CINXE.COM
Learn Milvus: Insights and Innovations in VectorDB Technology
<!DOCTYPE html><html lang="en"><head><meta charSet="utf-8"/><meta name="viewport" content="width=device-width"/><link rel="shortcut icon" href="/favicon.ico"/><link rel="icon" type="image/png" sizes="32x32" href="/favicon-32x32.png"/><meta name="image" property="og:image" content="https://assets.zilliz.com/meta_image_milvus_d6510e10e0.png"/><meta name="baidu-site-verification" content="codeva-bAvzh4ipX4"/><meta property="og:type" content="WebSite"/><meta name="keywords" content="milvus, vector database, milvus docs, milvus blogs"/><link rel="alternate" hrefLang="en" href="https://milvus.io/blog"/><title>Learn Milvus: Insights and Innovations in VectorDB Technology</title><meta name="description" content="Learn vector database fundamentals, Milvus features and capabilities, and technical tutorials on managing and optimizing vector search for modern AI apps."/><meta property="og:title" content="Learn Milvus: Insights and Innovations in VectorDB Technology"/><meta property="og:description" content="Learn vector database fundamentals, Milvus features and capabilities, and technical tutorials on managing and optimizing vector search for modern AI apps."/><meta property="og:url" content="https://milvus.io/blog"/><meta name="next-head-count" content="14"/><link rel="preconnect" href="https://fonts.googleapis.com"/><link rel="preconnect" href="https://fonts.gstatic.com"/><link rel="stylesheet" href="https://assets.zilliz.com/katex/katex.min.css"/><link rel="preconnect" href="https://fonts.gstatic.com" crossorigin /><link rel="preload" href="/_next/static/css/8c86470971238028.css" as="style"/><link rel="stylesheet" href="/_next/static/css/8c86470971238028.css" data-n-g=""/><link rel="preload" href="/_next/static/css/50cf83e36fd3c216.css" as="style"/><link rel="stylesheet" href="/_next/static/css/50cf83e36fd3c216.css" data-n-p=""/><link rel="preload" href="/_next/static/css/1267035f64123bee.css" as="style"/><link rel="stylesheet" href="/_next/static/css/1267035f64123bee.css" data-n-p=""/><link rel="preload" href="/_next/static/css/ae897f5b961640d8.css" as="style"/><link rel="stylesheet" href="/_next/static/css/ae897f5b961640d8.css" data-n-p=""/><noscript data-n-css=""></noscript><script defer="" nomodule="" src="/_next/static/chunks/polyfills-42372ed130431b0a.js"></script><script src="/_next/static/chunks/webpack-281ec69796c37d31.js" defer=""></script><script src="/_next/static/chunks/framework-b742ca6262bf02b6.js" defer=""></script><script src="/_next/static/chunks/main-e1ca4ae0b7d8e36e.js" defer=""></script><script src="/_next/static/chunks/pages/_app-b0a3eb5d837cd1db.js" defer=""></script><script src="/_next/static/chunks/4186-23042655333586c9.js" defer=""></script><script src="/_next/static/chunks/8418-dbef9aba6604b9fb.js" defer=""></script><script src="/_next/static/chunks/2411-c58b752e81c55f68.js" defer=""></script><script src="/_next/static/chunks/pages/blog-b3e8ac29104b7ae5.js" defer=""></script><script src="/_next/static/SWuVyeBuFzTkSMeqLwUio/_buildManifest.js" defer=""></script><script src="/_next/static/SWuVyeBuFzTkSMeqLwUio/_ssgManifest.js" defer=""></script><style data-href="https://fonts.googleapis.com/css2?family=Geist:wght@400;500;600;700&display=swap">@font-face{font-family:'Geist';font-style:normal;font-weight:400;font-display:swap;src:url(https://fonts.gstatic.com/s/geist/v1/gyBhhwUxId8gMGYQMKR3pzfaWI_RnOM4ng.woff) format('woff')}@font-face{font-family:'Geist';font-style:normal;font-weight:500;font-display:swap;src:url(https://fonts.gstatic.com/s/geist/v1/gyBhhwUxId8gMGYQMKR3pzfaWI_RruM4ng.woff) format('woff')}@font-face{font-family:'Geist';font-style:normal;font-weight:600;font-display:swap;src:url(https://fonts.gstatic.com/s/geist/v1/gyBhhwUxId8gMGYQMKR3pzfaWI_RQuQ4ng.woff) format('woff')}@font-face{font-family:'Geist';font-style:normal;font-weight:700;font-display:swap;src:url(https://fonts.gstatic.com/s/geist/v1/gyBhhwUxId8gMGYQMKR3pzfaWI_Re-Q4ng.woff) format('woff')}@font-face{font-family:'Geist';font-style:normal;font-weight:400;font-display:swap;src:url(https://fonts.gstatic.com/s/geist/v1/gyByhwUxId8gMEwSGFWNOITddY4.woff2) format('woff2');unicode-range:U+0100-02BA,U+02BD-02C5,U+02C7-02CC,U+02CE-02D7,U+02DD-02FF,U+0304,U+0308,U+0329,U+1D00-1DBF,U+1E00-1E9F,U+1EF2-1EFF,U+2020,U+20A0-20AB,U+20AD-20C0,U+2113,U+2C60-2C7F,U+A720-A7FF}@font-face{font-family:'Geist';font-style:normal;font-weight:400;font-display:swap;src:url(https://fonts.gstatic.com/s/geist/v1/gyByhwUxId8gMEwcGFWNOITd.woff2) format('woff2');unicode-range:U+0000-00FF,U+0131,U+0152-0153,U+02BB-02BC,U+02C6,U+02DA,U+02DC,U+0304,U+0308,U+0329,U+2000-206F,U+20AC,U+2122,U+2191,U+2193,U+2212,U+2215,U+FEFF,U+FFFD}@font-face{font-family:'Geist';font-style:normal;font-weight:500;font-display:swap;src:url(https://fonts.gstatic.com/s/geist/v1/gyByhwUxId8gMEwSGFWNOITddY4.woff2) format('woff2');unicode-range:U+0100-02BA,U+02BD-02C5,U+02C7-02CC,U+02CE-02D7,U+02DD-02FF,U+0304,U+0308,U+0329,U+1D00-1DBF,U+1E00-1E9F,U+1EF2-1EFF,U+2020,U+20A0-20AB,U+20AD-20C0,U+2113,U+2C60-2C7F,U+A720-A7FF}@font-face{font-family:'Geist';font-style:normal;font-weight:500;font-display:swap;src:url(https://fonts.gstatic.com/s/geist/v1/gyByhwUxId8gMEwcGFWNOITd.woff2) format('woff2');unicode-range:U+0000-00FF,U+0131,U+0152-0153,U+02BB-02BC,U+02C6,U+02DA,U+02DC,U+0304,U+0308,U+0329,U+2000-206F,U+20AC,U+2122,U+2191,U+2193,U+2212,U+2215,U+FEFF,U+FFFD}@font-face{font-family:'Geist';font-style:normal;font-weight:600;font-display:swap;src:url(https://fonts.gstatic.com/s/geist/v1/gyByhwUxId8gMEwSGFWNOITddY4.woff2) format('woff2');unicode-range:U+0100-02BA,U+02BD-02C5,U+02C7-02CC,U+02CE-02D7,U+02DD-02FF,U+0304,U+0308,U+0329,U+1D00-1DBF,U+1E00-1E9F,U+1EF2-1EFF,U+2020,U+20A0-20AB,U+20AD-20C0,U+2113,U+2C60-2C7F,U+A720-A7FF}@font-face{font-family:'Geist';font-style:normal;font-weight:600;font-display:swap;src:url(https://fonts.gstatic.com/s/geist/v1/gyByhwUxId8gMEwcGFWNOITd.woff2) format('woff2');unicode-range:U+0000-00FF,U+0131,U+0152-0153,U+02BB-02BC,U+02C6,U+02DA,U+02DC,U+0304,U+0308,U+0329,U+2000-206F,U+20AC,U+2122,U+2191,U+2193,U+2212,U+2215,U+FEFF,U+FFFD}@font-face{font-family:'Geist';font-style:normal;font-weight:700;font-display:swap;src:url(https://fonts.gstatic.com/s/geist/v1/gyByhwUxId8gMEwSGFWNOITddY4.woff2) format('woff2');unicode-range:U+0100-02BA,U+02BD-02C5,U+02C7-02CC,U+02CE-02D7,U+02DD-02FF,U+0304,U+0308,U+0329,U+1D00-1DBF,U+1E00-1E9F,U+1EF2-1EFF,U+2020,U+20A0-20AB,U+20AD-20C0,U+2113,U+2C60-2C7F,U+A720-A7FF}@font-face{font-family:'Geist';font-style:normal;font-weight:700;font-display:swap;src:url(https://fonts.gstatic.com/s/geist/v1/gyByhwUxId8gMEwcGFWNOITd.woff2) format('woff2');unicode-range:U+0000-00FF,U+0131,U+0152-0153,U+02BB-02BC,U+02C6,U+02DA,U+02DC,U+0304,U+0308,U+0329,U+2000-206F,U+20AC,U+2122,U+2191,U+2193,U+2212,U+2215,U+FEFF,U+FFFD}</style><style data-href="https://fonts.googleapis.com/css2?family=Geist%20Mono:wght@400;500;600;700&display=swap">@font-face{font-family:'Geist Mono';font-style:normal;font-weight:400;font-display:swap;src:url(https://fonts.gstatic.com/s/geistmono/v1/or3yQ6H-1_WfwkMZI_qYPLs1a-t7PU0AbeE9KJ5Q.woff) format('woff')}@font-face{font-family:'Geist Mono';font-style:normal;font-weight:500;font-display:swap;src:url(https://fonts.gstatic.com/s/geistmono/v1/or3yQ6H-1_WfwkMZI_qYPLs1a-t7PU0AbeEPKJ5Q.woff) format('woff')}@font-face{font-family:'Geist Mono';font-style:normal;font-weight:600;font-display:swap;src:url(https://fonts.gstatic.com/s/geistmono/v1/or3yQ6H-1_WfwkMZI_qYPLs1a-t7PU0AbeHjL55Q.woff) format('woff')}@font-face{font-family:'Geist Mono';font-style:normal;font-weight:700;font-display:swap;src:url(https://fonts.gstatic.com/s/geistmono/v1/or3yQ6H-1_WfwkMZI_qYPLs1a-t7PU0AbeHaL55Q.woff) format('woff')}@font-face{font-family:'Geist Mono';font-style:normal;font-weight:400;font-display:swap;src:url(https://fonts.gstatic.com/s/geistmono/v1/or3nQ6H-1_WfwkMZI_qYFrkdmhHkjkotbA.woff2) format('woff2');unicode-range:U+0100-02BA,U+02BD-02C5,U+02C7-02CC,U+02CE-02D7,U+02DD-02FF,U+0304,U+0308,U+0329,U+1D00-1DBF,U+1E00-1E9F,U+1EF2-1EFF,U+2020,U+20A0-20AB,U+20AD-20C0,U+2113,U+2C60-2C7F,U+A720-A7FF}@font-face{font-family:'Geist Mono';font-style:normal;font-weight:400;font-display:swap;src:url(https://fonts.gstatic.com/s/geistmono/v1/or3nQ6H-1_WfwkMZI_qYFrcdmhHkjko.woff2) format('woff2');unicode-range:U+0000-00FF,U+0131,U+0152-0153,U+02BB-02BC,U+02C6,U+02DA,U+02DC,U+0304,U+0308,U+0329,U+2000-206F,U+20AC,U+2122,U+2191,U+2193,U+2212,U+2215,U+FEFF,U+FFFD}@font-face{font-family:'Geist Mono';font-style:normal;font-weight:500;font-display:swap;src:url(https://fonts.gstatic.com/s/geistmono/v1/or3nQ6H-1_WfwkMZI_qYFrkdmhHkjkotbA.woff2) format('woff2');unicode-range:U+0100-02BA,U+02BD-02C5,U+02C7-02CC,U+02CE-02D7,U+02DD-02FF,U+0304,U+0308,U+0329,U+1D00-1DBF,U+1E00-1E9F,U+1EF2-1EFF,U+2020,U+20A0-20AB,U+20AD-20C0,U+2113,U+2C60-2C7F,U+A720-A7FF}@font-face{font-family:'Geist Mono';font-style:normal;font-weight:500;font-display:swap;src:url(https://fonts.gstatic.com/s/geistmono/v1/or3nQ6H-1_WfwkMZI_qYFrcdmhHkjko.woff2) format('woff2');unicode-range:U+0000-00FF,U+0131,U+0152-0153,U+02BB-02BC,U+02C6,U+02DA,U+02DC,U+0304,U+0308,U+0329,U+2000-206F,U+20AC,U+2122,U+2191,U+2193,U+2212,U+2215,U+FEFF,U+FFFD}@font-face{font-family:'Geist Mono';font-style:normal;font-weight:600;font-display:swap;src:url(https://fonts.gstatic.com/s/geistmono/v1/or3nQ6H-1_WfwkMZI_qYFrkdmhHkjkotbA.woff2) format('woff2');unicode-range:U+0100-02BA,U+02BD-02C5,U+02C7-02CC,U+02CE-02D7,U+02DD-02FF,U+0304,U+0308,U+0329,U+1D00-1DBF,U+1E00-1E9F,U+1EF2-1EFF,U+2020,U+20A0-20AB,U+20AD-20C0,U+2113,U+2C60-2C7F,U+A720-A7FF}@font-face{font-family:'Geist Mono';font-style:normal;font-weight:600;font-display:swap;src:url(https://fonts.gstatic.com/s/geistmono/v1/or3nQ6H-1_WfwkMZI_qYFrcdmhHkjko.woff2) format('woff2');unicode-range:U+0000-00FF,U+0131,U+0152-0153,U+02BB-02BC,U+02C6,U+02DA,U+02DC,U+0304,U+0308,U+0329,U+2000-206F,U+20AC,U+2122,U+2191,U+2193,U+2212,U+2215,U+FEFF,U+FFFD}@font-face{font-family:'Geist Mono';font-style:normal;font-weight:700;font-display:swap;src:url(https://fonts.gstatic.com/s/geistmono/v1/or3nQ6H-1_WfwkMZI_qYFrkdmhHkjkotbA.woff2) format('woff2');unicode-range:U+0100-02BA,U+02BD-02C5,U+02C7-02CC,U+02CE-02D7,U+02DD-02FF,U+0304,U+0308,U+0329,U+1D00-1DBF,U+1E00-1E9F,U+1EF2-1EFF,U+2020,U+20A0-20AB,U+20AD-20C0,U+2113,U+2C60-2C7F,U+A720-A7FF}@font-face{font-family:'Geist Mono';font-style:normal;font-weight:700;font-display:swap;src:url(https://fonts.gstatic.com/s/geistmono/v1/or3nQ6H-1_WfwkMZI_qYFrcdmhHkjko.woff2) format('woff2');unicode-range:U+0000-00FF,U+0131,U+0152-0153,U+02BB-02BC,U+02C6,U+02DA,U+02DC,U+0304,U+0308,U+0329,U+2000-206F,U+20AC,U+2122,U+2191,U+2193,U+2212,U+2215,U+FEFF,U+FFFD}</style><style data-href="https://fonts.googleapis.com/css2?family=Inter:wght@400..700&display=swap">@font-face{font-family:'Inter';font-style:normal;font-weight:400;font-display:swap;src:url(https://fonts.gstatic.com/s/inter/v18/UcCO3FwrK3iLTeHuS_nVMrMxCp50SjIw2boKoduKmMEVuLyfMZs.woff) format('woff')}@font-face{font-family:'Inter';font-style:normal;font-weight:500;font-display:swap;src:url(https://fonts.gstatic.com/s/inter/v18/UcCO3FwrK3iLTeHuS_nVMrMxCp50SjIw2boKoduKmMEVuI6fMZs.woff) format('woff')}@font-face{font-family:'Inter';font-style:normal;font-weight:600;font-display:swap;src:url(https://fonts.gstatic.com/s/inter/v18/UcCO3FwrK3iLTeHuS_nVMrMxCp50SjIw2boKoduKmMEVuGKYMZs.woff) format('woff')}@font-face{font-family:'Inter';font-style:normal;font-weight:700;font-display:swap;src:url(https://fonts.gstatic.com/s/inter/v18/UcCO3FwrK3iLTeHuS_nVMrMxCp50SjIw2boKoduKmMEVuFuYMZs.woff) format('woff')}@font-face{font-family:'Inter';font-style:normal;font-weight:400 700;font-display:swap;src:url(https://fonts.gstatic.com/s/inter/v18/UcC73FwrK3iLTeHuS_nVMrMxCp50SjIa2JL7W0Q5n-wU.woff2) format('woff2');unicode-range:U+0460-052F,U+1C80-1C8A,U+20B4,U+2DE0-2DFF,U+A640-A69F,U+FE2E-FE2F}@font-face{font-family:'Inter';font-style:normal;font-weight:400 700;font-display:swap;src:url(https://fonts.gstatic.com/s/inter/v18/UcC73FwrK3iLTeHuS_nVMrMxCp50SjIa0ZL7W0Q5n-wU.woff2) format('woff2');unicode-range:U+0301,U+0400-045F,U+0490-0491,U+04B0-04B1,U+2116}@font-face{font-family:'Inter';font-style:normal;font-weight:400 700;font-display:swap;src:url(https://fonts.gstatic.com/s/inter/v18/UcC73FwrK3iLTeHuS_nVMrMxCp50SjIa2ZL7W0Q5n-wU.woff2) format('woff2');unicode-range:U+1F00-1FFF}@font-face{font-family:'Inter';font-style:normal;font-weight:400 700;font-display:swap;src:url(https://fonts.gstatic.com/s/inter/v18/UcC73FwrK3iLTeHuS_nVMrMxCp50SjIa1pL7W0Q5n-wU.woff2) format('woff2');unicode-range:U+0370-0377,U+037A-037F,U+0384-038A,U+038C,U+038E-03A1,U+03A3-03FF}@font-face{font-family:'Inter';font-style:normal;font-weight:400 700;font-display:swap;src:url(https://fonts.gstatic.com/s/inter/v18/UcC73FwrK3iLTeHuS_nVMrMxCp50SjIa2pL7W0Q5n-wU.woff2) format('woff2');unicode-range:U+0102-0103,U+0110-0111,U+0128-0129,U+0168-0169,U+01A0-01A1,U+01AF-01B0,U+0300-0301,U+0303-0304,U+0308-0309,U+0323,U+0329,U+1EA0-1EF9,U+20AB}@font-face{font-family:'Inter';font-style:normal;font-weight:400 700;font-display:swap;src:url(https://fonts.gstatic.com/s/inter/v18/UcC73FwrK3iLTeHuS_nVMrMxCp50SjIa25L7W0Q5n-wU.woff2) format('woff2');unicode-range:U+0100-02BA,U+02BD-02C5,U+02C7-02CC,U+02CE-02D7,U+02DD-02FF,U+0304,U+0308,U+0329,U+1D00-1DBF,U+1E00-1E9F,U+1EF2-1EFF,U+2020,U+20A0-20AB,U+20AD-20C0,U+2113,U+2C60-2C7F,U+A720-A7FF}@font-face{font-family:'Inter';font-style:normal;font-weight:400 700;font-display:swap;src:url(https://fonts.gstatic.com/s/inter/v18/UcC73FwrK3iLTeHuS_nVMrMxCp50SjIa1ZL7W0Q5nw.woff2) format('woff2');unicode-range:U+0000-00FF,U+0131,U+0152-0153,U+02BB-02BC,U+02C6,U+02DA,U+02DC,U+0304,U+0308,U+0329,U+2000-206F,U+20AC,U+2122,U+2191,U+2193,U+2212,U+2215,U+FEFF,U+FFFD}</style></head><body><div id="__next"><header class="index_headerContainer__bg7s5 index_stickyHeader__lmTLG"><div class="index_bannerContainer__mlFqj"><p class="index_bannerText__TMQg_">🚀 Try Zilliz Cloud, the fully managed Milvus, for free—experience 10x faster performance! <a href="https://cloud.zilliz.com/signup?utm_source=milvusio&utm_medium=referral&utm_campaign=top_banner&utm_content=blog">Try Now>></a></p></div><div class="bg-white border-b-black4 border-b-[1px] border-solid max-[1280px]:border-none"><div class="hidden xl:flex h-[58px] px-10 items-center justify-between mx-auto"><div class="flex items-center gap-[40px] max-[1240px]:gap-[10px]"><div class="index_logoSection__ZC7jO"><a class="inline-flex items-center" href="/"><img src="/images/layout/milvus-logo.svg" height="24" alt="Milvus"/></a><div class="w-[1px] h-[20px] bg-black3 mx-[8px]"></div><a href="https://zilliz.com/" target="_blank" rel="noreferrer noopener"><img src="/images/layout/zilliz-logo.svg" alt="Zilliz" height="30"/></a></div><ul class="flex items-center list-none gap-[16px] max-[1080px]:gap-[10px]"><li class="shrink-0"><div class="group relative"><button class="group flex items-center gap-[4px] text-[14px] font-[500] h-[21px] text-black2 hover:text-black1 cursor-pointer font-mono uppercase text-[#667176]"><span class="inline-block leading-[16px]">Why Milvus</span><svg class="group-hover:rotate-180 transition-transform" xmlns="http://www.w3.org/2000/svg" width="16" height="16" viewBox="0 0 16 16" fill="none"><path d="M13.3295 6L9.08688 10.2426L4.84424 6" stroke="rgb(0, 19, 26, 0.7)"></path></svg></button><div class="invisible absolute opacity-0 group-hover:visible group-hover:z-10 group-hover:opacity-100 pt-[12px]"><ul class="flex flex-col items-stretch gap-[4px] bg-white py-[8px] -z-10 rounded-[4px] list-none shadow-nav-menu transition"><li><a class="flex items-center gap-[4px] text-[14px] leading-[40px] font-[400] px-[16px] no whitespace-nowrap cursor-pointer hover:bg-black/[0.04] font-mono uppercase text-[#667176]" href="/intro">What is Milvus</a></li><li><a class="flex items-center gap-[4px] text-[14px] leading-[40px] font-[400] px-[16px] no whitespace-nowrap cursor-pointer hover:bg-black/[0.04] font-mono uppercase text-[#667176]" href="/use-cases">Use Cases</a></li></ul></div></div></li><li><a class="block text-[14px] leading-[21px] font-[500] hover:text-black1 cursor-pointer font-mono uppercase text-black2" href="/docs">Docs</a></li><li class="shrink-0"><div class="group relative"><button class="group flex items-center gap-[4px] text-[14px] font-[500] h-[21px] text-black2 hover:text-black1 cursor-pointer font-mono uppercase text-[#667176]"><span class="inline-block leading-[16px]">Tutorials</span><svg class="group-hover:rotate-180 transition-transform" xmlns="http://www.w3.org/2000/svg" width="16" height="16" viewBox="0 0 16 16" fill="none"><path d="M13.3295 6L9.08688 10.2426L4.84424 6" stroke="rgb(0, 19, 26, 0.7)"></path></svg></button><div class="invisible absolute opacity-0 group-hover:visible group-hover:z-10 group-hover:opacity-100 pt-[12px]"><ul class="flex flex-col items-stretch gap-[4px] bg-white py-[8px] -z-10 rounded-[4px] list-none shadow-nav-menu transition"><li><a class="flex items-center gap-[4px] text-[14px] leading-[40px] font-[400] px-[16px] no whitespace-nowrap cursor-pointer hover:bg-black/[0.04] font-mono uppercase text-[#667176]" href="/bootcamp">Bootcamp</a></li><li><a class="flex items-center gap-[4px] text-[14px] leading-[40px] font-[400] px-[16px] no whitespace-nowrap cursor-pointer hover:bg-black/[0.04] font-mono uppercase text-[#667176]" href="/milvus-demos">Demos</a></li><li><a rel="noopener noreferrer" class="flex items-center gap-[4px] text-[14px] leading-[40px] font-[400] px-[16px] no whitespace-nowrap cursor-pointer hover:bg-black/[0.04] font-mono uppercase text-[#667176]" target="_blank" href="https://www.youtube.com/c/MilvusVectorDatabase">Video<svg xmlns="http://www.w3.org/2000/svg" width="14" height="14" viewBox="0 0 14 14" fill="none"><path d="M3.5 11L11.0833 3.41669M11.0833 3.41669V10.6967M11.0833 3.41669H3.80333" stroke="#00131A" stroke-linecap="round" stroke-linejoin="round"></path></svg></a></li></ul></div></div></li><li class="shrink-0"><div class="group relative"><button class="group flex items-center gap-[4px] text-[14px] font-[500] h-[21px] text-black2 hover:text-black1 cursor-pointer font-mono uppercase text-[#667176]"><span class="inline-block leading-[16px]">Tools</span><svg class="group-hover:rotate-180 transition-transform" xmlns="http://www.w3.org/2000/svg" width="16" height="16" viewBox="0 0 16 16" fill="none"><path d="M13.3295 6L9.08688 10.2426L4.84424 6" stroke="rgb(0, 19, 26, 0.7)"></path></svg></button><div class="invisible absolute opacity-0 group-hover:visible group-hover:z-10 group-hover:opacity-100 pt-[12px]"><ul class="flex flex-col items-stretch gap-[4px] bg-white py-[8px] -z-10 rounded-[4px] list-none shadow-nav-menu transition"><li><a rel="noopener noreferrer" class="flex items-center gap-[4px] text-[14px] leading-[40px] font-[400] px-[16px] no whitespace-nowrap cursor-pointer hover:bg-black/[0.04] font-mono uppercase text-[#667176]" target="_blank" href="https://github.com/zilliztech/attu">Attu<svg xmlns="http://www.w3.org/2000/svg" width="14" height="14" viewBox="0 0 14 14" fill="none"><path d="M3.5 11L11.0833 3.41669M11.0833 3.41669V10.6967M11.0833 3.41669H3.80333" stroke="#00131A" stroke-linecap="round" stroke-linejoin="round"></path></svg></a></li><li><a rel="noopener noreferrer" class="flex items-center gap-[4px] text-[14px] leading-[40px] font-[400] px-[16px] no whitespace-nowrap cursor-pointer hover:bg-black/[0.04] font-mono uppercase text-[#667176]" target="_blank" href="https://github.com/zilliztech/milvus_cli">Milvus CLI<svg xmlns="http://www.w3.org/2000/svg" width="14" height="14" viewBox="0 0 14 14" fill="none"><path d="M3.5 11L11.0833 3.41669M11.0833 3.41669V10.6967M11.0833 3.41669H3.80333" stroke="#00131A" stroke-linecap="round" stroke-linejoin="round"></path></svg></a></li><li><a class="flex items-center gap-[4px] text-[14px] leading-[40px] font-[400] px-[16px] no whitespace-nowrap cursor-pointer hover:bg-black/[0.04] font-mono uppercase text-[#667176]" href="/tools/sizing">Sizing Tool</a></li><li><a rel="noopener noreferrer" class="flex items-center gap-[4px] text-[14px] leading-[40px] font-[400] px-[16px] no whitespace-nowrap cursor-pointer hover:bg-black/[0.04] font-mono uppercase text-[#667176]" target="_blank" href="https://github.com/zilliztech/milvus-backup">Milvus Backup<svg xmlns="http://www.w3.org/2000/svg" width="14" height="14" viewBox="0 0 14 14" fill="none"><path d="M3.5 11L11.0833 3.41669M11.0833 3.41669V10.6967M11.0833 3.41669H3.80333" stroke="#00131A" stroke-linecap="round" stroke-linejoin="round"></path></svg></a></li><li><a rel="noopener noreferrer" class="flex items-center gap-[4px] text-[14px] leading-[40px] font-[400] px-[16px] no whitespace-nowrap cursor-pointer hover:bg-black/[0.04] font-mono uppercase text-[#667176]" target="_blank" href="https://github.com/zilliztech/vts">VTS<svg xmlns="http://www.w3.org/2000/svg" width="14" height="14" viewBox="0 0 14 14" fill="none"><path d="M3.5 11L11.0833 3.41669M11.0833 3.41669V10.6967M11.0833 3.41669H3.80333" stroke="#00131A" stroke-linecap="round" stroke-linejoin="round"></path></svg></a></li><li><a rel="noopener noreferrer" class="flex items-center gap-[4px] text-[14px] leading-[40px] font-[400] px-[16px] no whitespace-nowrap cursor-pointer hover:bg-black/[0.04] font-mono uppercase text-[#667176]" target="_blank" href="https://github.com/zilliztech/deep-searcher">Deep Searcher<svg xmlns="http://www.w3.org/2000/svg" width="14" height="14" viewBox="0 0 14 14" fill="none"><path d="M3.5 11L11.0833 3.41669M11.0833 3.41669V10.6967M11.0833 3.41669H3.80333" stroke="#00131A" stroke-linecap="round" stroke-linejoin="round"></path></svg></a></li></ul></div></div></li><li><a class="block text-[14px] leading-[21px] font-[500] hover:text-black1 cursor-pointer font-mono uppercase text-black2" href="/blog">Blog</a></li><li class="shrink-0"><div class="group relative"><button class="group flex items-center gap-[4px] text-[14px] font-[500] h-[21px] text-black2 hover:text-black1 cursor-pointer font-mono uppercase text-[#667176]"><span class="inline-block leading-[16px]">Community</span><svg class="group-hover:rotate-180 transition-transform" xmlns="http://www.w3.org/2000/svg" width="16" height="16" viewBox="0 0 16 16" fill="none"><path d="M13.3295 6L9.08688 10.2426L4.84424 6" stroke="rgb(0, 19, 26, 0.7)"></path></svg></button><div class="invisible absolute opacity-0 group-hover:visible group-hover:z-10 group-hover:opacity-100 pt-[12px]"><ul class="flex flex-col items-stretch gap-[4px] bg-white py-[8px] -z-10 rounded-[4px] list-none shadow-nav-menu transition"><li><a rel="noopener noreferrer" class="flex items-center gap-[4px] text-[14px] leading-[40px] font-[400] px-[16px] no whitespace-nowrap cursor-pointer hover:bg-black/[0.04] font-mono uppercase text-[#667176]" target="_blank" href="https://milvus.io/discord">Discord<svg xmlns="http://www.w3.org/2000/svg" width="14" height="14" viewBox="0 0 14 14" fill="none"><path d="M3.5 11L11.0833 3.41669M11.0833 3.41669V10.6967M11.0833 3.41669H3.80333" stroke="#00131A" stroke-linecap="round" stroke-linejoin="round"></path></svg></a></li><li><a rel="noopener noreferrer" class="flex items-center gap-[4px] text-[14px] leading-[40px] font-[400] px-[16px] no whitespace-nowrap cursor-pointer hover:bg-black/[0.04] font-mono uppercase text-[#667176]" target="_blank" href="https://github.com/milvus-io/milvus/discussions">GitHub<svg xmlns="http://www.w3.org/2000/svg" width="14" height="14" viewBox="0 0 14 14" fill="none"><path d="M3.5 11L11.0833 3.41669M11.0833 3.41669V10.6967M11.0833 3.41669H3.80333" stroke="#00131A" stroke-linecap="round" stroke-linejoin="round"></path></svg></a></li><li><a class="flex items-center gap-[4px] text-[14px] leading-[40px] font-[400] px-[16px] no whitespace-nowrap cursor-pointer hover:bg-black/[0.04] font-mono uppercase text-[#667176]" href="/community">More Channels</a></li></ul></div></div></li></ul></div><div class="flex gap-[20px] max-[1240px]:gap-[12px] max-[1080px]:gap-[10px] items-center font-mono"><div class=""><button type="button" role="combobox" aria-controls="radix-:R394m:" aria-expanded="false" aria-autocomplete="none" dir="ltr" data-state="closed" class="flex h-[20px] w-full items-center rounded-md border-slate-200 bg-white text-sm ring-offset-white placeholder:text-slate-500 focus:outline-none focus:ring-2 focus:ring-slate-950 focus:ring-offset-2 disabled:cursor-not-allowed disabled:bg-[#F9F9F9] disabled:text-[#667176] [&>span]:line-clamp-1 dark:border-slate-800 dark:bg-slate-950 dark:ring-offset-slate-950 dark:placeholder:text-slate-400 dark:focus:ring-slate-300 border-0 pl-0 gap-[4px] justify-start font-[600] text-black1 hover:text-black2"><svg xmlns="http://www.w3.org/2000/svg" width="16" height="17" viewBox="0 0 16 17" fill="none" class="transition-all"><g clip-path="url(#clip0_1804_386)"><rect width="16" height="16" transform="translate(0 0.5)" fill="white"></rect><path d="M8 16C7.03659 16 6.12919 15.8159 5.27778 15.4478C4.42637 15.0796 3.68489 14.5793 3.05333 13.9467C2.42074 13.3156 1.92037 12.5741 1.55222 11.7222C1.18407 10.8708 1 9.96341 1 9C1 8.034 1.18407 7.12581 1.55222 6.27544C1.92089 5.42507 2.42126 4.68411 3.05333 4.05256C3.68437 3.421 4.42585 2.92115 5.27778 2.553C6.12919 2.18433 7.03659 2 8 2C8.966 2 9.87419 2.18407 10.7246 2.55222C11.5749 2.92089 12.3159 3.42126 12.9474 4.05333C13.579 4.68489 14.0789 5.42559 14.447 6.27544C14.8157 7.12581 15 8.034 15 9C15 9.96341 14.8159 10.8708 14.4478 11.7222C14.0796 12.5736 13.5793 13.3151 12.9467 13.9467C12.3151 14.5787 11.5744 15.0791 10.7246 15.4478C9.87419 15.8159 8.966 16 8 16ZM8 15.2284C8.4563 14.642 8.83274 14.0662 9.12933 13.501C9.42541 12.9358 9.66626 12.3032 9.85189 11.6032H6.14811C6.35345 12.3426 6.59922 12.9949 6.88544 13.5601C7.17167 14.1253 7.54319 14.6814 8 15.2284ZM7.00989 15.1118C6.64693 14.684 6.31559 14.1551 6.01589 13.5251C5.71619 12.8956 5.49322 12.2547 5.347 11.6024H2.36344C2.80937 12.57 3.44119 13.3633 4.25889 13.9824C5.07711 14.6016 5.99385 14.978 7.00911 15.1118M8.98933 15.1118C10.0046 14.978 10.9213 14.6016 11.7396 13.9824C12.5578 13.3633 13.1896 12.57 13.635 11.6024H10.653C10.456 12.2646 10.2079 12.9107 9.90867 13.5407C9.60896 14.1701 9.30252 14.6939 8.98933 15.1118ZM2.04611 10.8247H5.18444C5.12533 10.5058 5.08385 10.1949 5.06 9.89211C5.03511 9.5893 5.02267 9.29193 5.02267 9C5.02267 8.70807 5.03485 8.4107 5.05922 8.10789C5.08359 7.80507 5.12507 7.49396 5.18367 7.17456H2.04767C1.96315 7.44419 1.89704 7.73793 1.84933 8.05578C1.80163 8.37363 1.77778 8.68837 1.77778 9C1.77778 9.31215 1.80137 9.62689 1.84856 9.94422C1.89626 10.2621 1.96237 10.5556 2.04689 10.8247M5.963 10.8247H10.037C10.0961 10.5058 10.1376 10.1999 10.1614 9.90689C10.1863 9.61444 10.1988 9.31215 10.1988 9C10.1988 8.68785 10.1866 8.38556 10.1622 8.09311C10.1379 7.80015 10.0964 7.49422 10.0378 7.17533H5.96222C5.90363 7.49422 5.86215 7.80015 5.83778 8.09311C5.81341 8.38556 5.80122 8.68785 5.80122 9C5.80122 9.31215 5.81341 9.61444 5.83778 9.90689C5.86215 10.1999 5.90441 10.5058 5.963 10.8247ZM10.8156 10.8247H13.9531C14.0376 10.555 14.1037 10.2616 14.1514 9.94422C14.1991 9.62689 14.2227 9.31215 14.2222 9C14.2222 8.68785 14.1986 8.37311 14.1514 8.05578C14.1037 7.73793 14.0376 7.44444 13.9531 7.17533H10.8148C10.8739 7.49422 10.9154 7.80507 10.9392 8.10789C10.9641 8.4107 10.9766 8.70807 10.9766 9C10.9766 9.29193 10.9644 9.5893 10.94 9.89211C10.9156 10.1949 10.8741 10.506 10.8156 10.8254M10.6538 6.39756H13.6358C13.18 5.4103 12.5557 4.61696 11.7629 4.01756C10.9696 3.41815 10.0453 3.03704 8.99011 2.87422C9.35307 3.35126 9.67948 3.89726 9.96933 4.51222C10.2587 5.12667 10.4868 5.75511 10.6538 6.39756ZM6.14811 6.39756H9.85189C9.64655 5.66748 9.39326 5.00741 9.092 4.41733C8.79126 3.82674 8.42726 3.27815 8 2.77156C7.57326 3.27815 7.20926 3.82674 6.908 4.41733C6.60726 5.00741 6.35396 5.66748 6.14811 6.39756ZM2.36422 6.39756H5.34622C5.51319 5.75511 5.74133 5.12667 6.03067 4.51222C6.32052 3.89726 6.64693 3.351 7.00989 2.87344C5.94485 3.03678 5.01826 3.42048 4.23011 4.02456C3.44196 4.62915 2.81974 5.41989 2.36344 6.39678" fill="currentColor"></path><path d="M7.00989 15.1118C6.64693 14.684 6.31559 14.1551 6.01589 13.5251C5.71619 12.8956 5.49322 12.2547 5.347 11.6024H2.36344C2.80937 12.57 3.44119 13.3633 4.25889 13.9824C5.07711 14.6016 5.99385 14.978 7.00911 15.1118M2.04611 10.8247H5.18444C5.12533 10.5058 5.08385 10.1949 5.06 9.89211C5.03511 9.5893 5.02267 9.29193 5.02267 9C5.02267 8.70807 5.03485 8.4107 5.05922 8.10789C5.08359 7.80507 5.12507 7.49396 5.18367 7.17456H2.04767C1.96315 7.44419 1.89704 7.73793 1.84933 8.05578C1.80163 8.37363 1.77778 8.68837 1.77778 9C1.77778 9.31215 1.80137 9.62689 1.84856 9.94422C1.89626 10.2621 1.96237 10.5556 2.04689 10.8247M10.8156 10.8247H13.9531C14.0376 10.555 14.1037 10.2616 14.1514 9.94422C14.1991 9.62689 14.2227 9.31215 14.2222 9C14.2222 8.68785 14.1986 8.37311 14.1514 8.05578C14.1037 7.73793 14.0376 7.44444 13.9531 7.17533H10.8148C10.8739 7.49422 10.9154 7.80507 10.9392 8.10789C10.9641 8.4107 10.9766 8.70807 10.9766 9C10.9766 9.29193 10.9644 9.5893 10.94 9.89211C10.9156 10.1949 10.8741 10.506 10.8156 10.8254M2.36422 6.39756H5.34622C5.51319 5.75511 5.74133 5.12667 6.03067 4.51222C6.32052 3.89726 6.64693 3.351 7.00989 2.87344C5.94485 3.03678 5.01826 3.42048 4.23011 4.02456C3.44196 4.62915 2.81974 5.41989 2.36344 6.39678M8 16C7.03659 16 6.12919 15.8159 5.27778 15.4478C4.42637 15.0796 3.68489 14.5793 3.05333 13.9467C2.42074 13.3156 1.92037 12.5741 1.55222 11.7222C1.18407 10.8708 1 9.96341 1 9C1 8.034 1.18407 7.12581 1.55222 6.27544C1.92089 5.42507 2.42126 4.68411 3.05333 4.05256C3.68437 3.421 4.42585 2.92115 5.27778 2.553C6.12919 2.18433 7.03659 2 8 2C8.966 2 9.87419 2.18407 10.7246 2.55222C11.5749 2.92089 12.3159 3.42126 12.9474 4.05333C13.579 4.68489 14.0789 5.42559 14.447 6.27544C14.8157 7.12581 15 8.034 15 9C15 9.96341 14.8159 10.8708 14.4478 11.7222C14.0796 12.5736 13.5793 13.3151 12.9467 13.9467C12.3151 14.5787 11.5744 15.0791 10.7246 15.4478C9.87419 15.8159 8.966 16 8 16ZM8 15.2284C8.4563 14.642 8.83274 14.0662 9.12933 13.501C9.42541 12.9358 9.66626 12.3032 9.85189 11.6032H6.14811C6.35344 12.3426 6.59922 12.9949 6.88544 13.5601C7.17167 14.1253 7.54319 14.6814 8 15.2284ZM8.98933 15.1118C10.0046 14.978 10.9213 14.6016 11.7396 13.9824C12.5578 13.3633 13.1896 12.57 13.635 11.6024H10.653C10.456 12.2646 10.2079 12.9107 9.90867 13.5407C9.60896 14.1701 9.30252 14.6939 8.98933 15.1118ZM5.963 10.8247H10.037C10.0961 10.5058 10.1376 10.1999 10.1614 9.90689C10.1863 9.61444 10.1988 9.31215 10.1988 9C10.1988 8.68785 10.1866 8.38556 10.1622 8.09311C10.1379 7.80015 10.0964 7.49422 10.0378 7.17533H5.96222C5.90363 7.49422 5.86215 7.80015 5.83778 8.09311C5.81341 8.38556 5.80122 8.68785 5.80122 9C5.80122 9.31215 5.81341 9.61444 5.83778 9.90689C5.86215 10.1999 5.90441 10.5058 5.963 10.8247ZM10.6538 6.39756H13.6358C13.18 5.4103 12.5557 4.61696 11.7629 4.01756C10.9696 3.41815 10.0453 3.03704 8.99011 2.87422C9.35307 3.35126 9.67948 3.89726 9.96933 4.51222C10.2587 5.12667 10.4868 5.75511 10.6538 6.39756ZM6.14811 6.39756H9.85189C9.64656 5.66748 9.39326 5.00741 9.092 4.41733C8.79126 3.82674 8.42726 3.27815 8 2.77156C7.57326 3.27815 7.20926 3.82674 6.908 4.41733C6.60726 5.00741 6.35396 5.66748 6.14811 6.39756Z" stroke="currentColor" stroke-width="0.5"></path></g><defs><clipPath id="clip0_1804_386"><rect width="16" height="16" fill="white" transform="translate(0 0.5)"></rect></clipPath></defs></svg><svg xmlns="http://www.w3.org/2000/svg" width="14" height="14" viewBox="0 0 14 14" fill="none" class="transition-all"><g clip-path="url(#clip0_3441_107)"><path d="M1.85228 5.0569L7 10.2046L12.1477 5.0569" stroke="#667176" stroke-width="1.3" stroke-linecap="round" stroke-linejoin="round"></path></g><defs><clipPath id="clip0_3441_107"><rect width="14" height="14" fill="white" transform="matrix(1 0 0 -1 0 14)"></rect></clipPath></defs></svg></button><select aria-hidden="true" tabindex="-1" style="position:absolute;border:0;width:1px;height:1px;padding:0;margin:-1px;overflow:hidden;clip:rect(0, 0, 0, 0);white-space:nowrap;word-wrap:normal"></select></div><a href="https://github.com/milvus-io/milvus" class="h-9 rounded-md flex items-center basis-[77px] flex-shrink-0 flex-grow-0 px-[6px] py-[3px] transition duration-200 ease-in-out text-black hover:text-black2" target="_blank"><svg xmlns="http://www.w3.org/2000/svg" width="14" height="14" viewBox="0 0 14 14" fill="currentColor"><g clip-path="url(#clip0_3441_111)"><path fill-rule="evenodd" clip-rule="evenodd" d="M7 0C3.1325 0 0 3.1325 0 7C0 10.0975 2.00375 12.7137 4.78625 13.6413C5.13625 13.7025 5.2675 13.4925 5.2675 13.3088C5.2675 13.1425 5.25875 12.5913 5.25875 12.005C3.5 12.3288 3.045 11.5763 2.905 11.1825C2.82625 10.9812 2.485 10.36 2.1875 10.1937C1.9425 10.0625 1.5925 9.73875 2.17875 9.73C2.73 9.72125 3.12375 10.2375 3.255 10.4475C3.885 11.5062 4.89125 11.2088 5.29375 11.025C5.355 10.57 5.53875 10.2638 5.74 10.0887C4.1825 9.91375 2.555 9.31 2.555 6.6325C2.555 5.87125 2.82625 5.24125 3.2725 4.75125C3.2025 4.57625 2.9575 3.85875 3.3425 2.89625C3.3425 2.89625 3.92875 2.7125 5.2675 3.61375C5.8275 3.45625 6.4225 3.3775 7.0175 3.3775C7.6125 3.3775 8.2075 3.45625 8.7675 3.61375C10.1063 2.70375 10.6925 2.89625 10.6925 2.89625C11.0775 3.85875 10.8325 4.57625 10.7625 4.75125C11.2087 5.24125 11.48 5.8625 11.48 6.6325C11.48 9.31875 9.84375 9.91375 8.28625 10.0887C8.54 10.3075 8.75875 10.7275 8.75875 11.3837C8.75875 12.32 8.75 13.0725 8.75 13.3088C8.75 13.4925 8.88125 13.7113 9.23125 13.6413C11.9963 12.7137 14 10.0887 14 7C14 3.1325 10.8675 0 7 0Z"></path></g><defs><clipPath id="clip0_3441_111"><rect width="14" height="14" fill="white"></rect></clipPath></defs></svg><span class="text-[12px] leading-[18px] ml-[2px] mr-[4px] whitespace-nowrap">Star</span><span class="text-[12px] font-[500] leading-[18px]">33.8K</span></a><a class="text-[12px] font-[500] leading-[18px] text-black1 hover:text-black2 transition duration-200 ease-in-out whitespace-pre" href="/contact">Contact Us</a><a target="_blank" href="https://cloud.zilliz.com/signup?utm_source=milvusio&utm_medium=referral&utm_campaign=nav_right&utm_content=blog"><div class="h-9 px-3 py-1.5 rounded-md bg-blue1 hover:bg-[#008DC8] justify-start items-center gap-1 inline-flex cursor-pointer transition duration-200 ease-in-out"><div class="text-center text-white text-sm font-medium leading-[21px] whitespace-nowrap">Try Managed Milvus</div></div></a></div></div></div><div class="block xl:hidden bg-white border-b border-solid border-gray-300"><div class="responsive_container__V94zl mobileHeader_mobileHeader__DN_uX"><div class="index_logoSection__ZC7jO"><a class="inline-flex items-center" href="/"><img src="/images/layout/milvus-logo.svg" height="24" alt="Milvus"/></a><div class="w-[1px] h-[20px] bg-black3 mx-[8px]"></div><a href="https://zilliz.com/" target="_blank" rel="noreferrer noopener"><img src="/images/layout/zilliz-logo.svg" alt="Zilliz" height="30"/></a></div><nav class="mobileHeader_navWrapper__ZO93B"><div class="responsive_container__V94zl mobileHeader_listWrapper__S6lVq"><div><style data-emotion="css 1iy2q10">.css-1iy2q10{list-style:none;margin:0;padding:0;position:relative;padding-top:8px;padding-bottom:8px;width:100%;}</style><nav class="MuiList-root MuiList-padding css-1iy2q10" aria-labelledby="nested-list-subheader"><a class="mobileHeader_menuLink__1b_qL" href="/docs"><style data-emotion="css 27xx2q">.css-27xx2q{display:-webkit-box;display:-webkit-flex;display:-ms-flexbox;display:flex;-webkit-box-flex:1;-webkit-flex-grow:1;-ms-flex-positive:1;flex-grow:1;-webkit-box-pack:start;-ms-flex-pack:start;-webkit-justify-content:flex-start;justify-content:flex-start;-webkit-align-items:center;-webkit-box-align:center;-ms-flex-align:center;align-items:center;position:relative;-webkit-text-decoration:none;text-decoration:none;min-width:0;box-sizing:border-box;text-align:left;padding-top:8px;padding-bottom:8px;-webkit-transition:background-color 150ms cubic-bezier(0.4, 0, 0.2, 1) 0ms;transition:background-color 150ms cubic-bezier(0.4, 0, 0.2, 1) 0ms;padding-left:16px;padding-right:16px;}.css-27xx2q:hover{-webkit-text-decoration:none;text-decoration:none;background-color:rgba(0, 0, 0, 0.04);}@media (hover: none){.css-27xx2q:hover{background-color:transparent;}}.css-27xx2q.Mui-selected{background-color:rgba(25, 118, 210, 0.08);}.css-27xx2q.Mui-selected.Mui-focusVisible{background-color:rgba(25, 118, 210, 0.2);}.css-27xx2q.Mui-selected:hover{background-color:rgba(25, 118, 210, 0.12);}@media (hover: none){.css-27xx2q.Mui-selected:hover{background-color:rgba(25, 118, 210, 0.08);}}.css-27xx2q.Mui-focusVisible{background-color:rgba(0, 0, 0, 0.12);}.css-27xx2q.Mui-disabled{opacity:0.38;}</style><style data-emotion="css 1uwabd6">.css-1uwabd6{display:-webkit-inline-box;display:-webkit-inline-flex;display:-ms-inline-flexbox;display:inline-flex;-webkit-align-items:center;-webkit-box-align:center;-ms-flex-align:center;align-items:center;-webkit-box-pack:center;-ms-flex-pack:center;-webkit-justify-content:center;justify-content:center;position:relative;box-sizing:border-box;-webkit-tap-highlight-color:transparent;background-color:transparent;outline:0;border:0;margin:0;border-radius:0;padding:0;cursor:pointer;-webkit-user-select:none;-moz-user-select:none;-ms-user-select:none;user-select:none;vertical-align:middle;-moz-appearance:none;-webkit-appearance:none;-webkit-text-decoration:none;text-decoration:none;color:inherit;display:-webkit-box;display:-webkit-flex;display:-ms-flexbox;display:flex;-webkit-box-flex:1;-webkit-flex-grow:1;-ms-flex-positive:1;flex-grow:1;-webkit-box-pack:start;-ms-flex-pack:start;-webkit-justify-content:flex-start;justify-content:flex-start;-webkit-align-items:center;-webkit-box-align:center;-ms-flex-align:center;align-items:center;position:relative;-webkit-text-decoration:none;text-decoration:none;min-width:0;box-sizing:border-box;text-align:left;padding-top:8px;padding-bottom:8px;-webkit-transition:background-color 150ms cubic-bezier(0.4, 0, 0.2, 1) 0ms;transition:background-color 150ms cubic-bezier(0.4, 0, 0.2, 1) 0ms;padding-left:16px;padding-right:16px;}.css-1uwabd6::-moz-focus-inner{border-style:none;}.css-1uwabd6.Mui-disabled{pointer-events:none;cursor:default;}@media print{.css-1uwabd6{-webkit-print-color-adjust:exact;color-adjust:exact;}}.css-1uwabd6:hover{-webkit-text-decoration:none;text-decoration:none;background-color:rgba(0, 0, 0, 0.04);}@media (hover: none){.css-1uwabd6:hover{background-color:transparent;}}.css-1uwabd6.Mui-selected{background-color:rgba(25, 118, 210, 0.08);}.css-1uwabd6.Mui-selected.Mui-focusVisible{background-color:rgba(25, 118, 210, 0.2);}.css-1uwabd6.Mui-selected:hover{background-color:rgba(25, 118, 210, 0.12);}@media (hover: none){.css-1uwabd6.Mui-selected:hover{background-color:rgba(25, 118, 210, 0.08);}}.css-1uwabd6.Mui-focusVisible{background-color:rgba(0, 0, 0, 0.12);}.css-1uwabd6.Mui-disabled{opacity:0.38;}</style><div class="MuiButtonBase-root MuiListItemButton-root MuiListItemButton-gutters MuiListItemButton-root MuiListItemButton-gutters css-1uwabd6" tabindex="0" role="button"><style data-emotion="css 1tsvksn">.css-1tsvksn{-webkit-flex:1 1 auto;-ms-flex:1 1 auto;flex:1 1 auto;min-width:0;margin-top:4px;margin-bottom:4px;}</style><div class="MuiListItemText-root css-1tsvksn"><style data-emotion="css yb0lig">.css-yb0lig{margin:0;font-family:"Roboto","Helvetica","Arial",sans-serif;font-weight:400;font-size:1rem;line-height:1.5;letter-spacing:0.00938em;display:block;}</style><span class="MuiTypography-root MuiTypography-body1 MuiListItemText-primary css-yb0lig">Docs</span></div></div></a><style data-emotion="css 39bbo6">.css-39bbo6{margin:0;-webkit-flex-shrink:0;-ms-flex-negative:0;flex-shrink:0;border-width:0;border-style:solid;border-color:rgba(0, 0, 0, 0.12);border-bottom-width:thin;}</style><hr class="MuiDivider-root MuiDivider-fullWidth css-39bbo6"/><div class="MuiButtonBase-root MuiListItemButton-root MuiListItemButton-gutters MuiListItemButton-root MuiListItemButton-gutters css-1uwabd6" tabindex="0" role="button"><div class="MuiListItemText-root css-1tsvksn"><span class="MuiTypography-root MuiTypography-body1 MuiListItemText-primary css-yb0lig">Tutorials</span></div><style data-emotion="css vubbuv">.css-vubbuv{-webkit-user-select:none;-moz-user-select:none;-ms-user-select:none;user-select:none;width:1em;height:1em;display:inline-block;fill:currentColor;-webkit-flex-shrink:0;-ms-flex-negative:0;flex-shrink:0;-webkit-transition:fill 200ms cubic-bezier(0.4, 0, 0.2, 1) 0ms;transition:fill 200ms cubic-bezier(0.4, 0, 0.2, 1) 0ms;font-size:1.5rem;}</style><svg class="MuiSvgIcon-root MuiSvgIcon-fontSizeMedium mobileHeader_expendIcon__6fVfE mobileHeader_static___wUnT css-vubbuv" focusable="false" aria-hidden="true" viewBox="0 0 24 24" data-testid="ExpandMoreIcon"><path d="M16.59 8.59 12 13.17 7.41 8.59 6 10l6 6 6-6z"></path></svg></div><hr class="MuiDivider-root MuiDivider-fullWidth css-39bbo6"/><div class="MuiButtonBase-root MuiListItemButton-root MuiListItemButton-gutters MuiListItemButton-root MuiListItemButton-gutters css-1uwabd6" tabindex="0" role="button"><div class="MuiListItemText-root css-1tsvksn"><span class="MuiTypography-root MuiTypography-body1 MuiListItemText-primary css-yb0lig">Tools</span></div><svg class="MuiSvgIcon-root MuiSvgIcon-fontSizeMedium mobileHeader_expendIcon__6fVfE mobileHeader_static___wUnT css-vubbuv" focusable="false" aria-hidden="true" viewBox="0 0 24 24" data-testid="ExpandMoreIcon"><path d="M16.59 8.59 12 13.17 7.41 8.59 6 10l6 6 6-6z"></path></svg></div><hr class="MuiDivider-root MuiDivider-fullWidth css-39bbo6"/><a class="mobileHeader_menuLink__1b_qL" href="/blog"><div class="MuiButtonBase-root MuiListItemButton-root MuiListItemButton-gutters MuiListItemButton-root MuiListItemButton-gutters css-1uwabd6" tabindex="0" role="button"><div class="MuiListItemText-root css-1tsvksn"><span class="MuiTypography-root MuiTypography-body1 MuiListItemText-primary css-yb0lig">Blog</span></div></div></a><hr class="MuiDivider-root MuiDivider-fullWidth css-39bbo6"/><div class="MuiButtonBase-root MuiListItemButton-root MuiListItemButton-gutters MuiListItemButton-root MuiListItemButton-gutters css-1uwabd6" tabindex="0" role="button"><div class="MuiListItemText-root css-1tsvksn"><span class="MuiTypography-root MuiTypography-body1 MuiListItemText-primary css-yb0lig">Community</span></div><svg class="MuiSvgIcon-root MuiSvgIcon-fontSizeMedium mobileHeader_expendIcon__6fVfE mobileHeader_static___wUnT css-vubbuv" focusable="false" aria-hidden="true" viewBox="0 0 24 24" data-testid="ExpandMoreIcon"><path d="M16.59 8.59 12 13.17 7.41 8.59 6 10l6 6 6-6z"></path></svg></div></nav></div><div class="mobileHeader_mobileStartBtnWrapper__U8ZJI"><a target="_self" class="block text-[14px] font-[500] leading-[40px] text-black1 px-[1rem] text-center h-[40px]" href="/contact">Contact Us</a><a target="_blank" href="https://cloud.zilliz.com/signup?utm_source=milvusio&utm_medium=referral&utm_campaign=nav_right&utm_content=blog"><button class="inline-flex items-center justify-center whitespace-nowrap rounded-md text-sm font-medium ring-offset-white transition-colors focus-visible:outline-none focus-visible:ring-2 focus-visible:ring-slate-950 focus-visible:ring-offset-2 disabled:pointer-events-none disabled:opacity-50 dark:ring-offset-slate-950 dark:focus-visible:ring-slate-300 font-mono bg-slate-900 text-slate-50 hover:bg-slate-900/90 dark:bg-slate-50 dark:text-slate-900 dark:hover:bg-slate-50/90 h-10 px-4 py-2 w-full">Try Managed Milvus</button></a></div></div></nav><div class="flex items-center gap-[12px]"><div class=""><button type="button" role="combobox" aria-controls="radix-:R3t4m:" aria-expanded="false" aria-autocomplete="none" dir="ltr" data-state="closed" class="flex h-[20px] w-full items-center rounded-md border-slate-200 bg-white text-sm ring-offset-white placeholder:text-slate-500 focus:outline-none focus:ring-2 focus:ring-slate-950 focus:ring-offset-2 disabled:cursor-not-allowed disabled:bg-[#F9F9F9] disabled:text-[#667176] [&>span]:line-clamp-1 dark:border-slate-800 dark:bg-slate-950 dark:ring-offset-slate-950 dark:placeholder:text-slate-400 dark:focus:ring-slate-300 border-0 pl-0 gap-[4px] justify-start font-[600] text-black1 hover:text-black2"><svg xmlns="http://www.w3.org/2000/svg" width="16" height="17" viewBox="0 0 16 17" fill="none" class="transition-all"><g clip-path="url(#clip0_1804_386)"><rect width="16" height="16" transform="translate(0 0.5)" fill="white"></rect><path d="M8 16C7.03659 16 6.12919 15.8159 5.27778 15.4478C4.42637 15.0796 3.68489 14.5793 3.05333 13.9467C2.42074 13.3156 1.92037 12.5741 1.55222 11.7222C1.18407 10.8708 1 9.96341 1 9C1 8.034 1.18407 7.12581 1.55222 6.27544C1.92089 5.42507 2.42126 4.68411 3.05333 4.05256C3.68437 3.421 4.42585 2.92115 5.27778 2.553C6.12919 2.18433 7.03659 2 8 2C8.966 2 9.87419 2.18407 10.7246 2.55222C11.5749 2.92089 12.3159 3.42126 12.9474 4.05333C13.579 4.68489 14.0789 5.42559 14.447 6.27544C14.8157 7.12581 15 8.034 15 9C15 9.96341 14.8159 10.8708 14.4478 11.7222C14.0796 12.5736 13.5793 13.3151 12.9467 13.9467C12.3151 14.5787 11.5744 15.0791 10.7246 15.4478C9.87419 15.8159 8.966 16 8 16ZM8 15.2284C8.4563 14.642 8.83274 14.0662 9.12933 13.501C9.42541 12.9358 9.66626 12.3032 9.85189 11.6032H6.14811C6.35345 12.3426 6.59922 12.9949 6.88544 13.5601C7.17167 14.1253 7.54319 14.6814 8 15.2284ZM7.00989 15.1118C6.64693 14.684 6.31559 14.1551 6.01589 13.5251C5.71619 12.8956 5.49322 12.2547 5.347 11.6024H2.36344C2.80937 12.57 3.44119 13.3633 4.25889 13.9824C5.07711 14.6016 5.99385 14.978 7.00911 15.1118M8.98933 15.1118C10.0046 14.978 10.9213 14.6016 11.7396 13.9824C12.5578 13.3633 13.1896 12.57 13.635 11.6024H10.653C10.456 12.2646 10.2079 12.9107 9.90867 13.5407C9.60896 14.1701 9.30252 14.6939 8.98933 15.1118ZM2.04611 10.8247H5.18444C5.12533 10.5058 5.08385 10.1949 5.06 9.89211C5.03511 9.5893 5.02267 9.29193 5.02267 9C5.02267 8.70807 5.03485 8.4107 5.05922 8.10789C5.08359 7.80507 5.12507 7.49396 5.18367 7.17456H2.04767C1.96315 7.44419 1.89704 7.73793 1.84933 8.05578C1.80163 8.37363 1.77778 8.68837 1.77778 9C1.77778 9.31215 1.80137 9.62689 1.84856 9.94422C1.89626 10.2621 1.96237 10.5556 2.04689 10.8247M5.963 10.8247H10.037C10.0961 10.5058 10.1376 10.1999 10.1614 9.90689C10.1863 9.61444 10.1988 9.31215 10.1988 9C10.1988 8.68785 10.1866 8.38556 10.1622 8.09311C10.1379 7.80015 10.0964 7.49422 10.0378 7.17533H5.96222C5.90363 7.49422 5.86215 7.80015 5.83778 8.09311C5.81341 8.38556 5.80122 8.68785 5.80122 9C5.80122 9.31215 5.81341 9.61444 5.83778 9.90689C5.86215 10.1999 5.90441 10.5058 5.963 10.8247ZM10.8156 10.8247H13.9531C14.0376 10.555 14.1037 10.2616 14.1514 9.94422C14.1991 9.62689 14.2227 9.31215 14.2222 9C14.2222 8.68785 14.1986 8.37311 14.1514 8.05578C14.1037 7.73793 14.0376 7.44444 13.9531 7.17533H10.8148C10.8739 7.49422 10.9154 7.80507 10.9392 8.10789C10.9641 8.4107 10.9766 8.70807 10.9766 9C10.9766 9.29193 10.9644 9.5893 10.94 9.89211C10.9156 10.1949 10.8741 10.506 10.8156 10.8254M10.6538 6.39756H13.6358C13.18 5.4103 12.5557 4.61696 11.7629 4.01756C10.9696 3.41815 10.0453 3.03704 8.99011 2.87422C9.35307 3.35126 9.67948 3.89726 9.96933 4.51222C10.2587 5.12667 10.4868 5.75511 10.6538 6.39756ZM6.14811 6.39756H9.85189C9.64655 5.66748 9.39326 5.00741 9.092 4.41733C8.79126 3.82674 8.42726 3.27815 8 2.77156C7.57326 3.27815 7.20926 3.82674 6.908 4.41733C6.60726 5.00741 6.35396 5.66748 6.14811 6.39756ZM2.36422 6.39756H5.34622C5.51319 5.75511 5.74133 5.12667 6.03067 4.51222C6.32052 3.89726 6.64693 3.351 7.00989 2.87344C5.94485 3.03678 5.01826 3.42048 4.23011 4.02456C3.44196 4.62915 2.81974 5.41989 2.36344 6.39678" fill="currentColor"></path><path d="M7.00989 15.1118C6.64693 14.684 6.31559 14.1551 6.01589 13.5251C5.71619 12.8956 5.49322 12.2547 5.347 11.6024H2.36344C2.80937 12.57 3.44119 13.3633 4.25889 13.9824C5.07711 14.6016 5.99385 14.978 7.00911 15.1118M2.04611 10.8247H5.18444C5.12533 10.5058 5.08385 10.1949 5.06 9.89211C5.03511 9.5893 5.02267 9.29193 5.02267 9C5.02267 8.70807 5.03485 8.4107 5.05922 8.10789C5.08359 7.80507 5.12507 7.49396 5.18367 7.17456H2.04767C1.96315 7.44419 1.89704 7.73793 1.84933 8.05578C1.80163 8.37363 1.77778 8.68837 1.77778 9C1.77778 9.31215 1.80137 9.62689 1.84856 9.94422C1.89626 10.2621 1.96237 10.5556 2.04689 10.8247M10.8156 10.8247H13.9531C14.0376 10.555 14.1037 10.2616 14.1514 9.94422C14.1991 9.62689 14.2227 9.31215 14.2222 9C14.2222 8.68785 14.1986 8.37311 14.1514 8.05578C14.1037 7.73793 14.0376 7.44444 13.9531 7.17533H10.8148C10.8739 7.49422 10.9154 7.80507 10.9392 8.10789C10.9641 8.4107 10.9766 8.70807 10.9766 9C10.9766 9.29193 10.9644 9.5893 10.94 9.89211C10.9156 10.1949 10.8741 10.506 10.8156 10.8254M2.36422 6.39756H5.34622C5.51319 5.75511 5.74133 5.12667 6.03067 4.51222C6.32052 3.89726 6.64693 3.351 7.00989 2.87344C5.94485 3.03678 5.01826 3.42048 4.23011 4.02456C3.44196 4.62915 2.81974 5.41989 2.36344 6.39678M8 16C7.03659 16 6.12919 15.8159 5.27778 15.4478C4.42637 15.0796 3.68489 14.5793 3.05333 13.9467C2.42074 13.3156 1.92037 12.5741 1.55222 11.7222C1.18407 10.8708 1 9.96341 1 9C1 8.034 1.18407 7.12581 1.55222 6.27544C1.92089 5.42507 2.42126 4.68411 3.05333 4.05256C3.68437 3.421 4.42585 2.92115 5.27778 2.553C6.12919 2.18433 7.03659 2 8 2C8.966 2 9.87419 2.18407 10.7246 2.55222C11.5749 2.92089 12.3159 3.42126 12.9474 4.05333C13.579 4.68489 14.0789 5.42559 14.447 6.27544C14.8157 7.12581 15 8.034 15 9C15 9.96341 14.8159 10.8708 14.4478 11.7222C14.0796 12.5736 13.5793 13.3151 12.9467 13.9467C12.3151 14.5787 11.5744 15.0791 10.7246 15.4478C9.87419 15.8159 8.966 16 8 16ZM8 15.2284C8.4563 14.642 8.83274 14.0662 9.12933 13.501C9.42541 12.9358 9.66626 12.3032 9.85189 11.6032H6.14811C6.35344 12.3426 6.59922 12.9949 6.88544 13.5601C7.17167 14.1253 7.54319 14.6814 8 15.2284ZM8.98933 15.1118C10.0046 14.978 10.9213 14.6016 11.7396 13.9824C12.5578 13.3633 13.1896 12.57 13.635 11.6024H10.653C10.456 12.2646 10.2079 12.9107 9.90867 13.5407C9.60896 14.1701 9.30252 14.6939 8.98933 15.1118ZM5.963 10.8247H10.037C10.0961 10.5058 10.1376 10.1999 10.1614 9.90689C10.1863 9.61444 10.1988 9.31215 10.1988 9C10.1988 8.68785 10.1866 8.38556 10.1622 8.09311C10.1379 7.80015 10.0964 7.49422 10.0378 7.17533H5.96222C5.90363 7.49422 5.86215 7.80015 5.83778 8.09311C5.81341 8.38556 5.80122 8.68785 5.80122 9C5.80122 9.31215 5.81341 9.61444 5.83778 9.90689C5.86215 10.1999 5.90441 10.5058 5.963 10.8247ZM10.6538 6.39756H13.6358C13.18 5.4103 12.5557 4.61696 11.7629 4.01756C10.9696 3.41815 10.0453 3.03704 8.99011 2.87422C9.35307 3.35126 9.67948 3.89726 9.96933 4.51222C10.2587 5.12667 10.4868 5.75511 10.6538 6.39756ZM6.14811 6.39756H9.85189C9.64656 5.66748 9.39326 5.00741 9.092 4.41733C8.79126 3.82674 8.42726 3.27815 8 2.77156C7.57326 3.27815 7.20926 3.82674 6.908 4.41733C6.60726 5.00741 6.35396 5.66748 6.14811 6.39756Z" stroke="currentColor" stroke-width="0.5"></path></g><defs><clipPath id="clip0_1804_386"><rect width="16" height="16" fill="white" transform="translate(0 0.5)"></rect></clipPath></defs></svg><svg xmlns="http://www.w3.org/2000/svg" width="14" height="14" viewBox="0 0 14 14" fill="none" class="transition-all"><g clip-path="url(#clip0_3441_107)"><path d="M1.85228 5.0569L7 10.2046L12.1477 5.0569" stroke="#667176" stroke-width="1.3" stroke-linecap="round" stroke-linejoin="round"></path></g><defs><clipPath id="clip0_3441_107"><rect width="14" height="14" fill="white" transform="matrix(1 0 0 -1 0 14)"></rect></clipPath></defs></svg></button><select aria-hidden="true" tabindex="-1" style="position:absolute;border:0;width:1px;height:1px;padding:0;margin:-1px;overflow:hidden;clip:rect(0, 0, 0, 0);white-space:nowrap;word-wrap:normal"></select></div><button class="mobileHeader_menuIconBtn__TPfKx"><svg width="24" height="24" viewBox="0 0 24 24"><rect x="2" y="5" width="20" height="2" fill="black"></rect><rect x="2" y="11" width="20" height="2" fill="black"></rect><rect x="2" y="17" width="20" height="2" fill="black"></rect></svg></button></div></div></div></header><main><section class="blog_banner__dRg4d"><div class="responsive_homeContainer__9_COi"><div class="blog_banner-detail__BxnhW"><section class="blog_recommend__W45TX"><span class="blog_trending__4Q7G5"><svg width="16" height="16" viewBox="0 0 16 16" fill="none" xmlns="http://www.w3.org/2000/svg"><path d="M14 4.66675L9.47333 9.19341C9.41136 9.2559 9.33762 9.3055 9.25638 9.33934C9.17515 9.37319 9.08801 9.39061 9 9.39061C8.91199 9.39061 8.82486 9.37319 8.74362 9.33934C8.66238 9.3055 8.58864 9.2559 8.52667 9.19341L6.80667 7.47341C6.74469 7.41093 6.67096 7.36133 6.58972 7.32749C6.50848 7.29364 6.42134 7.27622 6.33333 7.27622C6.24533 7.27622 6.15819 7.29364 6.07695 7.32749C5.99571 7.36133 5.92198 7.41093 5.86 7.47341L2 11.3334" stroke="currentColor" stroke-linecap="round" stroke-linejoin="round"></path><path d="M13.9997 7.33341V4.66675H11.333" stroke="currentColor" stroke-linecap="round" stroke-linejoin="round"></path></svg>TRENDING</span><a href="/blog/milvus-2025-roadmap-tell-us-what-you-think.md"><h1 class="blog_recommend-title__EEpci">Milvus 2025 Roadmap - Tell Us What You Think </h1></a><p class="blog_recommend-desc__qzthC">In 2025, we’re rolling out two major versions, Milvus 2.6 and Milvus 3.0, and many other technical features. We welcome you to share your thoughts with us.</p><div class="blog_recommend-extra__oyHs7"><p class="blog_blog-extra__ICdzc blog_dark___LE8C"><span class="blog_blog-extra-tags__Rf_FB">Announcements</span><span class="blog_blog-extra-separator__OqBcN"></span><span>Mar 27, 2025</span></p></div></section><div class="blog_recent__fcehD"><div><div class="blog_tabs-title__EJGTr"><div class="blog_tabs-title-item__V8Wxa blog_active__r5CED"><svg width="20" height="20" viewBox="0 0 20 20" fill="none" xmlns="http://www.w3.org/2000/svg"><path d="M10 2.5C11.4834 2.5 12.9334 2.93987 14.1668 3.76398C15.4001 4.58809 16.3614 5.75943 16.9291 7.12987C17.4968 8.50032 17.6453 10.0083 17.3559 11.4632C17.0665 12.918 16.3522 14.2544 15.3033 15.3033C14.2544 16.3522 12.918 17.0665 11.4632 17.3559C10.0083 17.6453 8.50032 17.4968 7.12987 16.9291C5.75943 16.3614 4.58809 15.4001 3.76398 14.1668C2.93987 12.9334 2.5 11.4834 2.5 10" stroke="currentColor" stroke-width="1.25" stroke-linecap="round" stroke-linejoin="round"></path><path d="M6.66699 10.0002H10.0003V5.8335" stroke="currentColor" stroke-width="1.25" stroke-linecap="round" stroke-linejoin="round"></path></svg>MOST RECENT</div><div class="blog_tabs-title-item__V8Wxa"><svg width="20" height="20" viewBox="0 0 20 20" fill="none" xmlns="http://www.w3.org/2000/svg"><path d="M13.1997 11.8167C13.6188 12.7758 13.722 13.8434 13.4942 14.8651C13.2665 15.8868 12.7198 16.8096 11.933 17.5L9.58301 15.15" stroke="currentColor" stroke-width="1.25" stroke-linecap="round" stroke-linejoin="round"></path><path d="M8.18333 6.79992C7.22416 6.38081 6.15654 6.27765 5.13486 6.50535C4.11319 6.73306 3.19042 7.27983 2.5 8.06659L4.85 10.4166" stroke="currentColor" stroke-width="1.25" stroke-linecap="round" stroke-linejoin="round"></path><path d="M8.90828 15.6584C15.5749 10.8167 17.1416 5.6584 17.4916 3.4584C17.5129 3.32848 17.5031 3.19535 17.4631 3.06994C17.423 2.94453 17.3538 2.83039 17.2611 2.73689C17.1684 2.64339 17.0549 2.57318 16.9298 2.53201C16.8048 2.49085 16.6717 2.4799 16.5416 2.50007C14.3666 2.8584 9.16661 4.39174 4.34161 11.0917L8.90828 15.6584ZM7.24161 16.3251L8.42494 15.1417L4.85828 11.6001L3.67494 12.7834C3.51974 12.9395 3.43262 13.1507 3.43262 13.3709C3.43262 13.5911 3.51974 13.8023 3.67494 13.9584L6.04161 16.3251C6.19775 16.4803 6.40896 16.5674 6.62911 16.5674C6.84927 16.5674 7.06048 16.4803 7.21661 16.3251H7.24161Z" stroke="currentColor" stroke-width="1.25" stroke-linecap="round" stroke-linejoin="round"></path></svg>RELEASES & EVENTS</div></div><div><div class="blog_recent-blog__Hvno_"><a href="/blog/parse-is-hard-solve-semantic-understanding-with-mistral-ocr-and-milvus.md"><h2 class="blog_recent-blog-title__2tqP7">Parsing is Hard: Solving Semantic Understanding with Mistral OCR and Milvus </h2></a><p class="blog_blog-extra__ICdzc"><span class="blog_blog-extra-tags__Rf_FB">Engineering</span><span class="blog_blog-extra-separator__OqBcN"></span><span>Apr 03, 2025</span></p></div><hr class="blog_recent-blog-separator__JJUbd"/><div class="blog_recent-blog__Hvno_"><a href="/blog/generate-more-creative-and-curated-ghibli-style-images-with-gpt-4o-and-milvus.md"><h2 class="blog_recent-blog-title__2tqP7">Generating More Creative and Curated Ghibli-Style Images with GPT-4o and Milvus</h2></a><p class="blog_blog-extra__ICdzc"><span class="blog_blog-extra-tags__Rf_FB">Engineering</span><span class="blog_blog-extra-separator__OqBcN"></span><span>Apr 01, 2025</span></p></div><hr class="blog_recent-blog-separator__JJUbd"/><div class="blog_recent-blog__Hvno_"><a href="/blog/deepseek-v3-0324-minor-update-thats-crushing-top-ai-models.md"><h2 class="blog_recent-blog-title__2tqP7">DeepSeek V3-0324: The "Minor Update" That's Crushing Top AI Models </h2></a><p class="blog_blog-extra__ICdzc"><span class="blog_blog-extra-tags__Rf_FB">Engineering</span><span class="blog_blog-extra-separator__OqBcN"></span><span>Mar 25, 2025</span></p></div></div></div></div></div><div class="blog_subscribe__RyRkx"><div class="blog_subscribe-info__IW8Kx"><h2 class="blog_subscribe-title__SVE8y">Be the first to know</h2><p class="blog_subscribe-desc___pFBE">Get updates on Milvus releases and tutorials from Zilliz, Milvus' creator and key maintainer.