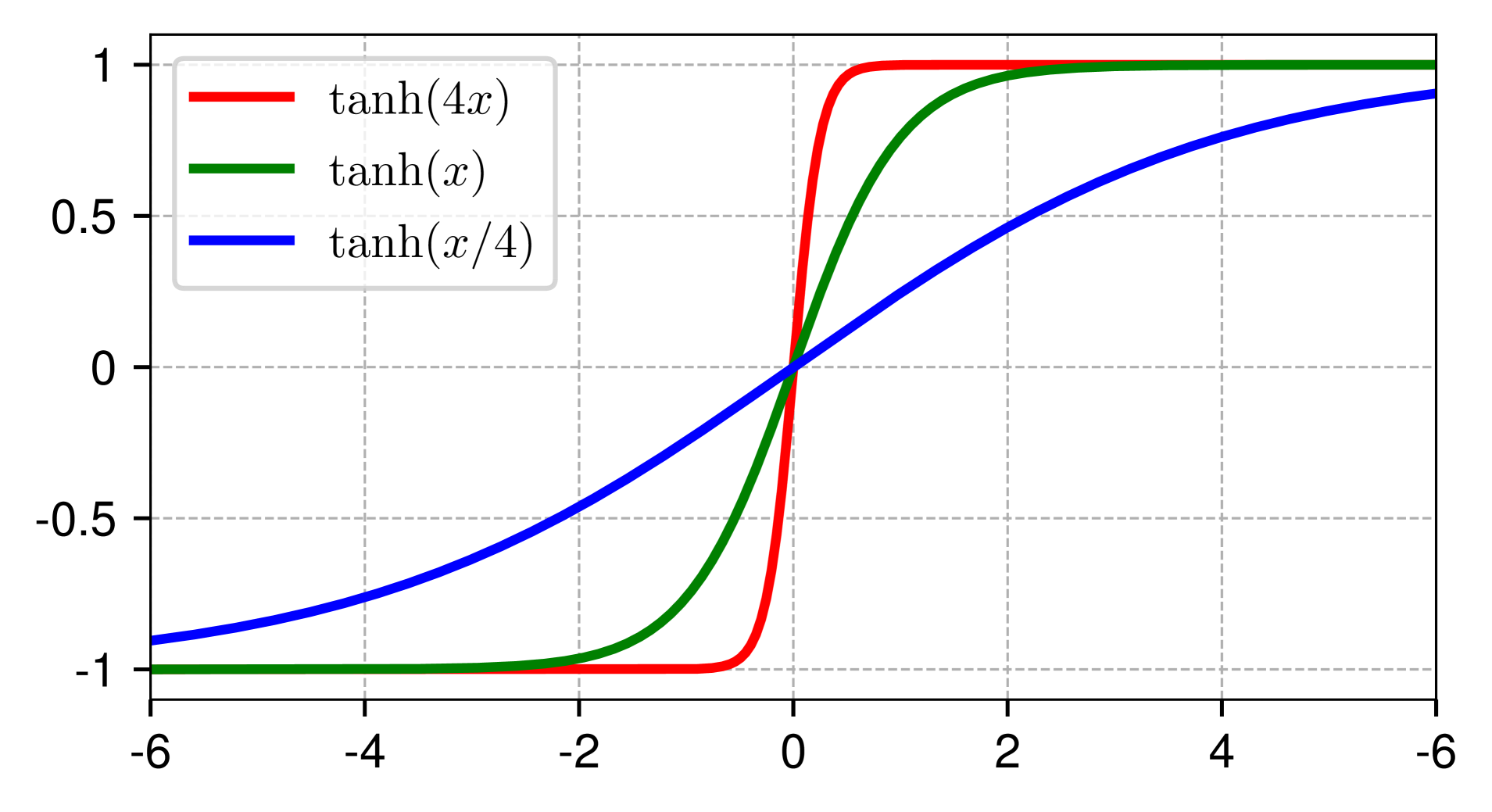\n*그림 3: 다양한 α 값을 가진 tanh 함수로, 더 큰 α 값이 어떻게 더 급격한 전이를 만드는지 보여줍니다.*\n\n그림 3에서 보듯이, 더 큰 α 값은 -1에서 1로의 전이를 더 급격하게 만들고, 더 작은 α는 더 점진적으로 만듭니다. 이러한 유연성을 통해 네트워크는 작업과 층의 깊이에 따라 값 압축의 정도를 조절할 수 있습니다.\n\n## 실험적 증거\n\n연구진은 정규화 층의 대체제로서 DyT의 효과를 검증하기 위해 다양한 작업과 도메인에 걸쳐 광범위한 실험을 수행했습니다. 이러한 실험들은 다음을 포함합니다:\n\n1. **비전 작업**:\n - Vision Transformers(ViT)와 ConvNeXt를 사용한 ImageNet 분류\n - MAE와 DINO를 사용한 자기지도학습\n\n2. **생성 모델**:\n - 이미지 생성을 위한 확산 모델(DiT)\n\n3. **대규모 언어 모델**:\n - 7B에서 70B 매개변수 규모의 LLaMA 사전학습\n\n4. **기타 도메인**:\n - wav2vec 2.0을 사용한 음성 처리\n - HyenaDNA와 Caduceus를 사용한 DNA 시퀀스 모델링\n\n결과는 일관되게 DyT를 사용한 트랜스포머가 정규화된 버전과 동등하거나 더 나은 성능을 보여주었습니다. 예를 들어, ImageNet 분류에서 Vision Transformers의 경우, DyT 변형이 LN 버전과 비슷한 정확도를 달성했습니다:\n\n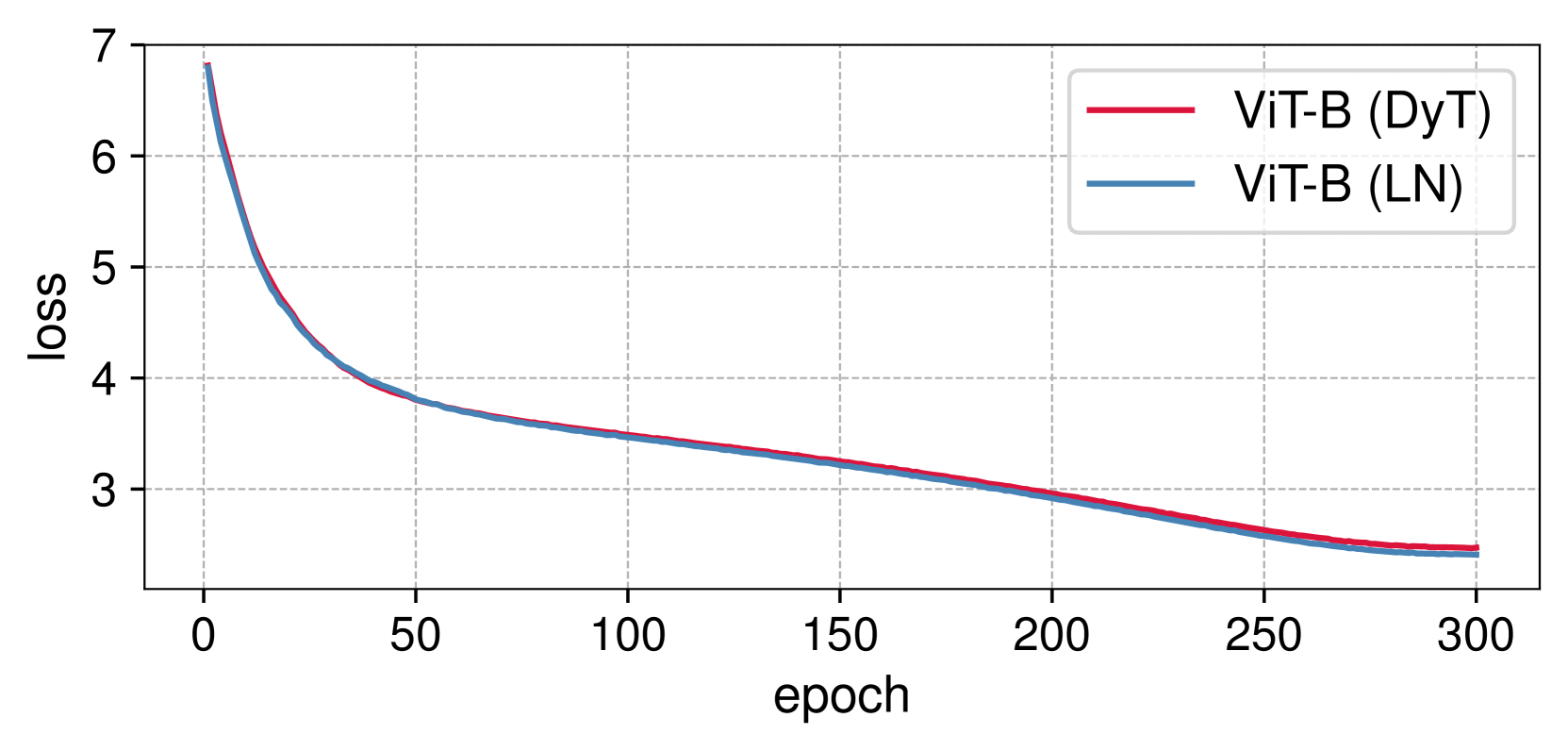\n*그림 4: Layer Normalization(LN)과 Dynamic Tanh(DyT)를 사용한 ViT-B의 학습 손실 곡선으로, 거의 동일한 수렴을 보여줍니다.*\n\n마찬가지로, 다양한 크기(7B에서 70B 매개변수)의 LLaMA 모델에서, DyT 변형은 RMSNorm 모델과 비교하여 비슷하거나 약간 더 나은 손실 값을 달성했습니다:\n\n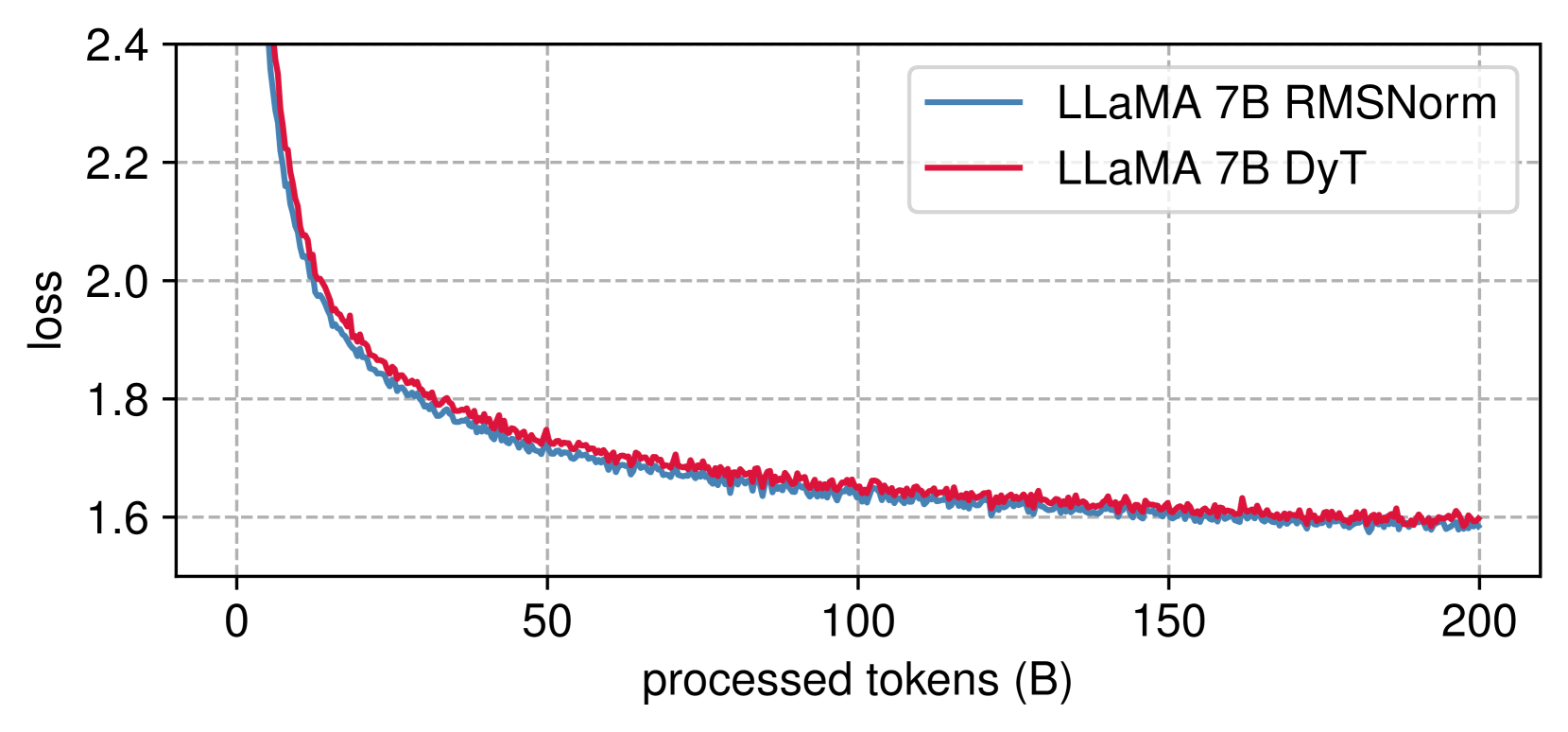\n*그림 5: RMSNorm과 DyT를 사용한 LLaMA 7B의 학습 손실 곡선으로, 비슷한 성능을 보여줍니다.*\n\n## 튜닝과 확장성\n\nDyT는 일반적으로 견고하고 최소한의 튜닝으로도 잘 작동하지만, 연구진은 더 큰 모델, 특히 대규모 언어 모델(LLM)의 경우 α의 신중한 초기화가 중요하다는 것을 발견했습니다. 그들은 LLaMA 아키텍처에 대한 초기화 값을 철저히 탐색했습니다:\n\n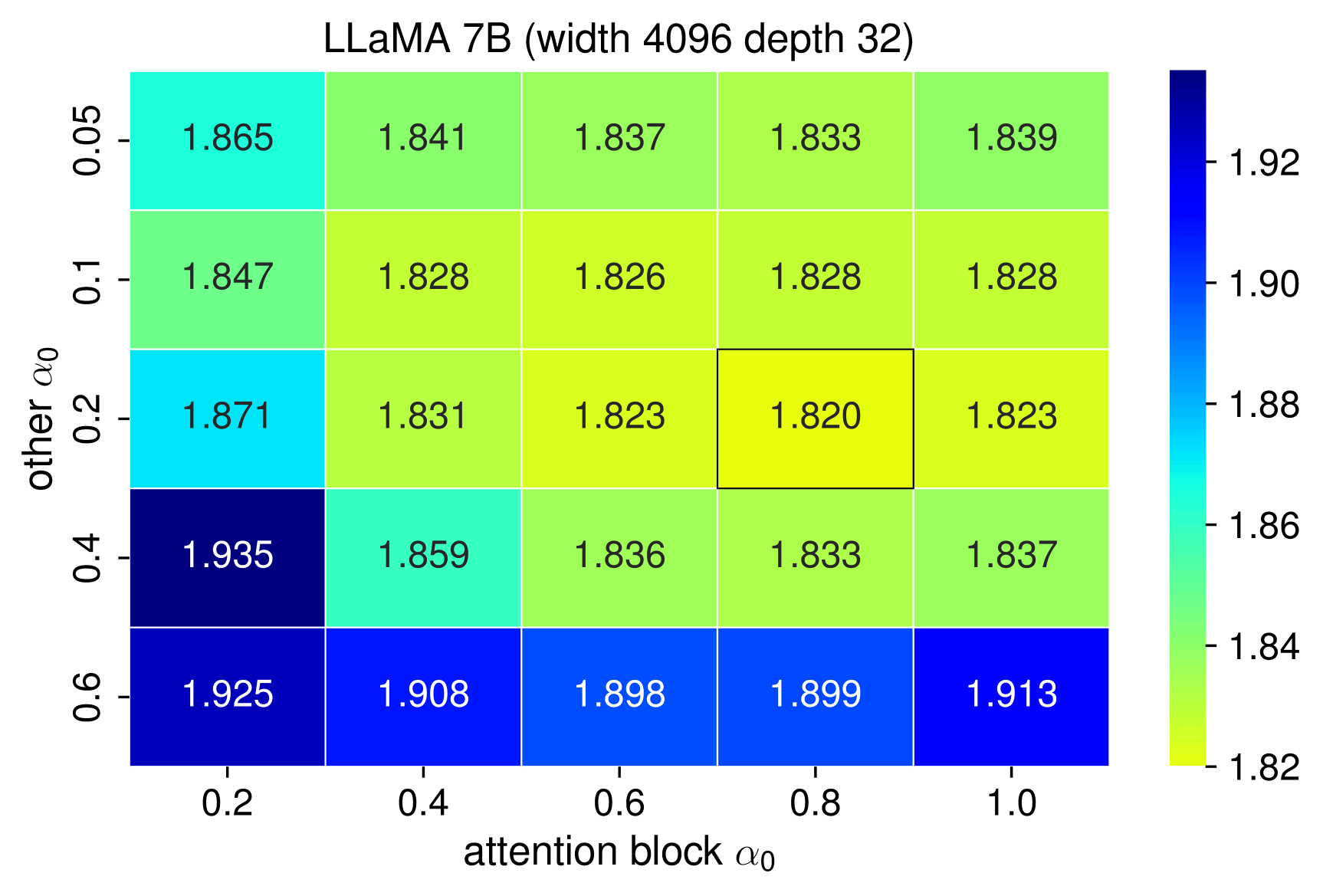\n*그림 6: 주의력과 피드포워드 블록에 대한 다양한 α 초기화 값에 따른 LLaMA 7B 성능을 보여주는 히트맵.*\n\nLLaMA 7B의 경우, 최적의 α 초기화는 주의력 블록에서 0.2, 다른 블록에서 0.2로 나타났으며, LLaMA 13B의 경우에는 주의력 블록에서 0.6, 다른 블록에서 0.15로 나타났습니다. 이는 더 큰 모델이 α 매개변수의 더 신중한 튜닝을 필요로 할 수 있다는 것을 시사합니다.\n\n연구진은 또한 다양한 깊이와 너비의 모델을 학습시켜 그들의 접근 방식의 확장성을 테스트했습니다:\n\n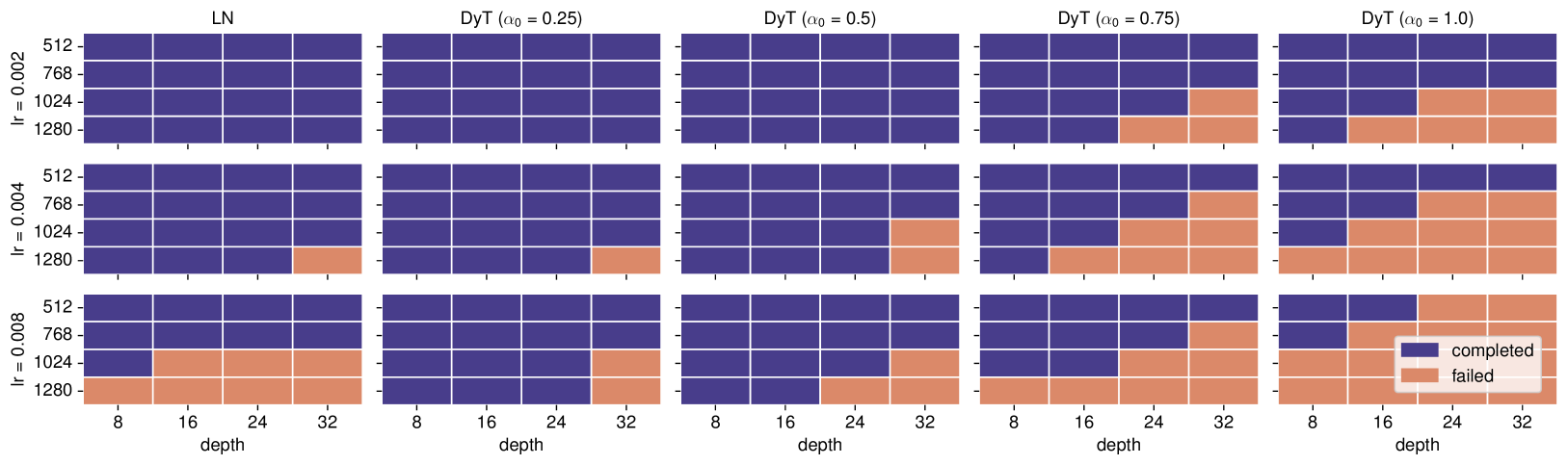\n*그림 7: 다양한 모델 깊이와 너비에 걸친 LN과 DyT 간의 학습 안정성 비교, 파란색은 성공적인 학습을, 주황색은 불안정성을 나타냅니다.*\n\n결과는 DyT 모델이 LN 모델과 비슷하게 확장될 수 있음을 보여주었지만, 더 큰 규모에서는 학습률에 대한 추가적인 민감도가 있는 것으로 나타났습니다.\n\n## 알파 매개변수 분석\n\n연구진은 DyT의 α 매개변수가 활성화의 통계적 특성과 어떻게 관련되는지 분석했습니다. 흥미롭게도, α가 층 활성화의 표준편차의 역수를 근사하는 것을 학습한다는 것을 발견했습니다:\n\n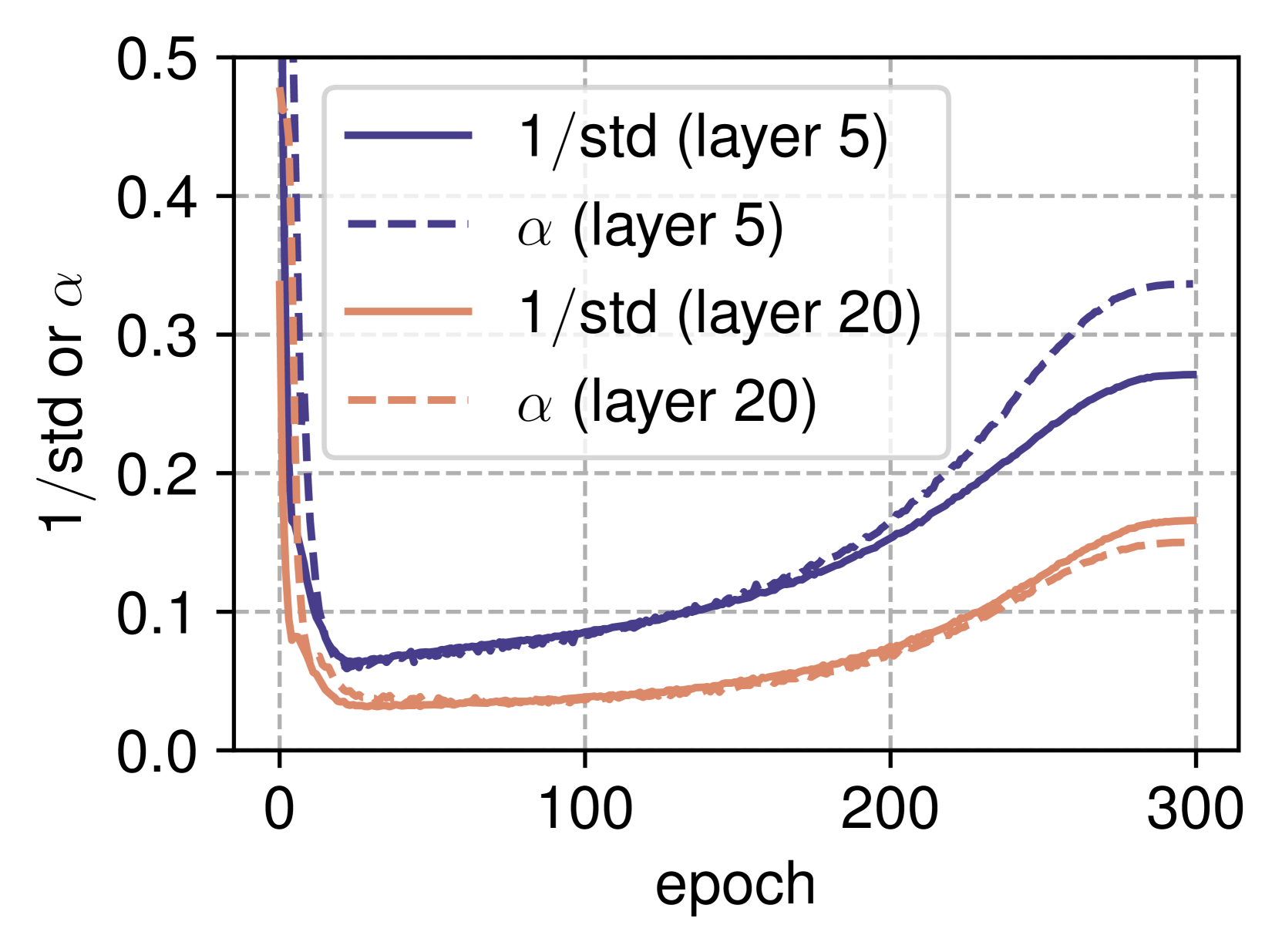\n*그림 8: 학습 에포크에 걸쳐 학습된 α 값과 활성화 표준편차의 역수(1/std)를 비교한 것으로, α가 정규화 동작을 부분적으로 모방하는 방식을 보여줍니다.*\n\n이러한 발견은 DyT가 통계를 명시적으로 계산하지 않고도 정규화 레이어와 유사한 적응형 스케일링을 암묵적으로 학습한다는 것을 시사합니다. α 매개변수는 활성화의 표준편차에 반비례하는 경향이 있어, 입력을 tanh 함수에 적합한 크기로 효과적으로 스케일링합니다.\n\n또한, 연구진은 서로 다른 레이어와 모델에서 학습된 α 값과 활성화의 역표준편차 사이에 일관된 상관관계를 관찰했습니다:\n\n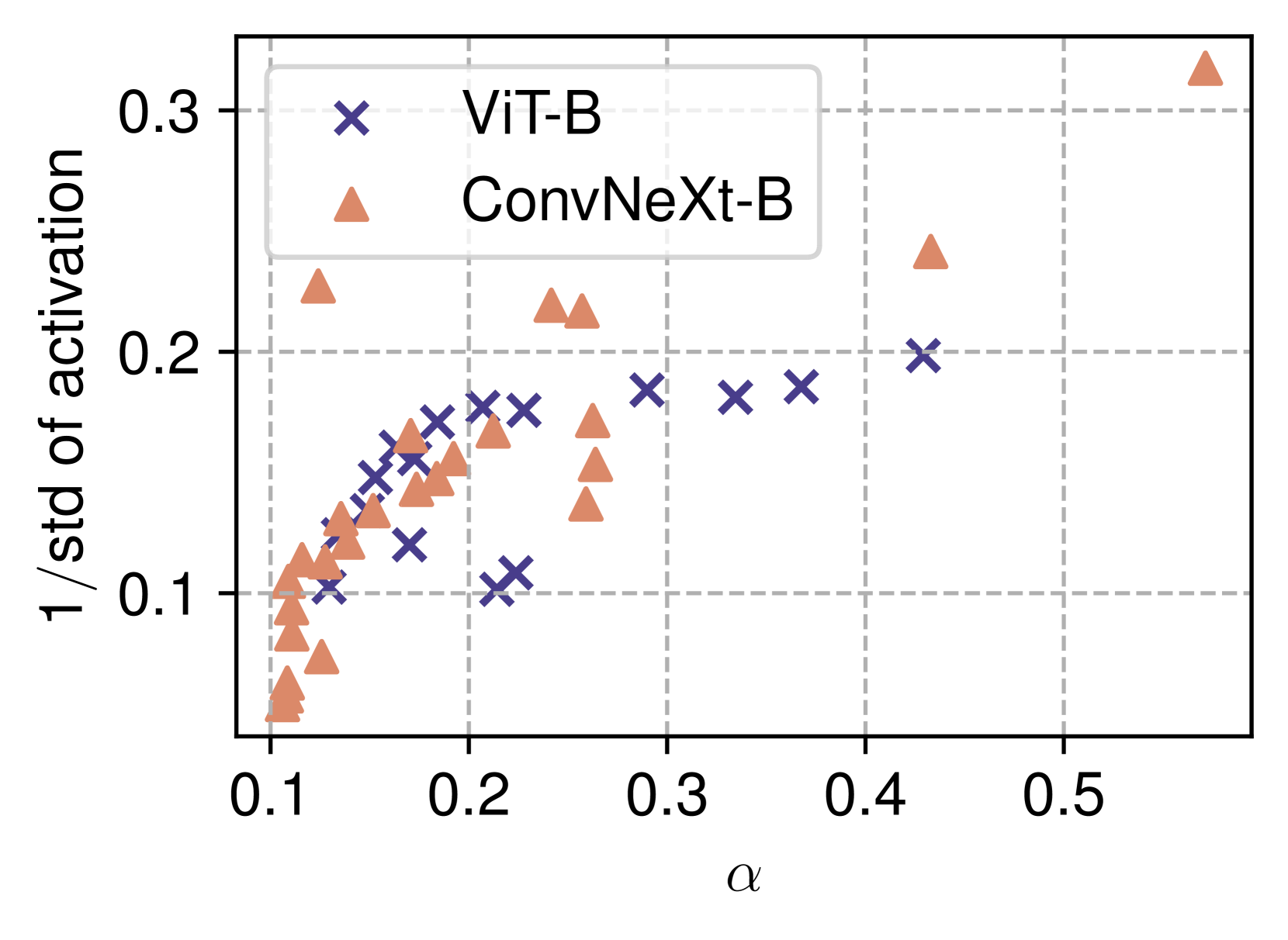\n*그림 9: ViT-B와 ConvNeXt-B 모델의 다양한 레이어에서 학습된 α 값과 활성화의 역표준편차 간의 관계를 보여주는 산점도.*\n\n## 다른 접근 방식과의 비교\n\n연구진은 DyT를 Fixup, SkipInit, σReparam을 포함한 정규화 없이 심층 네트워크를 훈련하기 위해 제안된 다른 방법들과 비교했습니다. 다양한 작업과 모델 아키텍처에서 DyT는 이러한 대안들을 일관되게 능가했습니다.\n\n또한 tanh 함수와 학습 가능한 스케일 매개변수 α의 중요성을 검증하기 위한 절제 연구도 수행했습니다. 이 연구들은 다음을 보여줍니다:\n\n1. tanh를 sigmoid나 hardtanh와 같은 다른 함수로 대체하면 성능이 저하되어 tanh의 특정 속성의 중요성을 강조했습니다.\n\n2. 학습 가능한 α 대신 고정된 α를 사용하면 성능이 크게 저하되어 적응성의 중요성을 입증했습니다.\n\n3. 비선형성을 완전히 제거하면(학습 가능한 스케일만 사용) 훈련이 불안정해져, tanh의 유계성이 중요하다는 것을 보여줍니다.\n\n다양한 작업에서 초기 α 값이 모델 성능에 미치는 영향도 연구되었습니다:\n\n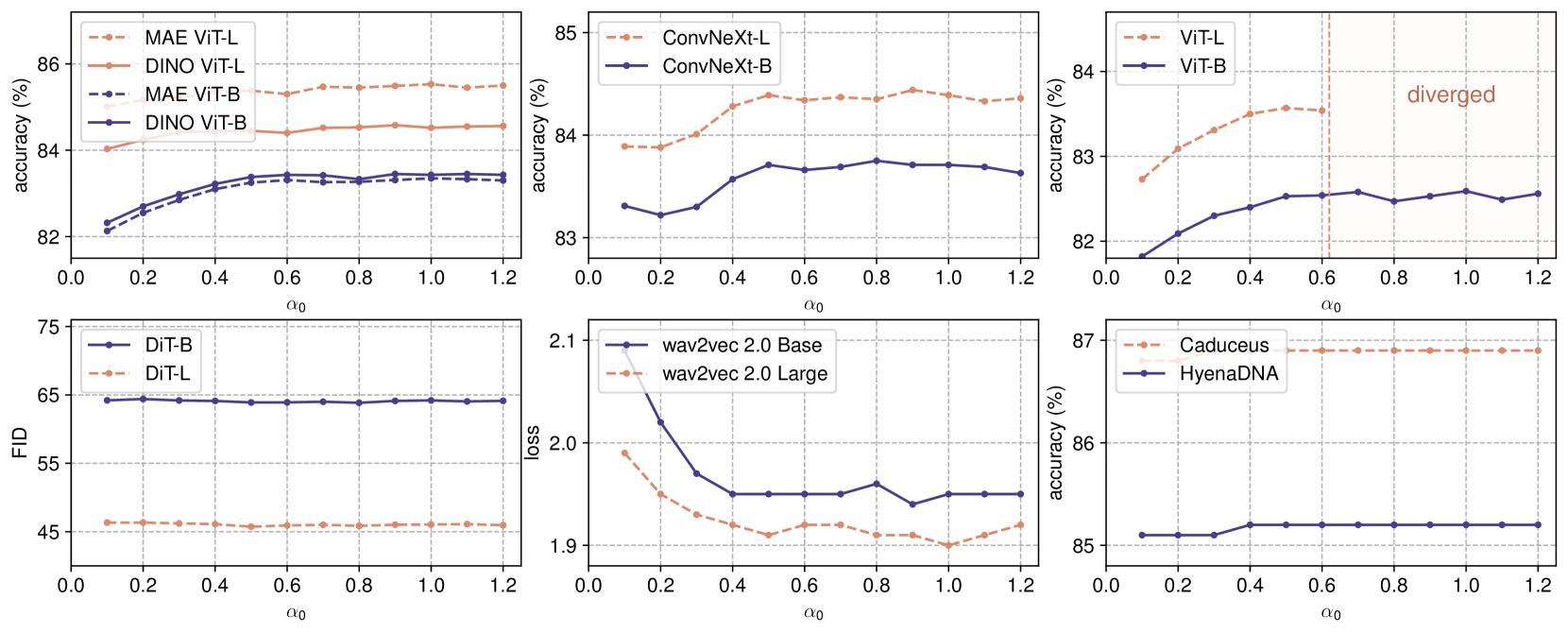\n*그림 10: 다양한 α 초기화 값(α₀)에 따른 여러 모델의 성능을 보여주며, 작업별 민감도를 나타냅니다.*\n\n## 의의와 응용\n\n이 연구의 발견은 여러 가지 중요한 의의를 가집니다:\n\n1. **아키텍처 단순화**: 정규화 레이어를 DyT로 대체함으로써 트랜스포머 아키텍처를 단순화할 수 있어, 더 해석하기 쉬운 모델로 이어질 수 있습니다.\n\n2. **계산 효율성**: 예비 측정 결과에 따르면 DyT는 통계 계산이 필요 없어 정규화 레이어에 비해 훈련 및 추론 속도를 향상시킬 수 있습니다.\n\n3. **이론적 이해**: DyT의 성공은 딥러닝에서 정규화의 근본적인 역할에 대한 통찰을 제공하며, 핵심 이점이 통계의 정규화보다는 S자 형태의 변환일 수 있음을 시사합니다.\n\n4. **다중 도메인 적용 가능성**: 다양한 도메인(시각, 언어, 음성, 생물학)에서 DyT의 일관된 성공은 딥러닝 최적화의 근본적인 원리를 포착했음을 시사합니다.\n\n저자들이 언급한 한 가지 한계점은 DyT가 추가 연구 없이는 배치 정규화를 사용하는 기존의 CNN 아키텍처에 직접 적용하기 어려울 수 있다는 것입니다. 그들의 연구는 주로 트랜스포머 아키텍처에 초점을 맞추었습니다.\n\n## 결론\n\n\"정규화 없는 트랜스포머\" 논문은 트랜스포머의 정규화 레이어를 간단한 동적 tanh(DyT) 연산으로 효과적으로 대체할 수 있음을 보여줌으로써 딥러닝 아키텍처 설계에 중요한 기여를 했습니다. 이는 정규화 레이어가 고성능 트랜스포머 훈련에 필수불가결하다는 기존의 통념에 도전합니다.\n\n제안된 DyT 접근 방식은 구현이 쉽고, 대개 최소한의 튜닝만 필요하며, 다양한 작업과 도메인에서 정규화된 모델의 성능과 대등하거나 이를 능가할 수 있는 매력적인 대안을 제시합니다. DyT의 α가 활성화 표준 편차의 역수를 근사하게 학습한다는 발견은 이 단순한 메커니즘이 어떻게 정규화의 특정 측면을 효과적으로 모방하는지에 대한 통찰을 제공합니다.\n\n이 연구는 신경망 아키텍처를 단순화하는 새로운 길을 열어주며, 전통적인 정규화 기법의 대안을 더욱 탐구하도록 영감을 줄 수 있습니다. 딥 러닝이 계속 발전함에 따라, 이러한 단순화는 더 효율적이고 해석 가능한 모델 개발에 기여할 수 있습니다.\n\n## 관련 인용문헌\n\nJimmy Lei Ba, Jamie Ryan Kiros, Geoffrey E Hinton. [계층 정규화](https://alphaxiv.org/abs/1607.06450). arXiv 사전인쇄 arXiv:1607.06450, 2016.\n\n * 이 논문은 특히 트랜스포머에서 학습 안정화를 위한 중요한 구성 요소인 계층 정규화(LN)를 소개합니다. 논문은 LN의 동작을 분석하고 대체제로 동적 탄젠트(DyT)를 제안하므로, 이 인용은 매우 관련성이 높습니다.\n\nAlexey Dosovitskiy, Lucas Beyer, Alexander Kolesnikov, Dirk Weissenborn, Xiaohua Zhai, Thomas Unterthiner, Mostafa Dehghani, Matthias Minderer, Georg Heigold, Sylvain Gelly, 외. 이미지는 16x16 단어의 가치가 있다: 대규모 이미지 인식을 위한 트랜스포머. arXiv 사전인쇄 arXiv:2010.11929, 2020.\n\n * 이 논문은 이미지 분류 작업에서 DyT의 효과를 벤치마킹하는 데 사용되는 주요 아키텍처인 비전 트랜스포머(ViT)를 소개합니다. 논문은 DyT가 계층 정규화를 대체할 수 있음을 보여주기 위해 ViT를 핵심 아키텍처로 사용합니다.\n\nBiao Zhang, Rico Sennrich. [제곱근 평균 계층 정규화](https://alphaxiv.org/abs/1910.07467). NeurIPS, 2019.\n\n * 이 연구는 계층 정규화의 대안인 RMSNorm을 소개하며, 특히 대규모 언어 모델 실험에서 DyT의 기준 비교로 사용됩니다. 논문은 DyT를 LN과 RMSNorm 모두의 대체제로 탐구합니다.\n\nHugo Touvron, Thibaut Lavril, Gautier Izacard, Xavier Martinet, Marie-Anne Lachaux, Timothée Lacroix, Baptiste Rozière, Naman Goyal, Eric Hambro, Faisal Azhar, 외. [Llama: 개방형 및 효율적인 기초 언어 모델](https://alphaxiv.org/abs/2302.13971). arXiv 사전인쇄 arXiv:2302.13971, 2023a.\n\n * 이 인용문은 대규모 언어 모델 맥락에서 DyT를 테스트하고 평가하기 위한 핵심 아키텍처로 사용되는 LLaMA 언어 모델을 소개합니다. 논문은 DyT의 일반화 가능성을 검증하기 위한 중요한 아키텍처로 LLaMA를 사용합니다.\n\nAshish Vaswani, Noam Shazeer, Niki Parmar, Jakob Uszkoreit, Llion Jones, Aidan N Gomez, Łukasz Kaiser, Illia Polosukhin. [주의력이 전부다](https://alphaxiv.org/abs/1706.03762). NeurIPS, 2017.\n\n * 이 기초적인 논문은 DyT 연구의 주요 초점인 트랜스포머 아키텍처를 소개합니다. 논문은 DyT가 어떻게 트랜스포머를 개선할 수 있는지 보여주는 데 중점을 둡니다."])</script><script>self.__next_f.push([1,"94:T40de,"])</script><script>self.__next_f.push([1,"# Transformers sin Normalización: Una Alternativa Simple con Tangente Hiperbólica Dinámica\n\n## Tabla de Contenidos\n- [Introducción](#introducción)\n- [Entendiendo las Capas de Normalización](#entendiendo-las-capas-de-normalización)\n- [La Solución de Tangente Hiperbólica Dinámica](#la-solución-de-tangente-hiperbólica-dinámica)\n- [Cómo Funciona DyT](#cómo-funciona-dyt)\n- [Evidencia Experimental](#evidencia-experimental)\n- [Ajuste y Escalabilidad](#ajuste-y-escalabilidad)\n- [Análisis del Parámetro Alfa](#análisis-del-parámetro-alfa)\n- [Comparación con Otros Enfoques](#comparación-con-otros-enfoques)\n- [Implicaciones y Aplicaciones](#implicaciones-y-aplicaciones)\n- [Conclusión](#conclusión)\n\n## Introducción\n\nLas capas de normalización han sido consideradas componentes esenciales en las redes neuronales modernas, particularmente en arquitecturas Transformer que dominan el procesamiento del lenguaje natural, visión por computadora y otros dominios. La Normalización de Capa (LN) y sus variantes son ubicuas en los Transformers, consideradas cruciales para estabilizar el entrenamiento y mejorar el rendimiento. Sin embargo, un nuevo artículo de investigadores de Meta AI, NYU, MIT y la Universidad de Princeton desafía esta suposición fundamental al demostrar que los Transformers pueden lograr un rendimiento equivalente o mejor sin las capas de normalización tradicionales.\n\n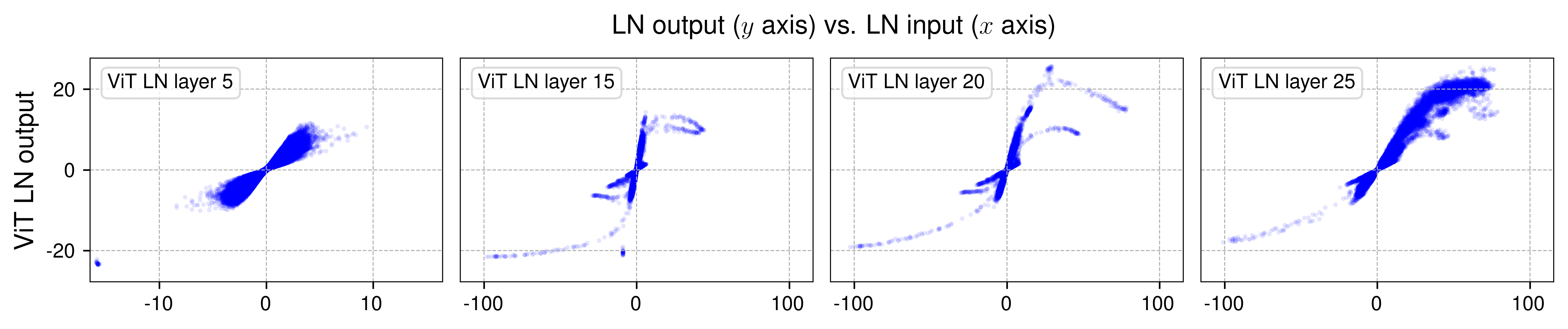\n*Figura 1: Visualización del comportamiento entrada-salida de la Normalización de Capa en varias capas ViT, mostrando relaciones en forma de S, similares a tanh.*\n\n## Entendiendo las Capas de Normalización\n\nTécnicas de normalización como la Normalización por Lotes, Normalización de Capa y RMSNorm se han convertido en práctica estándar en el aprendizaje profundo. Estos métodos típicamente normalizan las activaciones calculando estadísticas (media y/o desviación estándar) a través de dimensiones específicas, ayudando a estabilizar el entrenamiento al controlar la distribución de las activaciones de la red.\n\nEn los Transformers específicamente, la Normalización de Capa opera calculando la media y la desviación estándar a través de la dimensión de características para cada token o posición. Este proceso de normalización es computacionalmente costoso ya que requiere calcular estas estadísticas en cada capa durante el entrenamiento y la inferencia.\n\nLos autores observaron que la Normalización de Capa a menudo produce mapeos entrada-salida similares a tanh, en forma de S, como se muestra en la Figura 1. Esta observación llevó a su idea clave: quizás el efecto beneficioso de la normalización podría lograrse a través de un mecanismo más simple que imite este comportamiento en forma de S sin calcular estadísticas de activación.\n\n## La Solución de Tangente Hiperbólica Dinámica\n\nLos investigadores proponen la Tangente Hiperbólica Dinámica (DyT) como un reemplazo directo para las capas de normalización. DyT se define como:\n\n```\nDyT(x) = tanh(αx)\n```\n\nDonde α es un parámetro aprendible que controla la pendiente de la función tanh. Esta formulación simple elimina la necesidad de calcular estadísticas de activación mientras preserva la transformación en forma de S que parece ser importante para el rendimiento del Transformer.\n\n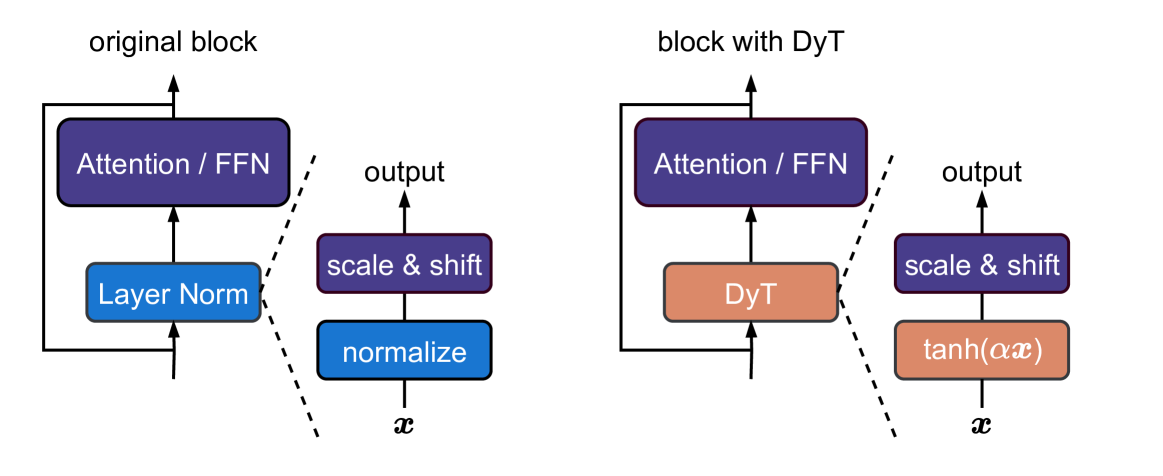\n*Figura 2: Izquierda: Bloque Transformer original con Normalización de Capa. Derecha: Bloque propuesto con reemplazo de Tangente Hiperbólica Dinámica (DyT).*\n\nLa belleza de este enfoque radica en su simplicidad - reemplazando operaciones complejas de normalización con una única operación elemento a elemento que tiene un parámetro aprendible. La Figura 2 muestra cómo el bloque Transformer tradicional con Normalización de Capa se compara con el bloque propuesto con DyT.\n\n## Cómo Funciona DyT\n\nLa Tangente Hiperbólica Dinámica funciona a través de dos mecanismos clave:\n\n1. **Compresión de Valores**: La función tanh comprime valores extremos, proporcionando una forma de regularización implícita similar a las capas de normalización. Esto evita que las activaciones crezcan demasiado durante los pases hacia adelante y hacia atrás.