</p></div><div class="blog_subscribe-form__BTIxd"><div class="index_subscribeContainer__KRyy9"><div class="index_subscribeSection__HxBZ7"><div class="index_inputWrapper__S93JG"><div class="customInput_container__QaET_ index_customInputContainer__E0Bx2 blog_subscribe-input-container____xpT"><span></span><input class="customInput_input__LWwEQ customInput_fullWidth__LQFVu index_customInput__jCRxv blog_subscribe-input___eKuF" placeholder="Email"/></div><p class="index_errorMessage__FXqEW blog_subscribe-input-message__Q6_QM"></p></div><button class="index_linkButton__KW4cr index_customSubscribeButton__qseMJ blog_subscribe-button__73_BR whitespace-nowrap index_contained__3m7DK index_primaryColor__GTYfK index_largeSize__w54YY">Subscribe</button></div></div></div></div></div></section><section class="blog_content__sDrVo"><div class="responsive_homeContainer__9_COi"><header class="blog_list-header__eF_ii"><div class="blog_filter__cFr7r"><div class="blog_filter-tags__YMAoG"><a href="/blog"><div class="blog_filter-tag__QykY9 blog_active__r5CED">ALL</div></a><a href="/blog?blog_tag=engineering"><div class="blog_filter-tag__QykY9">Engineering</div></a><a href="/blog?blog_tag=announcements"><div class="blog_filter-tag__QykY9">Announcements</div></a><a href="/blog?blog_tag=tutorials"><div class="blog_filter-tag__QykY9">Tutorials</div></a><a href="/blog?blog_tag=usecases"><div class="blog_filter-tag__QykY9">Use Cases</div></a></div></div><label class="blog_searcher__Aw9FA"><svg width="20" height="21" viewBox="0 0 20 21" fill="none" xmlns="http://www.w3.org/2000/svg" class="blog_searcher-icon__PYwvl"><path d="M12.917 13.4165L15.8337 16.3332" stroke="currentColor" stroke-width="1.25" stroke-linecap="round" stroke-linejoin="round"></path><path d="M4.16699 9.6665C4.16699 12.4279 6.40557 14.6665 9.16699 14.6665C10.5501 14.6665 11.8021 14.1049 12.7072 13.1973C13.6093 12.2929 14.167 11.0448 14.167 9.6665C14.167 6.90508 11.9284 4.6665 9.16699 4.6665C6.40557 4.6665 4.16699 6.90508 4.16699 9.6665Z" stroke="currentColor" stroke-width="1.25" stroke-linecap="round" stroke-linejoin="round"></path></svg><input class="blog_searcher-input__SPb93" placeholder="Search"/></label></header><section class="blog_list-detail__jRCpT"><a class="blog_blog-card__mTmhJ" title="Parsing is Hard: Solving Semantic Understanding with Mistral OCR and Milvus " href="/blog/parse-is-hard-solve-semantic-understanding-with-mistral-ocr-and-milvus.md"><div class="blog_blog-card-img__qyc2Q" style="background-image:url(https://assets.zilliz.com/Parsing_is_Hard_Solving_Semantic_Understanding_with_Mistral_OCR_and_Milvus_316ac013b6.png)"></div><p class="blog_blog-extra__ICdzc blog_blog-card-extra__G07HX"><span class="blog_blog-extra-tags__Rf_FB">Engineering</span><span class="blog_blog-extra-separator__OqBcN"></span><span>Apr 03, 2025</span></p><h3 class="blog_blog-card-title__OEYNA">Parsing is Hard: Solving Semantic Understanding with Mistral OCR and Milvus </h3></a><a class="blog_blog-card__mTmhJ" title="Generating More Creative and Curated Ghibli-Style Images with GPT-4o and Milvus" href="/blog/generate-more-creative-and-curated-ghibli-style-images-with-gpt-4o-and-milvus.md"><div class="blog_blog-card-img__qyc2Q" style="background-image:url(https://assets.zilliz.com/GPT_4opagephoto_5e934b89e5.png)"></div><p class="blog_blog-extra__ICdzc blog_blog-card-extra__G07HX"><span class="blog_blog-extra-tags__Rf_FB">Engineering</span><span class="blog_blog-extra-separator__OqBcN"></span><span>Apr 01, 2025</span></p><h3 class="blog_blog-card-title__OEYNA">Generating More Creative and Curated Ghibli-Style Images with GPT-4o and Milvus</h3></a><a class="blog_blog-card__mTmhJ" title="DeepSeek V3-0324: The "Minor Update" That's Crushing Top AI Models " href="/blog/deepseek-v3-0324-minor-update-thats-crushing-top-ai-models.md"><div class="blog_blog-card-img__qyc2Q" style="background-image:url(https://assets.zilliz.com/Deep_Seek_V3_0324_The_Minor_Update_That_s_Crushing_Top_AI_Models_391585994c.png)"></div><p class="blog_blog-extra__ICdzc blog_blog-card-extra__G07HX"><span class="blog_blog-extra-tags__Rf_FB">Engineering</span><span class="blog_blog-extra-separator__OqBcN"></span><span>Mar 25, 2025</span></p><h3 class="blog_blog-card-title__OEYNA">DeepSeek V3-0324: The "Minor Update" That's Crushing Top AI Models </h3></a><a class="blog_blog-card__mTmhJ" title="What Exactly is a Vector Database and How Does It Work" href="/blog/what-is-a-vector-database.md"><div class="blog_blog-card-img__qyc2Q" style="background-image:url(https://assets.zilliz.com/What_s_a_Vector_Database_and_How_Does_It_Work_cac0875415.png)"></div><p class="blog_blog-extra__ICdzc blog_blog-card-extra__G07HX"><span class="blog_blog-extra-tags__Rf_FB">Engineering</span><span class="blog_blog-extra-separator__OqBcN"></span><span>Mar 24, 2025</span></p><h3 class="blog_blog-card-title__OEYNA">What Exactly is a Vector Database and How Does It Work</h3></a><a class="blog_blog-card__mTmhJ" title="Stop Using Outdated RAG: DeepSearcher's Agentic RAG Approach Changes Everything " href="/blog/stop-use-outdated-rag-deepsearcher-agentic-rag-approaches-changes-everything.md"><div class="blog_blog-card-img__qyc2Q" style="background-image:url(https://assets.zilliz.com/Stop_Using_Outdated_RAG_Deep_Searcher_s_Agentic_RAG_Approach_Changes_Everything_b2eaa644cf.png)"></div><p class="blog_blog-extra__ICdzc blog_blog-card-extra__G07HX"><span class="blog_blog-extra-tags__Rf_FB">Engineering</span><span class="blog_blog-extra-separator__OqBcN"></span><span>Mar 23, 2025</span></p><h3 class="blog_blog-card-title__OEYNA">Stop Using Outdated RAG: DeepSearcher's Agentic RAG Approach Changes Everything </h3></a><a class="blog_blog-card__mTmhJ" title="Why Manual Sharding is a Bad Idea for Vector Database And How to Fix It" href="/blog/why-manual-sharding-is-a-bad-idea-for-vector-databases-and-how-to-fix-it.md"><div class="blog_blog-card-img__qyc2Q" style="background-image:url(https://assets.zilliz.com/Why_Manual_Sharding_is_a_Bad_Idea_for_Vector_Database_And_How_to_Fix_It_1_968a5be504.png)"></div><p class="blog_blog-extra__ICdzc blog_blog-card-extra__G07HX"><span class="blog_blog-extra-tags__Rf_FB">Engineering</span><span class="blog_blog-extra-separator__OqBcN"></span><span>Mar 18, 2025</span></p><h3 class="blog_blog-card-title__OEYNA">Why Manual Sharding is a Bad Idea for Vector Database And How to Fix It</h3></a><a class="blog_blog-card__mTmhJ" title="A Day in the Life of a Milvus Datum" href="/blog/a-day-in-the-life-of-milvus-datum.md"><div class="blog_blog-card-img__qyc2Q" style="background-image:url(https://assets.zilliz.com/a_day_in_the_life_of_a_milvus_datum_ca279f7f59.png)"></div><p class="blog_blog-extra__ICdzc blog_blog-card-extra__G07HX"><span class="blog_blog-extra-tags__Rf_FB">Engineering</span><span class="blog_blog-extra-separator__OqBcN"></span><span>Mar 17, 2025</span></p><h3 class="blog_blog-card-title__OEYNA">A Day in the Life of a Milvus Datum</h3></a><a class="blog_blog-card__mTmhJ" title="AI for Smarter Browsing: Filtering Web Content with Pixtral, Milvus, and Browser Use" href="/blog/ai-for-smarter-browsing-filtering-web-content-with-pirxtral-milvus-browser-use.md"><div class="blog_blog-card-img__qyc2Q" style="background-image:url(https://assets.zilliz.com/AI_for_Smarter_Browsing_Filtering_Web_Content_with_Pixtral_Milvus_and_Browser_Use_56d0154bbd.png)"></div><p class="blog_blog-extra__ICdzc blog_blog-card-extra__G07HX"><span class="blog_blog-extra-tags__Rf_FB">Engineering</span><span class="blog_blog-extra-separator__OqBcN"></span><span>Feb 25, 2025</span></p><h3 class="blog_blog-card-title__OEYNA">AI for Smarter Browsing: Filtering Web Content with Pixtral, Milvus, and Browser Use</h3></a><a class="blog_blog-card__mTmhJ" title="Introducing DeepSearcher: A Local Open Source Deep Research" href="/blog/introduce-deepsearcher-a-local-open-source-deep-research.md"><div class="blog_blog-card-img__qyc2Q" style="background-image:url(https://assets.zilliz.com/Introducing_Deep_Searcher_A_Local_Open_Source_Deep_Research_4d00da5b85.png)"></div><p class="blog_blog-extra__ICdzc blog_blog-card-extra__G07HX"><span class="blog_blog-extra-tags__Rf_FB">Announcements</span><span class="blog_blog-extra-separator__OqBcN"></span><span>Feb 21, 2025</span></p><h3 class="blog_blog-card-title__OEYNA">Introducing DeepSearcher: A Local Open Source Deep Research</h3></a><nav role="navigation" aria-label="pagination" class="mx-auto flex w-full justify-center blog_paginationWrapper__yui__"><ul class="flex flex-row items-center gap-1" style="gap:5px"><li class=""><button class="w-[32px] h-[32px] p-0 m-0 flex items-center justify-center blog_paginationLink__1DboH blog_disabledNavigationLink__mlo9D"><svg xmlns="http://www.w3.org/2000/svg" width="24" height="24" viewBox="0 0 24 24" fill="none" stroke="currentColor" stroke-width="2" stroke-linecap="round" stroke-linejoin="round" class="lucide lucide-chevron-left h-4 w-4"><path d="m15 18-6-6 6-6"></path></svg></button></li><li class=""><a aria-current="page" class="inline-flex items-center justify-center whitespace-nowrap rounded-md text-sm font-medium ring-offset-white transition-colors focus-visible:outline-none focus-visible:ring-2 focus-visible:ring-slate-950 focus-visible:ring-offset-2 disabled:pointer-events-none disabled:opacity-50 dark:ring-offset-slate-950 dark:focus-visible:ring-slate-300 font-mono border border-slate-200 bg-white hover:bg-slate-100 hover:text-slate-900 dark:border-slate-800 dark:bg-slate-950 dark:hover:bg-slate-800 dark:hover:text-slate-50 h-10 w-10 blog_paginationLink__1DboH blog_activePaginationLink__GSwbX" href="/blog">1</a></li><li class=""><a class="inline-flex items-center justify-center whitespace-nowrap rounded-md text-sm font-medium ring-offset-white transition-colors focus-visible:outline-none focus-visible:ring-2 focus-visible:ring-slate-950 focus-visible:ring-offset-2 disabled:pointer-events-none disabled:opacity-50 dark:ring-offset-slate-950 dark:focus-visible:ring-slate-300 font-mono hover:bg-slate-100 hover:text-slate-900 dark:hover:bg-slate-800 dark:hover:text-slate-50 h-10 w-10 blog_paginationLink__1DboH" href="/blog?page=2">2</a></li><li class=""><a class="inline-flex items-center justify-center whitespace-nowrap rounded-md text-sm font-medium ring-offset-white transition-colors focus-visible:outline-none focus-visible:ring-2 focus-visible:ring-slate-950 focus-visible:ring-offset-2 disabled:pointer-events-none disabled:opacity-50 dark:ring-offset-slate-950 dark:focus-visible:ring-slate-300 font-mono hover:bg-slate-100 hover:text-slate-900 dark:hover:bg-slate-800 dark:hover:text-slate-50 h-10 w-10 blog_paginationLink__1DboH" href="/blog?page=3">3</a></li><li class=""><span aria-hidden="true" class="flex h-9 w-9 items-end p-[6px] justify-center"><svg xmlns="http://www.w3.org/2000/svg" width="24" height="24" viewBox="0 0 24 24" fill="none" stroke="currentColor" stroke-width="2" stroke-linecap="round" stroke-linejoin="round" class="lucide lucide-ellipsis h-4 w-4"><circle cx="12" cy="12" r="1"></circle><circle cx="19" cy="12" r="1"></circle><circle cx="5" cy="12" r="1"></circle></svg><span class="sr-only">More pages</span></span></li><li class=""><a class="inline-flex items-center justify-center whitespace-nowrap rounded-md text-sm font-medium ring-offset-white transition-colors focus-visible:outline-none focus-visible:ring-2 focus-visible:ring-slate-950 focus-visible:ring-offset-2 disabled:pointer-events-none disabled:opacity-50 dark:ring-offset-slate-950 dark:focus-visible:ring-slate-300 font-mono hover:bg-slate-100 hover:text-slate-900 dark:hover:bg-slate-800 dark:hover:text-slate-50 h-10 w-10 blog_paginationLink__1DboH" href="/blog?page=18">18</a></li><li class=""><a class="inline-flex items-center justify-center whitespace-nowrap rounded-md text-sm font-medium ring-offset-white transition-colors focus-visible:outline-none focus-visible:ring-2 focus-visible:ring-slate-950 focus-visible:ring-offset-2 disabled:pointer-events-none disabled:opacity-50 dark:ring-offset-slate-950 dark:focus-visible:ring-slate-300 font-mono hover:bg-slate-100 hover:text-slate-900 dark:hover:bg-slate-800 dark:hover:text-slate-50 h-10 px-4 py-2 gap-1 pr-2.5 blog_paginationLink__1DboH" aria-label="Go to next page" href="/blog?page=2"><svg xmlns="http://www.w3.org/2000/svg" width="24" height="24" viewBox="0 0 24 24" fill="none" stroke="currentColor" stroke-width="2" stroke-linecap="round" stroke-linejoin="round" class="lucide lucide-chevron-right h-4 w-4"><path d="m9 18 6-6-6-6"></path></svg></a></li></ul></nav></section><section class="blog_ai-service__Tnhen"><div class="inkeep-custom-trigger-wrapper"><div class="blog_ai-service-wrapper__hp09B"><div class="blog_ai-service-logo__kp_aw" style="background-image:url(/images/ai-bird.png)"></div><div class="blog_ai-service-main__ZLIQZ"><h3 class="blog_ai-service-title__6Mdi_">Ask me anything about Milvus!</h3><p class="blog_ai-service-desc__D7eon">An AI assistant trained on documentation, help articles, and other content.</p></div><svg width="24" height="25" viewBox="0 0 24 25" fill="none" xmlns="http://www.w3.org/2000/svg" class="blog_ai-service-icon__K2TQc"><path d="M3 13.1621H9V11.1621H3V2.00781C3 1.73167 3.22386 1.50781 3.5 1.50781C3.58425 1.50781 3.66714 1.5291 3.74096 1.5697L22.2034 11.724C22.4454 11.8571 22.5337 12.1611 22.4006 12.4031C22.3549 12.4862 22.2865 12.5546 22.2034 12.6003L3.74096 22.7546C3.499 22.8877 3.19497 22.7994 3.06189 22.5575C3.02129 22.4836 3 22.4008 3 22.3165V13.1621Z" fill="currentColor"></path></svg></div></div></section><section class="index_zilliz-adv__Zvk5V"><div class="index_zilliz-adv-main__ajAaY"><h6 class="index_zilliz-adv-small-title__hCTTs">Zilliz Cloud</h6><h3 class="index_zilliz-adv-title___uc5u">Hassle-free and 10x faster than Milvus</h3><ul class="index_zilliz-adv-features__Wi4jc"><li class="index_zilliz-adv-features-item__zANFX"><svg width="20" height="21" viewBox="0 0 20 21" fill="none" xmlns="http://www.w3.org/2000/svg" class="index_zilliz-adv-features-item-icon__ZKiYr"><path d="M1 10.1137L4.5 14.2224L11.5 7.83105" stroke="currentColor" stroke-width="2"></path></svg>Use-case optimized compute units for your workload</li><li class="index_zilliz-adv-features-item__zANFX"><svg width="20" height="21" viewBox="0 0 20 21" fill="none" xmlns="http://www.w3.org/2000/svg" class="index_zilliz-adv-features-item-icon__ZKiYr"><path d="M1 10.1137L4.5 14.2224L11.5 7.83105" stroke="currentColor" stroke-width="2"></path></svg>Enterprise-grade security</li><li class="index_zilliz-adv-features-item__zANFX"><svg width="20" height="21" viewBox="0 0 20 21" fill="none" xmlns="http://www.w3.org/2000/svg" class="index_zilliz-adv-features-item-icon__ZKiYr"><path d="M1 10.1137L4.5 14.2224L11.5 7.83105" stroke="currentColor" stroke-width="2"></path></svg>Available on AWS, GCP, and Azure</li></ul><a target="_blank" class="index_zilliz-adv-btn__8VcHR" href="https://cloud.zilliz.com/signup?utm_source=milvusio&utm_medium=referral&utm_campaign=bottom_banner&utm_content=blog">Try Fully Managed Milvus Free<svg xmlns="http://www.w3.org/2000/svg" width="14" height="14" viewBox="0 0 14 14" fill="none"><path d="M3.5 11L11.0833 3.41669M11.0833 3.41669V10.6967M11.0833 3.41669H3.80333" stroke="#FFFFFF" stroke-linecap="round" stroke-linejoin="round"></path></svg></a></div><div class="index_zilliz-adv-logo__ljoQR" style="background-image:url(/images/supported.png)"></div></section><section class="blog_authors__yndC1"><header class="blog_authors-header__qH1_7"><h2>Most Contributing Authors</h2></header><div class="blog_authors-list__meDgP"><div class="blog_author__Z7ez4"><div class="blog_author-avatar__cPq06" style="background-image:url(/images/authors/stefanwebb.png)"></div><div><h4 class="blog_author-name__kl4zs">Stefan Webb</h4><p class="blog_author-title__Objc2">Developer Advocate, Zilliz</p></div></div><div class="blog_author__Z7ez4"><div class="blog_author-avatar__cPq06" style="background-image:url(/images/authors/davidwang.png)"></div><div><h4 class="blog_author-name__kl4zs">David Wang</h4><p class="blog_author-title__Objc2">Algorithm Engineer, Zilliz</p></div></div><div class="blog_author__Z7ez4"><div class="blog_author-avatar__cPq06" style="background-image:url(/images/authors/jiangchen.png)"></div><div><h4 class="blog_author-name__kl4zs">Jiang Chen</h4><p class="blog_author-title__Objc2">Engineering Lead, Zilliz</p></div></div></div></section></div></section></main><footer class="responsive_homeContainer__9_COi min-h-[390px] box-border bg-[#fff] border-t-[1px] border-solid border-[#ECECEE] font-mono"><div class="py-[80px]"><div class="flex flex-col lg:flex-row justify-between space-y-8 lg:space-y-0 lg:space-x-16"><div class="flex flex-col items-center max-phone:items-start sm:items-start lg:items-start flex-shrink-0 flex-grow-0 flex-[390px]"><img src="/images/layout/lf-ai-logo.svg" alt="LF_AI" class="h-[16px] w-[auto] mb-[10px]"/><div class="flex items-center gap-[8px] "><a href="/"><img alt="Milvus" class="h-[40px] w-[auto] max-phone:h-[30px]" src="/images/layout/milvus-logo.svg"/></a><span class="h-[40px] w-[1px] bg-black3"></span><a href="https://zilliz.com/" target="_blank" rel="noreferrer noopener"><img src="/images/layout/zilliz-logo.svg" alt="Zilliz" class="h-[50px] w-[auto] max-phone:h-[40px]"/></a></div><div class="flex items-center gap-[8px] mt-[12px] text-[14px] font-[400] leading-[1.5] text-black2 whitespace-nowrap flex-wrap">Made with Love <img width="16" height="16" src="/images/blue-heart.png" alt="Blue Heart Emoji" class="mx-[-4px]"/> by the Devs from <a href="https://zilliz.com/" target="_blank" rel="noreferrer" class="text-black2 hover:underline">Zilliz</a></div><div class="mt-[100px] w-full"><div class="index_subscribeContainer__KRyy9"><h3 class="index_title__zpjQ5">Get Milvus Updates</h3><div class="index_subscribeSection__HxBZ7"><div class="index_inputWrapper__S93JG"><div class="customInput_container__QaET_ index_customInputContainer__E0Bx2"><span></span><input class="customInput_input__LWwEQ customInput_fullWidth__LQFVu index_customInput__jCRxv" placeholder="Email"/></div><p class="index_errorMessage__FXqEW"></p></div><button class="index_linkButton__KW4cr index_customSubscribeButton__qseMJ whitespace-nowrap index_contained__3m7DK index_primaryColor__GTYfK index_largeSize__w54YY">Subscribe</button></div></div></div><div class="flex mt-[40px] space-x-[12px]"><div class="flex items-center justify-start gap-[12px]"><a target="_blank" rel="noopener noreferrer" href="https://github.com/milvus-io/milvus" class="index_linkButton__KW4cr index_iconButton__2Kcxl index_text__efKLY index_primaryColor__GTYfK index_mediumSize__QISKx"><svg width="60" height="60" viewBox="0 0 60 60" fill="none" xmlns="http://www.w3.org/2000/svg"><rect x="0.5" y="0.5" width="59" height="59" rx="11.5" fill="white"></rect><rect x="0.5" y="0.5" width="59" height="59" rx="11.5" stroke="#ECECEE"></rect><path fill-rule="evenodd" clip-rule="evenodd" d="M30.3035 18C23.5058 18 18 23.5058 18 30.3035C18 35.7478 21.5219 40.3463 26.4125 41.9765C27.0277 42.0842 27.2584 41.715 27.2584 41.3921C27.2584 41.0999 27.243 40.131 27.243 39.1005C24.1518 39.6696 23.352 38.347 23.106 37.6549C22.9675 37.3012 22.3678 36.2092 21.8449 35.917C21.4142 35.6863 20.7991 35.1173 21.8295 35.1019C22.7984 35.0865 23.4904 35.9939 23.7211 36.363C24.8285 38.2239 26.5971 37.701 27.3045 37.3781C27.4122 36.5783 27.7352 36.04 28.0889 35.7325C25.3514 35.4249 22.4908 34.3637 22.4908 29.6576C22.4908 28.3196 22.9675 27.2123 23.7519 26.351C23.6289 26.0434 23.1982 24.7823 23.8749 23.0906C23.8749 23.0906 24.9054 22.7676 27.2584 24.3517C28.2427 24.0749 29.2885 23.9365 30.3343 23.9365C31.3801 23.9365 32.4259 24.0749 33.4102 24.3517C35.7632 22.7522 36.7936 23.0906 36.7936 23.0906C37.4703 24.7823 37.0397 26.0434 36.9167 26.351C37.701 27.2123 38.1778 28.3042 38.1778 29.6576C38.1778 34.3791 35.3018 35.4249 32.5643 35.7325C33.0103 36.1169 33.3948 36.8552 33.3948 38.0086C33.3948 39.6542 33.3794 40.9768 33.3794 41.3921C33.3794 41.715 33.6101 42.0995 34.2253 41.9765C39.0852 40.3463 42.607 35.7325 42.607 30.3035C42.607 23.5058 37.1012 18 30.3035 18Z" fill="#00131A"></path></svg></a><a target="_blank" rel="noopener noreferrer" href="https://twitter.com/milvusio" class="index_linkButton__KW4cr index_iconButton__2Kcxl index_text__efKLY index_primaryColor__GTYfK index_mediumSize__QISKx"><svg width="60" height="60" viewBox="0 0 60 60" fill="none" xmlns="http://www.w3.org/2000/svg"><rect x="0.5" y="0.5" width="59" height="59" rx="11.5" fill="white"></rect><rect x="0.5" y="0.5" width="59" height="59" rx="11.5" stroke="#ECECEE"></rect><path d="M36.1088 20H39.4399L32.1624 28.4718L40.7239 40H34.0203L28.7698 33.0082L22.7621 40H19.429L27.213 30.9385L19 20H25.8737L30.6197 26.3908L36.1088 20ZM34.9397 37.9692H36.7855L24.8708 21.9241H22.89L34.9397 37.9692Z" fill="#00131A"></path></svg></a><a target="_blank" rel="noopener noreferrer" href="https://milvus.io/discord" class="index_linkButton__KW4cr index_iconButton__2Kcxl index_text__efKLY index_primaryColor__GTYfK index_mediumSize__QISKx"><svg width="60" height="60" viewBox="0 0 60 60" fill="none" xmlns="http://www.w3.org/2000/svg"><rect x="0.5" y="0.5" width="59" height="59" rx="11.5" fill="white"></rect><rect x="0.5" y="0.5" width="59" height="59" rx="11.5" stroke="#ECECEE"></rect><path d="M38.6777 21.675C37.0212 20.8925 35.25 20.3238 33.3982 20C33.1708 20.4151 32.9051 20.9735 32.7219 21.4176C30.7534 21.1187 28.803 21.1187 26.8708 21.4176C26.6876 20.9735 26.4159 20.4151 26.1864 20C24.3327 20.3238 22.5594 20.8946 20.9029 21.6791C17.5617 26.7767 16.6559 31.7476 17.1088 36.648C19.3249 38.3188 21.4725 39.3338 23.5839 39.9979C24.1052 39.2736 24.5701 38.5035 24.9707 37.692C24.2078 37.3994 23.4772 37.0382 22.7869 36.6189C22.97 36.482 23.1492 36.3387 23.3222 36.1914C27.533 38.1798 32.108 38.1798 36.2684 36.1914C36.4435 36.3387 36.6226 36.482 36.8038 36.6189C36.1114 37.0403 35.3788 37.4014 34.6159 37.6941C35.0165 38.5035 35.4794 39.2757 36.0027 40C38.1161 39.3358 40.2658 38.3209 42.4818 36.648C43.0132 30.9672 41.5741 26.0419 38.6777 21.675ZM25.5443 33.6343C24.2803 33.6343 23.2437 32.4429 23.2437 30.9921C23.2437 29.5413 24.2582 28.3479 25.5443 28.3479C26.8305 28.3479 27.8671 29.5392 27.8449 30.9921C27.8469 32.4429 26.8305 33.6343 25.5443 33.6343ZM34.0463 33.6343C32.7823 33.6343 31.7457 32.4429 31.7457 30.9921C31.7457 29.5413 32.7601 28.3479 34.0463 28.3479C35.3325 28.3479 36.3691 29.5392 36.3469 30.9921C36.3469 32.4429 35.3325 33.6343 34.0463 33.6343Z" fill="#00131A"></path></svg></a><a target="_blank" rel="noopener noreferrer" href="https://www.linkedin.com/company/the-milvus-project/" class="index_linkButton__KW4cr index_iconButton__2Kcxl index_text__efKLY index_primaryColor__GTYfK index_mediumSize__QISKx"><svg width="60" height="60" viewBox="0 0 60 60" fill="none" xmlns="http://www.w3.org/2000/svg"><rect x="0.5" y="0.5" width="59" height="59" rx="11.5" fill="white"></rect><rect x="0.5" y="0.5" width="59" height="59" rx="11.5" stroke="#ECECEE"></rect><path fill-rule="evenodd" clip-rule="evenodd" d="M23.8124 19.4062C23.8124 20.7351 22.7351 21.8124 21.4062 21.8124C20.0773 21.8124 19 20.7351 19 19.4062C19 18.0773 20.0773 17 21.4062 17C22.7351 17 23.8124 18.0773 23.8124 19.4062ZM37.3204 24.0524C37.303 24.0469 37.2859 24.0412 37.2688 24.0356C37.2346 24.0243 37.2003 24.013 37.1637 24.0029C37.0977 23.9877 37.0317 23.9754 36.9643 23.9644C36.7031 23.9121 36.4171 23.875 36.0816 23.875C33.2134 23.875 31.3943 25.9608 30.7948 26.7666V23.875H25.8751V38.9997H30.7948V30.7499C30.7948 30.7499 34.5127 25.5717 36.0816 29.3749V38.9997H40.9999V28.7933C40.9999 26.5081 39.4338 24.6037 37.3204 24.0524ZM19.0001 23.8753H23.9198V39.0001H19.0001V23.8753Z" fill="#00131A"></path></svg></a><a target="_blank" rel="noopener noreferrer" href="https://www.youtube.com/channel/UCMCo_F7pKjMHBlfyxwOPw-g" class="index_linkButton__KW4cr index_iconButton__2Kcxl index_text__efKLY index_primaryColor__GTYfK index_mediumSize__QISKx"><svg width="60" height="60" viewBox="0 0 60 60" fill="none" xmlns="http://www.w3.org/2000/svg"><rect x="0.5" y="0.5" width="59" height="59" rx="11.5" fill="white"></rect><rect x="0.5" y="0.5" width="59" height="59" rx="11.5" stroke="#ECECEE"></rect><path fill-rule="evenodd" clip-rule="evenodd" d="M38.866 21.1495C40.4244 21.2373 41.1898 21.468 41.895 22.7225C42.6295 23.9754 43 26.1334 43 29.9342V29.9391V29.9473C43 33.7303 42.6295 35.9045 41.8966 37.1444C41.1914 38.3989 40.426 38.6264 38.8676 38.732C37.3092 38.8214 33.3946 38.875 30.0033 38.875C26.6054 38.875 22.6891 38.8214 21.1324 38.7304C19.5773 38.6248 18.8119 38.3973 18.1001 37.1427C17.3737 35.9029 17 33.7286 17 29.9456V29.9424V29.9375V29.9326C17 26.1334 17.3737 23.9754 18.1001 22.7225C18.8119 21.4664 19.5789 21.2373 21.134 21.1479C22.6891 21.0439 26.6054 21 30.0033 21C33.3946 21 37.3092 21.0439 38.866 21.1495ZM34.875 29.9375L26.75 25.0625V34.8125L34.875 29.9375Z" fill="black"></path></svg></a></div></div><p class="mt-3 text-sm text-black1">Copyright © Milvus. 2025 All rights reserved.</p></div><div class="grid grid-cols-2 gap-8 text-sm sm:gap-x-0 flex-shrink-0 flex-grow-0 flex-[420px] max-tablet:flex-auto"><div><h3 class="text-[16px] font-[500] leading-[24px]">Resources</h3><ul class="mt-[20px] space-y-[8px]"><li class="list-none"><a class="text-[14px] font-[400] leading-[21px] text-black1 hover:opacity-[0.7]" href="/docs">Docs</a></li><li class="list-none"><a class="text-[14px] font-[400] leading-[21px] text-black1 hover:opacity-[0.7]" href="/blog">Blog</a></li><li class="list-none"><a class="inline-flex items-center gap-[4px] text-[14px] font-[400] leading-[21px] text-black1 hover:opacity-[0.7]" href="https://cloud.zilliz.com/signup?utm_source=milvusio&utm_medium=referral&utm_campaign=footer&utm_content=blog" target="_blank" rel="noopener noreferrer">Managed Milvus<svg xmlns="http://www.w3.org/2000/svg" width="14" height="14" viewBox="0 0 14 14" fill="none"><path d="M3.5 11L11.0833 3.41669M11.0833 3.41669V10.6967M11.0833 3.41669H3.80333" stroke="#00131A" stroke-linecap="round" stroke-linejoin="round"></path></svg></a></li><li class="list-none"><a class="text-[14px] font-[400] leading-[21px] text-black1 hover:opacity-[0.7]" href="/contact">Contact Us</a></li><li class="list-none"><a class="text-[14px] font-[400] leading-[21px] text-black1 hover:opacity-[0.7] text-transparent" href="/ai-quick-reference">AI Quick Reference </a></li></ul></div><div><h3 class="text-[16px] font-[500] leading-[24px]">Tutorials</h3><ul class="mt-[20px] space-y-[8px]"><li class="list-none"><a class="text-[14px] font-[400] leading-[21px] text-black1 hover:opacity-[0.7]" href="/bootcamp">Bootcamps</a></li><li class="list-none"><a class="text-[14px] font-[400] leading-[21px] text-black1 hover:opacity-[0.7]" href="/milvus-demos">Demo</a></li><li class="list-none"><a class="inline-flex items-center gap-[4px] text-[14px] font-[400] leading-[21px] text-black1 hover:opacity-[0.7]" href="https://www.youtube.com/c/MilvusVectorDatabase" target="_blank" rel="noopener noreferrer">Video</a></li></ul></div><div><h3 class="text-[16px] font-[500] leading-[24px]">Tools</h3><ul class="mt-[20px] space-y-[8px]"><li class="list-none"><a class="inline-flex items-center gap-[4px] text-[14px] font-[400] leading-[21px] text-black1 hover:opacity-[0.7]" href="https://github.com/zilliztech/attu" target="_blank" rel="noopener noreferrer">Attu</a></li><li class="list-none"><a class="inline-flex items-center gap-[4px] text-[14px] font-[400] leading-[21px] text-black1 hover:opacity-[0.7]" href="https://github.com/zilliztech/milvus_cli" target="_blank" rel="noopener noreferrer">Milvus CLI</a></li><li class="list-none"><a class="text-[14px] font-[400] leading-[21px] text-black1 hover:opacity-[0.7]" href="/tools/sizing">Milvus Sizing Tool</a></li><li class="list-none"><a class="inline-flex items-center gap-[4px] text-[14px] font-[400] leading-[21px] text-black1 hover:opacity-[0.7]" href="https://github.com/zilliztech/milvus-backup" target="_blank" rel="noopener noreferrer">Milvus Backup Tool</a></li><li class="list-none"><a class="inline-flex items-center gap-[4px] text-[14px] font-[400] leading-[21px] text-black1 hover:opacity-[0.7]" href="https://github.com/zilliztech/vts" target="_blank" rel="noopener noreferrer">Vector Transport Service (VTS)</a></li></ul></div><div><h3 class="text-[16px] font-[500] leading-[24px]">Community</h3><ul class="mt-[20px] space-y-[8px]"><li class="list-none"><a class="text-[14px] font-[400] leading-[21px] text-black1 hover:opacity-[0.7]" href="/community">Get Involved</a></li><li class="list-none"><a class="inline-flex items-center gap-[4px] text-[14px] font-[400] leading-[21px] text-black1 hover:opacity-[0.7]" href="https://milvus.io/discord" target="_blank" rel="noopener noreferrer">Discord<svg xmlns="http://www.w3.org/2000/svg" width="14" height="14" viewBox="0 0 14 14" fill="none"><path d="M3.5 11L11.0833 3.41669M11.0833 3.41669V10.6967M11.0833 3.41669H3.80333" stroke="#00131A" stroke-linecap="round" stroke-linejoin="round"></path></svg></a></li><li class="list-none"><a class="inline-flex items-center gap-[4px] text-[14px] font-[400] leading-[21px] text-black1 hover:opacity-[0.7]" href="https://github.com/milvus-io/milvus" target="_blank" rel="noopener noreferrer">Github<svg xmlns="http://www.w3.org/2000/svg" width="14" height="14" viewBox="0 0 14 14" fill="none"><path d="M3.5 11L11.0833 3.41669M11.0833 3.41669V10.6967M11.0833 3.41669H3.80333" stroke="#00131A" stroke-linecap="round" stroke-linejoin="round"></path></svg></a></li></ul></div></div></div></div></footer></div><script id="__NEXT_DATA__" type="application/json">{"props":{"pageProps":{"locale":"en","blogList":[{"id":"parse-is-hard-solve-semantic-understanding-with-mistral-ocr-and-milvus.md","author":"Stephen Batifol","desc":"Tackling the challenge head-on using the powerful combo of Mistral OCR and Milvus Vector DB, turning your doc parsing nightmares into a calm dream with searchable, semantically meaningful vector embeddings.","canonicalUrl":"https://milvus.io/blog/parse-is-hard-solve-semantic-understanding-with-mistral-ocr-and-milvus.md","date":"2025-04-03T00:00:00.000Z","cover":"https://assets.zilliz.com/Parsing_is_Hard_Solving_Semantic_Understanding_with_Mistral_OCR_and_Milvus_316ac013b6.png","tags":["Engineering"],"href":"/blog/parse-is-hard-solve-semantic-understanding-with-mistral-ocr-and-milvus.md","content":"\n\nLet's face it: parsing documents is hard—really hard. PDFs, images, reports, tables, messy handwriting; they're packed with valuable information that your users want to search for, but extracting that information and express that accurately in your search index is like solving a puzzle where the pieces keep changing shape: you thought you've solved it with an extra line of code but tomorrow a new doc gets ingested and you find another corner case to deal with.\n\nIn this post, we'll tackle this challenge head-on using the powerful combo of Mistral OCR and Milvus Vector DB, turning your doc parsing nightmares into a calm dream with searchable, semantically meaningful vector embeddings. \n\n\n## Why Rule-based Parsing Just Won't Cut It\n\nIf you've ever struggled with standard OCR tools, you probably know that they have all sorts of issues:\n\n- **Complex layouts**: Tables, lists, multi-column formats -- they can break or pose issues to most parsers.\n- **Semantic ambiguity**: Keywords alone don't tell you if \"apple\" means fruit or company.\n- The chanllege of scale and cost: Processing thousands of documents becomes painfully slow.\n\nWe need a smarter, more systematic approach that doesn’t just extract text—it _understands_ the content. And that’s exactly where Mistral OCR and Milvus come in.\n\n\n## Meet Your Dream Team\n\n### Mistral OCR: More than just text extraction\n\nMistral OCR isn’t your average OCR tool. It's designed to tackle a wide range of documents.\n\n- **Deep Understanding of Complex Documents**: Whether it's embedded images, mathematical equations, or tables, it can understand it all with a very high accuracy.\n- **Keeps original layouts:** Not only does it understand the different layouts in the documents, it also keeps the original layouts and structure intact. On top of that, it's also capable of parsing multi-page documents.\n- **Multilingual and Multimodal Mastery**: From English to Hindi to Arabic, Mistral OCR can comprehend documents across thousands of languages and scripts, making it invaluable for applications targeting a global user base.\n\n\n### Milvus: Your Vector Database Built for Scale\n\n- **Billion+ Scale**: [Milvus](https://milvus.io/) can scale to billions of vectors, making it perfect for storing large-scale documents.\n- **Full-Text Search: In addition to supporting dense vector embeddings**, Milvus also supports Full Text Search. Making it easy to run queries using text and get better results for your RAG system.\n\n\n## Examples:\n\nLet's take this handwritten note in English, for example. Using a regular OCR tool to extract this text would be a very hard task.\n\n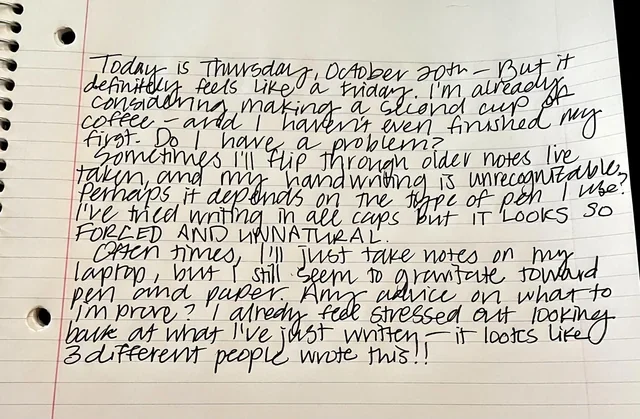\n\nWe process it with Mistral OCR\n\n```python\napi_key = os.getenv(\"MISTRAL_API_KEY\")\nclient = Mistral(api_key=api_key)\n\nurl = \"https://preview.redd.it/ocr-for-handwritten-documents-v0-os036yiv9xod1.png?width=640\u0026format=png\u0026auto=webp\u0026s=29461b68383534a3c1bf76cc9e36a2ba4de13c86\"\nresult = client.ocr.process(\n model=ocr_model, document={\"type\": \"image_url\", \"image_url\": url}\n )\nprint(f\"Result: {result.pages[0].markdown}\")\n```\n\nAnd we get the following output. It can recognize handwritten text well. We can see that it even keeps the capitalized format of the words \"FORCED AND UNNATURAL\"!\n\n```Markdown\nToday is Thursday, October 20th - But it definitely feels like a Friday. I'm already considering making a second cup of coffee - and I haven't even finished my first. Do I have a problem?\nSometimes I'll fly through older notes I've taken, and my handwriting is unrecamptable. Perhaps it depends on the type of pen I use. I've tried writing in all cups but it looks so FORCED AND UNNATURAL.\nOften times, I'll just take notes on my lapten, but I still seem to ermittelt forward pen and paper. Any advice on what to\nimprove? I already feel stressed at looking back at what I've just written - it looks like I different people wrote this!\n```\n\nNow we can then insert the text into Milvus for semantic search.\n\n```\nfrom pymilvus import MilvusClient \n\nCOLLECTION_NAME = \"document_ocr\"\n\nmilvus_client = MilvusClient(uri='http://localhost:19530')\n\"\"\"\nThis is where you would define the index, create a collection etc. For the sake of this example. I am skipping it. \n\nschema = CollectionSchema(...)\n\nmilvus_client.create_collection(\n collection_name=COLLECTION_NAME,\n schema=schema,\n )\n\n\"\"\"\n\nmilvus_client.insert(collection_name=COLLECTION_NAME, data=[result.pages[0].markdown])\n```\n\nBut Mistral can also understand documents in different languages or in more complex format, for example let's try this invoice in German that combines some item names in English.\n\n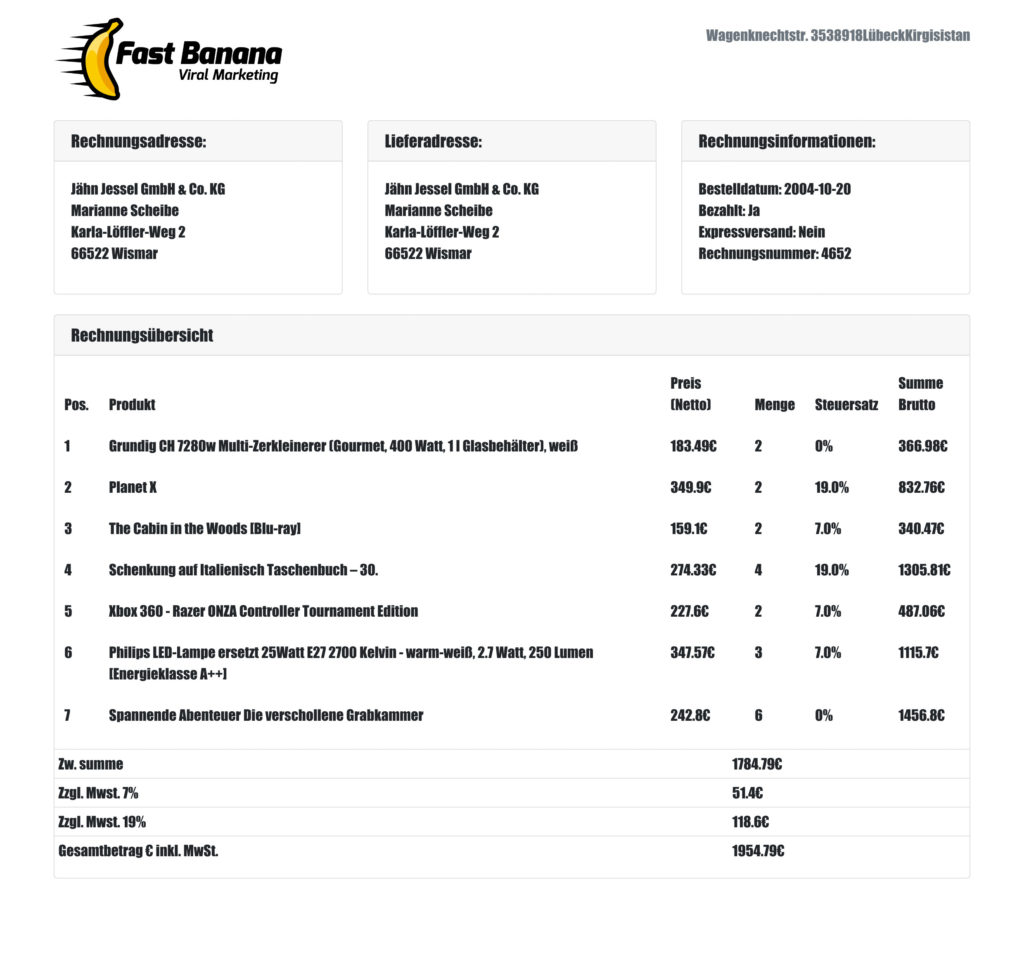\n\nMistral OCR is still capable of extracting all the information you have and it even creates the table structure in Markdown that represents the table from the scanned image.\n\n```\nRechnungsadresse:\n\nJähn Jessel GmbH a. Co. KG Marianne Scheibe Karla-Löffler-Weg 2 66522 Wismar\n\nLieferadresse:\n\nJähn Jessel GmbH a. Co. KG Marianne Scheibe Karla-Löffler-Weg 2 66522 Wismar\n\nRechnungsinformationen:\n\nBestelldatum: 2004-10-20\nBezahit: Ja\nExpressversand: Nein\nRechnungsnummer: 4652\n\nRechnungsübersicht\n\n| Pos. | Produkt | Preis \u003cbr\u003e (Netto) | Menge | Steuersatz | Summe \u003cbr\u003e Brutto |\n| :--: | :--: | :--: | :--: | :--: | :--: |\n| 1 | Grundig CH 7280w Multi-Zerkleinerer (Gourmet, 400 Watt, 11 Glasbehälter), weiß | 183.49 C | 2 | $0 \\%$ | 366.98 C |\n| 2 | Planet K | 349.9 C | 2 | $19.0 \\%$ | 832.76 C |\n| 3 | The Cabin in the Woods (Blu-ray) | 159.1 C | 2 | $7.0 \\%$ | 340.47 C |\n| 4 | Schenkung auf Italienisch Taschenbuch - 30. | 274.33 C | 4 | $19.0 \\%$ | 1305.81 C |\n| 5 | Xbox 360 - Razer 0N2A Controller Tournament Edition | 227.6 C | 2 | $7.0 \\%$ | 487.06 C |\n| 6 | Philips LED-Lampe ersetzt 25Watt E27 2700 Kelvin - warm-weiß, 2.7 Watt, 250 Lumen IEnergieklasse A++I | 347.57 C | 3 | $7.0 \\%$ | 1115.7 C |\n| 7 | Spannende Abenteuer Die verschollene Grabkammer | 242.8 C | 6 | $0 \\%$ | 1456.8 C |\n| Zw. summe | | 1784.79 C | | | |\n| Zzgl. Mwst. 7\\% | | 51.4 C | | | |\n| Zzgl. Mwst. 19\\% | | 118.6 C | | | |\n| Gesamtbetrag C inkl. MwSt. | | 1954.79 C | | | |\n```\n\n\n## Real-World Usage: A Case Study\n\nNow that we've seen that Mistral OCR can work on different documents, we could imagine how a legal firm that is drowning in case files and contracts leverage this tool. By implementing a RAG system with Mistral OCR and Milvus, what once took a paralegal countless hours, like manually scanning for specific clauses or comparing past cases, now is done by AI in only a couple of minutes.\n\n\n### Next Steps\n\nReady to extract all your content? Head over to the [notebook on GitHub](https://github.com/milvus-io/bootcamp/blob/master/bootcamp/tutorials/integration/mistral_ocr_with_milvus.ipynb) for the full example, join our [Discord](http://zilliz.com/discord) to chat with the community, and start building today! You can also check out [Mistral documentation](https://docs.mistral.ai/capabilities/document/) about their OCR model\u0026#x20;\n\nSay goodbye to parsing chaos, and hello to intelligent, scalable document understanding.\n","title":"Parsing is Hard: Solving Semantic Understanding with Mistral OCR and Milvus\n","metaData":{}},{"id":"generate-more-creative-and-curated-ghibli-style-images-with-gpt-4o-and-milvus.md","author":"Lumina Wang","desc":"Connecting Your Private Data with GPT-4o Using Milvus for More Curated Image Outputs","tags":["Engineering"],"canonicalUrl":"https://milvus.io/blog/generate-more-creative-and-curated-ghibli-style-images-with-gpt-4o-and-milvus.md","date":"2025-04-01T00:00:00.000Z","cover":"https://assets.zilliz.com/GPT_4opagephoto_5e934b89e5.png","href":"/blog/generate-more-creative-and-curated-ghibli-style-images-with-gpt-4o-and-milvus.md","content":"\n## Everyone Became an Artist Overnight with GPT-4o\n\n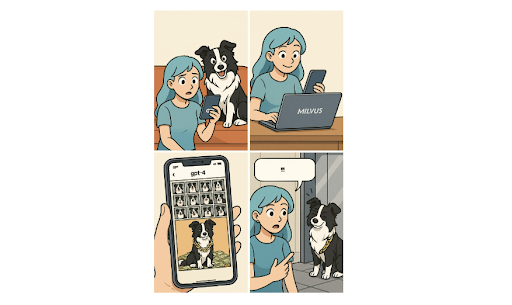\n\n_Believe it or not, the picture you just saw was AI-generated—specifically, by the newly released GPT-4o!_\n\nWhen OpenAI launched GPT-4o's native image generation feature on March 26th, no one could have predicted the creative tsunami that followed. Overnight, the internet exploded with AI-generated Ghibli-style portraits—celebrities, politicians, pets, and even users themselves were transformed into charming Studio Ghibli characters with just a few simple prompts. The demand was so overwhelming that Sam Altman himself had to \"plead\" with users to slow down, tweeting that the OpenAI's \"GPUs are melting.\"\n\n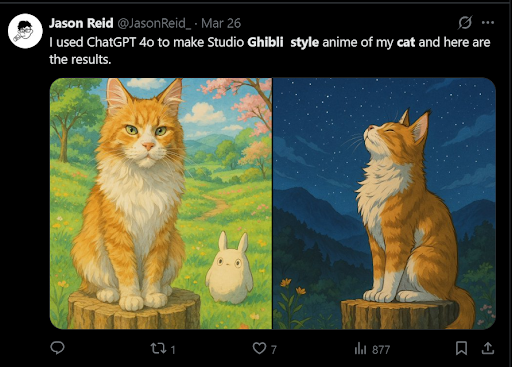\n\nExample of GPT-4o generated images (credit X\\@Jason Reid)\n\n## Why GPT-4o Changes Everything \n\nFor creative industries, this represents a paradigm shift. Tasks that once required an entire design team a whole day can now be completed in mere minutes. What makes GPT-4o different from previous image generators is **its remarkable visual consistency and intuitive interface**. It supports multi-turn conversations that let you refine images by adding elements, adjusting proportions, changing styles, or even transforming 2D into 3D—essentially putting a professional designer in your pocket.\n\nThe secret behind GPT-4o's superior performance? It’s autoregressive architecture. Unlike diffusion models (like Stable Diffusion) that degrade images into noise before reconstructing them, GPT-4o generates images sequentially—one token at a time—maintaining contextual awareness throughout the process. This fundamental architectural difference explains why GPT-4o produces more coherent results with more straightforward, more natural prompts.\n\nBut here's where things get interesting for developers: **An increasing number of signs point to a major trend—AI models themselves are becoming products. Simply put, most products that simply wrap large AI models around public domain data are at risk of being left behind.**\n\nThe true power of these advancements comes from combining general-purpose large models with **private, domain-specific data**. This combination may well be the optimal survival strategy for most companies in the era of large language models. As base models continue to evolve, the lasting competitive advantage will belong to those who can effectively integrate their proprietary datasets with these powerful AI systems.\n\nLet's explore how to connect your private data with GPT-4o using Milvus, an open-source and high-performance vector database.\n\n## Connecting Your Private Data with GPT-4o Using Milvus for More Curated Image Outputs \n\nVector databases are the key technology bridging your private data with AI models. They work by converting your content—whether images, text, or audio—into mathematical representations (vectors) that capture their meaning and characteristics. This allows for a semantic search based on similarity rather than just keywords.\n\nMilvus, as a leading open-source vector database, is particularly well-suited for connecting with generative AI tools like GPT-4o. Here's how I used it to solve a personal challenge.\n\n### Background\n\nOne day, I had this brilliant idea—turn all the mischief of my dog Cola, into a comic strip. But there was a catch: How could I sift through tens of thousands of photos from work, travels, and food adventures to find Cola's mischievous moments?\n\nThe answer? Import all my photos into Milvus and do an image search.\n\nLet's walk through the implementation step by step.\n\n#### Dependencies and Environment\n\nFirst, you need to get your environment ready with the right packages:\n\n```\npip install pymilvus --upgrade\npip install torch numpy scikit-learn pillow\n```\n\n#### Prepare the Data\n\nI'll use my photo library, which has about 30,000 photos, as the dataset in this guide. If you don't have any dataset at hand, download a sample dataset from Milvus and unzip it:\n\n```\n!wget https://github.com/milvus-io/pymilvus-assets/releases/download/imagedata/reverse_image_search.zip\n!unzip -q -o reverse_image_search.zip\n```\n\n#### Define the Feature Extractor\n\nWe'll use the ResNet-50 mode from the `timm` library to extract embedding vectors from our images. This model has been trained on millions of images and can extract meaningful features that represent the visual content.\n\n```\n import torch\n from PIL import Image\n import timm\n from sklearn.preprocessing import normalize\n from timm.data import resolve_data_config\n from timm.data.transforms_factory import create_transform\n class FeatureExtractor:\n def __init__(self, modelname):\n # Load the pre-trained model\n self.model = timm.create_model(\n modelname, pretrained=True, num_classes=0, global_pool=\"avg\"\n )\n self.model.eval()\n # Get the input size required by the model\n self.input_size = self.model.default_cfg[\"input_size\"]\n config = resolve_data_config({}, model=modelname)\n # Get the preprocessing function provided by TIMM for the model\n self.preprocess = create_transform(**config)\n def __call__(self, imagepath):\n # Preprocess the input image\n input_image = Image.open(imagepath).convert(\"RGB\") # Convert to RGB if needed\n input_image = self.preprocess(input_image)\n # Convert the image to a PyTorch tensor and add a batch dimension\n input_tensor = input_image.unsqueeze(0)\n # Perform inference\n with torch.no_grad():\n output = self.model(input_tensor)\n # Extract the feature vector\n feature_vector = output.squeeze().numpy()\n return normalize(feature_vector.reshape(1, -1), norm=\"l2\").flatten()\n```\n\n#### Create a Milvus Collection\n\nNext, we'll create a Milvus collection to store our image embeddings. Think of this as a specialized database explicitly designed for vector similarity search:\n\n```\n from pymilvus import MilvusClient\n client = MilvusClient(uri=\"example.db\")\n if client.has_collection(collection_name=\"image_embeddings\"):\n client.drop_collection(collection_name=\"image_embeddings\")\n\n client.create_collection(\n collection_name=\"image_embeddings\",\n vector_field_name=\"vector\",\n dimension=2048,\n auto_id=True,\n enable_dynamic_field=True,\n metric_type=\"COSINE\",\n )\n```\n\n**Notes on MilvusClient Parameters:**\n\n- **Local Setup:** Using a local file (e.g., `./milvus.db`) is the easiest way to get started—Milvus Lite will handle all your data.\n\n- **Scale Up:** For large datasets, set up a robust Milvus server using Docker or Kubernetes and use its URI (e.g., `http://localhost:19530`).\n\n- **Cloud Option:** If you’re into Zilliz Cloud (the fully managed service of Milvus), adjust your URI and token to match the public endpoint and API key.\n\n#### Insert Image Embeddings into Milvus\n\nNow comes the process of analyzing each image and storing its vector representation. This step might take some time depending on your dataset size, but it's a one-time process:\n\n```\n import os\n from some_module import FeatureExtractor # Replace with your feature extraction module\n extractor = FeatureExtractor(\"resnet50\")\n root = \"./train\" # Path to your dataset\n insert = True\n if insert:\n for dirpath, _, filenames in os.walk(root):\n for filename in filenames:\n if filename.endswith(\".jpeg\"):\n filepath = os.path.join(dirpath, filename)\n image_embedding = extractor(filepath)\n client.insert(\n \"image_embeddings\",\n {\"vector\": image_embedding, \"filename\": filepath},\n )\n```\n\n#### Conduct an Image Search\n\nWith our database populated, we can now search for similar images. This is where the magic happens—we can find visually similar photos using vector similarity:\n\n```\n from IPython.display import display\n from PIL import Image\n query_image = \"./search-image.jpeg\" # The image you want to search with\n results = client.search(\n \"image_embeddings\",\n data=[extractor(query_image)],\n output_fields=[\"filename\"],\n search_params={\"metric_type\": \"COSINE\"},\n limit=6, # Top-k results\n )\n images = []\n for result in results:\n for hit in result[:10]:\n filename = hit[\"entity\"][\"filename\"]\n img = Image.open(filename)\n img = img.resize((150, 150))\n images.append(img)\n width = 150 * 3\n height = 150 * 2\n concatenated_image = Image.new(\"RGB\", (width, height))\n for idx, img in enumerate(images):\n x = idx % 5\n y = idx // 5\n concatenated_image.paste(img, (x * 150, y * 150))\n\n display(\"query\")\n display(Image.open(query_image).resize((150, 150)))\n display(\"results\")\n display(concatenated_image)\n```\n\n**The returned images are shown as below:** \n\n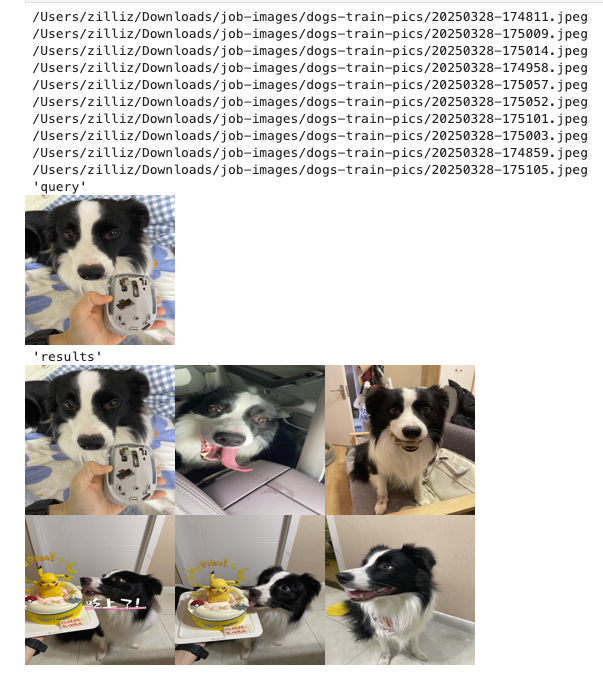\n\n### Combine Vector Search with GPT-4o: Generating Ghibli-Style Images with Images Returned by Milvus \n\nNow comes the exciting part: using our image search results as input for GPT-4o to generate creative content. In my case, I wanted to create comic strips featuring my dog Cola based on photos I've taken.\n\nThe workflow is simple but powerful:\n\n1. Use vector search to find relevant images of Cola from my collection\n\n2. Feed these images to GPT-4o with creative prompts\n\n3. Generate unique comics based on visual inspiration\n\nHere are some examples of what this combination can produce:\n\n**The prompts I use:** \n\n- _\"Generate a four-panel, full-color, hilarious comic strip featuring a Border Collie caught gnawing on a mouse—with an awkward moment when the owner finds out.\"\\\n _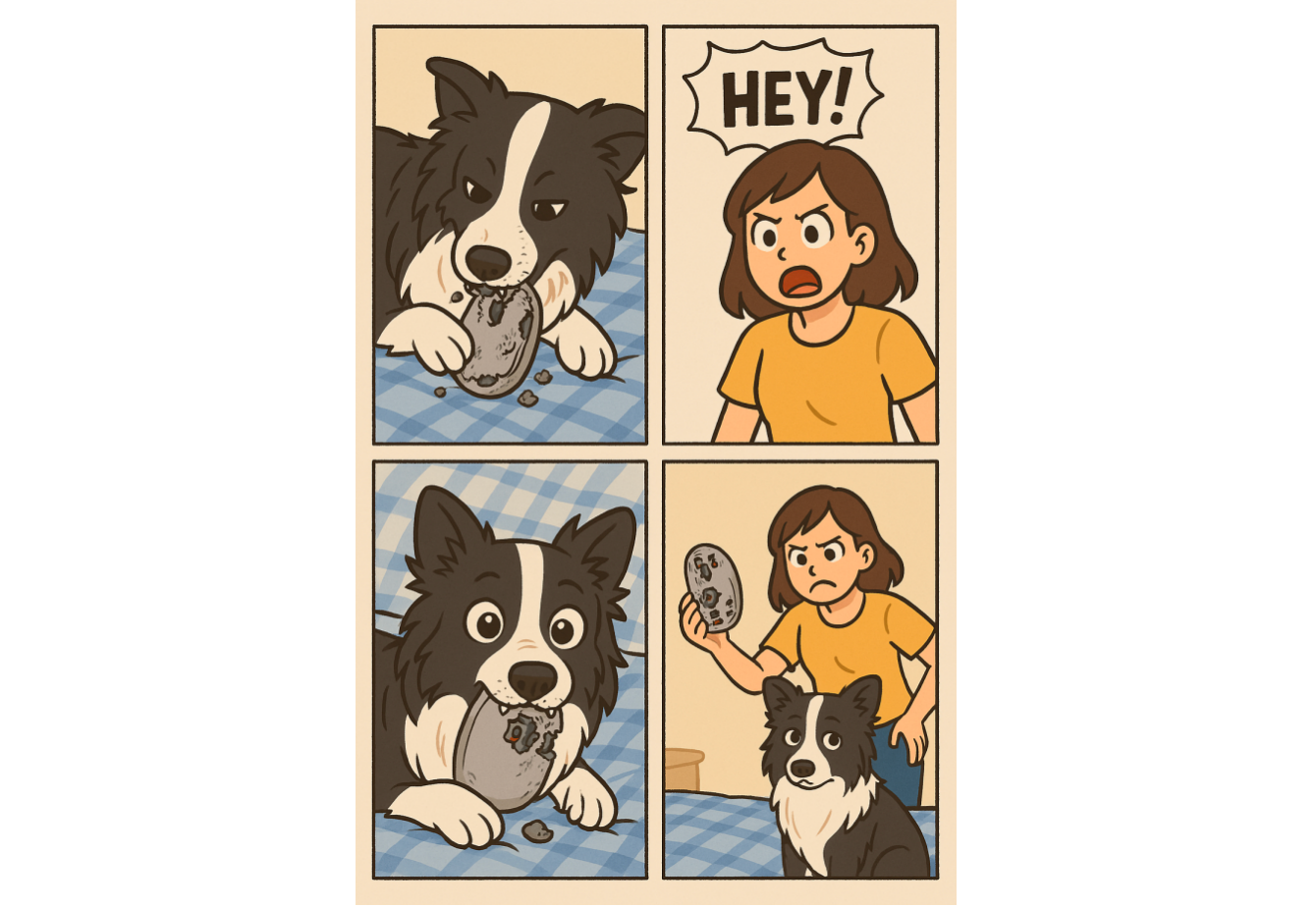__\n\n- _\"Draw a comic where this dog rocks a cute outfit.\"\\\n _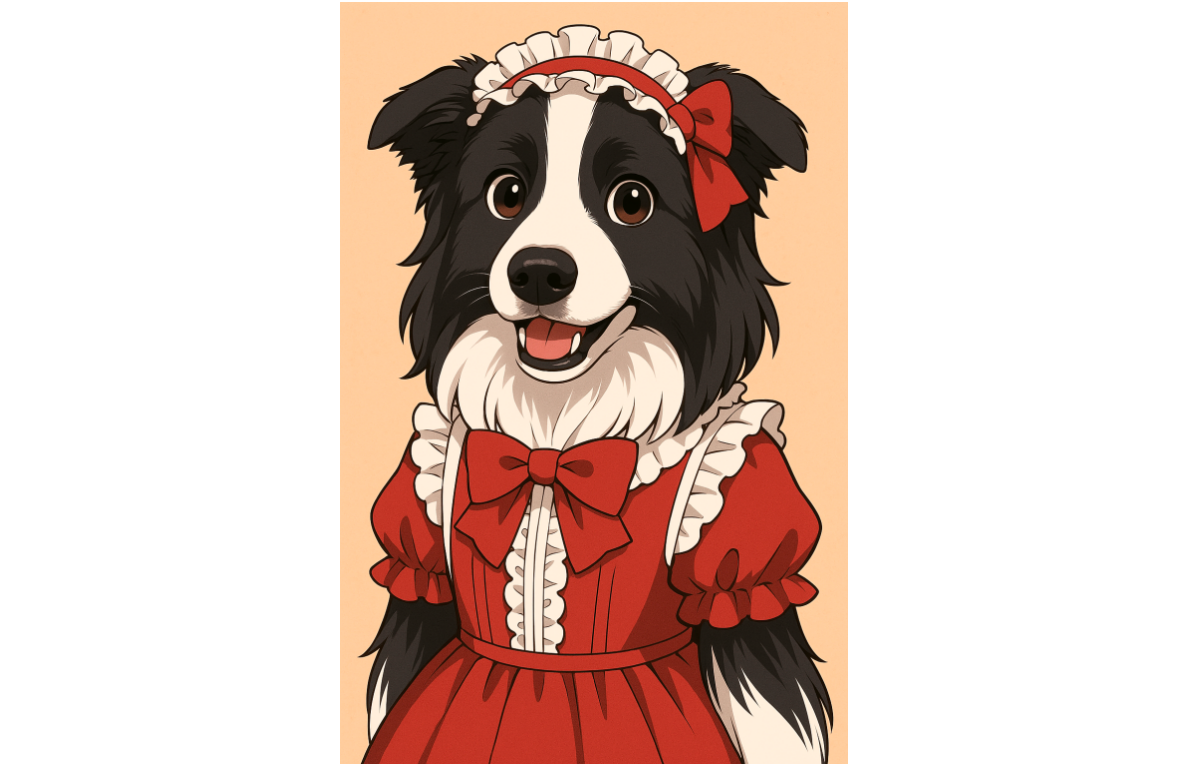__\n\n- _\"Using this dog as the model, create a comic strip of it attending Hogwarts School of Witchcraft and Wizardry.\"\\\n _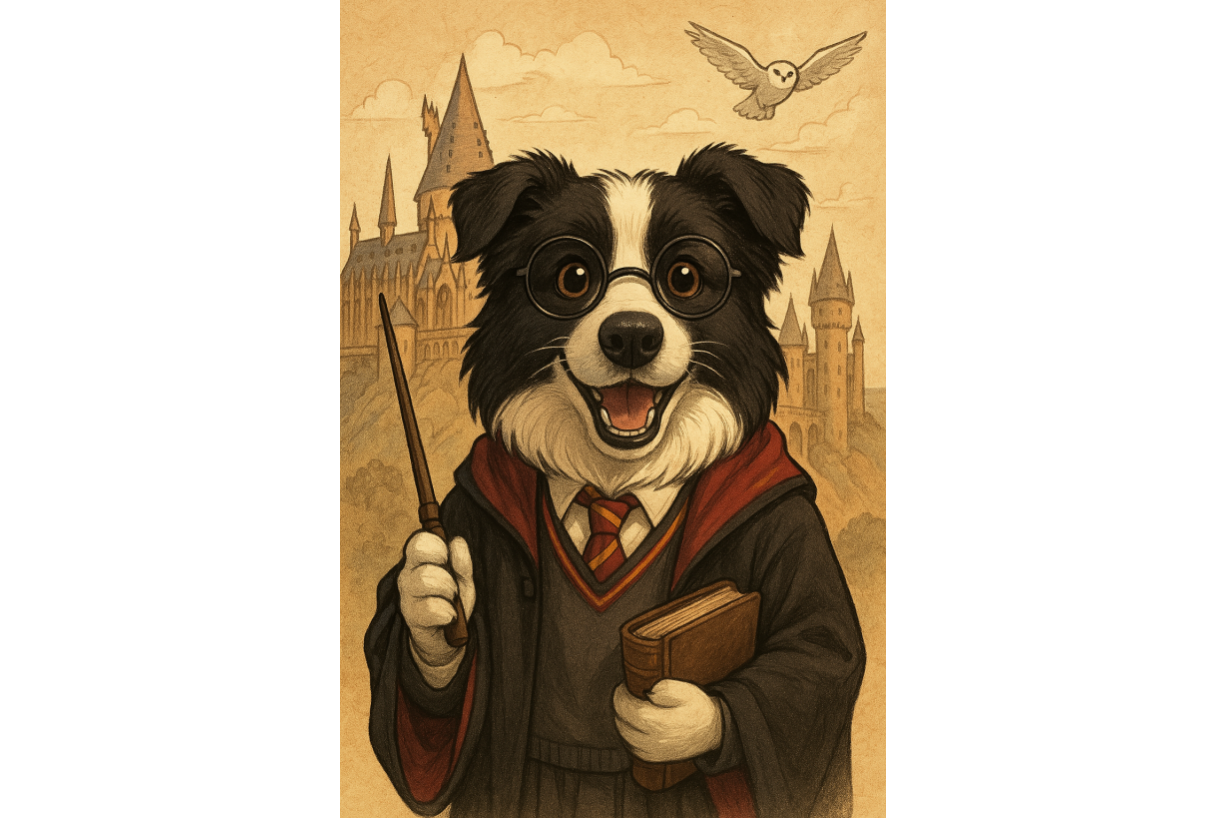__\n\n### A Few Quick Tips from My Experience of Image Generation:\n\n1. **Keep it simple**: Unlike those finicky diffusion models, GPT-4o works best with straightforward prompts. I found myself writing shorter and shorter prompts as I went along, and getting better results.\n\n2. **English works best**: I tried prompting in Chinese for some comics, but the results weren't great. I ended up writing my prompts in English and then translating the finished comics when needed.\n\n3. **Not good for Video Generation**: Don’t get your hopes too high with Sora yet—AI-generated videos still have a way to go when it comes to fluid movement and coherent storylines.\n\n## What's Next? My Perspective and Open for Discussion \n\nWith AI-generated images leading the charge, a quick look at OpenAI's major releases over the past six months shows a clear pattern: whether it's GPTs for app marketplaces, DeepResearch for report generation, GPT-4o for conversational image creation, or Sora for video magic - large AI models are stepping from behind the curtain into the spotlight. What was once experimental tech is now maturing into real, usable products.\n\nAs GPT-4o and similar models become widely accepted, most workflows and intelligent agents based on Stable Diffusion are heading toward obsolescence. However, the irreplaceable value of private data and human insight remains strong. For example, while AI won't completely replace creative agencies, integrating a Milvus vector database with GPT models enables agencies to quickly generate fresh, creative ideas inspired by their past successes. E-commerce platforms can design personalized clothing based on shopping trends, and academic institutions can instantly create visuals for research papers.\n\nThe era of products powered by AI models is here, and the race to mine the data goldmine is just getting started. For developers and businesses alike, the message is clear: combine your unique data with these powerful models or risk being left behind.\n","title":"Generating More Creative and Curated Ghibli-Style Images with GPT-4o and Milvus","metaData":{}},{"id":"milvus-2025-roadmap-tell-us-what-you-think.md","author":"Fendy Feng, Field Zhang","desc":"In 2025, we’re rolling out two major versions, Milvus 2.6 and Milvus 3.0, and many other technical features. We welcome you to share your thoughts with us.","recommend":true,"canonicalUrl":"https://milvus.io/blog/milvus-2025-roadmap-tell-us-what-you-think.md","date":"2025-03-27T00:00:00.000Z","cover":"https://assets.zilliz.com/2025_roadmap_04e6c5d1c3.png","tags":["Announcements"],"href":"/blog/milvus-2025-roadmap-tell-us-what-you-think.md","content":"\nHey, Milvus users and contributors!\n\nWe're excited to share our [**Milvus 2025 roadmap**](https://milvus.io/docs/roadmap.md) with you. 🚀 This technical plan highlights the key features and improvements we’re building to make Milvus even more powerful for your vector search needs.\n\nBut this is just the beginning—we want your insights! Your feedback helps shape Milvus, ensuring it evolves to meet real-world challenges. Let us know what you think and help us refine the roadmap as we move forward.\n\n\n## The Current Landscape\n\nOver the past year, we've seen many of you build impressive RAG and agent applications with Milvus, leveraging many of our popular features, such as our model integration, full-text search, and hybrid search. Your implementations have provided valuable insights into real-world vector search requirements.\n\nAs AI technologies evolve, your use cases are becoming more sophisticated - from basic vector search to complex multimodal applications spanning intelligent agents, autonomous systems, and embodied AI. These technical challenges are informing our roadmap as we continue to develop Milvus to meet your needs.\n\n\n## Two Major Releases in 2025: Milvus 2.6 and Milvus 3.0 \n\nIn 2025, we’re rolling out two major versions: Milvus 2.6 (Middle of CY25) and Milvus 3.0 (end of 2025). \n\n**Milvus 2.6** focuses on core architecture improvements you've been asking for:\n\n- Simpler deployment with fewer dependencies (goodbye, deployment headaches!)\n\n- Faster data ingestion pipelines\n\n- Lower storage costs (we hear your production cost concerns)\n\n- Better handling of large-scale data operations (delete/modify)\n\n- More efficient scalar and full-text search\n\n- Support for the latest embedding models you're working with\n\n**Milvus 3.0** is our bigger architectural evolution, introducing a vector data lake system for:\n\n- Seamless AI service integration\n\n- Next-level search capabilities\n\n- More robust data management\n\n- Better handling of those massive offline datasets you're working with\n\n\n## Technical Features We're Planning - We Need Your Feedback\n\nBelow are key technical features we are planning to add to Milvus. \n\n\n| **Key Feature Area** | **Technical Features** |\n|----------------------------------------|----------------------------------------------------------------------------------------------------------------------------------------------------------------------------------------|\n| **AI-Driven Unstructured Data Processing** | - Data-In/Out: Native integration with major model services for raw text ingestion\u003cbr\u003e- Original Data Handling: Text/URL reference support for raw data processing\u003cbr\u003e- Tensor Support: Vector list implementation (for ColBERT/CoPali/Video scenarios)\u003cbr\u003e- Extended Data Types: DateTime, Map, GIS support based on requirements\u003cbr\u003e- Iterative Search: Query vector refinement through user‘s feedback |\n| **Search Quality \u0026 Performance Improvements** | - Advanced Matching: phrase_match \u0026 multi_match capabilities\u003cbr\u003e- Analyzer Upgrade: Enhance Analyzer with expanded tokenizer support and improved observability\u003cbr\u003e- JSON Optimization: Faster filtering through improved indexing\u003cbr\u003e- Execution Sorting: Scalar field-based result ordering\u003cbr\u003e- Advanced Reranker: Model-based reranking \u0026 custom scoring functions\u003cbr\u003e- Iterative Search: Query vector refinement through user‘s feedback |\n| **Data Management Flexibility** | - Schema Change: Add/delete field, modify varchar length\u003cbr\u003e- Scalar Aggregations: count/distinct/min/max operations\u003cbr\u003e- Support UDF: Support user-defined function\u003cbr\u003e- Data Versioning: Snapshot-based rollback system\u003cbr\u003e- Data Clustering: Co-location through configuration\u003cbr\u003e- Data Sampling: Fast get results based on sampling data |\n| **Architectural Improvements** | - Stream Node: Simplified incremental data ingestion\u003cbr\u003e- MixCoord: Unified coordinator architecture\u003cbr\u003e- Logstore Independence: Reduced external dependencies like pulsar\u003cbr\u003e- PK Deduplication: Global primary key deduplication |\n| **Cost Efficiency \u0026 Architecture Improvements** | - Tiered Storage: Hot/cold data separation for lower storage cost\u003cbr\u003e- Data Evict Policy: Users can define their own data evict policy\u003cbr\u003e- Bulk Updates: Support field-specific value modifications, ETL, etc\u003cbr\u003e- Large TopK: Returns massive datasets\u003cbr\u003e- VTS GA: Connect to different sources of data\u003cbr\u003e- Advanced Quantization: Optimize memory consumption and performance based on quantization techniques\u003cbr\u003e- Resource Elasticity: Dynamically scale resources to accommodate varying write loads, read loads, and background task loads |\n\n\n\nAs we implement this roadmap, we'd appreciate your thoughts and feedback on the following:\n\n1. **Feature priorities:** Which features in our roadmap would have the most impact on your work?\n\n2. **Implementation ideas:** Any specific approaches you think would work well for these features?\n\n3. **Use case alignment:** How do these planned features align with your current and future use cases?\n\n4. **Performance considerations:** Any performance aspects we should focus on for your specific needs?\n\n**Your insights help us make Milvus better for everyone. Feel free to share your thoughts on our[ Milvus Discussion Forum](https://github.com/milvus-io/milvus/discussions/40263) or our [Discord Channel](https://discord.com/invite/8uyFbECzPX).**\n\n\n## Welcome to Contribute to Milvus\n\nAs an open-source project, Milvus always welcomes your contributions:\n\n- **Share feedback:** Report issues or suggest features through our [GitHub issue page](https://github.com/milvus-io/milvus/issues)\n\n- **Code contributions:** Submit pull requests (see our [Contributor's Guide](https://github.com/milvus-io/milvus/blob/82915a9630ab0ff40d7891b97c367ede5726ff7c/CONTRIBUTING.md))\n\n- **Spread the word:** Share your Milvus experiences and [star our GitHub repository](https://github.com/milvus-io/milvus)\n\nWe're excited to build this next chapter of Milvus with you. Your code, ideas, and feedback drive this project forward!\n\n-- The Milvus Team \n","title":"Milvus 2025 Roadmap - Tell Us What You Think\n","metaData":{}},{"id":"deepseek-v3-0324-minor-update-thats-crushing-top-ai-models.md","author":"Lumina Wang","desc":"DeepSeek v3-0324 is trained with larger parameters, has a longer context window and enhanced Reasoning, Coding, and Math capabilities.","tags":["Engineering"],"recommend":true,"canonicalUrl":"https://milvus.io/blog/deepseek-v3-0324-minor-update-thats-crushing-top-ai-models.md","date":"2025-03-25T00:00:00.000Z","cover":"https://assets.zilliz.com/Deep_Seek_V3_0324_The_Minor_Update_That_s_Crushing_Top_AI_Models_391585994c.png","href":"/blog/deepseek-v3-0324-minor-update-thats-crushing-top-ai-models.md","content":"\nDeepSeek quietly dropped a bombshell last night. Their latest release,[ DeepSeek v3-0324](https://huggingface.co/deepseek-ai/DeepSeek-V3-0324), was downplayed in the official announcement as just a **\"minor upgrade\"** with no API changes. But our extensive testing at [Zilliz](https://zilliz.com/) has revealed something more significant: this update represents a quantum leap in performance, particularly in logic reasoning, programming, and mathematical problem-solving.