\n\n2. **Escalado Adaptativo**: El parámetro α aprendible ajusta la pendiente de la función tanh, permitiendo que la red controle qué tan agresivamente se comprimen los valores. Esta adaptabilidad es crucial para el rendimiento.\n\nLa función tangente hiperbólica (tanh) está limitada entre -1 y 1, comprimiendo cualquier valor de entrada en este rango. La pendiente de esta compresión está controlada por α:\n\n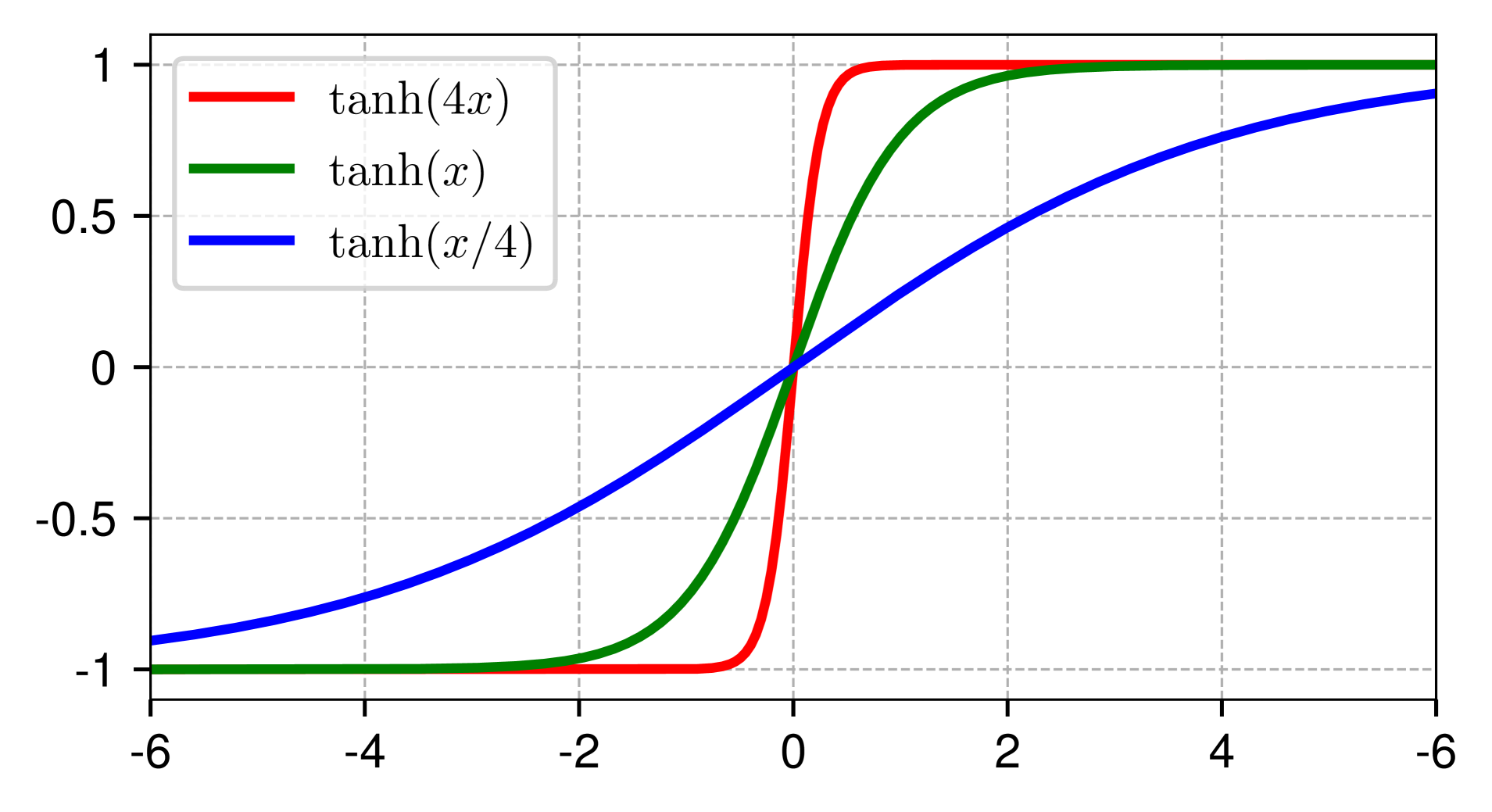\n*Figura 3: La función tanh con diferentes valores de α, mostrando cómo valores más grandes de α crean transiciones más pronunciadas.*\n\nComo se muestra en la Figura 3, un valor α más grande hace que la transición de -1 a 1 sea más pronunciada, mientras que un α más pequeño la hace más gradual. Esta flexibilidad permite que la red ajuste el grado de compresión de valores según la tarea y la profundidad de la capa.\n\n## Evidencia Experimental\n\nLos investigadores realizaron extensos experimentos en diversas tareas y dominios para validar la efectividad de DyT como reemplazo de las capas de normalización. Estos experimentos incluyeron:\n\n1. **Tareas de Visión**:\n - Clasificación ImageNet con Vision Transformers (ViT) y ConvNeXt\n - Aprendizaje auto-supervisado con MAE y DINO\n\n2. **Modelos Generativos**:\n - Modelos de difusión para generación de imágenes (DiT)\n\n3. **Modelos de Lenguaje Grandes**:\n - Preentrenamiento de LLaMA en escalas de 7B a 70B parámetros\n\n4. **Otros Dominios**:\n - Procesamiento de voz con wav2vec 2.0\n - Modelado de secuencias de ADN con HyenaDNA y Caduceus\n\nLos resultados mostraron consistentemente que los Transformers con DyT podían igualar o superar el rendimiento de sus contrapartes normalizadas. Por ejemplo, con Vision Transformers en clasificación ImageNet, la variante DyT logró una precisión comparable a la versión LN:\n\n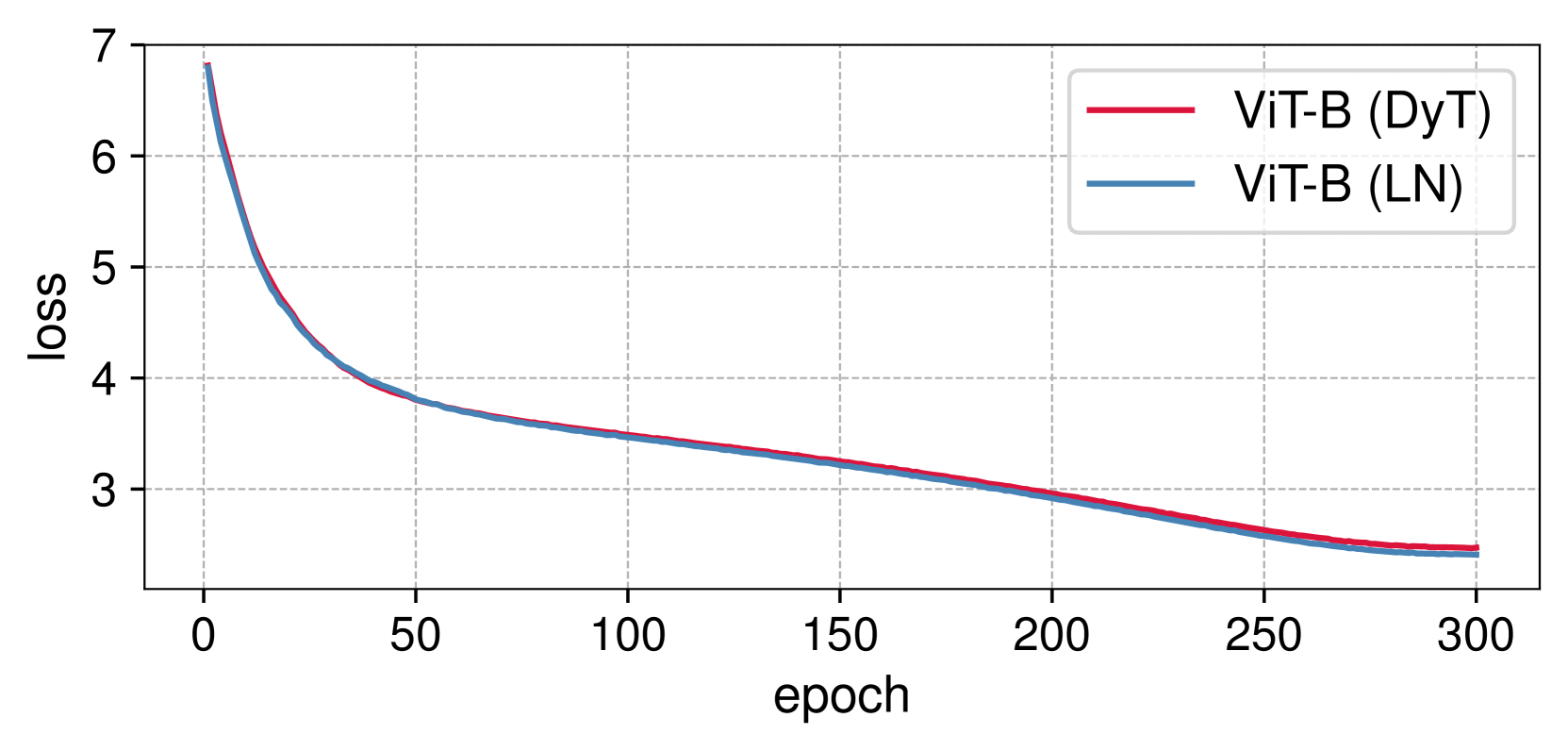\n*Figura 4: Curvas de pérdida de entrenamiento para ViT-B con Layer Normalization (LN) y Dynamic Tanh (DyT), mostrando una convergencia casi idéntica.*\n\nDe manera similar, para modelos LLaMA de varios tamaños (7B a 70B parámetros), las variantes DyT lograron valores de pérdida comparables o ligeramente mejores que los modelos RMSNorm:\n\n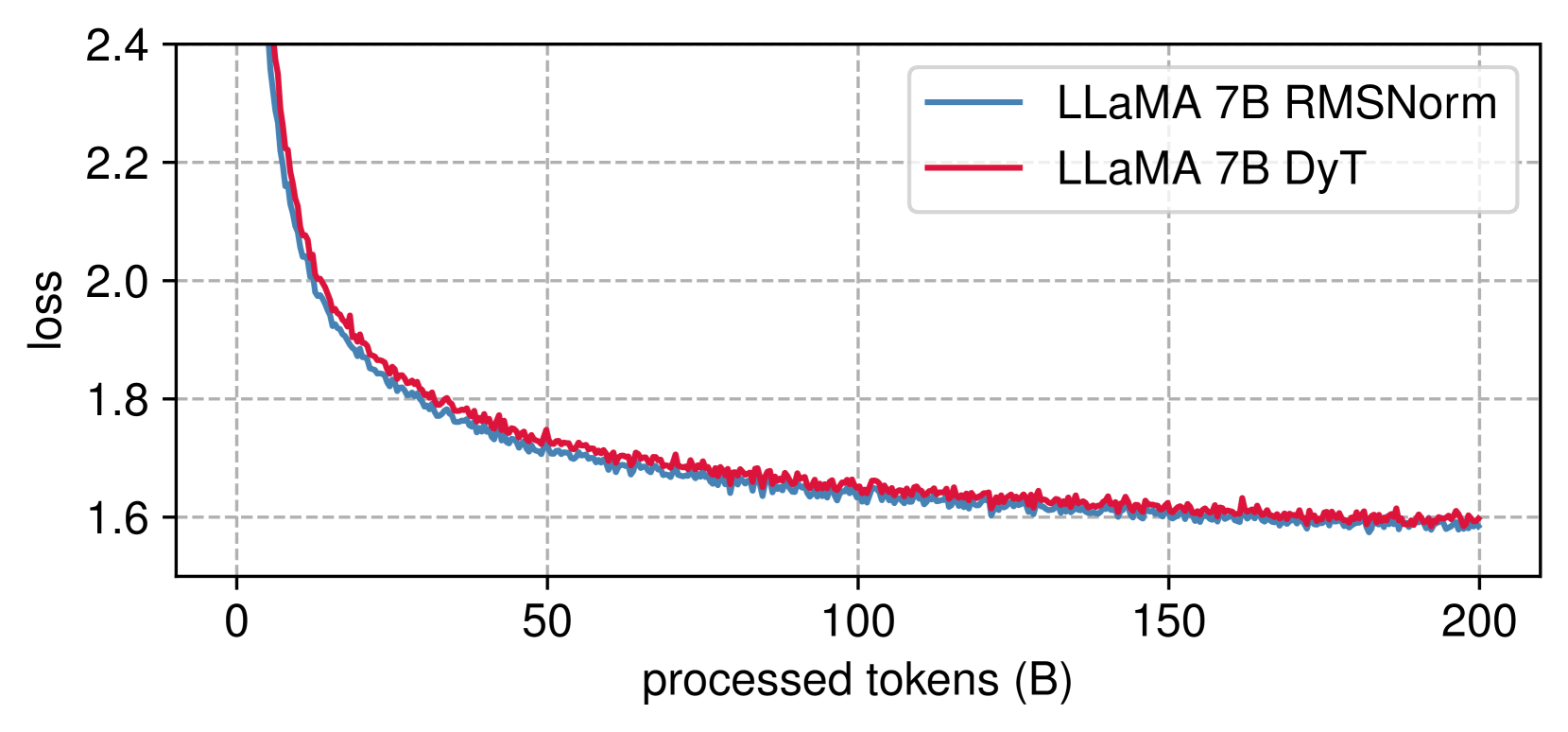\n*Figura 5: Curvas de pérdida de entrenamiento para LLaMA 7B con RMSNorm y DyT, mostrando rendimiento comparable.*\n\n## Ajuste y Escalabilidad\n\nSi bien DyT es generalmente robusto y funciona bien con un ajuste mínimo, los investigadores encontraron que para modelos más grandes, particularmente los Modelos de Lenguaje Grandes (LLMs), la inicialización cuidadosa de α es importante. Realizaron una exploración exhaustiva de valores de inicialización para la arquitectura LLaMA:\n\n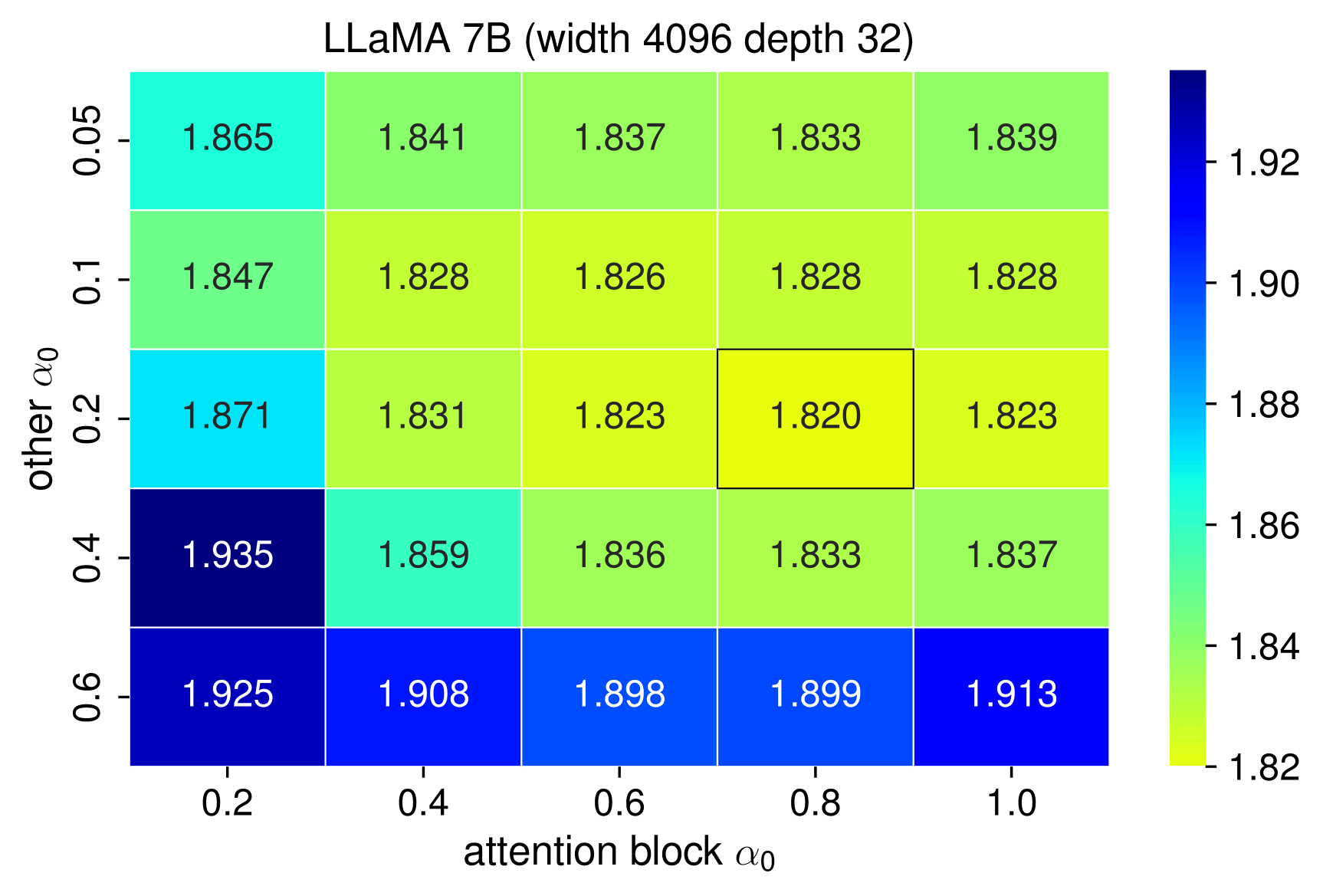\n*Figura 6: Mapa de calor que muestra el rendimiento de LLaMA 7B con diferentes valores de inicialización de α para bloques de atención y feedforward.*\n\nPara LLaMA 7B, la inicialización óptima de α resultó ser 0.2 para bloques de atención y 0.2 para otros bloques, mientras que para LLaMA 13B, fue 0.6 para bloques de atención y 0.15 para otros bloques. Esto sugiere que los modelos más grandes pueden requerir un ajuste más cuidadoso del parámetro α.\n\nLos investigadores también probaron la escalabilidad de su enfoque entrenando modelos de diferentes profundidades y anchuras:\n\n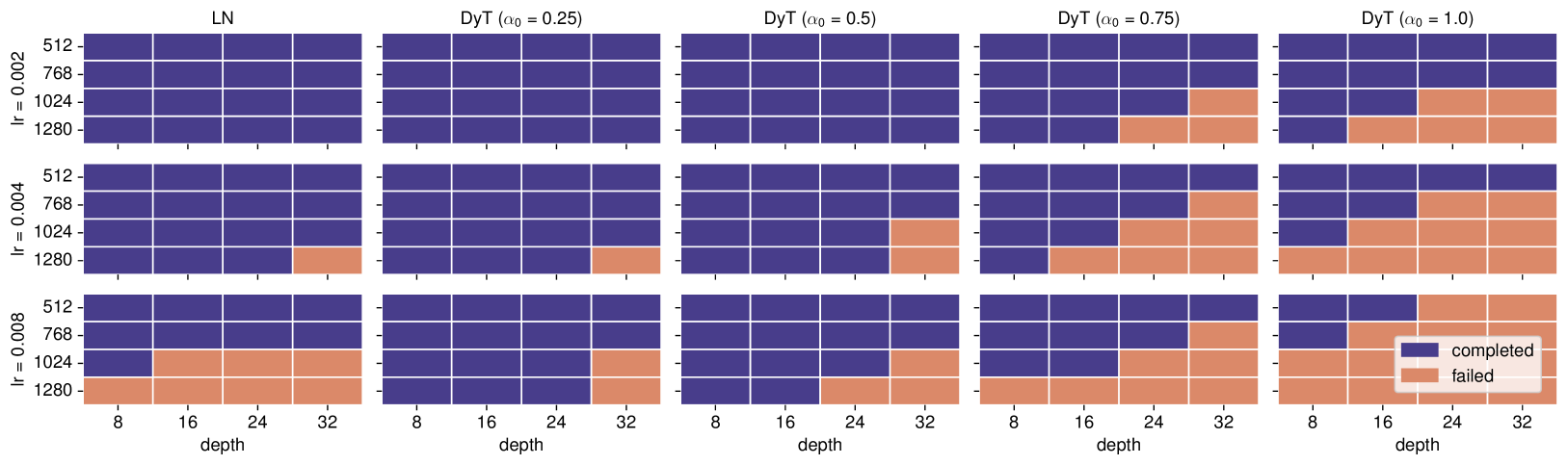\n*Figura 7: Comparación de estabilidad de entrenamiento entre LN y DyT a través de diferentes profundidades y anchuras de modelo, con azul indicando entrenamiento exitoso y naranja indicando inestabilidad.*\n\nLos resultados mostraron que los modelos DyT podían escalar de manera comparable a los modelos LN, aunque con algo más de sensibilidad a la tasa de aprendizaje en escalas mayores.\n\n## Análisis del Parámetro Alpha\n\nLos investigadores analizaron cómo el parámetro α en DyT se relaciona con las propiedades estadísticas de las activaciones. Interesantemente, encontraron que α aprende a aproximar el inverso de la desviación estándar de las activaciones de la capa:\n\n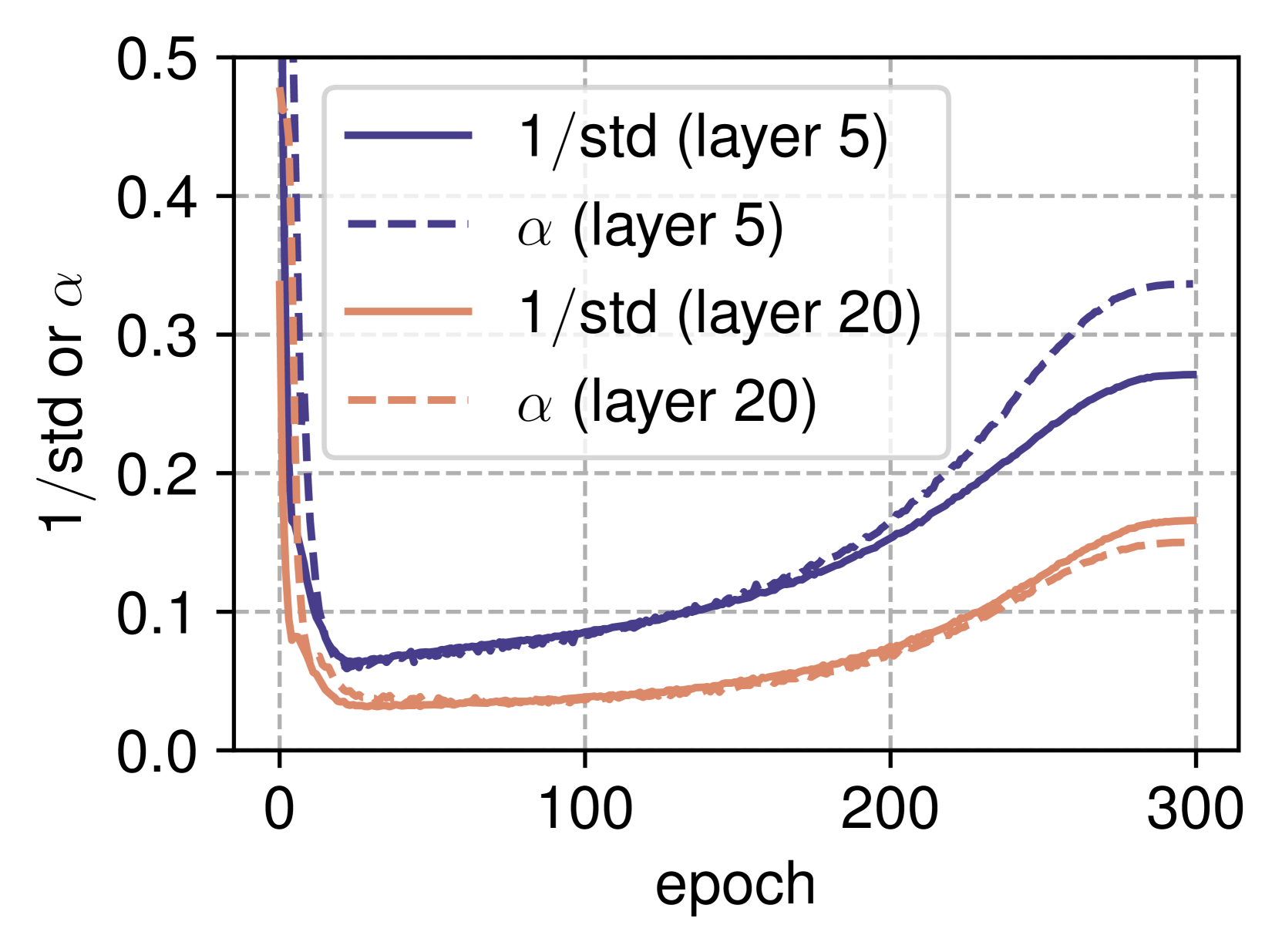\n*Figura 8: Comparación entre los valores α aprendidos y la inversa de la desviación estándar de activación (1/std) a lo largo de las épocas de entrenamiento, mostrando cómo α imita parcialmente el comportamiento de normalización.*\n\nEste hallazgo sugiere que DyT aprende implícitamente a realizar una forma de escalado adaptativo similar a las capas de normalización, pero sin calcular explícitamente estadísticas. El parámetro α tiende a ser inversamente proporcional a la desviación estándar de las activaciones, escalando efectivamente las entradas de manera que su magnitud sea apropiada para la función tanh.\n\nAdemás, observaron una correlación consistente entre los valores α aprendidos y la inversa de la desviación estándar de las activaciones a través de diferentes capas y modelos:\n\n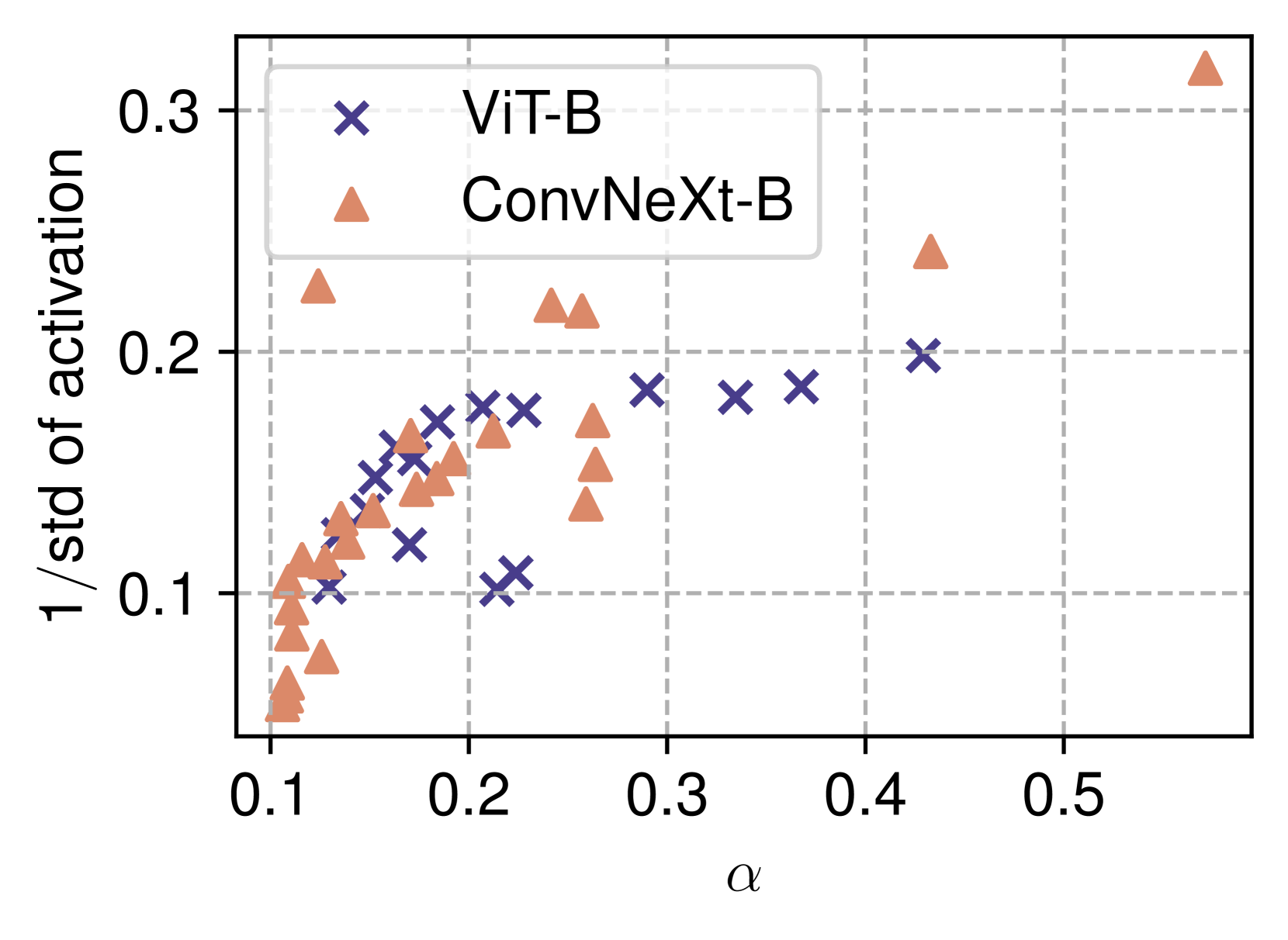\n*Figura 9: Gráfico de dispersión que muestra la relación entre los valores α aprendidos y la inversa de la desviación estándar de las activaciones a través de diferentes capas en modelos ViT-B y ConvNeXt-B.*\n\n## Comparación con Otros Enfoques\n\nLos investigadores compararon DyT con otros métodos propuestos para entrenar redes profundas sin normalización, incluyendo Fixup, SkipInit y σReparam. A través de varias tareas y arquitecturas de modelos, DyT superó consistentemente estas alternativas.\n\nTambién realizaron estudios de ablación para validar la importancia tanto de la función tanh como del parámetro de escala α aprendible. Estos estudios mostraron que:\n\n1. Reemplazar tanh con otras funciones como sigmoid o hardtanh llevó a un rendimiento reducido, destacando la importancia de las propiedades específicas de tanh.\n\n2. Usar un α fijo en lugar de uno aprendible degradó significativamente el rendimiento, demostrando la importancia de la adaptabilidad.\n\n3. Eliminar completamente la no linealidad (usando solo una escala aprendible) llevó a inestabilidad en el entrenamiento, indicando que la naturaleza acotada de tanh es crucial.\n\nTambién se estudió el impacto de los valores iniciales de α en el rendimiento del modelo a través de diferentes tareas:\n\n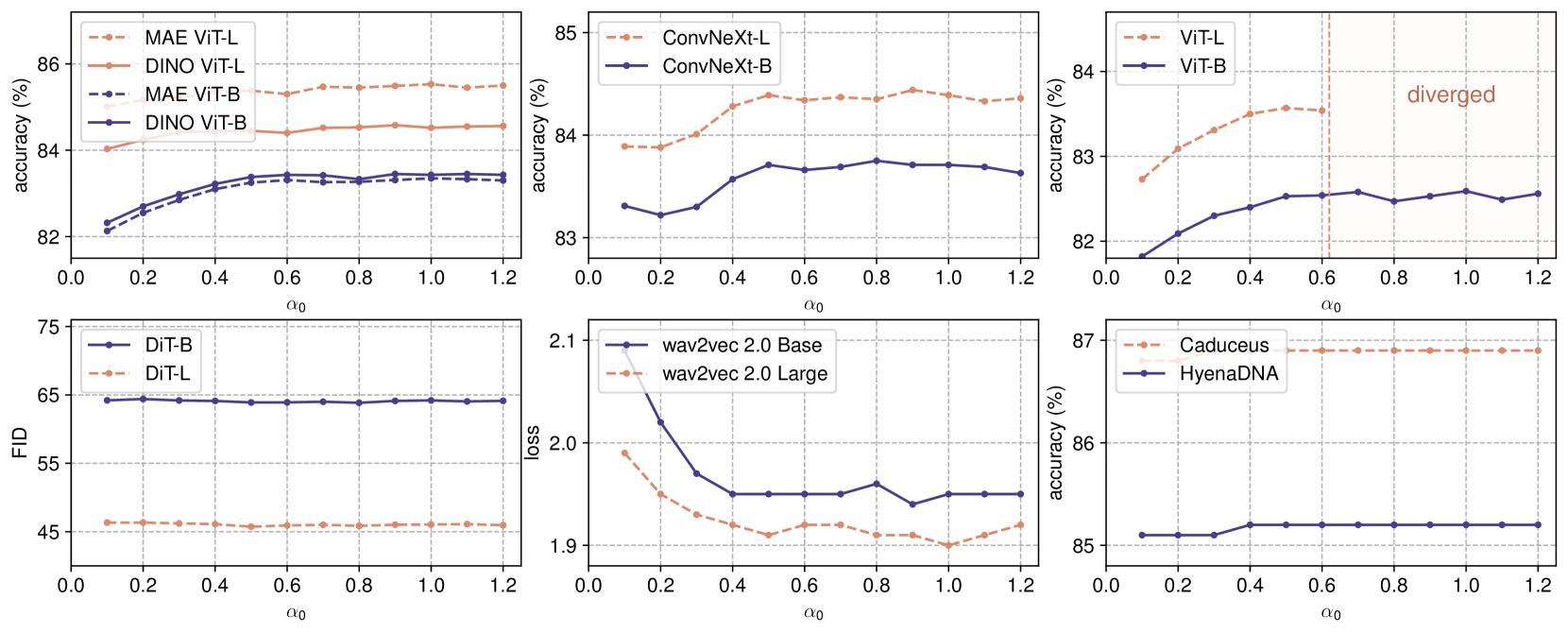\n*Figura 10: Rendimiento de varios modelos con diferentes valores de inicialización de α (α₀), mostrando sensibilidad dependiente de la tarea.*\n\n## Implicaciones y Aplicaciones\n\nLos hallazgos de esta investigación tienen varias implicaciones importantes:\n\n1. **Simplificación Arquitectónica**: Al reemplazar las capas de normalización con DyT, las arquitecturas Transformer pueden simplificarse, potencialmente llevando a modelos más interpretables.\n\n2. **Eficiencia Computacional**: Las mediciones preliminares sugieren que DyT puede mejorar la velocidad de entrenamiento e inferencia comparado con las capas de normalización, ya que elimina la necesidad de calcular estadísticas.\n\n3. **Comprensión Teórica**: El éxito de DyT proporciona información sobre el papel fundamental de la normalización en el aprendizaje profundo, sugiriendo que el beneficio clave puede ser la transformación en forma de S más que la normalización de estadísticas per se.\n\n4. **Aplicabilidad Entre Dominios**: El éxito consistente de DyT a través de diversos dominios (visión, lenguaje, habla, biología) sugiere que captura un principio fundamental de la optimización del aprendizaje profundo.\n\nUna limitación señalada por los autores es que DyT puede no ser directamente aplicable a arquitecturas CNN clásicas que usan normalización por lotes sin investigación adicional. El enfoque de su trabajo fue principalmente en arquitecturas Transformer.\n\n## Conclusión\n\nEl artículo \"Transformers without Normalization\" presenta una contribución significativa al diseño de arquitecturas de aprendizaje profundo al demostrar que las capas de normalización en Transformers pueden ser efectivamente reemplazadas con una simple operación de Tanh Dinámica (DyT). Esto desafía la sabiduría convencional de que las capas de normalización son indispensables para entrenar Transformers de alto rendimiento.\n\nEl enfoque propuesto de DyT ofrece una alternativa convincente que es fácil de implementar, a menudo requiere un ajuste mínimo y puede igualar o superar el rendimiento de los modelos normalizados en una amplia gama de tareas y dominios. El hallazgo de que α en DyT aprende a aproximar el inverso de la desviación estándar de activación proporciona información sobre cómo este simple mecanismo imita efectivamente ciertos aspectos de la normalización.\n\nEsta investigación abre nuevas vías para simplificar las arquitecturas de redes neuronales y puede inspirar una mayor exploración de alternativas a las técnicas de normalización tradicionales. A medida que el aprendizaje profundo continúa evolucionando, tales simplificaciones podrían contribuir a modelos más eficientes e interpretables.\n\n## Citas Relevantes\n\nJimmy Lei Ba, Jamie Ryan Kiros, y Geoffrey E Hinton. [Layer normalization](https://alphaxiv.org/abs/1607.06450). arXiv preprint arXiv:1607.06450, 2016.\n\n * Este artículo introduce la Normalización de Capas (LN), un componente crucial para estabilizar el entrenamiento en redes profundas, especialmente Transformers. El artículo analiza el comportamiento de LN y propone Dynamic Tanh (DyT) como reemplazo, haciendo esta cita altamente relevante.\n\nAlexey Dosovitskiy, Lucas Beyer, Alexander Kolesnikov, Dirk Weissenborn, Xiaohua Zhai, Thomas Unterthiner, Mostafa Dehghani, Matthias Minderer, Georg Heigold, Sylvain Gelly, et al. An image is worth 16x16 words: Transformers for image recognition at scale. arXiv preprint arXiv:2010.11929, 2020.\n\n * Este artículo introduce el Vision Transformer (ViT), una arquitectura prominente utilizada para evaluar la efectividad de DyT en tareas de clasificación de imágenes. El artículo utiliza ViT como arquitectura central para demostrar que DyT puede reemplazar la normalización de capas.\n\nBiao Zhang y Rico Sennrich. [Root mean square layer normalization](https://alphaxiv.org/abs/1910.07467). NeurIPS, 2019.\n\n * Este trabajo introduce RMSNorm, una alternativa a la Normalización de Capas, y se utiliza como comparación base para DyT, particularmente en experimentos con Modelos de Lenguaje Grandes. El artículo explora DyT como reemplazo tanto para LN como para RMSNorm.\n\nHugo Touvron, Thibaut Lavril, Gautier Izacard, Xavier Martinet, Marie-Anne Lachaux, Timothée Lacroix, Baptiste Rozière, Naman Goyal, Eric Hambro, Faisal Azhar, et al. [Llama: Open and efficient foundation language models](https://alphaxiv.org/abs/2302.13971). arXiv preprint arXiv:2302.13971, 2023a.\n\n * Esta cita introduce el modelo de lenguaje LLaMA, que sirve como arquitectura clave para probar y evaluar DyT en el contexto de modelos de lenguaje grandes. El artículo utiliza LLaMA como una arquitectura importante para verificar la generalización de DyT.\n\nAshish Vaswani, Noam Shazeer, Niki Parmar, Jakob Uszkoreit, Llion Jones, Aidan N Gomez, Łukasz Kaiser, e Illia Polosukhin. [Attention is all you need](https://alphaxiv.org/abs/1706.03762). NeurIPS, 2017.\n\n * Este artículo fundamental introduce la arquitectura Transformer, que es el enfoque principal del estudio DyT. El artículo se centra en mostrar cómo DyT puede mejorar los Transformers."])</script><script>self.__next_f.push([1,"95:T83e9,"])</script><script>self.__next_f.push([1,"# नॉर्मलाइज़ेशन के बिना ट्रांसफॉर्मर्स: डायनामिक टैन्ह के साथ एक सरल विकल्प\n\n## विषय-सूची\n- [परिचय](#परिचय)\n- [नॉर्मलाइज़ेशन लेयर्स को समझना](#नॉर्मलाइज़ेशन-लेयर्स-को-समझना)\n- [डायनामिक टैन्ह समाधान](#डायनामिक-टैन्ह-समाधान)\n- [DyT कैसे काम करता है](#dyt-कैसे-काम-करता-है)\n- [प्रायोगिक प्रमाण](#प्रायोगिक-प्रमाण)\n- [ट्यूनिंग और स्केलेबिलिटी](#ट्यूनिंग-और-स्केलेबिलिटी)\n- [अल्फा पैरामीटर का विश्लेषण](#अल्फा-पैरामीटर-का-विश्लेषण)\n- [अन्य दृष्टिकोणों से तुलना](#अन्य-दृष्टिकोणों-से-तुलना)\n- [निहितार्थ और अनुप्रयोग](#निहितार्थ-और-अनुप्रयोग)\n- [निष्कर्ष](#निष्कर्ष)\n\n## परिचय\n\nनॉर्मलाइज़ेशन लेयर्स को आधुनिक न्यूरल नेटवर्क्स में आवश्यक घटकों के रूप में माना जाता है, विशेष रूप से ट्रांसफॉर्मर आर्किटेक्चर में जो प्राकृतिक भाषा प्रसंस्करण, कंप्यूटर विजन और अन्य डोमेन में प्रमुख हैं। लेयर नॉर्मलाइज़ेशन (LN) और इसके विविध रूप ट्रांसफॉर्मर्स में सर्वव्यापी हैं, जिन्हें प्रशिक्षण को स्थिर करने और प्रदर्शन में सुधार के लिए महत्वपूर्ण माना जाता है। हालांकि, मेटा एआई, एनवाईयू, एमआईटी और प्रिंसटन विश्वविद्यालय के शोधकर्ताओं द्वारा एक नया पेपर इस मौलिक धारणा को चुनौती देता है, यह प्रदर्शित करते हुए कि ट्रांसफॉर्मर्स पारंपरिक नॉर्मलाइज़ेशन लेयर्स के बिना समान या बेहतर प्रदर्शन प्राप्त कर सकते हैं।\n\n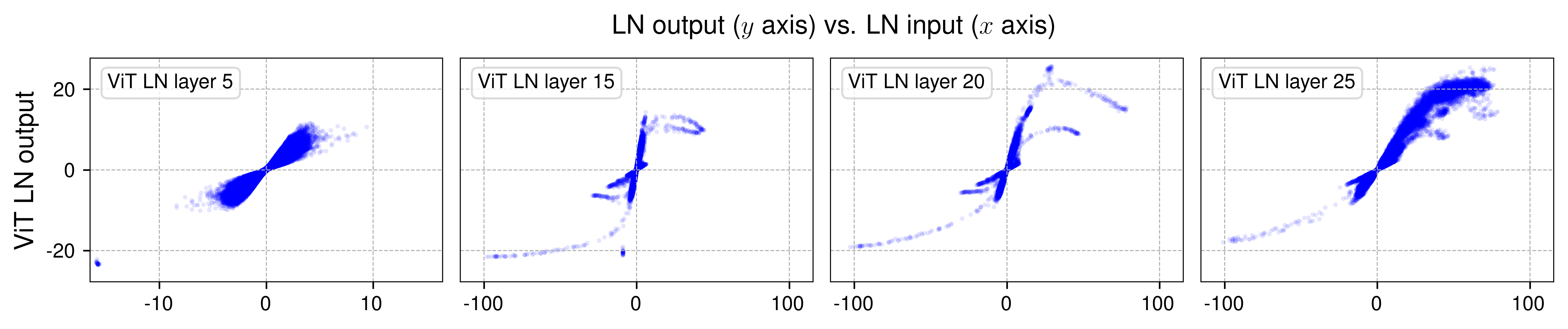\n*चित्र 1: विभिन्न ViT लेयर्स में लेयर नॉर्मलाइज़ेशन के इनपुट-आउटपुट व्यवहार का विज़ुअलाइज़ेशन, एस-आकार के, टैन्ह-जैसे संबंधों को दर्शाता है।*\n\n## नॉर्मलाइज़ेशन लेयर्स को समझना\n\nबैच नॉर्मलाइज़ेशन, लेयर नॉर्मलाइज़ेशन और RMSNorm जैसी नॉर्मलाइज़ेशन तकनीकें डीप लर्निंग में मानक प्रथा बन गई हैं। ये विधियां सामान्यतः निर्दिष्ट आयामों में सांख्यिकी (माध्य और/या मानक विचलन) की गणना करके सक्रियण को सामान्य करती हैं, जो नेटवर्क सक्रियण के वितरण को नियंत्रित करके प्रशिक्षण को स्थिर करने में मदद करती हैं।\n\nविशेष रूप से ट्रांसफॉर्मर्स में, लेयर नॉर्मलाइज़ेशन प्रत्येक टोकन या स्थिति के लिए फीचर आयाम में माध्य और मानक विचलन की गणना करके काम करता है। यह नॉर्मलाइज़ेशन प्रक्रिया कम्प्यूटेशनल रूप से महंगी है क्योंकि इसे प्रशिक्षण और अनुमान दोनों के दौरान प्रत्येक लेयर में इन आंकड़ों की गणना करने की आवश्यकता होती है।\n\nलेखकों ने देखा कि लेयर नॉर्मलाइज़ेशन अक्सर टैन्ह-जैसे, एस-आकार के इनपुट-आउटपुट मैपिंग उत्पन्न करता है, जैसा कि चित्र 1 में दिखाया गया है। इस अवलोकन ने उनकी मुख्य अंतर्दृष्टि की ओर ले जाया: शायद नॉर्मलाइज़ेशन का लाभकारी प्रभाव एक सरल तंत्र के माध्यम से प्राप्त किया जा सकता है जो सक्रियण आंकड़ों की गणना किए बिना इस एस-आकार के व्यवहार की नकल करता है।\n\n## डायनामिक टैन्ह समाधान\n\nशोधकर्ता नॉर्मलाइज़ेशन लेयर्स के लिए डायनामिक टैन्ह (DyT) को एक सीधा विकल्प के रूप में प्रस्तावित करते हैं। DyT को इस प्रकार परिभाषित किया जाता है:\n\n```\nDyT(x) = tanh(αx)\n```\n\nजहां α एक सीखने योग्य पैरामीटर है जो टैन्ह फंक्शन की तीव्रता को नियंत्रित करता है। यह सरल सूत्रीकरण सक्रियण आंकड़ों की गणना की आवश्यकता को समाप्त करता है जबकि एस-आकार के रूपांतरण को संरक्षित करता है जो ट्रांसफॉर्मर प्रदर्शन के लिए महत्वपूर्ण प्रतीत होता है।\n\n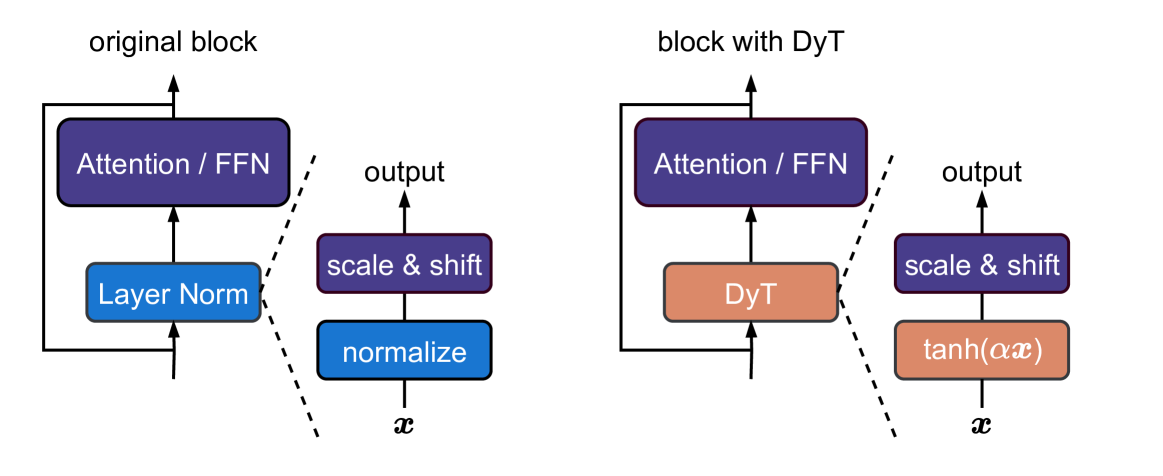\n*चित्र 2: बाएं: लेयर नॉर्मलाइज़ेशन के साथ मूल ट्रांसफॉर्मर ब्लॉक। दाएं: डायनामिक टैन्ह (DyT) प्रतिस्थापन के साथ प्रस्तावित ब्लॉक।*\n\n## DyT कैसे काम करता है\n\nडायनामिक टैन्ह दो प्रमुख तंत्रों के माध्यम से काम करता है:\n\n1. **मान दबाना**: टैन्ह फंक्शन चरम मानों को दबाता है, नॉर्मलाइज़ेशन लेयर्स के समान एक प्रकार का अंतर्निहित नियमितीकरण प्रदान करता है। यह फॉरवर्ड और बैकवर्ड पास के दौरान सक्रियण के बहुत बड़े होने से रोकता है।\n\n2. **अनुकूली स्केलिंग**: सीखने योग्य पैरामीटर α, tanh फंक्शन की तीव्रता को समायोजित करता है, जो नेटवर्क को यह नियंत्रित करने की अनुमति देता है कि मान कितनी आक्रामकता से दबाए जाएं। यह अनुकूलन प्रदर्शन के लिए महत्वपूर्ण है।\n\nहाइपरबोलिक टैनजेंट फंक्शन (tanh) -1 और 1 के बीच सीमित है, जो किसी भी इनपुट मान को इस रेंज में दबाता है। इस दबाव की तीव्रता α द्वारा नियंत्रित की जाती है:\n\n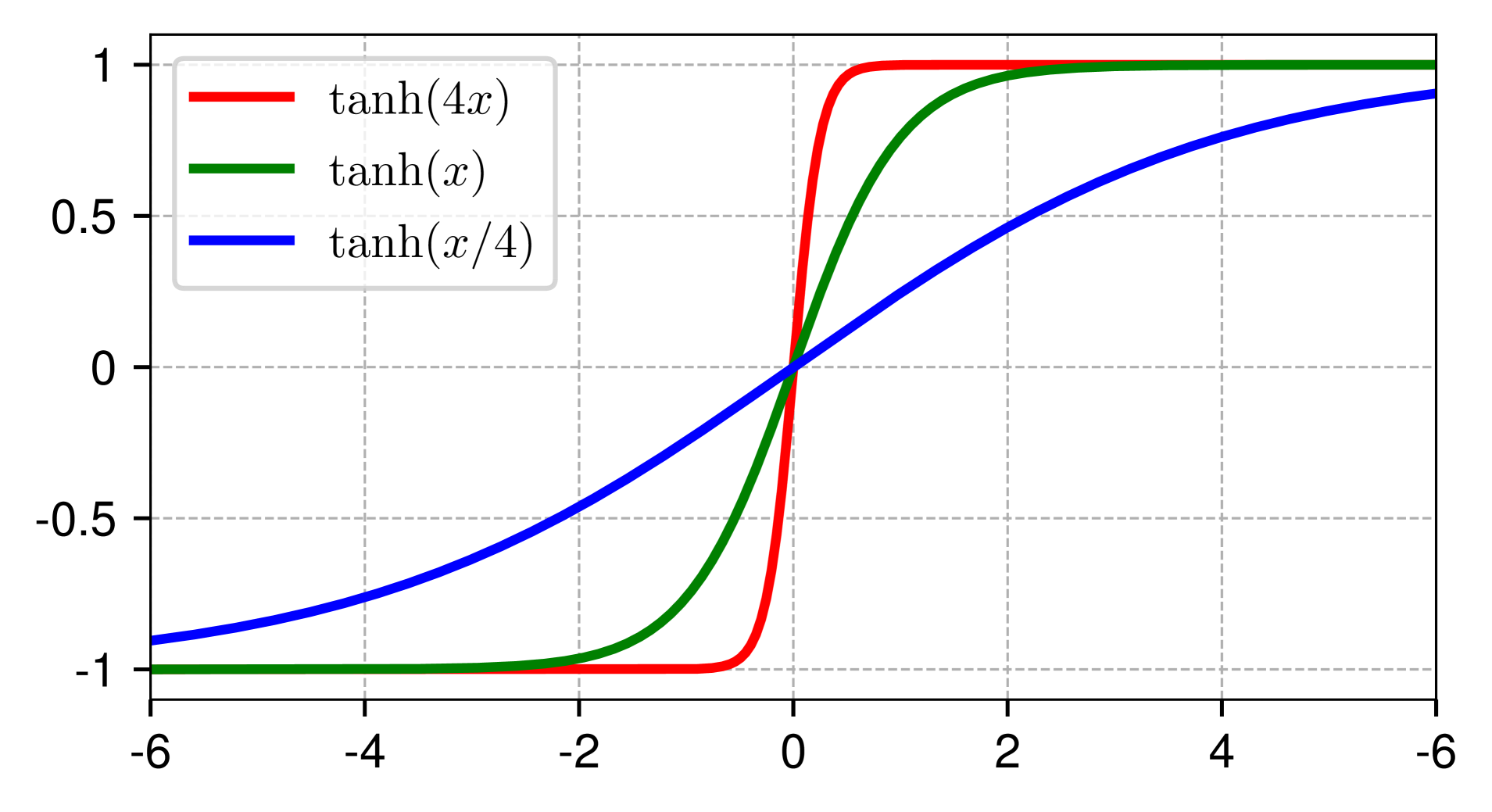\n*चित्र 3: विभिन्न α मानों के साथ tanh फंक्शन, जो दिखाता है कि कैसे बड़े α मान तेज संक्रमण बनाते हैं।*\n\nजैसा कि चित्र 3 में दिखाया गया है, बड़ा α मान -1 से 1 तक के संक्रमण को तेज बनाता है, जबकि छोटा α इसे अधिक क्रमिक बनाता है। यह लचीलापन नेटवर्क को कार्य और परत की गहराई के आधार पर मान दबाव की डिग्री को समायोजित करने की अनुमति देता है।\n\n## प्रायोगिक साक्ष्य\n\nशोधकर्ताओं ने नॉर्मलाइजेशन लेयर्स के विकल्प के रूप में DyT की प्रभावशीलता को मान्य करने के लिए विविध कार्यों और डोमेन में व्यापक प्रयोग किए। इन प्रयोगों में शामिल थे:\n\n1. **विजन टास्क**:\n - विजन ट्रांसफॉर्मर्स (ViT) और ConvNeXt के साथ ImageNet वर्गीकरण\n - MAE और DINO के साथ सेल्फ-सुपरवाइज्ड लर्निंग\n\n2. **जनरेटिव मॉडल्स**:\n - छवि निर्माण के लिए डिफ्यूजन मॉडल्स (DiT)\n\n3. **लार्ज लैंग्वेज मॉडल्स**:\n - 7B से 70B पैरामीटर्स तक के स्केल पर LLaMA प्रीट्रेनिंग\n\n4. **अन्य डोमेन**:\n - wav2vec 2.0 के साथ स्पीच प्रोसेसिंग\n - HyenaDNA और Caduceus के साथ DNA सीक्वेंस मॉडलिंग\n\nपरिणामों ने लगातार दिखाया कि DyT के साथ ट्रांसफॉर्मर्स उनके नॉर्मलाइज्ड समकक्षों के बराबर या बेहतर प्रदर्शन कर सकते हैं। उदाहरण के लिए, ImageNet वर्गीकरण पर विजन ट्रांसफॉर्मर्स के साथ, DyT वैरिएंट ने LN वर्जन के समान सटीकता प्राप्त की:\n\n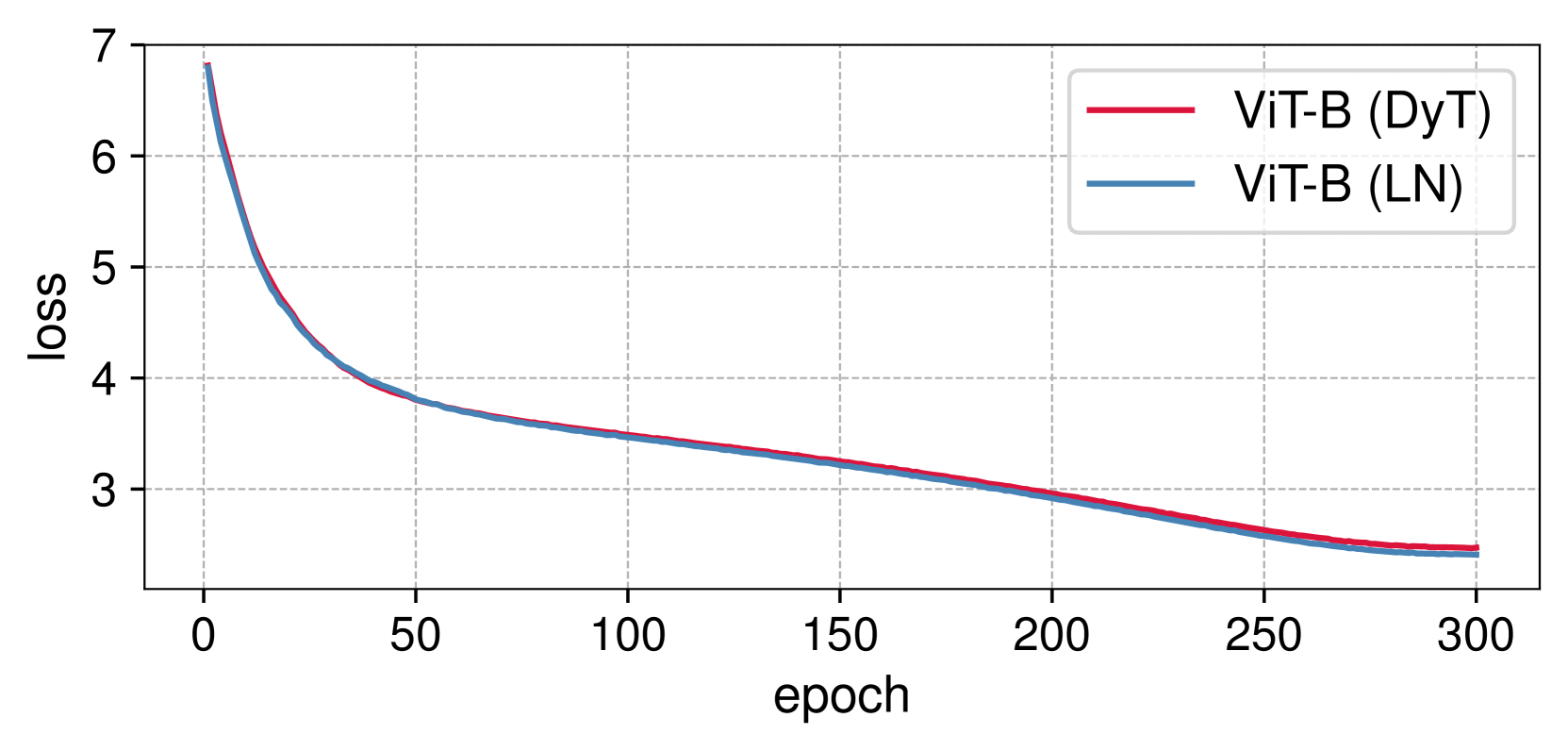\n*चित्र 4: लेयर नॉर्मलाइजेशन (LN) और डायनामिक टैन्ह (DyT) के साथ ViT-B के लिए प्रशिक्षण लॉस वक्र, जो लगभग समान कन्वर्जेंस दिखा रहे हैं।*\n\nइसी तरह, विभिन्न आकारों (7B से 70B पैरामीटर्स) के LLaMA मॉडल्स के लिए, DyT वैरिएंट्स ने RMSNorm मॉडल्स की तुलना में समान या थोड़ा बेहतर लॉस वैल्यू प्राप्त की:\n\n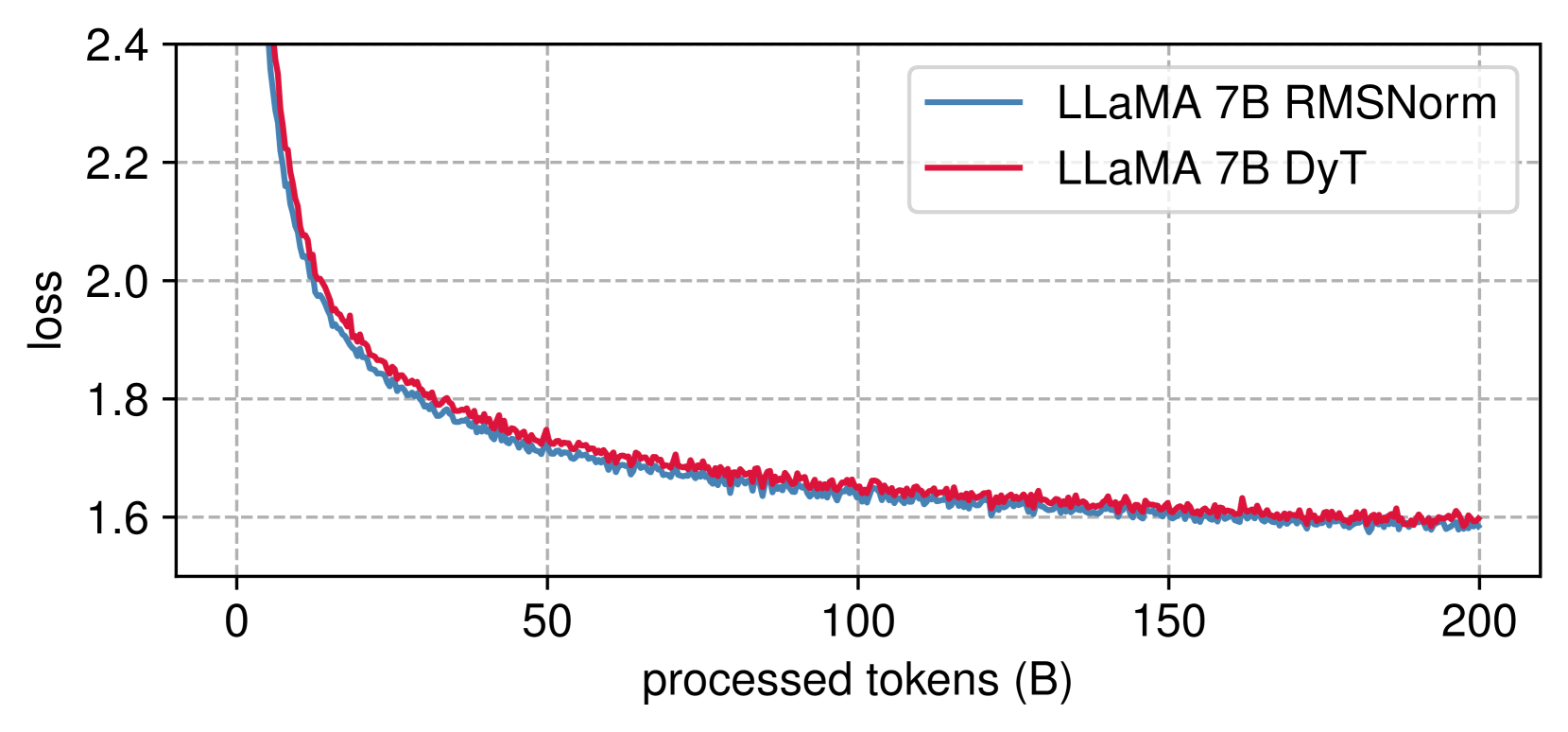\n*चित्र 5: RMSNorm और DyT के साथ LLaMA 7B के लिए प्रशिक्षण लॉस वक्र, जो तुलनीय प्रदर्शन दिखा रहे हैं।*\n\n## ट्यूनिंग और स्केलेबिलिटी\n\nहालांकि DyT सामान्यतः मजबूत है और न्यूनतम ट्यूनिंग के साथ अच्छा काम करता है, शोधकर्ताओं ने पाया कि बड़े मॉडल्स, विशेष रूप से लार्ज लैंग्वेज मॉडल्स (LLMs) के लिए, α का सावधानीपूर्वक इनिशियलाइजेशन महत्वपूर्ण है। उन्होंने LLaMA आर्किटेक्चर के लिए इनिशियलाइजेशन मानों का विस्तृत अन्वेषण किया:\n\n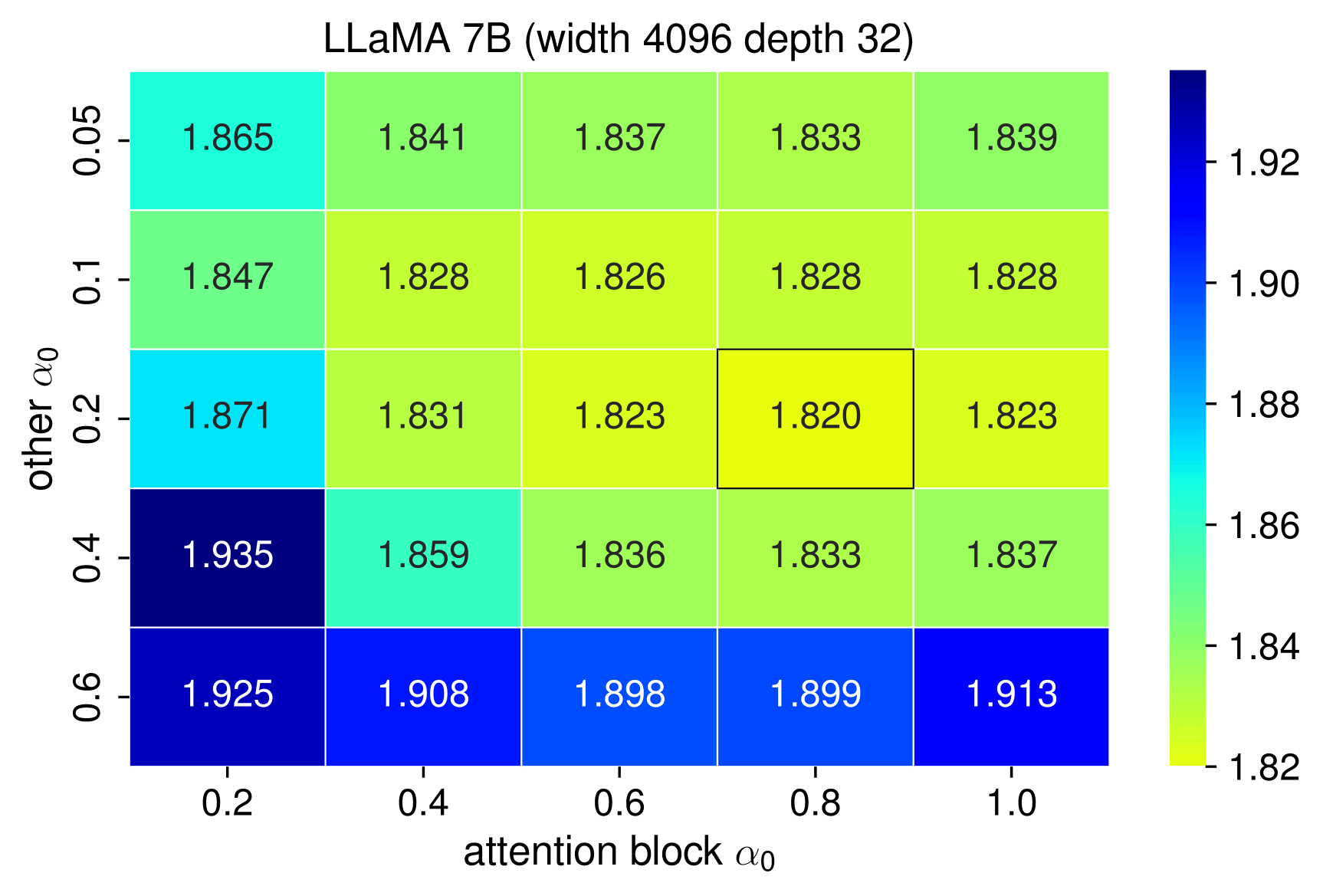\n*चित्र 6: ध्यान और फीडफॉरवर्ड ब्लॉक्स के लिए विभिन्न α इनिशियलाइजेशन मानों के साथ LLaMA 7B प्रदर्शन दिखाने वाला हीटमैप।*\n\nLLaMA 7B के लिए, इष्टतम α इनिशियलाइजेशन ध्यान ब्लॉक्स के लिए 0.2 और अन्य ब्लॉक्स के लिए 0.2 पाया गया, जबकि LLaMA 13B के लिए, यह ध्यान ब्लॉक्स के लिए 0.6 और अन्य ब्लॉक्स के लिए 0.15 था। यह सुझाव देता है कि बड़े मॉडल्स को α पैरामीटर की अधिक सावधानीपूर्वक ट्यूनिंग की आवश्यकता हो सकती है।\n\nशोधकर्ताओं ने विभिन्न गहराई और चौड़ाई के मॉडल्स को प्रशिक्षित करके अपने दृष्टिकोण की स्केलेबिलिटी का भी परीक्षण किया:\n\n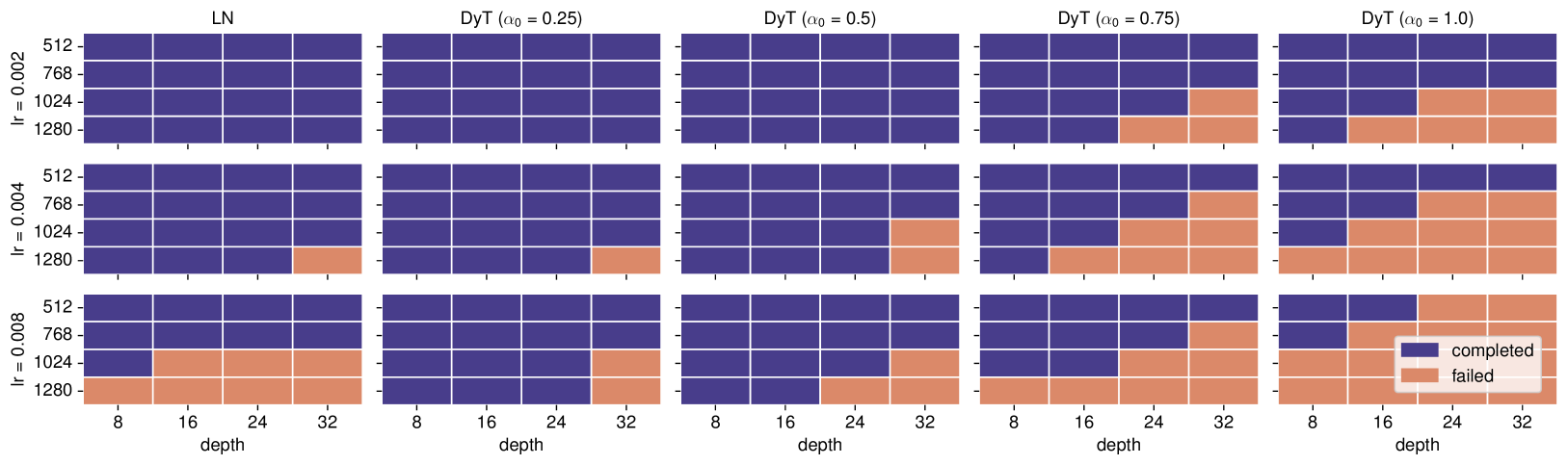\n*चित्र 7: विभिन्न मॉडल गहराई और चौड़ाई में LN और DyT के बीच प्रशिक्षण स्थिरता की तुलना, जहां नीला सफल प्रशिक्षण और नारंगी अस्थिरता को दर्शाता है।*\n\nपरिणामों ने दिखाया कि DyT मॉडल्स LN मॉडल्स के समान स्केल कर सकते हैं, हालांकि बड़े स्केल पर लर्निंग रेट के प्रति कुछ अतिरिक्त संवेदनशीलता के साथ।\n\n## अल्फा पैरामीटर का विश्लेषण\n\nशोधकर्ताओं ने विश्लेषण किया कि DyT में α पैरामीटर सक्रियण के सांख्यिकीय गुणों से कैसे संबंधित है। दिलचस्प बात यह है कि उन्होंने पाया कि α लेयर सक्रियण के मानक विचलन के व्युत्क्रम का अनुमान लगाना सीखता है:\n\n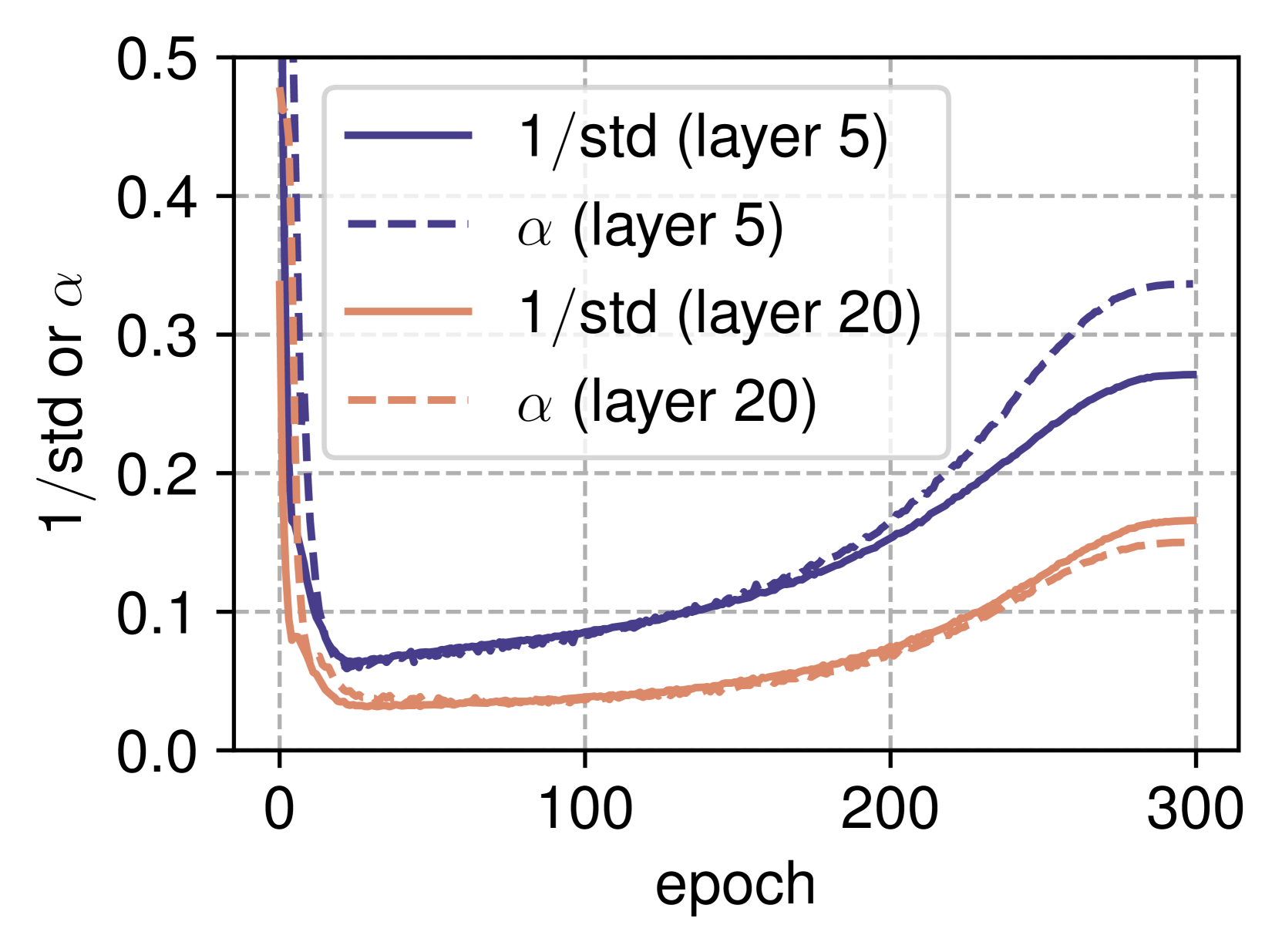\n*चित्र 8: प्रशिक्षण चरणों में सीखे गए α मूल्यों और सक्रियण मानक विचलन (1/std) के व्युत्क्रम के बीच तुलना, जो दर्शाती है कि α किस प्रकार आंशिक रूप से सामान्यीकरण व्यवहार की नकल करता है।*\n\nयह निष्कर्ष सुझाता है कि DyT अंतर्निहित रूप से सामान्यीकरण परतों के समान अनुकूली स्केलिंग करना सीखता है, लेकिन सांख्यिकी की स्पष्ट गणना किए बिना। α पैरामीटर सक्रियण के मानक विचलन के व्युत्क्रमानुपाती होता है, जो प्रभावी रूप से इनपुट को इस तरह से स्केल करता है कि उनका परिमाण tanh फंक्शन के लिए उपयुक्त हो।\n\nइसके अलावा, उन्होंने विभिन्न परतों और मॉडलों में सीखे गए α मूल्यों और सक्रियण के व्युत्क्रम मानक विचलन के बीच एक स्थिर सहसंबंध देखा:\n\n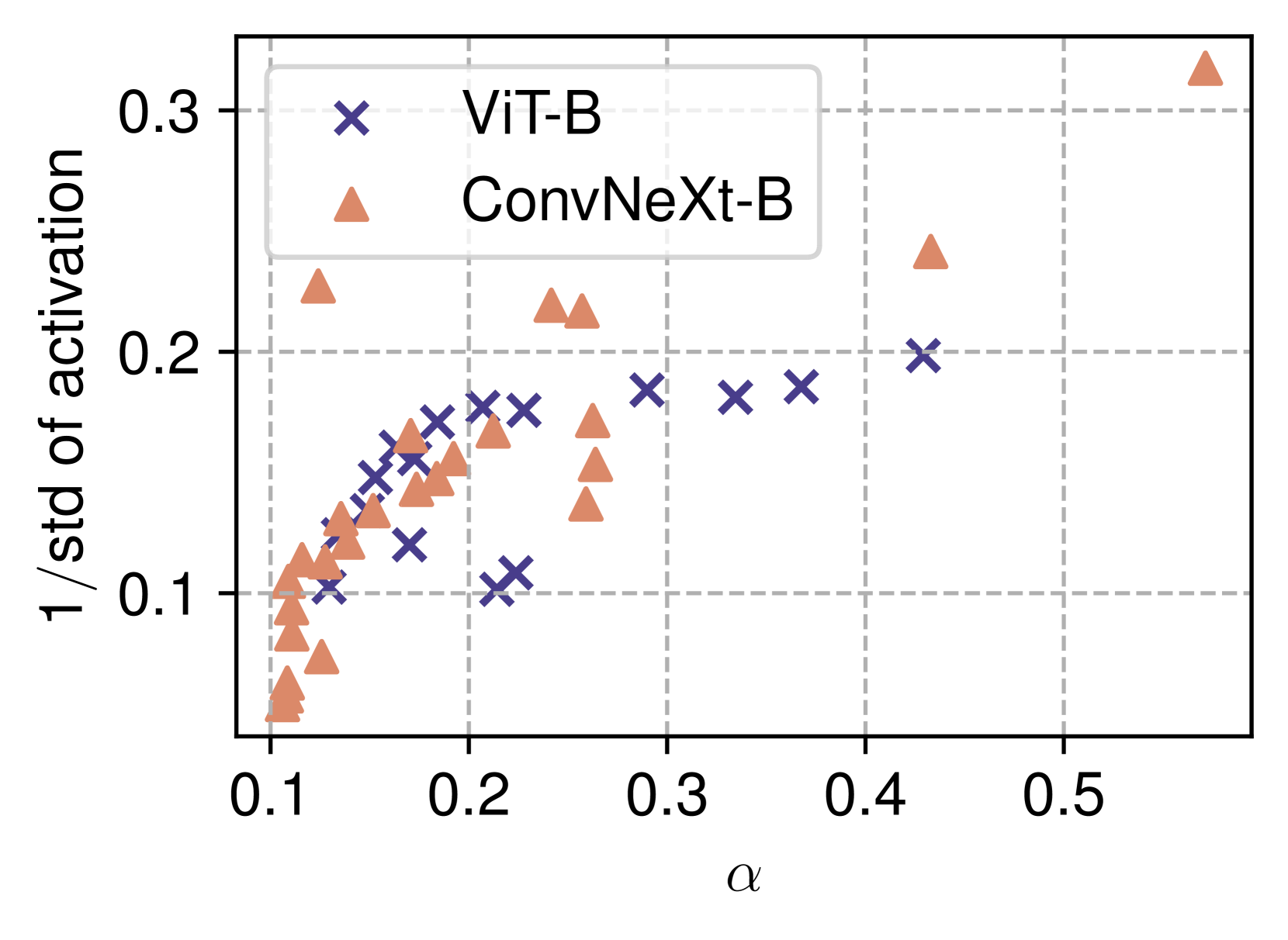\n*चित्र 9: ViT-B और ConvNeXt-B मॉडलों में विभिन्न परतों में सीखे गए α मूल्यों और सक्रियण के व्युत्क्रम मानक विचलन के बीच संबंध दर्शाने वाला स्कैटर प्लॉट।*\n\n## अन्य दृष्टिकोणों से तुलना\n\nशोधकर्ताओं ने सामान्यीकरण के बिना गहरे नेटवर्क के प्रशिक्षण के लिए प्रस्तावित अन्य विधियों जैसे Fixup, SkipInit, और σReparam के साथ DyT की तुलना की। विभिन्न कार्यों और मॉडल आर्किटेक्चर में, DyT ने लगातार इन विकल्पों से बेहतर प्रदर्शन किया।