\n\nWhat we're seeing isn't just incremental improvement – it's a fundamental shift that positions DeepSeek v3-0324 among the elite tier of language models. And it is open source. \n\n**This release deserves your immediate attention for developers and enterprises building AI-powered applications.**\n\n\n## What's New in DeepSeek v3-0324 and How Good Is It Really?\n\nDeepSeek v3-0324 introduces three major improvements over its predecessor, [DeepSeek v3](https://zilliz.com/blog/why-deepseek-v3-is-taking-the-ai-world-by-storm):\n\n- **Larger Model, More Power:** The parameter count has increased from 671 billion to 685 billion, allowing the model to handle more complex reasoning and generate more nuanced responses.\n\n- **A Massive Context Window:** With an upgraded 128K token context length, DeepSeek v3-0324 can retain and process significantly more information in a single query, making it ideal for long-form conversations, document analysis, and retrieval-based AI applications.\n\n- **Enhanced Reasoning, Coding, and Math:** This update brings a noticeable boost in logic, programming, and mathematical capabilities, making it a strong contender for AI-assisted coding, scientific research, and enterprise-grade problem-solving.\n\nBut the raw numbers don't tell the whole story. What's truly impressive is how DeepSeek has managed to simultaneously enhance reasoning capacity and generation efficiency—something that typically involves engineering tradeoffs.\n\n\n### The Secret Sauce: Architectural Innovation\n\nUnder the hood, DeepSeek v3-0324 retains its [Multi-head Latent Attention (MLA) ](https://arxiv.org/abs/2502.07864)architecture—an efficient mechanism that compresses Key-Value (KV) caches using latent vectors to reduce memory usage and computational overhead during inference. Additionally, it replaces traditional [Feed-Forward Networks (FFN)](https://zilliz.com/glossary/feedforward-neural-networks-(fnn)) with Mixture of Experts ([MoE](https://zilliz.com/learn/what-is-mixture-of-experts)) layers, optimizing compute efficiency by dynamically activating the best-performing experts for each token.\n\nHowever, the most exciting upgrade is **multi-token prediction (MTP),** which allows each token to predict multiple future tokens simultaneously. This overcomes a significant bottleneck in traditional autoregressive models, improving both accuracy and inference speed. \n\nTogether, these innovations create a model that doesn't just scale well – it scales intelligently, bringing professional-grade AI capabilities within reach of more development teams.\n\n\n## Build a RAG System with Milvus and DeepSeek v3-0324 in 5 Minutes\n\nDeepSeek v3-0324's powerful reasoning capabilities make it an ideal candidate for Retrieval-Augmented Generation (RAG) systems. In this tutorial, we'll show you how to build a complete RAG pipeline using DeepSeek v3-0324 and the [Milvus](https://zilliz.com/what-is-milvus) vector database in just five minutes. You'll learn how to retrieve and synthesize knowledge efficiently with minimal setup.\n\n\n### Setting Up Your Environment\n\nFirst, let's install the necessary dependencies:\n\n```\n! pip install --upgrade pymilvus[model] openai requests tqdm\n```\n\n**Note:** If you're using Google Colab, you'll need to restart the runtime after installing these packages. Click on the \"Runtime\" menu at the top of the screen and select \"Restart session\" from the dropdown menu.\n\nSince DeepSeek provides an OpenAI-compatible API, you'll need an API key. You can get one by signing up on the[ DeepSeek platform](https://platform.deepseek.com/api_keys):\n\n```\nimport os\n\nos.environ[\"DEEPSEEK_API_KEY\"] = \"***********\"\n```\n### Preparing Your Data\n\nFor this tutorial, we'll use the FAQ pages from the [Milvus Documentation 2.4.x](https://github.com/milvus-io/milvus-docs/releases/download/v2.4.6-preview/milvus_docs_2.4.x_en.zip) as our knowledge source: \n\n```\n! wget https://github.com/milvus-io/milvus-docs/releases/download/v2.4.6-preview/milvus_docs_2.4.x_en.zip\n! unzip -q milvus_docs_2.4.x_en.zip -d milvus_docs\n```\nNow, let's load and prepare the FAQ content from the markdown files:\n\n```\nfrom glob import glob\n\n# Load all markdown files from the FAQ directory\ntext_lines = []\nfor file_path in glob(\"milvus_docs/en/faq/*.md\", recursive=True):\n with open(file_path, \"r\") as file:\n file_text = file.read()\n # Split on headings to separate content sections\n text_lines += file_text.split(\"# \")\n```\n### Setting Up the Language and Embedding Models\n\nWe'll use [OpenRouter](https://openrouter.ai/) to access DeepSeek v3-0324. OpenRouter provides a unified API for multiple AI models, such as DeepSeek and Claude. By creating a free DeepSeek V3 API key on OpenRouter, you can easily try out DeepSeek V3 0324. \n\nhttps://assets.zilliz.com/Setting_Up_the_Language_and_Embedding_Models_8b00595a6b.png\n\n```\nfrom openai import OpenAI\n\ndeepseek_client = OpenAI(\n api_key=\"\u003cOPENROUTER_API_KEY\u003e\",\n base_url=\"https://openrouter.ai/api/v1\",\n)\n```\nFor text embeddings, we'll use Milvus' [built-in embedding model](https://milvus.io/docs/embeddings.md), which is lightweight and effective:\n\n```\nfrom pymilvus import model as milvus_model\n\n# Initialize the embedding model\nembedding_model = milvus_model.DefaultEmbeddingFunction()\n\n# Test the embedding model\ntest_embedding = embedding_model.encode_queries([\"This is a test\"])[0]\nembedding_dim = len(test_embedding)\nprint(f\"Embedding dimension: {embedding_dim}\")\nprint(f\"First 10 values: {test_embedding[:10]}\")\n```\n### Creating a Milvus Collection\n\nNow let's set up our vector database using Milvus:\n\n```\nfrom pymilvus import MilvusClient\n\n# Initialize Milvus client (using Milvus Lite for simplicity)\nmilvus_client = MilvusClient(uri=\"./milvus_demo.db\")\ncollection_name = \"my_rag_collection\"\n\n# Remove existing collection if it exists\nif milvus_client.has_collection(collection_name):\n milvus_client.drop_collection(collection_name)\n\n# Create a new collection\nmilvus_client.create_collection(\n collection_name=collection_name,\n dimension=embedding_dim,\n metric_type=\"IP\", # Inner product distance\n consistency_level=\"Strong\", # See https://milvus.io/docs/consistency.md for details\n)\n```\n\n**Pro Tip**: For different deployment scenarios, you can adjust your Milvus setup:\n\n- For local development: Use `uri=\"./milvus.db\"` with [Milvus Lite](https://milvus.io/docs/milvus_lite.md)\n\n- For larger datasets: Set up a Milvus server via [Docker/Kubernetes](https://milvus.io/docs/quickstart.md) and use `uri=\"http://localhost:19530\"`\n\n- For production: Use[ Zilliz Cloud](https://zilliz.com/cloud) with your cloud endpoint and API key.\n\n\n### Loading Data into Milvus\n\nLet's convert our text data into embeddings and store them in Milvus:\n\n```\nfrom tqdm import tqdm\n\n# Create embeddings for all text chunks\ndata = []\ndoc_embeddings = embedding_model.encode_documents(text_lines)\n\n# Create records with IDs, vectors, and text\nfor i, line in enumerate(tqdm(text_lines, desc=\"Creating embeddings\")):\n data.append({\"id\": i, \"vector\": doc_embeddings[i], \"text\": line})\n\n# Insert data into Milvus\nmilvus_client.insert(collection_name=collection_name, data=data)\n```\n\n```\nCreating embeddings: 0%| | 0/72 [00:00\u003c?, ?it/s]huggingface/tokenizers: The current process just got forked, after parallelism has already been used. Disabling parallelism to avoid deadlocks...\nTo disable this warning, you can either:\n - Avoid using `tokenizers` before the fork if possible\n - Explicitly set the environment variable TOKENIZERS_PARALLELISM=(true | false)\nCreating embeddings: 100%|██████████| 72/72 [00:00\u003c00:00, 246522.36it/s]\n\n\n\n\n\n{'insert_count': 72, 'ids': [0, 1, 2, 3, 4, 5, 6, 7, 8, 9, 10, 11, 12, 13, 14, 15, 16, 17, 18, 19, 20, 21, 22, 23, 24, 25, 26, 27, 28, 29, 30, 31, 32, 33, 34, 35, 36, 37, 38, 39, 40, 41, 42, 43, 44, 45, 46, 47, 48, 49, 50, 51, 52, 53, 54, 55, 56, 57, 58, 59, 60, 61, 62, 63, 64, 65, 66, 67, 68, 69, 70, 71], 'cost': 0}\n```\n\n### Building the RAG Pipeline\n\n#### Step 1: Retrieve Relevant Information\n\nLet's test our RAG system with a common question:\n\n```\nquestion = \"How is data stored in milvus?\"\n\n# Search for relevant information\nsearch_res = milvus_client.search(\n collection_name=collection_name,\n data=embedding_model.encode_queries([question]), # Convert question to embedding\n limit=3, # Return top 3 results\n search_params={\"metric_type\": \"IP\", \"params\": {}}, # Inner product distance\n output_fields=[\"text\"], # Return the text field\n)\n\n# Examine search results\nimport json\nretrieved_lines_with_distances = [\n (res[\"entity\"][\"text\"], res[\"distance\"]) for res in search_res[0]\n]\nprint(json.dumps(retrieved_lines_with_distances, indent=4))\n```\n\n```\n[\n [\n \" Where does Milvus store data?\\n\\nMilvus deals with two types of data, inserted data and metadata. \\n\\nInserted data, including vector data, scalar data, and collection-specific schema, are stored in persistent storage as incremental log. Milvus supports multiple object storage backends, including [MinIO](https://min.io/), [AWS S3](https://aws.amazon.com/s3/?nc1=h_ls), [Google Cloud Storage](https://cloud.google.com/storage?hl=en#object-storage-for-companies-of-all-sizes) (GCS), [Azure Blob Storage](https://azure.microsoft.com/en-us/products/storage/blobs), [Alibaba Cloud OSS](https://www.alibabacloud.com/product/object-storage-service), and [Tencent Cloud Object Storage](https://www.tencentcloud.com/products/cos) (COS).\\n\\nMetadata are generated within Milvus. Each Milvus module has its own metadata that are stored in etcd.\\n\\n###\",\n 0.6572665572166443\n ],\n [\n \"How does Milvus flush data?\\n\\nMilvus returns success when inserted data are loaded to the message queue. However, the data are not yet flushed to the disk. Then Milvus' data node writes the data in the message queue to persistent storage as incremental logs. If `flush()` is called, the data node is forced to write all data in the message queue to persistent storage immediately.\\n\\n###\",\n 0.6312146186828613\n ],\n [\n \"How does Milvus handle vector data types and precision?\\n\\nMilvus supports Binary, Float32, Float16, and BFloat16 vector types.\\n\\n- Binary vectors: Store binary data as sequences of 0s and 1s, used in image processing and information retrieval.\\n- Float32 vectors: Default storage with a precision of about 7 decimal digits. Even Float64 values are stored with Float32 precision, leading to potential precision loss upon retrieval.\\n- Float16 and BFloat16 vectors: Offer reduced precision and memory usage. Float16 is suitable for applications with limited bandwidth and storage, while BFloat16 balances range and efficiency, commonly used in deep learning to reduce computational requirements without significantly impacting accuracy.\\n\\n###\",\n 0.6115777492523193\n ]\n]\n```\n\n#### Step 2: Generate a Response with DeepSeek\n\nNow let's use DeepSeek to generate a response based on the retrieved information:\n\n```\n# Combine retrieved text chunks\ncontext = \"\\n\".join(\n [line_with_distance[0] for line_with_distance in retrieved_lines_with_distances]\n)\n\n# Define prompts for the language model\nSYSTEM_PROMPT = \"\"\"\nYou are an AI assistant. You are able to find answers to the questions from the contextual passage snippets provided.\n\"\"\"\n\nUSER_PROMPT = f\"\"\"\nUse the following pieces of information enclosed in \u003ccontext\u003e tags to provide an answer to the question enclosed in \u003cquestion\u003e tags.\n\n\u003ccontext\u003e\n{context}\n\u003c/context\u003e\n\n\u003cquestion\u003e\n{question}\n\u003c/question\u003e\n\"\"\"\n\n# Generate response with DeepSeek\nresponse = deepseek_client.chat.completions.create(\n model=\"deepseek-chat\",\n messages=[\n {\"role\": \"system\", \"content\": SYSTEM_PROMPT},\n {\"role\": \"user\", \"content\": USER_PROMPT},\n ],\n)\n\nprint(response.choices[0].message.content)\n```\n\n\n```\nIn Milvus, data is stored in two main categories: inserted data and metadata.\n\n1. **Inserted Data**: This includes vector data, scalar data, and collection-specific schema. The inserted data is stored in persistent storage as incremental logs. Milvus supports various object storage backends for this purpose, such as MinIO, AWS S3, Google Cloud Storage (GCS), Azure Blob Storage, Alibaba Cloud OSS, and Tencent Cloud Object Storage (COS).\n\n2. **Metadata**: Metadata is generated within Milvus and is specific to each Milvus module. This metadata is stored in etcd, a distributed key-value store.\n\nAdditionally, when data is inserted, it is first loaded into a message queue, and Milvus returns success at this stage. The data is then written to persistent storage as incremental logs by the data node. If the `flush()` function is called, the data node is forced to write all data in the message queue to persistent storage immediately.\n```\n\nAnd there you have it! You've successfully built a complete RAG pipeline with DeepSeek v3-0324 and Milvus. This system can now answer questions based on the Milvus documentation with high accuracy and contextual awareness.\n\n## Comparing DeepSeek-V3-0324: Original vs. RAG-Enhanced Version\n\nTheory is one thing, but real-world performance is what matters. We tested both the standard DeepSeek v3-0324 (with \"Deep Thinking\" disabled) and our RAG-enhanced version with the same prompt: *Write HTML code to create a fancy website about Milvus.*\n\n\n### Website Built with The Standard Model's Output Code \n\nHere's what the website looks like: \n\n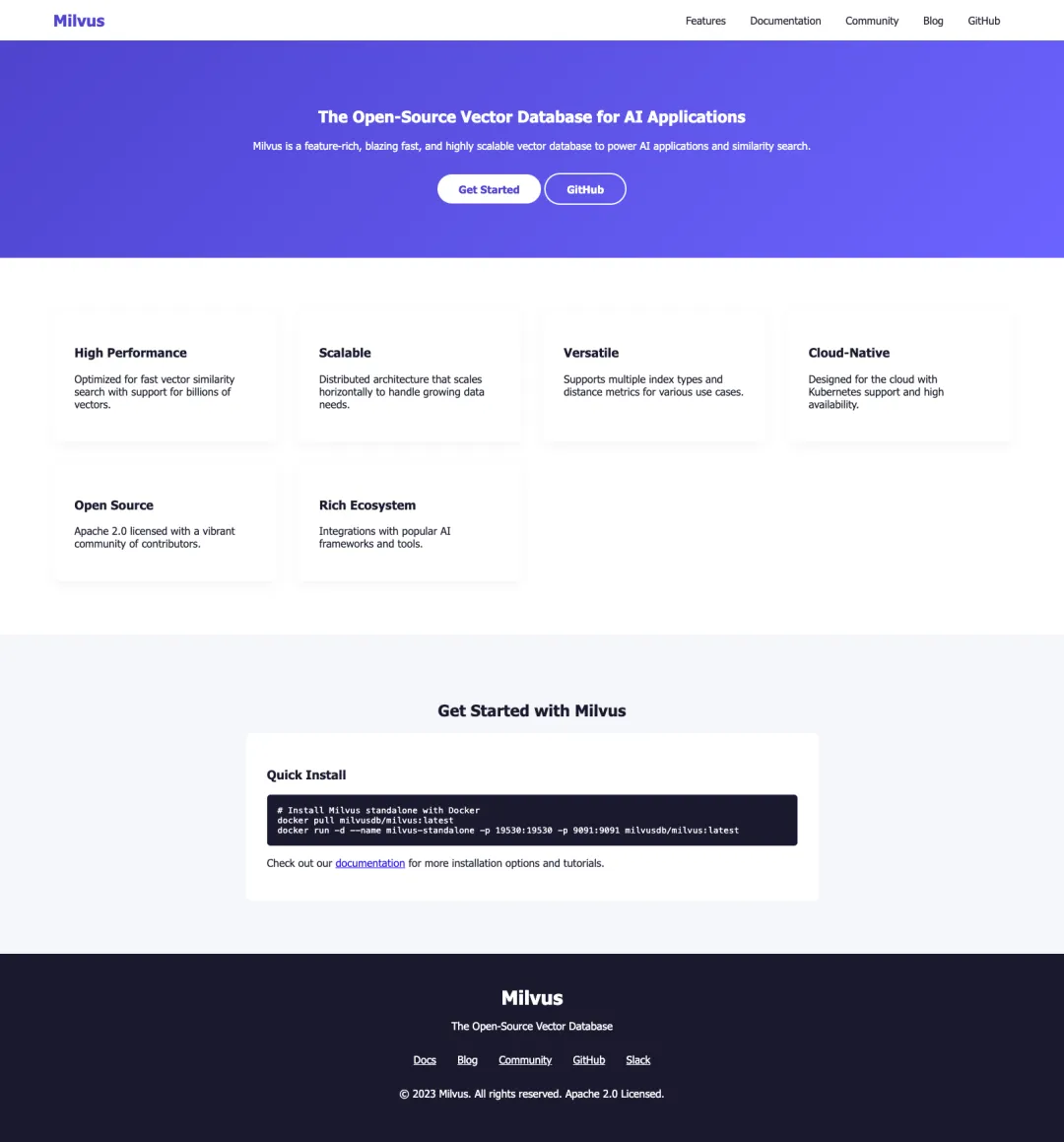\n\nWhile visually appealing, the content relies heavily on generic descriptions and misses many of Milvus' core technical features.\n\n\n### Website Built with Code Generated by the RAG-Enhanced Version\n\nWhen we integrated Milvus as the knowledge base, the results were dramatically different:\n\n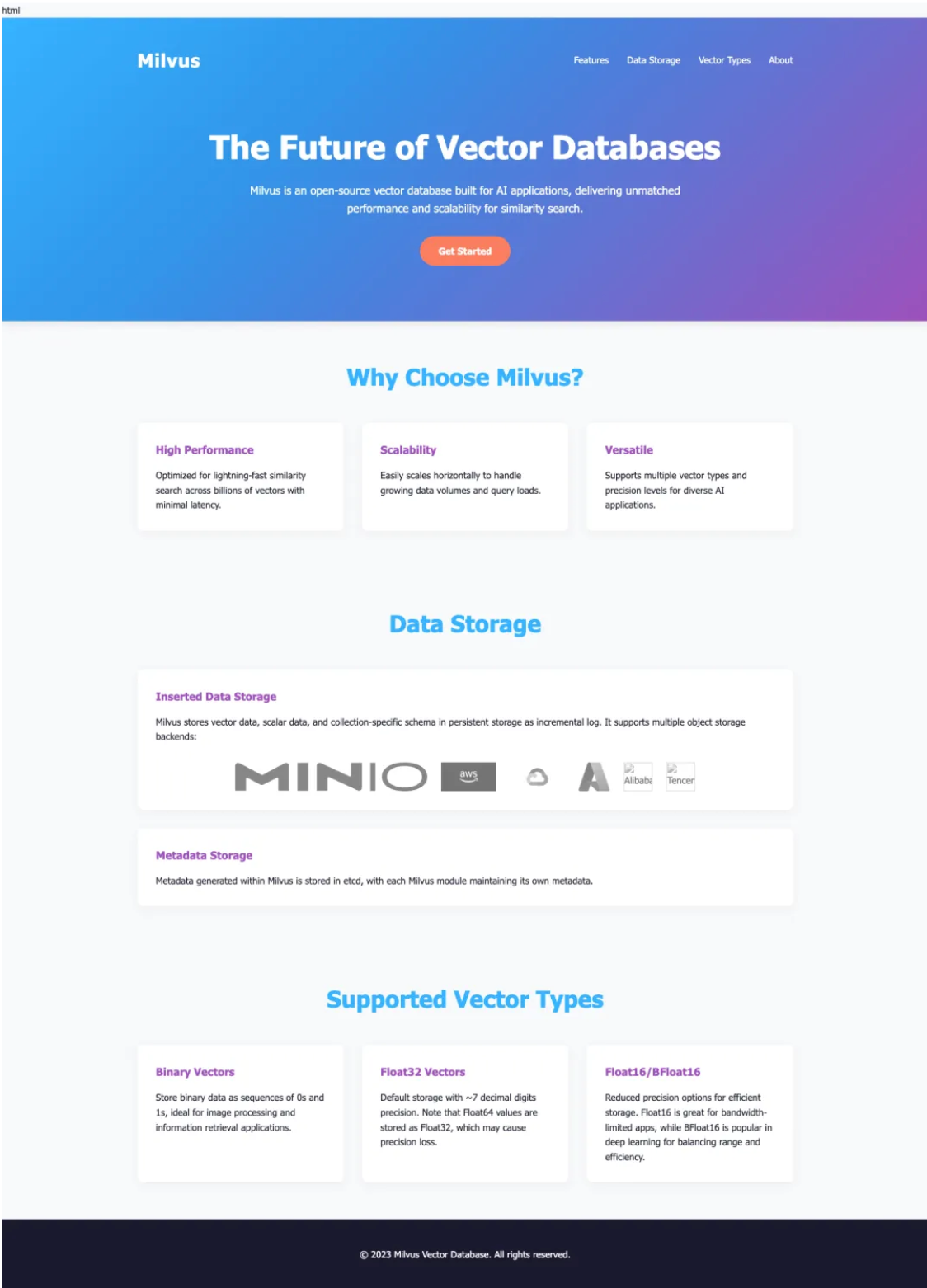\n\nThe latter website doesn't just look better – it demonstrates genuine understanding of Milvus' architecture, use cases, and technical advantages. \n\n\n## Can DeepSeek v3-0324 Replace Dedicated Reasoning Models?\n\nOur most surprising discovery came when comparing DeepSeek v3-0324 against specialized reasoning models like Claude 3.7 Sonnet and GPT-4 Turbo across mathematical, logical, and code reasoning tasks.\n\nWhile dedicated reasoning models excel at multi-step problem solving, they often do so at the cost of efficiency. Our benchmarks showed that reasoning-heavy models frequently overanalyze simple prompts, generating 2-3x more tokens than necessary and significantly increasing latency and API costs.\n\nDeepSeek v3-0324 takes a different approach. It demonstrates comparable logical consistency but with remarkably greater conciseness – often producing correct solutions with 40-60% fewer tokens. This efficiency doesn't come at the expense of accuracy; in our code generation tests, DeepSeek's solutions matched or exceeded the functionality of those from reasoning-focused competitors.\n\nFor developers balancing performance with budget constraints, this efficiency advantage translates directly to lower API costs and faster response times – crucial factors for production applications where user experience hinges on perceived speed.\n\n\n## The Future of AI Models: Blurring the Reasoning Divide\n\nDeepSeek v3-0324's performance challenges a core assumption in the AI industry: that reasoning and efficiency represent an unavoidable tradeoff. This suggests we may be approaching an inflection point where the distinction between reasoning and non-reasoning models begins to blur.\n\nLeading AI providers may eventually eliminate this distinction entirely, developing models that dynamically adjust their reasoning depth based on task complexity. Such adaptive reasoning would optimize both computational efficiency and response quality, potentially revolutionizing how we build and deploy AI applications.\n\nFor developers building RAG systems, this evolution promises more cost-effective solutions that deliver the reasoning depth of premium models without their computational overhead – expanding what's possible with open-source AI.\n","title":"DeepSeek V3-0324: The \"Minor Update\" That's Crushing Top AI Models\n","metaData":{}},{"id":"what-is-a-vector-database.md","author":"Zilliz","desc":"A vector database stores, indexes, and searches vector embeddings generated by machine learning models for fast information retrieval and similarity search.","canonicalUrl":"https://milvus.io/blog/parse-is-hard-solve-semantic-understanding-with-mistral-ocr-and-milvus.md","date":"2025-03-24T00:00:00.000Z","cover":"https://assets.zilliz.com/What_s_a_Vector_Database_and_How_Does_It_Work_cac0875415.png","tags":["Engineering"],"href":"/blog/what-is-a-vector-database.md","content":"\nA vector database indexes and stores vector embeddings for fast retrieval and similarity search, with capabilities like CRUD operations, metadata filtering, and horizontal scaling designed specifically for AI applications.\n\n\u003ciframe width=\"100%\" height=\"315\" src=\"https://www.youtube.com/embed/4yQjsY5iD9Q\" title=\"YouTube video player\" frameborder=\"0\" allow=\"accelerometer; autoplay; clipboard-write; encrypted-media; gyroscope; picture-in-picture; web-share\" allowfullscreen\u003e\u003c/iframe\u003e\n\n\n## Introduction: The Rise of Vector Databases in the AI Era\n\nIn the early days of ImageNet, it took 25,000 human curators to manually label the dataset. This staggering number highlights a fundamental challenge in AI: manually categorizing unstructured data simply doesn’t scale. With billions of images, videos, documents, and audio files generated daily, a paradigm shift was needed in how computers understand and interact with content.\n\n[Traditional relational database](https://zilliz.com/blog/relational-databases-vs-vector-databases) systems excel at managing structured data with predefined formats and executing precise search operations. In contrast, vector databases specialize in storing and retrieving [unstructured data ](https://zilliz.com/learn/introduction-to-unstructured-data)types, such as images, audio, videos, and textual content, through high-dimensional numerical representations known as vector embeddings. Vector databases support [large language models](https://zilliz.com/glossary/large-language-models-(llms)) by providing efficient data retrieval and management. Modern vector databases outperform traditional systems by 2-10x through hardware-aware optimization (AVX512, SIMD, GPUs, NVMe SSDs), highly optimized search algorithms (HNSW, IVF, DiskANN), and column-oriented storage design. Their cloud-native, decoupled architecture enables independent scaling of search, data insertion, and indexing components, allowing systems to efficiently handle billions of vectors while maintaining performance for enterprise AI applications at companies like Salesforce, PayPal, eBay, and NVIDIA.\n\nThis represents what experts call a “semantic gap”—traditional databases operate on exact matches and predefined relationships, while human understanding of content is nuanced, contextual, and multidimensional. This gap becomes increasingly problematic as AI applications demand:\n\n- Finding conceptual similarities rather than exact matches\n\n- Understanding contextual relationships between different pieces of content\n\n- Capturing the semantic essence of information beyond keywords\n\n- Processing multimodal data within a unified framework\n\nVector databases have emerged as the critical technology to bridge this gap, becoming an essential component in the modern AI infrastructure. They enhance the performance of machine learning models by facilitating tasks like clustering and classification.\n\n\u003ciframe style=\"border-radius:12px\" src=\"https://open.spotify.com/embed/episode/1T6K6wlZuryLbETUrafO9f?utm_source=generator\" width=\"100%\" height=\"152\" frameBorder=\"0\" allowfullscreen=\"\" allow=\"autoplay; clipboard-write; encrypted-media; fullscreen; picture-in-picture\" loading=\"lazy\"\u003e\u003c/iframe\u003e\n\n\n## Understanding Vector Embeddings: The Foundation\n\n[Vector embeddings](https://zilliz.com/glossary/vector-embeddings) serve as the critical bridge across the semantic gap. These high-dimensional numerical representations capture the semantic essence of unstructured data in a form computers can efficiently process. Modern embedding models transform raw content—whether text, images, or audio—into dense vectors where similar concepts cluster together in the vector space, regardless of surface-level differences.\n\nFor example, properly constructed embeddings would position concepts like “automobile,” “car,” and “vehicle” in proximity within the vector space, despite having different lexical forms. This property enables [semantic search](https://zilliz.com/glossary/semantic-search), [recommendation systems](https://zilliz.com/vector-database-use-cases/recommender-system), and AI applications to understand content beyond simple pattern matching.\n\nThe power of embeddings extends across modalities. Advanced vector databases support various unstructured data types—text, images, audio—in a unified system, enabling cross-modal searches and relationships that were previously impossible to model efficiently. These vector database capabilities are crucial for AI-driven technologies such as chatbots and image recognition systems, supporting advanced applications like semantic search and recommendation systems.\n\nHowever, storing, indexing, and retrieving embeddings at scale presents unique computational challenges that traditional databases weren’t built to address.\n\n\n## Vector Databases: Core Concepts\n\nVector databases represent a paradigm shift in how we store and query unstructured data. Unlike traditional relational database systems that excel at managing structured data with predefined formats, vector databases specialize in handling unstructured data through numerical vector representations.\n\nAt their core, vector databases are designed to solve a fundamental problem: enabling efficient similarity searches across massive datasets of unstructured data. They accomplish this through three key components:\n\n**Vector Embeddings**: High-dimensional numerical representations that capture semantic meaning of unstructured data (text, images, audio, etc.)\n\n**Specialized Indexing**: Algorithms optimized for high-dimensional vector spaces that enable fast approximate searches. Vector database indexes vectors to enhance the speed and efficiency of similarity searches, utilizing various ML algorithms to create indexes on vector embeddings.\n\n[**Distance Metrics**](https://zilliz.com/blog/similarity-metrics-for-vector-search): Mathematical functions that quantify similarity between vectors\n\nThe primary operation in a vector database is the [k-nearest neighbors](https://zilliz.com/blog/k-nearest-neighbor-algorithm-for-machine-learning) (KNN) query, which finds the k vectors most similar to a given query vector. For large-scale applications, these databases typically implement [approximate nearest neighbor](https://zilliz.com/glossary/anns) (ANN) algorithms, trading a small amount of accuracy for significant gains in search speed.\n\n\n### Mathematical Foundations of Vector Similarity\n\nUnderstanding vector databases requires grasping the mathematical principles behind vector similarity. Here are the foundational concepts:\n\n\n### Vector Spaces and Embeddings\n\nA [vector embedding](https://zilliz.com/learn/everything-you-should-know-about-vector-embeddings) is a fixed-length array of floating-point numbers (they can range from 100-32,768 dimensions!) that represents unstructured data in a numerical format. These embeddings position similar items closer together in a high-dimensional vector space.\n\nFor example, the words \"king\" and \"queen\" would have vector representations that are closer to each other than either is to \"automobile\" in a well-trained word embedding space.\n\n\n### Distance Metrics\n\nThe choice of distance metric fundamentally affects how similarity is calculated. Common distance metrics include:\n\n1. **Euclidean Distance**: The straight-line distance between two points in Euclidean space.\n\n2. **Cosine Similarity**: Measures the cosine of the angle between two vectors, focusing on orientation rather than magnitude\n\n3. **Dot Product**: For normalized vectors, represents how aligned two vectors are.\n\n4. **Manhattan Distance (L1 Norm)**: Sum of absolute differences between coordinates.\n\nDifferent use cases may require different distance metrics. For example, cosine similarity often works well for text embeddings, while Euclidean distance may be better suited for certain types of [image embeddings](https://zilliz.com/learn/image-embeddings-for-enhanced-image-search).\n\n[Semantic similarity](https://zilliz.com/glossary/semantic-similarity) between vectors in a vector space\n\n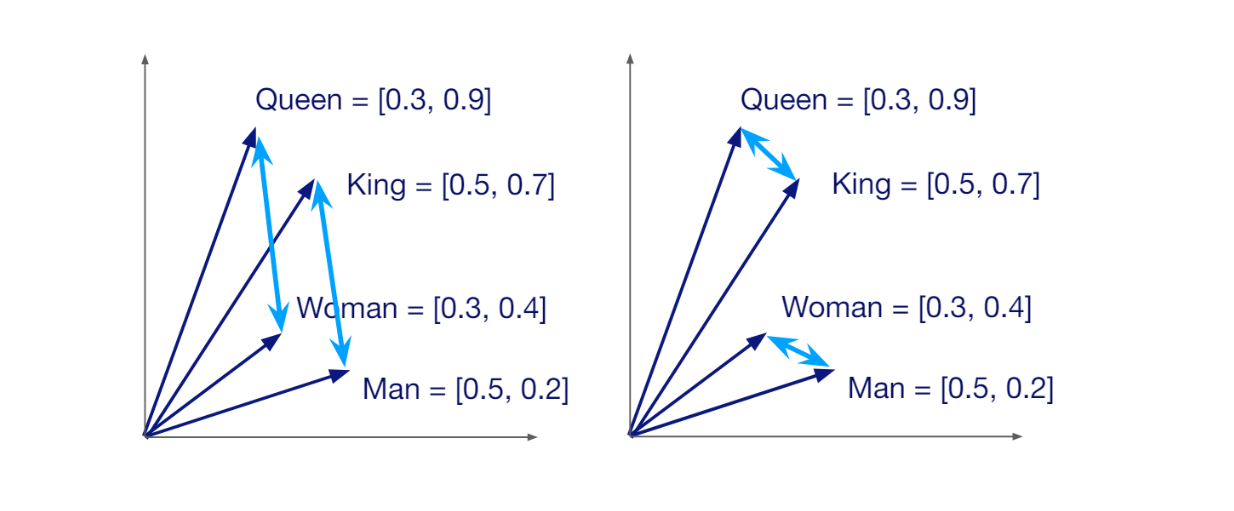\n\nUnderstanding these mathematical foundations leads to an important question about implementation: So just add a vector index to any database, right?\n\nSimply adding a vector index to a relational database isn't sufficient, nor is using a standalone [vector index library](https://zilliz.com/learn/comparing-vector-database-vector-search-library-and-vector-search-plugin). While vector indices provide the critical ability to find similar vectors efficiently, they lack the infrastructure needed for production applications:\n\n- They don't provide CRUD operations for managing vector data\n\n- They lack metadata storage and filtering capabilities\n\n- They offer no built-in scaling, replication, or fault tolerance\n\n- They require custom infrastructure for data persistence and management\n\nVector databases emerged to address these limitations, providing complete data management capabilities designed specifically for vector embeddings. They combine the semantic power of vector search with the operational capabilities of database systems.\n\nUnlike traditional databases that operate on exact matches, vector databases focus on semantic search—finding vectors that are \"most similar\" to a query vector according to specific distance metrics. This fundamental difference drives the unique architecture and algorithms that power these specialized systems.\n\n\n## Vector Database Architecture: A Technical Framework\n\nModern vector databases implement a sophisticated multi-layered architecture that separates concerns, enables scalability, and ensures maintainability. This technical framework goes far beyond simple search indices to create systems capable of handling production AI workloads. Vector databases work by processing and retrieving information for AI and ML applications, utilizing algorithms for approximate nearest neighbor searches, converting various types of raw data into vectors, and efficiently managing diverse data types through semantic searches.\n\n\n### Four-Tier Architecture\n\nA production vector database typically consists of four primary architectural layers:\n\n1. **Storage Layer**: Manages persistent storage of vector data and metadata, implements specialized encoding and compression strategies, and optimizes I/O patterns for vector-specific access.\n\n2. **Index Layer**: Maintains multiple indexing algorithms, manages their creation and updates, and implements hardware-specific optimizations for performance.\n\n3. **Query Layer**: Processes incoming queries, determines execution strategies, handles result processing, and implements caching for repeated queries.\n\n4. **Service Layer**: Manages client connections, handles request routing, provides monitoring and logging, and implements security and multi-tenancy.\n\n### Vector Search Workflow\n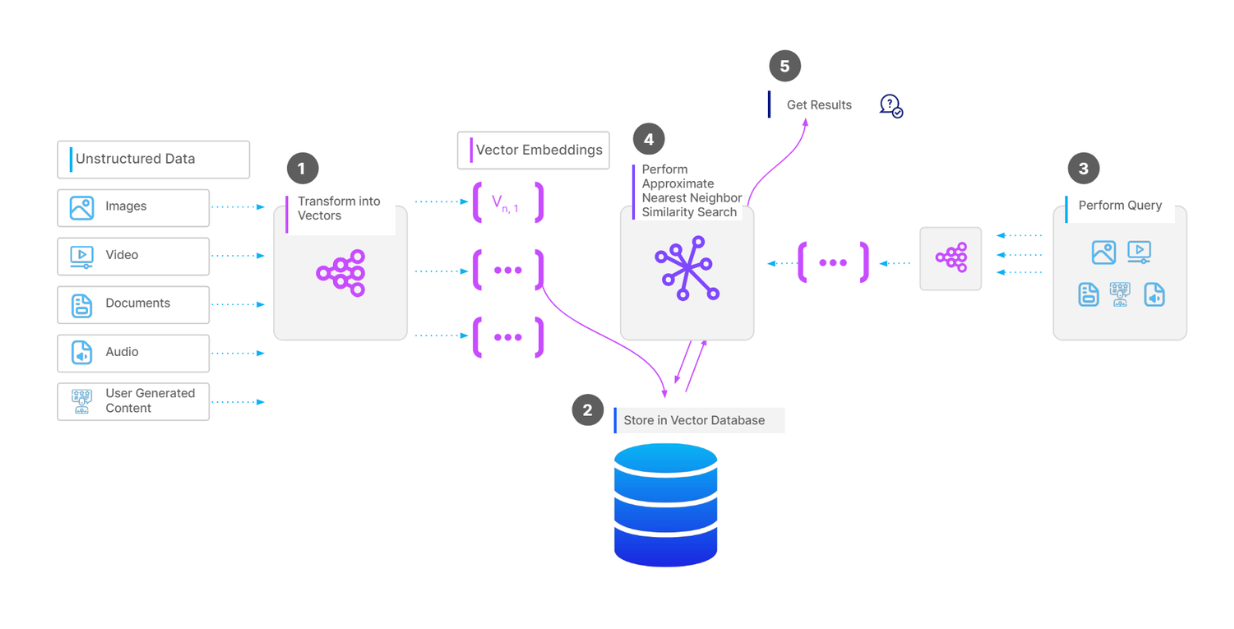\n\n\nA typical vector database implementation follows this workflow:\n\n1. A machine learning model transforms unstructured data (text, images, audio) into vector embeddings\n\n2. These vector embeddings are stored in the database along with relevant metadata\n\n3. When a user performs a query, it is converted into a vector embedding using the *same* model\n\n4. The database compares the query vector to stored vectors using an approximate nearest neighbor algorithm\n\n5. The system returns the top-K most relevant results based on vector similarity\n\n6. Optional post-processing may apply additional filters or reranking\n\nThis pipeline enables efficient semantic search across massive collections of unstructured data that would be impossible with traditional database approaches.\n\n\n#### Consistency in Vector Databases\n\nEnsuring consistency in distributed vector databases is a challenge due to the trade-off between performance and correctness. While eventual consistency is common in large-scale systems, strong consistency models are required for mission-critical applications like fraud detection and real-time recommendations. Techniques like quorum-based writes and distributed consensus (e.g., [Raft](https://zilliz.com/learn/raft-or-not), Paxos) ensure data integrity without excessive performance trade-offs.\n\nProduction implementations adopt a shared-storage architecture featuring storage and computing disaggregation. This separation follows the principle of data plane and control plane disaggregation, with each layer being independently scalable for optimal resource utilization.\n\n\n### Managing Connections, Security, and Multitenancy\n\nAs these databases are used in multi-user and multi-tenant environments, securing data and managing access control are critical for maintaining confidentiality.\n\nSecurity measures like encryption (both at rest and in transit) protect sensitive data, such as embeddings and metadata. Authentication and authorization ensure only authorized users can access the system, with fine-grained permissions for managing access to specific data.\n\nAccess control defines roles and permissions to restrict data access. This is particularly important for databases storing sensitive information like customer data or proprietary AI models.\n\nMultitenancy involves isolating each tenant's data to prevent unauthorized access while enabling resource sharing. This is achieved through sharding, partitioning, or row-level security to ensure scalable and secure access for different teams or clients.\n\nExternal identity and access management (IAM) systems integrate with vector databases to enforce security policies and ensure compliance with industry standards.\n\n## Advantages of Vector Databases\n\nVector databases offer several advantages over traditional databases, making them an ideal choice for handling vector data. Here are some of the key benefits:\n\n1. **Efficient Similarity Search**: One of the standout features of vector databases is their ability to perform efficient semantic searches. Unlike traditional databases that rely on exact matches, vector databases excel at finding data points that are similar to a given query vector. This capability is crucial for applications like recommendation systems, where finding items similar to a user’s past interactions can significantly enhance user experience.\n\n2. **Handling High-Dimensional Data**: Vector databases are specifically designed to manage high-dimensional data efficiently. This makes them particularly suitable for applications in natural language processing, [computer vision](https://zilliz.com/learn/what-is-computer-vision), and genomics, where data often exists in high-dimensional spaces. By leveraging advanced indexing and search algorithms, vector databases can quickly retrieve relevant data points, even in complex, vector embedding datasets.\n\n3. **Scalability**: Scalability is a critical requirement for modern AI applications, and vector databases are built to scale efficiently. Whether dealing with millions or billions of vectors, vector databases can handle the growing demands of AI applications through horizontal scaling. This ensures that performance remains consistent even as data volumes increase.\n\n4. **Flexibility**: Vector databases offer remarkable flexibility in terms of data representation. They can store and manage various types of data, including numerical features, embeddings from text or images, and even complex data like molecular structures. This versatility makes vector databases a powerful tool for a wide range of applications, from text analysis to scientific research.\n\n5. **Real-time Applications**: Many vector databases are optimized for real-time or near-real-time querying. This is particularly important for applications that require quick responses, such as fraud detection, real-time recommendations, and interactive AI systems. The ability to perform rapid similarity searches ensures that these applications can deliver timely and relevant results.\n\n## Use Cases for Vector Databases\n\nVector databases have a wide range of applications across various industries, demonstrating their versatility and power. Here are some notable use cases:\n\n1. **Natural Language Processing**: In the realm of natural language processing (NLP), vector databases play a crucial role. They are used for tasks such as text classification, sentiment analysis, and language translation. By converting text into high-dimensional vector embeddings, vector databases enable efficient similarity searches and semantic understanding, enhancing the performance of [NLP models](https://zilliz.com/learn/7-nlp-models).\n\n2. **Computer Vision**: Vector databases are also widely used in computer vision applications. Tasks like image recognition, [object detection](https://zilliz.com/learn/what-is-object-detection), and image segmentation benefit from the ability of vector databases to handle high-dimensional image embeddings. This allows for quick and accurate retrieval of visually similar images, making vector databases indispensable in fields like autonomous driving, medical imaging, and digital asset management.\n\n3. **Genomics**: In genomics, vector databases are used to store and analyze genetic sequences, protein structures, and other molecular data. The high-dimensional nature of this data makes vector databases an ideal choice for managing and querying large genomic datasets. Researchers can perform vector searches to find genetic sequences with similar patterns, aiding in the discovery of genetic markers and the understanding of complex biological processes.\n\n4. **Recommendation Systems**: Vector databases are a cornerstone of modern recommendation systems. By storing user interactions and item features as vector embeddings, these databases can quickly identify items that are similar to those a user has previously interacted with. This capability enhances the accuracy and relevance of recommendations, improving user satisfaction and engagement.\n\n5. **Chatbots and Virtual Assistants**: Vector databases are used in chatbots and virtual assistants to provide real-time contextual answers to user queries. By converting user inputs into vector embeddings, these systems can perform similarity searches to find the most relevant responses. This enables chatbots and virtual assistants to deliver more accurate and contextually appropriate answers, enhancing the overall user experience.\n\nBy leveraging the unique capabilities of vector databases, organizations across various industries can build more intelligent, responsive, and scalable AI applications.\n\n## Vector Search Algorithms: From Theory to Practice\n\nVector databases require specialized indexing [algorithms](https://zilliz.com/learn/vector-index) to enable efficient similarity search in high-dimensional spaces. The algorithm selection directly impacts accuracy, speed, memory usage, and scalability.\n\n\n### Graph-Based Approaches\n\n**HNSW (**[**Hierarchical Navigable Small World**](https://zilliz.com/learn/hierarchical-navigable-small-worlds-HNSW)**)** creates navigable structures by connecting similar vectors, enabling efficient traversal during search. HNSW limits maximum connections per node and search scope to balance performance and accuracy, making it one of the most widely used algorithms for vector similarity search.\n\n**Cagra** is a graph-based index optimized specifically for GPU acceleration. It constructs navigable graph structures that align with GPU processing patterns, enabling massively parallel vector comparisons. What makes Cagra particularly effective is its ability to balance recall and performance through configurable parameters like graph degree and search width. Using inference-grade GPUs with Cagra can be more cost-effective than expensive training-grade hardware while still delivering high throughput, especially for large-scale vector collections. However, it's worth noting that GPU indexes like Cagra may not necessarily reduce latency compared to CPU indexes unless operating under high query pressure.\n\n\n### Quantization Techniques\n\n[**Product Quantization (PQ)**](https://zilliz.com/learn/scalar-quantization-and-product-quantization) decomposes high-dimensional vectors into smaller subvectors, quantizing each separately. This significantly reduces storage needs (often by 90%+) but introduces some accuracy loss.\n\n**Scalar Quantization (SQ)** converts 32-bit floats to 8-bit integers, reducing memory usage by 75% with minimal accuracy impact.\n\n\n### On-Disk Indexing: Cost-Effective Scaling\n\nFor large-scale vector collections (100M+ vectors), in-memory indexes become prohibitively expensive. For example, 100 million 1024-dimensional vectors would require approximately 400GB of RAM. This is where on-disk indexing algorithms like DiskANN provide significant cost benefits.\n\n[DiskANN](https://zilliz.com/learn/DiskANN-and-the-Vamana-Algorithm), based on the Vamana graph algorithm, enables efficient vector search while storing most of the index on NVMe SSDs rather than RAM. This approach offers several cost advantages:\n\n- **Reduced hardware costs**: Organizations can deploy vector search at scale using commodity hardware with modest RAM configurations\n\n- **Lower operational expenses**: Less RAM means lower power consumption and cooling costs in data centers\n\n- **Linear cost scaling**: Memory costs scale linearly with data volume, while performance remains relatively stable\n\n- **Optimized I/O patterns**: DiskANN's specialized design minimizes disk reads through careful graph traversal strategies\n\nThe trade-off is typically a modest increase in query latency (often just 2-3ms) compared to purely in-memory approaches, which is acceptable for many production use cases.\n\n\n### Specialized Index Types\n\n**Binary Embedding Indexes** are specialized for computer vision, image fingerprinting, and recommendation systems where data can be represented as binary features. These indexes serve different application needs. For image deduplication, digital watermarking, and copyright detection where exact matching is critical, optimized binary indexes provide precise similarity detection. For high-throughput recommendation systems, content-based image retrieval, and large-scale feature matching where speed is prioritized over perfect recall, binary indexes offer exceptional performance advantages.\n\n**Sparse Vector Indexes** are optimized for vectors where most elements are zero, with only a few non-zero values. Unlike dense vectors (where most or all dimensions contain meaningful values), sparse vectors efficiently represent data with many dimensions but few active features. This representation is particularly common in text processing where a document might use only a small subset of all possible words in a vocabulary. Sparse Vector Indexes excel in natural language processing tasks like semantic document search, full-text querying, and topic modeling. These indexes are particularly valuable for enterprise search across large document collections, legal document discovery where specific terms and concepts must be efficiently located, and academic research platforms indexing millions of papers with specialized terminology.\n\n\n## Advanced Query Capabilities\n\nAt the core of vector databases lies their ability to perform efficient semantic searches. Vector search capabilities range from basic similarity matching to advanced techniques for improving relevance and diversity.\n\n\n### Basic ANN Search\n\nApproximate Nearest Neighbor (ANN) search is the foundational search method in vector databases. Unlike exact k-Nearest Neighbors (kNN) search, which compares a query vector against every vector in the database, ANN search uses indexing structures to quickly identify a subset of vectors likely to be most similar, dramatically improving performance.\n\nThe key components of ANN search include:\n\n- **Query vectors**: The vector representation of what you're searching for\n\n- **Index structures**: Pre-built data structures that organize vectors for efficient retrieval\n\n- **Metric types**: Mathematical functions like Euclidean (L2), Cosine, or Inner Product that measure similarity between vectors\n\n- **Top-K results**: The specified number of most similar vectors to return\n\nVector databases provide optimizations to improve search efficiency:\n\n- **Bulk vector search**: Searching with multiple query vectors in parallel\n\n- **Partitioned search**: Limiting search to specific data partitions\n\n- **Pagination**: Using limit and offset parameters for retrieving large result sets\n\n- **Output field selection**: Controlling which entity fields are returned with results\n\n\n### Advanced Search Techniques\n\n#### Range Search\n\nRange search improves result relevancy by restricting results to vectors with similarity scores falling within a specific range. Unlike standard ANN search which returns the top-K most similar vectors, range search defines an \"annular region\" using:\n\n- An outer boundary (radius) that sets the maximum allowable distance\n\n- An inner boundary (range_filter) that can exclude vectors that are too similar\n\nThis approach is particularly useful when you want to find \"similar but not identical\" items, such as product recommendations that are related but not exact duplicates of what a user has already viewed.\n\n\n#### Filtered Search\n\nFiltered search combines vector similarity with metadata constraints to narrow results to vectors that match specific criteria. For example, in a product catalog, you could find visually similar items but restrict results to a specific brand or price range.\n\nHighly Scalable vector databases support two filtering approaches:\n\n- **Standard filtering**: Applies metadata filters before vector search, significantly reducing the candidate pool\n\n- **Iterative filtering**: Performs vector search first, then applies filters to each result until reaching the desired number of matches\n\n\n#### Text Match\n\nText match enables precise document retrieval based on specific terms, complementing vector similarity search with exact text matching capabilities. Unlike semantic search, which finds conceptually similar content, text match focuses on finding exact occurrences of query terms.\n\nFor example, a product search might combine text match to find products that explicitly mention \"waterproof\" with vector similarity to find visually similar products, ensuring both semantic relevance and specific feature requirements are met.\n\n\n#### Grouping Search\n\nGrouping search aggregates results by a specified field to improve result diversity. For example, in a document collection where each paragraph is a separate vector, grouping ensures results come from different documents rather than multiple paragraphs from the same document.\n\nThis technique is valuable for:\n\n- Document retrieval systems where you want representation from different sources\n\n- Recommendation systems that need to present diverse options\n\n- Search systems where result diversity is as important as similarity\n\n\n#### Hybrid Search\n\nHybrid search combines results from multiple vector fields, each potentially representing different aspects of the data or using different embedding models. This enables:\n\n- **Sparse-dense vector combinations**: Combining semantic understanding (dense vectors) with keyword matching (sparse vectors) for more comprehensive text search\n\n- **Multimodal search**: Finding matches across different data types, such as searching for products using both image and text inputs\n\nHybrid search implementations use sophisticated reranking strategies to combine results:\n\n- **Weighted ranking**: Prioritizes results from specific vector fields\n\n- **Reciprocal Rank Fusion**: Balances results across all vector fields without specific emphasis\n\n\n#### Full-Text Search\n\nFull-text search capabilities in modern vector databases bridge the gap between traditional text search and vector similarity. These systems:\n\n- Automatically convert raw text queries into sparse embeddings\n\n- Retrieve documents containing specific terms or phrases\n\n- Rank results based on both term relevance and semantic similarity\n\n- Complement vector search by catching exact matches that semantic search might miss\n\nThis hybrid approach is particularly valuable for comprehensive [information retrieval](https://zilliz.com/learn/what-is-information-retrieval) systems that need both precise term matching and semantic understanding.\n\n\n## Performance Engineering: Metrics That Matter\n\nPerformance optimization in vector databases requires understanding key metrics and their tradeoffs.\n\n\n### The Recall-Throughput Tradeoff\n\nRecall measures the proportion of true nearest neighbors found among returned results. Higher recall requires more extensive search, reducing throughput (queries per second). Production systems balance these metrics based on application requirements, typically targeting 80-99% recall depending on use case.\n\nWhen evaluating vector database performance, standardized benchmarking environments like ANN-Benchmarks provide valuable comparative data. These tools measure critical metrics including:\n\n- Search recall: The proportion of queries for which true nearest neighbors are found among returned results\n\n- Queries per second (QPS): The rate at which the database processes queries under standardized conditions\n\n- Performance across different dataset sizes and dimensions\n\nAn alternative is an open source benchmark system called [VDB Bench](https://zilliz.com/vector-database-benchmark-tool?database=ZillizCloud%2CMilvus%2CElasticCloud%2CPgVector%2CPinecone%2CQdrantCloud%2CWeaviateCloud\u0026dataset=medium\u0026filter=none%2Clow%2Chigh\u0026tab=1). VectorDBBench is an [open-source benchmarking tool](https://github.com/zilliztech/VectorDBBench) designed to evaluate and compare the performance of mainstream vector databases such as Milvus and Zilliz Cloud using their own datasets. It also helps developers choose the most suitable vector database for their use cases.\n\nThese benchmarks allow organizations to identify the most suitable vector database implementation for their specific requirements, considering the balance between accuracy, speed, and scalability.\n\n\n### Memory Management\n\nEfficient memory management enables vector databases to scale to billions of vectors while maintaining performance:\n\n- **Dynamic allocation** adjusts memory usage based on workload characteristics\n\n- **Caching policies** retain frequently accessed vectors in memory\n\n- **Vector compression techniques** significantly reduce memory requirements\n\nFor datasets that exceed memory capacity, disk-based solutions provide a crucial capability. These algorithms optimize I/O patterns for NVMe SSDs through techniques like beam search and graph-based navigation.\n\n\n### Advanced Filtering and Hybrid Search\n\nVector databases combine semantic similarity with traditional filtering to create powerful query capabilities:\n\n- **Pre-filtering** applies metadata constraints before vector search, reducing the candidate set for similarity comparison\n\n- **Post-filtering** executes vector search first, then applies filters to results\n\n- **Metadata indexing** improves filtering performance through specialized indexes for different data types\n\nPerformant vector databases support complex query patterns combining multiple vector fields with scalar constraints. Multi-vector queries find entities similar to multiple reference points simultaneously, while negative vector queries exclude vectors similar to specified examples.\n\n\n## Scaling Vector Databases in Production\n\nVector databases require thoughtful deployment strategies to ensure optimal performance at different scales:\n\n- **Small-scale deployments** (millions of vectors) can operate effectively on a single machine with sufficient memory\n\n- **Mid-scale deployments** (tens to hundreds of millions) benefit from vertical scaling with high-memory instances and SSD storage\n\n- **Billion-scale deployments** require horizontal scaling across multiple nodes with specialized roles\n\nSharding and replication form the foundation of scalable vector database architecture:\n\n- **Horizontal sharding** divides collections across multiple nodes\n\n- **Replication** creates redundant copies of data, improving both fault tolerance and query throughput\n\nModern systems adjust replication factors dynamically based on query patterns and reliability requirements.\n\n\n## Real-World Impact\n\nThe flexibility of high performant vector databases is evident in their deployment options. Systems can run across a spectrum of environments, from lightweight installations on laptops for prototyping to massive distributed clusters managing tens of billions of vectors. This scalability has enabled organizations to move from concept to production without changing database technologies.\n\nCompanies like Salesforce, PayPal, eBay, NVIDIA, IBM, and Airbnb now rely on vector databases like open source [Milvus](https://milvus.io/) to power large-scale AI applications. These implementations span diverse use cases—from sophisticated product recommendation systems to content moderation, fraud detection, and customer support automation—all built on the foundation of vector search.\n\nIn recent years, vector databases became vital in addressing the hallucination issues common in LLMs by providing domain-specific, up-to-date, or confidential data. For example, [Zilliz Cloud](https://zilliz.com/cloud) stores specialized data as vector embeddings. When a user asks a question, it transforms the query into vectors, performs ANN searches for the most relevant results, and combines these with the original question to create a comprehensive context for the large language models. This framework serves as the foundation for developing reliable LLM-powered applications that produce more accurate and contextually relevant responses.\n\n\n## Conclusion\n\nThe rise of vector databases represents more than just a new technology—it signifies a fundamental shift in how we approach data management for AI applications. By bridging the gap between unstructured data and computational systems, vector databases have become an essential component of the modern AI infrastructure, enabling applications that understand and process information in increasingly human-like ways.\n\nThe key advantages of vector databases over traditional database systems include:\n\n- High-dimensional search: Efficient similarity searches on high-dimensional vectors used in machine learning and Generative AI applications\n\n- Scalability: Horizontal scaling for efficient storage and retrieval of large vector collections\n\n- Flexibility with hybrid search: Handling various vector data types, including sparse and dense vectors\n\n- Performance: Significantly faster vector similarity searches compared to traditional databases\n\n- Customizable indexing: Support for custom indexing schemes optimized for specific use cases and data types\n\nAs AI applications become increasingly sophisticated, the demands on vector databases continue to evolve. Modern systems must balance performance, accuracy, scaling, and cost-effectiveness while integrating seamlessly with the broader AI ecosystem. For organizations looking to implement AI at scale, understanding vector database technology isn't just a technical consideration—it's a strategic imperative.","title":"What Exactly is a Vector Database and How Does It Work","metaData":{}},{"id":"stop-use-outdated-rag-deepsearcher-agentic-rag-approaches-changes-everything.md","author":"Cheney Zhang","recommend":true,"canonicalUrl":"https://milvus.io/blog/stop-use-outdated-rag-deepsearcher-agentic-rag-approaches-changes-everything.md","date":"2025-03-23T00:00:00.000Z","cover":"https://assets.zilliz.com/Stop_Using_Outdated_RAG_Deep_Searcher_s_Agentic_RAG_Approach_Changes_Everything_b2eaa644cf.png","tags":["Engineering"],"href":"/blog/stop-use-outdated-rag-deepsearcher-agentic-rag-approaches-changes-everything.md","content":"\n\n## The Shift to AI-Powered Search with LLMs and Deep Research\n\nThe evolution of search technology has progressed dramatically over the decades—from keyword-based retrieval in the pre-2000s to personalized search experiences in the 2010s. We're witnessing the emergence of AI-powered solutions capable of handling complex queries requiring in-depth, professional analysis.\n\nOpenAI's Deep Research exemplifies this shift, using reasoning capabilities to synthesize large amounts of information and generate multi-step research reports. For example, when asked about \"What is Tesla's reasonable market cap?\" Deep Research can comprehensively analyze corporate finances, business growth trajectories, and market value estimations.\n\nDeep Research implements an advanced form of the RAG (Retrieval-Augmented Generation) framework at its core. Traditional RAG enhances language model outputs by retrieving and incorporating relevant external information. OpenAI's approach takes this further by implementing iterative retrieval and reasoning cycles. Instead of a single retrieval step, Deep Research dynamically generates multiple queries, evaluates intermediate results, and refines its search strategy—demonstrating how advanced or agentic RAG techniques can deliver high-quality, enterprise-level content that feels more like professional research than simple question-answering.\n\n\n## DeepSearcher: A Local Deep Research Bringing Agentic RAG to Everyone\n\nInspired by these advancements, developers worldwide have been creating their own implementations. Zilliz engineers built and open-sourced the [DeepSearcher](https://github.com/zilliztech/deep-searcher) project, which can be considered a local and open-source Deep Research. This project has garnered over 4,900 GitHub stars in less than a month. \n\nDeepSearcher redefines AI-powered enterprise search by combining the power of advanced reasoning models, sophisticated search features, and an integrated research assistant. Integrating local data via [Milvus](https://milvus.io/docs/overview.md) (a high-performance and open-source vector database), DeepSearcher delivers faster, more relevant results while allowing users to swap core models for a customized experience easily.\n\n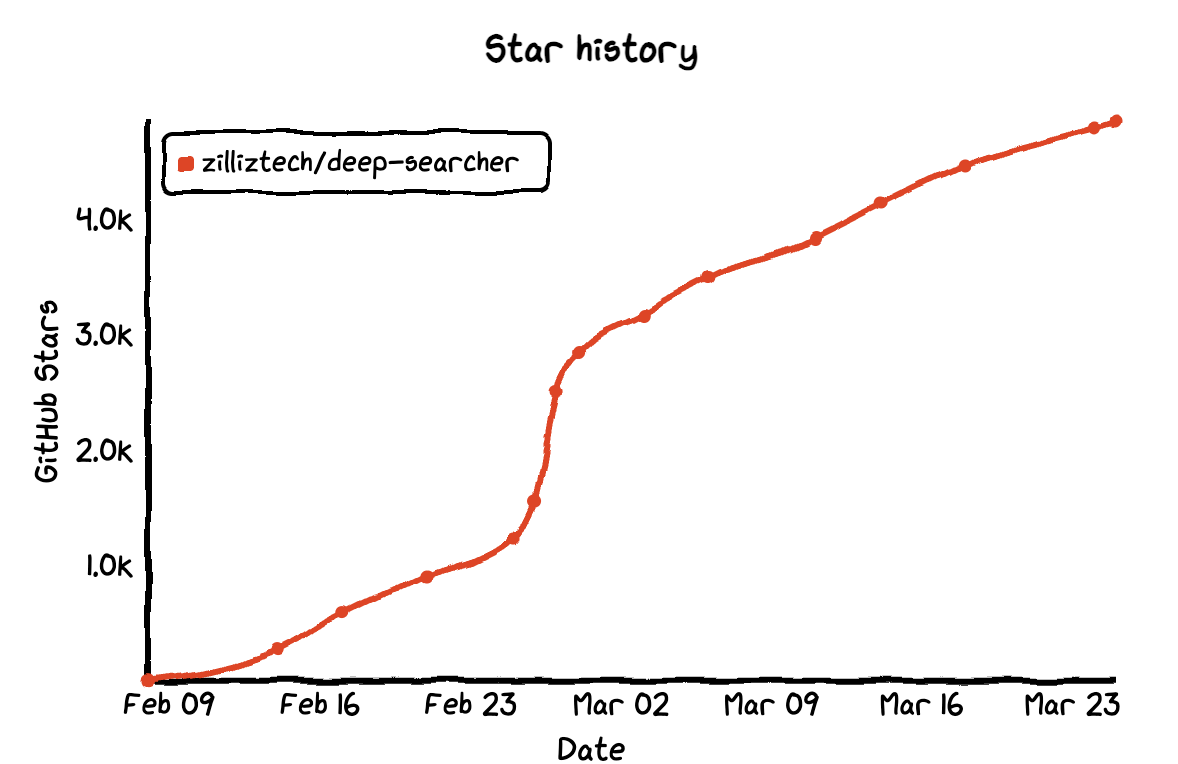\n\n_Figure 1:_ _DeepSearcher’s star history (_[_Source_](https://www.star-history.com/#zilliztech/deep-searcher\u0026Date)_)_\n\nIn this article, we'll explore the evolution from traditional RAG to Agentic RAG, exploring what specifically makes these approaches different on a technical level. We'll then discuss DeepSearcher's implementation, showing how it leverages intelligent agent capabilities to enable dynamic, multi-turn reasoning—and why this matters for developers building enterprise-level search solutions.\n\n\n## From Traditional RAG to Agentic RAG: The Power of Iterative Reasoning\n\nAgentic RAG enhances the traditional RAG framework by incorporating intelligent agent capabilities. DeepSearcher is a prime example of an agentic RAG framework. Through dynamic planning, multi-step reasoning, and autonomous decision-making, it establishes a closed-loop process that retrieves, processes, validates, and optimizes data to solve complex problems.\n\nThe growing popularity of Agentic RAG is driven by significant advancements in large language model (LLM) reasoning capabilities, particularly their improved ability to break down complex problems and maintain coherent chains of thought across multiple steps.