\n\nउन्होंने tanh फंक्शन और सीखने योग्य स्केल पैरामीटर α दोनों के महत्व को मान्य करने के लिए विलोपन अध्ययन भी किए। इन अध्ययनों ने दिखाया कि:\n\n1. tanh को sigmoid या hardtanh जैसे अन्य फंक्शन से बदलने से प्रदर्शन कम हुआ, जो tanh के विशिष्ट गुणों के महत्व को उजागर करता है।\n\n2. सीखने योग्य के बजाय एक निश्चित α का उपयोग करने से प्रदर्शन में काफी गिरावट आई, जो अनुकूलनशीलता के महत्व को दर्शाता है।\n\n3. गैर-रैखिकता को पूरी तरह से हटाने (केवल सीखने योग्य स्केल का उपयोग) से प्रशिक्षण अस्थिरता उत्पन्न हुई, जो दर्शाता है कि tanh की सीमित प्रकृति महत्वपूर्ण है।\n\nविभिन्न कार्यों में मॉडल प्रदर्शन पर प्रारंभिक α मूल्यों के प्रभाव का भी अध्ययन किया गया:\n\n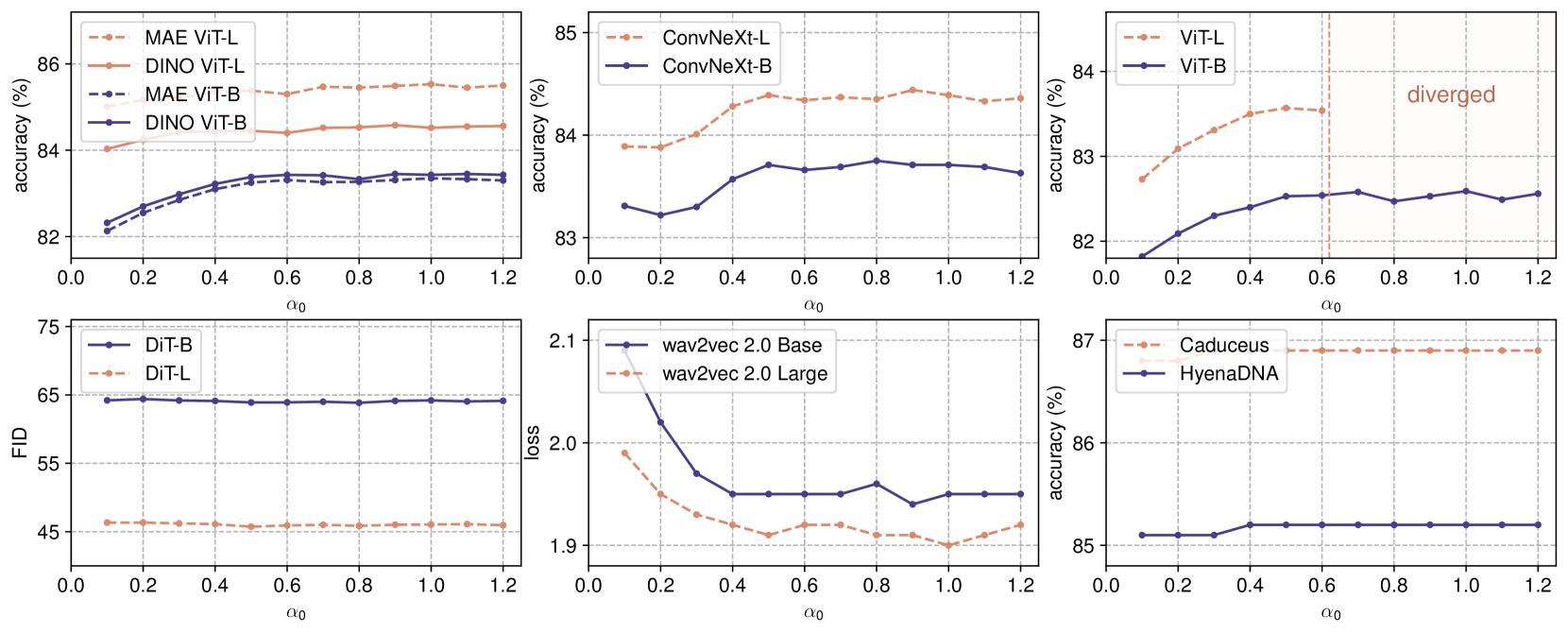\n*चित्र 10: विभिन्न α प्रारंभीकरण मूल्यों (α₀) के साथ विभिन्न मॉडलों का प्रदर्शन, कार्य-निर्भर संवेदनशीलता दिखाता है।*\n\n## निहितार्थ और अनुप्रयोग\n\nइस शोध के निष्कर्षों के कई महत्वपूर्ण निहितार्थ हैं:\n\n1. **आर्किटेक्चरल सरलीकरण**: सामान्यीकरण परतों को DyT से बदलकर, ट्रांसफॉर्मर आर्किटेक्चर को सरल बनाया जा सकता है, जो संभवतः अधिक व्याख्या योग्य मॉडल की ओर ले जाता है।\n\n2. **कम्प्यूटेशनल दक्षता**: प्रारंभिक मापों से संकेत मिलता है कि DyT सांख्यिकी की गणना की आवश्यकता को समाप्त करके सामान्यीकरण परतों की तुलना में प्रशिक्षण और अनुमान गति में सुधार कर सकता है।\n\n3. **सैद्धांतिक समझ**: DyT की सफलता डीप लर्निंग में सामान्यीकरण की मौलिक भूमिका में अंतर्दृष्टि प्रदान करती है, जो सुझाव देती है कि प्रमुख लाभ सांख्यिकी के सामान्यीकरण के बजाय S-आकार का परिवर्तन हो सकता है।\n\n4. **क्रॉस-डोमेन प्रयोज्यता**: विविध डोमेन (दृष्टि, भाषा, भाषण, जीवविज्ञान) में DyT की निरंतर सफलता सुझाव देती है कि यह डीप लर्निंग अनुकूलन के एक मौलिक सिद्धांत को पकड़ता है।\n\nलेखकों द्वारा नोट की गई एक सीमा यह है कि DyT आगे के शोध के बिना बैच सामान्यीकरण का उपयोग करने वाले क्लासिक CNN आर्किटेक्चर पर सीधे लागू नहीं हो सकता है। उनके काम का मुख्य फोकस ट्रांसफॉर्मर आर्किटेक्चर पर था।\n\n## निष्कर्ष\n\n\"ट्रांसफॉर्मर्स विदाउट नॉर्मलाइजेशन\" पेपर डीप लर्निंग आर्किटेक्चर डिजाइन में एक महत्वपूर्ण योगदान प्रस्तुत करता है, यह प्रदर्शित करके कि ट्रांसफॉर्मर्स में सामान्यीकरण परतों को प्रभावी ढंग से एक सरल डायनामिक टैन्ह (DyT) ऑपरेशन से बदला जा सकता है। यह इस पारंपरिक मान्यता को चुनौती देता है कि उच्च-प्रदर्शन ट्रांसफॉर्मर्स के प्रशिक्षण के लिए सामान्यीकरण परतें अपरिहार्य हैं।\n\nप्रस्तावित DyT दृष्टिकोण एक आकर्षक विकल्प प्रस्तुत करता है जो कार्यान्वयन में आसान है, अक्सर न्यूनतम ट्यूनिंग की आवश्यकता होती है, और कार्यों एवं डोमेन की एक विस्तृत श्रृंखला में सामान्यीकृत मॉडल के प्रदर्शन की बराबरी या उससे बेहतर कर सकता है। यह खोज कि DyT में α सक्रियण मानक विचलन के व्युत्क्रम का अनुमान लगाना सीखता है, यह समझने में मदद करता है कि यह सरल तंत्र कैसे प्रभावी रूप से सामान्यीकरण के कुछ पहलुओं की नकल करता है।\n\nयह शोध तंत्रिका नेटवर्क वास्तुकला को सरल बनाने के नए मार्ग खोलता है और पारंपरिक सामान्यीकरण तकनीकों के विकल्पों की और खोज को प्रेरित कर सकता है। जैसे-जैसे डीप लर्निंग विकसित होती रहती है, ऐसी सरलीकरण अधिक कुशल और व्याख्या योग्य मॉडल में योगदान कर सकते हैं।\n\n## संबंधित उद्धरण\n\nजिमी लेई बा, जेमी रयान किरोस, और जेफरी ई हिंटन। [लेयर नॉर्मलाइजेशन](https://alphaxiv.org/abs/1607.06450)।arXiv प्रिप्रिंट arXiv:1607.06450, 2016।\n\n * यह पेपर लेयर नॉर्मलाइजेशन (LN) को प्रस्तुत करता है, जो गहरे नेटवर्क में प्रशिक्षण को स्थिर करने के लिए एक महत्वपूर्ण घटक है, विशेष रूप से ट्रांसफॉर्मर्स में। पेपर LN के व्यवहार का विश्लेषण करता है और इसके प्रतिस्थापन के रूप में डायनामिक टैन्ह (DyT) का प्रस्ताव करता है, जो इस उद्धरण को अत्यधिक प्रासंगिक बनाता है।\n\nएलेक्सी डोसोविट्स्की, लुकास बेयर, अलेक्जेंडर कोलेस्निकोव, डिर्क वीसेनबॉर्न, शियाओहुआ झाई, थॉमस अंटरथिनर, मोस्तफा देहघानी, मैथियास माइंडरर, जॉर्ज हीगोल्ड, सिल्वेन जेली, एट अल। एन इमेज इज वर्थ 16x16 वर्ड्स: ट्रांसफॉर्मर्स फॉर इमेज रेकग्निशन एट स्केल।arXiv प्रिप्रिंट arXiv:2010.11929, 2020।\n\n * यह पेपर विजन ट्रांसफॉर्मर (ViT) को प्रस्तुत करता है, जो छवि वर्गीकरण कार्यों में DyT की प्रभावशीलता के बेंचमार्किंग के लिए उपयोग की जाने वाली एक प्रमुख वास्तुकला है। पेपर यह प्रदर्शित करने के लिए ViT को एक मुख्य वास्तुकला के रूप में उपयोग करता है कि DyT लेयर नॉर्मलाइजेशन को प्रतिस्थापित कर सकता है।\n\nबियाओ झांग और रिको सेनरिच। [रूट मीन स्क्वायर लेयर नॉर्मलाइजेशन](https://alphaxiv.org/abs/1910.07467)।NeurIPS, 2019।\n\n * यह कार्य लेयर नॉर्मलाइजेशन का एक विकल्प RMSNorm प्रस्तुत करता है, और DyT के लिए एक बेसलाइन तुलना के रूप में उपयोग किया जाता है, विशेष रूप से लार्ज लैंग्वेज मॉडल प्रयोगों में। पेपर DyT को LN और RMSNorm दोनों के प्रतिस्थापन के रूप में खोजता है।\n\nह्यूगो टूवरॉन, थिबॉट लावरिल, गौतियर इज़ाकार्ड, जेवियर मार्टिनेट, मैरी-एन लाचौक्स, टिमोथी लाक्रोइक्स, बैप्टिस्ट रोज़िएर, नमन गोयल, एरिक हैम्ब्रो, फैसल अज़हर, एट अल। [लामा: ओपन एंड एफिशिएंट फाउंडेशन लैंग्वेज मॉडल्स](https://alphaxiv.org/abs/2302.13971)। arXiv प्रिप्रिंट arXiv:2302.13971, 2023a।\n\n * यह उद्धरण LLaMA भाषा मॉडल को प्रस्तुत करता है, जो बड़े भाषा मॉडल के संदर्भ में DyT के परीक्षण और मूल्यांकन के लिए एक प्रमुख वास्तुकला के रूप में कार्य करता है। पेपर DyT की सामान्यीकरण क्षमता को सत्यापित करने के लिए LLaMA को एक महत्वपूर्ण वास्तुकला के रूप में उपयोग करता है।\n\nआशीष वास्वानी, नोम शज़ीर, निकी परमार, जैकब उज़कोरेइट, लियोन जोन्स, एडन एन गोमेज़, लुकाश कैसर, और इलिया पोलोसुखिन। [अटेंशन इज़ ऑल यू नीड](https://alphaxiv.org/abs/1706.03762)।NeurIPS, 2017।\n\n * यह मौलिक पेपर ट्रांसफॉर्मर वास्तुकला को प्रस्तुत करता है, जो DyT अध्ययन का प्राथमिक फोकस है। पेपर यह दिखाने पर केंद्रित है कि DyT कैसे ट्रांसफॉर्मर्स को सुधार सकता है।"])</script><script>self.__next_f.push([1,"96:T440,Normalization layers are ubiquitous in modern neural networks and have long\nbeen considered essential. This work demonstrates that Transformers without\nnormalization can achieve the same or better performance using a remarkably\nsimple technique. We introduce Dynamic Tanh (DyT), an element-wise operation\n$DyT($x$) = \\tanh(\\alpha $x$)$, as a drop-in replacement for normalization\nlayers in Transformers. DyT is inspired by the observation that layer\nnormalization in Transformers often produces tanh-like, $S$-shaped input-output\nmappings. By incorporating DyT, Transformers without normalization can match or\nexceed the performance of their normalized counterparts, mostly without\nhyperparameter tuning. We validate the effectiveness of Transformers with DyT\nacross diverse settings, ranging from recognition to generation, supervised to\nself-supervised learning, and computer vision to language models. These\nfindings challenge the conventional understanding that normalization layers are\nindispensable in modern neural networks, and offer new insights into their role\nin deep networks."])</script><script>self.__next_f.push([1,"9:[\"$\",\"$L13\",null,{\"state\":{\"mutations\":[],\"queries\":[{\"state\":\"$6:props:state:queries:0:state\",\"queryKey\":\"$6:props:state:queries:0:queryKey\",\"queryHash\":\"[\\\"my_communities\\\"]\"},{\"state\":\"$6:props:state:queries:1:state\",\"queryKey\":\"$6:props:state:queries:1:queryKey\",\"queryHash\":\"[\\\"user\\\"]\"},{\"state\":\"$6:props:state:queries:2:state\",\"queryKey\":\"$6:props:state:queries:2:queryKey\",\"queryHash\":\"[\\\"paper\\\",\\\"2303.13814\\\",\\\"metadata\\\"]\"},{\"state\":\"$6:props:state:queries:3:state\",\"queryKey\":\"$6:props:state:queries:3:queryKey\",\"queryHash\":\"[\\\"paper\\\",\\\"2303.13814\\\",\\\"comments\\\"]\"},{\"state\":{\"data\":\"Mozilla/4.0 (compatible; MSIE 7.0; Windows NT 6.0; SLCC1; .NET CLR 2.0.50727; .NET CLR 3.0.04506; .NET CLR 3.5.21022; .NET CLR 1.0.3705; .NET CLR 1.1.4322)\",\"dataUpdateCount\":77,\"dataUpdatedAt\":1744228241989,\"error\":null,\"errorUpdateCount\":0,\"errorUpdatedAt\":0,\"fetchFailureCount\":0,\"fetchFailureReason\":null,\"fetchMeta\":null,\"isInvalidated\":false,\"status\":\"success\",\"fetchStatus\":\"idle\"},\"queryKey\":\"$6:props:state:queries:4:queryKey\",\"queryHash\":\"[\\\"user-agent\\\"]\"},{\"state\":\"$6:props:state:queries:5:state\",\"queryKey\":\"$6:props:state:queries:5:queryKey\",\"queryHash\":\"[\\\"paper\\\",\\\"2303.13396\\\",\\\"metadata\\\"]\"},{\"state\":\"$6:props:state:queries:6:state\",\"queryKey\":\"$6:props:state:queries:6:queryKey\",\"queryHash\":\"[\\\"paper\\\",\\\"2303.13396\\\",\\\"comments\\\"]\"},{\"state\":\"$6:props:state:queries:7:state\",\"queryKey\":\"$6:props:state:queries:7:queryKey\",\"queryHash\":\"[\\\"paper\\\",\\\"2210.14955\\\",\\\"metadata\\\"]\"},{\"state\":\"$6:props:state:queries:8:state\",\"queryKey\":\"$6:props:state:queries:8:queryKey\",\"queryHash\":\"[\\\"paper\\\",\\\"2210.14955\\\",\\\"comments\\\"]\"},{\"state\":\"$6:props:state:queries:9:state\",\"queryKey\":\"$6:props:state:queries:9:queryKey\",\"queryHash\":\"[\\\"paper\\\",\\\"2303.13505\\\",\\\"metadata\\\"]\"},{\"state\":\"$6:props:state:queries:10:state\",\"queryKey\":\"$6:props:state:queries:10:queryKey\",\"queryHash\":\"[\\\"paper\\\",\\\"2303.13505\\\",\\\"comments\\\"]\"},{\"state\":\"$6:props:state:queries:11:state\",\"queryKey\":\"$6:props:state:queries:11:queryKey\",\"queryHash\":\"[\\\"paper\\\",\\\"2303.13502\\\",\\\"metadata\\\"]\"},{\"state\":\"$6:props:state:queries:12:state\",\"queryKey\":\"$6:props:state:queries:12:queryKey\",\"queryHash\":\"[\\\"paper\\\",\\\"2303.13502\\\",\\\"comments\\\"]\"},{\"state\":\"$6:props:state:queries:13:state\",\"queryKey\":\"$6:props:state:queries:13:queryKey\",\"queryHash\":\"[\\\"paper\\\",\\\"1801.06263\\\",\\\"metadata\\\"]\"},{\"state\":\"$6:props:state:queries:14:state\",\"queryKey\":\"$6:props:state:queries:14:queryKey\",\"queryHash\":\"[\\\"paper\\\",\\\"1801.06263\\\",\\\"comments\\\"]\"},{\"state\":\"$6:props:state:queries:15:state\",\"queryKey\":\"$6:props:state:queries:15:queryKey\",\"queryHash\":\"[\\\"paper\\\",\\\"hep-ph/9901208\\\",\\\"metadata\\\"]\"},{\"state\":\"$6:props:state:queries:16:state\",\"queryKey\":\"$6:props:state:queries:16:queryKey\",\"queryHash\":\"[\\\"paper\\\",\\\"hep-ph/9901208\\\",\\\"comments\\\"]\"},{\"state\":\"$6:props:state:queries:17:state\",\"queryKey\":\"$6:props:state:queries:17:queryKey\",\"queryHash\":\"[\\\"paper\\\",\\\"2303.13495\\\",\\\"metadata\\\"]\"},{\"state\":\"$6:props:state:queries:18:state\",\"queryKey\":\"$6:props:state:queries:18:queryKey\",\"queryHash\":\"[\\\"paper\\\",\\\"2303.13495\\\",\\\"comments\\\"]\"},{\"state\":\"$6:props:state:queries:19:state\",\"queryKey\":\"$6:props:state:queries:19:queryKey\",\"queryHash\":\"[\\\"paper\\\",\\\"2303.13491\\\",\\\"metadata\\\"]\"},{\"state\":\"$6:props:state:queries:20:state\",\"queryKey\":\"$6:props:state:queries:20:queryKey\",\"queryHash\":\"[\\\"paper\\\",\\\"2303.13491\\\",\\\"comments\\\"]\"},{\"state\":\"$6:props:state:queries:21:state\",\"queryKey\":\"$6:props:state:queries:21:queryKey\",\"queryHash\":\"[\\\"paper\\\",\\\"2303.13490\\\",\\\"metadata\\\"]\"},{\"state\":\"$6:props:state:queries:22:state\",\"queryKey\":\"$6:props:state:queries:22:queryKey\",\"queryHash\":\"[\\\"paper\\\",\\\"2303.13490\\\",\\\"comments\\\"]\"},{\"state\":\"$6:props:state:queries:23:state\",\"queryKey\":\"$6:props:state:queries:23:queryKey\",\"queryHash\":\"[\\\"paper\\\",\\\"2303.13471\\\",\\\"metadata\\\"]\"},{\"state\":\"$6:props:state:queries:24:state\",\"queryKey\":\"$6:props:state:queries:24:queryKey\",\"queryHash\":\"[\\\"paper\\\",\\\"2303.13471\\\",\\\"comments\\\"]\"},{\"state\":\"$6:props:state:queries:25:state\",\"queryKey\":\"$6:props:state:queries:25:queryKey\",\"queryHash\":\"[\\\"paper\\\",\\\"2303.13462\\\",\\\"metadata\\\"]\"},{\"state\":\"$6:props:state:queries:26:state\",\"queryKey\":\"$6:props:state:queries:26:queryKey\",\"queryHash\":\"[\\\"paper\\\",\\\"2303.13462\\\",\\\"comments\\\"]\"},{\"state\":\"$6:props:state:queries:27:state\",\"queryKey\":\"$6:props:state:queries:27:queryKey\",\"queryHash\":\"[\\\"paper\\\",\\\"2303.13450\\\",\\\"metadata\\\"]\"},{\"state\":\"$6:props:state:queries:28:state\",\"queryKey\":\"$6:props:state:queries:28:queryKey\",\"queryHash\":\"[\\\"paper\\\",\\\"2303.13450\\\",\\\"comments\\\"]\"},{\"state\":\"$6:props:state:queries:29:state\",\"queryKey\":\"$6:props:state:queries:29:queryKey\",\"queryHash\":\"[\\\"paper\\\",\\\"2303.13455\\\",\\\"metadata\\\"]\"},{\"state\":\"$6:props:state:queries:30:state\",\"queryKey\":\"$6:props:state:queries:30:queryKey\",\"queryHash\":\"[\\\"paper\\\",\\\"2303.13455\\\",\\\"comments\\\"]\"},{\"state\":\"$6:props:state:queries:31:state\",\"queryKey\":\"$6:props:state:queries:31:queryKey\",\"queryHash\":\"[\\\"paper\\\",\\\"2205.11912\\\",\\\"metadata\\\"]\"},{\"state\":\"$6:props:state:queries:32:state\",\"queryKey\":\"$6:props:state:queries:32:queryKey\",\"queryHash\":\"[\\\"paper\\\",\\\"2205.11912\\\",\\\"comments\\\"]\"},{\"state\":\"$6:props:state:queries:33:state\",\"queryKey\":\"$6:props:state:queries:33:queryKey\",\"queryHash\":\"[\\\"paper\\\",\\\"2303.13425\\\",\\\"metadata\\\"]\"},{\"state\":\"$6:props:state:queries:34:state\",\"queryKey\":\"$6:props:state:queries:34:queryKey\",\"queryHash\":\"[\\\"paper\\\",\\\"2303.13425\\\",\\\"comments\\\"]\"},{\"state\":\"$6:props:state:queries:35:state\",\"queryKey\":\"$6:props:state:queries:35:queryKey\",\"queryHash\":\"[\\\"paper\\\",\\\"2406.07987\\\",\\\"metadata\\\"]\"},{\"state\":\"$6:props:state:queries:36:state\",\"queryKey\":\"$6:props:state:queries:36:queryKey\",\"queryHash\":\"[\\\"paper\\\",\\\"2406.07987\\\",\\\"comments\\\"]\"},{\"state\":\"$6:props:state:queries:37:state\",\"queryKey\":\"$6:props:state:queries:37:queryKey\",\"queryHash\":\"[\\\"paper\\\",\\\"2209.09485\\\",\\\"metadata\\\"]\"},{\"state\":\"$6:props:state:queries:38:state\",\"queryKey\":\"$6:props:state:queries:38:queryKey\",\"queryHash\":\"[\\\"paper\\\",\\\"2209.09485\\\",\\\"comments\\\"]\"},{\"state\":\"$6:props:state:queries:39:state\",\"queryKey\":\"$6:props:state:queries:39:queryKey\",\"queryHash\":\"[\\\"paper\\\",\\\"2412.01851\\\",\\\"metadata\\\"]\"},{\"state\":\"$6:props:state:queries:40:state\",\"queryKey\":\"$6:props:state:queries:40:queryKey\",\"queryHash\":\"[\\\"paper\\\",\\\"2412.01851\\\",\\\"comments\\\"]\"},{\"state\":\"$6:props:state:queries:41:state\",\"queryKey\":\"$6:props:state:queries:41:queryKey\",\"queryHash\":\"[\\\"paper\\\",\\\"2303.13416\\\",\\\"metadata\\\"]\"},{\"state\":\"$6:props:state:queries:42:state\",\"queryKey\":\"$6:props:state:queries:42:queryKey\",\"queryHash\":\"[\\\"paper\\\",\\\"2303.13416\\\",\\\"comments\\\"]\"},{\"state\":\"$6:props:state:queries:43:state\",\"queryKey\":\"$6:props:state:queries:43:queryKey\",\"queryHash\":\"[\\\"paper\\\",\\\"2303.13417\\\",\\\"metadata\\\"]\"},{\"state\":\"$6:props:state:queries:44:state\",\"queryKey\":\"$6:props:state:queries:44:queryKey\",\"queryHash\":\"[\\\"paper\\\",\\\"2303.13417\\\",\\\"comments\\\"]\"},{\"state\":\"$6:props:state:queries:45:state\",\"queryKey\":\"$6:props:state:queries:45:queryKey\",\"queryHash\":\"[\\\"paper\\\",\\\"2304.14489\\\",\\\"metadata\\\"]\"},{\"state\":\"$6:props:state:queries:46:state\",\"queryKey\":\"$6:props:state:queries:46:queryKey\",\"queryHash\":\"[\\\"paper\\\",\\\"2304.14489\\\",\\\"comments\\\"]\"},{\"state\":\"$6:props:state:queries:47:state\",\"queryKey\":\"$6:props:state:queries:47:queryKey\",\"queryHash\":\"[\\\"paper\\\",\\\"2303.13571\\\",\\\"metadata\\\"]\"},{\"state\":\"$6:props:state:queries:48:state\",\"queryKey\":\"$6:props:state:queries:48:queryKey\",\"queryHash\":\"[\\\"paper\\\",\\\"2303.13571\\\",\\\"comments\\\"]\"},{\"state\":\"$6:props:state:queries:49:state\",\"queryKey\":\"$6:props:state:queries:49:queryKey\",\"queryHash\":\"[\\\"paper\\\",\\\"2205.15448\\\",\\\"metadata\\\"]\"},{\"state\":\"$6:props:state:queries:50:state\",\"queryKey\":\"$6:props:state:queries:50:queryKey\",\"queryHash\":\"[\\\"paper\\\",\\\"2205.15448\\\",\\\"comments\\\"]\"},{\"state\":\"$6:props:state:queries:51:state\",\"queryKey\":\"$6:props:state:queries:51:queryKey\",\"queryHash\":\"[\\\"paper\\\",\\\"2301.02933\\\",\\\"metadata\\\"]\"},{\"state\":\"$6:props:state:queries:52:state\",\"queryKey\":\"$6:props:state:queries:52:queryKey\",\"queryHash\":\"[\\\"paper\\\",\\\"2301.02933\\\",\\\"comments\\\"]\"},{\"state\":\"$6:props:state:queries:53:state\",\"queryKey\":\"$6:props:state:queries:53:queryKey\",\"queryHash\":\"[\\\"paper\\\",\\\"2210.14064\\\",\\\"metadata\\\"]\"},{\"state\":\"$6:props:state:queries:54:state\",\"queryKey\":\"$6:props:state:queries:54:queryKey\",\"queryHash\":\"[\\\"paper\\\",\\\"2210.14064\\\",\\\"comments\\\"]\"},{\"state\":\"$6:props:state:queries:55:state\",\"queryKey\":\"$6:props:state:queries:55:queryKey\",\"queryHash\":\"[\\\"paper\\\",\\\"2205.10747\\\",\\\"metadata\\\"]\"},{\"state\":\"$6:props:state:queries:56:state\",\"queryKey\":\"$6:props:state:queries:56:queryKey\",\"queryHash\":\"[\\\"paper\\\",\\\"2205.10747\\\",\\\"comments\\\"]\"},{\"state\":\"$6:props:state:queries:57:state\",\"queryKey\":\"$6:props:state:queries:57:queryKey\",\"queryHash\":\"[\\\"paper\\\",\\\"2502.09984\\\",\\\"metadata\\\"]\"},{\"state\":\"$6:props:state:queries:58:state\",\"queryKey\":\"$6:props:state:queries:58:queryKey\",\"queryHash\":\"[\\\"paper\\\",\\\"2502.09984\\\",\\\"comments\\\"]\"},{\"state\":\"$6:props:state:queries:59:state\",\"queryKey\":\"$6:props:state:queries:59:queryKey\",\"queryHash\":\"[\\\"paper\\\",\\\"1903.02925\\\",\\\"metadata\\\"]\"},{\"state\":\"$6:props:state:queries:60:state\",\"queryKey\":\"$6:props:state:queries:60:queryKey\",\"queryHash\":\"[\\\"paper\\\",\\\"1903.02925\\\",\\\"comments\\\"]\"},{\"state\":\"$6:props:state:queries:61:state\",\"queryKey\":\"$6:props:state:queries:61:queryKey\",\"queryHash\":\"[\\\"paper\\\",\\\"2012.03831\\\",\\\"metadata\\\"]\"},{\"state\":\"$6:props:state:queries:62:state\",\"queryKey\":\"$6:props:state:queries:62:queryKey\",\"queryHash\":\"[\\\"paper\\\",\\\"2012.03831\\\",\\\"comments\\\"]\"},{\"state\":\"$6:props:state:queries:63:state\",\"queryKey\":\"$6:props:state:queries:63:queryKey\",\"queryHash\":\"[\\\"paper\\\",\\\"2303.13319\\\",\\\"metadata\\\"]\"},{\"state\":\"$6:props:state:queries:64:state\",\"queryKey\":\"$6:props:state:queries:64:queryKey\",\"queryHash\":\"[\\\"paper\\\",\\\"2303.13319\\\",\\\"comments\\\"]\"},{\"state\":\"$6:props:state:queries:65:state\",\"queryKey\":\"$6:props:state:queries:65:queryKey\",\"queryHash\":\"[\\\"paper\\\",\\\"2411.07321\\\",\\\"metadata\\\"]\"},{\"state\":\"$6:props:state:queries:66:state\",\"queryKey\":\"$6:props:state:queries:66:queryKey\",\"queryHash\":\"[\\\"paper\\\",\\\"2411.07321\\\",\\\"comments\\\"]\"},{\"state\":\"$6:props:state:queries:67:state\",\"queryKey\":\"$6:props:state:queries:67:queryKey\",\"queryHash\":\"[\\\"paper\\\",\\\"2208.02541\\\",\\\"metadata\\\"]\"},{\"state\":\"$6:props:state:queries:68:state\",\"queryKey\":\"$6:props:state:queries:68:queryKey\",\"queryHash\":\"[\\\"paper\\\",\\\"2208.02541\\\",\\\"comments\\\"]\"},{\"state\":\"$6:props:state:queries:69:state\",\"queryKey\":\"$6:props:state:queries:69:queryKey\",\"queryHash\":\"[\\\"paper\\\",\\\"2102.11742\\\",\\\"metadata\\\"]\"},{\"state\":\"$6:props:state:queries:70:state\",\"queryKey\":\"$6:props:state:queries:70:queryKey\",\"queryHash\":\"[\\\"paper\\\",\\\"2102.11742\\\",\\\"comments\\\"]\"},{\"state\":\"$6:props:state:queries:71:state\",\"queryKey\":\"$6:props:state:queries:71:queryKey\",\"queryHash\":\"[\\\"paper\\\",\\\"2110.08991\\\",\\\"metadata\\\"]\"},{\"state\":\"$6:props:state:queries:72:state\",\"queryKey\":\"$6:props:state:queries:72:queryKey\",\"queryHash\":\"[\\\"paper\\\",\\\"2110.08991\\\",\\\"comments\\\"]\"},{\"state\":\"$6:props:state:queries:73:state\",\"queryKey\":\"$6:props:state:queries:73:queryKey\",\"queryHash\":\"[\\\"paper\\\",\\\"2302.13537\\\",\\\"metadata\\\"]\"},{\"state\":\"$6:props:state:queries:74:state\",\"queryKey\":\"$6:props:state:queries:74:queryKey\",\"queryHash\":\"[\\\"paper\\\",\\\"2302.13537\\\",\\\"comments\\\"]\"},{\"state\":\"$6:props:state:queries:75:state\",\"queryKey\":\"$6:props:state:queries:75:queryKey\",\"queryHash\":\"[\\\"paper\\\",\\\"2412.20556\\\",\\\"metadata\\\"]\"},{\"state\":\"$6:props:state:queries:76:state\",\"queryKey\":\"$6:props:state:queries:76:queryKey\",\"queryHash\":\"[\\\"paper\\\",\\\"2412.20556\\\",\\\"comments\\\"]\"},{\"state\":\"$6:props:state:queries:77:state\",\"queryKey\":\"$6:props:state:queries:77:queryKey\",\"queryHash\":\"[\\\"paper\\\",\\\"2404.04925\\\",\\\"metadata\\\"]\"},{\"state\":\"$6:props:state:queries:78:state\",\"queryKey\":\"$6:props:state:queries:78:queryKey\",\"queryHash\":\"[\\\"paper\\\",\\\"2404.04925\\\",\\\"comments\\\"]\"},{\"state\":\"$6:props:state:queries:79:state\",\"queryKey\":\"$6:props:state:queries:79:queryKey\",\"queryHash\":\"[\\\"paper\\\",\\\"2408.03111\\\",\\\"metadata\\\"]\"},{\"state\":\"$6:props:state:queries:80:state\",\"queryKey\":\"$6:props:state:queries:80:queryKey\",\"queryHash\":\"[\\\"paper\\\",\\\"2408.03111\\\",\\\"comments\\\"]\"},{\"state\":\"$6:props:state:queries:81:state\",\"queryKey\":\"$6:props:state:queries:81:queryKey\",\"queryHash\":\"[\\\"paper\\\",\\\"2412.21089\\\",\\\"metadata\\\"]\"},{\"state\":\"$6:props:state:queries:82:state\",\"queryKey\":\"$6:props:state:queries:82:queryKey\",\"queryHash\":\"[\\\"paper\\\",\\\"2412.21089\\\",\\\"comments\\\"]\"},{\"state\":\"$6:props:state:queries:83:state\",\"queryKey\":\"$6:props:state:queries:83:queryKey\",\"queryHash\":\"[\\\"paper\\\",\\\"2305.01779\\\",\\\"metadata\\\"]\"},{\"state\":\"$6:props:state:queries:84:state\",\"queryKey\":\"$6:props:state:queries:84:queryKey\",\"queryHash\":\"[\\\"paper\\\",\\\"2305.01779\\\",\\\"comments\\\"]\"},{\"state\":\"$6:props:state:queries:85:state\",\"queryKey\":\"$6:props:state:queries:85:queryKey\",\"queryHash\":\"[\\\"paper\\\",\\\"2502.13576\\\",\\\"metadata\\\"]\"},{\"state\":\"$6:props:state:queries:86:state\",\"queryKey\":\"$6:props:state:queries:86:queryKey\",\"queryHash\":\"[\\\"paper\\\",\\\"2502.13576\\\",\\\"comments\\\"]\"},{\"state\":\"$6:props:state:queries:87:state\",\"queryKey\":\"$6:props:state:queries:87:queryKey\",\"queryHash\":\"[\\\"paper\\\",\\\"2209.01552\\\",\\\"metadata\\\"]\"},{\"state\":\"$6:props:state:queries:88:state\",\"queryKey\":\"$6:props:state:queries:88:queryKey\",\"queryHash\":\"[\\\"paper\\\",\\\"2209.01552\\\",\\\"comments\\\"]\"},{\"state\":\"$6:props:state:queries:89:state\",\"queryKey\":\"$6:props:state:queries:89:queryKey\",\"queryHash\":\"[\\\"paper\\\",\\\"2208.07743\\\",\\\"metadata\\\"]\"},{\"state\":\"$6:props:state:queries:90:state\",\"queryKey\":\"$6:props:state:queries:90:queryKey\",\"queryHash\":\"[\\\"paper\\\",\\\"2208.07743\\\",\\\"comments\\\"]\"},{\"state\":\"$6:props:state:queries:91:state\",\"queryKey\":\"$6:props:state:queries:91:queryKey\",\"queryHash\":\"[\\\"paper\\\",\\\"2303.13216\\\",\\\"metadata\\\"]\"},{\"state\":\"$6:props:state:queries:92:state\",\"queryKey\":\"$6:props:state:queries:92:queryKey\",\"queryHash\":\"[\\\"paper\\\",\\\"2303.13216\\\",\\\"comments\\\"]\"},{\"state\":\"$6:props:state:queries:93:state\",\"queryKey\":\"$6:props:state:queries:93:queryKey\",\"queryHash\":\"[\\\"paper\\\",\\\"2301.12900\\\",\\\"metadata\\\"]\"},{\"state\":\"$6:props:state:queries:94:state\",\"queryKey\":\"$6:props:state:queries:94:queryKey\",\"queryHash\":\"[\\\"paper\\\",\\\"2301.12900\\\",\\\"comments\\\"]\"},{\"state\":\"$6:props:state:queries:95:state\",\"queryKey\":\"$6:props:state:queries:95:queryKey\",\"queryHash\":\"[\\\"paper\\\",\\\"2211.11595\\\",\\\"metadata\\\"]\"},{\"state\":\"$6:props:state:queries:96:state\",\"queryKey\":\"$6:props:state:queries:96:queryKey\",\"queryHash\":\"[\\\"paper\\\",\\\"2211.11595\\\",\\\"comments\\\"]\"},{\"state\":\"$6:props:state:queries:97:state\",\"queryKey\":\"$6:props:state:queries:97:queryKey\",\"queryHash\":\"[\\\"paper\\\",\\\"2303.13273\\\",\\\"metadata\\\"]\"},{\"state\":\"$6:props:state:queries:98:state\",\"queryKey\":\"$6:props:state:queries:98:queryKey\",\"queryHash\":\"[\\\"paper\\\",\\\"2303.13273\\\",\\\"comments\\\"]\"},{\"state\":\"$6:props:state:queries:99:state\",\"queryKey\":\"$6:props:state:queries:99:queryKey\",\"queryHash\":\"[\\\"paper\\\",\\\"2209.03188\\\",\\\"metadata\\\"]\"},{\"state\":\"$6:props:state:queries:100:state\",\"queryKey\":\"$6:props:state:queries:100:queryKey\",\"queryHash\":\"[\\\"paper\\\",\\\"2209.03188\\\",\\\"comments\\\"]\"},{\"state\":\"$6:props:state:queries:101:state\",\"queryKey\":\"$6:props:state:queries:101:queryKey\",\"queryHash\":\"[\\\"paper\\\",\\\"2303.13175\\\",\\\"metadata\\\"]\"},{\"state\":\"$6:props:state:queries:102:state\",\"queryKey\":\"$6:props:state:queries:102:queryKey\",\"queryHash\":\"[\\\"paper\\\",\\\"2303.13175\\\",\\\"comments\\\"]\"},{\"state\":\"$6:props:state:queries:103:state\",\"queryKey\":\"$6:props:state:queries:103:queryKey\",\"queryHash\":\"[\\\"paper\\\",\\\"2303.01247\\\",\\\"metadata\\\"]\"},{\"state\":\"$6:props:state:queries:104:state\",\"queryKey\":\"$6:props:state:queries:104:queryKey\",\"queryHash\":\"[\\\"paper\\\",\\\"2303.01247\\\",\\\"comments\\\"]\"},{\"state\":\"$6:props:state:queries:105:state\",\"queryKey\":\"$6:props:state:queries:105:queryKey\",\"queryHash\":\"[\\\"paper\\\",\\\"2303.13101\\\",\\\"metadata\\\"]\"},{\"state\":\"$6:props:state:queries:106:state\",\"queryKey\":\"$6:props:state:queries:106:queryKey\",\"queryHash\":\"[\\\"paper\\\",\\\"2303.13101\\\",\\\"comments\\\"]\"},{\"state\":\"$6:props:state:queries:107:state\",\"queryKey\":\"$6:props:state:queries:107:queryKey\",\"queryHash\":\"[\\\"paper\\\",\\\"2303.13117\\\",\\\"metadata\\\"]\"},{\"state\":\"$6:props:state:queries:108:state\",\"queryKey\":\"$6:props:state:queries:108:queryKey\",\"queryHash\":\"[\\\"paper\\\",\\\"2303.13117\\\",\\\"comments\\\"]\"},{\"state\":\"$6:props:state:queries:109:state\",\"queryKey\":\"$6:props:state:queries:109:queryKey\",\"queryHash\":\"[\\\"paper\\\",\\\"2403.06149\\\",\\\"metadata\\\"]\"},{\"state\":\"$6:props:state:queries:110:state\",\"queryKey\":\"$6:props:state:queries:110:queryKey\",\"queryHash\":\"[\\\"paper\\\",\\\"2403.06149\\\",\\\"comments\\\"]\"},{\"state\":\"$6:props:state:queries:111:state\",\"queryKey\":\"$6:props:state:queries:111:queryKey\",\"queryHash\":\"[\\\"paper\\\",\\\"2212.03441\\\",\\\"metadata\\\"]\"},{\"state\":\"$6:props:state:queries:112:state\",\"queryKey\":\"$6:props:state:queries:112:queryKey\",\"queryHash\":\"[\\\"paper\\\",\\\"2212.03441\\\",\\\"comments\\\"]\"},{\"state\":\"$6:props:state:queries:113:state\",\"queryKey\":\"$6:props:state:queries:113:queryKey\",\"queryHash\":\"[\\\"paper\\\",\\\"2404.16870\\\",\\\"metadata\\\"]\"},{\"state\":\"$6:props:state:queries:114:state\",\"queryKey\":\"$6:props:state:queries:114:queryKey\",\"queryHash\":\"[\\\"paper\\\",\\\"2404.16870\\\",\\\"comments\\\"]\"},{\"state\":\"$6:props:state:queries:115:state\",\"queryKey\":\"$6:props:state:queries:115:queryKey\",\"queryHash\":\"[\\\"paper\\\",\\\"2412.20834\\\",\\\"metadata\\\"]\"},{\"state\":\"$6:props:state:queries:116:state\",\"queryKey\":\"$6:props:state:queries:116:queryKey\",\"queryHash\":\"[\\\"paper\\\",\\\"2412.20834\\\",\\\"comments\\\"]\"},{\"state\":\"$6:props:state:queries:117:state\",\"queryKey\":\"$6:props:state:queries:117:queryKey\",\"queryHash\":\"[\\\"paper\\\",\\\"2006.06704\\\",\\\"metadata\\\"]\"},{\"state\":\"$6:props:state:queries:118:state\",\"queryKey\":\"$6:props:state:queries:118:queryKey\",\"queryHash\":\"[\\\"paper\\\",\\\"2006.06704\\\",\\\"comments\\\"]\"},{\"state\":\"$6:props:state:queries:119:state\",\"queryKey\":\"$6:props:state:queries:119:queryKey\",\"queryHash\":\"[\\\"paper\\\",\\\"2303.13076\\\",\\\"metadata\\\"]\"},{\"state\":\"$6:props:state:queries:120:state\",\"queryKey\":\"$6:props:state:queries:120:queryKey\",\"queryHash\":\"[\\\"paper\\\",\\\"2303.13076\\\",\\\"comments\\\"]\"},{\"state\":\"$6:props:state:queries:121:state\",\"queryKey\":\"$6:props:state:queries:121:queryKey\",\"queryHash\":\"[\\\"paper\\\",\\\"2306.11149\\\",\\\"metadata\\\"]\"},{\"state\":\"$6:props:state:queries:122:state\",\"queryKey\":\"$6:props:state:queries:122:queryKey\",\"queryHash\":\"[\\\"paper\\\",\\\"2306.11149\\\",\\\"comments\\\"]\"},{\"state\":\"$6:props:state:queries:123:state\",\"queryKey\":\"$6:props:state:queries:123:queryKey\",\"queryHash\":\"[\\\"paper\\\",\\\"2303.13035\\\",\\\"metadata\\\"]\"},{\"state\":\"$6:props:state:queries:124:state\",\"queryKey\":\"$6:props:state:queries:124:queryKey\",\"queryHash\":\"[\\\"paper\\\",\\\"2303.13035\\\",\\\"comments\\\"]\"},{\"state\":\"$6:props:state:queries:125:state\",\"queryKey\":\"$6:props:state:queries:125:queryKey\",\"queryHash\":\"[\\\"paper\\\",\\\"2303.16149\\\",\\\"metadata\\\"]\"},{\"state\":\"$6:props:state:queries:126:state\",\"queryKey\":\"$6:props:state:queries:126:queryKey\",\"queryHash\":\"[\\\"paper\\\",\\\"2303.16149\\\",\\\"comments\\\"]\"},{\"state\":\"$6:props:state:queries:127:state\",\"queryKey\":\"$6:props:state:queries:127:queryKey\",\"queryHash\":\"[\\\"paper\\\",\\\"2303.13029\\\",\\\"metadata\\\"]\"},{\"state\":\"$6:props:state:queries:128:state\",\"queryKey\":\"$6:props:state:queries:128:queryKey\",\"queryHash\":\"[\\\"paper\\\",\\\"2303.13029\\\",\\\"comments\\\"]\"},{\"state\":\"$6:props:state:queries:129:state\",\"queryKey\":\"$6:props:state:queries:129:queryKey\",\"queryHash\":\"[\\\"paper\\\",\\\"2209.01759\\\",\\\"metadata\\\"]\"},{\"state\":\"$6:props:state:queries:130:state\",\"queryKey\":\"$6:props:state:queries:130:queryKey\",\"queryHash\":\"[\\\"paper\\\",\\\"2209.01759\\\",\\\"comments\\\"]\"},{\"state\":\"$6:props:state