\n\n| | | |\n| --------------------------- | ------------------------------------------------------------------------------ | --------------------------------------------------------------------------------------------------------------------------------------------------------------- |\n| **Comparison Dimension** | **Traditional RAG** | **Agentic RAG** |\n| Core Approach | Passive and reactive | Proactive, agent-driven |\n| Process Flow | Single-step retrieval and generation (one-time process) | Dynamic, multi-step retrieval and generation (iterative refinement) |\n| Retrieval Strategy | Fixed keyword search, dependent on initial query | Adaptive retrieval (e.g., keyword refinement, data source switching) |\n| Complex Query Handling | Direct generation; prone to errors with conflicting data | Task decomposition → targeted retrieval → answer synthesis |\n| Interaction Capability | Relies entirely on user input; no autonomy | Proactive engagement (e.g., clarifying ambiguities, requesting details) |\n| Error Correction \u0026 Feedback | No self-correction; limited by initial results | Iterative validation → self-triggered re-retrieval for accuracy |\n| Ideal Use Cases | Simple Q\u0026A, factual lookups | Complex reasoning, multi-stage problem-solving, open-ended tasks |\n| Example | User asks: \"What is quantum computing?\" → System returns a textbook definition | User asks: \"How can quantum computing optimize logistics?\" → System retrieves quantum principles and logistics algorithms, then synthesizes actionable insights |\n\nUnlike traditional RAG, which relies on a single, query-based retrieval, Agentic RAG breaks down a query into multiple sub-questions and iteratively refines its search until it reaches a satisfactory answer. This evolution offers three primary benefits:\n\n- **Proactive Problem-Solving:** The system transitions from passively reacting to actively solving problems.\n\n- **Dynamic, Multi-Turn Retrieval:** Instead of performing a one-time search, the system continually adjusts its queries and self-corrects based on ongoing feedback.\n\n- **Broader Applicability:** It extends beyond basic fact-checking to handle complex reasoning tasks and generate comprehensive reports.\n\nBy leveraging these capabilities, Agentic RAG apps like DeepSearcher operate much like a human expert—delivering not only the final answer but also a complete, transparent breakdown of its reasoning process and execution details.\n\nIn the long term, Agentic RAG is set to overtake baseline RAG systems. Conventional approaches often struggle to address the underlying logic in user queries, which require iterative reasoning, reflection, and continuous optimization.\n\n\n## What Does an Agentic RAG Architecture Look Like? DeepSearcher as an Example \n\nNow that we’ve understood the power of agentic RAG systems, what does their architecture look like? Let’s take DeepSearcher as an example. \n\n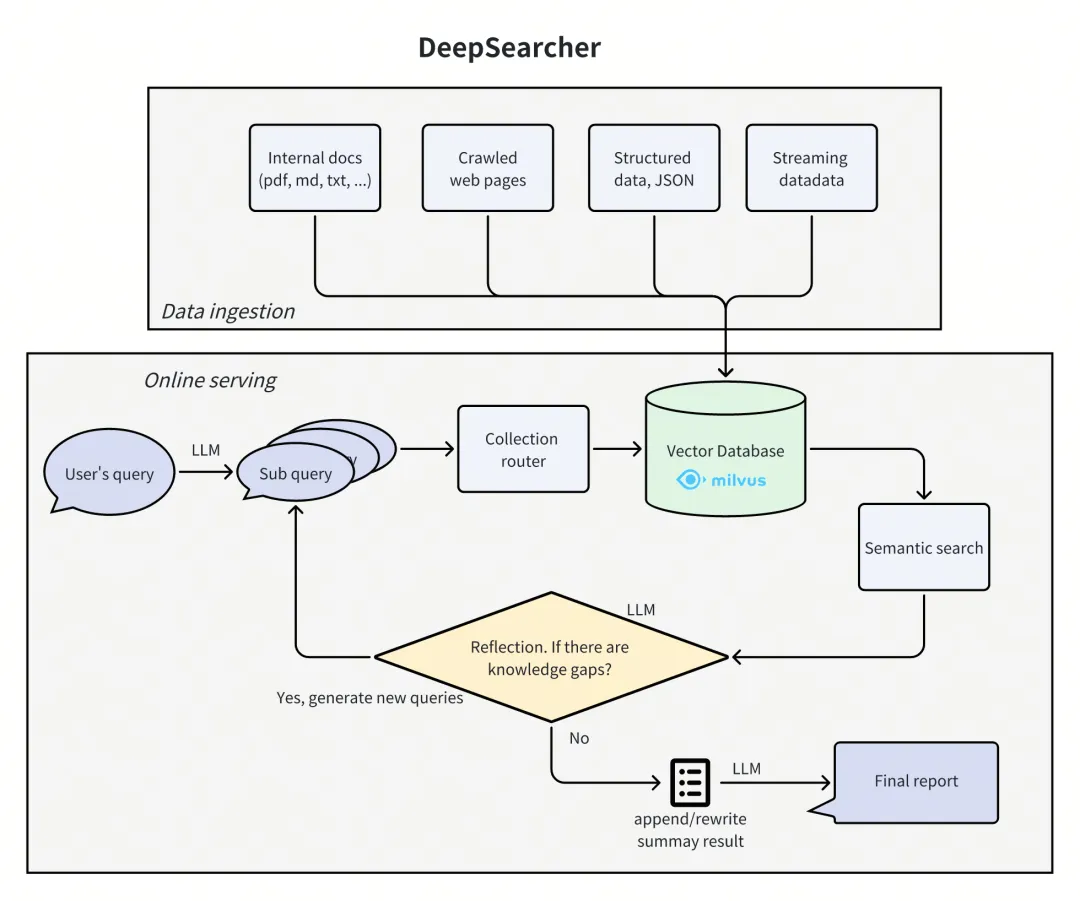\n\n\n_Figure 2: Two Modules Within DeepSearcher_\n\nDeepSearcher's architecture consists of two primary modules:\n\n\n### 1. Data Ingestion Module\n\nThis module connects various third-party proprietary data sources via a Milvus vector database. It is especially valuable for enterprise environments that rely on proprietary datasets. The module handles:\n\n- Document parsing and chunking\n\n- Embedding generation\n\n- Vector storage and indexing\n\n- Metadata management for efficient retrieval\n\n\n### 2. Online Reasoning and Query Module\n\nThis component implements diverse agent strategies within the RAG framework to deliver precise, insightful responses. It operates on a dynamic, iterative loop—after each data retrieval, the system reflects on whether the accumulated information sufficiently answers the original query. If not, another iteration is triggered; if yes, the final report is generated.\n\nThis ongoing cycle of \"follow-up\" and \"reflection\" represents a fundamental improvement over other basic RAG approaches. While traditional RAG performs a one-shot retrieval and generation process, DeepSearcher's iterative approach mirrors how human researchers work—asking initial questions, evaluating the information received, identifying gaps, and pursuing new lines of inquiry. \n\n\n## How Effective is DeepSearcher, and What Use Cases is It Best Suited For?\n\nOnce installed and configured, DeepSearcher indexes your local files through the Milvus vector database. When you submit a query, it performs a comprehensive, in-depth search of this indexed content. A key advantage for developers is that the system logs every step of its search and reasoning process, providing transparency into how it arrived at its conclusions—a critical feature for debugging and optimizing RAG systems.\n\n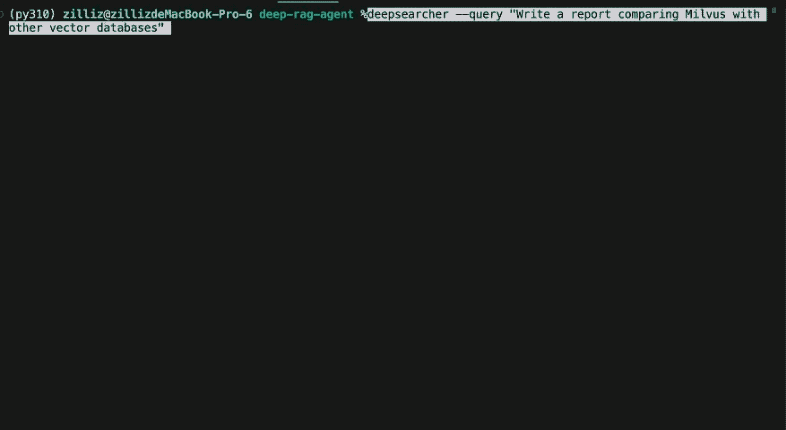\n\n_Figure 3: Accelerated Playback of DeepSearcher Iteration_\n\nThis approach consumes more computational resources than traditional RAG but delivers better results for complex queries. Let's discuss two specific use cases where DeepSearcher is best suited for. \n\n\n### 1. Overview-Type Queries\n\nOverview-type queries—such as generating reports, drafting documents, or summarizing trends—provide a brief topic but require an exhaustive, detailed output.\n\nFor example, when querying \"How has The Simpsons changed over time?\", DeepSearcher first generates an initial set of sub-queries:\n\n\n```\n_Break down the original query into new sub queries: [_\n\n_'How has the cultural impact and societal relevance of The Simpsons evolved from its debut to the present?',_\n\n_'What changes in character development, humor, and storytelling styles have occurred across different seasons of The Simpsons?',_\n\n_'How has the animation style and production technology of The Simpsons changed over time?',_\n\n_'How have audience demographics, reception, and ratings of The Simpsons shifted throughout its run?']_\n```\n\nIt retrieves relevant information, and then iterates with feedback to refine its search, generating the next sub-queries:\n\n```\n_New search queries for next iteration: [_\n\n_\"How have changes in The Simpsons' voice cast and production team influenced the show's evolution over different seasons?\",_\n\n_\"What role has The Simpsons' satire and social commentary played in its adaptation to contemporary issues across decades?\",_\n\n_'How has The Simpsons addressed and incorporated shifts in media consumption, such as streaming services, into its distribution and content strategies?']_\n```\n\nEach iteration builds on the previous one, culminating in a comprehensive report that covers multiple facets of the subject, structured with sections like:\n\n\n\n```\n**Report: The Evolution of _The Simpsons_ (1989–Present)**\n**1. Cultural Impact and Societal Relevance** \n_The Simpsons_ debuted as a subversive critique of American middle-class life, gaining notoriety for its bold satire in the 1990s. Initially a countercultural phenomenon, it challenged norms with episodes tackling religion, politics, and consumerism. Over time, its cultural dominance waned as competitors like _South Park_ and _Family Guy_ pushed boundaries further. By the 2010s, the show transitioned from trendsetter to nostalgic institution, balancing legacy appeal with attempts to address modern issues like climate change and LGBTQ+ rights, albeit with less societal resonance.\n**2. Character Development and Storytelling Shifts** \nEarly seasons featured nuanced character arcs (e.g., Lisa’s activism, Marge’s resilience), but later seasons saw \"Flanderization\" (exaggerating traits, e.g., Homer’s stupidity, Ned Flanders’ piety). Humor evolved from witty, character-driven satire to reliance on pop culture references and meta-humor. Serialized storytelling in early episodes gave way to episodic, gag-focused plots, often sacrificing emotional depth for absurdity.\n[...]\n**12. Merchandising and Global Reach** \nThe 1990s merchandise boom (action figures, _Simpsons_-themed cereals) faded, but the franchise persists via collaborations (e.g., _Fortnite_ skins, Lego sets). International adaptations include localized dubbing and culturally tailored episodes (e.g., Japanese _Itchy \u0026 Scratchy_ variants).\n**Conclusion** \n_The Simpsons_ evolved from a radical satire to a television institution, navigating shifts in technology, politics, and audience expectations. While its golden-age brilliance remains unmatched, its adaptability—through streaming, updated humor, and global outreach—secures its place as a cultural touchstone. The show’s longevity reflects both nostalgia and a pragmatic embrace of change, even as it grapples with the challenges of relevance in a fragmented media landscape.\n```\n\n_(For brevity, only excerpts of the process and final report are shown)_\n\nThe final report provides a thorough analysis with proper citations and structured organization.\n\n\n### 2. Complex Reasoning Queries\n\nComplex queries involve multiple layers of logic and interconnected entities.\n\nConsider the query: \"Which film has the director who is older, God's Gift To Women or Aldri annet enn bråk?\"\n\nWhile this might seem simple to a human, simple RAG systems struggle with it because the answer isn't stored directly in the knowledge base. DeepSearcher tackles this challenge by decomposing the query into smaller sub-questions:\n\n```\n[\"Who is the director of God'S Gift To Women?\", 'Who is the director of Aldri annet enn bråk?', 'What are the ages of the respective directors?', 'Which director is older?']\n```\n\nIt first retrieves information on the directors of both films, \n\n```\nThe director of \"God's Gift To Women\" is Michael Curtiz, the director of \"Aldri annet enn bråk\" is Edith Carlmar\n```\n\nthen generates sub-queries: \n\n```\n[\"Find the birthdate of Michael Curtiz, the director of God's Gift To Women\", 'Find the birthdate of Edith Carlmar, the director of Aldri annet enn bråk']\n```\n\nand then extracts their birth dates, and finally compares them to determine the correct answer:\n\n```\nThe director of \"God's Gift To Women\" is Michael Curtiz, who was born on December 24, 1886 and passed away on April 11, 1962. He was a Hungarian-born American film director known for his prolific career in Hollywood.\nOn the other hand, the director of \"Aldri annet enn bråk\" is Edith Carlmar, who was born on November 15, 1911 and passed away on May 17, 2003.\nTherefore, Michael Curtiz is older than Edith Carlmar.\n```\n\nIn contrast, a conventional RAG system might miss key details due to limited retrieval in a single pass, potentially resulting in [hallucinated](https://zilliz.com/blog/decoding-llm-hallucinations-deep-dive-into-llm-errors) or inaccurate answers:\n\n```\n1. **Aldri annet enn bråk** (1954) is directed by **Edith Carlmar**, who was born on November 15, 1911.\n2. The related chunks did not provide specific details about the director of \"God's Gift to Women.\" However, if we look at external sources for \"God's Gift to Women,\" the film was directed by **L. M. (Lyman) Steinberg**, who was born on December 19, 1905.\nBy comparing their birth dates:\n- Edith Carlmar: November 15, 1911\n- L. M. Steinberg: December 19, 1905\n**Conclusion**: L. M. Steinberg, the director of \"God's Gift to Women,\" is older than Edith Carlmar, the director of \"Aldri annet enn bråk.\"\n```\n\nDeepSearcher stands out by performing deep, iterative searches on imported local data. It logs each step of its reasoning process and ultimately delivers a comprehensive and unified report. This makes it particularly effective for overview-type queries—such as generating detailed reports or summarizing trends—and for complex reasoning queries that require breaking down a question into smaller sub-questions and aggregating data through multiple feedback loops.\n\nIn the next section, we will compare DeepSearcher with other RAG systems, exploring how its iterative approach and flexible model integration stack up against traditional methods.\n\n\n## Quantitative Comparison: DeepSearcher vs. Traditional RAG\n\nIn the DeepSearcher GitHub repository, we've made available code for quantitative testing. For this analysis, we used the popular 2WikiMultiHopQA dataset. (Note: We evaluated only the first 50 entries to manage API token consumption, but the overall trends remain clear.)\n\n\n### Recall Rate Comparison\n\nAs shown in Figure 4, the recall rate improves significantly as the number of maximum iterations increases:\n\n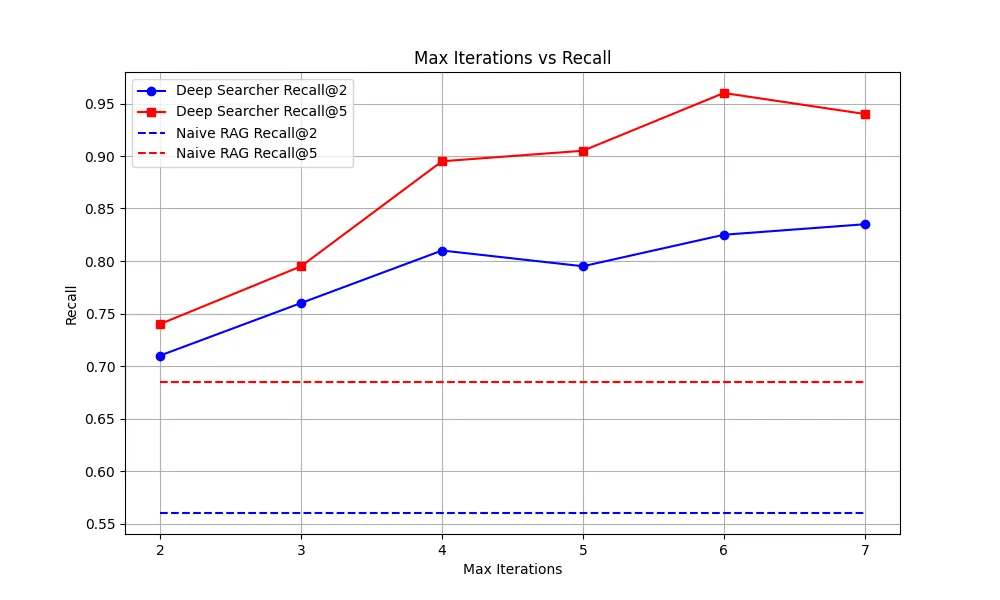\n\n_Figure 4: Max Iterations vs. Recall_\n\nAfter a certain point, the marginal improvements taper off—hence, we typically set the default to 3 iterations, though this can be adjusted based on specific needs.\n\n\n### Token Consumption Analysis\n\nWe also measured the total token usage for 50 queries across different iteration counts:\n\n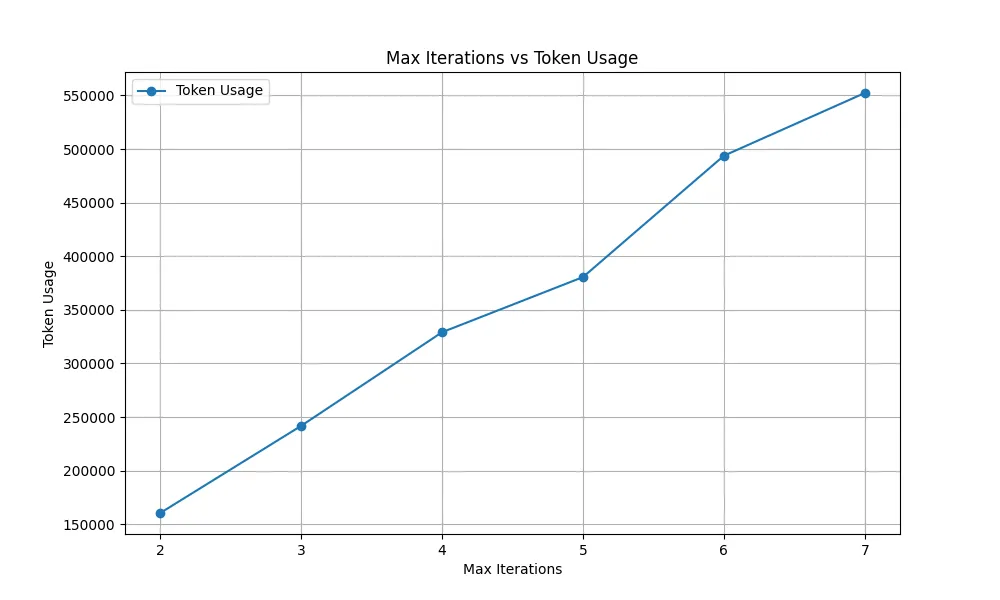\n\n_Figure 5: Max Iterations vs. Token Usage_\n\nThe results show that token consumption increases linearly with more iterations. For example, with 4 iterations, DeepSearcher consumes roughly 0.3M tokens. Using a rough estimate based on OpenAI's gpt-4o-mini pricing of $0.60/1M output tokens, this equates to an average cost of about $0.0036 per query (or roughly $0.18 for 50 queries).\n\nFor more resource-intensive inference models, the costs would be several times higher due to both higher per-token pricing and larger token outputs.\n\n\n### Model Performance Comparison\n\nA significant advantage of DeepSearcher is its flexibility in switching between different models. We tested various inference models and non-inference models (like gpt-4o-mini). Overall, inference models—especially Claude 3.7 Sonnet—tended to perform the best, although the differences weren't dramatic.\n\n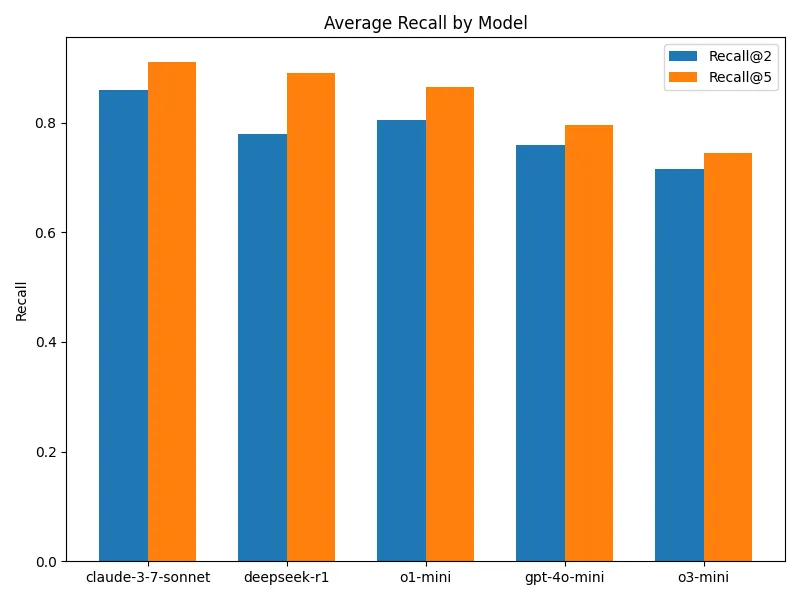\n\n_Figure 6: Average Recall by Model_\n\nNotably, some smaller non-inference models sometimes couldn't complete the full agent query process because of their limited ability to follow instructions—a common challenge for many developers working with similar systems.\n\n\n## DeepSearcher (Agentic RAG) vs. Graph RAG\n\n[Graph RAG](https://zilliz.com/blog/graphrag-explained-enhance-rag-with-knowledge-graphs) is also able to handle complex queries, particularly multi-hop queries. Then, what is the difference between DeepSearcher (Agentic RAG) and Graph RAG? \n\nGraph RAG is designed to query documents based on explicit relational links, making it particularly strong in multi-hop queries. For instance, when processing a long novel, Graph RAG can precisely extract the intricate relationships between characters. However, this method requires substantial token usage during data import to map out these relationships, and its query mode tends to be rigid—typically effective only for single-relationship queries.\n\n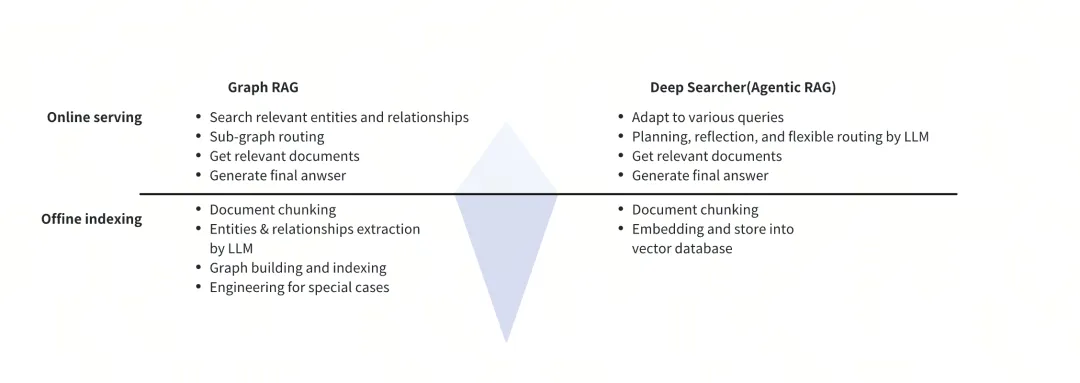\n\n_Figure 7: Graph RAG vs. DeepSearcher_\n\nIn contrast, Agentic RAG—as exemplified by DeepSearcher—takes a fundamentally different approach. It minimizes token consumption during data import and instead invests computational resources during query processing. This design choice creates important technical tradeoffs:\n\n1. Lower upfront costs: DeepSearcher requires less preprocessing of documents, making initial setup faster and less expensive\n\n2. Dynamic query handling: The system can adjust its retrieval strategy on-the-fly based on intermediate findings\n\n3. Higher per-query costs: Each query requires more computation than Graph RAG, but delivers more flexible results\n\nFor developers, this distinction is crucial when designing systems with different usage patterns. Graph RAG may be more efficient for applications with predictable query patterns and high query volume, while DeepSearcher's approach excels in scenarios requiring flexibility and handling unpredictable, complex queries.\n\nLooking ahead, as the cost of LLMs drops and inference performance continues to improve, Agentic RAG systems like DeepSearcher are likely to become more prevalent. The computational cost disadvantage will diminish, while the flexibility advantage will remain.\n\n\n## DeepSearcher vs. Deep Research\n\nUnlike OpenAI's Deep Research, DeepSearcher is specifically tailored for the deep retrieval and analysis of private data. By leveraging a vector database, DeepSearcher can ingest diverse data sources, integrate various data types, and store them uniformly in a vector-based knowledge repository. Its robust semantic search capabilities enable it to efficiently search through vast amounts of offline data.\n\nFurthermore, DeepSearcher is completely open source. While Deep Research remains a leader in content generation quality, it comes with a monthly fee and operates as a closed-source product, meaning its internal processes are hidden from users. In contrast, DeepSearcher provides full transparency—users can examine the code, customize it to suit their needs, or even deploy it in their own production environments.\n\n\n## Technical Insights \n\nThroughout the development and subsequent iterations of DeepSearcher, we've gathered several important technical insights:\n\n\n### Inference Models: Effective but Not Infallible\n\nOur experiments reveal that while inference models perform well as agents, they sometimes overanalyze straightforward instructions, leading to excessive token consumption and slower response times. This observation aligns with the approach of major AI providers like OpenAI, which no longer distinguish between inference and non-inference models. Instead, model services should automatically determine the necessity of inference based on specific requirements to conserve tokens.\n\n\n### The Imminent Rise of Agentic RAG\n\nFrom a demand perspective, deep content generation is essential; technically, enhancing RAG effectiveness is also crucial. In the long run, cost is the primary barrier to the widespread adoption of Agentic RAG. However, with the emergence of cost-effective, high-quality LLMs like DeepSeek-R1 and the cost reductions driven by Moore's Law, the expenses associated with inference services are expected to decrease.\n\n\n### The Hidden Scaling Limit of Agentic RAG\n\nA critical finding from our research concerns the relationship between performance and computational resources. Initially, we hypothesized that simply increasing the number of iterations and token allocation would proportionally improve results for complex queries.\n\nOur experiments revealed a more nuanced reality: while performance does improve with additional iterations, we observed clear diminishing returns. Specifically:\n\n- Performance increased sharply from 1 to 3 iterations\n\n- Improvements from 3 to 5 iterations were modest\n\n- Beyond 5 iterations, gains were negligible despite significant increases in token consumption\n\nThis finding has important implications for developers: simply throwing more computational resources at RAG systems isn't the most efficient approach. The quality of the retrieval strategy, the decomposition logic, and the synthesis process often matter more than raw iteration count. This suggests that developers should focus on optimizing these components rather than just increasing token budgets.\n\n\n### The Evolution Beyond Traditional RAG\n\nTraditional RAG offers valuable efficiency with its low-cost, single-retrieval approach, making it suitable for straightforward question-answering scenarios. Its limitations become apparent, however, when handling queries with complex implicit logic.\n\nConsider a user query like \"How to earn 100 million in a year.\" A traditional RAG system might retrieve content about high-earning careers or investment strategies, but would struggle to:\n\n1. Identify unrealistic expectations in the query\n\n2. Break down the problem into feasible sub-goals\n\n3. Synthesize information from multiple domains (business, finance, entrepreneurship)\n\n4. Present a structured, multi-path approach with realistic timelines\n\nThis is where Agentic RAG systems like DeepSearcher show their strength. By decomposing complex queries and applying multi-step reasoning, they can provide nuanced, comprehensive responses that better address the user's underlying information needs. As these systems become more efficient, we expect to see their adoption accelerate across enterprise applications.\n\n\n## Conclusion\n\nDeepSearcher represents a significant evolution in RAG system design, offering developers a powerful framework for building more sophisticated search and research capabilities. Its key technical advantages include:\n\n1. Iterative reasoning: The ability to break down complex queries into logical sub-steps and progressively build toward comprehensive answers\n\n2. Flexible architecture: Support for swapping underlying models and customizing the reasoning process to suit specific application needs\n\n3. Vector database integration: Seamless connection to Milvus for efficient storage and retrieval of vector embeddings from private data sources\n\n4. Transparent execution: Detailed logging of each reasoning step, enabling developers to debug and optimize system behavior\n\nOur performance testing confirms that DeepSearcher delivers superior results for complex queries compared to traditional RAG approaches, though with clear tradeoffs in computational efficiency. The optimal configuration (typically around 3 iterations) balances accuracy against resource consumption.\n\nAs LLM costs continue to decrease and reasoning capabilities improve, the Agentic RAG approach implemented in DeepSearcher will become increasingly practical for production applications. For developers working on enterprise search, research assistants, or knowledge management systems, DeepSearcher offers a powerful open-source foundation that can be customized to specific domain requirements.\n\nWe welcome contributions from the developer community and invite you to explore this new paradigm in RAG implementation by checking out our [GitHub repository](https://github.com/zilliztech/deep-searcher).\n","title":"Stop Using Outdated RAG: DeepSearcher's Agentic RAG Approach Changes Everything\n","metaData":{}},{"id":"why-manual-sharding-is-a-bad-idea-for-vector-databases-and-how-to-fix-it.md","author":"James Luan","desc":"Discover why manual vector database sharding creates bottlenecks and how Milvus's automated scaling eliminates engineering overhead for seamless growth.","tags":["Engineering"],"recommend":true,"canonicalUrl":"https://milvus.io/blog/why-manual-sharding-is-a-bad-idea-for-vector-databases-and-how-to-fix-it.md","date":"2025-03-18T00:00:00.000Z","cover":"https://assets.zilliz.com/Why_Manual_Sharding_is_a_Bad_Idea_for_Vector_Database_And_How_to_Fix_It_1_968a5be504.png","href":"/blog/why-manual-sharding-is-a-bad-idea-for-vector-databases-and-how-to-fix-it.md","content":"\n_\"We initially built our semantic search on pgvector instead of Milvus because all our relational data was already in PostgreSQL,\"_ recalls Alex, CTO of an enterprise AI SaaS startup. _\"But as soon as we hit product-market fit, our growth ran into serious hurdles on the engineering side. It quickly became clear that pgvector wasn’t designed for scalability. Simple tasks such as rolling out schema updates across multiple shards turned into tedious, error-prone processes that consumed days of engineering effort. When we reached 100 million vector embeddings, query latency spiked to over a second, something far beyond what our customers would tolerate. After moving to Milvus, sharding manually felt like stepping into the stone age. It’s no fun juggling shard servers as if they were fragile artifacts. No company should have to endure that.\"_\n\n\n## A Common Challenge for AI Companies\n\nAlex's experience isn't unique to pgvector users. Whether you're using pgvector, Qdrant, Weaviate, or any other vector database that relies on manual sharding, the scaling challenges remain the same. What starts as a manageable solution quickly turns into a tech debt as data volumes grow.\n\nFor startups today, **scalability isn't optional—it's mission-critical**. This is especially true for AI products powered by Large Language Models(LLM) and vector databases, where the leap from early adoption to exponential growth can happen overnight. Achieving product-market fit often triggers a surge in user growth, overwhelming data inflows, and skyrocketing query demands. But if the database infrastructure can't keep up, slow queries and operational inefficiencies can stall momentum and hinder business success.\n\nA short-term technical decision could lead to long-term bottleneck, forcing engineering teams to constantly address urgent performance issues, database crashes, and system failures instead of focusing on innovation. The worst-case scenario? A costly, time-consuming database re-architecture—precisely when a company should be scaling.\n\n\n## Isn’t Sharding a Natural Solution to Scalability?\n\nScalability can be addressed in multiple ways. The most straightforward approach, **Scaling Up**, involves enhancing a single machine’s resources by adding more CPU, memory, or storage to accommodate growing data volumes. While simple, this method has clear limitations. In a Kubernetes environment, for example, large pods are inefficient, and relying on a single node increases the risk of failure, potentially leading to significant downtime.\n\nWhen Scaling Up is no longer viable, businesses naturally turn to **Scaling Out**, distributing data across multiple servers. At first glance, **sharding** appears to be a simple solution—splitting a database into smaller, independent databases to increase capacity and enable multiple writable primary nodes.\n\nHowever, while conceptually straightforward, sharding quickly becomes a complex challenge in practice. Most applications are initially designed to work with a single, unified database. The moment a vector database is divided into multiple shards, every part of the application that interacts with data must be modified or entirely rewritten, introducing significant development overhead. Designing an effective sharding strategy becomes crucial, as does implementing routing logic to ensure data is directed to the correct shard. Managing atomic transactions across multiple shards often requires restructuring applications to avoid cross-shard operations. Additionally, failure scenarios must be handled gracefully to prevent disruptions when certain shards become unavailable.\n\n\n## Why Manual Sharding Becomes a Burden\n\n_\"We originally estimated implementing manual sharding for our pgvector database would take two engineers about six months,\"_ Alex remembers. _\"What we didn't realize was that those engineers would_ **_always_** _be needed. Every schema change, data rebalancing operation, or scaling decision required their specialized expertise. We were essentially committing to a permanent 'sharding team' just to keep our database running.\"_\n\nReal-world challenges with sharded vector databases include:\n\n1. **Data Distribution Imbalance (Hotspots)**: In multi-tenant use cases, data distribution can range from hundreds to billions of vectors per tenant. This imbalance creates hotspots where certain shards become overloaded while others sit idle.\n\n2. **The Resharding Headache**: Choosing the right number of shards is nearly impossible. Too few leads to frequent and costly resharding operations. Too many creates unnecessary metadata overhead, increasing complexity and reducing performance.\n\n3. **Schema Change Complexity**: Many vector databases implement sharding by managing multiple underlying databases. This makes synchronizing schema changes across shards cumbersome and error-prone, slowing development cycles.\n\n4. **Resource Waste**: In storage-compute coupled databases, you must meticulously allocate resources across every node while anticipating future growth. Typically, when resource utilization reaches 60-70%, you need to start planning for resharding.\n\nSimply put, **managing shards manually is bad for your business**. Instead of locking your engineering team into constant shard management, consider investing in a vector database designed to scale automatically—without the operational burden.\n\n\n## How Milvus Solves the Scalability Problem\n\nMany developers—from startups to enterprises—have recognized the significant overhead associated with manual database sharding. Milvus takes a fundamentally different approach, enabling seamless scaling from millions to billions of vectors without the complexity.\n\n\n### Automated Scaling Without the Tech Debt\n\nMilvus leverages Kubernetes and a disaggregated storage-compute architecture to support seamless expansion. This design enables:\n\n- Rapid scaling in response to changing demands\n\n- Automatic load balancing across all available nodes\n\n- Independent resource allocation, letting you adjust compute, memory, and storage separately\n\n- Consistent high performance, even during periods of rapid growth\n\n\n### Distributed Architecture Designed from the Ground Up\n\nMilvus achieves its scaling capabilities through two key innovations:\n\n**Segment-Based Architecture:** At its core, Milvus organizes data into \"segments\"—the smallest units of data management:\n\n- Growing Segments reside on StreamNodes, optimizing data freshness for real-time queries\n\n- Sealed Segments are managed by QueryNodes, utilizing powerful indexes to accelerate search\n\n- These segments are evenly distributed across nodes to optimize parallel processing\n\n**Two-Layer Routing**: Unlike traditional databases where each shard lives on a single machine, Milvus distributes data in one shard dynamically across multiple nodes:\n\n- Each shard can store over 1 billion data points\n\n- Segments within each shard are automatically balanced across machines\n\n- Expanding collections is as simple as increasing the number of shards\n\n- The upcoming Milvus 3.0 will introduce dynamic shard splitting, eliminating even this minimal manual step\n\n\n### Query Processing at Scale\n\nWhen executing a query, Milvus follows an efficient process:\n\n1. The Proxy identifies relevant shards for the requested collection\n\n2. The Proxy gathers data from both StreamNodes and QueryNodes\n\n3. StreamNodes handle real-time data while QueryNodes process historical data concurrently\n\n4. Results are aggregated and returned to the user\n\n\n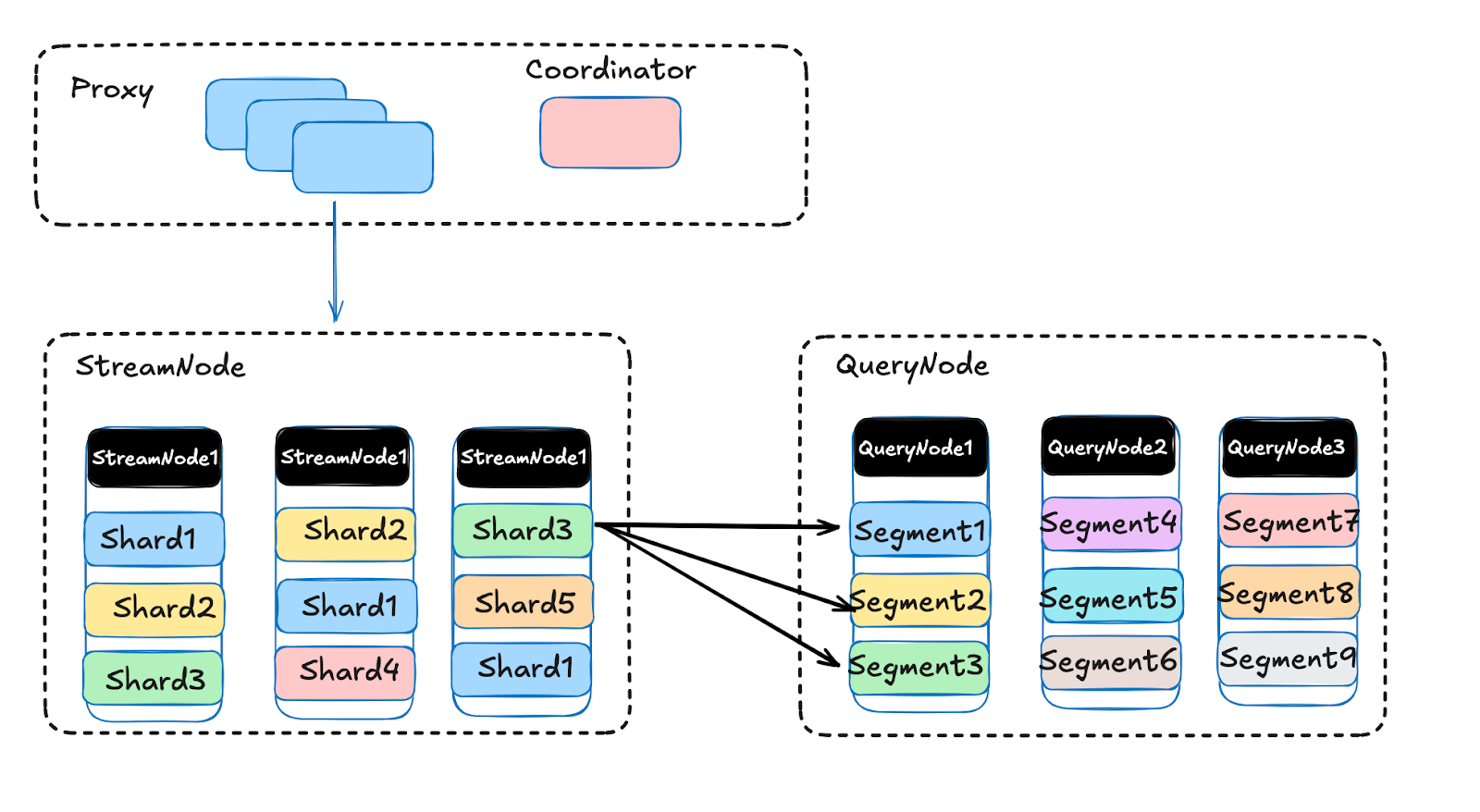\n\n## A Different Engineering Experience\n\n_\"When scalability is built into the database itself, all those headaches just... disappear,\"_ says Alex, reflecting on his team's transition to Milvus. _\"My engineers are back to building features customers love instead of babysitting database shards.\"_\n\nIf you're grappling with the engineering burden of manual sharding, performance bottlenecks at scale, or the daunting prospect of database migrations, it's time to rethink your approach. Visit our [docs page](https://milvus.io/docs/overview.md#What-Makes-Milvus-so-Scalable) to learn more about Milvus architecture, or experience effortless scalability firsthand with fully-managed Milvus at [zilliz.com/cloud](https://zilliz.com/cloud).\n\nWith the right vector database foundation, your innovation knows no limits.\n","title":"Why Manual Sharding is a Bad Idea for Vector Database And How to Fix It","metaData":{}},{"id":"a-day-in-the-life-of-milvus-datum.md","author":"Stefan Webb, Anthony Tu","desc":"So, let’s take a stroll in a day in the life of Dave, the Milvus datum.","tags":["Engineering"],"recommend":true,"canonicalUrl":"https://milvus.io/blog/a-day-in-the-life-of-milvus-datum.md","date":"2025-03-17T00:00:00.000Z","cover":"https://assets.zilliz.com/a_day_in_the_life_of_a_milvus_datum_ca279f7f59.png","href":"/blog/a-day-in-the-life-of-milvus-datum.md","content":"\n\nBuilding a performant [vector database](https://zilliz.com/learn/what-is-vector-database) like Milvus that scales to billions of vectors and handles web-scale traffic is no simple feat. It requires the careful, intelligent design of a distributed system. Necessarily, there will be a trade-off between performance vs simplicity in the internals of a system like this.\n\nWhile we have tried to well balance this trade-off, some aspects of the internals have remained opaque. This article aims to dispel any mystery around how Milvus breaks down data insertion, indexing, and serving across nodes. Understanding these processes at a high level is essential for effectively optimizing query performance, system stability, and debugging-related issues.\n\nSo, let’s take a stroll in a day in the life of Dave, the Milvus datum. Imagine you insert Dave into your collection in a [Milvus Distributed deployment](https://milvus.io/docs/install-overview.md#Milvus-Distributed) (see the diagram below). As far as you are concerned, he goes directly into the collection. Behind the scenes, however, many steps occur across independent sub-systems.\n\n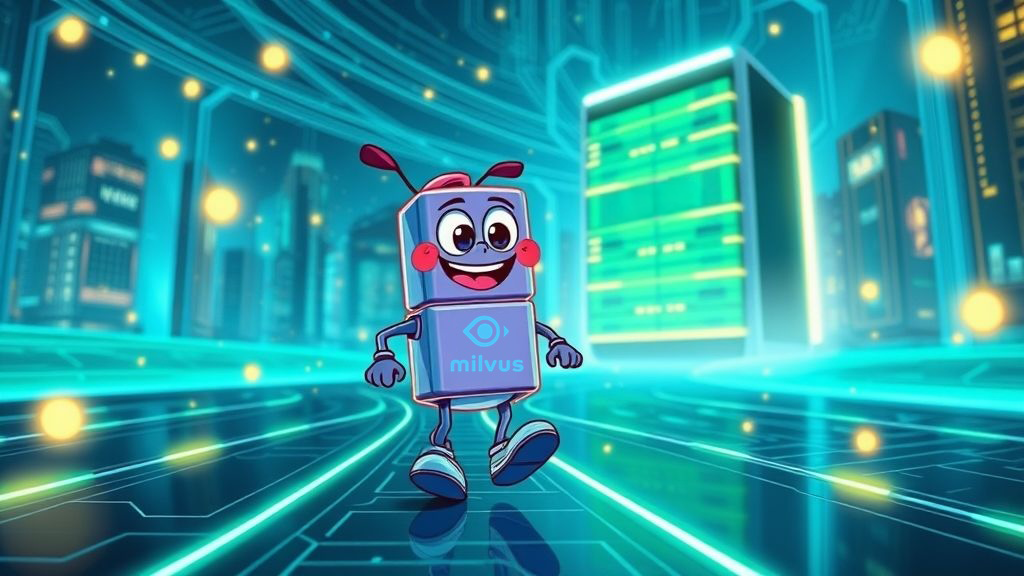\n\n\n## Proxy Nodes and the Message Queue\n\n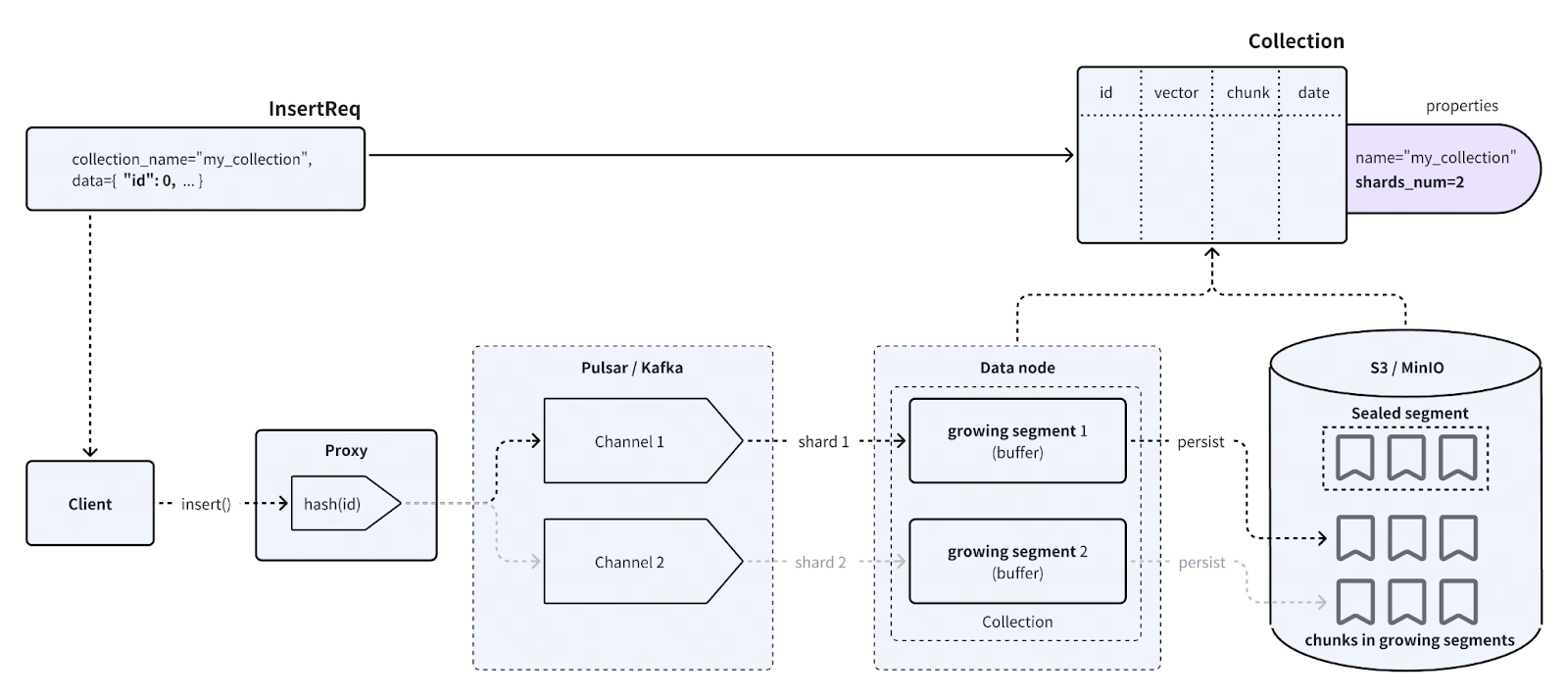\n\nInitially, you call the MilvusClient object, for example, via the PyMilvus library, and send an `_insert()`_ request to a _proxy node_. Proxy nodes are the gateway between the user and the database system, performing operations like load balancing on incoming traffic and collating multiple outputs before they are returned to the user.\n\nA hash function is applied to the item’s primary key to determine which _channel_ to send it to. Channels, implemented with either Pulsar or Kafka topics, represent a holding ground for streaming data, which can then be sent onwards to subscribers of the channel.\n\n\n## Data Nodes, Segments, and Chunks\n\n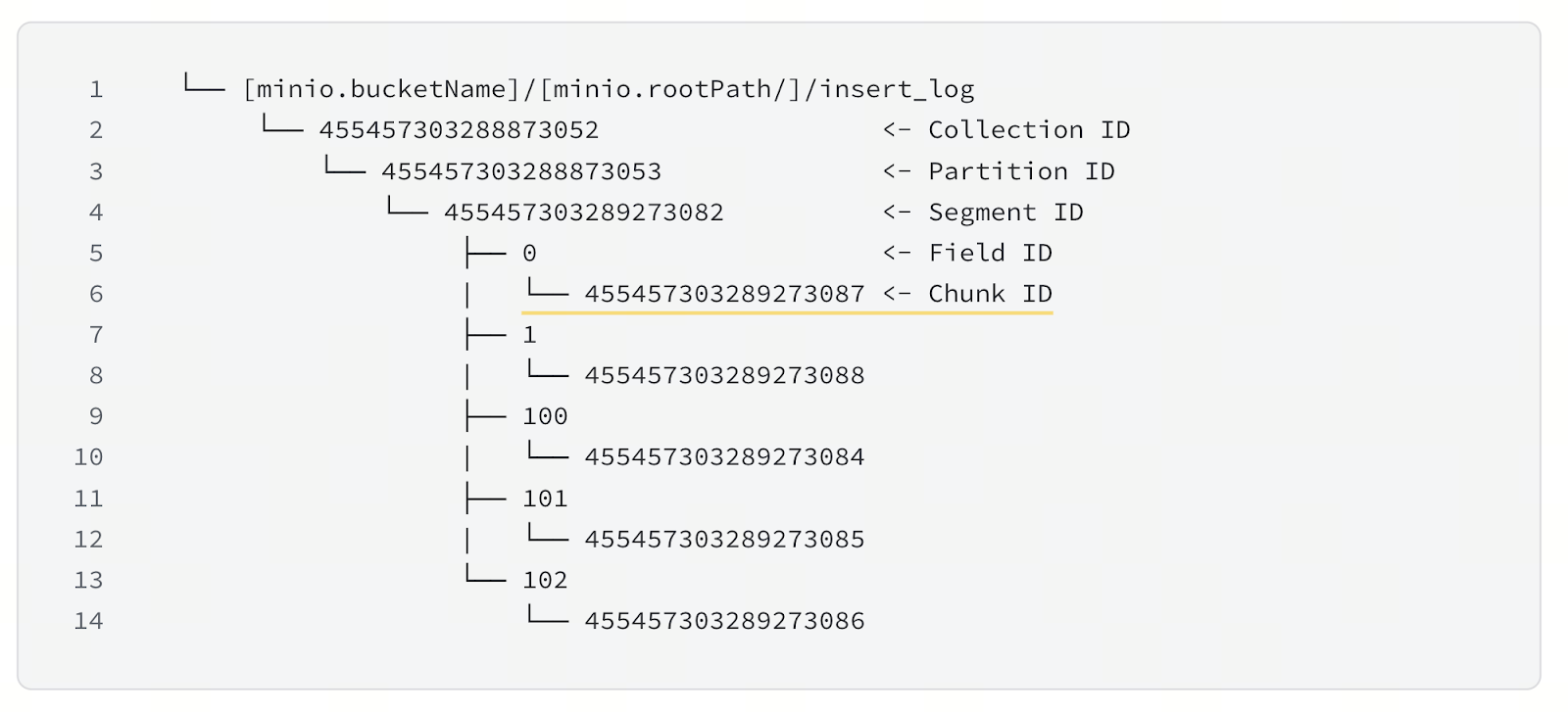\n\nAfter the data has been sent to the appropriate channel, the channel then sends it to the corresponding segment in the _data node_. Data nodes are responsible for storing and managing data buffers called _growing segments_. There is one growing segment per shard.\n\nAs data is inserted into a segment, the segment grows towards a maximum size, defaulting to 122MB. During this time, smaller parts of the segment, by default 16MB and known as _chunks_, are pushed to persistent storage, for example, using AWS’s S3 or other compatible storage like MinIO. Each chunk is a physical file on the object storage and there is a separate file per field. See the figure above illustrating the file hierarchy on object storage.\n\nSo to summarize, a collection’s data is split across data nodes, within which it is split into segments for buffering, which are further split into per-field chunks for persistent storage. The two diagrams above make this clearer. By dividing the incoming data in this way, we fully exploit the cluster’s parallelism of network bandwidth, compute, and storage.\n\n\n## Sealing, Merging, and Compacting Segments\n\n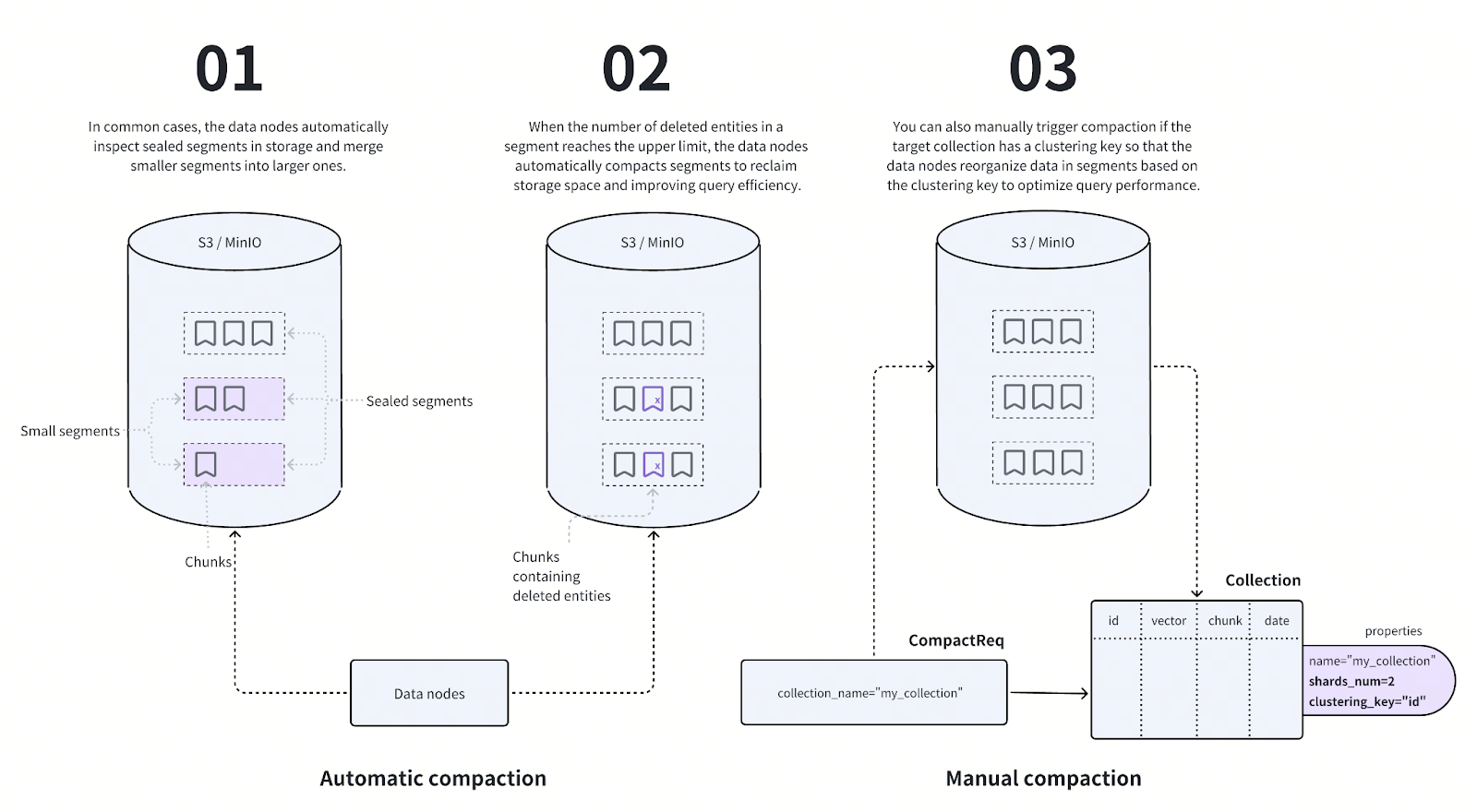\n\nThus far we have told the story of how our friendly datum Dave makes his way from an `_insert()`_ query into persistent storage. Of course, his story does not end there. There are further steps to make the search and indexing process more efficient. By managing the size and number of segments, the system fully exploits the cluster’s parallelism.\n\nOnce a segment reaches its maximum size on a data node, by default 122MB, it is said to be _sealed_. What this means is that the buffer on the data node is cleared to make way for a new segment, and the corresponding chunks in persistent storage are marked as belonging to a closed segment.\n\nThe data nodes periodically look for smaller sealed segments and merge them into larger ones until they have reached a maximum size of 1GB (by default) per segment. Recall that when an item is deleted in Milvus, it is simply marked with a deletion flag - think of it as Death Row for Dave. When the number of deleted items in a segment passes a given threshold, by default 20%, the segment is reduced in size, an operation we call _compaction_.\n\n\nIndexing and Searching through Segments\n\n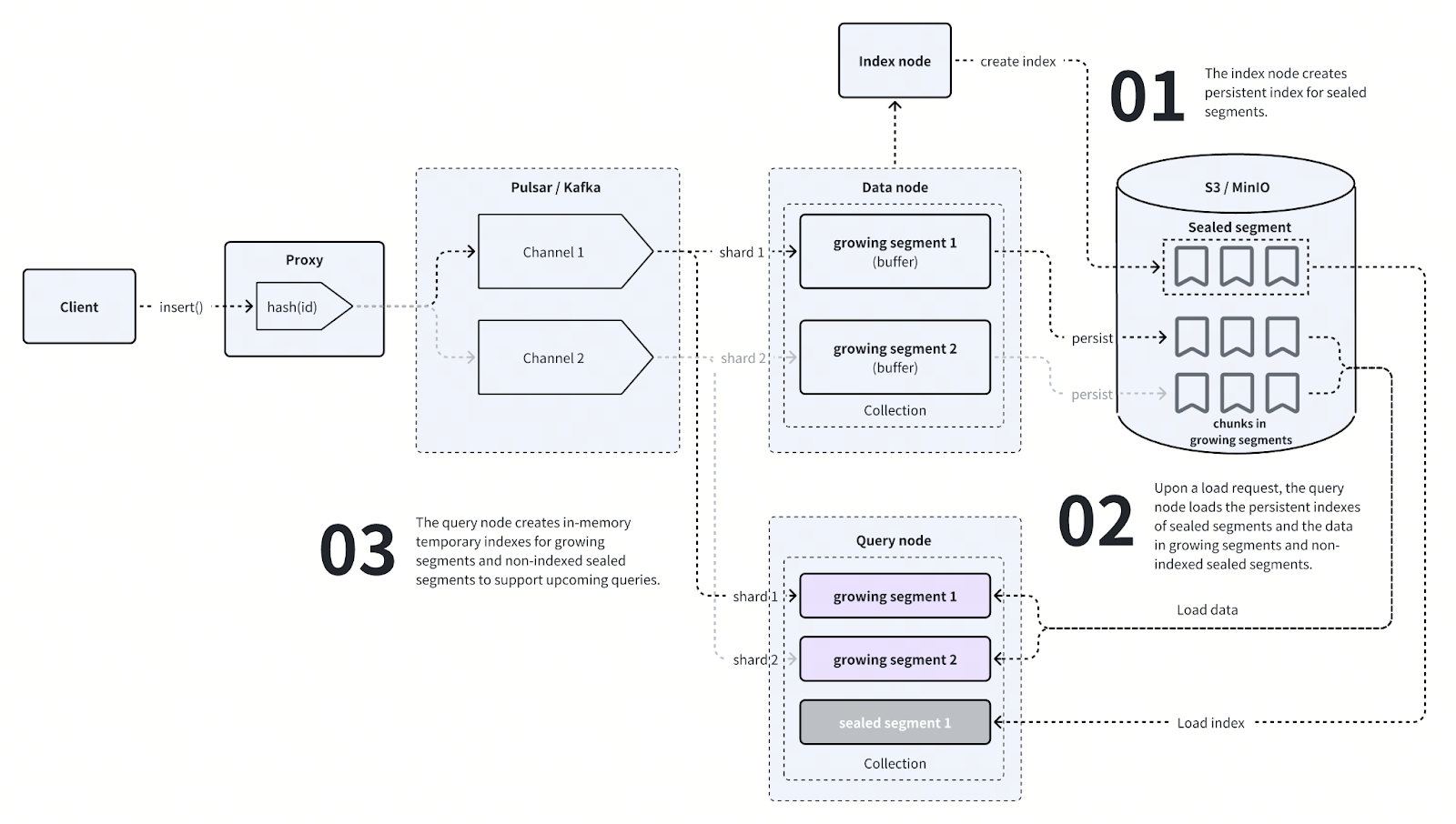\n\n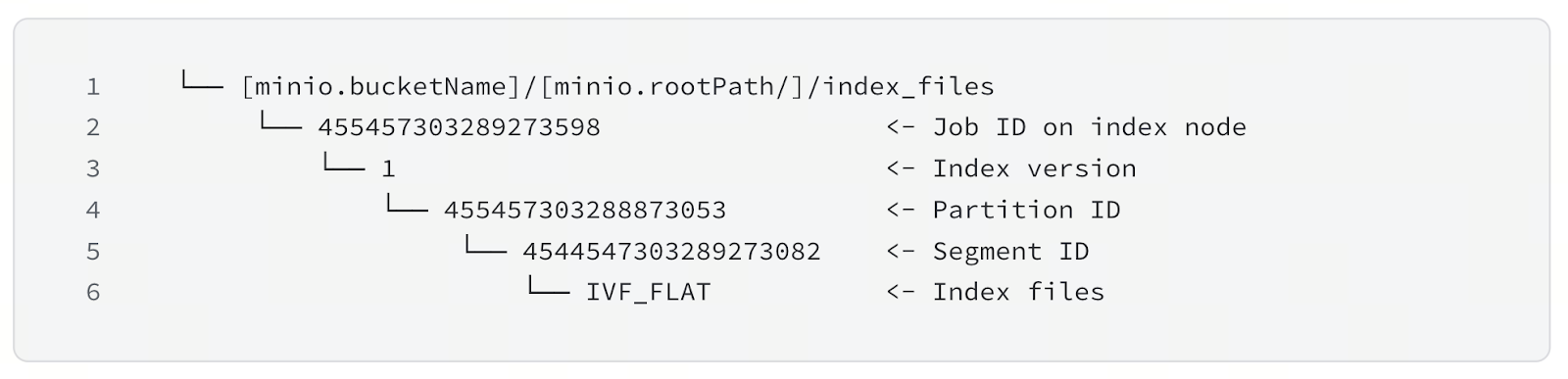\n\nThere is an additional node type, the _index node_, that is responsible for building indexes for sealed segments. When the segment is sealed, the data node sends a request for an index node to construct an index. The index node then sends the completed index to object storage. Each sealed segment has its own index stored in a separate file. You can examine this file manually by accessing the bucket - see the figure above for the file hierarchy.\n\nQuery nodes - not only data nodes - subscribe to the message queue topics for the corresponding shards. The growing segments are replicated on the query nodes, and the node loads into memory sealed segments belonging to the collection as required. It builds an index for each growing segment as data comes in, and loads the finalized indexes for sealed segments from the data store.\n\nImagine now that you call the MilvusClient object with a _search()_ request that encompasses Dave. After being routed to all query nodes via the proxy node, each query node performs a vector similarity search (or another one of the search methods like query, range search, or grouping search), iterating over the segments one by one. The results are collated across nodes in a MapReduce-like fashion and sent back to the user, Dave being happy to find himself reunited with you at last.\n\n\n## Discussion\n\nWe have covered a day in the life of Dave the datum, both for `_insert()`_ and `_search()`_ operations. Other operations like `_delete()`_ and `_upsert()`_ work similarly. Inevitably, we have had to simplify our discussion and omit finer details. On the whole, though, you should now have a sufficient picture of how Milvus is designed for parallelism across nodes in a distributed system to be robust and efficient, and how you can use this for optimization and debugging. \n\n_An important takeaway from this article: Milvus is designed with a separation of concerns across node types. Each node type has a specific, mutually exclusive function, and there is a separation of storage and compute._ The result is that each component can be scaled independently with parameters tweakable according to the use case and traffic patterns. For example, you can scale the number of query nodes to serve increased traffic without scaling data and index nodes. With that flexibility, there are Milvus users that handle billions of vectors and serve web-scale traffic, with sub-100ms query latency.\n\nYou can also enjoy the benefits of Milvus’ distributed design without even deploying a distributed cluster through [Zilliz Cloud](https://zilliz.com/cloud), a fully managed service of Milvus. [Sign up today for the free-tier of Zilliz Cloud and put Dave into action!](https://cloud.zilliz.com/signup)\n","title":"A Day in the Life of a Milvus Datum","metaData":{}},{"id":"ai-for-smarter-browsing-filtering-web-content-with-pirxtral-milvus-browser-use.md","author":"Stephen Batifol","desc":"Learn how to build an intelligent assistant that filters content by combining Pixtral for image analysis, Milvus vector database for storage, and Browser Use for web navigation.","tags":["Engineering"],"recommend":true,"canonicalUrl":"https://milvus.io/blog/ai-for-smarter-browsing-filtering-web-content-with-pixtral-milvus-browser-use.md","date":"2025-02-25T00:00:00.000Z","cover":"https://assets.zilliz.com/AI_for_Smarter_Browsing_Filtering_Web_Content_with_Pixtral_Milvus_and_Browser_Use_56d0154bbd.png","href":"/blog/ai-for-smarter-browsing-filtering-web-content-with-pirxtral-milvus-browser-use.md","content":"\n\u003ciframe width=\"100%\" height=\"480\" src=\"https://www.youtube.com/embed/4Xf4_Wfjk_Y\" title=\"How to Build a Smart Social Media Agent with Milvus, Pixtral \u0026amp; Browser Use\" frameborder=\"0\" allow=\"accelerometer; autoplay; clipboard-write; encrypted-media; gyroscope; picture-in-picture; web-share\" referrerpolicy=\"strict-origin-when-cross-origin\" allowfullscreen\u003e\u003c/iframe\u003e\n\nAs a Developer Advocate for Milvus, I spend a lot of time on Socials, listening to what people have to say about us and if I can help as well. There is a slight clash of worlds though when you look for \"Milvus\". It is both a Vector DB and genus of bird, meaning that one moment I'm deep in a thread about vector similarity algorithms, the next I'm admiring stunning photographs of black birds flying through the sky.\n\nWhile both topics are interesting, mixing them up isn't really helpful in my case, what if there was a smart way to solve this problem without me having to check manually?\n\nLet's build something smarter - by combining visual understanding with context awareness, we can build an assistant that knows the difference between a black kite's migration patterns and a new article from us.\n\n\n## The tech stack\n\nWe combine three different technologies:\n\n- **Browser-Use:** This tool navigates various websites (e.g., Twitter) to fetch content.\n- **Pixtral**: A vision-language model that analyzes images and context. In this example, it distinguishes between a technical diagram about our Vector DB and a stunning bird photograph.\n- **Milvus:** A high performance and open-source Vector DB. His is where we will store the relevant posts for later querying.\n\n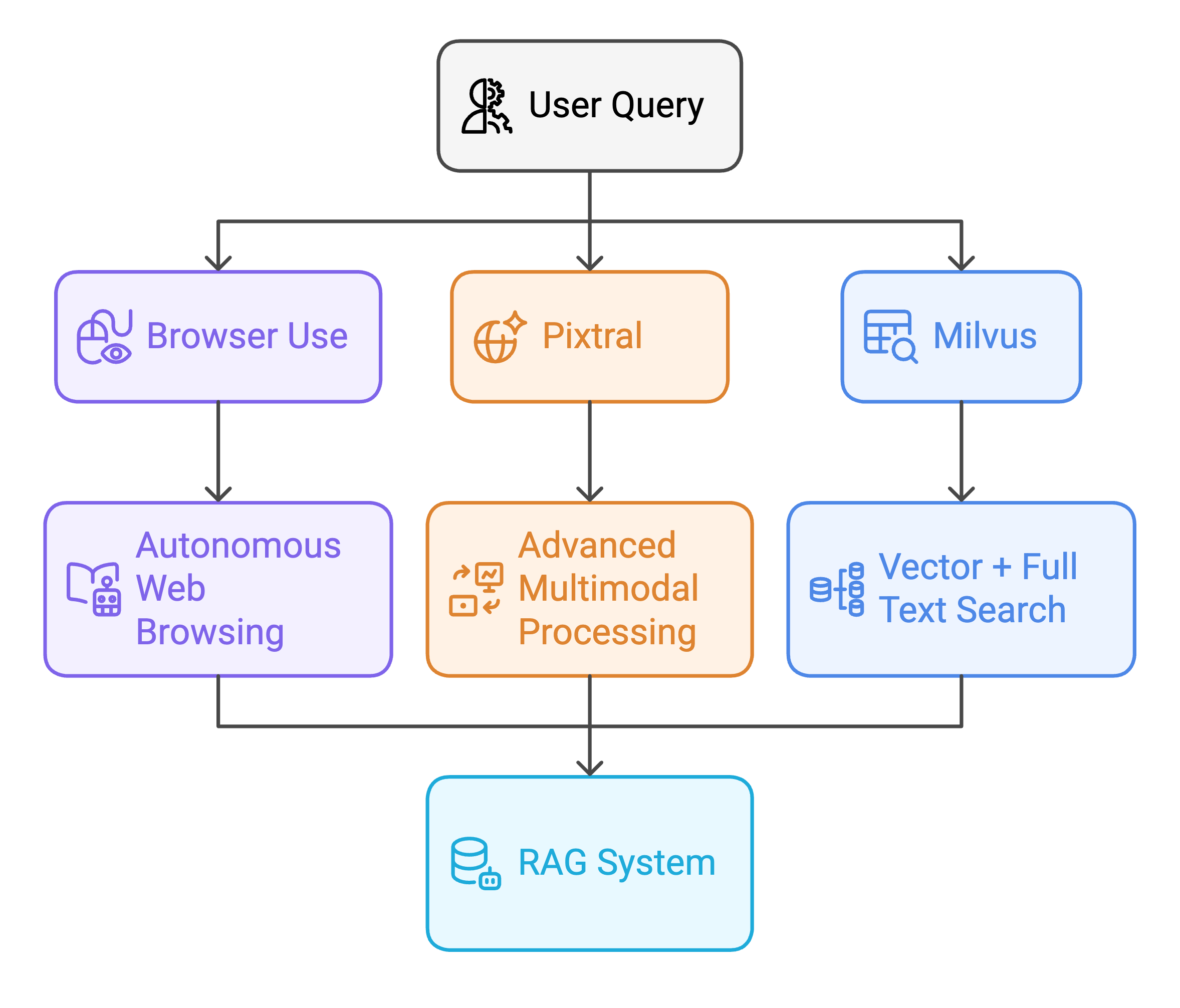\n\n\n## Seeing it in action\n\nLet's have a look at those 2 posts:\n\n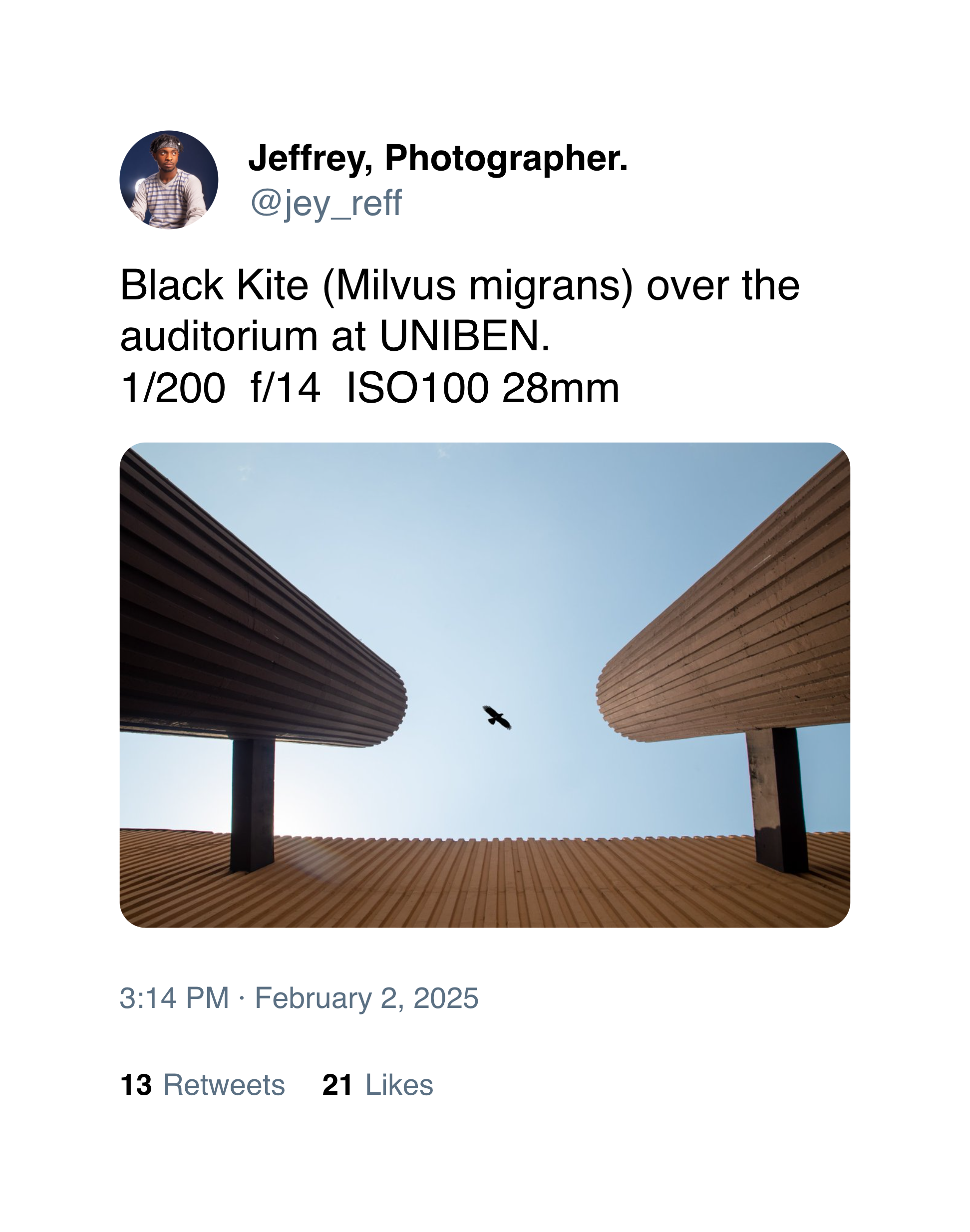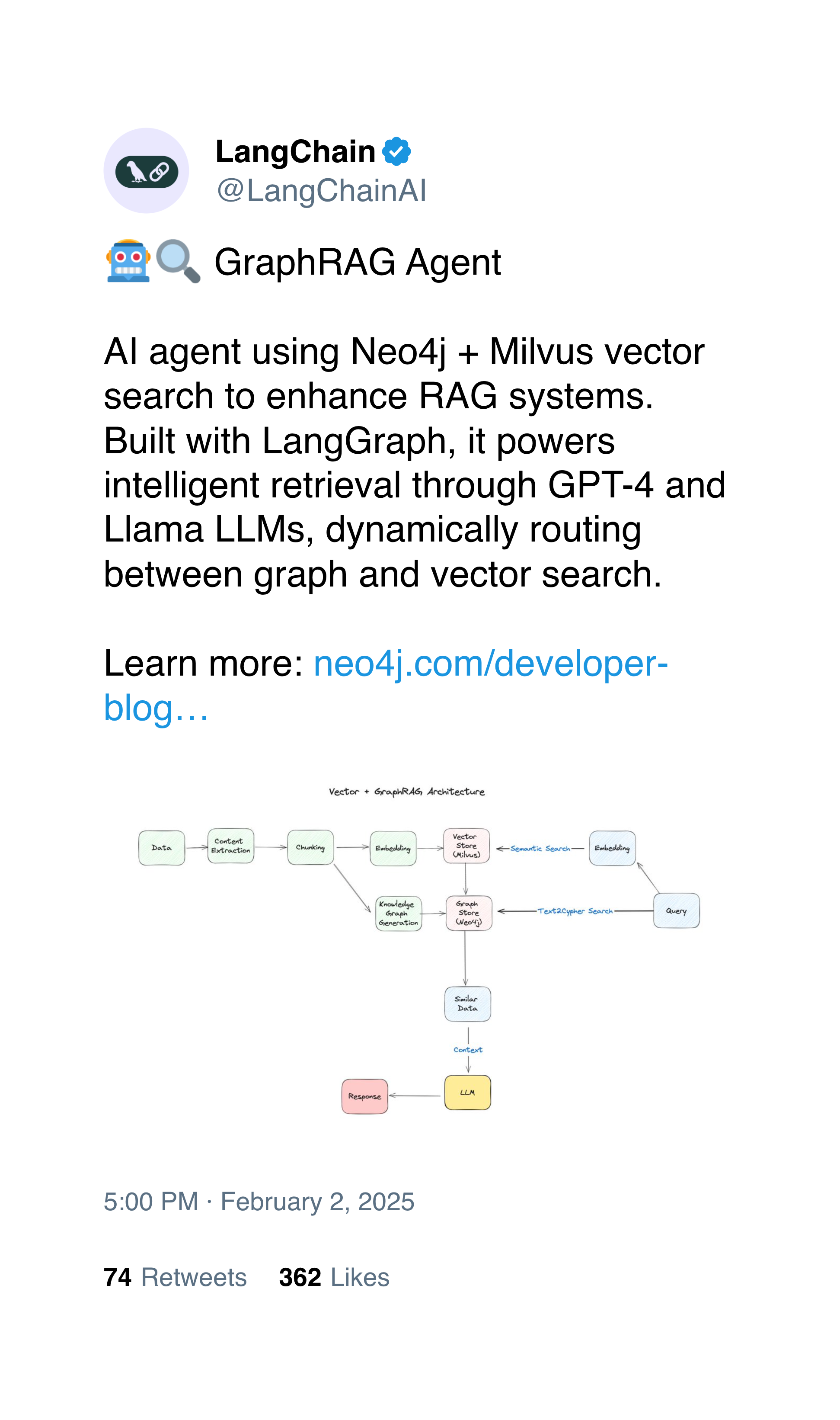\n\nFor the one on the left, Pixtral recognizes that this is a post about Milvus, the Vector DB. It mentioned some implementation details, but also an image showing a system diagram, indicating that this is indeed, about the Vector DB. We can see below that Pixtral also thinks the same. \n\n```Shell\nINFO [src.agent.custom_agent] 🧠 New Memory: 1. The post by LangChainAI discusses the 'GraphRAG Agent' which uses Neo4j and Milvus vector search to enhance RAG systems.\n2. Replies include praise for the use of Milvus in intelligent retrieval and vector search.\n3. Specific mention of Milvus as a key component in innovative RAG systems.\n4. No content related to birds or photography was found.\nINFO [src.agent.custom_agent] ⏳ Task Progress:\n1. Navigated to the post URL. 2. Extracted relevant post content. 3. Analyzed the content for relevance to Milvus, the Vector DB.\nINFO [src.agent.custom_agent] 📋 Future Plans:\n1. Summarize the findings and confirm the relevance to Milvus. 2. Complete the task by providing a clear and concise report.\nINFO [src.agent.custom_agent] 🤔 Thought: The task required analyzing the post content for relevance to Milvus, the Vector DB, ignoring any content about birds or photography. The extracted content is entirely relevant to Milvus and its applications in vector search and RAG systems. I will now summarize the findings and complete the task.\nINFO [src.agent.custom_agent] 🎯 Summary: The post and its replies are relevant to Milvus, the Vector DB, focusing on its use in intelligent retrieval and vector search. No content about birds or photography was found.\nINFO [src.agent.custom_agent] 🛠️ Action 1/1: {\"done\":{\"text\":\"The post by LangChainAI discusses the 'GraphRAG Agent,' which uses Neo4j and Milvus vector search to enhance RAG systems. Replies highlight the innovative use of Milvus for intelligent retrieval and vector search, with no mention of birds or photography. The content is entirely relevant to Milvus, the Vector DB.\"}}\nINFO [src.agent.custom_agent] 🧠 All Memory:\n1. The post by LangChainAI discusses the 'GraphRAG Agent' which uses Neo4j and Milvus vector search to enhance RAG systems.\n2. Replies focus on the innovative use of Milvus for intelligent retrieval and vector search alongside other technologies.\n3. No posts or replies are related to birds or photography.\n1. The post by LangChainAI discusses the 'GraphRAG Agent' which uses Neo4j and Milvus vector search to enhance RAG systems.\n2. Replies include praise for the use of Milvus in intelligent retrieval and vector search.\n3. Specific mention of Milvus as a key component in innovative RAG systems.\n4. No content related to birds or photography was found.\n```\n\nThe one on the right on the other hand isn't, we can see that this picture, as beautiful as it is, isn't about a Vector DB. We can see a bird flying in the sky, therefore, Pixtral will consider this one irrelevant.\n\n```Shell\nINFO [src.agent.custom_agent] 🧠 New Memory: The post and comments primarily discuss photography and birds. No references to Milvus the Vector Database are found.\nINFO [src.agent.custom_agent] ⏳ Task Progress:\nNavigated to the post. Analyzed the content for relevance to Milvus, the Vector DB. No relevant information found.\nINFO [src.agent.custom_agent] 🤔 Thought: The content of the post and comments only discusses birds and photography. Since the task specifies ignoring such topics, there is no relevant information for Milvus, the Vector DB. The task can now be concluded.\nINFO [src.agent.custom_agent] 🎯 Summary: The post does not contain relevant information for Milvus, the Vector DB. I will conclude the task.\nINFO [src.agent.custom_agent] 🛠️ Action 1/1: {\"done\":{\"text\":\"The post and comments focus on birds and photography. No relevant information related to Milvus, the Vector DB, was found.\"}}\nINFO [src.agent.custom_agent] 🧠 All Memory:\nThe post and comments primarily discuss photography and birds. No references to Milvus the Vector Database are found.\n```\n\nNow that we have filtered out the posts we don't want, we can save the relevant ones in Milvus. Making it possible to query them later using either Vector Search or Full Text Search.\n\n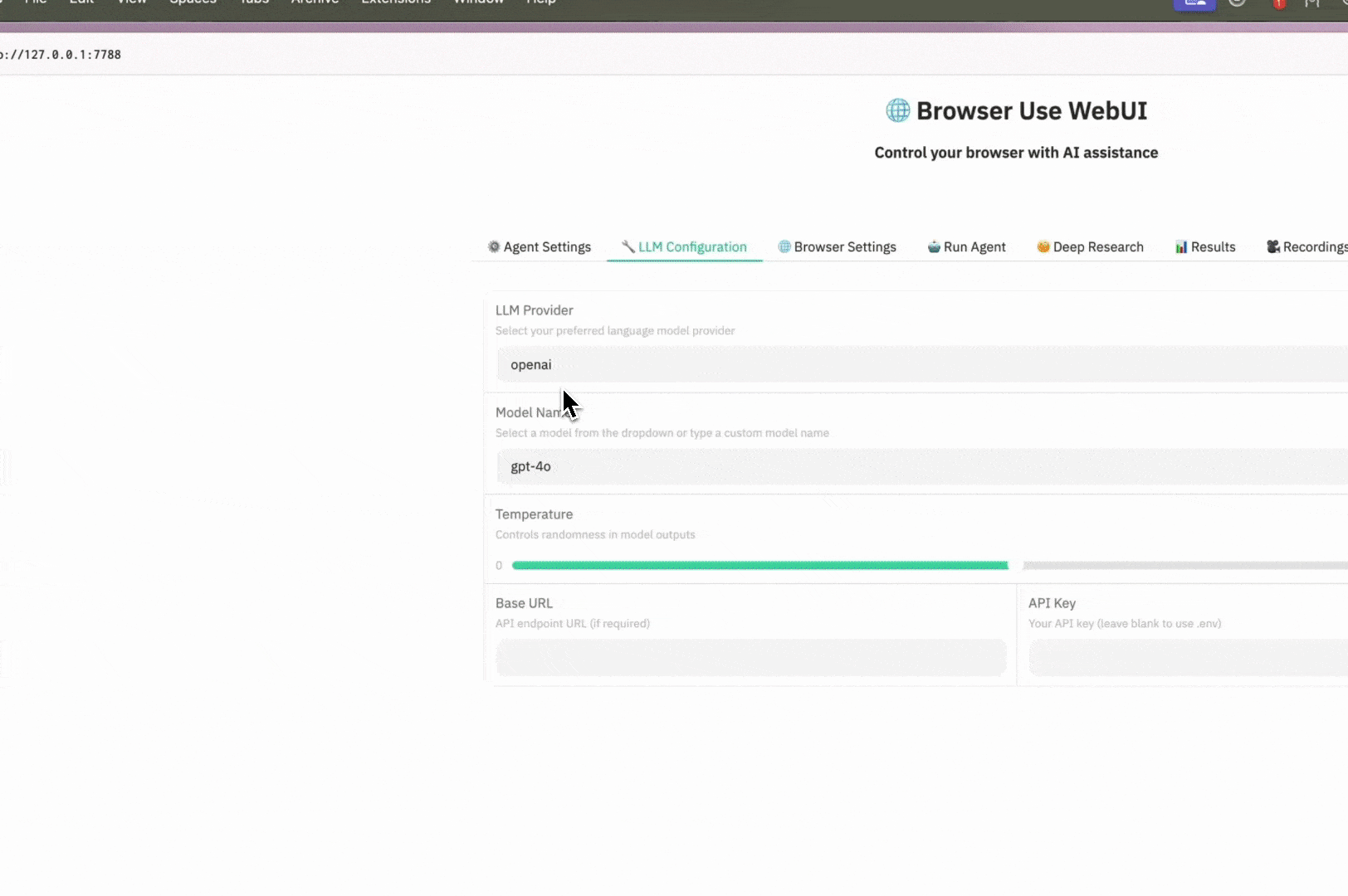\n\n\n## Storing Data in Milvus\n\n[Dynamic Fields](https://milvus.io/docs/enable-dynamic-field.md#Dynamic-Field) are a must in this case because it's not always possible to respect the schema that Milvus expects. With Milvus, you just use `enable_dynamic_field=True` when creating your schema, and that's it. Here is a code snippet to showcase the process:\n\n```Python\nfrom pymilvus import MilvusClient\n\n# Connect to Milvus\nclient = MilvusClient(uri=\"http://localhost:19530\")\n\n# Create a Schema that handles Dynamic Fields\nschema = self.client.create_schema(enable_dynamic_field=True)\nschema.add_field(field_name=\"id\", datatype=DataType.INT64, is_primary=True, auto_id=True)\nschema.add_field(field_name=\"text\", datatype=DataType.VARCHAR, max_length=65535, enable_analyzer=True)\nschema.add_field(field_name=\"vector\", datatype=DataType.FLOAT_VECTOR, dim=384)\nschema.add_field(field_name=\"sparse\", datatype=DataType.SPARSE_FLOAT_VECTOR)\n```\n\nThen we define the data we want to have access to:\n\n```Python\n# Prepare data with dynamic fields\ndata = {\n 'text': content_str,\n 'vector': embedding,\n 'url': url,\n 'type': content_type,\n 'metadata': json.dumps(metadata or {})\n}\n\n# Insert into Milvus\nself.client.insert(\n collection_name=self.collection_name,\n data=[data]\n)\n```\n\nThis simple setup means you don’t have to worry about every field being perfectly defined upfront. Just set up the schema to allow for dynamic additions and let Milvus do the heavy lifting.\n\n\n## Conclusion\n\nBy combining Browser Use's web navigation, Pixtral's visual understanding, and Milvus's efficient storage, we've built an intelligent assistant that truly understands context. Now I am using it to distinguish between birds and vector DB, but the same approach could help with another problem you may be facing.\n\nOn my end, I wanna continue working on agents that can help me in my daily work in order to reduce my cognitive load 😌\n\n\n## We'd Love to Hear What You Think!\n\nIf you like this blog post, please consider:\n\n- ⭐ Giving us a star on [GitHub](https://github.com/milvus-io/milvus)\n- 💬 Joining our [Milvus Discord community](https://discord.gg/FG6hMJStWu) to share your experiences or if you need help building Agents\n- 🔍 Exploring our [Bootcamp repository](https://github.com/milvus-io/bootcamp) for examples of applications using Milvus\n\n","title":"AI for Smarter Browsing: Filtering Web Content with Pixtral, Milvus, and Browser Use","metaData":{}},{"id":"introduce-deepsearcher-a-local-open-source-deep-research.md","author":"Stefan Webb","desc":"In contrast to OpenAI’s Deep Research, this example ran locally, using only open-source models and tools like Milvus and LangChain.","tags":["Announcements"],"recommend":true,"canonicalUrl":"https://zilliz.com/blog/introduce-deepsearcher-a-local-open-source-deep-research","date":"2025-02-21T00:00:00.000Z","cover":"https://assets.zilliz.com/Introducing_Deep_Searcher_A_Local_Open_Source_Deep_Research_4d00da5b85.png","href":"/blog/introduce-deepsearcher-a-local-open-source-deep-research.md","content":"\n\n\n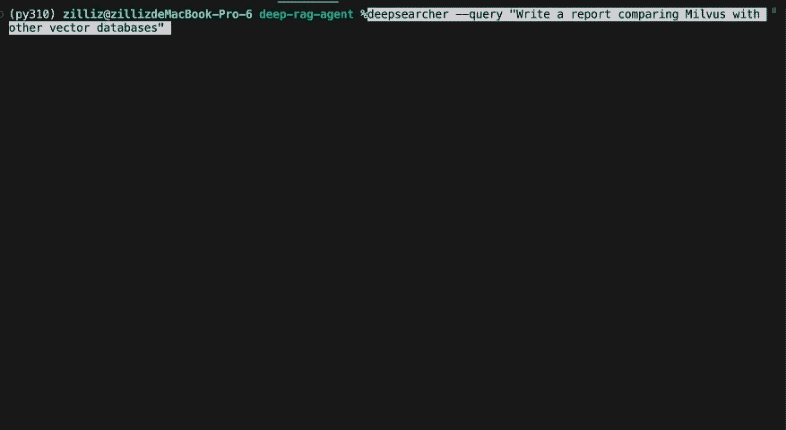\n\nIn the previous post, [_“I Built a Deep Research with Open Source—and So Can You!”_](https://milvus.io/blog/i-built-a-deep-research-with-open-source-so-can-you.md), we explained some of the principles underlying research agents and constructed a simple prototype that generates detailed reports on a given topic or question. The article and corresponding notebook demonstrated the fundamental concepts of _tool use_, _query decomposition_, _reasoning_, and _reflection_. The example in our previous post, in contrast to OpenAI’s Deep Research, ran locally, using only open-source models and tools like [Milvus](https://milvus.io/docs) and LangChain. (I encourage you to read the [above article](https://milvus.io/blog/i-built-a-deep-research-with-open-source-so-can-you.md) before continuing.) \n\nIn the following weeks, there was an explosion of interest in understanding and reproducing OpenAI’s Deep Research. See, for example, [Perplexity Deep Research](https://www.perplexity.ai/hub/blog/introducing-perplexity-deep-research) and [Hugging Face's Open DeepResearch](https://huggingface.co/blog/open-deep-research). These tools differ in architecture and methodology although sharing an objective: iteratively research a topic or question by surfing the web or internal documents and output a detailed, informed, and well-structured report. Importantly, the underlying agent automates reasoning about what action to take at each intermediate step.\n\nIn this post, we build upon our previous post and present Zilliz’s [DeepSearcher](https://github.com/zilliztech/deep-searcher) open-source project. Our agent demonstrates additional concepts: _query routing, conditional execution flow_, and _web crawling as a tool_. It is presented as a Python library and command-line tool rather than a Jupyter notebook and is more fully-featured than our previous post. For example, it can input multiple source documents and can set the embedding model and vector database used via a configuration file. While still relatively simple, DeepSearcher is a great showcase of agentic RAG and is a further step towards a state-of-the-art AI applications.\n\nAdditionally, we explore the need for faster and more efficient inference services. Reasoning models make use of “inference scaling”, that is, extra computation, to improve their output, and that combined with the fact that a single report may require hundreds or thousands of LLM calls results in inference bandwidth being the primary bottleneck. We use the [DeepSeek-R1 reasoning model on SambaNova’s custom-built hardware](https://sambanova.ai/press/fastest-deepseek-r1-671b-with-highest-efficiency), which is twice as fast in output tokens-per-second as the nearest competitor (see figure below).\n\nSambaNova Cloud also provides inference-as-a-service for other open-source models including Llama 3.x, Qwen2.5, and QwQ. The inference service runs on SambaNova’s custom chip called the reconfigurable dataflow unit (RDU), which is specially designed for efficient inference on Generative AI models, lowering cost and increasing inference speed. [Find out more on their website.](https://sambanova.ai/technology/sn40l-rdu-ai-chip)\n\n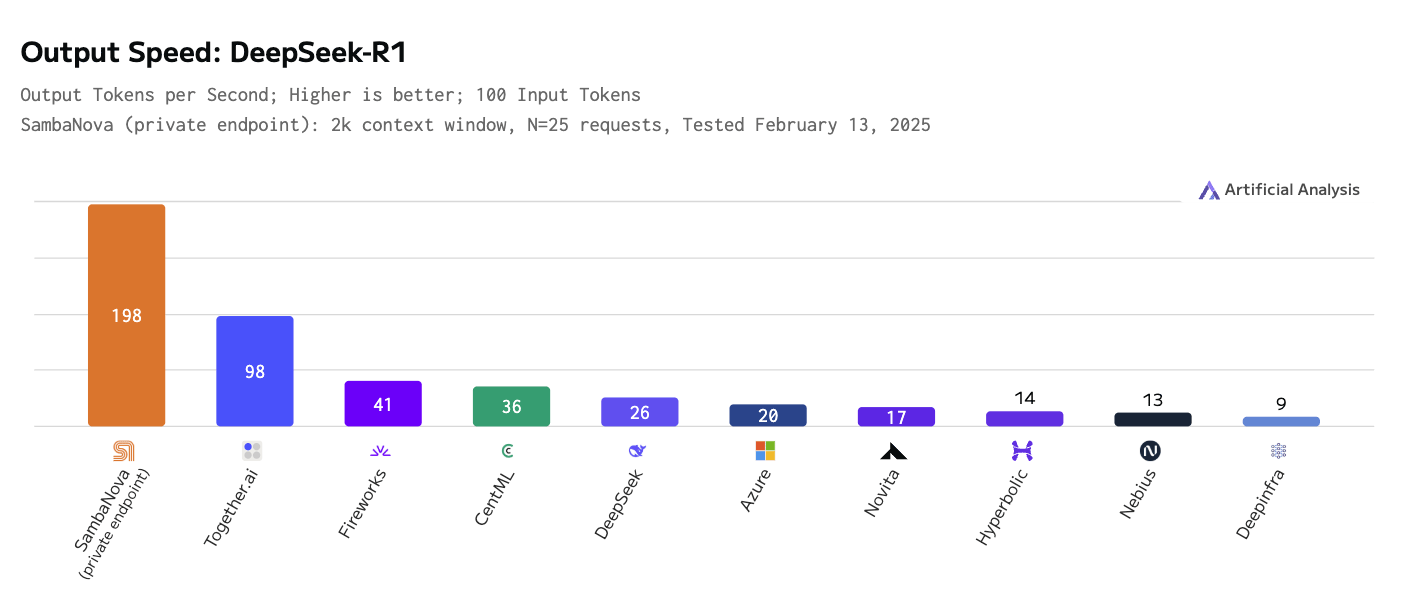\n\n\n## DeepSearcher Architecture\n\n\n\nThe architecture of [DeepSearcher](https://github.com/zilliztech/deep-searcher) follows our previous post by breaking the problem up into four steps - _define/refine the question_, _research_, _analyze_, _synthesize_ - although this time with some overlap. We go through each step, highlighting [DeepSearcher](https://github.com/zilliztech/deep-searcher)’s improvements.\n\n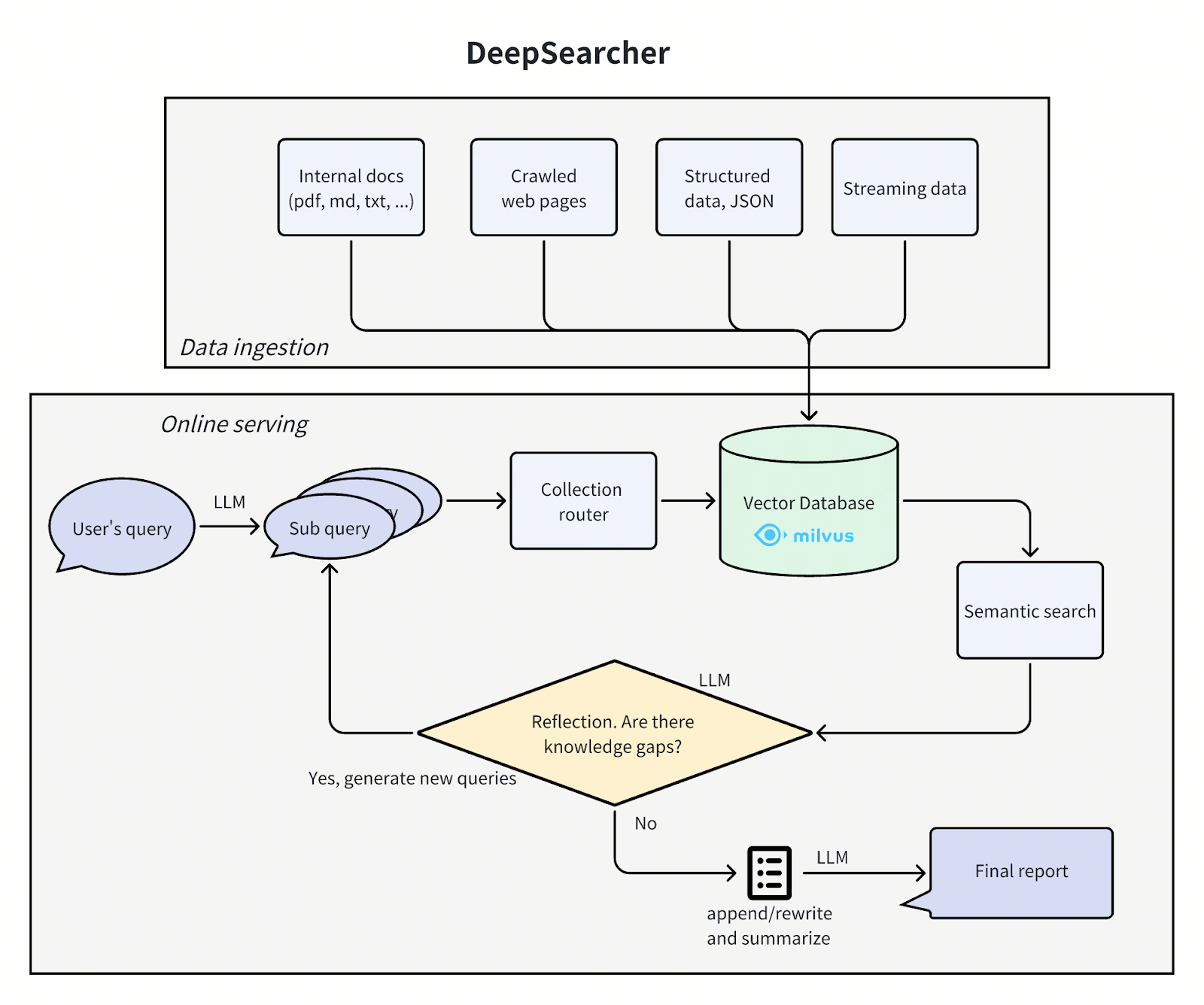\n\n### Define and Refine the Question\n\n```txt\nBreak down the original query into new sub queries: [\n 'How has the cultural impact and societal relevance of The Simpsons evolved from its debut to the present?',\n 'What changes in character development, humor, and storytelling styles have occurred across different seasons of The Simpsons?', \n 'How has the animation style and production technology of The Simpsons changed over time?',\n 'How have audience demographics, reception, and ratings of The Simpsons shifted throughout its run?']\n```\n\n\nIn the design of DeepSearcher, the boundaries between researching and refining the question are blurred. The initial user query is decomposed into sub-queries, much like the previous post. See above for initial subqueries produced from the query “How has The Simpsons changed over time?”. However, the following research step will continue to refine the question as needed.\n\n\n### Research and Analyze\n\nHaving broken down the query into sub-queries, the research portion of the agent begins. It has, roughly speaking, four steps: _routing_, _search_, _reflection, and conditional repeat_.\n\n\n#### Routing\n\nOur database contains multiple tables or collections from different sources. It would be more efficient if we could restrict our semantic search to only those sources that are relevant to the query at hand. A query router prompts an LLM to decide from which collections information should be retrieved.\n\nHere is the method to form the query routing prompt:\n\n```python\ndef get_vector_db_search_prompt(\n question: str,\n collection_names: List[str],\n collection_descriptions: List[str],\n context: List[str] = None,\n):\n sections = []\n # common prompt\n common_prompt = f\"\"\"You are an advanced AI problem analyst. Use your reasoning ability and historical conversation information, based on all the existing data sets, to get absolutely accurate answers to the following questions, and generate a suitable question for each data set according to the data set description that may be related to the question.\n\nQuestion: {question}\n\"\"\"\n sections.append(common_prompt)\n \n # data set prompt\n data_set = []\n for i, collection_name in enumerate(collection_names):\n data_set.append(f\"{collection_name}: {collection_descriptions[i]}\")\n data_set_prompt = f\"\"\"The following is all the data set information. The format of data set information is data set name: data set description.\n\nData Sets And Descriptions:\n\"\"\"\n sections.append(data_set_prompt + \"\\n\".join(data_set))\n \n # context prompt\n if context:\n context_prompt = f\"\"\"The following is a condensed version of the historical conversation. This information needs to be combined in this analysis to generate questions that are closer to the answer. You must not generate the same or similar questions for the same data set, nor can you regenerate questions for data sets that have been determined to be unrelated.\n\nHistorical Conversation:\n\"\"\"\n sections.append(context_prompt + \"\\n\".join(context))\n \n # response prompt\n response_prompt = f\"\"\"Based on the above, you can only select a few datasets from the following dataset list to generate appropriate related questions for the selected datasets in order to solve the above problems. The output format is json, where the key is the name of the dataset and the value is the corresponding generated question.\n\nData Sets:\n\"\"\"\n sections.append(response_prompt + \"\\n\".join(collection_names))\n \n footer = \"\"\"Respond exclusively in valid JSON format matching exact JSON schema.\n\nCritical Requirements:\n- Include ONLY ONE action type\n- Never add unsupported keys\n- Exclude all non-JSON text, markdown, or explanations\n- Maintain strict JSON syntax\"\"\"\n sections.append(footer)\n return \"\\n\\n\".join(sections)\n```\n\n\n\nWe make the LLM return structured output as JSON in order to easily convert its output to a decision on what to do next.\n\n\n#### Search\n\nHaving selected various database collections via the previous step, the search step performs a similarity search with [Milvus](https://milvus.io/docs). Much like the previous post, the source data has been specified in advance, chunked, embedded, and stored in the vector database. For DeepSearcher, the data sources, both local and online, must be manually specified. We leave online search for future work.\n\n\n#### Reflection\n\nUnlike the previous post, DeepSearcher illustrates a true form of agentic reflection, inputting the prior outputs as context into a prompt that “reflects” on whether the questions asked so far and the relevant retrieved chunks contain any informational gaps. This can be seen as an analysis step.\n\nHere is the method to create the prompt:\n\n\n```python\ndef get_reflect_prompt(\n question: str,\n mini_questions: List[str],\n mini_chuncks: List[str],\n):\n mini_chunk_str = \"\"\n for i, chunk in enumerate(mini_chuncks):\n mini_chunk_str += f\"\"\"\u003cchunk_{i}\u003e\\n{chunk}\\n\u003c/chunk_{i}\u003e\\n\"\"\"\n reflect_prompt = f\"\"\"Determine whether additional search queries are needed based on the original query, previous sub queries, and all retrieved document chunks. If further research is required, provide a Python list of up to 3 search queries. If no further research is required, return an empty list.\n\nIf the original query is to write a report, then you prefer to generate some further queries, instead return an empty list.\n\n Original Query: {question}\n Previous Sub Queries: {mini_questions}\n Related Chunks: \n {mini_chunk_str}\n \"\"\"\n \n \n footer = \"\"\"Respond exclusively in valid List of str format without any other text.\"\"\"\n return reflect_prompt + footer\n```\n\n\n\nOnce more, we make the LLM return structured output, this time as Python-interpretable data.\n\nHere is an example of new sub-queries “discovered” by reflection after answering the initial sub-queries above:\n\n```\nNew search queries for next iteration: [\n \"How have changes in The Simpsons' voice cast and production team influenced the show's evolution over different seasons?\",\n \"What role has The Simpsons' satire and social commentary played in its adaptation to contemporary issues across decades?\",\n 'How has The Simpsons addressed and incorporated shifts in media consumption, such as streaming services, into its distribution and content strategies?']\n```\n\n\n\n#### Conditional Repeat\n\nUnlike our previous post, DeepSearcher illustrates conditional execution flow. After reflecting on whether the questions and answers so far are complete, if there are additional questions to be asked the agent repeats the above steps. Importantly, the execution flow (a while loop) is a function of the LLM output rather than being hard-coded. In this case there is only a binary choice: _repeat research_ or _generate a report_. In more complex agents there may be several such as: _follow hyperlink_, _retrieve chunks, store in memory, reflect_ etc. In this way, the question continues to be refined as the agent sees fit until it decides to exit the loop and generate the report. In our Simpsons example, DeepSearcher performs two more rounds of filling the gaps with extra sub-queries.\n\n\n### Synthesize\n\nFinally, the fully decomposed question and retrieved chunks are synthesized into a report with a single prompt. Here is the code to create the prompt:\n\n```python\ndef get_final_answer_prompt(\n question: str, \n mini_questions: List[str],\n mini_chuncks: List[str],\n):\n mini_chunk_str = \"\"\n for i, chunk in enumerate(mini_chuncks):\n mini_chunk_str += f\"\"\"\u003cchunk_{i}\u003e\\n{chunk}\\n\u003c/chunk_{i}\u003e\\n\"\"\"\n summary_prompt = f\"\"\"You are an AI content analysis expert, good at summarizing content. Please summarize a specific and detailed answer or report based on the previous queries and the retrieved document chunks.\n\n Original Query: {question}\n Previous Sub Queries: {mini_questions}\n Related Chunks: \n {mini_chunk_str}\n \"\"\"\n return summary_prompt\n```\n\n\nThis approach has the advantage over our prototype, which analyzed each question separately and simply concatenated the output, of producing a report where all sections are consistent with each other, i.e., containing no repeated or contradictory information. A more complex system could combine aspects of both, using a conditional execution flow to structure the report, summarize, rewrite, reflect and pivot, and so on, which we leave for future work.\n\n\n## Results\n\nHere is a sample from the report generated by the query “How has The Simpsons changed over time?” with DeepSeek-R1 passing the Wikipedia page on The Simpsons as source material:\n\n```txt\nReport: The Evolution of The Simpsons (1989–Present)\n1. Cultural Impact and Societal Relevance\nThe Simpsons debuted as a subversive critique of American middle-class life, gaining notoriety for its bold satire in the 1990s. Initially a countercultural phenomenon, it challenged norms with episodes tackling religion, politics, and consumerism. Over time, its cultural dominance waned as competitors like South Park and Family Guy pushed boundaries further. By the 2010s, the show transitioned from trendsetter to nostalgic institution, balancing legacy appeal with attempts to address modern issues like climate change and LGBTQ+ rights, albeit with less societal resonance.\n…\nConclusion\nThe Simpsons evolved from a radical satire to a television institution, navigating shifts in technology, politics, and audience expectations. While its golden-age brilliance remains unmatched, its adaptability—through streaming, updated humor, and global outreach—secures its place as a cultural touchstone. The show’s longevity reflects both nostalgia and a pragmatic embrace of change, even as it grapples with the challenges of relevance in a fragmented media landscape.\n```\n\n\nFind [the full report here](https://drive.google.com/file/d/1GE3rvxFFTKqro67ctTkknryUf-ojhduN/view?usp=sharing), and [a report produced by DeepSearcher with GPT-4o mini](https://drive.google.com/file/d/1EGd16sJDNFnssk9yTd5o9jzbizrY_NS_/view?usp=sharing) for comparison.\n\n\n## Discussion\n\nWe presented [DeepSearcher](https://github.com/zilliztech/deep-searcher), an agent for performing research and writing reports. Our system is built upon the idea in our previous article, adding features like conditional execution flow, query routing, and an improved interface. We switched from local inference with a small 4-bit quantized reasoning model to an online inference service for the massive DeepSeek-R1 model, qualitatively improving our output report. DeepSearcher works with most inference services like OpenAI, Gemini, DeepSeek and Grok 3 (coming soon!).\n\nReasoning models, especially as used in research agents, are inference-heavy, and we were fortunate to be able to use the fastest offering of DeepSeek-R1 from SambaNova running on their custom hardware. For our demonstration query, we made sixty-five calls to SambaNova’s DeepSeek-R1 inference service, inputting around 25k tokens, outputting 22k tokens, and costing $0.30. We were impressed with the speed of inference given that the model contains 671-billion parameters and is 3/4 of a terabyte large. [Find out more details here!](https://sambanova.ai/press/fastest-deepseek-r1-671b-with-highest-efficiency)\n\nWe will continue to iterate on this work in future posts, examining additional agentic concepts and the design space of research agents. In the meanwhile, we invite everyone to try out [DeepSearcher](https://github.com/zilliztech/deep-searcher), [star us on GitHub](https://github.com/zilliztech/deep-searcher), and share your feedback!\n\n\n## Resources\n\n- [**Zilliz’s DeepSearcher**](https://github.com/zilliztech/deep-searcher)\n\n- Background reading: [**_“I Built a Deep Research with Open Source—and So Can You!”_**](https://milvus.io/blog/i-built-a-deep-research-with-open-source-so-can-you.md)\n\n- _“_[__SambaNova Launches the Fastest DeepSeek-R1 671B with the Highest Efficiency__](https://sambanova.ai/press/fastest-deepseek-r1-671b-with-highest-efficiency)_”_\n\n- DeepSearcher: [DeepSeek-R1 report on The Simpsons](https://drive.google.com/file/d/1GE3rvxFFTKqro67ctTkknryUf-ojhduN/view?usp=sharing)\n\n- DeepSearcher: [GPT-4o mini report on The Simpsons](https://drive.google.com/file/d/1EGd16sJDNFnssk9yTd5o9jzbizrY_NS_/view?usp=sharing)\n\n- [Milvus Open-Source Vector Database](https://milvus.io/docs)\n","title":"Introducing DeepSearcher: A Local Open Source Deep Research","metaData":{}},{"id":"what-milvus-taught-us-in-2024.md","author":"Stefan Webb","desc":"Check out the top asked questions about Milvus in our Discord.","tags":["Engineering"],"recommend":true,"canonicalUrl":"https://milvus.io/blog/what-milvus-taught-us-in-2024.md","date":"2025-02-18T00:00:00.000Z","cover":"https://assets.zilliz.com/What_Milvus_Users_Taught_Us_in_2024_db63863725.png","href":"/blog/what-milvus-taught-us-in-2024.md","content":"\n## Overview\n\nAs Milvus flourished in 2024 with major releases and a thriving open-source ecosystem, a hidden treasure trove of user insights was quietly forming in our community on [Discord](https://discord.gg/xwqmFDURcz). This compilation of community discussions presented a unique opportunity to understand our users' challenges firsthand. Intrigued by this untapped resource, I embarked on a comprehensive analysis of every discussion thread from the year, searching for patterns that could help us compile a frequently asked questions resource for Milvus users.\n\n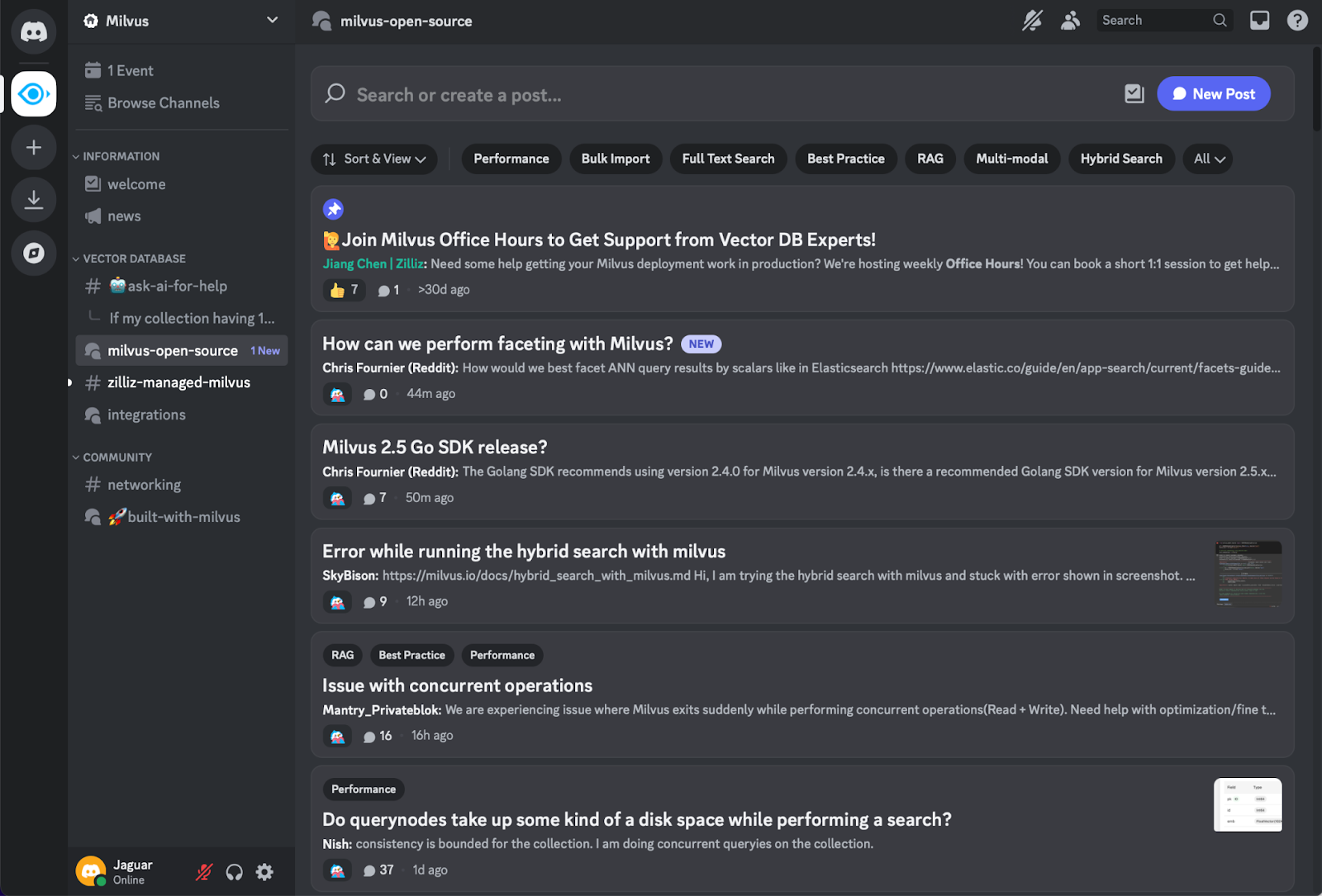\n\nMy analysis revealed three primary areas where users consistently sought guidance: **Performance Optimization**, **Deployment Strategies**, and **Data Management**. Users frequently discussed how to fine-tune Milvus for production environments and track performance metrics effectively. When it came to deployment, the community grappled with selecting appropriate deployments, choosing optimal search indices, and resolving issues in distributed setups. The data management conversations centered around service-to-service data migration strategies and the selection of embedding models.\n\nLet’s examine each of these areas in more detail.\n\n## Deployment\n\n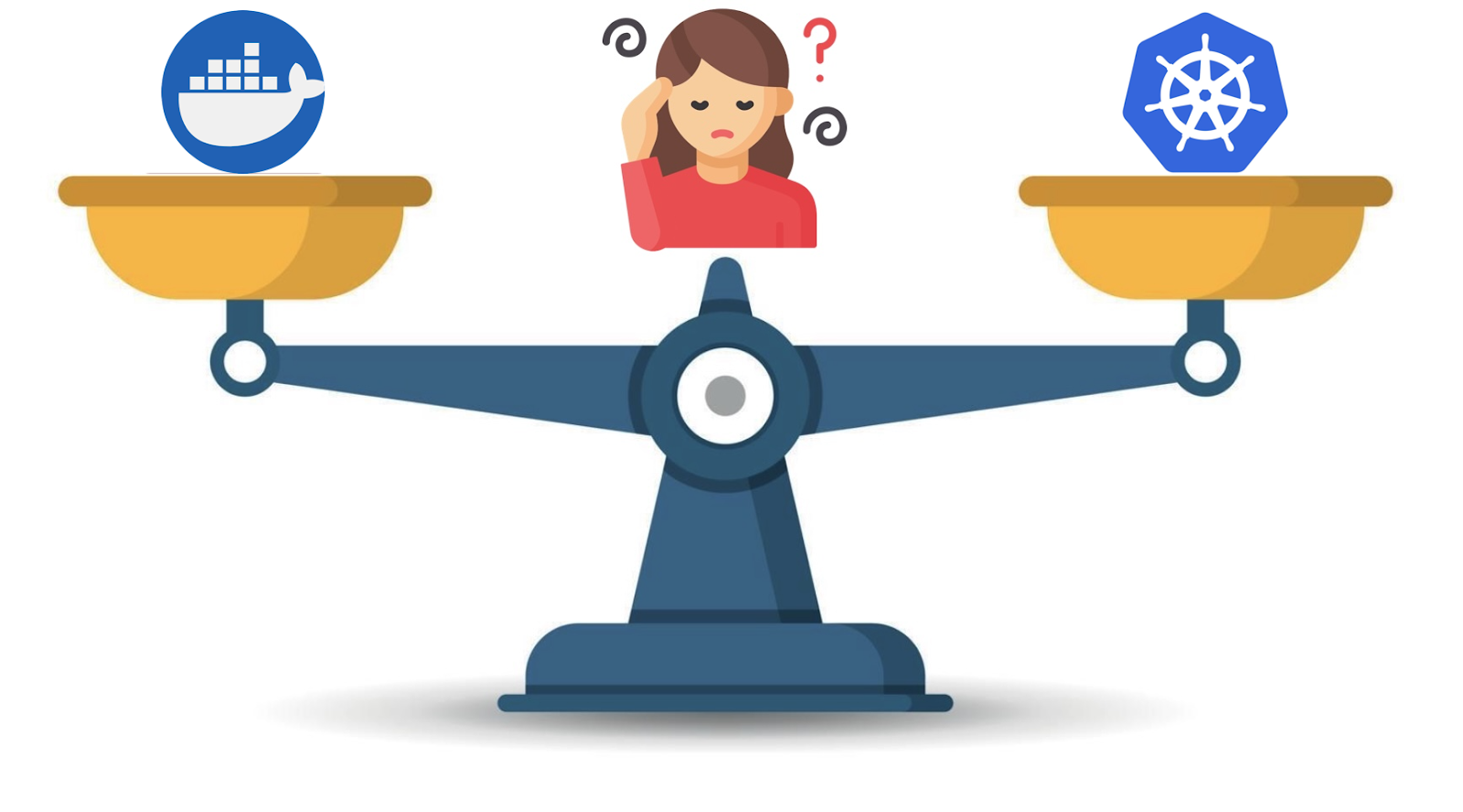\n\nMilvus provides flexible deployment modes to fit various use cases. However, some users do find it challenging to find the right choice, and want to feel comfortable that they are doing so “correctly.”\n\n\n### Which deployment type should I choose?\n\nA very frequent question is which deployment to choose out of Milvus [Lite](https://milvus.io/docs/milvus_lite.md), [Standalone](https://milvus.io/docs/prerequisite-docker.md), and [Distributed](https://milvus.io/docs/prerequisite-helm.md). The answer primarily depends on how large your vector database needs to be and how much traffic it will serve: \n\n\n#### Milvus Lite\n\nWhen prototyping on your local system with up to a few million vectors, or looking for an embedded vector db for unit testing and CI/CD, you can use Milvus Lite. Note that some more advanced features like full-text search are not yet available within Milvus Lite but coming soon.\n\n\n#### Milvus Standalone\n\nIf your system needs to serve production traffic and / or you need to store between a few million and a hundred-million vectors, you should use Milvus Standalone, which packs all components of Milvus into a single Docker image. There is a variation which just takes its persistent storage (minio) and metadata store (etcd) dependencies out as separate images. \n\n\n#### Milvus Distributed\n\nFor any larger scale deployments serving production traffic, like serving billions of vectors at thousands of QPS, you should use Milvus Distributed. Some users may want to perform offline batch processing at scale, for example, for data deduplication or record linkage, and the future version of Milvus 3.0 will provide a more efficient way of doing this by what we term a vector lake.\n\n\n#### Fully Managed Service\n\nFor developers who want to focus on the application development without worrying about DevOps, [Zilliz Cloud](https://cloud.zilliz.com/signup) is the fully managed Milvus that offers a free tier.\n\nSee [“Overview of Milvus Deployments”](https://milvus.io/docs/install-overview.md#Choose-the-Right-Deployment-for-Your-Use-Case) for more information.\n\n\n### How much memory, storage, and compute will I require?\n\nThis question comes up a lot, not only for existing Milvus users but also those who are considering whether Milvus is appropriate for their application. The exact combination of how much memory, storage, and compute a deployment will require depends on complex interaction of factors.\n\nVector embeddings differ in dimensionality due to the model that is used. And some vector search indexes are stored entirely in memory, whereas others store data to disk. Also, many search indexes are able to store a compressed (quantized) copy of the embeddings and require additional memory for graph data structures. These are just a few factors that affect the memory and storage.\n\n\n#### Milvus Resource Sizing Tool\n\nLuckily, Zilliz (the team that maintains Milvus) has built [a resource sizing tool](https://milvus.io/tools/sizing) that does a fantastic job of answering this question. Input your vector dimensionality, index type, deployment options, and so on and the tool estimates CPU, memory, and storage needed across the various types of Milvus nodes and its dependencies. Your mileage may vary so a real load testing with your data and sample traffic is always a good idea. \n\n\n### Which vector index or distance metric should I choose?\n\nMany users are uncertain which index they should choose and how to set the hyperparameters. First, it is always possible to defer the choice of index type to Milvus by selecting AUTOINDEX. If you wish to select a specific index type, however, a few rules of thumb provide a starting point.\n\n\n#### In-Memory Indexes\n\nWould you like to pay the cost to fit your index entirely into memory? An in-memory index is typically the fastest but also expensive. See [“In-memory indexes”](https://milvus.io/docs/index.md?tab=floating) for a list of the ones supported by Milvus and the tradeoffs they make in terms of latency, memory, and recall.\n\nKeep in mind that your index size is not simply the number of vectors times their dimensionality and floating point size. Most indexes quantize the vectors to reduce memory usage, but require memory for additional data structures. Other non-vector data (scalar) and their index also takes up memory space.\n\n\n#### On-Disk Indexes\n\nWhen your index does not fit in memory, you can use one of the [“On-disk indexes”](https://milvus.io/docs/disk_index.md) provided by Milvus. Two choices with very different latency/resource tradeoffs are [DiskANN](https://milvus.io/docs/disk_index.md) and [MMap](https://milvus.io/docs/mmap.md#MMap-enabled-Data-Storage). \n\nDiskANN stores a highly compressed copy of the vectors in memory, and the uncompressed vectors and graph search structures on disk. It uses some clever ideas to search the vector space while minimizing disk reads and takes advantage of the fast random access speed of SSDs. For minimum latency, the SSD must be connected via NVMe rather than SATA for best I/O performance. \n\nTechnically speaking, MMap is not an index type, but refers to the use of virtual memory with an in-memory index. With virtual memory, pages can be swapped between disk and RAM as required, which allows a much larger index to be used efficiently if the access patterns are such that only a small portion of the data is used at a time.\n\nDiskANN has excellent and consistent latency. MMap has even better latency when it is accessing a page in-memory, but frequent page-swapping will cause latency spikes. Thus MMap can have a higher variability in latency, depending on the memory access patterns.\n\n\n#### GPU Indexes\n\nA third option is to construct [an index using GPU memory and compute](https://milvus.io/docs/gpu_index.md). Milvus’ GPU support is contributed by the Nvidia [RAPIDS](https://rapids.ai/) team. GPU vector search may have lower latency than a corresponding CPU search, although it usually takes hundreds or thousands of search QPS to fully exploit the parallelism of GPU. Also, GPUs typically have less memory than the CPU RAM and are more costly to run.\n\n\n#### Distance Metrics\n\nAn easier question to answer is which distance metric should you choose to measure similarity between vectors. It is recommended to choose the same distance metric that your embedding model was trained with, which is typically COSINE (or IP when inputs are normalized). The source of your model (e.g. the model page on HuggingFace) will provide clarification on which distance metric was used. Zilliz also put together a convenient [table](https://zilliz.com/ai-models) to look that up.\n\nTo summarize, I think a lot of the uncertainty around index choice revolves around uncertainty about how these choices affect the latency/resource usage/recall tradeoff of your deployment. I recommend using the rules of thumb above to decide between in-memory, on-disk, or GPU indexes, and then using the tradeoff guidelines given in the Milvus documentation to pick a particular one.\n\n\n### Can you fix my broken Milvus Distributed deployment?\n\nMany questions revolve around issues getting a Milvus Distributed deployment up and running, with questions relating to configuration, tooling, and debugging logs. It’s hard to give a single fix as each question seems different from the last, although luckily Milvus has [a vibrant Discord](https://milvus.io/discord) where you can seek help, and we also offer [1-on-1 office hours with an expert](https://milvus.io/blog/join-milvus-office-hours-to-get-support-from-vectordb-experts.md).\n\n\n### How do I deploy Milvus on Windows?\n\nA question that has come up several times is how to deploy Milvus on Windows machines. Based on your feedback, we have rewritten the documentation for this: see [Run Milvus in Docker (Windows)](https://milvus.io/docs/install_standalone-windows.md) for how to do this, using [Windows Subsystem for Linux 2 (WSL2)](https://learn.microsoft.com/en-us/windows/wsl/about#what-is-wsl-2).\n\n\n## Performance and Profiling\n\n\n\nHaving chosen a deployment type and got it running, users want to feel comfortable that they have made optimal decisions and would like to profile their deployment’s performance and state. Many questions related to how to profile performance, observe state, and get an insight into what and why. \n\n\n### How do I measure performance?\n\nUsers want to check metrics related to the performance of their deployment so they can understand and remedy bottlenecks. Metrics mentioned include average query latency, distribution of latencies, query volume, memory usage, disk storage, and so on. While obtaining these metrics with [the legacy monitoring system](https://milvus.io/docs/monitor_overview.md) has been challenging, Milvus 2.5 introduces a new system called [WebUI](https://milvus.io/docs/milvus-webui.md#Milvus-WebUI) (feedback welcome!), which allows you to access all this information from a user-friendly web interface.\n\n\n### What’s happening inside Milvus right now (i.e. observe state)?\n\nRelatedly, users want to observe the internal state of their deployment. Issues raised include understanding why a search index is taking so long to build, how to determine if the cluster is healthy, and understanding how a query is executed across nodes. Many of these questions can be answered with the new [WebUI](https://milvus.io/docs/milvus-webui.md#Milvus-WebUI) that gives transparency to what the system is doing internally.\n\n\n### How does some (complex) aspect of the internals work?\n\nAdvanced users often want some understanding of Milvus internals, for example, having an understanding of the sealing of segments or memory management. The underlying goal is typically to improve performance and sometimes to debug issues. The documentation, particularly under the sections “Concepts” and “Administration Guide\" is helpful here, for instance see the pages [“Milvus Architecture Overview”](https://milvus.io/docs/architecture_overview.md) and [“Clustering Compaction”](https://milvus.io/docs/clustering-compaction.md). We will continue to improve the documentation on Milvus internals, make it easier to understand, and welcome any feedback or requests via [Discord](https://milvus.io/discord).\n\n\n### Which embedding model should I choose?\n\nA question related to performance that has come up multiple times in meetups, office hours, and on Discord is how to choose an embedding model. This is a difficult question to give a definitive answer although we recommend starting with default models like [all-MiniLM-L6-v2](https://huggingface.co/sentence-transformers/all-MiniLM-L6-v2). \n\nSimilar to the choice of search index, there are tradeoffs between compute, storage, and recall. An embedding model with larger output dimension will require more storage, all else held equal, although probably result in higher recall of relevant items. Larger embedding models, for a fixed dimension, typically outperform smaller ones in terms of recall, although at the cost of increased compute and time. Leaderboards that rank embedding model performance such as [MTEB](https://huggingface.co/spaces/mteb/leaderboard) are based on benchmarks that may not align with your specific data and task.\n\nSo, it does not make sense to think of a “best” embedding model. Start with one that has acceptable recall and meets your compute and time budget for calculating embeddings. Further optimizations like fine-tuning on your data or exploring the compute/recall tradeoff empirically can be deferred to after you have a working system in production.\n\n\n## Data Management\n\n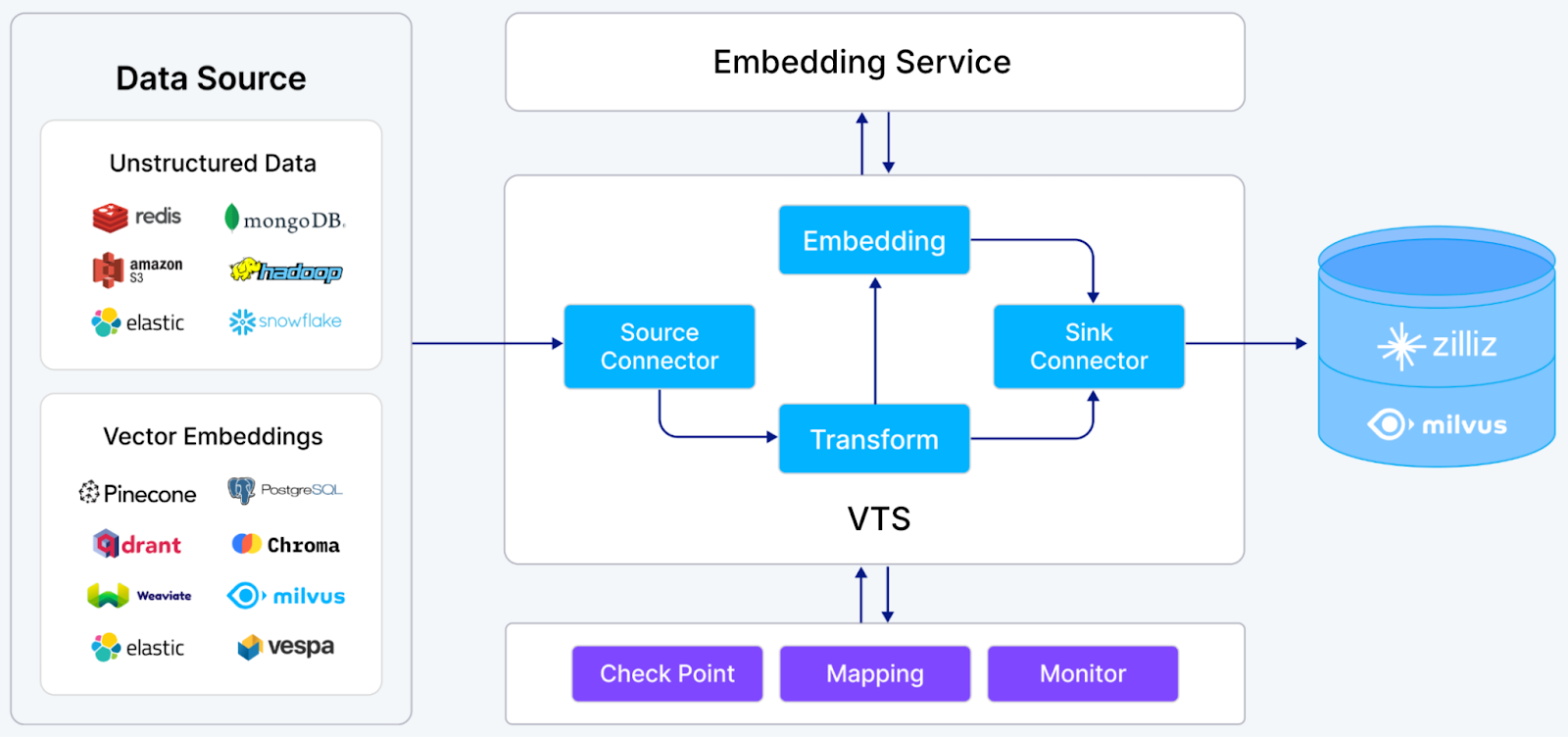\n\nHow to move data into and out of a Milvus deployment is another main theme in the Discord discussions, which is no surprise given how central this task is to putting an application into production.\n\n\n### How do I migrate data from X to Milvus? How do I migrate data from Standalone to Distributed? How do I migrate from 2.4.x to 2.5.x?\n\nA new user commonly wants to get existing data into Milvus from another platform, including traditional search engines like [Elasticsearch](https://docs.zilliz.com/docs/migrate-from-elasticsearch) and other vector databases like [Pinecone](https://docs.zilliz.com/docs/migrate-from-pinecone) or [Qdrant](https://docs.zilliz.com/docs/migrate-from-qdrant). Existing users may also want to migrate their data from one Milvus deployment to another, or [from self-hosted Milvus to fully managed Zilliz Cloud](https://docs.zilliz.com/docs/migrate-from-milvus).\n\nThe [Vector Transport Service (VTS)](https://github.com/zilliztech/vts) and the managed [Migration](https://docs.zilliz.com/docs/migrations) service on Zilliz Cloud are designed for this purpose.\n\n\n### How do I save and load data backups? How do I export data from Milvus?\n\nMilvus has a dedicated tool, [milvus-backup](https://github.com/zilliztech/milvus-backup), to take snapshots on permanent storage and restore them.\n\n\n## Next Steps\n\nI hope this has given you some pointers on how to tackle common challenges faced when building with a vector database. This definitely helped us to take another look at our documentation and feature roadmap to keep working on things that can help our community best succeed with Milvus. A key takeaway that I would like to emphasize, is that your choices put you within different points of a tradeoff space between compute, storage, latency, and recall. _You cannot maximize all of these performance criteria simultaneously - there is no “optimal” deployment. Yet, by understanding more about how vector search and distributed database systems work you can make an informed decision._\n\nAfter trawling through the large number of posts from 2024, it got me thinking: why should a human do this? Has not Generative AI promised to solve such a task of crunching large amounts of text and extracting insight? Join me in the second part of this blog post (coming soon), where I investigate the design and implementation of _a multi-agent system for extracting insight from discussion forums._\n\nThanks again and hope to see you in the community [Discord](https://milvus.io/discord) and our next [Unstructured Data](https://lu.ma/unstructured-data-meetup) meetups. For more hands-on assistance, we welcome you to book a [1-on-1 office hour](https://milvus.io/blog/join-milvus-office-hours-to-get-support-from-vectordb-experts.md). _Your feedback is essential to improving Milvus!_\n","title":"What Milvus Users Taught Us in 2024","metaData":{}},{"id":"i-built-a-deep-research-with-open-source-so-can-you.md","author":"Stefan Webb","desc":"Learn how to create a Deep Research-style agent using open-source tools like Milvus, DeepSeek R1, and LangChain.","tags":["Tutorials"],"recommend":true,"canonicalUrl":"https://milvus.io/blog/i-built-a-deep-research-with-open-source-so-can-you.md","date":"2025-02-06T00:00:00.000Z","cover":"https://assets.zilliz.com/I_Built_a_Deep_Research_with_Open_Source_and_So_Can_You_7eb2a38078.png","href":"/blog/i-built-a-deep-research-with-open-source-so-can-you.md","content":"\n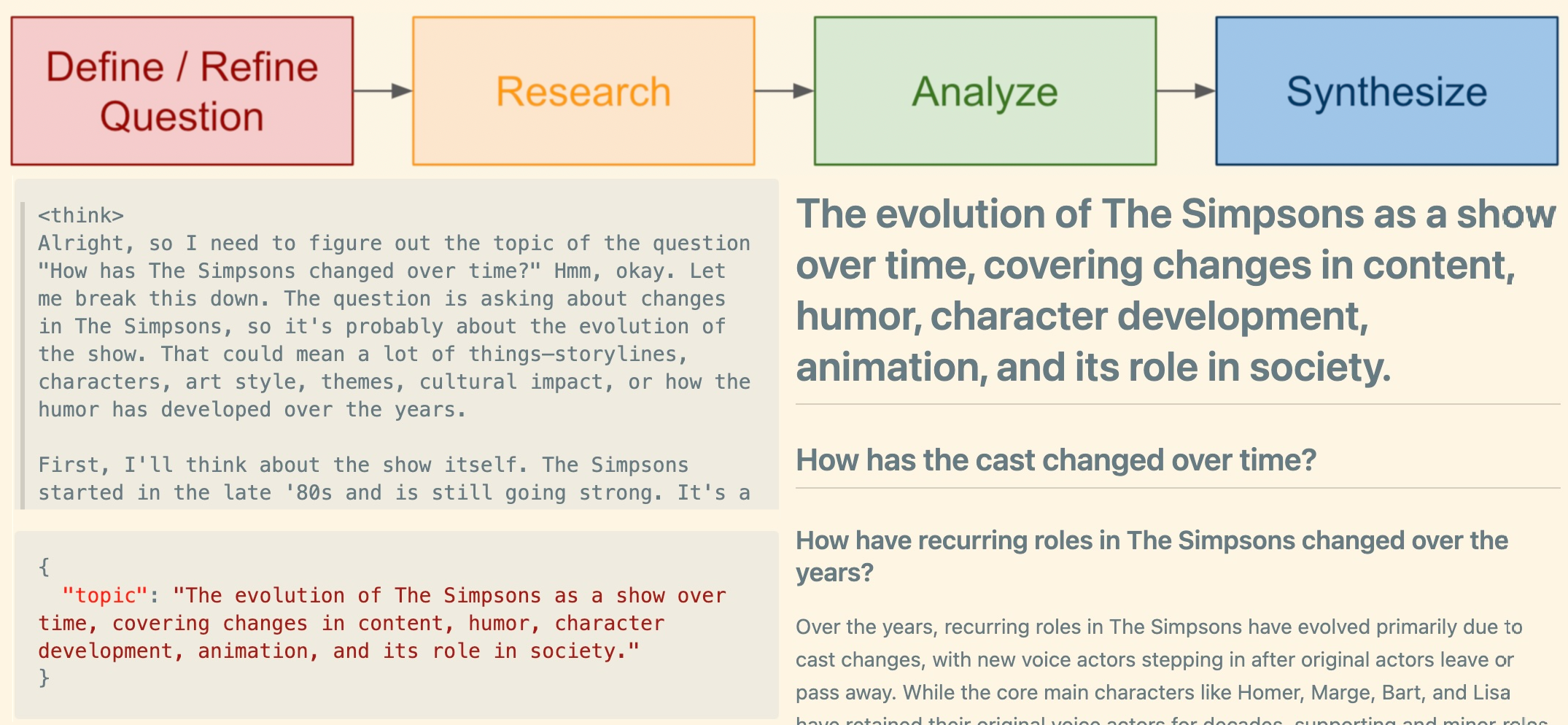\n\nWell actually, a minimally scoped agent that can reason, plan, use tools, etc. to perform research using Wikipedia. Still, not bad for a few hours of work…\n\nUnless you reside under a rock, in a cave, or in a remote mountain monastery, you will have heard about OpenAI’s release of _Deep Research_ on Feb 2, 2025. This new product promises to revolutionize how we answer questions requiring the synthesis of large amounts of diverse information.\n\nYou type in your query, select the Deep Research option, and the platform autonomously searches the web, performs reasoning on what it discovers, and synthesizes multiple sources into a coherent, fully-cited report. It takes several orders of magnitude longer to produce its output relative to a standard chatbot, but the result is more detailed, more informed, and more nuanced.\n\n\n## How does it work?\n\nBut how does this technology work, and why is Deep Research a noticeable improvement over previous attempts (like Google’s _Deep Research_ - incoming trademark dispute alert)? We’ll leave the latter for a future post. As for the former, there is no doubt much “secret sauce” underlying Deep Research. We can glean a few details from OpenAI’s release post, which I summarize.\n\n**Deep Research exploits recent advances in foundation models specialized for reasoning tasks:**\n\n- “...fine-tuned on the upcoming OpenAI o3 reasoning model…”\n\n- “...leverages reasoning to search, interpret, and analyze massive amounts of text…”\n\n**Deep Research makes use of a sophisticated agentic workflow with planning, reflection, and memory:**\n\n- “...learned to plan and execute a multi-step trajectory…”\n\n- “...backtracking and reacting to real-time information…”\n\n- “...pivoting as needed in reaction to information it encounters…”\n\n**Deep Research is trained on proprietary data, using several types of fine-tuning, which is likely a key component in its performance:**\n\n- “...trained using end-to-end reinforcement learning on hard browsing and reasoning tasks across a range of domains…”\n\n- “...optimized for web browsing and data analysis…”\n\nThe exact design of the agentic workflow is a secret, however, we can build something ourselves based on well-established ideas about how to structure agents.\n\n**One note before we begin**: It is easy to be swept away by Generative AI fever, especially when a new product that seems a step-improvement is released. However, Deep Research, as OpenAI acknowledges, has limitations common to Generative AI technology. We should remember to think critically about the output in that it may contain false facts (“hallucinations”), incorrect formatting and citations, and vary significantly in quality based on the random seed.\n\n\n## Can I build my own?\n\nWhy certainly! Let’s build our own “Deep Research”, running locally and with open-source tools. We’ll be armed with just a basic knowledge of Generative AI, common sense, a couple of spare hours, a GPU, and the open-source [Milvus](https://milvus.io/docs), [DeepSeek R1](https://huggingface.co/unsloth/DeepSeek-R1-Distill-Llama-8B-unsloth-bnb-4bit), and [LangChain](https://python.langchain.com/docs/introduction/).\n\nWe cannot hope to replicate OpenAI’s performance of course, but our prototype will minimally demonstrate some of the key ideas likely underlying their technology, combining advances in reasoning models with advances in agentic workflows. Importantly, and unlike OpenAI, we will be using only open-source tools, and be able to deploy our system locally - open-source certainly provides us great flexibility!\n\nWe will make a few simplifying assumptions to reduce the scope of our project:\n\n- We will use an open-source reasoning mode distilled then [quantized](https://zilliz.com/learn/unlock-power-of-vector-quantization-techniques-for-efficient-data-compression-and-retrieval) for 4-bits that can be run locally.\n\n- We will not perform additional fine-tuning on our reasoning model ourselves.\n\n- The only tool our agent has is the ability to download and read a Wikipedia page and perform separate RAG queries (we will not have access to the entire web).\n\n- Our agent will only process text data, not images, PDFs, etc.\n\n- Our agent will not backtrack or consider pivots.\n\n- Our agent will (not yet) control its execution flow based on its output.\n\n- Wikipedia contains the truth, the whole truth and nothing but the truth.\n\nWe will use [Milvus](https://milvus.io/docs) for our vector database, [DeepSeek R1](https://huggingface.co/unsloth/DeepSeek-R1-Distill-Llama-8B-unsloth-bnb-4bit) as our reasoning model, and [LangChain](https://python.langchain.com/docs/introduction/) to implement RAG. Let’s get started! \n\n\n# A Minimal Agent for Online Research\n\nWe will use our mental model of how humans conduct research to design the agentic workflow:\n\n\n### Define/Refine Question\n\nResearch starts by defining a question. We take the question to be the user’s query, however, we use our reasoning model to ensure the question is expressed in a way that is specific, clear, and focused. That is, our first step is to rewrite the prompt and extract any subqueries or subquestions. We make effective use of our foundation models specialization for reasoning, and a simple method for JSON structured output.\n\nHere is an example reasoning trace as DeepSeek refines the question “How has the cast changed over time?”:\n\n```text\n\u003cthink\u003e\n\nAlright, so I need to break down the question \"How has the cast changed over time?\" related to the evolution of The Simpsons. Let me think about what aspects are involved here.\n\nFirst, I know that the cast has changed a lot, but I need to figure out the intermediate questions to approach this. The main question is about changes in the cast over time, so I should consider different areas that contribute to this change.\n\nI guess the first sub-question would be about the original cast members. Who were the main voices and how did they evolve? Then, there might be new cast additions over the years, so another sub-question about that.\n\nAlso, some original voice actors have left, so I should include a sub-question about departures. Then, new voice actors joining would be another point.\n\nThe show has been popular for a long time, so recurring roles changing might be another aspect. Additionally, the role of the show in society might have influenced casting choices, so a sub-question about that.\n\nLastly, the overall impact on the cast's careers could be another angle. So, I should list these as sub-questions to cover all aspects.\n\n\u003c/think\u003e\n```\n\n### Search\n\nNext, we conduct a “literature review” of Wikipedia articles. For now, we read a single article and leave navigating links to a future iteration. We discovered during prototyping that link exploration can become very expensive if each link requires a call to the reasoning model. We parse the article, and store its data in our vector database, Milvus, akin to taking notes.\n\nHere is a code snippet showing how we store our Wikipedia page in Milvus using its LangChain integration:\n\n```python\nwiki_wiki = wikipediaapi.Wikipedia(user_agent='MilvusDeepResearchBot (\u003cinsert your email\u003e)', language='en')\npage_py = wiki_wiki.page(page_title)\n\ntext_splitter = RecursiveCharacterTextSplitter(chunk_size=2000, chunk_overlap=200)\ndocs = text_splitter.create_documents([page_py.text])\n\nvectorstore = Milvus.from_documents( # or Zilliz.from_documents\n documents=docs,\n embedding=embeddings,\n connection_args={\n \"uri\": \"./milvus_demo.db\",\n },\n drop_old=True, \n index_params={\n \"metric_type\": \"COSINE\",\n \"index_type\": \"FLAT\", \n \"params\": {},\n },\n)\n```\n\n### Analyze\n\nThe agent returns to its questions and answers them based on the relevant information in the document. We will leave a multi-step analysis/reflection workflow for future work, as well as any critical thinking on the credibility and bias of our sources.\n\nHere is a code snippet illustrating constructing a RAG with LangChain and answering our subquestions separately.\n\n```python\n# Define the RAG chain for response generation\nrag_chain = (\n {\"context\": retriever | format_docs, \"question\": RunnablePassthrough()}\n | prompt\n | llm\n | StrOutputParser()\n)\n\n# Prompt the RAG for each question\nanswers = {}\ntotal = len(leaves(breakdown))\n\npbar = tqdm(total=total)\nfor k, v in breakdown.items():\n if v == []:\n print(k)\n answers[k] = rag_chain.invoke(k).split('\u003c/think\u003e')[-1].strip()\n pbar.update(1)\n else:\n for q in v:\n print(q)\n answers[q] = rag_chain.invoke(q).split('\u003c/think\u003e')[-1].strip()\n pbar.update(1)\n```\n\n### Synthesize\n\nAfter the agent has performed its research, it creates a structured outline, or rather, a skeleton, of its findings to summarize in a report. It then completes each section, filling it in with a section title and the corresponding content. We leave a more sophisticated workflow with reflection, reordering, and rewriting for a future iteration. This part of the agent involves planning, tool usage, and memory.\n\nSee [accompanying notebook](https://drive.google.com/file/d/1waKX_NTgiY-47bYE0cI6qD8Cjn3zjrL6/view?usp=sharing) for the full code and the [saved report file](https://drive.google.com/file/d/15xeEe_EqY-29V2IlAvDy5yGdJdEPSHOh/view?usp=drive_link) for example output.\n\n\n## Results\n\nOur query for testing is _“How has The Simpsons changed over time?”_ and the data source is the Wikipedia article for “The Simpsons”. Here is one section of the [generated report](https://drive.google.com/file/d/15xeEe_EqY-29V2IlAvDy5yGdJdEPSHOh/view?usp=sharing):\n\n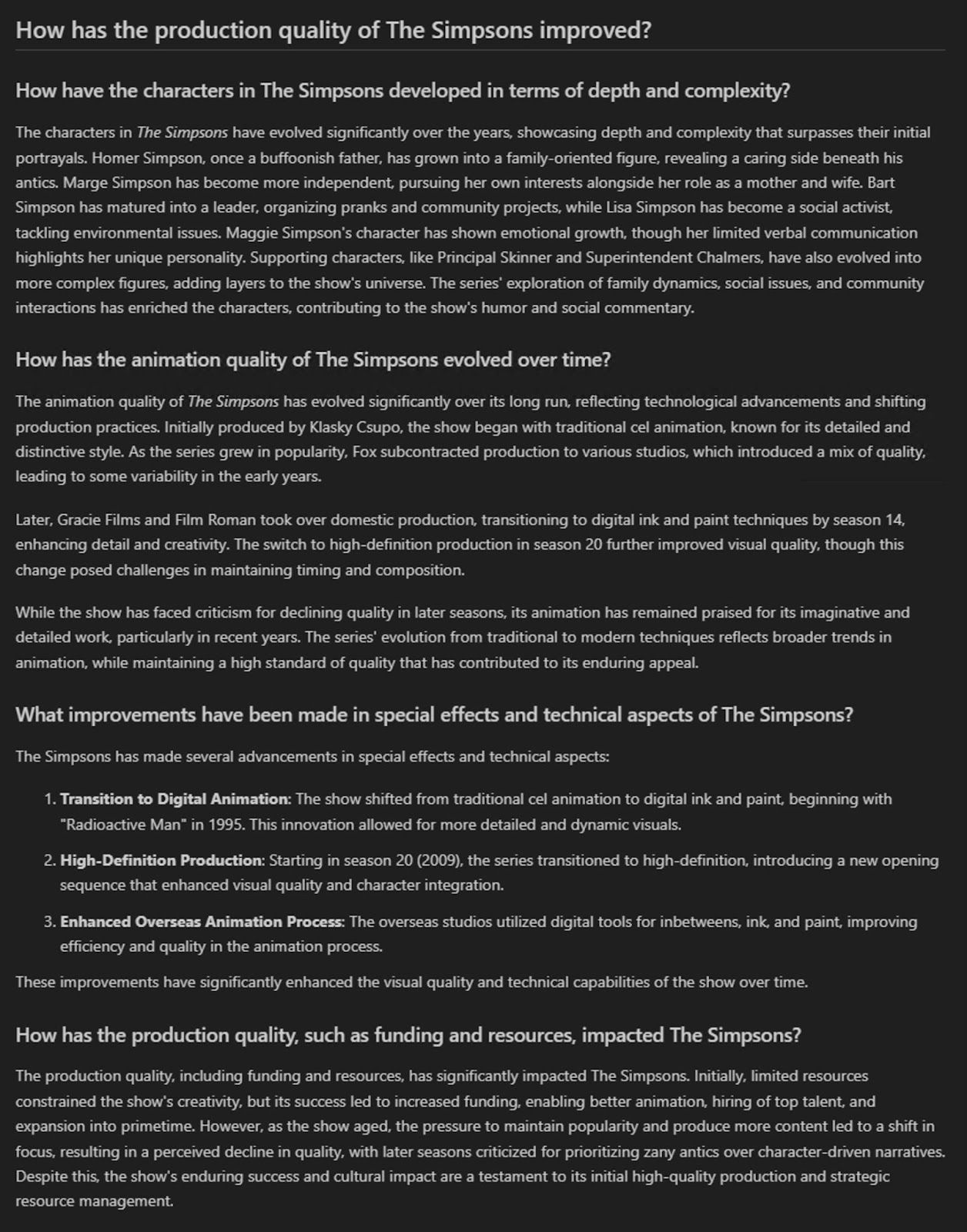\n\n\n## Summary: What we built and what’s next\n\nIn just a few hours, we have designed a basic agentic workflow that can reason, plan, and retrieve information from Wikipedia to generate a structured research report. While this prototype is far from OpenAI’s Deep Research, it demonstrates the power of open-source tools like Milvus, DeepSeek, and LangChain in building autonomous research agents. \n\nOf course, there’s plenty of room for improvement. Future iterations could:\n\n- Expand beyond Wikipedia to search multiple sources dynamically\n\n- Introduce backtracking and reflection to refine responses\n\n- Optimize execution flow based on the agent's own reasoning\n\nOpen-source gives us flexibility and control that closed source doesn’t. Whether for academic research, content synthesis, or AI-powered assistance, building our own research agents open up exciting possibilities. Stay tuned for the next post where we explore adding real-time web retrieval, multi-step reasoning, and conditional execution flow!\n\n\n## Resources\n\n- Notebook: _“_[_Baseline for An Open-Source Deep Research_](https://colab.research.google.com/drive/1W5tW8SqWXve7ZwbSb9pVdbt5R2wq105O?usp=sharing)_”_\n\n- Report: _“_[_The evolution of The Simpsons as a show over time, covering changes in content, humor, character development, animation, and its role in society._](https://drive.google.com/file/d/15xeEe_EqY-29V2IlAvDy5yGdJdEPSHOh/view?usp=drive_link)_”_\n\n- [Milvus vector database documentation](https://milvus.io/docs)\n\n- [Distilled and quantized DeepSeek R1 model page](https://huggingface.co/unsloth/DeepSeek-R1-Distill-Llama-8B-unsloth-bnb-4bit)\n\n- [️🔗 LangChain](https://python.langchain.com/docs/introduction/)\n\n- [Deep Research FAQ | OpenAI Help Center](https://help.openai.com/en/articles/10500283-deep-research-faq)\n\n\n\n","title":"I Built a Deep Research with Open Source—and So Can You!","metaData":{}},{"id":"multimodal-semantic-search-with-images-and-text.md","author":"Stefan Webb","desc":"Learn how to build a semantic search app using multimodal AI that understands text-image relationships, beyond basic keyword matching.","tags":["Engineering"],"recommend":true,"canonicalUrl":"https://milvus.io/blog/multimodal-semantic-search-with-images-and-text.md","date":"2025-02-03T00:00:00.000Z","cover":"https://assets.zilliz.com/Multimodal_Semantic_Search_with_Images_and_Text_1_3da9b83015.png","href":"/blog/multimodal-semantic-search-with-images-and-text.md","content":"\n\u003ciframe width=\"100%\" height=\"315\" src=\"https://www.youtube.com/embed/bxE0_QYX_sU?si=PkOHFcZto-rda1Fv\" title=\"YouTube video player\" frameborder=\"0\" allow=\"accelerometer; autoplay; clipboard-write; encrypted-media; gyroscope; picture-in-picture; web-share\" referrerpolicy=\"strict-origin-when-cross-origin\" allowfullscreen\u003e\u003c/iframe\u003e\n\nAs humans, we interpret the world through our senses. We hear sounds, we see images, video, and text, often layered on top of each other. We understand the world through these multiple modalities and the relationship between them. For artificial intelligence to truly match or exceed human capabilities, it must develop this same ability to understand the world through multiple lenses simultaneously.\n\n\nIn this post and accompanying video (above) and [notebook](https://github.com/milvus-io/bootcamp/blob/master/bootcamp/tutorials/quickstart/multimodal_retrieval_amazon_reviews.ipynb), we'll showcase recent breakthroughs in models that can process both text and images together. We'll demonstrate this by building a semantic search application that goes beyond simple keyword matching - it understands the relationship between what users are asking for and the visual content they're searching through.\n\nWhat makes this project particularly exciting is that it's built entirely with open-source tools: the Milvus vector database, HuggingFace's machine learning libraries, and a dataset of Amazon customer reviews. It's remarkable to think that just a decade ago, building something like this would have required significant proprietary resources. Today, these powerful components are freely available and can be combined in innovative ways by anyone with the curiosity to experiment.\n\n\n# Overview\n\n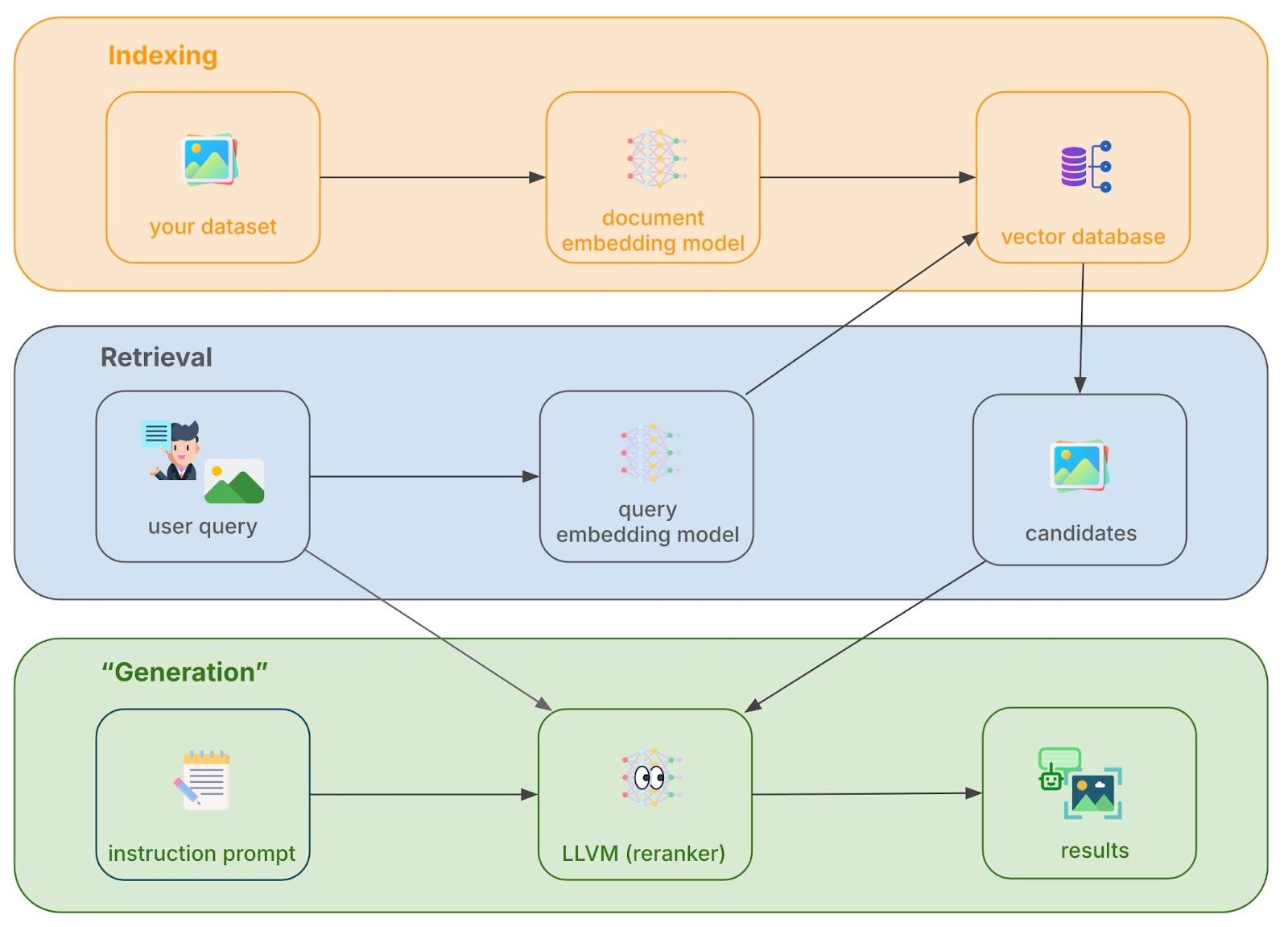\n\nOur multimodal search application is of the type _retrieve-and-rerank._ If you are familiar with _retrieval-augmented-generation_ (RAG) it is very similar, only that the final output is a list of images that were reranked by a large language-vision model (LLVM). The user’s search query contains both text and image, and the target is a set of images indexed in a vector database. The architecture has three steps - _indexing_, _retrieval_, and _reranking_ (akin to “generation”) - which we summarize in turn.\n\n\n## Indexing\n\nOur search application must have something to search. In our case, we use a small subset of the “Amazon Reviews 2023” dataset, which contains both text and images from Amazon customer reviews across all types of products. You can imagine a semantic search like that that we are building as being a useful addition to an ecommerce website. We use 900 images and discard the text, although observe that this notebook can scale to production-size with the right database and inference deployments.\n\nThe first piece of “magic” in our pipeline is the choice of embedding model. We use a recently developed multimodal model called [Visualized BGE](https://huggingface.co/BAAI/bge-visualized) that is able to embed text and images jointly, or either separately, into the same space with a single model where points that are close are semantically similar. Other such models have been developed recently, for instance [MagicLens](https://github.com/google-deepmind/magiclens).\n\n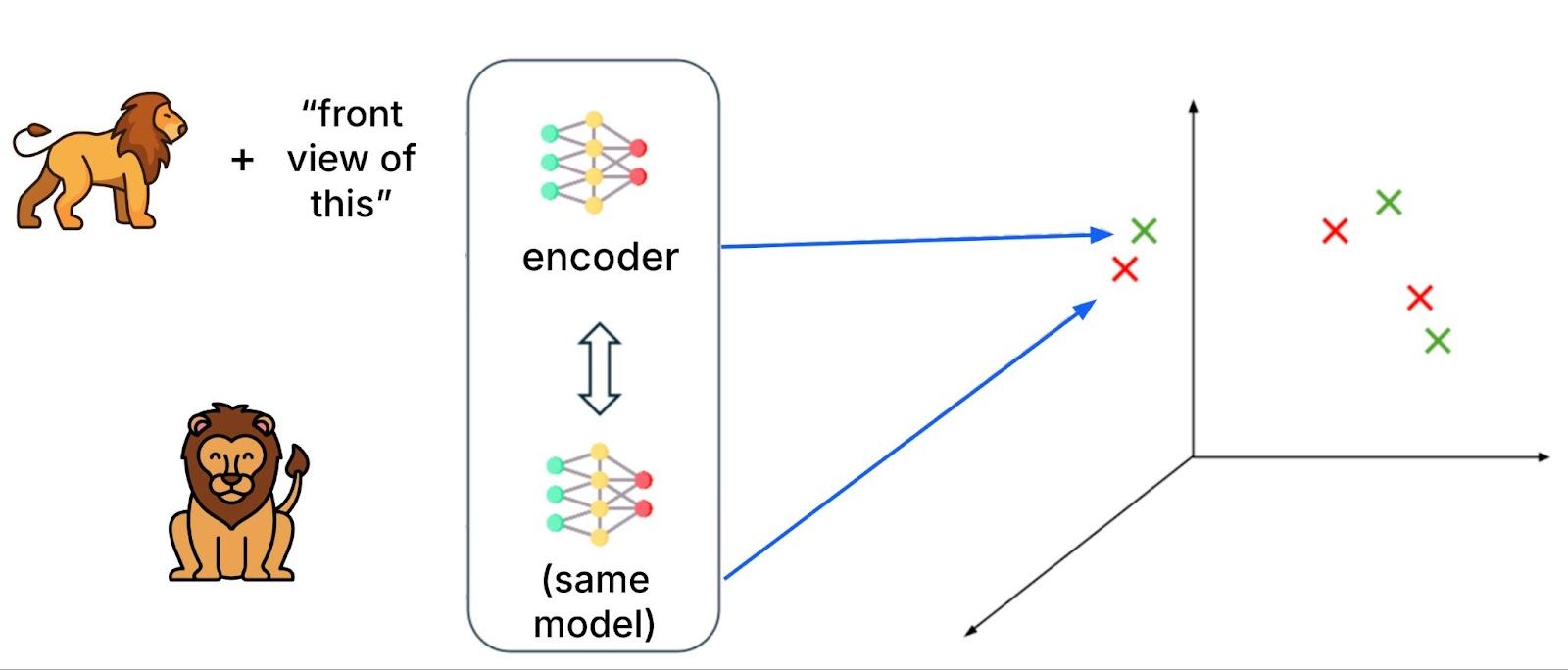\n\nThe figure above illustrates: the embedding for [an image of a lion side-on] plus the text “front view of this”, is close to an embedding for [an image of a lion front-on] without text. The same model is used for both text plus image inputs and image-only inputs (as well as text-only inputs). _In this way, the model is able to understand the user’s intent in how the query text relates to the query image._\n\nWe embed our 900 product images without corresponding text and store the embeddings in a vector database using [Milvus](https://milvus.io/docs).\n\n\n## Retrieval\n\nNow that our database is built, we can serve a user query. Imagine a user comes along with the query: “a phone case with this” plus [an image of a Leopard]. That is, they are searching for phone cases with Leopard skin prints.\n\nNote that the text of the user’s query said “this” rather than “a Leopard’s skin”. Our embedding model must be able to connect “this” to what it refers to, which is an impressive feat given that the previous iteration of models were not able to handle such open-ended instructions. The [MagicLens paper](https://arxiv.org/abs/2403.19651) gives further examples.\n\n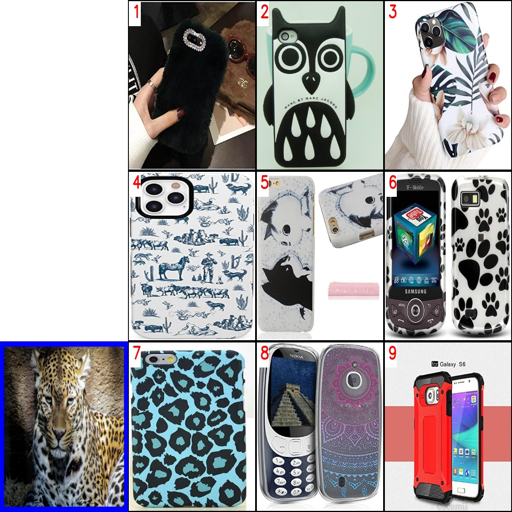\n\nWe embed the query text and image jointly and perform a similarity search of our vector database, returning the top nine hits. The results are shown in the figure above, along with the query image of the leopard. It appears that the top hit is not the one that is most relevant to the query. The seventh result appears to be most relevant - it is a phone cover with a leopard skin print.\n\n\n## Generation\n\nIt appears our search has failed in that the top result is not the most relevant. However, we can fix this with a reranking step. You may be familiar with reranking of retrieved items as being an important step in many RAG pipelines. We use [Phi-3 Vision](https://huggingface.co/microsoft/Phi-3-vision-128k-instruct) as the re-ranker model.\n\nWe first ask a LLVM to generate a caption of the query image. The LLVM outputs:\n\n_“The image shows a close-up of a leopard's face with a focus on its spotted fur and green eyes.”_\n\n \n\nWe then feed this caption, a single image with the nine results and query image, and construct a text prompt asking the model to re-rank the results, giving the answer as a list and providing a reason for the choice of the top match.\n\n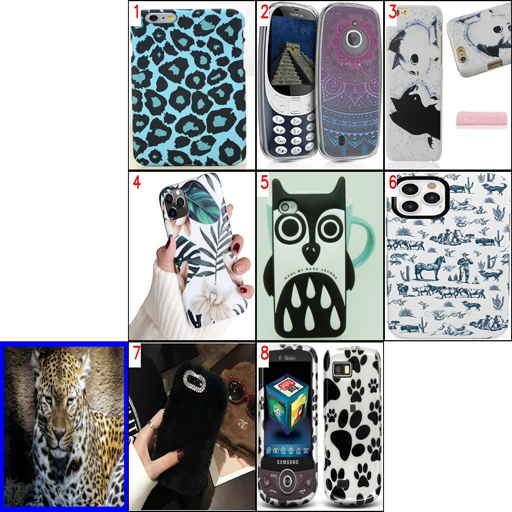\n\nThe output is visualized in the figure above - the most relevant item is now the top match - and the reason given is:\n\n_“The most suitable item is the one with the leopard theme, which matches the user's query instruction for a phone case with a similar theme.”_\n\nOur LLVM re-ranker was able to perform understanding across images and text, and improve the relevance of the search results. _One interesting artifact is that the re-ranker only gave eight results and has dropped one, which highlights the need for guardrails and structured output._\n\n\n## Summary\n\nIn this post and the accompanying [video](https://www.youtube.com/watch?v=bxE0_QYX_sU) and [notebook](https://github.com/milvus-io/bootcamp/blob/master/bootcamp/tutorials/quickstart/multimodal_retrieval_amazon_reviews.ipynb), we have constructed an application for multimodal semantic search across text and images. The embedding model was able to embed text and images jointly or separately into the same space, and the foundation model was able to input text and image while generating text in response. _Importantly, the embedding model was able to relate the user’s intent of an open-ended instruction to the query image and in that way specify how the user wanted the results to relate to the input image._\n\nThis is just a taste of what is to come in the near future. We will see many applications of multimodal search, multimodal understanding and reasoning, and so on across diverse modalities: image, video, audio, molecules, social networks, tabular data, time-series, the potential is boundless.\n\nAnd at the core of these systems is a vector database holding the system’s external “memory”. Milvus is an excellent choice for this purpose. It is open-source, fully featured (see [this article on full-text search in Milvus 2.5](https://milvus.io/blog/get-started-with-hybrid-semantic-full-text-search-with-milvus-2-5.md)) and scales efficiently to the billions of vectors with web-scale traffic and sub-100ms latency. Find out more at the [Milvus docs](https://milvus.io/docs), join our [Discord](https://milvus.io/discord) community, and hope to see you at our next [Unstructured Data meetup](https://lu.ma/unstructured-data-meetup). Until then!\n\n\n## Resources\n\n- Notebook: [“Multimodal Search with Amazon Reviews and LLVM Reranking](https://github.com/milvus-io/bootcamp/blob/master/bootcamp/tutorials/quickstart/multimodal_retrieval_amazon_reviews.ipynb)”\n\n- [Youtube AWS Developers video](https://www.youtube.com/watch?v=bxE0_QYX_sU)\n\n- [Milvus documentation](https://milvus.io/docs)\n\n- [Unstructured Data meetup](https://lu.ma/unstructured-data-meetup)\n\n- Embedding model: [Visualized BGE model card](https://huggingface.co/BAAI/bge-visualized)\n\n- Alt. embedding model: [MagicLens model repo](https://github.com/google-deepmind/magiclens)\n\n- LLVM: [Phi-3 Vision model card](https://huggingface.co/microsoft/Phi-3-vision-128k-instruct)\n\n- Paper: “[MagicLens: Self-Supervised Image Retrieval with Open-Ended Instructions](https://arxiv.org/abs/2403.19651)”\n\n- Dataset: [Amazon Reviews 2023](https://amazon-reviews-2023.github.io/)\n\n","title":"Multimodal Semantic Search with Images and Text","metaData":{}},{"id":"how-to-get-started-with-milvus.md","author":"Ruben Winastwan","tags":["Engineering"],"recommend":false,"canonicalUrl":"https://milvus.io/blog/how-to-get-started-with-milvus.md","date":"2025-01-17T00:00:00.000Z","cover":"https://assets.zilliz.com/How_To_Get_Started_With_Milvus_20230517_084248_28560b1efc.png","href":"/blog/how-to-get-started-with-milvus.md","content":"\n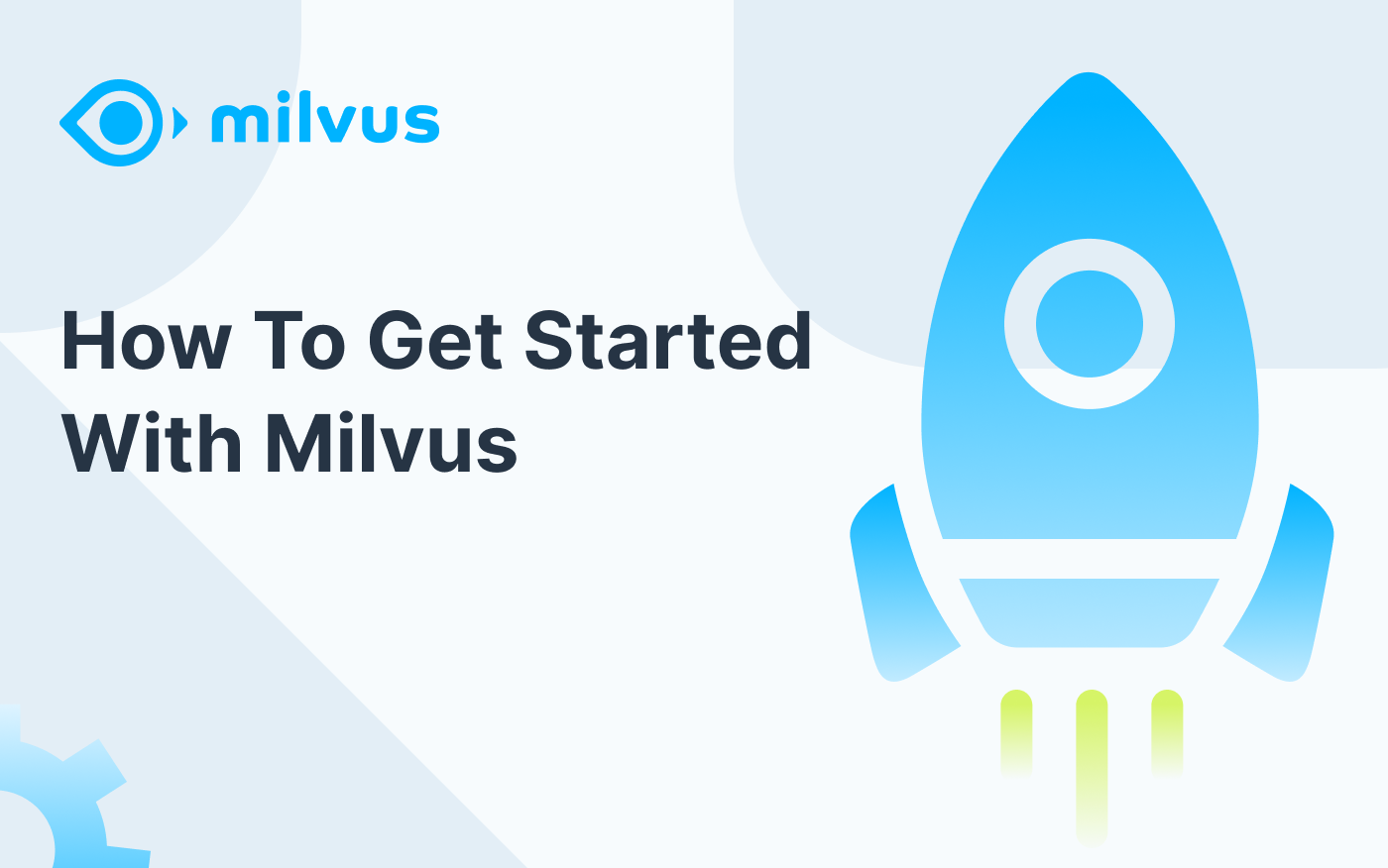\n\n**_Last updated January 2025_**\n\nThe advancements in Large Language Models ([LLMs](https://zilliz.com/glossary/large-language-models-(llms))) and the increasing volume of data necessitate a flexible and scalable infrastructure to store massive amounts of information, such as a database. However, [traditional databases](https://zilliz.com/blog/relational-databases-vs-vector-databases) are designed to store tabular and structured data, while the information commonly useful for leveraging the power of sophisticated LLMs and information retrieval algorithms is [unstructured](https://zilliz.com/learn/introduction-to-unstructured-data), such as text, images, videos, or audio.\n\n[Vector databases](https://zilliz.com/learn/what-is-vector-database) are database systems specifically designed for unstructured data. Not only can we store massive amounts of unstructured data with vector databases, but we can also perform [vector searches](https://zilliz.com/learn/vector-similarity-search) with them. Vector databases have advanced indexing methods such as Inverted File Index (IVFFlat) or Hierarchical Navigable Small World ([HNSW](https://zilliz.com/learn/hierarchical-navigable-small-worlds-HNSW)) to perform fast and efficient vector search and information retrieval processes.\n\n**Milvus** is an open-source vector database that we can use to leverage all of the beneficial features a vector database can offer. Here are what we’ll cover in this post: \n\n- [An Overview of Milvus](https://milvus.io/blog/how-to-get-started-with-milvus.md#What-is-Milvus)\n\n- [Milvus deployment options](https://milvus.io/blog/how-to-get-started-with-milvus.md#Milvus-Deployment-Options) \n\n- [Getting started with Milvus Lite](https://milvus.io/blog/how-to-get-started-with-milvus.md#Getting-Started-with-Milvus-Lite)\n\n- [Getting started with Milvus Standalone](https://milvus.io/blog/how-to-get-started-with-milvus.md#Getting-Started-with-Milvus-Standalone)\n\n- [Fully Managed Milvus ](https://milvus.io/blog/how-to-get-started-with-milvus.md#Fully-Managed-Milvus)\n\n\n## What is Milvus?\n\n[**Milvus** ](https://milvus.io/docs/overview.md)is an open-source vector database that enables us to store massive amounts of unstructured data and perform fast and efficient vector searches on them. Milvus is highly useful for many popular GenAI applications, such as recommendation systems, personalized chatbots, anomaly detection, image search, natural language processing, and retrieval augmented generation ([RAG](https://zilliz.com/learn/Retrieval-Augmented-Generation)).\n\nThere are several advantages that you can get by using Milvus as a vector database:\n\n- Milvus offers multiple deployment options that you can choose from depending on your use case and the size of the applications you want to build.\n\n- Milvus supports a diverse array of indexing methods to meet various data and performance needs, including in-memory options like FLAT, IVFFlat, HNSW, and [SCANN](https://zilliz.com/learn/what-is-scann-scalable-nearest-neighbors-google), quantized variants for memory efficiency, the on-disk [DiskANN](https://zilliz.com/learn/DiskANN-and-the-Vamana-Algorithm) for large datasets, and GPU-optimized indexes such as GPU_CAGRA, GPU_IVF_FLAT, and GPU_IVF_PQ for accelerated, memory-efficient searches. \n\n- Milvus also offers hybrid search, where we can use a combination of dense embeddings, sparse embeddings, and metadata filtering during vector search operations, leading to more accurate retrieval results. Additionally, [Milvus 2.5](https://milvus.io/blog/introduce-milvus-2-5-full-text-search-powerful-metadata-filtering-and-more.md) now supports a hybrid [full-text search](https://milvus.io/blog/get-started-with-hybrid-semantic-full-text-search-with-milvus-2-5.md) and vector search, making your retrieval even more accurate. \n\n- Milvus can be fully used on the cloud via [Zilliz Cloud](https://zilliz.com/cloud), where you can optimize its operational costs and vector search speed due to four advanced features: logical clusters, streaming and historical data disaggregation, tiered storage, autoscaling, and multi-tenancy hot-cold separation. \n\nWhen using Milvus as your vector database, you can choose three different deployment options, each with its strengths and benefits. We’ll talk about each of them in the next section.\n\n\n## Milvus Deployment Options\n\nWe can choose from four deployment options to start using Milvus: **Milvus Lite, Milvus Standalone, Milvus Distributed, and Zilliz Cloud (managed Milvus).** Each deployment option is designed to suit various scenarios in our use case, such as the size of our data, the purpose of our application, and the scale of our application.\n\n\n### Milvus Lite\n\n[**Milvus Lite**](https://milvus.io/docs/quickstart.md) is a lightweight version of Milvus and the easiest way for us to get started. In the next section, we'll see how we can run Milvus Lite in action, and all we need to do to get started is to install the Pymilvus library with pip. After that, we can perform most of the core functionalities of Milvus as a vector database. \n\nMilvus Lite is perfect for quick prototyping or learning purposes and can be run in a Jupyter notebook without any complicated setup. In terms of vector storage, Milvus Lite is suitable for storing roughly up to a million vector embeddings. Due to its lightweight feature and storage capacity, Milvus Lite is a perfect deployment option for working with edge devices, such as private documents search engine, on-device object detection, etc.\n\n\n### Milvus Standalone\n\nMilvus Standalone is a single-machine server deployment packed in a Docker image. Therefore, all we need to do to get started is to install Milvus in Docker, and then start the Docker container. We'll also see the detailed implementation of Milvus Standalone in the next section.\n\nMilvus Standalone is ideal for building and productionizing small to medium-scale applications, as it's able to store up to 10M vector embeddings. Additionally, Milvus Standalone offers high availability through a primary backup mode, making it highly dependable for use in production-ready applications. \n\nWe can also use Milvus Standalone, for example, after performing quick prototyping and learning Milvus functionalities with Milvus Lite, as both Milvus Standalone and Milvus Lite share the same client-side API.\n\n\n### Milvus Distributed\n\nMilvus Distributed is a deployment option that leverages a cloud-based architecture, where data ingestion and retrieval are handled separately, allowing for a highly scalable and efficient application.\n\nTo run Milvus Distributed, we typically need to use a Kubernetes cluster to allow the container to run on multiple machines and environments. The application of a Kubernetes cluster ensures the scalability and flexibility of Milvus Distributed in customizing the allocated resources depending on demand and workload. This also means that if one part fails, others can take over, ensuring the entire system remains uninterrupted.\n\nMilvus Distributed is able to handle up to tens of billions of vector embeddings and is specially designed for use cases where the data are too big to be stored in a single server machine. Therefore, this deployment option is perfect for Enterprise clients that serve a large user base.\n\n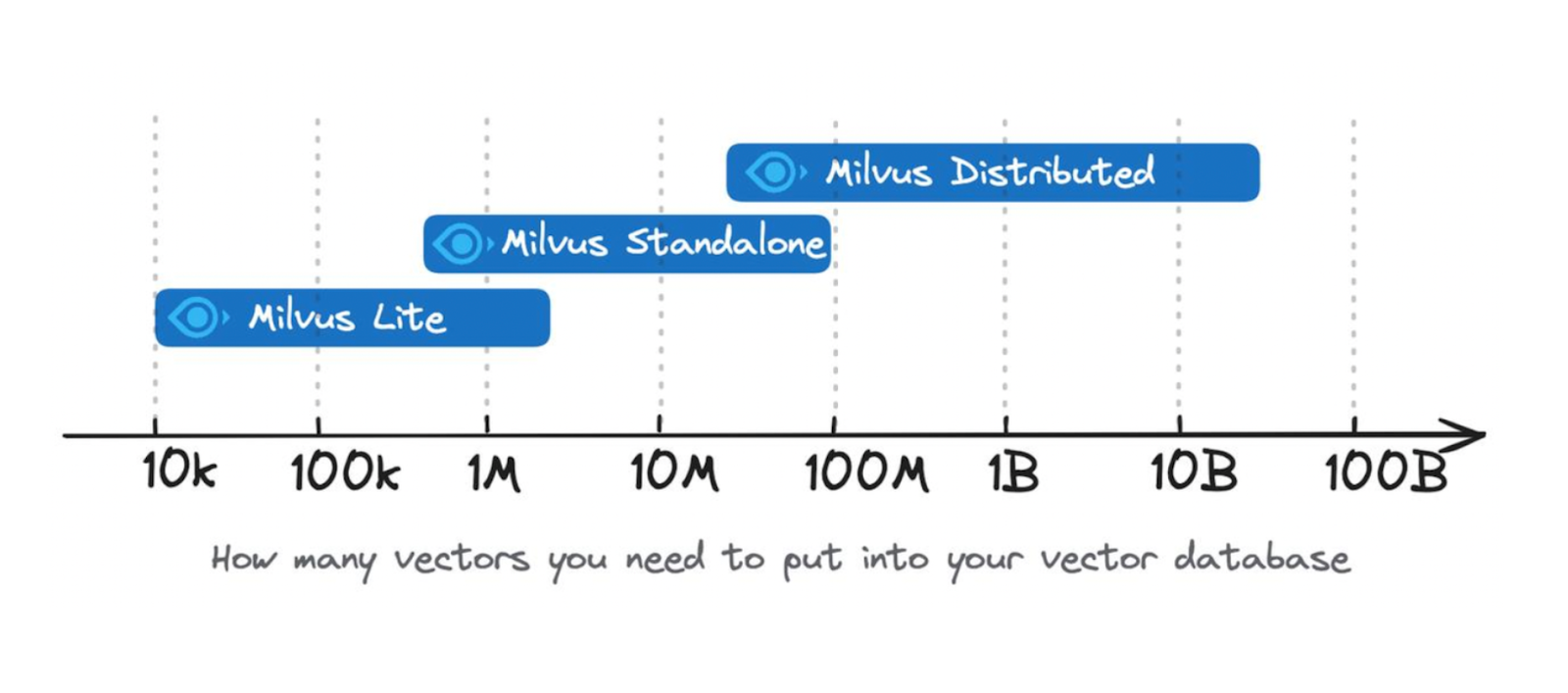\n\n_Figure: Vector embedding storage capability of different Milvus deployment options._\n\nIn this article, we're going to show you how to get started with both Milvus Lite and Milvus Standalone, as you can get started quickly with both methods without complicated setup. Milvus Distributed, however, is more complicated to set up. Once we set Milvus Distributed up, the code and logical process to create collections, ingest data, perform vector search, etc. are similar to Milvus Lite and Milvus Standalone, as they share the same client-side API.\n\nIn addition to the three deployment options mentioned above, you can also try the managed Milvus on [Zilliz Cloud](https://zilliz.com/cloud) for a hassle-free experience. We'll also talk about Zilliz Cloud later in this article.\n\n\n## Getting Started with Milvus Lite\n\nMilvus Lite can be implemented straightaway with Python by importing a library called Pymilvus using pip. Before installing Pymilvus, ensure that your environment meets the following requirements:\n\n- Ubuntu \u003e= 20.04 (x86_64 and arm64)\n\n- MacOS \u003e= 11.0 (Apple Silicon M1/M2 and x86_64)\n\n- Python 3.7 or later\n\nOnce these requirements are fulfilled, you can install Milvus Lite and the necessary dependencies for demonstration using the following command:\n\n```\n!pip install -U pymilvus\n!pip install \"pymilvus[model]\"\n```\n\n- `!pip install -U pymilvus`: This command installs or upgrades the `pymilvus` library, the Python SDK of Milvus. Milvus Lite is bunded with PyMilvus, so this single line of code is all you need to install Milvus Lite. \n\n- `!pip install \"pymilvus[model]\"`: This command adds advanced features and extra tools pre-integrated with Milvus, including machine learning models like Hugging Face Transformers, Jina AI embedding models, and reranking models.\n\nHere are the steps we're going to follow with Milvus Lite:\n\n1. Transform text data into their embedding representation using an embedding model.\n\n2. Create a schema in our Milvus database to store our text data and their embedding representations.\n\n3. Store and index our data into our schema.\n\n4. Perform a simple vector search on the stored data.\n\n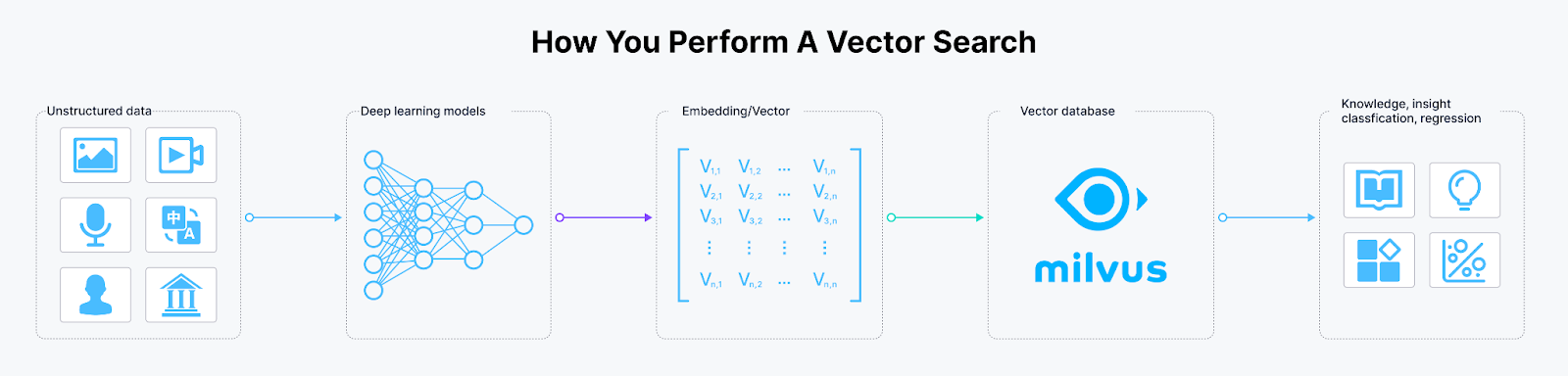\n\n_Figure: Workflow of vector search operation._\n\nTo transform text data into vector embeddings, we'll use an [embedding model](https://zilliz.com/ai-models) from SentenceTransformers called 'all-MiniLM-L6-v2'. This embedding model transforms our text into a 384-dimensional vector embedding. Let's load the model, transform our text data, and pack everything together.\n\n```python\nfrom pymilvus import model\n\ndocs = [\n \"Artificial intelligence was founded as an academic discipline in 1956.\",\n \"Alan Turing was the first person to conduct substantial research in AI.\",\n \"Born in Maida Vale, London, Turing was raised in southern England.\",\n]\n\nsentence_transformer_ef = model.dense.SentenceTransformerEmbeddingFunction(\n model_name='all-MiniLM-L6-v2', \n device='cpu' \n)\n\nvectors = sentence_transformer_ef.encode_documents(docs)\ndata = [ {\"id\": i, \"vector\": vectors[i], \"text\": docs[i]} for i in range(len(vectors)) ]\n```\n\n\nNext, let’s create a schema to store all of the data above into Milvus. As you can see above, our data consists of three fields: ID, vector, and text. Therefore, we’re going to create a schema with these three fields.\n\n```python\nfrom pymilvus import MilvusClient, DataType\n\nschema = MilvusClient.create_schema(\n auto_id=False,\n enable_dynamic_field=True,\n)\n\n# Add fields to schema\nschema.add_field(field_name=\"id\", datatype=DataType.INT64, is_primary=True)\nschema.add_field(field_name=\"vector\", datatype=DataType.FLOAT_VECTOR, dim=384)\nschema.add_field(field_name=\"text\", datatype=DataType.VARCHAR, max_length=512)\n```\n\n\nWith Milvus Lite, we can easily create a collection on a particular database based on the schema defined above, as well as inserting and indexing the data into the collection in just a few lines of code.\n\n```python\nclient = MilvusClient(\"./milvus_demo.db\")\n\nindex_params = client.prepare_index_params()\n\n# Add indexes\nindex_params.add_index(\n field_name=\"vector\", \n index_type=\"AUTOINDEX\",\n metric_type=\"COSINE\"\n)\n\n# Create collection\nclient.create_collection(\n collection_name=\"demo_collection\",\n schema=schema,\n index_params=index_params\n)\n\n# Insert data into collection\nres = client.insert(\n collection_name=\"demo_collection\",\n data=data\n)\n```\n\n\nIn the code above, we create a collection called \"demo_collection\" inside a Milvus database named \"milvus_demo\". Next, we index all of our data into the \"demo_collection\" that we just created.\n\nNow that we have our data inside the database, we can perform a vector search on them for any given query. Let's say we have a query: \"_Who is Alan Turing?_\". We can get the most appropriate answer to the query by implementing the following steps:\n\n1. Transform our query into a vector embedding using the same embedding model that we used to transform our data in the database into embeddings.\n\n2. Calculate the similarity between our query embedding and the embedding of each entry in the database using metrics like cosine similarity or Euclidean distance.\n\n3. Fetch the most similar entry as the appropriate answer to our query.\n\nBelow is the implementation of the above steps with Milvus:\n\n```python\nquery = [\"Who is Alan Turing\"]\nquery_embedding = sentence_transformer_ef.encode_queries(query)\n\n# Load collection\nclient.load_collection(\n collection_name=\"demo_collection\"\n)\n\n# Vector search\nres = client.search(\n collection_name=\"demo_collection\",\n data=query_embedding,\n limit=1,\n output_fields=[\"text\"],\n)\nprint(res)\n\"\"\"\nOutput:\ndata: [\"[{'id': 1, 'distance': 0.7199002504348755, 'entity': {'text': 'Alan Turing was the first person to conduct substantial research in AI.'}}]\"] \n\"\"\"\n```\n\n\nAnd that's it! You can also learn more about other functionalities that Milvus offers, such as managing databases, inserting and deleting collections, choosing the right indexing method, and performing more advanced vector searches with metadata filtering and hybrid search in [Milvus documentation](https://milvus.io/docs/).\n\n\n## Getting Started with Milvus Standalone\n\nMilvus Standalone is a deployment option in which everything is packed in a Docker container. Therefore, we need to install Milvus in Docker and then start the Docker container to get started with Milvus Standalone.\n\nBefore installing Milvus Standalone, make sure that both your hardware and software fulfill the requirements described on [this page](https://milvus.io/docs/prerequisite-docker.md). Also, ensure that you've installed Docker. To install Docker, refer to [this page](https://docs.docker.com/get-started/get-docker/).\n\nOnce our system fulfills the requirements and we have installed Docker, we can proceed with Milvus installation in Docker using the following command:\n\n```shell\n# Download the installation script\n$ curl -sfL \u003chttps://raw.githubusercontent.com/milvus-io/milvus/master/scripts/standalone_embed.sh\u003e -o standalone_embed.sh\n\n# Start the Docker container\n$ bash standalone_embed.sh start\n```\n\n\nIn the above code, we also start the Docker container and once it’s started, you’ll get similar output as below:\n\n\n\n_Figure: Message after successful starting of the Docker container._\n\nAfter running the installation script “standalone_embed.sh” above, a Docker container named “milvus” is started at port 19530. Therefore, we can create a new database as well as access all the things related to the Milvus database by pointing to this port when initiating the client. \n\nLet’s say we want to create a database called “milvus_demo”, similar to what we have done in Milvus Lite above. We can do so as follows:\n\n```python\nfrom pymilvus import MilvusClient\n\nclient = MilvusClient(\n uri=\"http://localhost:19530\",\n token=\"root:Milvus\",\n)\nclient.create_database(\"milvus_demo\")\n```\n\n\nNext, you can verify whether the newly created database called \"milvus_demo\" truly exists in your Milvus instance by accessing the [Milvus Web UI](https://milvus.io/docs/milvus-webui.md). As the name suggests, Milvus Web UI is a graphical user interface provided by Milvus to observe the statistics and metrics of the components, check the list and details of databases, collections, and configurations. You can access Milvus Web UI once you've started the Docker container above at http://127.0.0.1:9091/webui/.\n\nIf you access the above link, you'll see a landing page like this:\n\n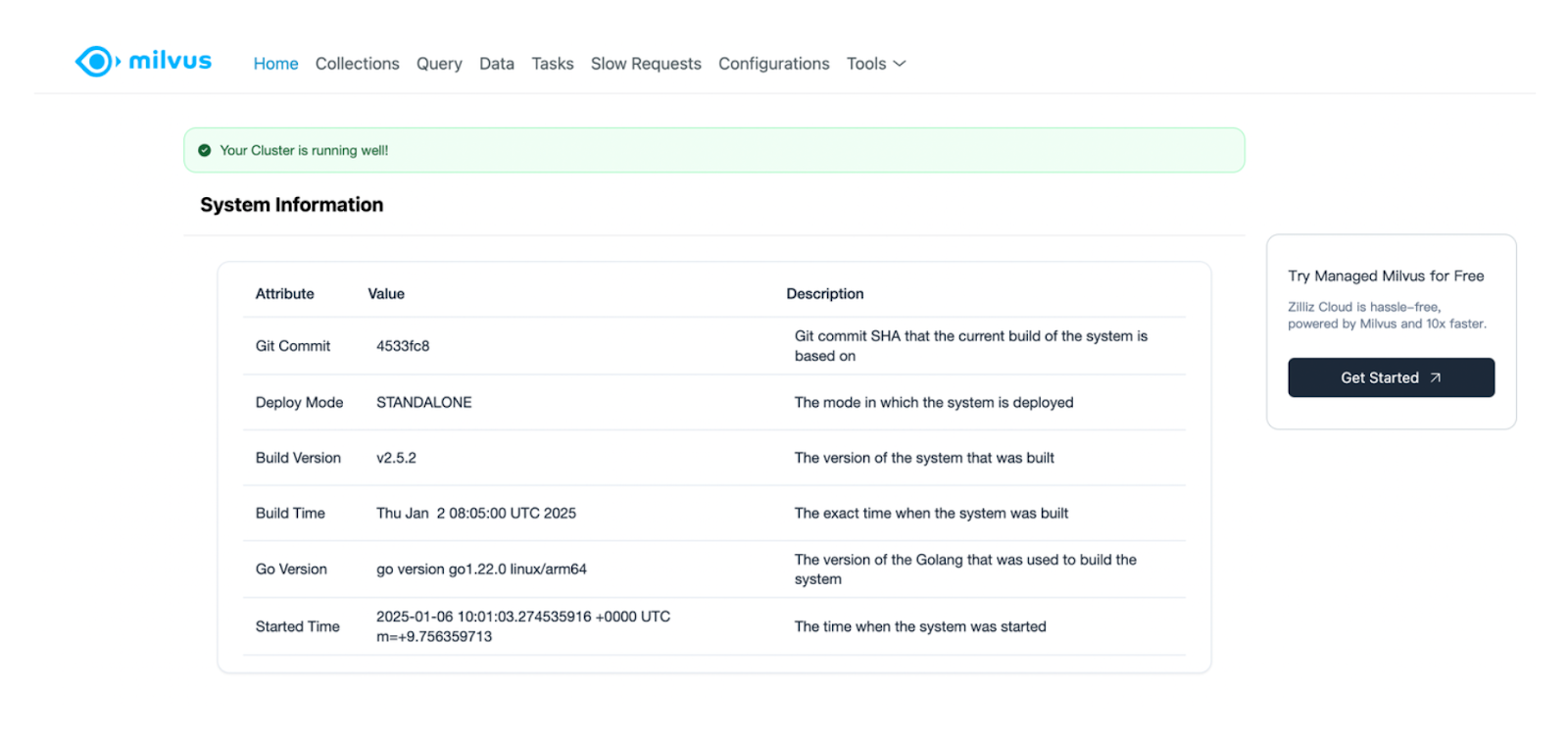\n\nUnder the \"Collections\" tab, you'll see that our \"milvus_demo\" database has been successfully created. As you can see, you can also check other things such as the list of collections, configurations, the queries you've performed, etc., with this Web UI.\n\n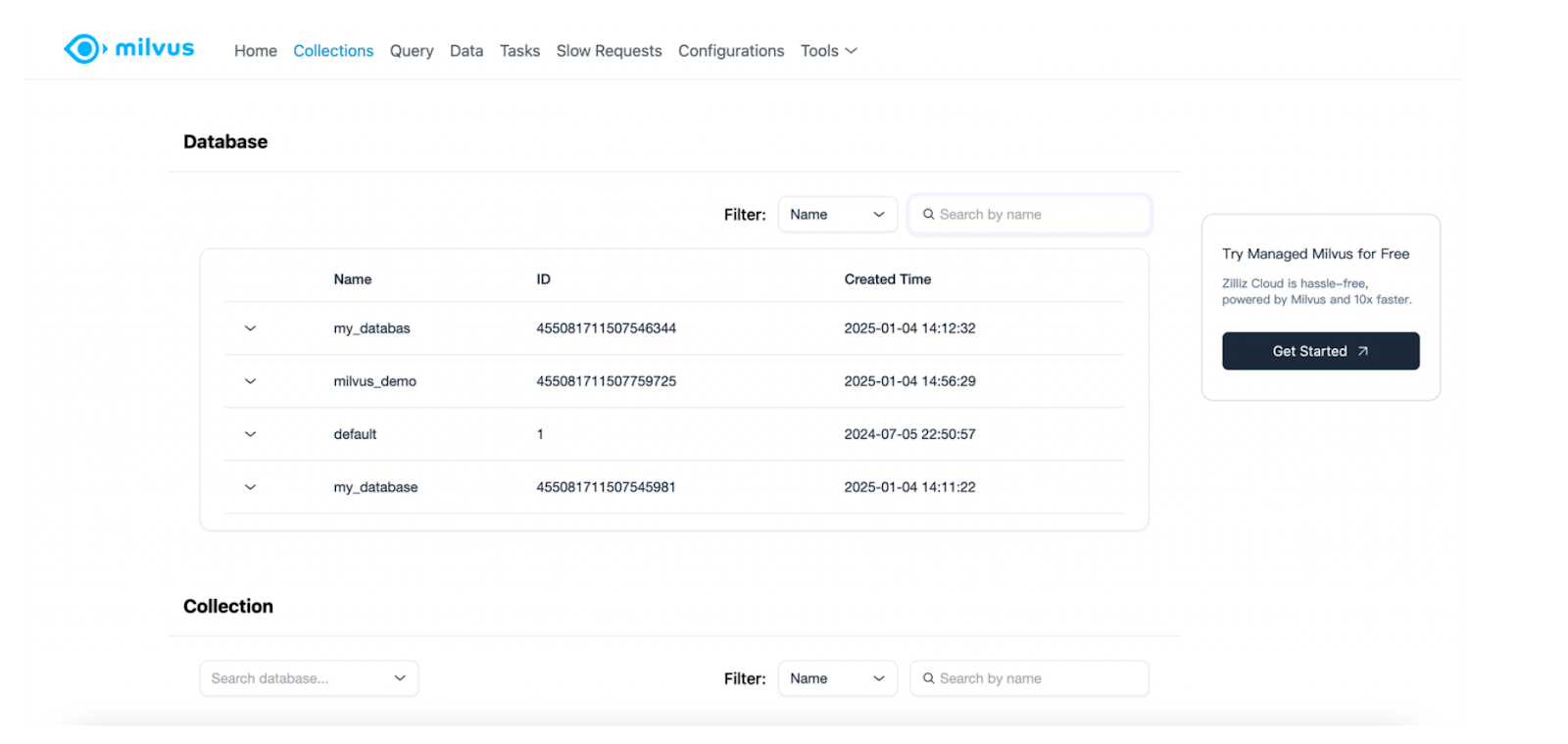\n\nNow we can perform everything exactly as we've seen in the Milvus Lite section above. Let's create a collection called \"demo_collection\" inside the \"milvus_demo\" database that consists of three fields, the same as what we had in the Milvus Lite section before. Then, we'll insert our data into the collection.\n\n\n```python\nindex_params = client.prepare_index_params()\n\n# Add indexes\nindex_params.add_index(\n field_name=\"vector\", \n index_type=\"AUTOINDEX\",\n metric_type=\"COSINE\"\n)\n\n# Create collection\nclient.create_collection(\n collection_name=\"demo_collection\",\n schema=schema,\n index_params=index_params\n)\n\n# Insert data into collection\nres = client.insert(\n collection_name=\"demo_collection\",\n data=data\n)\n```\n\nThe code to perform a vector search operation is also the same as Milvus Lite, as you can see in the below code:\n\n```python\nquery = [\"Who is Alan Turing\"]\nquery_embedding = sentence_transformer_ef.encode_queries(query)\n\n# Load collection\nclient.load_collection(\n collection_name=\"demo_collection\"\n)\n\n# Vector search\nres = client.search(\n collection_name=\"demo_collection\",\n data=query_embedding,\n limit=1,\n output_fields=[\"text\"],\n)\nprint(res)\n\"\"\"\nOutput:\ndata: [\"[{'id': 1, 'distance': 0.7199004292488098, 'entity': {'text': 'Alan Turing was the first person to conduct substantial research in AI.'}}]\"] \n\"\"\"\n```\n\nAside from using Docker, you can also use Milvus Standalone with [Docker Compose](https://milvus.io/docs/install_standalone-docker-compose.md) (for Linux) and [Docker Desktop](https://milvus.io/docs/install_standalone-windows.md) (for Windows).\n\nWhen we’re not using our Milvus instance anymore, we can stop Milvus Standalone with the following command:\n\n```shell\n$ bash standalone_embed.sh stop\n```\n\n\n\n## Fully Managed Milvus \n\nAn alternative way to get started with Milvus is through a native cloud-based infrastructure in [Zilliz Cloud](https://zilliz.com/cloud), where you can get a hassle-free, 10x faster experience. \n\nZilliz Cloud offers dedicated clusters with dedicated environments and resources to support your AI application. Since it is a cloud-based database built on Milvus, we do not need to set up and manage local infrastructure. Zilliz Cloud also provides more advanced features, such as separation between vector storage and computation, data backup to popular object storage systems like S3, and data caching to speed up vector search and retrieval operations.\n\nHowever, one thing to consider when considering cloud-based services is the operational cost. In most cases, we still need to pay even when the cluster is idle with no data ingestion or vector search activity. If you want to optimize your application's operational cost and performance further, Zilliz Cloud Serverless would be an excellent option.\n\n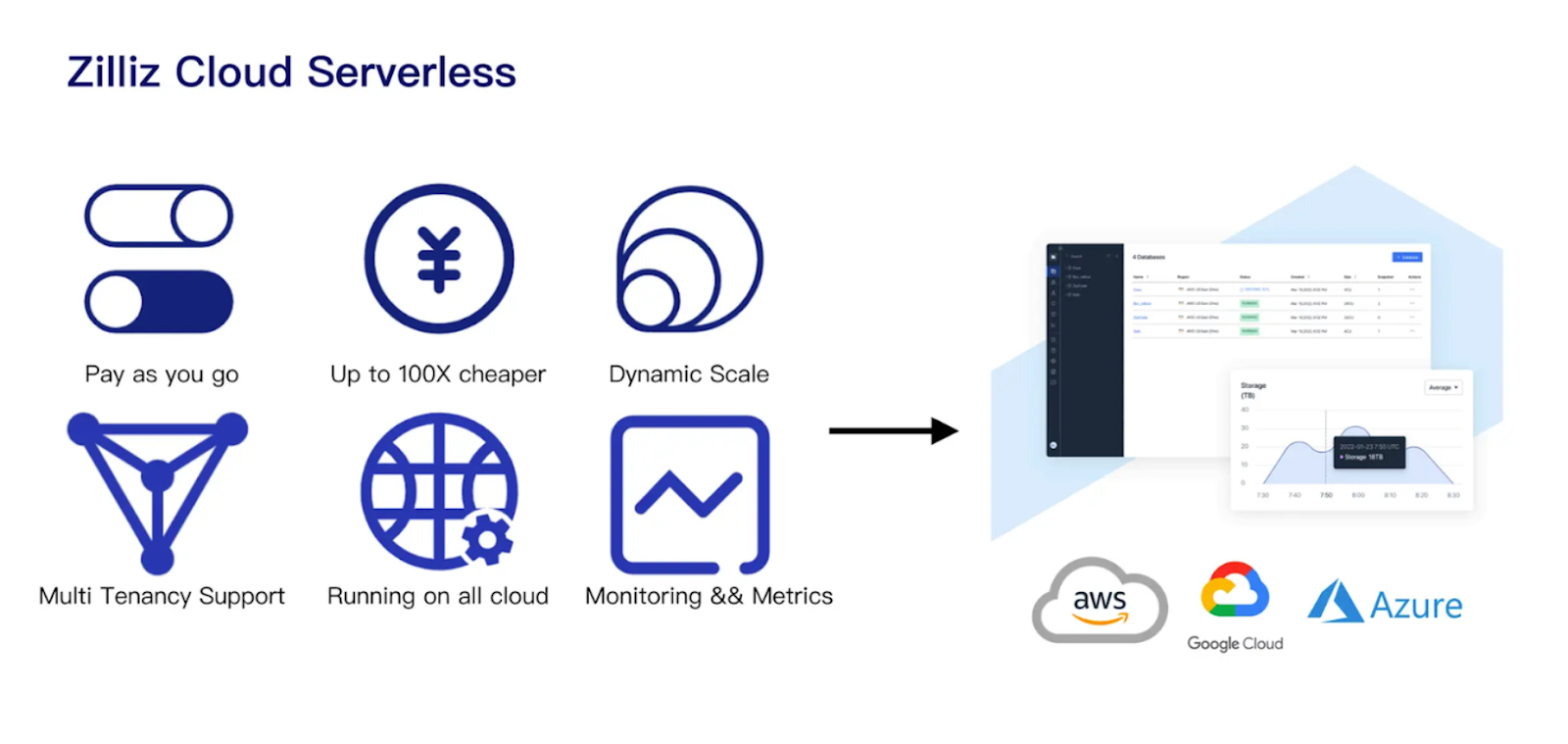\n\n_Figure: Key benefits of using Zilliz Cloud Serverless._\n\nZilliz Cloud Serverless is available on major cloud providers such as AWS, Azure, and GCP. It offers features like pay-as-you-go pricing, meaning you only pay when you use the cluster. \n\nZilliz Cloud Serverless also implements advanced technologies such as logical clusters, auto-scaling, tiered storage, disaggregation of streaming and historical data, and hot-cold data separation. These features enable Zilliz Cloud Serverless to achieve up to 50x cost savings and approximately 10x faster vector search operations compared to in-memory Milvus.\n\n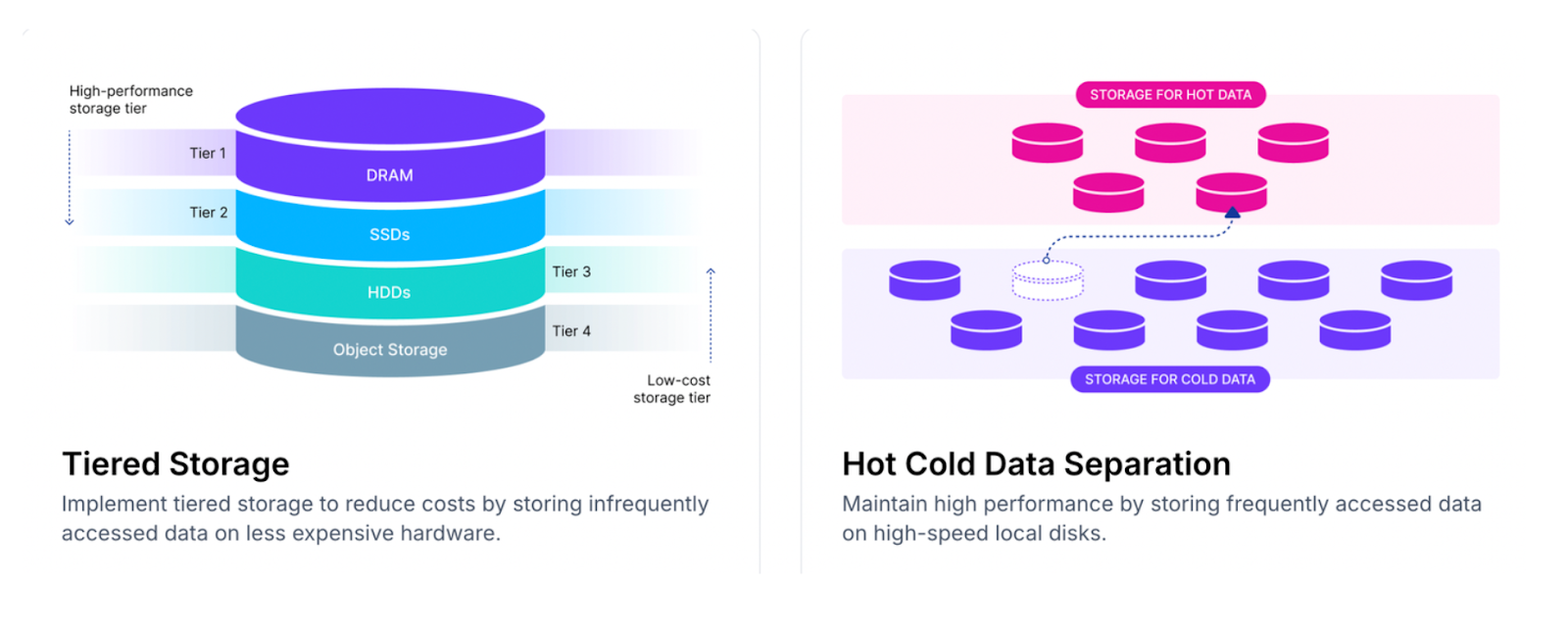\n\n_Figure: Illustration of tiered storage and hot-cold data separation._\n\nIf you'd like to get started with Zilliz Cloud Serverless, check out [this page](https://zilliz.com/serverless) for more information.\n\n\n## Conclusion\n\nMilvus stands out as a versatile and powerful vector database designed to meet the challenges of managing unstructured data and performing fast, efficient vector search operations in modern AI applications. With deployment options such as Milvus Lite for quick prototyping, Milvus Standalone for small to medium-scale applications, and Milvus Distributed for enterprise-level scalability, it offers flexibility to match any project's size and complexity.\n\nAdditionally, Zilliz Cloud Serverless extends Milvus's capabilities into the cloud and provides a cost-effective, pay-as-you-go model that eliminates the need for local infrastructure. With advanced features like tiered storage and auto-scaling, Zilliz Cloud Serverless ensures faster vector search operations while optimizing costs.\n","title":"How to Get Started with Milvus","metaData":{}},{"id":"elasticsearch-is-dead-long-live-lexical-search.md","author":"James Luan","tags":["Engineering"],"recommend":false,"canonicalUrl":"https://milvus.io/blog/elasticsearch-is-dead-long-live-lexical-search.md","date":"2024-12-17T00:00:00.000Z","cover":"https://assets.zilliz.com/Elasticsearch_is_Dead_Long_Live_Lexical_Search_0fa15cd6d7.png","href":"/blog/elasticsearch-is-dead-long-live-lexical-search.md","content":"\n\nBy now, everyone knows that hybrid search has improved [RAG](https://zilliz.com/learn/Retrieval-Augmented-Generation) (Retrieval-Augmented Generation) search quality. While [dense embedding](https://zilliz.com/learn/sparse-and-dense-embeddings) search has shown impressive capabilities in capturing deep semantic relationships between queries and documents, it still has notable limitations. These include a lack of explainability and suboptimal performance with long-tail queries and rare terms.\n\nMany RAG applications struggle because pre-trained models often lack domain-specific knowledge. In some scenarios, simple BM25 keyword matching outperforms these sophisticated models. This is where hybrid search bridges the gap, combining the semantic understanding of dense vector retrieval with the precision of keyword matching.\n\n\n## Why Hybrid Search is Complex in Production\n\nWhile frameworks like [LangChain](https://zilliz.com/learn/LangChain) or [LlamaIndex](https://zilliz.com/learn/getting-started-with-llamaindex) make building a proof-of-concept hybrid retriever easy, scaling to production with massive datasets is challenging. Traditional architectures require separate vector databases and search engines, leading to several key challenges:\n\n- High infrastructure maintenance costs and operational complexity\n\n- Data redundancy across multiple systems\n\n- Difficult data consistency management\n\n- Complex security and access control across systems\n\nThe market needs a unified solution that supports lexical and semantic search while reducing system complexity and cost.\n\n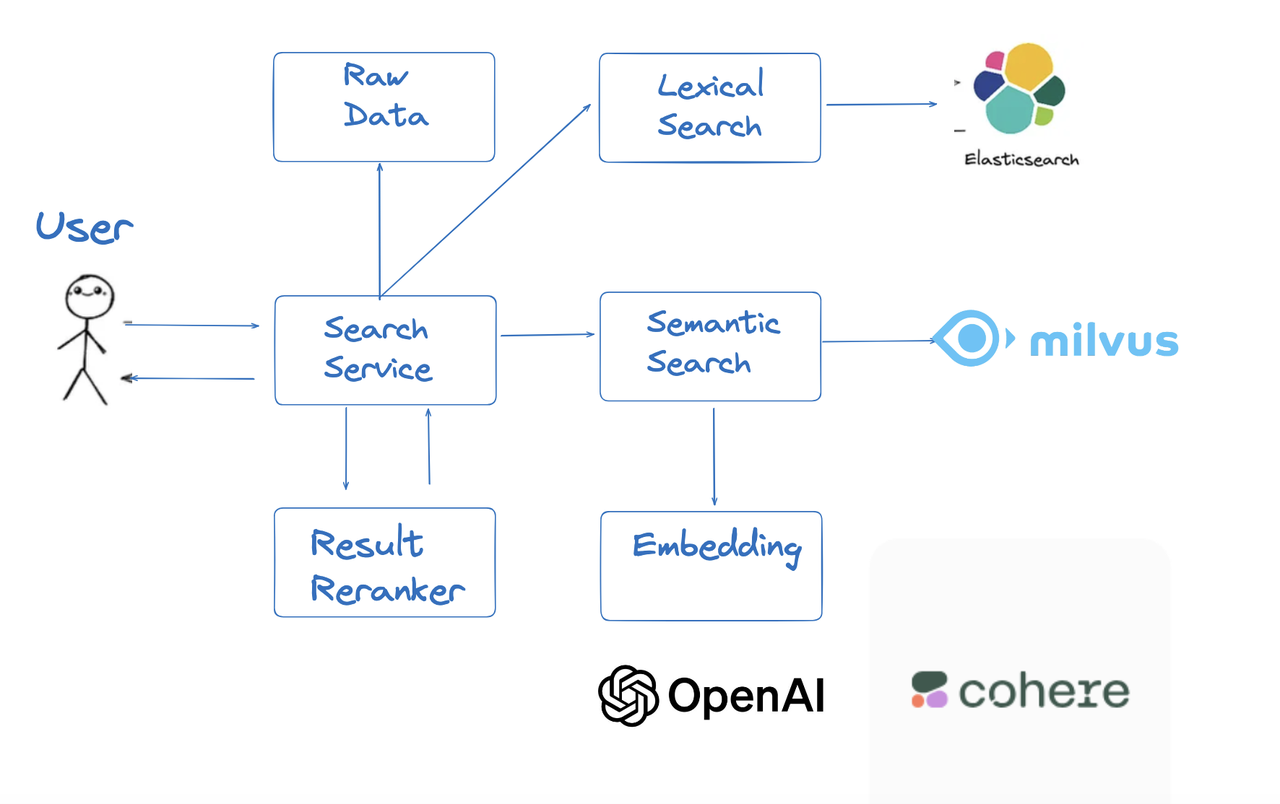\n\n\n## The Pain Points of Elasticsearch\n\nElasticsearch has been one of the past decade's most influential open-source search projects. Built on Apache Lucene, it gained popularity through its high performance, scalability, and distributed architecture. While it added vector ANN search in version 8.0, production deployments face several critical challenges:\n\n**High Update and Indexing Costs:** Elasticsearch's architecture doesn't fully decouple write operations, index building, and querying. This leads to significant CPU and I/O overhead during write operations, especially in bulk updates. The resource contention between indexing and querying impacts performance, creating a major bottleneck for high-frequency update scenarios.\n\n**Poor Real-time Performance:** As a \"near real-time\" search engine, Elasticsearch introduces noticeable latency in data visibility. This latency becomes particularly problematic for AI applications, such as Agent systems, where high-frequency interactions and dynamic decision-making require immediate data access.\n\n**Difficult Shard Management:** While Elasticsearch uses sharding for distributed architecture, shard management poses significant challenges. The lack of dynamic sharding support creates a dilemma: too many shards in small datasets lead to poor performance, while too few shards in large datasets limit scalability and cause uneven data distribution.\n\n**Non-Cloud-Native Architecture:** Developed before cloud-native architectures became prevalent, Elasticsearch's design tightly couples storage and compute, limiting its integration with modern infrastructure like public clouds and Kubernetes. Resource scaling requires simultaneous increases in both storage and compute, reducing flexibility. In multi-replica scenarios, each shard must build its index independently, increasing computational costs and reducing resource efficiency.\n\n**Poor Vector Search Performance:** Though Elasticsearch 8.0 introduced vector ANN search, its performance significantly lags behind that of dedicated vector engines like Milvus. Based on the Lucene kernel, its index structure proves inefficient for high-dimensional data, struggling with large-scale vector search requirements. Performance becomes particularly unstable in complex scenarios involving scalar filtering and multi-tenancy, making it challenging to support high-load or diverse business needs.\n\n**Excessive Resource Consumption:** Elasticsearch places extreme demands on memory and CPU, especially when processing large-scale data. Its JVM dependency requires frequent heap size adjustments and garbage collection tuning, severely impacting memory efficiency. Vector search operations require intensive SIMD-optimized computations, for which the JVM environment is far from ideal.\n\nThese fundamental limitations become increasingly problematic as organizations scale their AI infrastructure, making Elasticsearch particularly challenging for modern AI applications requiring high performance and reliability.\n\n\n## Introducing Sparse-BM25: Reimagining Lexical Search\n\n[Milvus 2.5](https://milvus.io/blog/introduce-milvus-2-5-full-text-search-powerful-metadata-filtering-and-more.md) introduces native lexical search support through Sparse-BM25, building upon the hybrid search capabilities introduced in version 2.4. This innovative approach includes the following key components:\n\n- Advanced tokenization and preprocessing via Tantivy\n\n- Distributed vocabulary and term frequency management\n\n- Sparse vector generation using corpus TF and query TF-IDF\n\n- Inverted index support with WAND algorithm (Block-Max WAND and graph index support in development)\n\nCompared to Elasticsearch, Milvus offers significant advantages in algorithm flexibility. Its vector distance-based similarity computation enables more sophisticated matching, including implementing TW-BERT (Term Weighting BERT) based on \"End-to-End Query Term Weighting\" research. This approach has demonstrated superior performance in both in-domain and out-domain testing.\n\nAnother crucial advantage is cost efficiency. By leveraging both inverted index and dense embedding compression, Milvus achieves a fivefold performance improvement with less than 1% recall degradation. Through tail-term pruning and vector quantization, memory usage has been reduced by over 50%.\n\nLong query optimization stands out as a particular strength. Where traditional WAND algorithms struggle with longer queries, Milvus excels by combining sparse embeddings with graph indices, delivering a tenfold performance improvement in high-dimensional sparse vector search scenarios.\n\n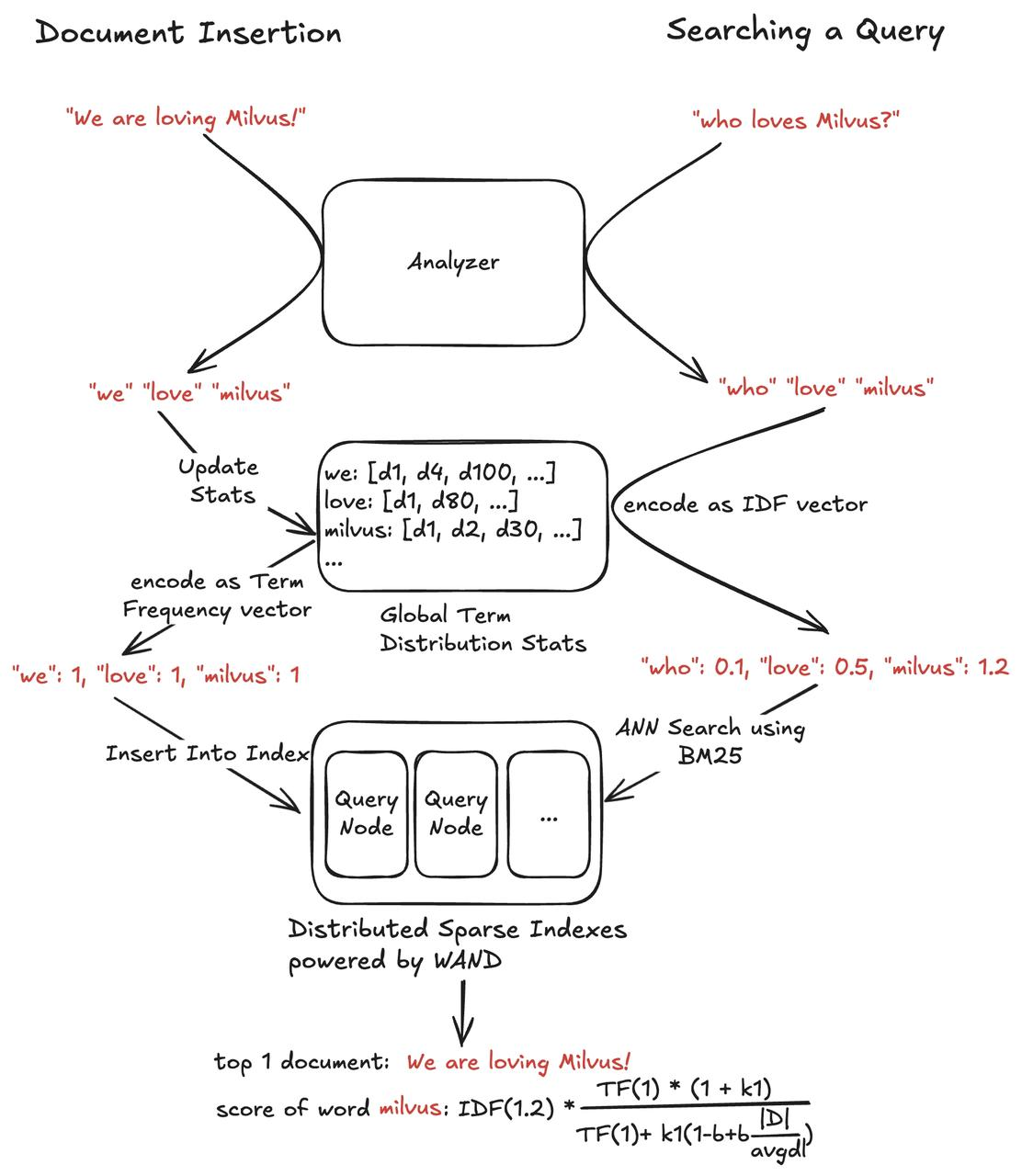\n\n\n## Milvus: The Ultimate Vector Database for RAG\n\nMilvus is the premier choice for RAG applications through its comprehensive feature set. Key advantages include:\n\n- Rich metadata support with dynamic schema capabilities and powerful filtering options\n\n- Enterprise-grade multi-tenancy with flexible isolation through collections, partitions, and partition keys\n\n- Industry-first disk vector index support with multi-tier storage from memory to S3\n\n- Cloud-native scalability supporting seamless scaling from 10M to 1B+ vectors\n\n- Comprehensive search capabilities, including grouping, range, and hybrid search\n\n- Deep ecosystem integration with LangChain, LlamaIndex, Dify, and other AI tools\n\nThe system's diverse search capabilities encompass grouping, range, and hybrid search methodologies. Deep integration with tools like LangChain, LlamaIndex, and Dify, as well as support for numerous AI products, places Milvus at the center of the modern AI infrastructure ecosystem.\n\n\n## Looking Forward\n\nAs AI transitions from POC to production, Milvus continues to evolve. We focus on making vector search more accessible and cost-effective while enhancing search quality. Whether you're a startup or an enterprise, Milvus reduces the technical barriers to AI application development.\n\nThis commitment to accessibility and innovation has led us to another major step forward. While our open-source solution continues to serve as the foundation for thousands of applications worldwide, we recognize that many organizations need a fully managed solution that eliminates operational overhead.\n\n\n## Zilliz Cloud: The Managed Solution\n\nWe've built [Zilliz Cloud](https://zilliz.com/cloud), a fully managed vector database service based on Milvus, over the past three years. Through a cloud-native reimplementation of the Milvus protocol, it offers enhanced usability, cost efficiency, and security.\n\nDrawing from our experience maintaining the world's largest vector search clusters and supporting thousands of AI application developers, Zilliz Cloud significantly reduces operational overhead and costs compared to self-hosted solutions.\n\nReady to experience the future of vector search? Start your free trial today with up to $200 in credits, no credit card required.\n","title":"Elasticsearch is Dead, Long Live Lexical Search","metaData":{}},{"id":"get-started-with-hybrid-semantic-full-text-search-with-milvus-2-5.md","author":"Stefan Webb","tags":["Engineering"],"recommend":false,"canonicalUrl":"https://milvus.io/blog/get-started-with-hybrid-semantic-full-text-search-with-milvus-2-5.md","date":"2024-12-17T00:00:00.000Z","cover":"https://assets.zilliz.com/Full_Text_Search_with_Milvus_2_5_7ba74461be.png","href":"/blog/get-started-with-hybrid-semantic-full-text-search-with-milvus-2-5.md","content":"\nIn this article, we will show you how to quickly get up and running with the new full-text search feature and combine it with the conventional semantic search based on vector embeddings.\n\n\n\u003ciframe width=\"100%\" height=\"480\" src=\"https://www.youtube.com/embed/3bftbAjQF7Q\" title=\"Beyond Keywords: Hybrid Search with Milvus 2.5\" frameborder=\"0\" allow=\"accelerometer; autoplay; clipboard-write; encrypted-media; gyroscope; picture-in-picture; web-share\" referrerpolicy=\"strict-origin-when-cross-origin\" allowfullscreen\u003e\u003c/iframe\u003e\n\n\n## Requirement\n\nFirst, ensure you have installed Milvus 2.5:\n\n\n```\npip install -U pymilvus[model]\n```\n\nand have a running instance of Milvus Standalone (e.g. on your local machine) using the [installation instructions in the Milvus docs](https://milvus.io/docs/prerequisite-docker.md).\n\n## Building the Data Schema and Search Indices\n\nWe import the required classes and functions:\n\n```\nfrom pymilvus import MilvusClient, DataType, Function, FunctionType, model\n```\n\nYou may have noticed two new entries for Milvus 2.5, `Function` and `FunctionType`, which we will explain shortly.\n\nNext we open the database with Milvus Standalone, that is, locally, and create the data schema. The schema comprises an integer primary key, a text string, a dense vector of dimension 384, and a sparse vector (of unlimited dimensionality).\nNote that Milvus Lite does not currently support full-text search, only Milvus Standalone and Milvus Distributed.\n\n```\nclient = MilvusClient(uri=\"http://localhost:19530\")\n\nschema = client.create_schema()\n\nschema.add_field(field_name=\"id\", datatype=DataType.INT64, is_primary=True, auto_id=True)\nschema.add_field(field_name=\"text\", datatype=DataType.VARCHAR, max_length=1000, enable_analyzer=True)\nschema.add_field(field_name=\"dense\", datatype=DataType.FLOAT_VECTOR, dim=768),\nschema.add_field(field_name=\"sparse\", datatype=DataType.SPARSE_FLOAT_VECTOR)\n```\n\n```\n{'auto_id': False, 'description': '', 'fields': [{'name': 'id', 'description': '', 'type': \u003cDataType.INT64: 5\u003e, 'is_primary': True, 'auto_id': True}, {'name': 'text', 'description': '', 'type': \u003cDataType.VARCHAR: 21\u003e, 'params': {'max_length': 1000, 'enable_analyzer': True}}, {'name': 'dense', 'description': '', 'type': \u003cDataType.FLOAT_VECTOR: 101\u003e, 'params': {'dim': 768}}, {'name': 'sparse', 'description': '', 'type': \u003cDataType.SPARSE_FLOAT_VECTOR: 104\u003e}], 'enable_dynamic_field': False}\n```\n\nYou may have noticed the `enable_analyzer=True` parameter. This tells Milvus 2.5 to enable the lexical parser on this field and build a list of tokens and token frequencies, which are required for full-text search. The `sparse` field will hold a vector representation of the documentation as a bag-of-words produced from the parsing `text`.\n\nBut how do we connect the `text` and `sparse` fields, and tell Milvus how `sparse` should be calculated from `text`? This is where we need to invoke the `Function` object and add it to the schema:\n\n\n```\nbm25_function = Function(\n name=\"text_bm25_emb\", # Function name\n input_field_names=[\"text\"], # Name of the VARCHAR field containing raw text data\n output_field_names=[\"sparse\"], # Name of the SPARSE_FLOAT_VECTOR field reserved to store generated embeddings\n function_type=FunctionType.BM25,\n)\n\nschema.add_function(bm25_function)\n```\n\n\n```\n{'auto_id': False, 'description': '', 'fields': [{'name': 'id', 'description': '', 'type': \u003cDataType.INT64: 5\u003e, 'is_primary': True, 'auto_id': True}, {'name': 'text', 'description': '', 'type': \u003cDataType.VARCHAR: 21\u003e, 'params': {'max_length': 1000, 'enable_analyzer': True}}, {'name': 'dense', 'description': '', 'type': \u003cDataType.FLOAT_VECTOR: 101\u003e, 'params': {'dim': 768}}, {'name': 'sparse', 'description': '', 'type': \u003cDataType.SPARSE_FLOAT_VECTOR: 104\u003e, 'is_function_output': True}], 'enable_dynamic_field': False, 'functions': [{'name': 'text_bm25_emb', 'description': '', 'type': \u003cFunctionType.BM25: 1\u003e, 'input_field_names': ['text'], 'output_field_names': ['sparse'], 'params': {}}]}\n```\n\nThe abstraction of the `Function` object is more general than that of applying full-text search. In the future, it may be used for other cases where one field needs to be a function of another field. In our case, we specify that `sparse` is a function of `text` via the function `FunctionType.BM25`. `BM25` refers to a common metric in information retrieval used for calculating a query's similarity to a document (relative to a collection of documents).\n\nWe use the default embedding model in Milvus, which is [paraphrase-albert-small-v2](https://huggingface.co/GPTCache/paraphrase-albert-small-v2):\n\n\n```\nembedding_fn = model.DefaultEmbeddingFunction()\n```\n\nThe next step is to add our search indices. We have one for the dense vector and a separate one for the sparse vector. The index type is `SPARSE_INVERTED_INDEX` with `BM25` since full-text search requires a different search method than those for standard dense vectors.\n\n\n```\nindex_params = client.prepare_index_params()\n\nindex_params.add_index(\n field_name=\"dense\",\n index_type=\"AUTOINDEX\", \n metric_type=\"COSINE\"\n)\n\nindex_params.add_index(\n field_name=\"sparse\",\n index_type=\"SPARSE_INVERTED_INDEX\", \n metric_type=\"BM25\"\n)\n```\n\nFinally, we create our collection:\n\n```\nclient.drop_collection('demo')\nclient.list_collections()\n```\n\n```\n[]\n```\n\n```\nclient.create_collection(\n collection_name='demo', \n schema=schema, \n index_params=index_params\n)\n\nclient.list_collections()\n```\n\n\n```\n['demo']\n```\nAnd with that, we have an empty database set up to accept text documents and perform semantic and full-text searches!\n\n## Inserting Data and Performing Full-Text Search\n\nInserting data is no different than previous versions of Milvus:\n\n```\ndocs = [\n 'information retrieval is a field of study.',\n 'information retrieval focuses on finding relevant information in large datasets.',\n 'data mining and information retrieval overlap in research.'\n]\n\nembeddings = embedding_fn(docs)\n\nclient.insert('demo', [\n {'text': doc, 'dense': vec} for doc, vec in zip(docs, embeddings)\n])\n```\n\n```\n{'insert_count': 3, 'ids': [454387371651630485, 454387371651630486, 454387371651630487], 'cost': 0}\n```\n\nLet's first illustrate a full-text search before we move on to hybrid search:\n\n```\nsearch_params = {\n 'params': {'drop_ratio_search': 0.2},\n}\n\nresults = client.search(\n collection_name='demo', \n data=['whats the focus of information retrieval?'],\n output_fields=['text'],\n anns_field='sparse',\n limit=3,\n search_params=search_params\n)\n```\n\nThe search parameter `drop_ratio_search` refers to the proportion of lower-scoring documents to drop during the search algorithm.\n\nLet's view the results:\n\n```\nfor hit in results[0]:\n print(hit)\n```\n\n```\n{'id': 454387371651630485, 'distance': 1.3352930545806885, 'entity': {'text': 'information retrieval is a field of study.'}}\n{'id': 454387371651630486, 'distance': 0.29726022481918335, 'entity': {'text': 'information retrieval focuses on finding relevant information in large datasets.'}}\n{'id': 454387371651630487, 'distance': 0.2715056240558624, 'entity': {'text': 'data mining and information retrieval overlap in research.'}}\n```\n\n## Performing Hybrid Semantic and Full-Text Search\n\nLet's now combine what we've learned to perform a hybrid search that combines separate semantic and full-text searches with a reranker:\n\n```\nfrom pymilvus import AnnSearchRequest, RRFRanker\nquery = 'whats the focus of information retrieval?'\nquery_dense_vector = embedding_fn([query])\n\nsearch_param_1 = {\n \"data\": query_dense_vector,\n \"anns_field\": \"dense\",\n \"param\": {\n \"metric_type\": \"COSINE\",\n },\n \"limit\": 3\n}\nrequest_1 = AnnSearchRequest(**search_param_1)\n\nsearch_param_2 = {\n \"data\": [query],\n \"anns_field\": \"sparse\",\n \"param\": {\n \"metric_type\": \"BM25\",\n \"params\": {\"drop_ratio_build\": 0.0}\n },\n \"limit\": 3\n}\nrequest_2 = AnnSearchRequest(**search_param_2)\n\nreqs = [request_1, request_2]\n```\n\n```\nranker = RRFRanker()\n\nres = client.hybrid_search(\n collection_name=\"demo\",\n output_fields=['text'],\n reqs=reqs,\n ranker=ranker,\n limit=3\n)\nfor hit in res[0]:\n print(hit)\n```\n\n```\n{'id': 454387371651630485, 'distance': 0.032786883413791656, 'entity': {'text': 'information retrieval is a field of study.'}}\n{'id': 454387371651630486, 'distance': 0.032258063554763794, 'entity': {'text': 'information retrieval focuses on finding relevant information in large datasets.'}}\n{'id': 454387371651630487, 'distance': 0.0317460335791111, 'entity': {'text': 'data mining and information retrieval overlap in research.'}}\n```\n\nAs you may have noticed, this is no different than a hybrid search with two separate semantic fields (available since Milvus 2.4). The results are identical to full-text search in this simple example, but for larger databases and keyword specific searches hybrid search typically has higher recall.\n\n## Summary\n\nYou're now equipped with all the knowledge needed to perform full-text and hybrid semantic/full-text search with Milvus 2.5. See the following articles for more discussion on how full-text search works and why it is complementary to semantic search:\n\n- [Introducing Milvus 2.5: Full-Text Search, More Powerful Metadata Filtering, and Usability Improvements!](https://milvus.io/blog/introduce-milvus-2-5-full-text-search-powerful-metadata-filtering-and-more.md)\n- [Semantic Search v.s. Full-Text Search: Which Do I Choose in Milvus 2.5?](https://milvus.io/blog/semantic-search-vs-full-text-search-which-one-should-i-choose-with-milvus-2-5.md)\n","title":"Getting Started with Hybrid Semantic / Full-Text Search with Milvus 2.5","metaData":{}},{"id":"introduce-milvus-2-5-full-text-search-powerful-metadata-filtering-and-more.md","author":"Ken Zhang, Stefan Webb, Jiang Chen","tags":["Engineering"],"recommend":true,"canonicalUrl":"https://milvus.io/blog/introduce-milvus-2-5-full-text-search-powerful-metadata-filtering-and-more.md","date":"2024-12-17T00:00:00.000Z","cover":"https://assets.zilliz.com/Introducing_Milvus_2_5_e4968e1cdb.png","href":"/blog/introduce-milvus-2-5-full-text-search-powerful-metadata-filtering-and-more.md","content":"\n\n## Overview\n\nWe are thrilled to present the latest version of Milvus, 2.5, which introduces a powerful new capability: [full-text search](https://milvus.io/docs/full-text-search.md#Full-Text-Search), also known as lexical or keyword search. If you are new to search, full-text search allows you to find documents by searching for specific words or phrases within them, similar to how you search in Google. This complements our existing semantic search capabilities, which understand the meaning behind your search rather than just matching exact words.\n\nWe use the industry-standard BM25 metric for document similarity, and our implemention is based on sparse vectors, allowing for more efficient storage and retrieval. For those unfamiliar with the term, sparse vectors are a way to represent text where most values are zero, making them very efficient to store and process—imagine a huge spreadsheet where only a few cells contain numbers, and the rest are empty. This approach fits well into Milvus's product philosophy where the vector is the core search entity.\n\nAn additional noteworthy aspect of our implementation is the capability to insert and query text _directly_ rather than having users first manually convert text into sparse vectors. This takes Milvus one step closer towards fully processing unstructured data.\n\nBut this is just the beginning. With the release of 2.5, we updated the [Milvus product roadmap](https://milvus.io/docs/roadmap.md). In future product iterations of Milvus, our focus will be on evolving Milvus's capabilities in four key directions:\n\n- Streamlined unstructured data processing;\n- Better search quality and efficiency;\n- Easier data management;\n- Lowering costs through algorithmic and design advances\n\nOur aim is to build data infrastructure that can both efficiently store and effectively retrieve information in the AI era.\n\n\n## Full-text Search via Sparse-BM25\n\nAlthough semantic search typically has better contexual awareness and intent understanding, when a user needs to search for specific proper nouns, serial numbers, or a completely-matching phrase, full-text retrieval with keyword matching often produces more accurate results.\n\nTo illustrate this with an example:\n\n- Semantic search excels when you ask: \"Find documents about renewable energy solutions\"\n- Full-text search is better when you need: \"Find documents mentioning _Tesla Model 3 2024_\"\n\nIn our previous version (Milvus 2.4), users had to pre-process their text using a separate tool (the BM25EmbeddingFunction module to the PyMilvus) on their own machines before they could search it This approach had several limitations: it couldn't handle growing datasets well, required extra setup steps, and made the whole process more complicated than necessary. For the technically minded, the key limitations were that it could only work on a single machine; the vocabulary and other corpus statistics used for BM25 scoring couldn't be updated as the corpus changed; and converting text to vectors on the client side is less intuitive working withtext directly.\n\nMilvus 2.5 simplifies everything. Now you can work with your text directly:\n\n- Store your original text documents as they are\n- Search using natural language queries\n- Get results back in readable form\n\nBehind the scenes, Milvus handles all the complex vector conversions automatically making it easier to work with text data. This is what we call our \"Doc in, Doc out\" approach—you work with readable text, and we handle the rest.\n\n\n### Techical Implementation\n\nFor those interested in the technical details, Milvus 2.5 adds the full-text search capability through its built-in Sparse-BM25 implementation, including:\n\n- **A Tokenizer built on tantivy**: Milvus now integrates with the thriving tantivy ecosystem\n- **Capability to ingest and retrieve raw documents**: Support for direct ingestion and query of text data\n- **BM25 relevance scoring**: Internalize BM25 scoring, implemented based on sparse vector\n\nWe chose to work with the well-developed tantivy ecosystem and build the Milvus text tokenizer on tantivy. In the future, Milvus will support more tokenizers and expose the tokenization process to help users better understand the retrieval quality. We will also explore deep learning-based tokenizers and stemmer strategies to further optimize the performance of full-text search. Below is sample code for using and configuring the tokenizer:\n\n```Python\n# Tokenizer configuration\nschema.add_field(\n field_name='text',\n datatype=DataType.VARCHAR,\n max_length=65535,\n enable_analyzer=True, # Enable tokenizer on this column\n analyzer_params={\"type\": \"english\"}, # Configure tokenizer parameters, here we choose the english template, fine-grained configuration is also supported\n enable_match=True, # Build an inverted index for Text_Match\n)\n```\n\nAfter configuring the tokenizer in the collection schema, users can register the text to bm25 function via add_function method. This will run internally in the Milvus server. All subsequent data flows such as additions, deletions, modifications, and queries can be completed by operating on the raw text string, as opposed to the vector representation. See below code example for how to ingest text and conduct full-text search with the new API:\n\n```Python\n# Define the mapping relationship between raw text data and vectors on the schema\nbm25_function = Function(\n name=\"text_bm25_emb\",\n input_field_names=[\"text\"], # Input text field\n output_field_names=[\"sparse\"], # Internal mapping sparse vector field\n function_type=FunctionType.BM25, # Model for processing mapping relationship\n)\n\nschema.add_function(bm25_function)\n...\n# Support for raw text in/out\nMilvusClient.insert('demo', [\n {'text': 'Artificial intelligence was founded as an academic discipline in 1956.'},\n {'text': 'Alan Turing was the first person to conduct substantial research in AI.'},\n {'text': 'Born in Maida Vale, London, Turing was raised in southern England.'},\n])\n\nMilvusClient.search(\n collection_name='demo',\n data=['Who started AI research?'],\n anns_field='sparse',\n limit=3\n)\n```\n\nWe have adopted an implementation of BM25 relevance scoring that represents queries and documents as sparse vectors, called **Sparse-BM25**. This unlocks many optimizations based on sparse vector, such as:\n\nMilvus achieves hybrid search capabilities through its cutting-edge **Sparse-BM25 implementation**, which integrates full-text search into the vector database architecture. By representing term frequencies as sparse vectors instead of traditional inverted indexes, Sparse-BM25 enables advanced optimizations, such as **graph indexing**, **product quantization (PQ)**, and **scalar quantization (SQ)**. These optimizations minimize memory usage and accelerate search performance. Similar to inverted index approach, Milvus supports taking raw text as input and generating sparse vectors internally. This make it able to work with any tokenizer and grasp any word shown in the dynamicly changing corpus.\n\nAdditionally, heuristic-based pruning discards low-value sparse vectors, further enhancing efficiency without compromising accuracy. Unlike previous approach using sparse vector, it can adapt to a growing corpus, not the accuracy of BM25 scoring.\n\n1. Building graph indexes on the sparse vector, which performs better than inverted index on queries with long text as inverted index needs more steps to finish matching the tokens in the query;\n2. Leveraging approximation techniques to speed up search with only minor impact on retrieval quality, such as vector quantization and heuristic based pruning;\n3. Unifying the interface and data model for performing semantic search and full-text search, thus enhancing the user experience.\n\n```Python\n# Creating an index on the sparse column\nindex_params.add_index(\n field_name=\"sparse\",\n index_type=\"AUTOINDEX\", # Default WAND index\n metric_type=\"BM25\" # Configure relevance scoring through metric_type\n)\n\n# Configurable parameters at search time to speed up search\nsearch_params = {\n 'params': {'drop_ratio_search': 0.6}, # WAND search parameter configuration can speed up search\n}\n```\n\nIn summary, Milvus 2.5 has expanded its search capability beyond semantic search by introducing full-text retrieval, making it easier for users to build high quality AI applications. These are just initial steps in the space of Sparse-BM25 search and we anticipate that there will be further optimization measures to try in the future.\n\n\n## Text Matching Search Filters\n\nA second text search feature released with Milvus 2.5 is **Text Match**, which allows the user to filter the search to entries containing a specific text string. This feature is also built on the basis of tokenization and is activated with `enable_match=True`.\n\nIt is worth noting that with Text Match, the processing of the query text is based on the logic of OR after tokenization. For example, in the example below, the result will return all documents (using the 'text' field) that contain either 'vector' or 'database'.\n\n```Python\nfilter = \"TEXT_MATCH(text, 'vector database')\"\n```\n\nIf your scenario requires matching both 'vector' and 'database', then you need to write two separate Text Matches and overlay them with AND to achieve your goal.\n\n```Python\nfilter = \"TEXT_MATCH(text, 'vector') and TEXT_MATCH(text, 'database')\"\n```\n\n\n## Significant Enhancement in Scalar Filtering Performance\n\nOur emphasis on scalar filtering performance originates from our discovery that the combination of vector retrieval and metadata filtering can greatly improve query performance and accuracy in various scenarios. These scenarios range from image search applications such as corner case identification in autonomous driving to complex RAG scenarios in enterprise knowledge bases. Thus, it is highly suitable for enterprise users to implement in large-scale data application scenarios.\n\nIn practice, many factors like how much data you're filtering, how your data is organized, and how you're searching can affect performance. To address this, Milvus 2.5 introduces three new types of indexes—BitMap Index, Array Inverted Index, and the Inverted Index after tokenizing the Varchar text field. These new indexes can significantly improve performance in real-world use cases.\n\nSpecifically:\n\n1. **BitMap Index** can be used to accelerate tag filtering (common operators include in, array_contains, etc.), and is suitable for scenarios with fewer field category data (data cardinality). The principle is to determine whether a row of data has a certain value on a column, with 1 for yes and 0 for no, and then maintain a BitMap list. The following chart shows the performance test comparison we conducted based on a customer's business scenario. In this scenario, the data volume is 500 million, the data category is 20, different values have different distribution proportions (1%, 5%, 10%, 50%), and the performance under different filtering amounts also varies. With 50% filtering, we can achieve a 6.8-fold performance gain through BitMap Index. It's worth noting that as cardinality increases, compared to BitMap Index, Inverted Index will show more balanced performance. \n\n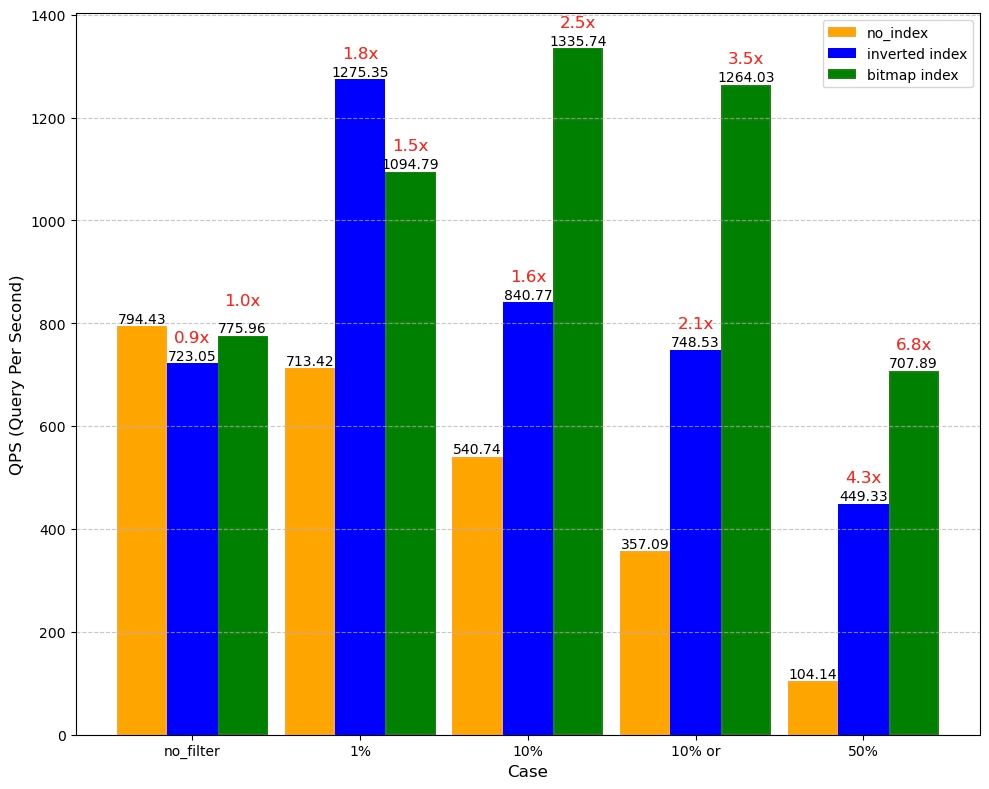\n\n\n2. **Text Match** is based on the Inverted Index after the text field is tokenized. Its performance far exceeds the Wildcard Match (i.e., like + %) function we provided in 2.4. According to our internal test results, the advantages of Text Match are very clear, especially in concurrent query scenarios, where it can achieve up to a 400-fold QPS increase. \n\n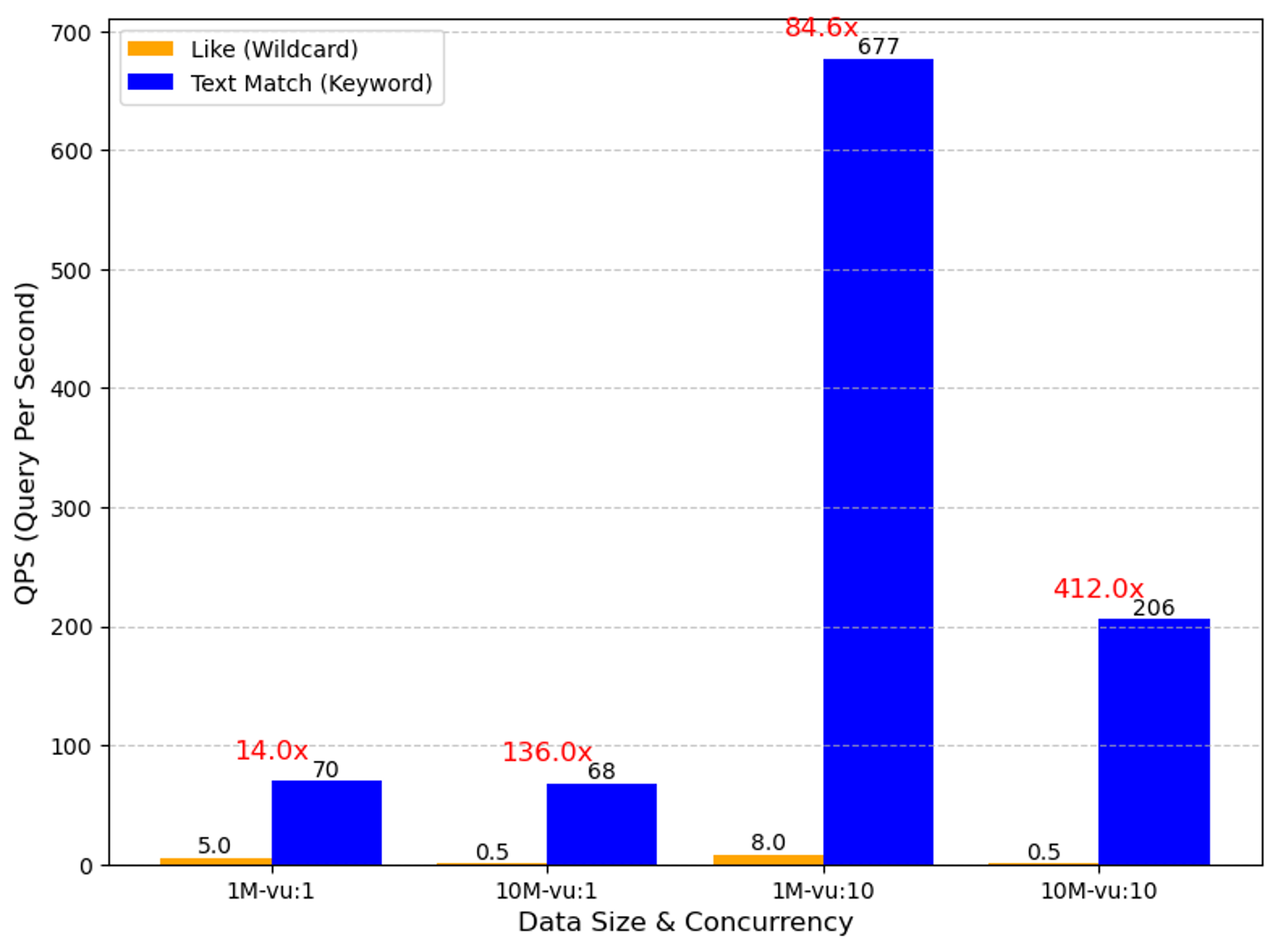\n\nIn terms of JSON data processing, we plan to introduce in subsequent versions of 2.5.x the building of inverted indices for user-specified keys and default location information recording for all keys to speed up parsing. We expect both of these areas to significantly enhance the query performance of JSON and Dynamic Field. We plan to showcase more information in future release notes and technical blogs, so stay tuned!\n\n\n## New Management Interface\n\nManaging a database shouldn't require a computer science degree, but we know database administrators need powerful tools. That's why we've introduced the **Cluster Management WebUI**, a new web-based interface accessible at your cluster's address on port 9091/webui. This observability tool provides:\n\n- Real-time monitoring dashboards showing cluster-wide metrics\n- Detailed memory and performance analytics per node\n- Segment information and slow query tracking\n- System health indicators and node status\n- Easy-to-use troubleshooting tools for complex system issues\n\nWhile this interface is still in beta, we're actively developing it based on user feedback from database administrators. Future updates will include AI-assisted diagnostics, more interactive management features, and enhanced cluster observability capabilities.\n\n\n## Documentation and Developer Experience\n\nWe've completely revamped our **documentation** and **SDK/API** experience to make Milvus more accessible while maintaining depth for experienced users. The improvements include:\n\n- A restructured documentation system with clearer progression from basic to advanced concepts\n- Interactive tutorials and real-world examples that showcase practical implementations\n- Comprehensive API references with practical code samples\n- A more user-friendly SDK design that simplifies common operations\n- Illustrated guides that make complex concepts easier to understand\n- An AI-powered documentation assistant (ASK AI) for quick answers\n\nThe updated SDK/API focuses on improving developer experience through more intuitive interfaces and better integration with the documentation. We believe you'll notice these improvements when working with the 2.5.x series.\n\nHowever, we know documentation and SDK development is an ongoing process. We'll continue optimizing both the content structure and SDK design based on community feedback. Join our Discord channel to share your suggestions and help us improve further.\n\n\n## **Summary**\n\nMilvus 2.5 contains 13 new features and several system-level optimizations, contributed not just by Zilliz but the open-source community. We have only touched on a few of them in this post and encourage you to visit our [release note](https://milvus.io/docs/release_notes.md) and [official documents](https://milvus.io/docs) for more information!\n","title":"Introducing Milvus 2.5: Full-Text Search, More Powerful Metadata Filtering, and Usability Improvements!","metaData":{}},{"id":"semantic-search-vs-full-text-search-which-one-should-i-choose-with-milvus-2-5.md","author":"David Wang, Jiang Chen","tags":["Engineering"],"recommend":false,"canonicalUrl":"https://milvus.io/blog/semantic-search-vs-full-text-search-which-one-should-i-choose-with-milvus-2-5.md","date":"2024-12-17T00:00:00.000Z","cover":"https://assets.zilliz.com/Semantic_Search_v_s_Full_Text_Search_5d93431c56.png","href":"/blog/semantic-search-vs-full-text-search-which-one-should-i-choose-with-milvus-2-5.md","content":"\n\nMilvus, a leading high-performance vector database, has long specialized in semantic search using vector embeddings from deep learning models. This technology powers AI applications like Retrieval-Augmented Generation (RAG), search engines, and recommender systems. With the rising popularity of RAG and other text search applications, the community has recognized the advantages of combining traditional text-matching methods with semantic search, known as hybrid search. This approach is particularly beneficial in scenarios that heavily rely on keyword matching. To address this need, Milvus 2.5 introduces full-text search (FTS) functionality and integrates it with the sparse vector search and hybrid search capabilities already available since version 2.4, creating a powerful synergy.\n\nHybrid search is a method that combines results from multiple search paths. Users can search different data fields in various ways, then merge and rank the results to obtain a comprehensive outcome. In popular RAG scenarios today, a typical hybrid approach combines semantic search with full-text search. Specifically, this involves merging results from dense embedding-based semantic search and BM25-based lexical matching using RRF (Reciprocal Rank Fusion) to enhance result ranking.\n\nIn this article, we will demonstrate this using a dataset provided by Anthropic, which consists of code snippets from nine code repositories. This resembles a popular use case of RAG: an AI-assisted coding bot. Because code data contains a lot of definitions, keywords, and other information, text-based search can be particularly effective in this context. Meanwhile, dense embedding models trained on large code datasets can capture higher-level semantic information. Our goal is to observe the effects of combining these two approaches through experimentation.\n\nWe will analyze specific cases to develop a clearer understanding of hybrid search. As the baseline, we will use an advanced dense embedding model (voyage-2) trained on a large volume of code data. We will then select examples where hybrid search outperforms both semantic and full-text search results (top 5) to analyze the characteristics behind these cases.\n\n| Method | Pass@5 |\n| :--------------------------: | :-----: |\n| Full-text Search | 0.7318 |\n| Semantic Search | 0.8096 |\n| Hybrid Search | 0.8176 |\n| Hybrid Search (add stopword) | 0.8418 |\n\nIn addition to analyzing the quality on a case-by-case basis, we broadened our evaluation by calculating the Pass@5 metric across the entire dataset. This metric measures the proportion of relevant results found in the top 5 results of each query. Our findings show that while advanced embedding models establish a solid baseline, integrating them with full-text search yields even better results. Further improvements are possible by examining BM25 results and fine-tuning parameters for specific scenarios, which can lead to significant performance gains.\n\n\n# Discussion\n\nWe examine the specific results retrieved for three different search queries, comparing semantic and full-text search to hybrid search. You can also check out [the full code in this repo](https://github.com/wxywb/milvus_fts_exps). \n\n\n## Case 1: **Hybrid Search Outperforms Semantic Search**\n\n**Query:** How is the log file created? \n\nThis query aims to inquire about creating a log file, and the correct answer should be a snippet of Rust code that creates a log file. In the semantic search results, we saw some code introducing the log header file and the C++ code for obtaining the logger. However, the key here is the \"logfile\" variable. In the hybrid search result #hybrid 0, we found this relevant result, which is naturally from the full-text search since hybrid search merges semantic and full-text search results.\n\nIn addition to this result, we can find unrelated test mock code in #hybrid 2, especially the repeated phrase, \"long string to test how those are handled.\" This requires understanding the principles behind the BM25 algorithm used in full-text search. Full-text search aims to match more infrequent words (since common words reduce the distinctiveness of the text and hinder object discrimination). Suppose we perform a statistical analysis on a large corpus of natural text. In that case, it is easy to conclude that \"how\" is a very common word and contributes very little to the relevance score. However, in this case, the dataset consists of code, and there aren't many occurrences of the word \"how\" in the code, making it a key search term in this context.\n\n**Ground Truth:** The correct answer is the Rust code that creates a log file.\n\n```C++\nuse {\n crate::args::LogArgs,\n anyhow::{anyhow, Result},\n simplelog::{Config, LevelFilter, WriteLogger},\n std::fs::File,\n};\n\npub struct Logger;\n\nimpl Logger {\n pub fn init(args: \u0026impl LogArgs) -\u003e Result\u003c()\u003e {\n let filter: LevelFilter = args.log_level().into();\n if filter != LevelFilter::Off {\n let logfile = File::create(args.log_file())\n .map_err(|e| anyhow!(\"Failed to open log file: {e:}\"))?;\n WriteLogger::init(filter, Config::default(), logfile)\n .map_err(|e| anyhow!(\"Failed to initalize logger: {e:}\"))?;\n }\n Ok(())\n }\n}\n```\n\n\n### Semantic Search Results\n\n```C++\n##dense 0 0.7745316028594971 \n/*\n * Licensed to the Apache Software Foundation (ASF) under one or more\n * contributor license agreements. See the NOTICE file distributed with\n * this work for additional information regarding copyright ownership.\n * The ASF licenses this file to You under the Apache License, Version 2.0\n * (the \"License\"); you may not use this file except in compliance with\n * the License. You may obtain a copy of the License at\n *\n * http://www.apache.org/licenses/LICENSE-2.0\n *\n * Unless required by applicable law or agreed to in writing, software\n * distributed under the License is distributed on an \"AS IS\" BASIS,\n * WITHOUT WARRANTIES OR CONDITIONS OF ANY KIND, either express or implied.\n * See the License for the specific language governing permissions and\n * limitations under the License.\n */\n#include \"logunit.h\"\n#include \u003clog4cxx/logger.h\u003e\n#include \u003clog4cxx/simplelayout.h\u003e\n#include \u003clog4cxx/fileappender.h\u003e\n#include \u003clog4cxx/helpers/absolutetimedateformat.h\u003e\n\n\n\n ##dense 1 0.769859254360199 \n void simple()\n {\n LayoutPtr layout = LayoutPtr(new SimpleLayout());\n AppenderPtr appender = FileAppenderPtr(new FileAppender(layout, LOG4CXX_STR(\"output/simple\"), false));\n root-\u003eaddAppender(appender);\n common();\n\n LOGUNIT_ASSERT(Compare::compare(LOG4CXX_FILE(\"output/simple\"), LOG4CXX_FILE(\"witness/simple\")));\n }\n\n std::string createMessage(int i, Pool \u0026 pool)\n {\n std::string msg(\"Message \");\n msg.append(pool.itoa(i));\n return msg;\n }\n\n void common()\n {\n int i = 0;\n\n // In the lines below, the logger names are chosen as an aid in\n // remembering their level values. In general, the logger names\n // have no bearing to level values.\n LoggerPtr ERRlogger = Logger::getLogger(LOG4CXX_TEST_STR(\"ERR\"));\n ERRlogger-\u003esetLevel(Level::getError());\n\n\n\n ##dense 2 0.7591114044189453 \n log4cxx::spi::LoggingEventPtr logEvt = std::make_shared\u003clog4cxx::spi::LoggingEvent\u003e(LOG4CXX_STR(\"foo\"),\n Level::getInfo(),\n LOG4CXX_STR(\"A Message\"),\n log4cxx::spi::LocationInfo::getLocationUnavailable());\n FMTLayout layout(LOG4CXX_STR(\"{d:%Y-%m-%d %H:%M:%S} {message}\"));\n LogString output;\n log4cxx::helpers::Pool pool;\n layout.format( output, logEvt, pool);\n\n\n\n ##dense 3 0.7562235593795776 \n#include \"util/compare.h\"\n#include \"util/transformer.h\"\n#include \"util/absolutedateandtimefilter.h\"\n#include \"util/iso8601filter.h\"\n#include \"util/absolutetimefilter.h\"\n#include \"util/relativetimefilter.h\"\n#include \"util/controlfilter.h\"\n#include \"util/threadfilter.h\"\n#include \"util/linenumberfilter.h\"\n#include \"util/filenamefilter.h\"\n#include \"vectorappender.h\"\n#include \u003clog4cxx/fmtlayout.h\u003e\n#include \u003clog4cxx/propertyconfigurator.h\u003e\n#include \u003clog4cxx/helpers/date.h\u003e\n#include \u003clog4cxx/spi/loggingevent.h\u003e\n#include \u003ciostream\u003e\n#include \u003ciomanip\u003e\n\n#define REGEX_STR(x) x\n#define PAT0 REGEX_STR(\"\\\\[[0-9A-FXx]*]\\\\ (DEBUG|INFO|WARN|ERROR|FATAL) .* - Message [0-9]\\\\{1,2\\\\}\")\n#define PAT1 ISO8601_PAT REGEX_STR(\" \") PAT0\n#define PAT2 ABSOLUTE_DATE_AND_TIME_PAT REGEX_STR(\" \") PAT0\n#define PAT3 ABSOLUTE_TIME_PAT REGEX_STR(\" \") PAT0\n#define PAT4 RELATIVE_TIME_PAT REGEX_STR(\" \") PAT0\n#define PAT5 REGEX_STR(\"\\\\[[0-9A-FXx]*]\\\\ (DEBUG|INFO|WARN|ERROR|FATAL) .* : Message [0-9]\\\\{1,2\\\\}\")\n\n\n ##dense 4 0.7557586431503296 \n std::string msg(\"Message \");\n\n Pool pool;\n\n // These should all log.----------------------------\n LOG4CXX_FATAL(ERRlogger, createMessage(i, pool));\n i++; //0\n LOG4CXX_ERROR(ERRlogger, createMessage(i, pool));\n i++;\n\n LOG4CXX_FATAL(INF, createMessage(i, pool));\n i++; // 2\n LOG4CXX_ERROR(INF, createMessage(i, pool));\n i++;\n LOG4CXX_WARN(INF, createMessage(i, pool));\n i++;\n LOG4CXX_INFO(INF, createMessage(i, pool));\n i++;\n\n LOG4CXX_FATAL(INF_UNDEF, createMessage(i, pool));\n i++; //6\n LOG4CXX_ERROR(INF_UNDEF, createMessage(i, pool));\n i++;\n LOG4CXX_WARN(INF_UNDEF, createMessage(i, pool));\n i++;\n LOG4CXX_INFO(INF_UNDEF, createMessage(i, pool));\n i++;\n\n LOG4CXX_FATAL(INF_ERR, createMessage(i, pool));\n i++; // 10\n LOG4CXX_ERROR(INF_ERR, createMessage(i, pool));\n i++;\n\n LOG4CXX_FATAL(INF_ERR_UNDEF, createMessage(i, pool));\n i++;\n LOG4CXX_ERROR(INF_ERR_UNDEF, createMessage(i, pool));\n i++;\n\n\n```\n\n\n### Hybrid Search Results\n\n```C++\n##hybrid 0 0.016393441706895828 \nuse {\n crate::args::LogArgs,\n anyhow::{anyhow, Result},\n simplelog::{Config, LevelFilter, WriteLogger},\n std::fs::File,\n};\n\npub struct Logger;\n\nimpl Logger {\n pub fn init(args: \u0026impl LogArgs) -\u003e Result\u003c()\u003e {\n let filter: LevelFilter = args.log_level().into();\n if filter != LevelFilter::Off {\n let logfile = File::create(args.log_file())\n .map_err(|e| anyhow!(\"Failed to open log file: {e:}\"))?;\n WriteLogger::init(filter, Config::default(), logfile)\n .map_err(|e| anyhow!(\"Failed to initalize logger: {e:}\"))?;\n }\n Ok(())\n }\n}\n\n \n##hybrid 1 0.016393441706895828 \n/*\n * Licensed to the Apache Software Foundation (ASF) under one or more\n * contributor license agreements. See the NOTICE file distributed with\n * this work for additional information regarding copyright ownership.\n * The ASF licenses this file to You under the Apache License, Version 2.0\n * (the \"License\"); you may not use this file except in compliance with\n * the License. You may obtain a copy of the License at\n *\n * http://www.apache.org/licenses/LICENSE-2.0\n *\n * Unless required by applicable law or agreed to in writing, software\n * distributed under the License is distributed on an \"AS IS\" BASIS,\n * WITHOUT WARRANTIES OR CONDITIONS OF ANY KIND, either express or implied.\n * See the License for the specific language governing permissions and\n * limitations under the License.\n */\n#include \"logunit.h\"\n#include \u003clog4cxx/logger.h\u003e\n#include \u003clog4cxx/simplelayout.h\u003e\n#include \u003clog4cxx/fileappender.h\u003e\n#include \u003clog4cxx/helpers/absolutetimedateformat.h\u003e\n\n\n \n##hybrid 2 0.016129031777381897 \n \"long string to test how those are handled. Here goes more text. \"\n \"long string to test how those are handled. Here goes more text. \"\n \"long string to test how those are handled. Here goes more text. \"\n \"long string to test how those are handled. Here goes more text. \"\n \"long string to test how those are handled. Here goes more text. \"\n \"long string to test how those are handled. Here goes more text. \"\n \"long string to test how those are handled. Here goes more text. \"\n \"long string to test how those are handled. Here goes more text. \"\n \"long string to test how those are handled. Here goes more text. \"\n \"long string to test how those are handled. Here goes more text. \"\n \"long string to test how those are handled. Here goes more text. \"\n \"long string to test how those are handled. Here goes more text. \"\n \"long string to test how those are handled. Here goes more text. \"\n };\n}\n\n\n \n##hybrid 3 0.016129031777381897 \n void simple()\n {\n LayoutPtr layout = LayoutPtr(new SimpleLayout());\n AppenderPtr appender = FileAppenderPtr(new FileAppender(layout, LOG4CXX_STR(\"output/simple\"), false));\n root-\u003eaddAppender(appender);\n common();\n\n LOGUNIT_ASSERT(Compare::compare(LOG4CXX_FILE(\"output/simple\"), LOG4CXX_FILE(\"witness/simple\")));\n }\n\n std::string createMessage(int i, Pool \u0026 pool)\n {\n std::string msg(\"Message \");\n msg.append(pool.itoa(i));\n return msg;\n }\n\n void common()\n {\n int i = 0;\n\n // In the lines below, the logger names are chosen as an aid in\n // remembering their level values. In general, the logger names\n // have no bearing to level values.