:queries:131:state\",\"queryKey\":\"$6:props:state:queries:131:queryKey\",\"queryHash\":\"[\\\"paper\\\",\\\"2303.13027\\\",\\\"metadata\\\"]\"},{\"state\":\"$6:props:state:queries:132:state\",\"queryKey\":\"$6:props:state:queries:132:queryKey\",\"queryHash\":\"[\\\"paper\\\",\\\"2303.13027\\\",\\\"comments\\\"]\"},{\"state\":\"$6:props:state:queries:133:state\",\"queryKey\":\"$6:props:state:queries:133:queryKey\",\"queryHash\":\"[\\\"paper\\\",\\\"2303.13026\\\",\\\"metadata\\\"]\"},{\"state\":\"$6:props:state:queries:134:state\",\"queryKey\":\"$6:props:state:queries:134:queryKey\",\"queryHash\":\"[\\\"paper\\\",\\\"2303.13026\\\",\\\"comments\\\"]\"},{\"state\":\"$6:props:state:queries:135:state\",\"queryKey\":\"$6:props:state:queries:135:queryKey\",\"queryHash\":\"[\\\"paper\\\",\\\"2303.13034\\\",\\\"metadata\\\"]\"},{\"state\":\"$6:props:state:queries:136:state\",\"queryKey\":\"$6:props:state:queries:136:queryKey\",\"queryHash\":\"[\\\"paper\\\",\\\"2303.13034\\\",\\\"comments\\\"]\"},{\"state\":\"$6:props:state:queries:137:state\",\"queryKey\":\"$6:props:state:queries:137:queryKey\",\"queryHash\":\"[\\\"paper\\\",\\\"2502.13650\\\",\\\"metadata\\\"]\"},{\"state\":\"$6:props:state:queries:138:state\",\"queryKey\":\"$6:props:state:queries:138:queryKey\",\"queryHash\":\"[\\\"paper\\\",\\\"2502.13650\\\",\\\"comments\\\"]\"},{\"state\":\"$6:props:state:queries:139:state\",\"queryKey\":\"$6:props:state:queries:139:queryKey\",\"queryHash\":\"[\\\"paper\\\",\\\"2409.17322\\\",\\\"metadata\\\"]\"},{\"state\":\"$6:props:state:queries:140:state\",\"queryKey\":\"$6:props:state:queries:140:queryKey\",\"queryHash\":\"[\\\"paper\\\",\\\"2409.17322\\\",\\\"comments\\\"]\"},{\"state\":\"$6:props:state:queries:141:state\",\"queryKey\":\"$6:props:state:queries:141:queryKey\",\"queryHash\":\"[\\\"paper\\\",\\\"1704.02553\\\",\\\"metadata\\\"]\"},{\"state\":\"$6:props:state:queries:142:state\",\"queryKey\":\"$6:props:state:queries:142:queryKey\",\"queryHash\":\"[\\\"paper\\\",\\\"1704.02553\\\",\\\"comments\\\"]\"},{\"state\":\"$6:props:state:queries:143:state\",\"queryKey\":\"$6:props:state:queries:143:queryKey\",\"queryHash\":\"[\\\"paper\\\",\\\"2411.01808\\\",\\\"metadata\\\"]\"},{\"state\":\"$6:props:state:queries:144:state\",\"queryKey\":\"$6:props:state:queries:144:queryKey\",\"queryHash\":\"[\\\"paper\\\",\\\"2411.01808\\\",\\\"comments\\\"]\"},{\"state\":{\"data\":{\"data\":{\"paper_version\":{\"_id\":\"67d3840793513844c2f69c12\",\"paper_group_id\":\"67d3840793513844c2f69c11\",\"version_label\":\"v1\",\"version_order\":1,\"title\":\"Transformers without Normalization\",\"abstract\":\"$8b\",\"author_ids\":[\"67322f44cd1e32a6e7f0a500\",\"672bbe60986a1370676d5732\",\"672bbcf6986a1370676d513f\",\"672bbd99986a1370676d53d0\",\"672bbf22986a1370676d59f4\"],\"publication_date\":\"2025-03-13T17:59:06.000Z\",\"license\":\"http://arxiv.org/licenses/nonexclusive-distrib/1.0/\",\"created_at\":\"2025-03-14T01:19:03.761Z\",\"updated_at\":\"2025-03-14T01:19:03.761Z\",\"is_deleted\":false,\"is_hidden\":false,\"universal_paper_id\":\"2503.10622\",\"imageURL\":\"image/2503.10622v1.png\"},\"paper_group\":{\"_id\":\"67d3840793513844c2f69c11\",\"universal_paper_id\":\"2503.10622\",\"title\":\"Transformers without Normalization\",\"created_at\":\"2025-03-14T01:19:03.080Z\",\"updated_at\":\"2025-03-14T01:19:03.080Z\",\"categories\":[\"Computer Science\"],\"subcategories\":[\"cs.LG\",\"cs.AI\",\"cs.CL\",\"cs.CV\"],\"author_user_ids\":[],\"source\":{\"name\":\"alphaXiv\",\"url\":\"https://arxiv.org/abs/2503.10622\"},\"metrics\":{\"activity_rank\":0,\"questions_count\":0,\"responses_count\":0,\"upvotes_count\":0,\"downvotes_count\":0,\"total_votes\":69,\"public_total_votes\":1580,\"visits_count\":{\"last24Hours\":357,\"last7Days\":2218,\"last30Days\":67738,\"last90Days\":67738,\"all\":203215},\"timeline\":[{\"date\":\"2025-03-31T14:00:01.489Z\",\"views\":2427},{\"date\":\"2025-03-28T02:00:01.489Z\",\"views\":7536},{\"date\":\"2025-03-24T14:00:01.489Z\",\"views\":13297},{\"date\":\"2025-03-21T02:00:01.489Z\",\"views\":42914},{\"date\":\"2025-03-17T14:00:01.489Z\",\"views\":67995},{\"date\":\"2025-03-14T02:00:01.489Z\",\"views\":66176},{\"date\":\"2025-03-10T14:00:01.489Z\",\"views\":4},{\"date\":\"2025-03-07T02:00:01.513Z\",\"views\":0},{\"date\":\"2025-03-03T14:00:01.536Z\",\"views\":2},{\"date\":\"2025-02-28T02:00:01.562Z\",\"views\":2},{\"date\":\"2025-02-24T14:00:01.585Z\",\"views\":1},{\"date\":\"2025-02-21T02:00:01.609Z\",\"views\":0},{\"date\":\"2025-02-17T14:00:01.632Z\",\"views\":1},{\"date\":\"2025-02-14T02:00:01.654Z\",\"views\":0},{\"date\":\"2025-02-10T14:00:01.676Z\",\"views\":0},{\"date\":\"2025-02-07T02:00:01.699Z\",\"views\":0},{\"date\":\"2025-02-03T14:00:01.722Z\",\"views\":0},{\"date\":\"2025-01-31T02:00:01.745Z\",\"views\":1},{\"date\":\"2025-01-27T14:00:02.255Z\",\"views\":0},{\"date\":\"2025-01-24T02:00:02.405Z\",\"views\":1},{\"date\":\"2025-01-20T14:00:02.439Z\",\"views\":2},{\"date\":\"2025-01-17T02:00:02.473Z\",\"views\":0},{\"date\":\"2025-01-13T14:00:02.499Z\",\"views\":1},{\"date\":\"2025-01-10T02:00:02.525Z\",\"views\":1},{\"date\":\"2025-01-06T14:00:02.578Z\",\"views\":1},{\"date\":\"2025-01-03T02:00:02.601Z\",\"views\":2},{\"date\":\"2024-12-30T14:00:02.709Z\",\"views\":2},{\"date\":\"2024-12-27T02:00:02.732Z\",\"views\":0},{\"date\":\"2024-12-23T14:00:02.754Z\",\"views\":1},{\"date\":\"2024-12-20T02:00:02.777Z\",\"views\":0},{\"date\":\"2024-12-16T14:00:02.799Z\",\"views\":1},{\"date\":\"2024-12-13T02:00:02.821Z\",\"views\":1},{\"date\":\"2024-12-09T14:00:02.843Z\",\"views\":1},{\"date\":\"2024-12-06T02:00:02.866Z\",\"views\":1},{\"date\":\"2024-12-02T14:00:02.891Z\",\"views\":1},{\"date\":\"2024-11-29T02:00:02.913Z\",\"views\":0},{\"date\":\"2024-11-25T14:00:02.936Z\",\"views\":0},{\"date\":\"2024-11-22T02:00:02.958Z\",\"views\":0},{\"date\":\"2024-11-18T14:00:02.981Z\",\"views\":2},{\"date\":\"2024-11-15T02:00:03.005Z\",\"views\":2},{\"date\":\"2024-11-11T14:00:03.027Z\",\"views\":1},{\"date\":\"2024-11-08T02:00:03.049Z\",\"views\":1},{\"date\":\"2024-11-04T14:00:03.071Z\",\"views\":0},{\"date\":\"2024-11-01T02:00:03.094Z\",\"views\":2},{\"date\":\"2024-10-28T14:00:03.117Z\",\"views\":0},{\"date\":\"2024-10-25T02:00:03.139Z\",\"views\":0},{\"date\":\"2024-10-21T14:00:03.162Z\",\"views\":1},{\"date\":\"2024-10-18T02:00:03.364Z\",\"views\":0},{\"date\":\"2024-10-14T14:00:03.387Z\",\"views\":0},{\"date\":\"2024-10-11T02:00:03.413Z\",\"views\":2},{\"date\":\"2024-10-07T14:00:03.435Z\",\"views\":2},{\"date\":\"2024-10-04T02:00:03.457Z\",\"views\":0},{\"date\":\"2024-09-30T14:00:03.481Z\",\"views\":1},{\"date\":\"2024-09-27T02:00:03.503Z\",\"views\":0},{\"date\":\"2024-09-23T14:00:03.526Z\",\"views\":1},{\"date\":\"2024-09-20T02:00:03.548Z\",\"views\":2},{\"date\":\"2024-09-16T14:00:03.570Z\",\"views\":2},{\"date\":\"2024-09-13T02:00:03.592Z\",\"views\":1}],\"weighted_visits\":{\"last24Hours\":0.08546401402303275,\"last7Days\":674.0502376869846,\"last30Days\":67738,\"last90Days\":67738,\"hot\":674.0502376869846}},\"is_hidden\":false,\"first_publication_date\":\"2025-03-13T17:59:06.000Z\",\"organizations\":[\"67be6377aa92218ccd8b1008\",\"67be6376aa92218ccd8b0f98\",\"67be637aaa92218ccd8b1158\",\"67be6379aa92218ccd8b10c6\"],\"detailedReport\":\"$8c\",\"paperSummary\":{\"summary\":\"Researchers from Meta FAIR, NYU, MIT, and Princeton demonstrate that Transformer models can achieve equal or better performance without normalization layers by introducing Dynamic Tanh (DyT), a simple learnable activation function that reduces computation time while maintaining model stability across vision, diffusion, and language tasks.\",\"originalProblem\":[\"Normalization layers like Layer Normalization are considered essential for training Transformers but add computational overhead\",\"Previous attempts to remove normalization layers often required complex architectural changes or showed limited success across different domains\"],\"solution\":[\"Replace normalization layers with Dynamic Tanh (DyT), defined as tanh(αx) where α is learnable\",\"Directly substitute DyT for normalization layers without changing model architecture or training protocols\"],\"keyInsights\":[\"Trained normalization layers exhibit tanh-like behavior, which DyT explicitly models\",\"The learnable scale parameter α automatically adapts to approximate 1/std of input activations\",\"The tanh function is crucial for stability while the learnable scale enables performance\"],\"results\":[\"DyT matches or exceeds performance of normalized Transformers across vision, diffusion, and language tasks\",\"Reduces computation time compared to RMSNorm in both training and inference\",\"Outperforms other normalization-free methods like Fixup and SkipInit\",\"Shows some sensitivity to α initialization in large language models\"]},\"resources\":{\"github\":{\"url\":\"https://github.com/jiachenzhu/DyT\",\"description\":\"Code release for DynamicTanh (DyT)\",\"language\":\"Python\",\"stars\":166}},\"citation\":{\"bibtex\":\"@Inproceedings{Zhu2025TransformersWN,\\n author = {Jiachen Zhu and Xinlei Chen and Kaiming He and Yann LeCun and Zhuang Liu},\\n title = {Transformers without Normalization},\\n year = {2025}\\n}\\n\"},\"custom_categories\":[\"attention-mechanisms\",\"transformers\",\"representation-learning\",\"self-supervised-learning\",\"optimization-methods\",\"parameter-efficient-training\"],\"overview\":{\"created_at\":\"2025-03-19T18:55:22.695Z\",\"text\":\"$8d\",\"translations\":{\"fr\":{\"text\":\"$8e\",\"created_at\":\"2025-03-27T21:50:07.014Z\"},\"ja\":{\"text\":\"$8f\",\"created_at\":\"2025-03-27T21:51:41.070Z\"},\"de\":{\"text\":\"$90\",\"created_at\":\"2025-03-27T21:51:48.028Z\"},\"ru\":{\"text\":\"$91\",\"created_at\":\"2025-03-27T21:52:29.014Z\"},\"zh\":{\"text\":\"$92\",\"created_at\":\"2025-03-27T21:55:22.083Z\"},\"ko\":{\"text\":\"$93\",\"created_at\":\"2025-03-27T22:13:29.928Z\"},\"es\":{\"text\":\"$94\",\"created_at\":\"2025-03-27T22:13:34.107Z\"},\"hi\":{\"text\":\"$95\",\"created_at\":\"2025-03-27T22:14:04.065Z\"}}},\"paperVersions\":{\"_id\":\"67d3840793513844c2f69c12\",\"paper_group_id\":\"67d3840793513844c2f69c11\",\"version_label\":\"v1\",\"version_order\":1,\"title\":\"Transformers without Normalization\",\"abstract\":\"$96\",\"author_ids\":[\"67322f44cd1e32a6e7f0a500\",\"672bbe60986a1370676d5732\",\"672bbcf6986a1370676d513f\",\"672bbd99986a1370676d53d0\",\"672bbf22986a1370676d59f4\"],\"publication_date\":\"2025-03-13T17:59:06.000Z\",\"license\":\"http://arxiv.org/licenses/nonexclusive-distrib/1.0/\",\"created_at\":\"2025-03-14T01:19:03.761Z\",\"updated_at\":\"2025-03-14T01:19:03.761Z\",\"is_deleted\":false,\"is_hidden\":false,\"universal_paper_id\":\"2503.10622\",\"imageURL\":\"image/2503.10622v1.png\"},\"verifiedAuthors\":[],\"authors\":[{\"_id\":\"672bbcf6986a1370676d513f\",\"full_name\":\"Kaiming He\",\"affiliation\":null,\"orcid\":null,\"semantic_scholarid\":null,\"user_id\":null},{\"_id\":\"672bbd99986a1370676d53d0\",\"full_name\":\"Yann LeCun\",\"affiliation\":null,\"orcid\":null,\"semantic_scholarid\":null,\"user_id\":null},{\"_id\":\"672bbe60986a1370676d5732\",\"full_name\":\"Xinlei Chen\",\"affiliation\":null,\"orcid\":null,\"semantic_scholarid\":null,\"user_id\":null},{\"_id\":\"672bbf22986a1370676d59f4\",\"full_name\":\"Zhuang Liu\",\"affiliation\":null,\"orcid\":null,\"semantic_scholarid\":null,\"user_id\":null},{\"_id\":\"67322f44cd1e32a6e7f0a500\",\"full_name\":\"Jiachen Zhu\",\"affiliation\":null,\"orcid\":null,\"semantic_scholarid\":null,\"user_id\":null}]},\"max_version_order\":1,\"verified_authors\":[],\"authors\":[{\"_id\":\"672bbcf6986a1370676d513f\",\"full_name\":\"Kaiming He\",\"affiliation\":null,\"orcid\":null,\"semantic_scholarid\":null,\"user_id\":null},{\"_id\":\"672bbd99986a1370676d53d0\",\"full_name\":\"Yann LeCun\",\"affiliation\":null,\"orcid\":null,\"semantic_scholarid\":null,\"user_id\":null},{\"_id\":\"672bbe60986a1370676d5732\",\"full_name\":\"Xinlei Chen\",\"affiliation\":null,\"orcid\":null,\"semantic_scholarid\":null,\"user_id\":null},{\"_id\":\"672bbf22986a1370676d59f4\",\"full_name\":\"Zhuang Liu\",\"affiliation\":null,\"orcid\":null,\"semantic_scholarid\":null,\"user_id\":null},{\"_id\":\"67322f44cd1e32a6e7f0a500\",\"full_name\":\"Jiachen Zhu\",\"affiliation\":null,\"orcid\":null,\"semantic_scholarid\":null,\"user_id\":null}],\"pdf_info\":{\"fetcher_url\":\"https://fetcher.alphaxiv.org/v2/pdf/2503.10622v1\"}}},\"dataUpdateCount\":1,\"dataUpdatedAt\":1744228241992,\"error\":null,\"errorUpdateCount\":0,\"errorUpdatedAt\":0,\"fetchFailureCount\":0,\"fetchFailureReason\":null,\"fetchMeta\":null,\"isInvalidated\":false,\"status\":\"success\",\"fetchStatus\":\"idle\"},\"queryKey\":[\"paper\",\"2503.10622\",\"metadata\"],\"queryHash\":\"[\\\"paper\\\",\\\"2503.10622\\\",\\\"metadata\\\"]\"},{\"state\":{\"data\":{\"data\":[{\"_id\":\"67dacc63682dc31851f8cc2d\",\"user_id\":\"674da0f7e57dd4be770d8437\",\"username\":\"Facundo Quiroga\",\"institution\":null,\"orcid_id\":\"\",\"gscholar_id\":\"kGvlEYAAAAAJ\",\"reputation\":17,\"is_author\":false,\"author_responded\":false,\"title\":\"Comment\",\"body\":\"\u003cp\u003eShould be α\u003c/p\u003e\",\"date\":\"2025-03-19T13:53:39.469Z\",\"responses\":[],\"annotation\":{\"type\":\"highlight\",\"highlightRects\":[{\"pageIndex\":4,\"rects\":[{\"x1\":429.28729940362206,\"y1\":441.2918839634941,\"x2\":457.5247065856217,\"y2\":450.86685136896995}]}],\"anchorPosition\":{\"pageIndex\":4,\"spanIndex\":93,\"offset\":14},\"focusPosition\":{\"pageIndex\":4,\"spanIndex\":93,\"offset\":20},\"selectedText\":\"alpha \"},\"tag\":\"general\",\"upvotes\":2,\"has_upvoted\":false,\"has_downvoted\":false,\"has_flagged\":false,\"edit_history\":[],\"paper_id\":\"2503.10622v1\",\"moderation\":{\"is_addressed\":true,\"is_closed\":true,\"is_flag_addressed\":false},\"paper_group_id\":\"67d3840793513844c2f69c11\",\"paper_version_id\":\"67d3840793513844c2f69c12\",\"endorsements\":[]},{\"_id\":\"67d74f93102025d39caee3d9\",\"user_id\":\"67d07c50bf20247b75453059\",\"username\":\"ZEN PUNK\",\"institution\":null,\"orcid_id\":\"\",\"gscholar_id\":\"\",\"reputation\":1,\"is_author\":false,\"author_responded\":false,\"title\":\"Podcast for paper\",\"body\":\"\u003cp\u003eAwesome work!\u003c/p\u003e\u003cp\u003e\u003cbr\u003e\u003ca target=\\\"_blank\\\" rel=\\\"noopener noreferrer nofollow\\\" href=\\\"https://open.spotify.com/episode/25KuT75TD1lVR6TJn3H11F?si=wC7aneiFRWqyjt8o71-2xw\\\"\u003eTransformers without Normalization podcast\u003c/a\u003e\u003cbr\u003e\u003c/p\u003e\",\"date\":\"2025-03-16T22:24:19.304Z\",\"responses\":[],\"annotation\":null,\"tag\":\"general\",\"upvotes\":1,\"has_upvoted\":false,\"has_downvoted\":false,\"has_flagged\":false,\"edit_history\":[{\"date\":\"2025-03-16T22:24:37.720Z\",\"body\":\"\u003cp\u003e\u003ca target=\\\"_blank\\\" href=\\\"https://open.spotify.com/episode/25KuT75TD1lVR6TJn3H11F?si=wC7aneiFRWqyjt8o71-2xw\\\"\u003eTransformers without Normalization podcast\u003c/a\u003e\u003cbr /\u003e \u003c/p\u003e\"}],\"paper_id\":\"2503.10622v1\",\"moderation\":{\"is_addressed\":true,\"is_closed\":true,\"is_flag_addressed\":false},\"paper_group_id\":\"67d3840793513844c2f69c11\",\"paper_version_id\":\"67d3840793513844c2f69c12\",\"endorsements\":[]}]},\"dataUpdateCount\":1,\"dataUpdatedAt\":1744228241990,\"error\":null,\"errorUpdateCount\":0,\"errorUpdatedAt\":0,\"fetchFailureCount\":0,\"fetchFailureReason\":null,\"fetchMeta\":null,\"isInvalidated\":false,\"status\":\"success\",\"fetchStatus\":\"idle\"},\"queryKey\":[\"paper\",\"2503.10622\",\"comments\"],\"queryHash\":\"[\\\"paper\\\",\\\"2503.10622\\\",\\\"comments\\\"]\"}]},\"data-sentry-element\":\"Hydrate\",\"data-sentry-component\":\"Layout\",\"data-sentry-source-file\":\"layout.tsx\",\"children\":[[\"$\",\"$L97\",null,{\"paperId\":\"2503.10622\",\"data-sentry-element\":\"UpdateGlobalPaperId\",\"data-sentry-source-file\":\"layout.tsx\"}],\"$L98\",[\"$\",\"$L99\",null,{\"data-sentry-element\":\"TopNavigation\",\"data-sentry-source-file\":\"layout.tsx\"}],[\"$\",\"$L9a\",null,{\"isMobileServer\":false,\"data-sentry-element\":\"CommentsProvider\",\"data-sentry-source-file\":\"layout.tsx\",\"children\":[\"$\",\"$L7\",null,{\"parallelRouterKey\":\"children\",\"segmentPath\":[\"children\",\"(paper)\",\"children\",\"$0:f:0:1:2:children:2:children:0\",\"children\"],\"error\":\"$undefined\",\"errorStyles\":\"$undefined\",\"errorScripts\":\"$undefined\",\"template\":[\"$\",\"$L8\",null,{}],\"templateStyles\":\"$undefined\",\"templateScripts\":\"$undefined\",\"notFound\":\"$undefined\",\"forbidden\":\"$undefined\",\"unauthorized\":\"$undefined\"}]}]]}]\n"])</script><script>self.__next_f.push([1,"9b:T96c,"])</script><script>self.__next_f.push([1,"{\"@context\":\"https://schema.org\",\"@type\":\"ScholarlyArticle\",\"headline\":\"Transformers without Normalization\",\"abstract\":\"Normalization layers are ubiquitous in modern neural networks and have long\\nbeen considered essential. This work demonstrates that Transformers without\\nnormalization can achieve the same or better performance using a remarkably\\nsimple technique. We introduce Dynamic Tanh (DyT), an element-wise operation\\n$DyT($x$) = \\\\tanh(\\\\alpha $x$)$, as a drop-in replacement for normalization\\nlayers in Transformers. DyT is inspired by the observation that layer\\nnormalization in Transformers often produces tanh-like, $S$-shaped input-output\\nmappings. By incorporating DyT, Transformers without normalization can match or\\nexceed the performance of their normalized counterparts, mostly without\\nhyperparameter tuning. We validate the effectiveness of Transformers with DyT\\nacross diverse settings, ranging from recognition to generation, supervised to\\nself-supervised learning, and computer vision to language models. These\\nfindings challenge the conventional understanding that normalization layers are\\nindispensable in modern neural networks, and offer new insights into their role\\nin deep networks.\",\"author\":[{\"@type\":\"Person\",\"name\":\"Kaiming He\"},{\"@type\":\"Person\",\"name\":\"Yann LeCun\"},{\"@type\":\"Person\",\"name\":\"Xinlei Chen\"},{\"@type\":\"Person\",\"name\":\"Zhuang Liu\"},{\"@type\":\"Person\",\"name\":\"Jiachen Zhu\"}],\"datePublished\":\"2025-03-13T17:59:06.000Z\",\"url\":\"https://www.alphaxiv.org/abs/67d3840793513844c2f69c11\",\"citation\":{\"@type\":\"CreativeWork\",\"identifier\":\"67d3840793513844c2f69c11\"},\"publisher\":{\"@type\":\"Organization\",\"name\":\"arXiv\"},\"discussionUrl\":\"https://www.alphaxiv.org/abs/67d3840793513844c2f69c11\",\"interactionStatistic\":[{\"@type\":\"InteractionCounter\",\"interactionType\":{\"@type\":\"ViewAction\",\"url\":\"https://schema.org/ViewAction\"},\"userInteractionCount\":203215},{\"@type\":\"InteractionCounter\",\"interactionType\":{\"@type\":\"LikeAction\",\"url\":\"https://schema.org/LikeAction\"},\"userInteractionCount\":1580}],\"commentCount\":2,\"comment\":[{\"@type\":\"Comment\",\"text\":\"Should be α\",\"dateCreated\":\"2025-03-19T13:53:39.469Z\",\"author\":{\"@type\":\"Person\",\"name\":\"Facundo Quiroga\"},\"upvoteCount\":2},{\"@type\":\"Comment\",\"text\":\"Awesome work!Transformers without Normalization podcast\",\"dateCreated\":\"2025-03-16T22:24:19.304Z\",\"author\":{\"@type\":\"Person\",\"name\":\"ZEN PUNK\"},\"upvoteCount\":1}]}"])</script><script>self.__next_f.push([1,"98:[\"$\",\"script\",null,{\"data-alphaxiv-id\":\"json-ld-paper-detail-view\",\"type\":\"application/ld+json\",\"dangerouslySetInnerHTML\":{\"__html\":\"$9b\"}}]\n"])</script></body></html>