\n LoggerPtr ERRlogger = Logger::getLogger(LOG4CXX_TEST_STR(\"ERR\"));\n ERRlogger-\u003esetLevel(Level::getError());\n\n\n \n##hybrid 4 0.01587301678955555 \nstd::vector\u003cstd::string\u003e MakeStrings() {\n return {\n \"a\", \"ab\", \"abc\", \"abcd\",\n \"long string to test how those are handled. Here goes more text. \"\n \"long string to test how those are handled. Here goes more text. \"\n \"long string to test how those are handled. Here goes more text. \"\n \"long string to test how those are handled. Here goes more text. \"\n \"long string to test how those are handled. Here goes more text. \"\n \"long string to test how those are handled. Here goes more text. \"\n```\n\n\n## Case 2: Hybrid Search Outperforms Full-Text Search\n\n**Query:** How do you initialize the logger? \n\nThis query is quite similar to the previous one, and the correct answer is also the same code snippet, but in this case, hybrid search found the answer (via semantic search), while full-text search did not. The reason for this discrepancy lies in the statistical weightings of words in the corpus, which do not align with our intuitive understanding of the question. The model failed to recognize that the match for the word \"how\" was not as important here. The word \"logger\" appeared more frequently in the code than \"how,\" which led to \"how\" becoming more significant in the full-text search ranking.\n\n**GroundTruth**\n\n```C++\nuse {\n crate::args::LogArgs,\n anyhow::{anyhow, Result},\n simplelog::{Config, LevelFilter, WriteLogger},\n std::fs::File,\n};\n\npub struct Logger;\n\nimpl Logger {\n pub fn init(args: \u0026impl LogArgs) -\u003e Result\u003c()\u003e {\n let filter: LevelFilter = args.log_level().into();\n if filter != LevelFilter::Off {\n let logfile = File::create(args.log_file())\n .map_err(|e| anyhow!(\"Failed to open log file: {e:}\"))?;\n WriteLogger::init(filter, Config::default(), logfile)\n .map_err(|e| anyhow!(\"Failed to initalize logger: {e:}\"))?;\n }\n Ok(())\n }\n}\n```\n\n\n### **Full Text Search Results**\n\n```C++\n##sparse 0 10.17311954498291 \n \"long string to test how those are handled. Here goes more text. \"\n \"long string to test how those are handled. Here goes more text. \"\n \"long string to test how those are handled. Here goes more text. \"\n \"long string to test how those are handled. Here goes more text. \"\n \"long string to test how those are handled. Here goes more text. \"\n \"long string to test how those are handled. Here goes more text. \"\n \"long string to test how those are handled. Here goes more text. \"\n \"long string to test how those are handled. Here goes more text. \"\n \"long string to test how those are handled. Here goes more text. \"\n \"long string to test how those are handled. Here goes more text. \"\n \"long string to test how those are handled. Here goes more text. \"\n \"long string to test how those are handled. Here goes more text. \"\n \"long string to test how those are handled. Here goes more text. \"\n };\n}\n\n\n\n ##sparse 1 9.775702476501465 \nstd::vector\u003cstd::string\u003e MakeStrings() {\n return {\n \"a\", \"ab\", \"abc\", \"abcd\",\n \"long string to test how those are handled. Here goes more text. \"\n \"long string to test how those are handled. Here goes more text. \"\n \"long string to test how those are handled. Here goes more text. \"\n \"long string to test how those are handled. Here goes more text. \"\n \"long string to test how those are handled. Here goes more text. \"\n \"long string to test how those are handled. Here goes more text. \"\n\n\n ##sparse 2 7.638711452484131 \n// union (\"x|y\"), grouping (\"(xy)\"), brackets (\"[xy]\"), and\n// repetition count (\"x{5,7}\"), among others.\n//\n// Below is the syntax that we do support. We chose it to be a\n// subset of both PCRE and POSIX extended regex, so it's easy to\n// learn wherever you come from. In the following: 'A' denotes a\n// literal character, period (.), or a single \\\\ escape sequence;\n// 'x' and 'y' denote regular expressions; 'm' and 'n' are for\n\n\n ##sparse 3 7.1208391189575195 \n/*\n * Licensed to the Apache Software Foundation (ASF) under one or more\n * contributor license agreements. See the NOTICE file distributed with\n * this work for additional information regarding copyright ownership.\n * The ASF licenses this file to You under the Apache License, Version 2.0\n * (the \"License\"); you may not use this file except in compliance with\n * the License. You may obtain a copy of the License at\n *\n * http://www.apache.org/licenses/LICENSE-2.0\n *\n * Unless required by applicable law or agreed to in writing, software\n * distributed under the License is distributed on an \"AS IS\" BASIS,\n * WITHOUT WARRANTIES OR CONDITIONS OF ANY KIND, either express or implied.\n * See the License for the specific language governing permissions and\n * limitations under the License.\n */\n#include \"logunit.h\"\n#include \u003clog4cxx/logger.h\u003e\n#include \u003clog4cxx/simplelayout.h\u003e\n#include \u003clog4cxx/fileappender.h\u003e\n#include \u003clog4cxx/helpers/absolutetimedateformat.h\u003e\n\n\n\n ##sparse 4 7.066349029541016 \n/*\n * Licensed to the Apache Software Foundation (ASF) under one or more\n * contributor license agreements. See the NOTICE file distributed with\n * this work for additional information regarding copyright ownership.\n * The ASF licenses this file to You under the Apache License, Version 2.0\n * (the \"License\"); you may not use this file except in compliance with\n * the License. You may obtain a copy of the License at\n *\n * http://www.apache.org/licenses/LICENSE-2.0\n *\n * Unless required by applicable law or agreed to in writing, software\n * distributed under the License is distributed on an \"AS IS\" BASIS,\n * WITHOUT WARRANTIES OR CONDITIONS OF ANY KIND, either express or implied.\n * See the License for the specific language governing permissions and\n * limitations under the License.\n */\n#include \u003clog4cxx/filter/denyallfilter.h\u003e\n#include \u003clog4cxx/logger.h\u003e\n#include \u003clog4cxx/spi/filter.h\u003e\n#include \u003clog4cxx/spi/loggingevent.h\u003e\n#include \"../logunit.h\"\n```\n\n\n### **Hybrid Search Results**\n\n```C++\n\n ##hybrid 0 0.016393441706895828 \nuse {\n crate::args::LogArgs,\n anyhow::{anyhow, Result},\n simplelog::{Config, LevelFilter, WriteLogger},\n std::fs::File,\n};\n\npub struct Logger;\n\nimpl Logger {\n pub fn init(args: \u0026impl LogArgs) -\u003e Result\u003c()\u003e {\n let filter: LevelFilter = args.log_level().into();\n if filter != LevelFilter::Off {\n let logfile = File::create(args.log_file())\n .map_err(|e| anyhow!(\"Failed to open log file: {e:}\"))?;\n WriteLogger::init(filter, Config::default(), logfile)\n .map_err(|e| anyhow!(\"Failed to initalize logger: {e:}\"))?;\n }\n Ok(())\n }\n}\n\n \n##hybrid 1 0.016393441706895828 \n \"long string to test how those are handled. Here goes more text. \"\n \"long string to test how those are handled. Here goes more text. \"\n \"long string to test how those are handled. Here goes more text. \"\n \"long string to test how those are handled. Here goes more text. \"\n \"long string to test how those are handled. Here goes more text. \"\n \"long string to test how those are handled. Here goes more text. \"\n \"long string to test how those are handled. Here goes more text. \"\n \"long string to test how those are handled. Here goes more text. \"\n \"long string to test how those are handled. Here goes more text. \"\n \"long string to test how those are handled. Here goes more text. \"\n \"long string to test how those are handled. Here goes more text. \"\n \"long string to test how those are handled. Here goes more text. \"\n \"long string to test how those are handled. Here goes more text. \"\n };\n}\n\n\n \n##hybrid 2 0.016129031777381897 \nstd::vector\u003cstd::string\u003e MakeStrings() {\n return {\n \"a\", \"ab\", \"abc\", \"abcd\",\n \"long string to test how those are handled. Here goes more text. \"\n \"long string to test how those are handled. Here goes more text. \"\n \"long string to test how those are handled. Here goes more text. \"\n \"long string to test how those are handled. Here goes more text. \"\n \"long string to test how those are handled. Here goes more text. \"\n \"long string to test how those are handled. Here goes more text. \"\n\n \n##hybrid 3 0.016129031777381897 \n LoggerPtr INF = Logger::getLogger(LOG4CXX_TEST_STR(\"INF\"));\n INF-\u003esetLevel(Level::getInfo());\n\n LoggerPtr INF_ERR = Logger::getLogger(LOG4CXX_TEST_STR(\"INF.ERR\"));\n INF_ERR-\u003esetLevel(Level::getError());\n\n LoggerPtr DEB = Logger::getLogger(LOG4CXX_TEST_STR(\"DEB\"));\n DEB-\u003esetLevel(Level::getDebug());\n\n // Note: categories with undefined level\n LoggerPtr INF_UNDEF = Logger::getLogger(LOG4CXX_TEST_STR(\"INF.UNDEF\"));\n LoggerPtr INF_ERR_UNDEF = Logger::getLogger(LOG4CXX_TEST_STR(\"INF.ERR.UNDEF\"));\n LoggerPtr UNDEF = Logger::getLogger(LOG4CXX_TEST_STR(\"UNDEF\"));\n\n\n \n##hybrid 4 0.01587301678955555 \n// union (\"x|y\"), grouping (\"(xy)\"), brackets (\"[xy]\"), and\n// repetition count (\"x{5,7}\"), among others.\n//\n// Below is the syntax that we do support. We chose it to be a\n// subset of both PCRE and POSIX extended regex, so it's easy to\n// learn wherever you come from. In the following: 'A' denotes a\n// literal character, period (.), or a single \\\\ escape sequence;\n// 'x' and 'y' denote regular expressions; 'm' and 'n' are for\n```\n\nIn our observations, we found that in the sparse vector search, many low-quality results were caused by matching low-information words like \"How\" and \"What.\" By examining the data, we realized that these words caused interference in the results. One approach to mitigate this issue is to add these words to a stopword list and ignore them during the matching process. This would help eliminate the negative impact of these common words and improve the quality of the search results.\n\n\n## Case 3: **Hybrid Search (with Stopword Addition) Outperforms Semantic Search**\n\nAfter adding the stopwords to filter out low-information words like \"How\" and \"What,\" we analyzed a case where a fine-tuned hybrid search performed better than a semantic search. The improvement in this case was due to matching the term \"RegistryClient\" in the query, which allowed us to find results not recalled by the semantic search model alone.\n\nFurthermore, we noticed that hybrid search reduced the number of low-quality matches in the results. In this case, the hybrid search method successfully integrated the semantic search with the full-text search, leading to more relevant results with improved accuracy.\n\n**Query:** How is the RegistryClient instance created in the test methods?\n\nThe hybrid search effectively retrieved the answer related to creating the \"RegistryClient\" instance, which semantic search alone failed to find. Adding stopwords helped avoid irrelevant results from terms like \"How,\" leading to better-quality matches and fewer low-quality results.\n\n```C++\n/** Integration tests for {@link BlobPuller}. */\npublic class BlobPullerIntegrationTest {\n\n private final FailoverHttpClient httpClient = new FailoverHttpClient(true, false, ignored -\u003e {});\n\n @Test\n public void testPull() throws IOException, RegistryException {\n RegistryClient registryClient =\n RegistryClient.factory(EventHandlers.NONE, \"gcr.io\", \"distroless/base\", httpClient)\n .newRegistryClient();\n V22ManifestTemplate manifestTemplate =\n registryClient\n .pullManifest(\n ManifestPullerIntegrationTest.KNOWN_MANIFEST_V22_SHA, V22ManifestTemplate.class)\n .getManifest();\n\n DescriptorDigest realDigest = manifestTemplate.getLayers().get(0).getDigest();\n```\n\n\n### Semantic Search Results\n\n```C++\n\n \n\n##dense 0 0.7411458492279053 \n Mockito.doThrow(mockRegistryUnauthorizedException)\n .when(mockJibContainerBuilder)\n .containerize(mockContainerizer);\n\n try {\n testJibBuildRunner.runBuild();\n Assert.fail();\n\n } catch (BuildStepsExecutionException ex) {\n Assert.assertEquals(\n TEST_HELPFUL_SUGGESTIONS.forHttpStatusCodeForbidden(\"someregistry/somerepository\"),\n ex.getMessage());\n }\n }\n\n\n\n ##dense 1 0.7346029877662659 \n verify(mockCredentialRetrieverFactory).known(knownCredential, \"credentialSource\");\n verify(mockCredentialRetrieverFactory).known(inferredCredential, \"inferredCredentialSource\");\n verify(mockCredentialRetrieverFactory)\n .dockerCredentialHelper(\"docker-credential-credentialHelperSuffix\");\n }\n\n\n\n ##dense 2 0.7285804748535156 \n when(mockCredentialRetrieverFactory.dockerCredentialHelper(anyString()))\n .thenReturn(mockDockerCredentialHelperCredentialRetriever);\n when(mockCredentialRetrieverFactory.known(knownCredential, \"credentialSource\"))\n .thenReturn(mockKnownCredentialRetriever);\n when(mockCredentialRetrieverFactory.known(inferredCredential, \"inferredCredentialSource\"))\n .thenReturn(mockInferredCredentialRetriever);\n when(mockCredentialRetrieverFactory.wellKnownCredentialHelpers())\n .thenReturn(mockWellKnownCredentialHelpersCredentialRetriever);\n\n\n\n ##dense 3 0.7279614210128784 \n @Test\n public void testBuildImage_insecureRegistryException()\n throws InterruptedException, IOException, CacheDirectoryCreationException, RegistryException,\n ExecutionException {\n InsecureRegistryException mockInsecureRegistryException =\n Mockito.mock(InsecureRegistryException.class);\n Mockito.doThrow(mockInsecureRegistryException)\n .when(mockJibContainerBuilder)\n .containerize(mockContainerizer);\n\n try {\n testJibBuildRunner.runBuild();\n Assert.fail();\n\n } catch (BuildStepsExecutionException ex) {\n Assert.assertEquals(TEST_HELPFUL_SUGGESTIONS.forInsecureRegistry(), ex.getMessage());\n }\n }\n\n\n\n ##dense 4 0.724872350692749 \n @Test\n public void testBuildImage_registryCredentialsNotSentException()\n throws InterruptedException, IOException, CacheDirectoryCreationException, RegistryException,\n ExecutionException {\n Mockito.doThrow(mockRegistryCredentialsNotSentException)\n .when(mockJibContainerBuilder)\n .containerize(mockContainerizer);\n\n try {\n testJibBuildRunner.runBuild();\n Assert.fail();\n\n } catch (BuildStepsExecutionException ex) {\n Assert.assertEquals(TEST_HELPFUL_SUGGESTIONS.forCredentialsNotSent(), ex.getMessage());\n }\n }\n```\n\n\n### Hybrid Search Results\n\n```C++\n\n ##hybrid 0 0.016393441706895828 \n/** Integration tests for {@link BlobPuller}. */\npublic class BlobPullerIntegrationTest {\n\n private final FailoverHttpClient httpClient = new FailoverHttpClient(true, false, ignored -\u003e {});\n\n @Test\n public void testPull() throws IOException, RegistryException {\n RegistryClient registryClient =\n RegistryClient.factory(EventHandlers.NONE, \"gcr.io\", \"distroless/base\", httpClient)\n .newRegistryClient();\n V22ManifestTemplate manifestTemplate =\n registryClient\n .pullManifest(\n ManifestPullerIntegrationTest.KNOWN_MANIFEST_V22_SHA, V22ManifestTemplate.class)\n .getManifest();\n\n DescriptorDigest realDigest = manifestTemplate.getLayers().get(0).getDigest();\n\n\n \n##hybrid 1 0.016393441706895828 \n Mockito.doThrow(mockRegistryUnauthorizedException)\n .when(mockJibContainerBuilder)\n .containerize(mockContainerizer);\n\n try {\n testJibBuildRunner.runBuild();\n Assert.fail();\n\n } catch (BuildStepsExecutionException ex) {\n Assert.assertEquals(\n TEST_HELPFUL_SUGGESTIONS.forHttpStatusCodeForbidden(\"someregistry/somerepository\"),\n ex.getMessage());\n }\n }\n\n\n \n##hybrid 2 0.016129031777381897 \n verify(mockCredentialRetrieverFactory).known(knownCredential, \"credentialSource\");\n verify(mockCredentialRetrieverFactory).known(inferredCredential, \"inferredCredentialSource\");\n verify(mockCredentialRetrieverFactory)\n .dockerCredentialHelper(\"docker-credential-credentialHelperSuffix\");\n }\n\n\n \n##hybrid 3 0.016129031777381897 \n @Test\n public void testPull_unknownBlob() throws IOException, DigestException {\n DescriptorDigest nonexistentDigest =\n DescriptorDigest.fromHash(\n \"aaaaaaaaaaaaaaaaaaaaaaaaaaaaaaaaaaaaaaaaaaaaaaaaaaaaaaaaaaaaaaaa\");\n\n RegistryClient registryClient =\n RegistryClient.factory(EventHandlers.NONE, \"gcr.io\", \"distroless/base\", httpClient)\n .newRegistryClient();\n\n try {\n registryClient\n .pullBlob(nonexistentDigest, ignored -\u003e {}, ignored -\u003e {})\n .writeTo(ByteStreams.nullOutputStream());\n Assert.fail(\"Trying to pull nonexistent blob should have errored\");\n\n } catch (IOException ex) {\n if (!(ex.getCause() instanceof RegistryErrorException)) {\n throw ex;\n }\n MatcherAssert.assertThat(\n ex.getMessage(),\n CoreMatchers.containsString(\n \"pull BLOB for gcr.io/distroless/base with digest \" + nonexistentDigest));\n }\n }\n}\n\n \n##hybrid 4 0.01587301678955555 \n when(mockCredentialRetrieverFactory.dockerCredentialHelper(anyString()))\n .thenReturn(mockDockerCredentialHelperCredentialRetriever);\n when(mockCredentialRetrieverFactory.known(knownCredential, \"credentialSource\"))\n .thenReturn(mockKnownCredentialRetriever);\n when(mockCredentialRetrieverFactory.known(inferredCredential, \"inferredCredentialSource\"))\n .thenReturn(mockInferredCredentialRetriever);\n when(mockCredentialRetrieverFactory.wellKnownCredentialHelpers())\n .thenReturn(mockWellKnownCredentialHelpersCredentialRetriever);\n```\n\n\n## Conclusions\n\nFrom our analysis, we can draw several conclusions about the performance of different retrieval methods. For most of the cases, the semantic search model helps us obtain good results by grasping the overall intention of the query, but it falls short when the query contains specific keywords we want to match.\n\nIn these cases, the embedding model doesn't explicitly represent this intent. On the other hand, Full-text search can address this issue directly. However, it also brings the problem of irrelevant results despite matching words, which can degrade the overall result quality. Therefore, it’s crucial to identify and handle these negative cases by analyzing specific results and applying targeted strategies to improve search quality. A hybrid search with ranking strategies such as RRF or weighted reranker is usually a good baseline option.\n\nWith the release of the full-text search functionality in Milvus 2.5, we aim to provide the community with flexible and diverse information retrieval solutions. This will allow users to explore various combinations of search methods and address the increasingly complex and varied search demands in the GenAI era. Check out the code example on [how to implement full-text search and hybrid search with Milvus 2.5](https://milvus.io/docs/full_text_search_with_milvus.md).\n","title":"Semantic Search vs. Full-Text Search: Which Do I Choose in Milvus 2.5?","metaData":{}},{"id":"build-multi-tenancy-rag-with-milvus-best-practices-part-one.md","author":"Robert Guo","tags":["Engineering"],"recommend":true,"canonicalUrl":"https://zilliz.com/blog/build-multi-tenancy-rag-with-milvus-best-practices-part-one","date":"2024-12-04T00:00:00.000Z","cover":"https://assets.zilliz.com/Designing_Multi_Tenancy_RAG_with_Milvus_40b3737145.png","href":"/blog/build-multi-tenancy-rag-with-milvus-best-practices-part-one.md","content":"\n\n## Introduction \n\nOver the past couple of years, [Retrieval-Augmented Generation (RAG)](https://zilliz.com/learn/Retrieval-Augmented-Generation) has emerged as a trusted solution for large organizations to enhance their [LLM](https://zilliz.com/glossary/large-language-models-(llms))-powered applications, especially those with diverse users. As such applications grow, implementing a multi-tenancy framework becomes essential. **Multi-tenancy** provides secure, isolated access to data for different user groups, ensuring user trust, meeting regulatory standards, and improving operational efficiency.\n\n[Milvus](https://zilliz.com/what-is-milvus) is an open-source [vector database](https://zilliz.com/learn/what-is-vector-database) built to handle high-dimensional [vector data](https://zilliz.com/glossary/vector-embeddings). It is an indispensable infrastructure component of RAG, storing and retrieving contextual information for LLMs from external sources. Milvus offers [flexible multi-tenancy strategies](https://milvus.io/docs/multi_tenancy.md) for various needs, including **database-level, collection-level, and partition-level multi-tenancy**. \n\nIn this post, we’ll cover: \n\n- What is Multi-Tenancy and Why It Matters\n\n- Multi-Tenancy Strategies in Milvus\n\n- Example: Multi-Tenancy Strategy for a RAG-Powered Enterprise Knowledge Base\n\n\n## What is Multi-Tenancy and Why It Matters\n\n[**Multi-tenancy**](https://milvus.io/docs/multi_tenancy.md) is an architecture where multiple customers or teams, known as \"**tenants,**\" share a single instance of an application or system. Each tenant’s data and configurations are logically isolated, ensuring privacy and security, while all tenants share the same underlying infrastructure. \n\nImagine a SaaS platform that provides knowledge-based solutions to multiple companies. Each company is a tenant. \n\n- Tenant A is a healthcare organization storing patient-facing FAQs and compliance documents.\n\n- Tenant B is a tech company managing internal IT troubleshooting workflows.\n\n- Tenant C is a retail business with customer service FAQs for product returns.\n\nEach tenant operates in a completely isolated environment, ensuring that no data from Tenant A leaks into Tenant B’s system or vice versa. Furthermore, resource allocation, query performance, and scaling decisions are tenant-specific, ensuring high performance regardless of workload spikes in one tenant.\n\nMulti-tenancy also works for systems serving different teams within the same organization. Imagine a large company using a RAG-powered knowledge base to serve its internal departments, such as HR, Legal, and Marketing. Each **department is a tenant** with isolated data and resources in this setup. \n\nMulti-tenancy offers significant benefits, including **cost efficiency, scalability, and robust data security**. By sharing a single infrastructure, service providers can reduce overhead costs and ensure more effective resource consumption. This approach also scales effortlessly—onboarding new tenants requires far fewer resources than creating separate instances for each one, as with single-tenancy models. Importantly, multi-tenancy maintains robust data security by ensuring strict data isolation for each tenant, with access controls and encryption protecting sensitive information from unauthorized access. Additionally, updates, patches, and new features can be deployed across all tenants simultaneously, simplifying system maintenance and reducing the burden on administrators while ensuring that security and compliance standards are consistently upheld. \n\n\n## Multi-Tenancy Strategies in Milvus\n\nTo understand how Milvus supports multi-tenancy, it's important to first look at how it organizes user data. \n\n\n### How Milvus Organizes User Data\n\nMilvus structures data across three layers, moving from broad to granular: [**Database**](https://milvus.io/docs/manage_databases.md), [**Collection**](https://milvus.io/docs/manage-collections.md), and [**Partition/Partition Key**](https://milvus.io/docs/manage-partitions.md). \n\n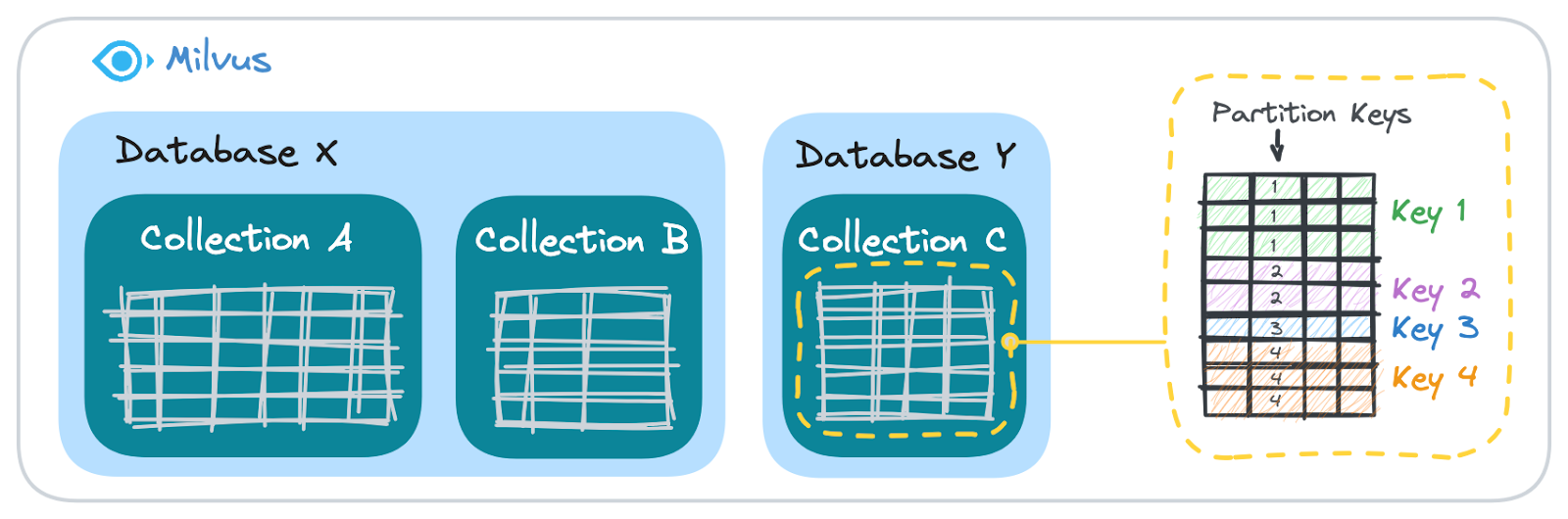\n\n_Figure: How Milvus organizes user data_ \n\n- **Database**: This acts as a logical container, similar to a database in traditional relational systems.\n\n- **Collection**: Comparable to a table within a database, a collection organizes data into manageable groups.\n\n- **Partition/Partition Key**: Within a collection, data can be further segmented by **Partitions**. Using a **Partition Key**, data with the same key is grouped together. For example, if you use a **user ID** as the **Partition Key**, all data for a specific user will be stored in the same logical segment. This makes it straightforward to retrieve data tied to individual users.\n\nAs you move from **Database** to **Collection** to **Partition Key**, the granularity of data organization becomes progressively finer. \n\nTo ensure stronger data security and proper access control, Milvus also provides robust [**Role-Based Access Control (RBAC)**](https://zilliz.com/blog/enabling-fine-grained-access-control-with-milvus-row-level-rbac), allowing administrators to define specific permissions for each user. Only authorized users can access certain data. \n\nMilvus supports [multiple strategies](https://milvus.io/docs/multi_tenancy.md) for implementing multi-tenancy, offering flexibility based on the needs of your application: **database-level, collection-level, and partition-level multi-tenancy**. \n\n\n### Database-Level Multi-Tenancy\n\nWith the database-level multi-tenancy approach, each tenant is assigned their own database within the same Milvus cluster. This strategy provides strong data isolation and ensures optimal search performance. However, it can lead to inefficient resource utilization if certain tenants remain inactive.\n\n\n### Collection-Level Multi-Tenancy\n\nHere, in collection-level multi-tenancy, we can organize data for tenants in two ways. \n\n- **One Collection for All Tenants**: All tenants share a single collection, with tenant-specific fields used for filtering. While simple to implement, this approach can encounter performance bottlenecks as the number of tenants increases. \n\n- **One Collection per Tenant**: Each tenant can have a dedicated collection, improving isolation and performance but requiring more resources. This setup may face scalability limitations if the number of tenants exceeds Milvus's collection capacity.\n\n\n### Partition-Level Multi-Tenancy\n\nPartition-Level Multi-Tenancy focuses on organizing tenants within a single collection. Here, we also have two ways to organize tenant data. \n\n- **One Partition per Tenant**: Tenants share a collection, but their data is stored in separate partitions. We can isolate data by assigning each tenant a dedicated partition, balancing isolation and search performance. However, this approach is constrained by Milvus's maximum partition limit. \n\n- **Partition-Key-Based Multi-Tenancy**: This is a more scalable option in which a single collection uses partition keys to distinguish tenants. This method simplifies resource management and supports higher scalability but does not support bulk data inserts.\n\nThe table below summarizes the key differences between key multi-tenancy approaches. \n\n| **Granularity** | **Database-level** | **Collection-level** | **Partition Key-level** |\n| ----------------------------- | ---------------------------------------------------------------- | ----------------------------------------------------------------- | ----------------------------------------------------------------- |\n| Max Tenants Supported | ~1,000 | ~10,000 | ~10,000,000 |\n| Data Organization Flexibility | High: Users can define multiple collections with custom schemas. | Medium: Users are limited to one collection with a custom schema. | Low: All users share a collection, requiring a consistent schema. |\n| Cost per User | High | Medium | Low |\n| Physical Resource Isolation | Yes | Yes | No |\n| RBAC | Yes | Yes | No |\n| Search Performance | Strong | Medium | Strong |\n\n\n## Example: Multi-Tenancy Strategy for a RAG-Powered Enterprise Knowledge Base\n\nWhen designing the multi-tenancy strategy for a RAG system, it's essential to align your approach with the specific needs of your business and your tenants. Milvus offers various multi-tenancy strategies, and choosing the right one depends on the number of tenants, their requirements, and the level of data isolation needed. Here's a practical guide for making these decisions, taking a RAG-powered enterprise knowledge base as an example. \n\n\n### Understanding Tenant Structure Before Choosing a Multi-Tenancy Strategy \n\nA RAG-powered enterprise knowledge base often serves a small number of tenants. These tenants are usually independent business units like IT, Sales, Legal, and Marketing, each requiring distinct knowledge base services. For example, the HR Department manages sensitive employee information like onboarding guides and benefits policies, which should be confidential and accessible only to HR personnel.\n\nIn this case, each business unit should be treated as a separate tenant and a **Database-level multi-tenancy strategy** is often the most suitable. By assigning dedicated databases to each tenant, organizations can achieve strong logical isolation, simplifying management and enhancing security. This setup provides tenants with significant flexibility—they can define custom data models within collections, create as many collections as needed, and independently manage access control for their collections.\n\n\n### Enhancing Security with Physical Resource Isolation\n\nIn situations where data security is highly prioritized, logical isolation at the database level may not be enough. For example, some business units might handle critical or highly sensitive data, requiring stronger guarantees against interference from other tenants. In such cases, we can implement a [physical isolation approach](https://milvus.io/docs/resource_group.md) on top of a database-level multi-tenancy structure. \n\nMilvus enables us to map logical components, such as databases and collections, to physical resources. This method ensures that the activities of other tenants do not impact critical operations. Let's explore how this approach works in practice. \n\n\n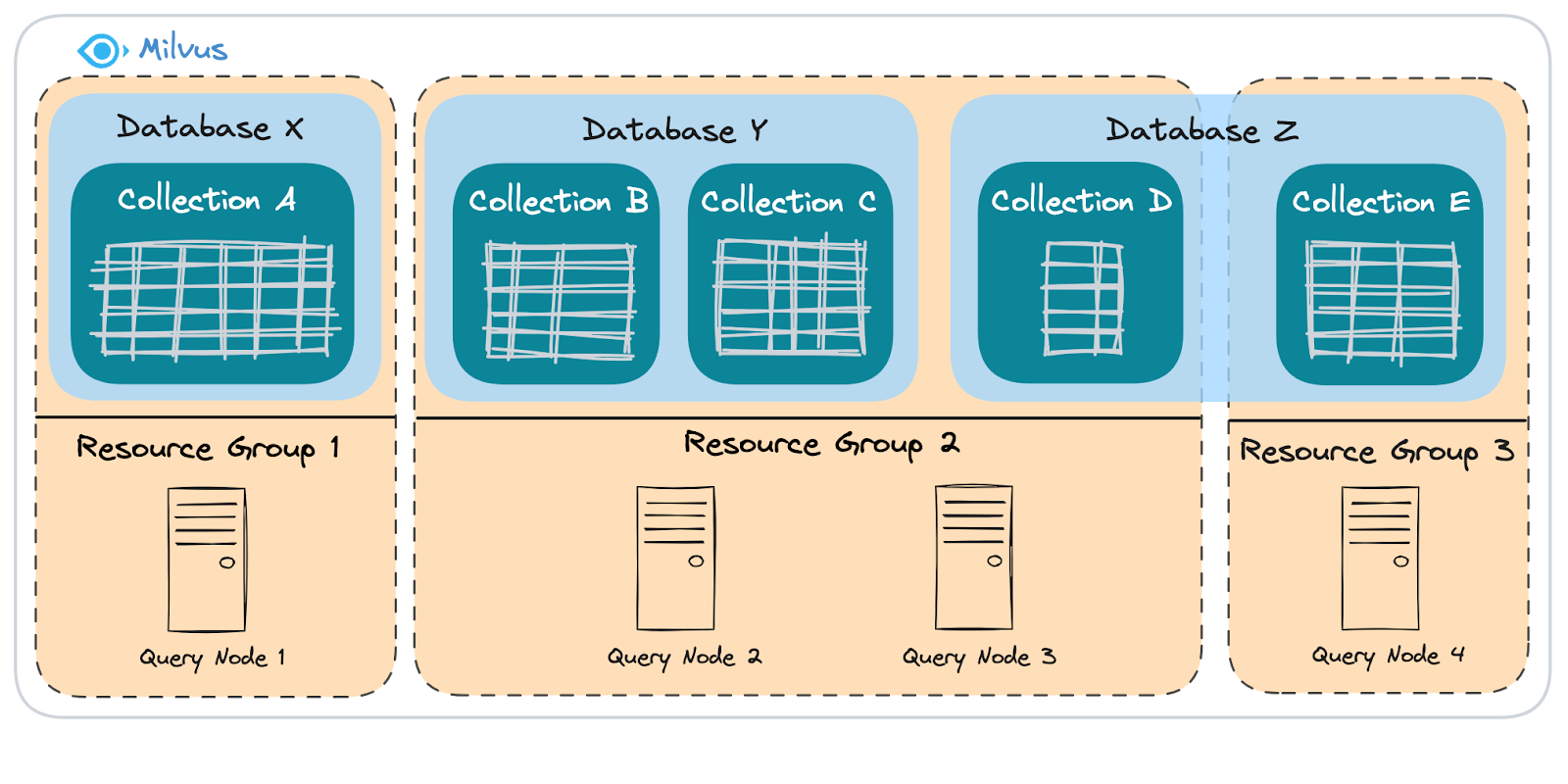\n\n\nFigure: How Milvus manages physical resources\n\nAs shown in the diagram above, there are three layers of resource management in Milvus: **Query Node**, **Resource Group**, and **Database**. \n\n- **Query Node**: The component that processes query tasks. It runs on a physical machine or container (e.g., a pod in Kubernetes).\n\n- **Resource Group**: A collection of Query Nodes that acts as a bridge between logical components (databases and collections) and physical resources. You can allocate one or more databases or collections to a single Resource Group.\n\nIn the example shown in the diagram above, there are three logical **Databases**: X, Y, and Z. \n\n- **Database X**: Contains **Collection A**.\n\n- **Database Y**: Contains **Collections B** and **C**.\n\n- **Database Z**: Contains **Collections D** and **E**.\n\nLet’s say **Database X** holds a critical knowledge base that we don’t want to be affected by the load from **Database Y** or **Database Z**. To ensure data isolation:\n\n- **Database X** is assigned its own **Resource Group** to guarantee that its critical knowledge base is unaffected by workloads from other databases.\n\n- **Collection E** is also allocated to a separate **Resource Group** within its parent database (**Z**). This provides isolation at the collection level for specific critical data within a shared database.\n\nMeanwhile, the remaining collections in **Databases Y** and **Z** share the physical resources of **Resource Group 2**. \n\nBy carefully mapping logical components to physical resources, organizations can achieve a flexible, scalable, and secure multi-tenancy architecture tailored to their specific business needs.\n\n\n### Designing End User-Level Access \n\nNow that we've learned the best practices for choosing a multi-tenancy strategy for an enterprise RAG, let's explore how to design user-level access in such systems. \n\nIn these systems, end users usually interact with the knowledge base in a read-only mode through LLMs. However, organizations still need to track such Q\u0026A data generated by users and link it to specific users for various purposes, such as improving the knowledge base’s accuracy or offering personalized services.\n\nTake a hospital's smart consultation service desk as an example. Patients might ask questions like, “Are there any available appointments with the specialist today?” or \"Is there any specific preparation needed for my upcoming surgery?\" While these questions don’t directly impact the knowledge base, it’s important for the hospital to track such interactions to improve services. These Q\u0026A pairs are usually stored in a separate database (it doesn't necessarily have to be a vector database) dedicated to logging interactions.\n\n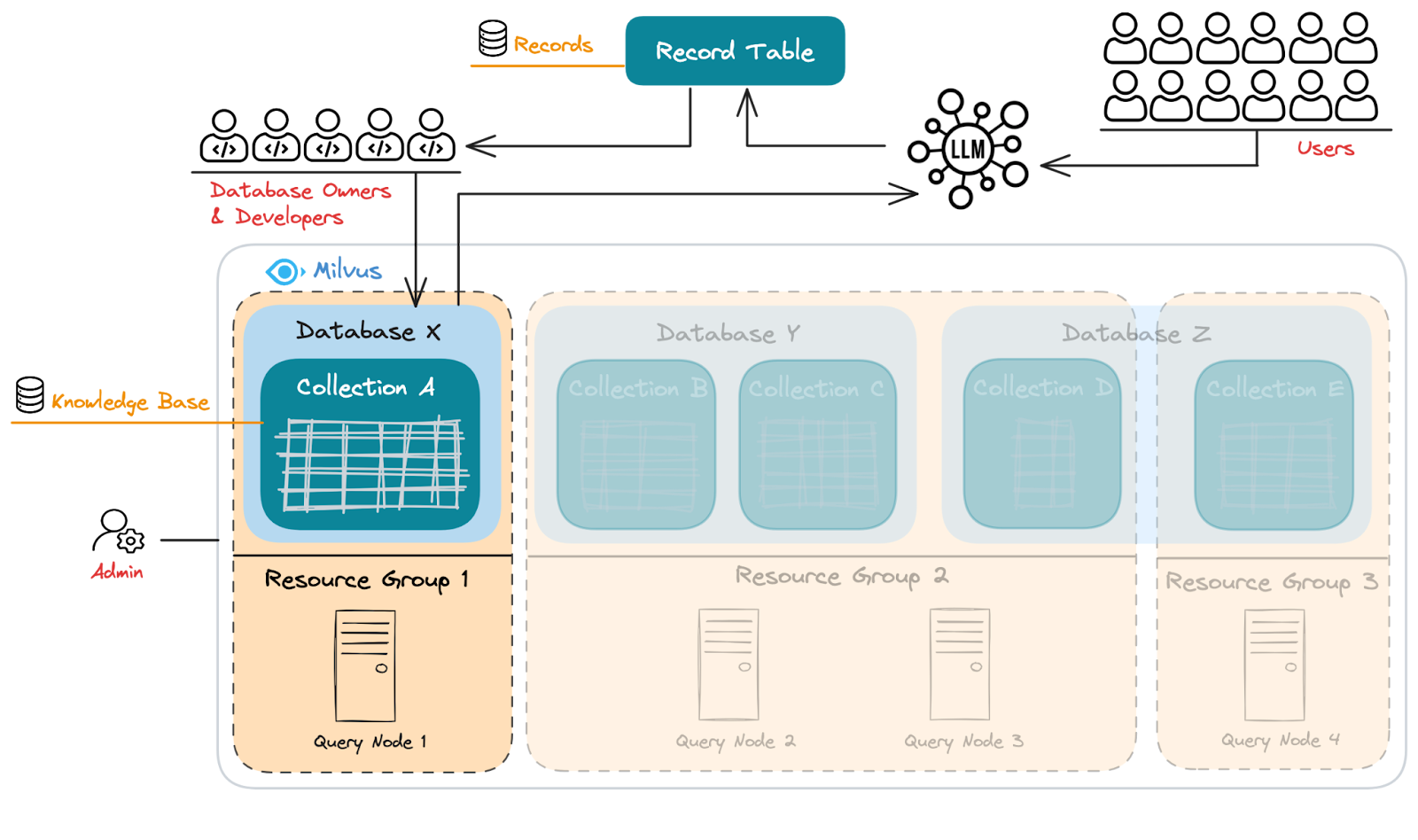\n\n_Figure: The multi-tenancy architecture for an enterprise RAG knowledge base_ \n\nThe diagram above shows the multi-tenancy architecture of an enterprise RAG system. \n\n- **System Administrators** oversee the RAG system, manage resource allocation, assign databases, map them to resource groups, and ensure scalability. They handle the physical infrastructure, as shown in the diagram, where each resource group (e.g., Resource Group 1, 2, and 3) is mapped to physical servers (query nodes).\n\n- **Tenants (Database owners and developers)** manage the knowledge base, iterating on it based on the user-generated Q\u0026A data, as shown in the diagram. Different databases (Database X, Y, Z) contain collections with different knowledge base content (Collection A, B, etc.).\n\n- **End Users** interact with the system in a read-only manner through the LLM. As they query the system, their questions are logged in the separate Q\u0026A record table (a separate database), continuously feeding valuable data back into the system.\n\nThis design ensures that each process layer—from user interaction to system administration—works seamlessly, helping the organization build a robust and continuously improving knowledge base.\n\n\n## Summary \n\nIn this blog, we’ve explored how [**multi-tenancy**](https://milvus.io/docs/multi_tenancy.md) frameworks play a critical role in the scalability, security, and performance of RAG-powered knowledge bases. By isolating data and resources for different tenants, businesses can ensure privacy, regulatory compliance, and optimized resource allocation across a shared infrastructure. [Milvus](https://milvus.io/docs/overview.md), with its flexible multi-tenancy strategies, allows businesses to choose the right level of data isolation—from database level to partition level—depending on their specific needs. Choosing the right multi-tenancy approach ensures companies can provide tailored services to tenants, even when dealing with diverse data and workloads. \n\nBy following the best practices outlined here, organizations can effectively design and manage multi-tenancy RAG systems that not only deliver superior user experiences but also scale effortlessly as business needs grow. Milvus’ architecture ensures that enterprises can maintain high levels of isolation, security, and performance, making it a crucial component in building enterprise-grade, RAG-powered knowledge bases.\n\n\n## Stay Tuned for More Insights into Multi-Tenancy RAG\n\nIn this blog, we’ve discussed how Milvus’ multi-tenancy strategies are designed to manage tenants, but not end users within those tenants. End-user interactions usually happen at the application layer, while the vector database itself remains unaware of those users.\n\nYou might be wondering: _If I want to provide more precise answers based on each end user’s query history, doesn’t Milvus need to maintain a personalized Q\u0026A context for each user?_\n\nThat’s a great question, and the answer really depends on the use case. For example, in an on-demand consultation service, queries are random, and the main focus is on the quality of the knowledge base rather than on keeping track of a user's historical context.\n\nHowever, in other cases, RAG systems must be context-aware. When this is required, Milvus needs to collaborate with the application layer to maintain a personalized memory of each user's context. This design is especially important for applications with massive end users, which we’ll explore in greater detail in my next post. Stay tuned for more insights!\n","title":"Designing Multi-Tenancy RAG with Milvus: Best Practices for Scalable Enterprise Knowledge Bases","metaData":{}},{"id":"how-to-contribute-to-milvus-a-quick-start-for-developers.md","author":"Shaoting Huang","tags":["Engineering"],"recommend":true,"canonicalUrl":"https://milvus.io/blog/how-to-contribute-to-milvus-a-quick-start-for-developers.md","date":"2024-12-01T00:00:00.000Z","cover":"https://assets.zilliz.com/How_to_Contribute_to_Milvus_91e1432163.png","href":"/blog/how-to-contribute-to-milvus-a-quick-start-for-developers.md","content":"\n\n\n[**Milvus**](https://github.com/milvus-io/milvus) is an open-source [vector database](https://zilliz.com/learn/what-is-vector-database) designed to manage high-dimensional vector data. Whether you're building intelligent search engines, recommendation systems, or next-gen AI solutions such as retrieval augmented generation ([RAG](https://zilliz.com/learn/Retrieval-Augmented-Generation)), Milvus is a powerful tool at your fingertips. \n\nBut what truly drives Milvus forward isn’t just its advanced technology—it’s the vibrant, passionate [developer community](https://zilliz.com/community) behind it. As an open-source project, Milvus thrives and evolves thanks to the contributions of developers like you. Every bug fix, feature addition, and performance enhancement from the community makes Milvus faster, more scalable, and more reliable.\n\nWhether you're passionate about open-source, eager to learn, or want to make a lasting impact in AI, Milvus is the perfect place to contribute. This guide will walk you through the process—from setting up your development environment to submitting your first pull request. We’ll also highlight common challenges you might face and provide solutions to overcome them.\n\nReady to dive in? Let’s make Milvus even better together!\n\n\n## Setting Up Your Milvus Development Environment\n\nFirst thing first: setting up your development environment. You can either install Milvus on your local machine or use Docker—both methods are straightforward, but you’ll also need to install a few third-party dependencies to get everything running.\n\n\n### Building Milvus Locally\n\nIf you like building things from scratch, building Milvus on your local machine is a breeze. Milvus makes it easy by bundling all the dependencies in the `install_deps.sh` script. Here’s the quick setup:\n\n\n```\n# Install third-party dependencies.\n$ cd milvus/\n$ ./scripts/install_deps.sh\n\n# Compile Milvus.\n$ make\n```\n\n### Building Milvus with Docker\n\nIf you prefer Docker, there are two ways to go about it: you can either run commands in a pre-built container or spin up a dev container for a more hands-on approach.\n\n\n\n```\n# Option 1: Run commands in a pre-built Docker container \nbuild/builder.sh make \n\n# Option 2: Spin up a dev container \n./scripts/devcontainer.sh up \ndocker-compose -f docker-compose-devcontainer.yml ps \ndocker exec -ti milvus-builder-1 bash \nmake milvus \n```\n\n\n\n**Platform Notes:** If you’re on Linux, you’re good to go—compilation issues are pretty rare. However, Mac users, especially with M1 chips, might run into some bumps along the way. Don’t sweat it, though—we have a guide to help you work through the most common issues. \n\n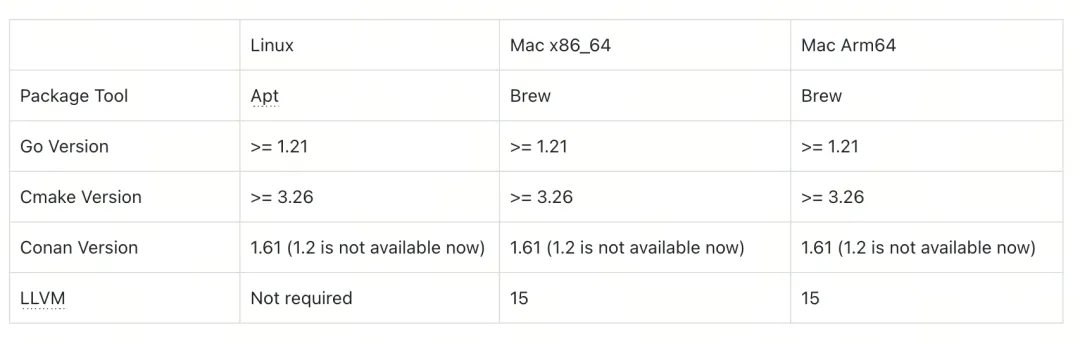\n\n_Figure: OS configuration_ \n\nFor the full setup guide, check out the official [Milvus Development Guide](https://github.com/milvus-io/milvus/blob/master/DEVELOPMENT.md).\n\n\n### Common Issues and How to Fix Them\n\nSometimes, setting up your Milvus development environment doesn’t go as smoothly as planned. Don’t worry—here’s a quick rundown of common issues you might hit and how to fix them fast.\n\n\n#### Homebrew: Unexpected Disconnect While Reading Sideband Packet\n\nIf you’re using Homebrew and see an error like this:\n\n\n```\n==\u003e Tapping homebrew/core\nremote: Enumerating objects: 1107077, done.\nremote: Counting objects: 100% (228/228), done.\nremote: Compressing objects: 100% (157/157), done.\nerror: 545 bytes of body are still expected.44 MiB | 341.00 KiB/s\nfetch-pack: unexpected disconnect while reading sideband packet\nfatal: early EOF\nfatal: index-pack failed\nFailed during: git fetch --force origin refs/heads/master:refs/remotes/origin/master\nmyuser~ %\n```\n\n\n\n**Fix:** Increase the `http.postBuffer` size:\n\n\n```\ngit config --global http.postBuffer 1M\n```\n\n\nIf you also run into `Brew: command not found` after installing Homebrew, you might need to set up your Git user configuration:\n\n\n```\ngit config --global user.email xxxgit config --global user.name xxx\n```\n\n\n\n#### Docker: Error Getting Credentials\n\nWhen working with Docker, you might see this:\n\n\n```\nerror getting credentials - err: exit status 1, out: `` \n```\n\n\n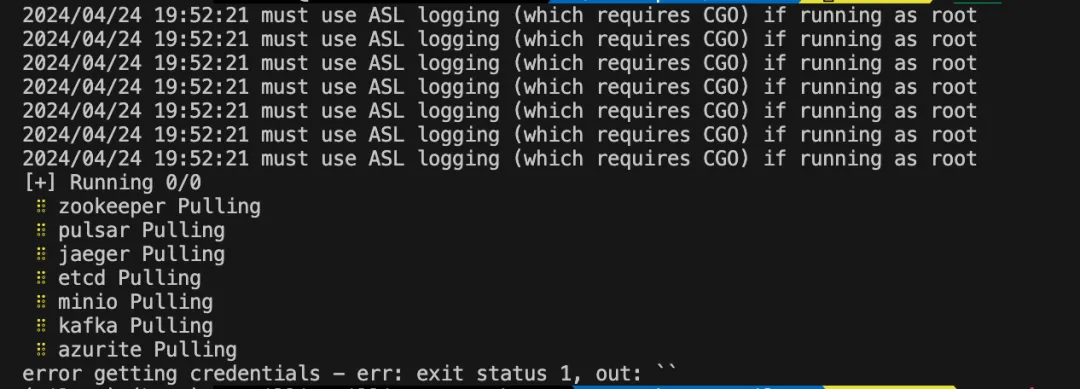\n\n\n\n**Fix:** Open` ~/.docker/config.json` and remove the `credsStore` field.\n\n\n#### Python: No Module Named 'imp'\n\nIf Python throws this error, it’s because Python 3.12 removed the `imp` module, which some older dependencies still use.\n\n\n 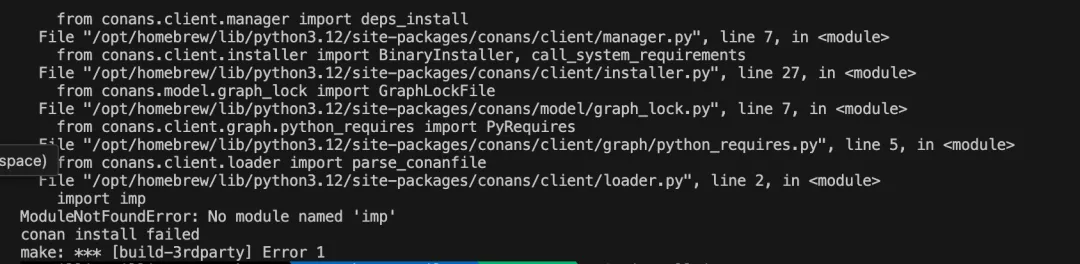\n\n**Fix:** Downgrade to Python 3.11:\n\n\n\n```\nbrew install python@3.11 \n```\n\n\n#### Conan: Unrecognized Arguments or Command Not Found\n\n**Issue:** If you see `Unrecognized arguments: --install-folder conan`, you’re likely using an incompatible Conan version.\n\n\n\n**Fix:** Downgrade to Conan 1.61:\n\n\n```\npip install conan==1.61 \n```\n\n\n**Issue:** If you see `Conan command not found`, it means your Python environment isn’t properly set up.\n\n**Fix:** Add Python’s bin directory to your `PATH`:\n\n\n```\nexport PATH=\"/path/to/python/bin:$PATH\"\n```\n\n\n\n#### LLVM: Use of Undeclared Identifier 'kSecFormatOpenSSL'\n\nThis error usually means your LLVM dependencies are outdated.\n\n\n\n**Fix:** Reinstall LLVM 15 and update your environment variables:\n\n\n```\nbrew reinstall llvm@15\nexport LDFLAGS=\"-L/opt/homebrew/opt/llvm@15/lib\"\nexport CPPFLAGS=\"-I/opt/homebrew/opt/llvm@15/include\"\n```\n\n\n**Pro Tips**\n\n- Always double-check your tool versions and dependencies.\n\n- If something still doesn’t work, the[ Milvus GitHub Issues page](https://github.com/milvus-io/milvus/issues) is a great place to find answers or ask for help.\n\n\n### Configuring VS Code for C++ and Go Integration\n\nGetting C++ and Go to work together in VS Code is easier than it sounds. With the right setup, you can streamline your development process for Milvus. Just tweak your `user.settings` file with the configuration below:\n\n\n```\n{\n \"go.toolsEnvVars\": {\n \"PKG_CONFIG_PATH\": \"/Users/zilliz/milvus/internal/core/output/lib/pkgconfig:/Users/zilliz/workspace/milvus/internal/core/output/lib64/pkgconfig\",\n \"LD_LIBRARY_PATH\": \"/Users/zilliz/workspace/milvus/internal/core/output/lib:/Users/zilliz/workspace/milvus/internal/core/output/lib64\",\n \"RPATH\": \"/Users/zilliz/workspace/milvus/internal/core/output/lib:/Users/zilliz/workspace/milvus/internal/core/output/lib64\"\n },\n \"go.testEnvVars\": {\n \"PKG_CONFIG_PATH\": \"/Users/zilliz/workspace/milvus/internal/core/output/lib/pkgconfig:/Users/zilliz/workspace/milvus/internal/core/output/lib64/pkgconfig\",\n \"LD_LIBRARY_PATH\": \"/Users/zilliz/workspace/milvus/internal/core/output/lib:/Users/zilliz/workspace/milvus/internal/core/output/lib64\",\n \"RPATH\": \"/Users/zilliz/workspace/milvus/internal/core/output/lib:/Users/zilliz/workspace/milvus/internal/core/output/lib64\"\n },\n \"go.buildFlags\": [\n \"-ldflags=-r /Users/zilliz/workspace/milvus/internal/core/output/lib\"\n ],\n \"terminal.integrated.env.linux\": {\n \"PKG_CONFIG_PATH\": \"/Users/zilliz/workspace/milvus/internal/core/output/lib/pkgconfig:/Users/zilliz/workspace/milvus/internal/core/output/lib64/pkgconfig\",\n \"LD_LIBRARY_PATH\": \"/Users/zilliz/workspace/milvus/internal/core/output/lib:/Users/zilliz/workspace/milvus/internal/core/output/lib64\",\n \"RPATH\": \"/Users/zilliz/workspace/milvus/internal/core/output/lib:/Users/zilliz/workspace/milvus/internal/core/output/lib64\"\n },\n \"go.useLanguageServer\": true,\n \"gopls\": {\n \"formatting.gofumpt\": true\n },\n \"go.formatTool\": \"gofumpt\",\n \"go.lintTool\": \"golangci-lint\",\n \"go.testTags\": \"dynamic\",\n \"go.testTimeout\": \"10m\"\n}\n```\n\n\n\nHere’s what this configuration does:\n\n- **Environment Variables:** Sets up paths for `PKG_CONFIG_PATH`, `LD_LIBRARY_PATH`, and `RPATH`, which are critical for locating libraries during builds and tests.\n\n- **Go Tools Integration:** Enables Go’s language server (`gopls`) and configures tools like `gofumpt` for formatting and `golangci-lint` for linting.\n\n- **Testing Setup:** Adds `testTags` and increases the timeout for running tests to 10 minutes.\n\nOnce added, this setup ensures a seamless integration between C++ and Go workflows. It’s perfect for building and testing Milvus without constant environment tweaking.\n\n\n**Pro Tip**\n\nAfter setting this up, run a quick test build to confirm everything works. If something feels off, double-check the paths and VS Code’s Go extension version.\n\n\n## Deploying Milvus\n\nMilvus supports [three deployment modes](https://milvus.io/docs/install-overview.md)—**Lite, Standalone,** and **Distributed.** \n\n- [**Milvus Lite**](https://milvus.io/blog/introducing-milvus-lite.md) is a Python library and an ultra-lightweight version of Milvus. It’s perfect for rapid prototyping in Python or notebook environments and for small-scale local experiments. \n\n- **Milvus Standalone** is the single-node deployment option for Milvus, using a client-server model. It is the Milvus equivalent of MySQL, while Milvus Lite is like SQLite. \n\n- **Milvus Distributed** is the distributed mode of Milvus, which is ideal for enterprise users building large-scale vector database systems or vector data platforms. \n\nAll these deployments rely on three core components:\n\n- **Milvus:** The vector database engine that drives all operations.\n\n- **Etcd:** The metadata engine that manages Milvus's internal metadata.\n\n- **MinIO:** The storage engine that ensures data persistence.\n\nWhen running in **Distributed** mode, Milvus also incorporates **Pulsar** for distributed message processing using a Pub/Sub mechanism, making it scalable for high-throughput environments.\n\n\n### Milvus Standalone\n\nThe Standalone mode is tailored for single-instance setups, making it perfect for testing and small-scale applications. Here’s how to get started:\n\n\n```\n# Deploy Milvus Standalone \nsudo docker-compose -f deployments/docker/dev/docker-compose.yml up -d\n# Start the standalone service \nbash ./scripts/start_standalone.sh\n```\n\n\n\n### Milvus Distributed (previously known as Milvus Cluster) \n\nFor larger datasets and higher traffic, the Distributed mode offers horizontal scalability. It combines multiple Milvus instances into a single cohesive system. Deployment is made easy with the **Milvus Operator**, which runs on Kubernetes and manages the entire Milvus stack for you.\n\nWant step-by-step guidance? Check out the [Milvus Installation Guide](https://milvus.io/docs/install_cluster-milvusoperator.md).\n\n\n## Running End-to-End (E2E) Tests\n\nOnce your Milvus deployment is up and running, testing its functionality is a breeze with E2E tests. These tests cover every part of your setup to ensure everything works as expected. Here’s how to run them:\n\n\n```\n# Navigate to the test directory \ncd tests/python_client \n\n# Install dependencies \npip install -r requirements.txt \n\n# Run E2E tests \npytest --tags=L0 -n auto \n```\n\n\n\nFor in-depth instructions and troubleshooting tips, refer to the [Milvus Development Guide](https://github.com/milvus-io/milvus/blob/master/DEVELOPMENT.md#e2e-tests).\n\n**Pro Tip**\n\nIf you're new to Milvus, start with Milvus Lite or Standalone mode to get a feel for its capabilities before scaling up to Distributed mode for production-level workloads.\n\n\n## Submitting Your Code\n\nCongrats! You’ve cleared all unit and E2E tests (or debugged and recompiled as needed). While the first build can take some time, future ones will be much faster—so no need to worry. With everything passing, you’re ready to submit your changes and contribute to Milvus!\n\n\n### Link Your Pull Request (PR) to an Issue\n\nEvery PR to Milvus needs to be tied to a relevant issue. Here's how to handle this:\n\n- **Check for Existing Issues:** Look through the[ Milvus issue tracker](https://github.com/milvus-io/milvus/issues) to see if there’s already an issue related to your changes.\n\n- **Create a New Issue:** If no relevant issue exists, open a new one and explain the problem you’re solving or the feature you’re adding.\n\n\n### Submitting Your Code\n\n1. **Fork the Repository:** Start by forking the[ Milvus repo](https://github.com/milvus-io/milvus) to your GitHub account.\n\n2. **Create a Branch:** Clone your fork locally and make a new branch for your changes.\n\n3. **Commit with Signed-off-by Signature:** Ensure your commits include a `Signed-off-by` signature to comply with open-source licensing:\n\n\n```\ngit commit -m \"Commit of your change\" -s\n```\n\n\nThis step certifies your contribution is in line with the Developer Certificate of Origin (DCO).\n\n\n#### **Helpful Resources**\n\nFor detailed steps and best practices, check out the[ Milvus Contribution Guide](https://github.com/milvus-io/milvus/blob/master/CONTRIBUTING.md).\n\n\n## Opportunities to Contribute\n\nCongrats—you’ve got Milvus up and running! You’ve explored its deployment modes, run your tests, and maybe even dug into the code. Now it’s time to level up: contribute to [Milvus](https://github.com/milvus-io/milvus) and help shape the future of AI and [unstructured data](https://zilliz.com/learn/introduction-to-unstructured-data).\n\nNo matter your skillset, there’s a place for you in the Milvus community! Whether you’re a developer who loves solving complex challenges, a tech writer who loves writing clean documentation or engineering blogs, or a Kubernetes enthusiast looking to improve deployments, there’s a way for you to make an impact.\n\nTake a look at the opportunities below and find your perfect match. Every contribution helps move Milvus forward—and who knows? Your next pull request might just power the next wave of innovation. So, what are you waiting for? Let’s get started! 🚀\n\n| Projects | Suitable for | Guidelines |\n| ----------------------------------------------------------------------------------------------------------------------------------------------------------------------------------- | ---------------------------------------- | --------------------------------------------------------------------------------------------------- |\n| [milvus](https://github.com/milvus-io/milvus), [milvus-sdk-go](https://github.com/milvus-io/milvus-sdk-go) | Go developers | / |\n| [milvus](https://github.com/milvus-io/milvus), [knowhere](https://github.com/milvus-io/knowhere) | CPP developers | / |\n| [pymilvus](https://github.com/milvus-io/pymilvus), [milvus-sdk-node](https://github.com/milvus-io/milvus-sdk-node), [milvus-sdk-java](https://github.com/milvus-io/milvus-sdk-java) | Developers interested in other languages | [Contributing to PyMilvus](https://github.com/milvus-io/pymilvus/blob/master/CONTRIBUTING.md) |\n| [milvus-helm](https://github.com/milvus-io/milvus-helm) | Kubernetes enthusiasts | / |\n| [Milvus-docs](https://github.com/milvus-io/milvus-docs), [milvus-io/community/blog](https://github.com/milvus-io/community) | Tech writers | [Contributing to milvus docs](https://github.com/milvus-io/milvus-docs/blob/v2.0.0/CONTRIBUTING.md) |\n| [milvus-insight](https://github.com/zilliztech/milvus-insight) | Web developers | / |\n\n\n## A Final Word\n\nMilvus offers various SDKs—[Python](https://milvus.io/docs/install-pymilvus.md) (PyMilvus), [Java](https://milvus.io/docs/install-java.md), [Go](https://milvus.io/docs/install-go.md), and [Node.js](https://milvus.io/docs/install-node.md)—that make it simple to start building. Contributing to Milvus isn’t just about code—it’s about joining a vibrant and innovative community. \n\n🚀Welcome to the Milvus developer community, and happy coding! We can’t wait to see what you’ll create. \n\n\n## Further Reading\n\n- [Join the Milvus Community of AI Developers](https://zilliz.com/community)\n\n- [What are Vector Databases and How Do They Work?](https://zilliz.com/learn/what-is-vector-database)\n\n- [Milvus Lite vs. Standalone vs. Distributed: Which Mode is Right for You? ](https://zilliz.com/blog/choose-the-right-milvus-deployment-mode-ai-applications)\n\n- [Build AI Apps with Milvus: Tutorials \u0026 Notebooks](https://zilliz.com/learn/milvus-notebooks)\n\n- [Top Performing AI Models for Your GenAI Apps | Zilliz](https://zilliz.com/ai-models)\n\n- [What is RAG?](https://zilliz.com/learn/Retrieval-Augmented-Generation)\n\n- [Generative AI Resource Hub | Zilliz](https://zilliz.com/learn/generative-ai)","title":"How to Contribute to Milvus: A Quick Start for Developers","metaData":{}},{"id":"getting-started-with-hnswlib.md","author":"Haziqa Sajid","desc":"HNSWlib, a library implementing HNSW, is highly efficient and scalable, performing well even with millions of points. Learn how to implement it in minutes.","metaTitle":"Getting Started with HNSWlib","tags":["Engineering"],"recommend":true,"canonicalUrl":"https://milvus.io/blog/getting-started-with-hnswlib.md","date":"2024-11-25T00:00:00.000Z","cover":"https://assets.zilliz.com/Getting_Started_with_HNS_Wlib_30922def3e.png","href":"/blog/getting-started-with-hnswlib.md","content":"\r\n\r\n\r\n[Semantic search](https://zilliz.com/glossary/semantic-search) allows machines to understand language and yield better search results, which is essential in AI and data analytics. Once the language is represented as [embeddings](https://zilliz.com/learn/everything-you-should-know-about-vector-embeddings), the search can be performed using exact or approximate methods. Approximate Nearest Neighbor ([ANN](https://zilliz.com/glossary/anns)) search is a method used to quickly find points in a dataset that are closest to a given query point, unlike [exact nearest neighbor search](https://zilliz.com/blog/k-nearest-neighbor-algorithm-for-machine-learning), which can be computationally expensive for high-dimensional data. ANN allows faster retrieval by providing results that are approximately close to the nearest neighbors. \r\n\r\nOne of the algorithms for Approximate Nearest Neighbor (ANN) search is [HNSW](https://zilliz.com/learn/hierarchical-navigable-small-worlds-HNSW) (Hierarchical Navigable Small Worlds), implemented under [HNSWlib](https://zilliz.com/learn/learn-hnswlib-graph-based-library-for-fast-anns), which will be the focus of today's discussion. In this blog, we will:\r\n\r\n- Understand the HNSW algorithm.\r\n\r\n- Explore HNSWlib and its key features.\r\n\r\n- Set up HNSWlib, covering index building and search implementation.\r\n\r\n- Compare it with Milvus.\r\n\r\n\r\n## Understanding HNSW\r\n\r\n**Hierarchical Navigable Small Worlds (**[**HNSW**](https://zilliz.com/learn/hierarchical-navigable-small-worlds-HNSW)**)** is a graph-based data structure that allows efficient similarity searches, particularly in high-dimensional spaces, by building a multi-layered graph of \"small world\" networks. Introduced in [2016](https://arxiv.org/abs/1603.09320), HNSW addresses the scalability issues associated with traditional search methods like brute-force and tree-based searches. It is ideal for applications involving large datasets, such as recommendation systems, image recognition, and [retrieval-augmented generation (RAG)](https://zilliz.com/vector-database-use-cases/llm-retrieval-augmented-generation).\r\n\r\n\r\n### Why HNSW Matters\r\n\r\nHNSW significantly enhances the performance of nearest-neighbor search in high-dimensional spaces. Combining the hierarchical structure with small-world navigability avoids the computational inefficiency of older methods, enabling it to perform well even with massive, complex datasets. To understand this better, let’s look at how it works now.\r\n\r\n\r\n### How HNSW Works\r\n\r\n1. **Hierarchical Layers:** HNSW organizes data into a hierarchy of layers, where each layer contains nodes connected by edges. The top layers are sparser, allowing for broad \"skips\" across the graph, much like zooming out on a map to see only major highways between cities. Lower layers increase in density, providing finer detail and more connections between closer neighbors.\r\n\r\n2. **Navigable Small Worlds Concept:** Each layer in HNSW builds on the concept of a \"small world\" network, where nodes (data points) are only a few \"hops\" away from each other. The search algorithm begins at the highest, sparsest layer and works downward, moving to progressively denser layers to refine the search. This approach is like moving from a global view down to neighborhood-level details, gradually narrowing the search area.\r\n\r\n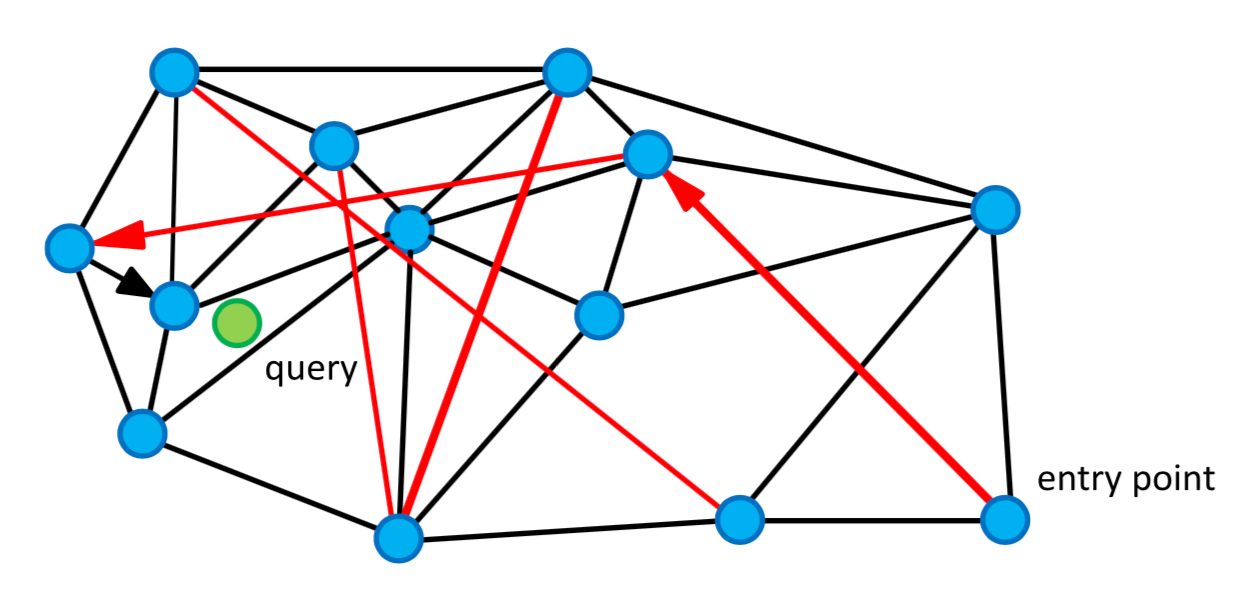\r\n\r\n[Fig 1](https://daniel-at-world.blogspot.com/2019/04/navigable-small-world-graphs-for.html): An Example of a Navigable Small World Graph\r\n\r\n3. **Skip List-Like Structure:** The hierarchical aspect of HNSW resembles a skip list, a probabilistic data structure where higher layers have fewer nodes, allowing for faster initial searches. \r\n\r\n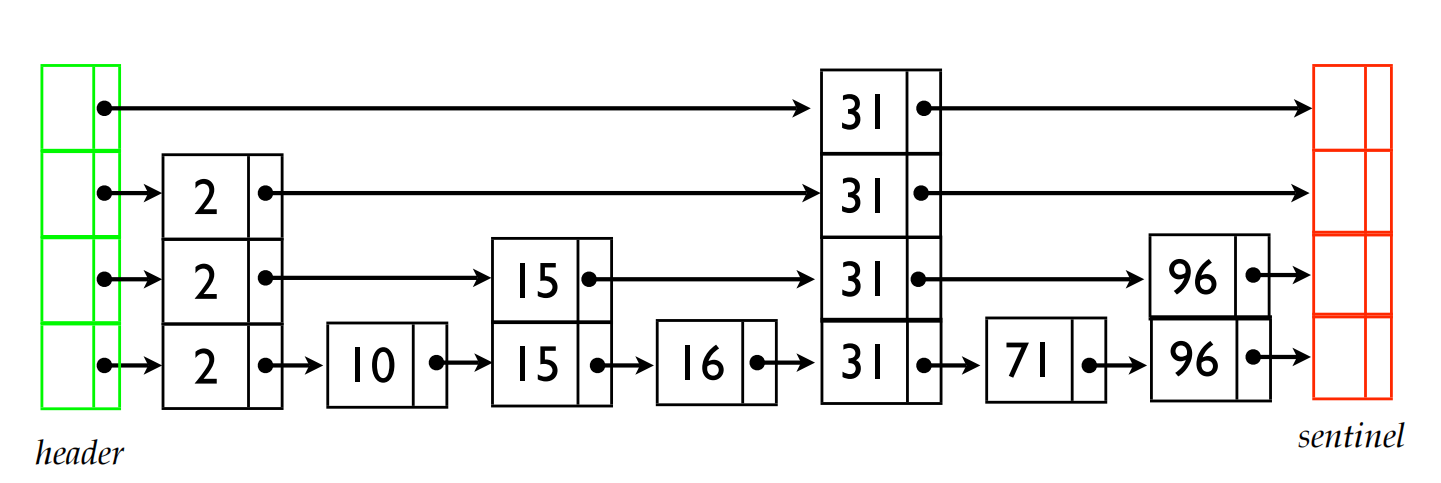\r\n\r\n[Fig 2](https://www.cs.cmu.edu/~ckingsf/bioinfo-lectures/skiplists.pdf): An Example of Skip List Structure\r\n\r\nTo search for 96 in the given skip list, we begin at the top level on the far left at the header node. Moving to the right, we encounter 31, less than 96, so we continue to the next node. Now, we need to move down a level where we see 31 again; since it’s still less than 96, we descend another level. Finding 31 once more, we then move right and reach 96, our target value. Thus, we locate 96 without needing to descend to the lowest levels of the skip list.\r\n\r\n4. **Search Efficiency:** The HNSW algorithm starts from an entry node at the highest layer, progressing to closer neighbors with each step. It descends through the layers, using each one for coarse-to-fine-grained exploration, until it reaches the lowest layer where the most similar nodes are likely found. This layered navigation reduces the number of nodes and edges that need to be explored, making the search fast and accurate.\r\n\r\n5. **Insertion and Maintenance**: When adding a new node, the algorithm determines its entry layer based on probability and connects it to nearby nodes using a neighbor selection heuristic. The heuristic aims to optimize connectivity, creating links that improve navigability while balancing graph density. This approach keeps the structure robust and adaptable to new data points.\r\n\r\nWhile we have a foundational understanding of the HNSW algorithm, implementing it from scratch can be overwhelming. Fortunately, the community has developed libraries like [HNSWlib](https://github.com/nmslib/hnswlib) to simplify usage, making it accessible without scratching your head. So, let’s take a closer look at HNSWlib.\r\n\r\n\r\n## Overview of HNSWlib\r\n\r\nHNSWlib, a popular library implementing HNSW, is highly efficient and scalable, performing well even with millions of points. It achieves sublinear time complexity by allowing quick jumps between graph layers and optimizing the search for dense, high-dimensional data. Here are the key features of HNSWlib include:\r\n\r\n- **Graph-Based Structure:** A multi-layered graph represents data points, allowing fast, nearest-neighbor searches.\r\n\r\n- **High-Dimensional Efficiency:** Optimized for high-dimensional data, providing quick and accurate approximate searches.\r\n\r\n- **Sublinear Search Time:** Achieves sublinear complexity by skipping layers, improving speed significantly.\r\n\r\n- **Dynamic Updates:** Supports real-time insertion and deletion of nodes without requiring a complete graph rebuild.\r\n\r\n- **Memory Efficiency:** Efficient memory usage, suitable for large datasets.\r\n\r\n- **Scalability:** Scales well to millions of data points, making it ideal for medium-scale applications like recommendation systems.\r\n\r\n**Note:** HNSWlib is excellent for creating simple prototypes for vector search applications. However, due to scalability limitations, there may be better choices such as [purpose-built vector databases](https://zilliz.com/blog/what-is-a-real-vector-database) for more complex scenarios involving hundreds of millions or even billions of data points. Let’s see that in action.\r\n\r\n\r\n## Getting Started with HNSWlib: A Step-by-Step Guide\r\n\r\nThis section will demonstrate using HNSWlib as a [vector search library](https://zilliz.com/learn/comparing-vector-database-vector-search-library-and-vector-search-plugin) by creating an HNSW index, inserting data, and performing searches. Let’s start with installation:\r\n\r\n\r\n### Setup and Imports\r\n\r\nTo get started with HNSWlib in Python, first install it using pip:\r\n\r\n```\r\npip install hnswlib\r\n```\r\n\r\nThen, import the necessary libraries:\r\n\r\n```\r\nimport hnswlib \r\nimport numpy as np\r\n```\r\n\r\n### Preparing Data\r\n\r\nIn this example, we’ll use `NumPy`to generate a random dataset with 10,000 elements, each with a dimension size 256.\r\n\r\n```\r\ndim = 256 # Dimensionality of your vectors\r\nnum_elements = 10000 # Number of elements to insert\r\n```\r\n\r\nLet’s create the data:\r\n\r\n```\r\ndata = np.random.rand(num_elements, dim).astype(np.float32) # Example data\r\n```\r\n\r\nNow our data is ready, let’s build an index.\r\n\r\n\r\n### Building an Index\r\n\r\nIn building an index, we need to define the dimensionality of the vectors and the space type. Let’s create an index:\r\n\r\n```\r\np = hnswlib.Index(space='l2', dim=dim)\r\n```\r\n- `space='l2'`: This parameter defines the distance metric used for similarity. Setting it to `'l2'` means using the Euclidean distance (L2 norm). If you instead set it to `'ip'`, it would use the inner product, which is helpful for tasks like cosine similarity.\r\n\r\n* `dim=dim`: This parameter specifies the dimensionality of the data points you’ll be working with. It must match the dimension of the data you plan to add to the index.\r\n\r\nHere’s how to initialize an index:\r\n\r\n```\r\np.init_index(max_elements=num_elements, ef_construction=200, M=16)\r\n```\r\n\r\n- `max_elements=num_elements`: This sets the maximum number of elements that can be added to the index. `Num_elements` is the maximum capacity, so we set this to 10,000 as we are working with 10,000 data points.\r\n\r\n* `ef_construction=200`: This parameter controls the accuracy vs. construction speed trade-off during index creation. A higher value improves recall (accuracy) but increases memory usage and build time. Common values range from 100 to 200.\r\n\r\n- `M=16`: This parameter determines the number of bi-directional links created for each data point, influencing accuracy and search speed. Typical values are between 12 and 48; 16 is often a good balance for moderate accuracy and speed.\r\n\r\n```\r\np.set_ef(50) # This parameter controls the speed/accuracy trade-off\r\n```\r\n\r\n- `ef`: The `ef` parameter, short for “exploration factor,” determines how many neighbors are examined during a search. A higher `ef` value results in more neighbors being explored, which generally increases the accuracy (recall) of the search but also makes it slower. Conversely, a lower `ef` value can search faster but might reduce accuracy.\r\n\r\nIn this case, Setting `ef` to 50 means the search algorithm will evaluate up to 50 neighbors when finding the most similar data points.\r\n\r\nNote: `ef_construction` sets neighbor search effort during index creation, enhancing accuracy but slowing construction. `ef` controls search effort during querying, balancing speed and recall dynamically for each query.\r\n\r\n\r\n### Performing Searches\r\n\r\nTo perform a nearest neighbor search using HNSWlib, we first create a random query vector. In this example, the vector's dimensionality matches the indexed data.\r\n\r\n```\r\nquery_vector = np.random.rand(dim).astype(np.float32) # Example query\r\n\r\nlabels, distances = p.knn_query(query_vector, k=5) # k is the number of nearest neighbors\r\n```\r\n\r\n- `query_vector`: This line generates a random vector with the same dimensionality as the indexed data, ensuring compatibility for the nearest neighbor search.\r\n- `knn_query`: The method searches for the `k` nearest neighbors of the `query_vector` within the index `p`. It returns two arrays: `labels`, which contain the indices of the nearest neighbors, and `distances`, which indicate the distances from the query vector to each of these neighbors. Here, `k=5` specifies that we want to find the five closest neighbors.\r\n\r\nHere are the results after printing the labels and distances:\r\n\r\n```\r\nprint(\"Nearest neighbors' labels:\", labels)\r\nprint(\"Distances:\", distances)\r\n```\r\n\r\n```\r\n\u003e Nearest neighbors' labels: [[4498 1751 5647 4483 2471]]\r\n\u003e Distances: [[33.718 35.484592 35.627766 35.828312 35.91495 ]]\r\n```\r\n\r\nHere we have it, a simple guide to get your wheels started with HNSWlib. \r\n\r\nAs mentioned, HNSWlib is a great vector search engine for prototyping or experimenting with medium-sized datasets. If you have higher scalability requirements or need other enterprise-level features, you may need to choose a purpose-built vector database like the open-source [Milvus](https://zilliz.com/what-is-milvus) or its fully managed service on [Zilliz Cloud](https://zilliz.com/cloud). So, in the following section, we will compare HNSWlib with Milvus. \r\n\r\n\r\n## HNSWlib vs. Purpose-Built Vector Databases Like Milvus\r\n\r\nA [vector database](https://zilliz.com/learn/what-is-vector-database) stores data as mathematical representations, enabling [machine learning models](https://zilliz.com/ai-models) to power search, recommendations, and text generation by identifying data through [similarity metrics](https://zilliz.com/blog/similarity-metrics-for-vector-search) for contextual understanding. \r\n\r\nVector indices libraries like HNSWlib improve v[ector search](https://zilliz.com/learn/vector-similarity-search) and retrieval but lack the management features of a full database. On the other hand, vector databases, like [Milvus](https://milvus.io/), are designed to handle vector embeddings at scale, providing advantages in data management, indexing, and querying capabilities that standalone libraries typically lack. Here are some other benefits of using Milvus:\r\n\r\n- **High-Speed Vector Similarity Search**: Milvus provides millisecond-level search performance across billion-scale vector datasets, ideal for applications like image retrieval, recommendation systems, natural language processing ([NLP](https://zilliz.com/learn/A-Beginner-Guide-to-Natural-Language-Processing)), and retrieval augmented generation ([RAG](https://zilliz.com/learn/Retrieval-Augmented-Generation)). \r\n\r\n- **Scalability and High Availability:** Built to handle massive data volumes, Milvus scales horizontally and includes replication and failover mechanisms for reliability.\r\n\r\n- **Distributed Architecture:** Milvus uses a distributed, scalable architecture that separates storage and computing across multiple nodes for flexibility and robustness.\r\n\r\n- [**Hybrid search**](https://zilliz.com/blog/a-review-of-hybrid-search-in-milvus)**:** Milvus supports multimodal search, hybrid [sparse and dense search](https://zilliz.com/learn/sparse-and-dense-embeddings), and hybrid dense and [full-text search](https://thenewstack.io/elasticsearch-was-great-but-vector-databases-are-the-future/), offering versatile and flexible search functionality.\r\n\r\n- **Flexible Data Support**: Milvus supports various data types—vectors, scalars, and structured data—allowing seamless management and analysis within a single system.\r\n\r\n- [**Active Community**](https://discord.com/invite/8uyFbECzPX) **and Support**: A thriving community provides regular updates, tutorials, and support, ensuring Milvus remains aligned with user needs and advances in the field. \r\n\r\n- [AI integration](https://milvus.io/docs/integrations_overview.md): Milvus has integrated with various popular AI frameworks and technologies, making it easier for developers to build applications with their familiar tech stacks. \r\n\r\nMilvus also provides a fully managed service on [Ziliz Cloud](https://zilliz.com/cloud), which is hassle-free and 10x faster than Milvus. \r\n\r\n\r\n### Comparison: Milvus vs. HNSWlib\r\n\r\n| **Feature** | **Milvus** | **HNSWlib** |\r\n| :---------: | :---------------------------------------------------------------------------------: | :---------------------------------------------: |\r\n| Scalability | Handles billions of vectors with ease | Fit for smaller datasets due to RAM usage |\r\n| Ideal for | Prototyping, experimenting, and enterprise-level applications | Focuses on prototypes and lightweight ANN tasks |\r\n| Indexing | Supports 10+ indexing algorithms, including HNSW, DiskANN, Quantization, and Binary | Uses a graph-based HNSW only |\r\n| Integration | Offers APIs and cloud-native services | Serves as a lightweight, standalone library |\r\n| Performance | Optimizes for large data, distributed queries | Offers high speed but limited scalability |\r\n\r\nOverall, Milvus is generally preferable for large-scale, production-grade applications with complex indexing needs, while HNSWlib is ideal for prototyping and more straightforward use cases.\r\n\r\n\r\n## Conclusion\r\n\r\nSemantic search can be resource-intensive, so internal data structuring, like that performed by HNSW, is essential for faster data retrieval. Libraries like HNSWlib care about the implementation, so the developers have the recipes ready to prototype vector capabilities. With just a few lines of code, we can build up our own index and perform searches.\r\n\r\nHNSWlib is a great way to start. However, if you want to build complex and production-ready AI applications, purpose-built vector databases are the best option. For example, [Milvus](https://milvus.io/) is an open-source vector database with many enterprise-ready features such as high-speed vector search, scalability, availability, and flexibility in terms of data types and programming language. \r\n\r\n\r\n## Further Reading\r\n\r\n- [What is Faiss (Facebook AI Similarity Search)? ](https://zilliz.com/learn/faiss)\r\n\r\n- [What is HNSWlib? A Graph-based Library for Fast ANN Search ](https://zilliz.com/learn/learn-hnswlib-graph-based-library-for-fast-anns)\r\n\r\n- [What is ScaNN (Scalable Nearest Neighbors)? ](https://zilliz.com/learn/what-is-scann-scalable-nearest-neighbors-google)\r\n\r\n- [VectorDBBench: An Open-Source VectorDB Benchmark Tool](https://zilliz.com/vector-database-benchmark-tool?database=ZillizCloud%2CMilvus%2CElasticCloud%2CPgVector%2CPinecone%2CQdrantCloud%2CWeaviateCloud\u0026dataset=medium\u0026filter=none%2Clow%2Chigh\u0026tab=1)\r\n\r\n- [Generative AI Resource Hub | Zilliz](https://zilliz.com/learn/generative-ai)\r\n\r\n- [What are Vector Databases and How Do They Work? ](https://zilliz.com/learn/what-is-vector-database)\r\n\r\n- [What is RAG? ](https://zilliz.com/learn/Retrieval-Augmented-Generation)\r\n\r\n- [Top Performing AI Models for Your GenAI Apps | Zilliz](https://zilliz.com/ai-models)\r\n\r\n","title":"Getting Started with HNSWlib","metaData":{}},{"id":"join-milvus-office-hours-to-get-support-from-vectordb-experts.md","author":"Emily Kurze","tags":["Events"],"recommend":true,"canonicalUrl":"https://milvus.io/blog/join-milvus-office-hours-to-get-support-from-vectordb-experts.md","date":"2024-11-08T00:00:00.000Z","cover":"https://assets.zilliz.com/Join_Milvus_Office_Hours_to_Get_Support_from_Vector_DB_Experts_1_64f88f0607.png","href":"/blog/join-milvus-office-hours-to-get-support-from-vectordb-experts.md","content":"\nGet expert help with your Milvus [vector database](https://zilliz.com/learn/what-is-vector-database) projects. Book a 20-minute one-on-one session to get insights, guidance, and answers to your questions. Whether you’re just getting started or need help with a specific issue, we’re here to help you make the most out of Milvus.\n\n[](https://meetings.hubspot.com/chloe-williams1/milvus-office-hours)\n\n\n## Why Book a Session?\n\nHere are a few great reasons to book time during our office hours:\n\n- **Optimizing Milvus Performance:** Get tips on tuning configurations to boost database performance.\n\n- **Data Schema Design Advice:** Learn best practices for data schema and index types to best fit your use case.\n\n- **Scaling Your Milvus Deployment:** Get advice on managing and scaling Milvus for larger data volumes.\n\n- **Troubleshooting Specific Issues:** Stuck on an error or unusual behavior? We can help troubleshoot and resolve it.\n\n- **Integration Assistance:** Need guidance on using Milvus with other integrated frameworks like LangChain, LlamaIndex, Spark, or your data processing pipelines?\n\n- **Learning about New Features:** Stay updated with the latest Milvus capabilities and how they could benefit your project.\n\nDon’t miss this opportunity to level up your Milvus implementation with expert guidance.\n\nIf you’re unable to join us live, don’t worry—there are still plenty of ways to get support! The Milvus community is active and ready to help on both [Discord](https://discord.com/invite/8uyFbECzPX) and[ GitHub Discussions](https://github.com/search?q=milvus\u0026type=discussions). Whether you prefer live chat or asynchronous discussions, these platforms are a great place to ask questions, share knowledge, and connect with other Milvus users and contributors.\n\n","title":"Join Milvus Office Hours to Get Support from Vector DB Experts!","metaData":{}},{"id":"matryoshka-embeddings-detail-at-multiple-scales.md","author":"Stefan Webb, David Wang","desc":"Embeddings with shortened dimensions without sacrificing semantic integrity, ideal for more efficient search and storage.","metaTitle":"What are Matryoshka Embeddings?","tags":["Engineering"],"recommend":true,"canonicalUrl":"https://milvus.io/blog/matryoshka-embeddings-detail-at-multiple-scales","date":"2024-10-30T00:00:00.000Z","cover":"https://assets.zilliz.com/Introduction_to_Matryoshka_Embedding_e5a5bc2056.png","href":"/blog/matryoshka-embeddings-detail-at-multiple-scales.md","content":"\n\n## What are Matryoshka Embeddings? \n\nWhen building efficient [vector search](https://zilliz.com/learn/vector-similarity-search) systems, one key challenge is managing storage costs while maintaining acceptable latency and recall. Modern [embedding models](https://zilliz.com/blog/choosing-the-right-embedding-model-for-your-data) output vectors with hundreds or thousands of dimensions, creating significant storage and computational overhead for the raw vector and index.\n\nTraditionally, the storage requirements are reduced by applying a quantization or dimensionality reduction method just before building the index. For instance, we can save storage by lowering the precision using [Product Quantization](https://zilliz.com/learn/scalar-quantization-and-product-quantization) (PQ) or the number of dimensions using Principal Component Analysis (PCA). These methods analyze the entire vector set to find a more compact one that maintains the semantic relationships between vectors.\n\nWhile effective, these standard approaches reduce precision or dimensionality only once and at a single scale. But what if we could maintain multiple layers of detail simultaneously, like a pyramid of increasingly precise representations?\n\nEnter [**Matryoshka embeddings**](https://arxiv.org/abs/2205.13147). Named after Russian nesting dolls (see illustration), these clever constructs embed multiple scales of representation within a single vector. Unlike traditional post-processing methods, Matryoshka embeddings learn this multi-scale structure during the initial training process. The result is remarkable: **not only does the full embedding capture input semantics, but each nested subset prefix (first half, first quarter, etc.) provides a coherent, if less detailed, representation.**\n\n\n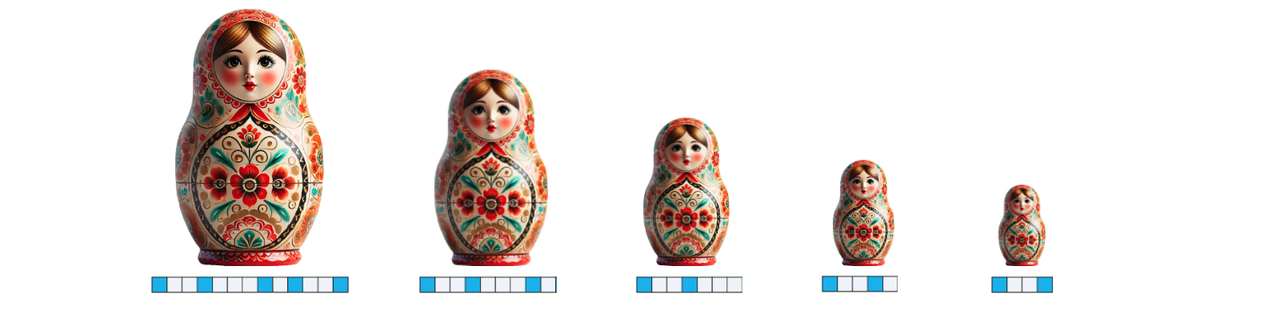\n\n_Figure: Visualization of Matryoshka embeddings with multiple layers of detail_\n\nThis approach contrasts sharply with conventional [embeddings](https://zilliz.com/glossary/vector-embeddings), where using arbitrary subsets of the vector dimensions typically destroys semantic meaning. With Matryoshka embeddings, you can choose the granularity that best balances your specific task's precision and computational cost. \n\nNeed a quick approximate search? Use the smallest \"doll.\" Need maximum accuracy? Use the full embedding. This flexibility makes them particularly valuable for systems adapting to different performance requirements or resource constraints.\n\n\n## Inference\n\nA valuable application of Matryoshka embeddings is accelerating similarity searches without sacrificing recall. By leveraging smaller subsets of query and database embeddings—such as the first 1/32 of their dimensions—we can build an index over this reduced space that still preserves much of the similarity information. Initial results from this smaller embedding space can be used directly. Still, there’s also a technique to boost recall and account for any minor reduction from the dimensional cutback, making this approach both efficient and effective for similarity search tasks.\n\n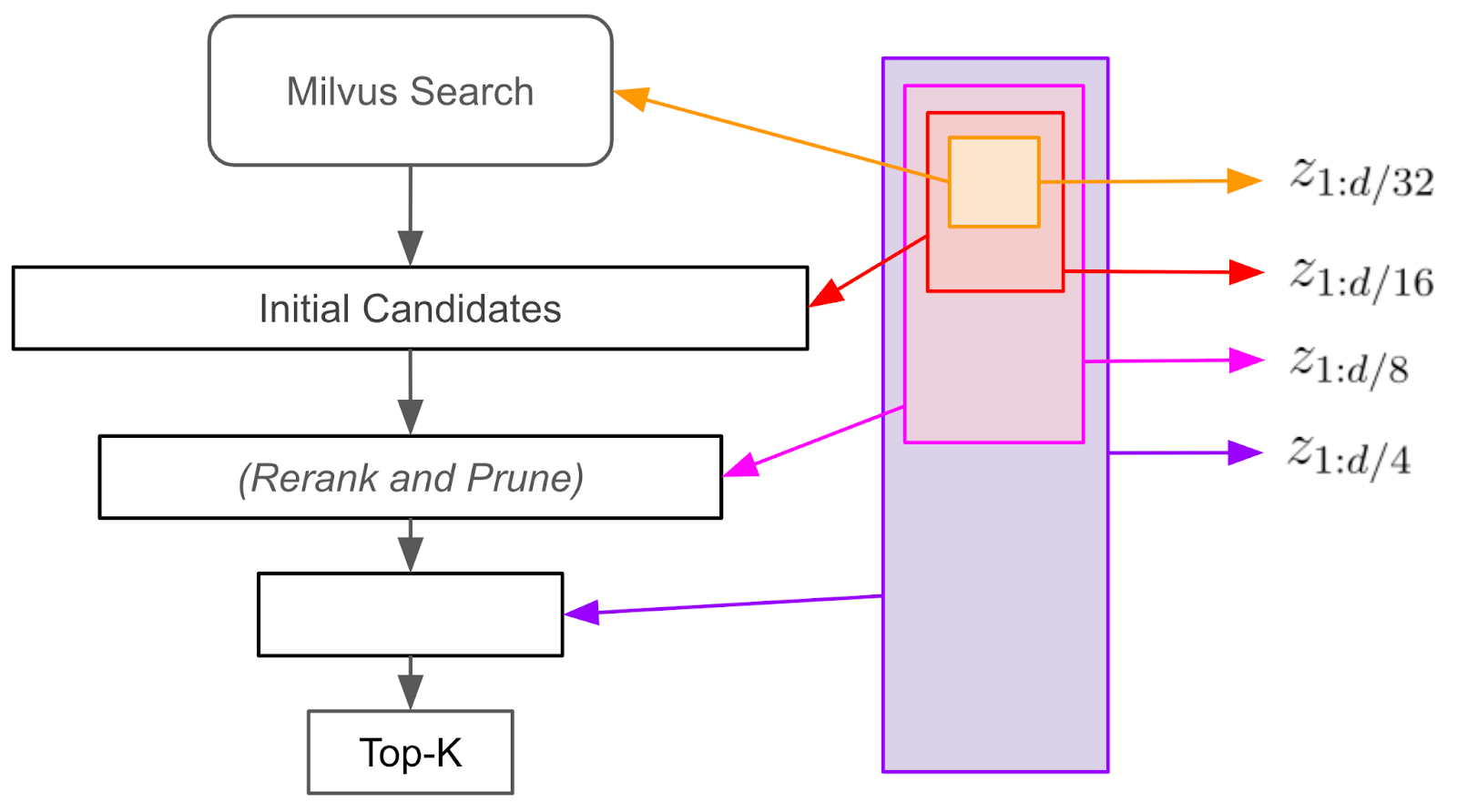\n\n_Figure: How the funnel search works with Matryoshka embeddings_ \n\nTo efficiently speed up similarity search while maintaining accuracy, we can use a \"funnel search\" approach. First, we perform an initial similarity search using only the first 1/32 of the embedding dimensions, generating a broad pool of candidate items. We then rerank these candidates based on their similarity to the query using the first 1/16 of the dimensions, pruning a portion of the list. This process continues iteratively, reranking and pruning with increasingly larger subsets of the embedding dimensions—1/8, 1/4, and so on. Importantly, we only perform one initial similarity search in this lower-dimensional space, and a single pass of the embedding model computes the query embedding. This funneling process narrows down candidates at each step and is faster and more efficient than directly searching in the full-dimensional space. Drawing many matches from the 1/32-dimensional space and refining them through funnel search can significantly accelerate similarity search while preserving strong recall.\n\n\n## Training\n\nLet’s go into a few of the technical details. The method is very simple to apply. Consider the context of fine-tuning a [BERT model](https://zilliz.com/learn/what-is-bert) for sentence embedding. To convert a BERT model, which has been pre-trained on the masked-token loss, into a sentence embedding model, we form the sentence embedding as the average of the final layer, that is, the average of the per-token contextualized embeddings. \n\nOne choice of training objective is the [Cosine Sentence (CoSENT) loss](https://sbert.net/docs/package_reference/sentence_transformer/losses.html#cosentloss), $L(u, v; s)$. It inputs a pair of sentence embeddings, $u,v$, and their desired similarity score, $s$ (see the link above for the formula). Now, to learn Matryoshka embeddings, we make a small modification to the training objective:\n\n$L_M(u, v) = w_0L(u_{1:d}, v_{1:d}) + w_1L(u_{1:d/2}, v_{1:d/2}) + w_2L(u_{1:d/4}, v_{1:d/4}) + \\cdots$\n\nwhere the sum is continued by calculating the loss on half of the input to the previous term until an information bottleneck is reached. The authors suggest setting\n\n$w_0=w_1=\\cdots=1$.\n\n_Simply put, the Matryoshka loss is a weighted sum of the original loss over recursive subsets of the input._\n\nOne key takeaway from the equation above is that the Matryoshka loss achieves efficient learning of representations at multiple scales by sharing weights across the embedding models (the same model is used to encode, for example, $u_{1:d}$ and $u_{1:d/2}$) and sharing dimensions across scales ($u_{1:d/2}$ is a subset of $u$).\n\n\n## Matryoshka Embeddings and Milvus\n\nMilvus seamlessly supports any Matryoshka embedding model that can be loaded via standard libraries such as [pymilvus.model](https://milvus.io/docs/embeddings.md), [sentence-transformers](https://milvus.io/docs/integrate_with_sentencetransformers.md), or other similar tools. From the system’s perspective, there’s no functional difference between a regular embedding model and one specifically trained to generate Matryoshka embeddings. \n\nPopular Matryoshka embedding models include: \n\n- OpenAI's [`text-embedding-3-large`](https://zilliz.com/ai-models/text-embedding-3-large)\n\n- Nomic’s [`nomic-embed-text-v1`](https://huggingface.co/nomic-ai/nomic-embed-text-v1)\n\n- Alibaba’s [`gte-multilingual-base`](https://huggingface.co/Alibaba-NLP/gte-multilingual-base)\n\nFor a complete guide on using Matryoshka embeddings with Milvus, see the notebook _[Funnel Search with Matryoshka Embeddings](https://github.com/milvus-io/bootcamp/blob/master/bootcamp/tutorials/quickstart/funnel_search_with_matryoshka.ipynb)_.\n\n\n## Summary\n\nMatryoshka embedding lets developers create shortened embeddings without sacrificing semantic integrity, making them ideal for more efficient search and storage. While you can modify an existing model, pre-trained options, such as those from [OpenAI](https://zilliz.com/ai-models) and [Hugging Face](https://zilliz.com/ai-models), are also available. \n\nHowever, a current limitation is the scarcity of open-source Matryoshka embeddings, with few available on the Hugging Face hub. Additionally, these models are often not explicitly labeled as “Matryoshka,” making them harder to locate. Hopefully, with growing interest, broader availability and clearer labeling may soon follow. \n\nReady to streamline your search capabilities? Get started with Milvus + Matryoshka embeddings today!\n\n\n## Resources\n\n- Notebook: [Funnel Search with Matryoshka Embeddings](https://github.com/milvus-io/bootcamp/blob/master/bootcamp/tutorials/quickstart/funnel_search_with_matryoshka.ipynb) \n\n- Paper: [Matryoshka Representation Learning](https://arxiv.org/abs/2205.13147)\n\n- Paper: [mGTE: Generalized Long-Context Text Representation and Reranking Models for Multilingual Text Retrieval](https://arxiv.org/pdf/2407.19669)\n\n- [Introducing PyMilvus Integration with Embedding Models ](https://milvus.io/blog/introducing-pymilvus-integrations-with-embedding-models.md)\n\n- [Exploring BGE-M3: The Future of Information Retrieval with Milvus ](https://zilliz.com/learn/Exploring-BGE-M3-the-future-of-information-retrieval-with-milvus)\n\n- [Nomic Embed: Training a Reproducible Long Context Text Embedder](https://static.nomic.ai/reports/2024_Nomic_Embed_Text_Technical_Report.pdf)\n\n- [Training Matryoshka Embeddings with Sentence Transformers Library](https://sbert.net/examples/training/matryoshka/README.html)\n\n- [Milvus Bootcamp](https://milvus.io/bootcamp)\n\n","title":"Matryoshka Embeddings: Detail at Multiple Scales","metaData":{}},{"id":"how-to-use-milvus-backup-tool-step-by-step-guide.md","author":"Michael Mo","desc":"This guide will walk you through the process of using Milvus Backup, ensuring that you can confidently handle your backup needs.","tags":["Engineering"],"recommend":true,"canonicalUrl":"https://milvus.io/blog/how-to-use-milvus-backup-tool-step-by-step-guide.md","date":"2024-09-27T00:00:00.000Z","cover":"https://assets.zilliz.com/How_to_Use_the_Milvus_Backup_Tool_A_Step_by_Step_Guide_411029fa4b.png","href":"/blog/how-to-use-milvus-backup-tool-step-by-step-guide.md","content":"\n[**Milvus**](https://milvus.io/docs/overview.md) is an open-source, high-performance, and highly scalable [vector database](https://zilliz.com/learn/what-is-vector-database) that can store, index, and search billion-scale [unstructured data](https://zilliz.com/learn/introduction-to-unstructured-data) through high-dimensional [vector embeddings](https://zilliz.com/glossary/vector-embeddings). It is perfect for building modern AI applications such as retrieval augmented generation ([RAG](https://zilliz.com/learn/Retrieval-Augmented-Generation)), semantic search, [multimodal search](https://zilliz.com/blog/multimodal-rag-expanding-beyond-text-for-smarter-ai), and recommendation systems. Milvus runs efficiently across various [environments](https://zilliz.com/blog/choose-the-right-milvus-deployment-mode-ai-applications), from laptops to large-scale distributed systems. It is available as open-source software and a cloud service.\n\n[**Milvus Backup**](https://milvus.io/docs/milvus_backup_overview.md) is a tool for backing up and restoring Milvus data. It provides both CLI and API to accommodate different application scenarios. This guide will walk you through the process of using Milvus Backup, ensuring that you can confidently handle your backup needs.\n\n\n## Preparation\n\nBefore starting the backup or restore process, you need to set up your environment:\n\n**1. Download the latest binary** from the[ Milvus-backup repository releases](https://github.com/zilliztech/milvus-backup/releases). Select the appropriate version for your operating system:\n\n- For macOS: `milvus-backup_Darwin_arm64.tar.gz` or `milvus-backup_Darwin_x86_64.tar.gz`\n\n- For Linux: `milvus-backup_Linux_arm64.tar.gz` or `milvus-backup_Linux_x86_64.tar.gz`\n\n**2. Download the configuration file** from [GitHub](https://github.com/zilliztech/milvus-backup/blob/main/configs/backup.yaml).\n\n**3. Extract the tar file** to your preferred directory and place the `backup.yaml` in the `configs/` directory within the same extracted folder. Ensure your directory structure appears as follows:\n\n```\n├── configs\n│ └── backup.yaml\n├── milvus-backup\n└── README.md\n```\n\n\n## Command Overview\n\nNavigate to your terminal and familiarize yourself with the tool’s commands:\n\n**1. General Help**: Type `milvus-backup help` to view the available commands and flags.\n\n```\nmilvus-backup is a backup\u0026restore tool for milvus.\n\nUsage:\n milvus-backup [flags]\n milvus-backup [command]\n\nAvailable Commands:\n check check if the connects is right.\n create create subcommand create a backup.\n delete delete subcommand delete backup by name.\n get get subcommand get backup by name.\n help Help about any command\n list list subcommand shows all backup in the cluster.\n restore restore subcommand restore a backup.\n server server subcommand start milvus-backup RESTAPI server.\n\nFlags:\n --config string config YAML file of milvus (default \"backup.yaml\")\n -h, --help help for milvus-backup\n\nUse \"milvus-backup [command] --help\" for more information about a command.\n```\n\n**2. Creating a Backup**: Get specific help for creating a backup by typing `milvus-backup create --help`.\n\n```\nUsage:\n milvus-backup create [flags]\n\nFlags:\n -n, --name string backup name, if unset will generate a name automatically\n -c, --colls string collectionNames to backup, use ',' to connect multiple collections\n -d, --databases string databases to backup\n -a, --database_collections string databases and collections to backup, json format: {\"db1\":[\"c1\", \"c2\"],\"db2\":[]}\n -f, --force force backup, will skip flush, should make sure data has been stored into disk when using it\n --meta_only only backup collection meta instead of data\n -h, --help help for create\n```\n\n**3. Restoring a Backup**: To understand how to restore a backup, use `milvus-backup restore --help`.\n\n```\nUsage:\n milvus-backup restore [flags]\n\nFlags:\n -n, --name string backup name to restore\n -c, --collections string collectionNames to restore\n -s, --suffix string add a suffix to collection name to restore\n -r, --rename string rename collections to new names, format: db1.collection1:db2.collection1_new,db1.collection2:db2.collection2_new\n -d, --databases string databases to restore, if not set, restore all databases\n -a, --database_collections string databases and collections to restore, json format: {\"db1\":[\"c1\", \"c2\"],\"db2\":[]}\n --meta_only if true, restore meta only\n --restore_index if true, restore index\n --use_auto_index if true, replace vector index with autoindex\n --drop_exist_collection if true, drop existing target collection before create\n --drop_exist_index if true, drop existing index of target collection before create\n --skip_create_collection if true, will skip collection, use when collection exist, restore index or data\n -h, --help help for restore\n```\n\n\n## Backup/Restore Use Cases\n\nThere are several use cases in which the milvus-backup tool can be applied effectively, depending on your specific needs and configurations:\n\n1. **Within a Single Milvus Instance:** Copy a collection to a new one within the same Milvus service.\n\n2. **Between Milvus Instances in a Single S3 with One Bucket:** Transfer a collection between Milvus instances with different root paths but using the same S3 bucket.\n\n3. **Between Milvus Instances Across Different S3 Buckets:** Transfer a collection between different S3 buckets within the same S3 service.\n\n4. **Across Different S3 Services:** Copy a collection between Milvus instances that are using different S3 services.\n\nLet’s explore each use case in details. \n\n\n## Use Case 1: Backup and Restore Within One Milvus Instance\n\nBackup and restore a collection within the same Milvus instance. Assume a collection named \"coll\" is backed up and restored as \"coll_bak\" using the same S3 bucket.\n\n\n### Configuration:\n\n- **Milvus** uses the `bucket_A` for storage.\n\n- **MinIO Configuration:**\n\n```\nminio:\n address: localhost # Address of MinIO/S3\n port: 9000 # Port of MinIO/S3\n accessKeyID: minioadmin # accessKeyID of MinIO/S3\n secretAccessKey: minioadmin # MinIO/S3 encryption string\n useSSL: false # Access to MinIO/S3 with SSL\n ssl:\n tlsCACert: /path/to/public.crt # path to your CACert file, ignore when it is empty\n bucketName: bucket_A # Bucket name in MinIO/S3\n rootPath: files # The root path where the message is stored in MinIO/S3\n```\n\n\n### Backup Workflow\n\n1\\. Configure `backup.yaml` to point Milvus and MinIO to the correct locations.\n\n```\n# Related configuration of minio, which is responsible for data persistence for Milvus.\nminio:\n # cloudProvider: \"minio\" # deprecated use storageType instead\n storageType: \"minio\" # support storage type: local, minio, s3, aws, gcp, ali(aliyun), azure, tc(tencent)\n address: localhost # Address of MinIO/S3\n port: 9000 # Port of MinIO/S3\n accessKeyID: minioadmin # accessKeyID of MinIO/S3\n secretAccessKey: minioadmin # MinIO/S3 encryption string\n useSSL: false # Access to MinIO/S3 with SSL\n useIAM: false\n iamEndpoint: \"\"\n bucketName: \"bucket_A\" # Milvus Bucket name in MinIO/S3, make it the same as your milvus instance\n rootPath: \"files\" # Milvus storage root path in MinIO/S3, make it the same as your milvus instance\n\n # only for azure\n backupAccessKeyID: minioadmin # accessKeyID of MinIO/S3\n backupSecretAccessKey: minioadmin # MinIO/S3 encryption string\n backupBucketName: \"bucket_A\" # Bucket name to store backup data. Backup data will store to backupBucketName/backupRootPath\n backupRootPath: \"backup\" # Rootpath to store backup data. Backup data will store to backupBucketName/backupRootPath\n```\n\n2\\. Create a backup using the command.\n\n```\n./milvus-backup create -c coll -n my_backup\n```\n\nThis command places the backup in `bucket_A/backup/my_backup`. \n\n3\\. Restore the backup to a new collection. \n\n```\n./milvus-backup restore -c coll -n my_backup -s _bak\n```\n\nThis restores \"coll\" as \"coll_bak\" within the same Milvus instance.\n\n\n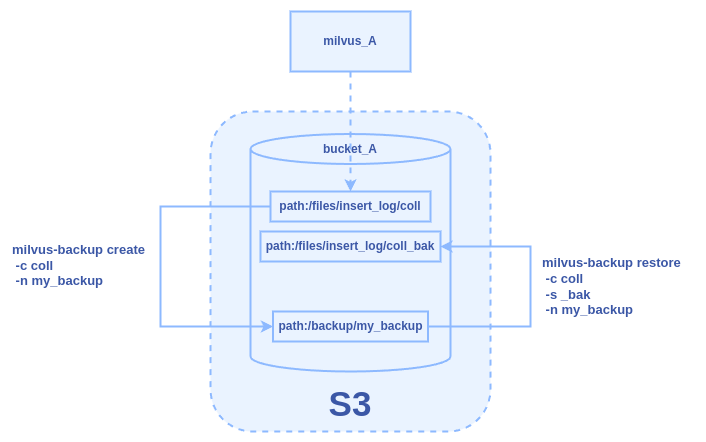\n\nFigure: The Backup and Restore Workflow Within One Milvus Instance\n\n\n## Use Case 2: Backup and Restore Between Two Milvus Instances Sharing One S3 Bucket\n\nBack up a collection from one Milvus instance and restore it to another using the same S3 bucket but with different root paths. Assuming there is a collection named \"coll\" in the milvus_A, we back up and restore it to a new collection named \"coll_bak\" to milvus_B. The two Milvus instances share the same bucket \"bucket_A\" as storage, but they have different root paths.\n\n\n### Configuration\n\n- **Milvus A** uses `files_A` as the root path.\n\n- **Milvus B** uses `files_B` as the root path.\n\n- **MinIO Configuration for Milvus A:**\n\n```\nminio:\n address: localhost # Address of MinIO/S3\n port: 9000 # Port of MinIO/S3\n accessKeyID: minioadmin # accessKeyID of MinIO/S3\n secretAccessKey: minioadmin # MinIO/S3 encryption string\n useSSL: false # Access to MinIO/S3 with SSL\n ssl:\n tlsCACert: /path/to/public.crt # path to your CACert file, ignore when it is empty\n bucketName: bucket_A # Bucket name in MinIO/S3\n rootPath: files_A # The root path where the message is stored in MinIO/S3\n```\n\n- **MinIO Configuration for Milvus B:** \n\n```\nminio:\n address: localhost # Address of MinIO/S3\n port: 9000 # Port of MinIO/S3\n accessKeyID: minioadmin # accessKeyID of MinIO/S3\n secretAccessKey: minioadmin # MinIO/S3 encryption string\n useSSL: false # Access to MinIO/S3 with SSL\n ssl:\n tlsCACert: /path/to/public.crt # path to your CACert file, ignore when it is empty\n bucketName: bucket_A # Bucket name in MinIO/S3\n rootPath: files_B # The root path where the message is stored in MinIO/S3\n```\n\n\n### Backup Workflow\n\n**1. Backup Configuration for Milvus A**\n\n```\n# milvus proxy address, compatible to milvus.yaml\nmilvus:\n address: milvus_A\n port: 19530\n authorizationEnabled: false\n # tls mode values [0, 1, 2]\n # 0 is close, 1 is one-way authentication, 2 is two-way authentication.\n tlsMode: 0\n user: \"root\"\n password: \"Milvus\"\n # Related configuration of minio, which is responsible for data persistence for Milvus.\nminio:\n # cloudProvider: \"minio\" # deprecated use storageType instead\n storageType: \"minio\" # support storage type: local, minio, s3, aws, gcp, ali(aliyun), azure, tc(tencent)\n address: milvus_A # Address of MinIO/S3\n port: 9000 # Port of MinIO/S3\n accessKeyID: minioadmin # accessKeyID of MinIO/S3\n secretAccessKey: minioadmin # MinIO/S3 encryption string\n useSSL: false # Access to MinIO/S3 with SSL\n useIAM: false\n iamEndpoint: \"\"\n bucketName: \"bucket_A\" # Milvus Bucket name in MinIO/S3, make it the same as your milvus instance\n rootPath: \"files_A\" # Milvus storage root path in MinIO/S3, make it the same as your milvus instance\n\n # only for azure\n backupAccessKeyID: minioadmin # accessKeyID of MinIO/S3\n backupSecretAccessKey: minioadmin # MinIO/S3 encryption string\n backupBucketName: \"bucket_A\" # Bucket name to store backup data. Backup data will store to backupBucketName/backupRootPath\n backupRootPath: \"backup\" # Rootpath to store backup data. Backup data will store to backupBucketName/backupRootPath\n```\n\n**2. Execute the backup command:**\n\n```\n./milvus-backup create -c coll -n my_backup\n```\n\n**3. Restore Configuration for Milvus B**\n\nModify `backup.yaml` to point to Milvus B and adjust the MinIO root path:\n\n```\n# milvus proxy address, compatible to milvus.yaml\nmilvus:\n address: milvus_B\n port: 19530\n authorizationEnabled: false\n # tls mode values [0, 1, 2]\n # 0 is close, 1 is one-way authentication, 2 is two-way authentication.\n tlsMode: 0\n user: \"root\"\n password: \"Milvus\"\n # Related configuration of minio, which is responsible for data persistence for Milvus.\nminio:\n # cloudProvider: \"minio\" # deprecated use storageType instead\n storageType: \"minio\" # support storage type: local, minio, s3, aws, gcp, ali(aliyun), azure, tc(tencent)\n address: milvus_B # Address of MinIO/S3\n port: 9000 # Port of MinIO/S3\n accessKeyID: minioadmin # accessKeyID of MinIO/S3\n secretAccessKey: minioadmin # MinIO/S3 encryption string\n useSSL: false # Access to MinIO/S3 with SSL\n useIAM: false\n iamEndpoint: \"\"\n bucketName: \"bucket_A\" # Milvus Bucket name in MinIO/S3, make it the same as your milvus instance\n rootPath: \"files_B\" # Milvus storage root path in MinIO/S3, make it the same as your milvus instance\n\n # only for azure\n backupAccessKeyID: minioadmin # accessKeyID of MinIO/S3\n backupSecretAccessKey: minioadmin # MinIO/S3 encryption string\n backupBucketName: \"bucket_A\" # Bucket name to store backup data. Backup data will store to backupBucketName/backupRootPath\n backupRootPath: \"backup\" # Rootpath to store backup data. Backup data will store to backupBucketName/backupRootPath\n```\n\n**4. Execute the restore command:**\n\n```\n./milvus-backup restore -c coll -n my_backup -s _bak\n```\n\n\n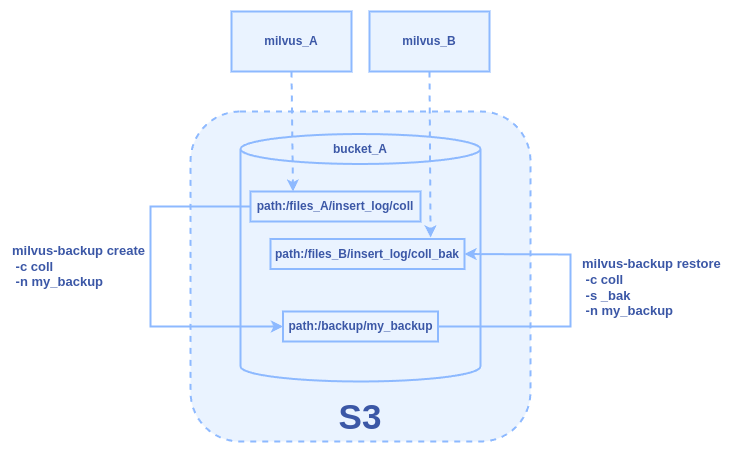\n\n\n\n\n## Use Case 3: Backup and Restore Between Two Milvus Instances in One S3, Different Buckets\n\nBackup a collection from a Milvus instance (Milvus_A) and restore it to another Milvus instance (Milvus_B) within the same S3 service but using different buckets.\n\n\n### Configuration:\n\n- **Milvus** uses the `bucket_A` for storage.\n\n- **MinIO Configuration for Milvus A:**\n\n```\nminio:\n address: localhost # Address of MinIO/S3\n port: 9000 # Port of MinIO/S3\n accessKeyID: minioadmin # accessKeyID of MinIO/S3\n secretAccessKey: minioadmin # MinIO/S3 encryption string\n useSSL: false # Access to MinIO/S3 with SSL\n ssl:\n tlsCACert: /path/to/public.crt # path to your CACert file, ignore when it is empty\n bucketName: bucket_A # Bucket name in MinIO/S3\n rootPath: files # The root path where the message is stored in MinIO/S3\n```\n\n- **MinIO Configuration for Milvus B:** \n\n```\nminio:\n address: localhost # Address of MinIO/S3\n port: 9000 # Port of MinIO/S3\n accessKeyID: minioadmin # accessKeyID of MinIO/S3\n secretAccessKey: minioadmin # MinIO/S3 encryption string\n useSSL: false # Access to MinIO/S3 with SSL\n ssl:\n tlsCACert: /path/to/public.crt # path to your CACert file, ignore when it is empty\n bucketName: bucket_B # Bucket name in MinIO/S3\n rootPath: files # The root path where the message is stored in MinIO/S3\n```\n\n\n### Backup and Restore Workflow\n\n**1. Backup Configuration for Milvus A**\n\n```\n# milvus proxy address, compatible to milvus.yaml\nmilvus:\n address: milvus_A\n port: 19530\n authorizationEnabled: false\n # tls mode values [0, 1, 2]\n # 0 is close, 1 is one-way authentication, 2 is two-way authentication.\n tlsMode: 0\n user: \"root\"\n password: \"Milvus\"\n # Related configuration of minio, which is responsible for data persistence for Milvus.\nminio:\n # cloudProvider: \"minio\" # deprecated use storageType instead\n storageType: \"minio\" # support storage type: local, minio, s3, aws, gcp, ali(aliyun), azure, tc(tencent)\n address: localhost # Address of MinIO/S3\n port: 9000 # Port of MinIO/S3\n accessKeyID: minioadmin # accessKeyID of MinIO/S3\n secretAccessKey: minioadmin # MinIO/S3 encryption string\n useSSL: false # Access to MinIO/S3 with SSL\n useIAM: false\n iamEndpoint: \"\"\n bucketName: \"bucket_A\" # Milvus Bucket name in MinIO/S3, make it the same as your milvus instance\n rootPath: \"files\" # Milvus storage root path in MinIO/S3, make it the same as your milvus instance\n\n # only for azure\n backupAccessKeyID: minioadmin # accessKeyID of MinIO/S3\n backupSecretAccessKey: minioadmin # MinIO/S3 encryption string\n backupBucketName: \"bucket_B\" # Bucket name to store backup data. Backup data will store to backupBucketName/backupRootPath\n backupRootPath: \"backup\" # Rootpath to store backup data. Backup data will store to backupBucketName/backupRootPath\n```\n\n**2. Execute the backup command:**\n\n```\n./milvus-backup create -c coll -n my_backup\n```\n\n**3. Restore Configuration for Milvus B**\n\n```\n# milvus proxy address, compatible to milvus.yaml\nmilvus:\n address: milvus_B\n port: 19530\n authorizationEnabled: false\n # tls mode values [0, 1, 2]\n # 0 is close, 1 is one-way authentication, 2 is two-way authentication.\n tlsMode: 0\n user: \"root\"\n password: \"Milvus\"\n # Related configuration of minio, which is responsible for data persistence for Milvus.\nminio:\n # cloudProvider: \"minio\" # deprecated use storageType instead\n storageType: \"minio\" # support storage type: local, minio, s3, aws, gcp, ali(aliyun), azure, tc(tencent)\n address: localhost # Address of MinIO/S3\n port: 9000 # Port of MinIO/S3\n accessKeyID: minioadmin # accessKeyID of MinIO/S3\n secretAccessKey: minioadmin # MinIO/S3 encryption string\n useSSL: false # Access to MinIO/S3 with SSL\n useIAM: false\n iamEndpoint: \"\"\n bucketName: \"bucket_B\" # Milvus Bucket name in MinIO/S3, make it the same as your milvus instance\n rootPath: \"files\" # Milvus storage root path in MinIO/S3, make it the same as your milvus instance\n\n # only for azure\n backupAccessKeyID: minioadmin # accessKeyID of MinIO/S3\n backupSecretAccessKey: minioadmin # MinIO/S3 encryption string\n backupBucketName: \"bucket_B\" # Bucket name to store backup data. Backup data will store to backupBucketName/backupRootPath\n backupRootPath: \"backup\" # Rootpath to store backup data. Backup data will store to backupBucketName/backupRootPath\n```\n\n**4. Execute the restore command:**\n\n```\n./milvus-backup restore -c coll -n my_backup -s _bak\n```\n\n\n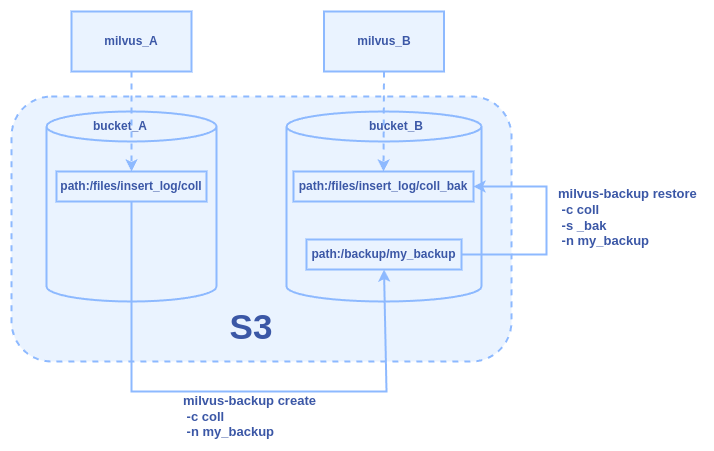\n\nFigure: The Backup and Restore Workflow Between Two Milvus Instances in One S3, Different Buckets\n\n\n## Use Case 4: Backup and Restore Between Two Milvus Instances Across Different S3 Services\n\nTo facilitate the backup of a collection named \"coll\" from Milvus_A using one S3 service (MinIO_A) and restore it into Milvus_B using a different S3 service (MinIO_B), with each instance utilizing different storage buckets.\n\n\n### Configuration\n\n- **MinIO Configuration for Milvus A:**\n\n```\nminio:\n address: minio_A # Address of MinIO/S3\n port: 9000 # Port of MinIO/S3\n accessKeyID: minioadmin # accessKeyID of MinIO/S3\n secretAccessKey: minioadmin # MinIO/S3 encryption string\n useSSL: false # Access to MinIO/S3 with SSL\n ssl:\n tlsCACert: /path/to/public.crt # path to your CACert file, ignore when it is empty\n bucketName: bucket_A # Bucket name in MinIO/S3\n rootPath: files # The root path where the message is stored in MinIO/S3\n```\n\n- **MinIO Configuration for Milvus B**\n\n```\n minio:\n address: minio_B # Address of MinIO/S3\n port: 9000 # Port of MinIO/S3\n accessKeyID: minioadmin # accessKeyID of MinIO/S3\n secretAccessKey: minioadmin # MinIO/S3 encryption string\n useSSL: false # Access to MinIO/S3 with SSL\n ssl:\n tlsCACert: /path/to/public.crt # path to your CACert file, ignore when it is empty\n bucketName: bucket_B # Bucket name in MinIO/S3\n rootPath: files # The root path where the message is stored in MinIO/S3\n```\n\n\n### Backup and Restore Workflow\n\n**1. Backup Configuration for Milvus A**\n\n```\n# milvus proxy address, compatible to milvus.yaml\nmilvus:\n address: milvus_A\n port: 19530\n authorizationEnabled: false\n # tls mode values [0, 1, 2]\n # 0 is close, 1 is one-way authentication, 2 is two-way authentication.\n tlsMode: 0\n user: \"root\"\n password: \"Milvus\"\n # Related configuration of minio, which is responsible for data persistence for Milvus.\nminio:\n # cloudProvider: \"minio\" # deprecated use storageType instead\n storageType: \"minio\" # support storage type: local, minio, s3, aws, gcp, ali(aliyun), azure, tc(tencent)\n address: minio_A # Address of MinIO/S3\n port: 9000 # Port of MinIO/S3\n accessKeyID: minioadmin # accessKeyID of MinIO/S3\n secretAccessKey: minioadmin # MinIO/S3 encryption string\n useSSL: false # Access to MinIO/S3 with SSL\n useIAM: false\n iamEndpoint: \"\"\n bucketName: \"bucket_A\" # Milvus Bucket name in MinIO/S3, make it the same as your milvus instance\n rootPath: \"files\" # Milvus storage root path in MinIO/S3, make it the same as your milvus instance\n\n # only for azure\n backupAccessKeyID: minioadmin # accessKeyID of MinIO/S3\n backupSecretAccessKey: minioadmin # MinIO/S3 encryption string\n backupBucketName: \"bucket_A\" # Bucket name to store backup data. Backup data will store to backupBucketName/backupRootPath\n backupRootPath: \"backup\" # Rootpath to store backup data. Backup data will store to backupBucketName/backupRootPath\n```\n\n**2. Execute the backup command:**\n\n```\n./milvus-backup create -c coll -n my_backup\n```\n\n**3.** Transfer the Backup\n\nManually copy the backup from `minio_A:bucket_A/backup/my_backup` to `minio_B:bucket_B/backup/my_backup` using an S3 compatible tool or SDK.\n\n4\\. **Restore Configuration for Milvus B**\n\n```\n# milvus proxy address, compatible to milvus.yaml\nmilvus:\n address: milvus_B\n port: 19530\n authorizationEnabled: false\n # tls mode values [0, 1, 2]\n # 0 is close, 1 is one-way authentication, 2 is two-way authentication.\n tlsMode: 0\n user: \"root\"\n password: \"Milvus\"\n # Related configuration of minio, which is responsible for data persistence for Milvus.\nminio:\n # cloudProvider: \"minio\" # deprecated use storageType instead\n storageType: \"minio\" # support storage type: local, minio, s3, aws, gcp, ali(aliyun), azure, tc(tencent)\n address: minio_B # Address of MinIO/S3\n port: 9000 # Port of MinIO/S3\n accessKeyID: minioadmin # accessKeyID of MinIO/S3\n secretAccessKey: minioadmin # MinIO/S3 encryption string\n useSSL: false # Access to MinIO/S3 with SSL\n useIAM: false\n iamEndpoint: \"\"\n bucketName: \"bucket_B\" # Milvus Bucket name in MinIO/S3, make it the same as your milvus instance\n rootPath: \"files\" # Milvus storage root path in MinIO/S3, make it the same as your milvus instance\n\n # only for azure\n backupAccessKeyID: minioadmin # accessKeyID of MinIO/S3\n backupSecretAccessKey: minioadmin # MinIO/S3 encryption string\n backupBucketName: \"bucket_B\" # Bucket name to store backup data. Backup data will store to backupBucketName/backupRootPath\n backupRootPath: \"backup\" # Rootpath to store backup data. Backup data will store to backupBucketName/backupRootPath\n```\n\n5\\. **Execute the restore command:**\n\n```\n./milvus-backup restore -c coll -n my_backup -s _bak\n```\n\n\n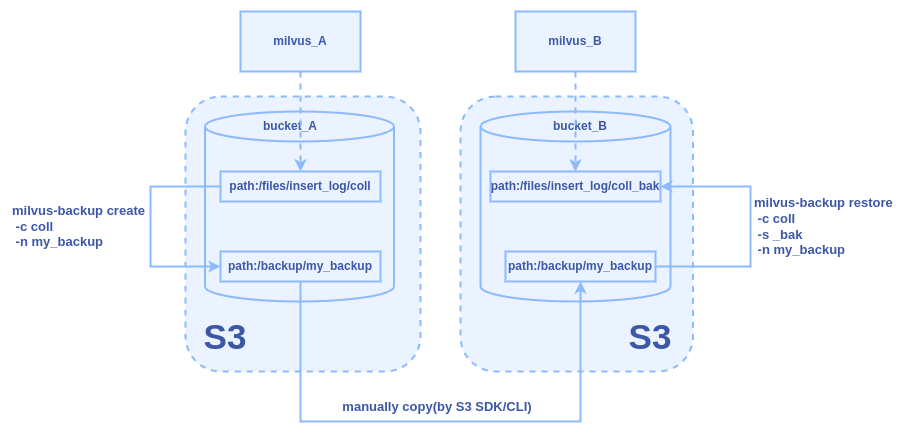\n\nFigure: The Backup and Restore Workflow Between Two Milvus Instances Across Different S3 Services\n\n\n## Configuration File Explanation\n\nEdit the `configs/backup.yaml` file to tailor the backup settings to your environment. Here’s a breakdown of the configuration options:\n\n**Logging**: Configure logging levels and output preferences.\n\n```\n# Configures the system log output.\nlog:\n level: info # Only supports debug, info, warn, error, panic, or fatal. Default 'info'.\n console: true # whether print log to console\n file:\n rootPath: \"logs/backup.log\"\n```\n\n**Milvus Connection**: Set the connection details for your Milvus instance.\n\n```\n# milvus proxy address, compatible to milvus.yaml\nmilvus:\n address: localhost\n port: 19530\n authorizationEnabled: false\n # tls mode values [0, 1, 2]\n # 0 is close, 1 is one-way authentication, 2 is two-way authentication.\n tlsMode: 0\n user: \"root\"\n password: \"Milvus\"\n```\n\n**MinIO Configuration**: Define how backups interact with MinIO or other S3-compatible storage.\n\n```\n# Related configuration of minio, which is responsible for data persistence for Milvus.\nminio:\n # cloudProvider: \"minio\" # deprecated use storageType instead\n storageType: \"minio\" # support storage type: local, minio, s3, aws, gcp, ali(aliyun), azure, tc(tencent)\n address: localhost # Address of MinIO/S3\n port: 9000 # Port of MinIO/S3\n accessKeyID: minioadmin # accessKeyID of MinIO/S3\n secretAccessKey: minioadmin # MinIO/S3 encryption string\n useSSL: false # Access to MinIO/S3 with SSL\n useIAM: false\n iamEndpoint: \"\"\n bucketName: \"a-bucket\" # Milvus Bucket name in MinIO/S3, make it the same as your milvus instance\n rootPath: \"files\" # Milvus storage root path in MinIO/S3, make it the same as your milvus instance\n\n # only for azure\n backupAccessKeyID: minioadmin # accessKeyID of MinIO/S3\n backupSecretAccessKey: minioadmin # MinIO/S3 encryption string\n backupBucketName: \"a-bucket\" # Bucket name to store backup data. Backup data will store to backupBucketName/backupRootPath\n backupRootPath: \"backup\" # Rootpath to store backup data. Backup data will store to backupBucketName/backupRootPath\n```\n\n\n## Conclusion\n\nThe [**Milvus Backup**](https://milvus.io/docs/milvus_backup_overview.md) tool provides a robust solution for backing up and restoring collections within and across Milvus instances. Whether you're managing backups within a single instance, between instances in the same S3 service, or across different S3 services, milvus-backup handles it all with flexibility and precision.\n\n\n### Key Takeaways\n\n1. **Versatility:** Milvus-backup supports multiple scenarios, from simple intra-instance backups to complex cross-service restorations.\n\n2. **Configuration Flexibility:** By configuring the `backup.yaml` file appropriately, users can customize the backup and restore processes to fit specific needs, accommodating different storage setups and network configurations.\n\n3. **Security and Control:** Direct manipulation of S3 buckets and paths allows for control over data storage and security, ensuring backups are both safe and accessible only to authorized users.\n\nEffective data management is crucial for leveraging Milvus's full potential in your applications. By mastering the Milvus backup tool, you can ensure data durability and availability, even in complex distributed environments. This guide empowers users to implement robust backup strategies, promoting best practices and efficient data handling techniques.\n\nWhether you're a developer, a data engineer, or an IT professional, understanding and utilizing the Milvus-backup tool can significantly contribute to your project's success by providing reliable and efficient data management solutions.\n","title":"How to Use the Milvus Backup Tool: A Step-by-Step Guide","metaData":{}},{"id":"deploy-milvus-on-kubernetes-step-by-step-guide-for-k8s-users.md","author":"Gael Gu","desc":"This guide will provide a clear, step-by-step walkthrough for setting up Milvus on Kubernetes using the Milvus Operator.","tags":["Engineering"],"recommend":true,"canonicalUrl":"https://milvus.io/blog/deploy-milvus-on-kubernetes-step-by-step-guide-for-k8s-users.md","date":"2024-09-26T00:00:00.000Z","cover":"https://assets.zilliz.com/Deploying_Milvus_on_Kubernetes_A_Step_by_Step_Guide_for_Kubernetes_Users_4193487867.png","href":"/blog/deploy-milvus-on-kubernetes-step-by-step-guide-for-k8s-users.md","content":"\n\n[**Milvus**](https://zilliz.com/what-is-milvus) is an open-source [vector database](https://zilliz.com/learn/what-is-vector-database) designed to store, index, and search massive amounts of [unstructured data](https://zilliz.com/learn/introduction-to-unstructured-data) through vector representations, making it perfect for AI-driven applications, such as similarity search, [semantic search](https://zilliz.com/glossary/semantic-search), retrieval augmented generation ([RAG](https://zilliz.com/learn/Retrieval-Augmented-Generation)), recommendation engines, and other machine learning tasks. \n\nBut what makes Milvus even more powerful is its seamless integration with Kubernetes. If you're a Kubernetes aficionado, you know the platform is perfect for orchestrating scalable, distributed systems. Milvus takes full advantage of Kubernetes' capabilities, allowing you to easily deploy, scale, and manage distributed Milvus clusters. This guide will provide a clear, step-by-step walkthrough for setting up Milvus on Kubernetes using the Milvus Operator. \n\n\n## Prerequisites\n\nBefore we begin, ensure you have the following prerequisites in place:\n\n- A Kubernetes cluster up and running. If you're testing locally, `minikube` is a great choice.\n\n- `kubectl` installed and configured to interact with your Kubernetes cluster.\n\n- Familiarity with basic Kubernetes concepts like pods, services, and deployments.\n\n\n## Step 1: Installing Minikube (For Local Testing)\n\nIf you need to set up a local Kubernetes environment, `minikube` is the tool for you. Official installation instructions are on the [minikube getting started page](https://minikube.sigs.k8s.io/docs/start/).\n\n\n### 1. Install Minikube\n\nVisit the[ minikube releases page](https://github.com/kubernetes/minikube/releases) and download the appropriate version for your operating system. For macOS/Linux, you can use the following command:\n\n```\n$ curl -Lo minikube https://storage.googleapis.com/minikube/releases/latest/minikube-linux-amd64\n$ sudo install minikube-linux-amd64 /usr/local/bin/minikube \u0026\u0026 rm minikube-linux-amd64\n```\n\n\n### 2. Start Minikube\n\n```\n$ minikube start\n```\n\n\n### 3. Interact with Cluster\n\nNow, you can interact with your clusters with the kubectl inside minikube. If you haven’t installed kubectl, minikube will download the appropriate version by default.\n\n```\n$ minikube kubectl cluster-info\n```\n\nAlternatively, you can create a symbolic link to minikube’s binary named `kubectl` for easier usage.\n\n```\n$ sudo ln -s $(which minikube) /usr/local/bin/kubectl\n$ kubectl cluster-info\n```\n\n\n## Step 2: Configuring the StorageClass\n\nIn Kubernetes, a **StorageClass** defines the types of storage available for your workloads, providing flexibility in managing different storage configurations. Before proceeding, you must ensure a default StorageClass is available in your cluster. Here’s how to check and configure one if necessary.\n\n\n### 1. Check Installed StorageClasses\n\nTo see the available StorageClasses in your Kubernetes cluster, run the following command:\n\n```\n$ kubectl get sc\n```\n\nThis will display the list of storage classes installed in your cluster. If a default StorageClass is already configured, it will be marked with `(default)`.\n\n\n\n\n### 2. Configure a Default StorageClass (if necessary)\n\nIf no default StorageClass is set, you can create one by defining it in a YAML file. Use the following example to create a default StorageClass:\n\n```\napiVersion: storage.k8s.io/v1\nkind: StorageClass\nmetadata:\n name: default-storageclass\nprovisioner: k8s.io/minikube-hostpath\n```\n\nThis YAML configuration defines a `StorageClass` called `default-storageclass` that uses the `minikube-hostpath` provisioner, commonly used in local development environments.\n\n\n### 3. Apply the StorageClass\n\nOnce the `default-storageclass.yaml` file is created, apply it to your cluster using the following command:\n\n```\n$ kubectl apply -f default-storageclass.yaml\n```\n\nThis will set up the default StorageClass for your cluster, ensuring that your storage needs are properly managed in the future.\n\n\n## Step 3: Installing Milvus Using the Milvus Operator\n\nThe Milvus Operator simplifies deploying Milvus on Kubernetes, managing the deployment, scaling, and updates. Before installing the Milvus Operator, you'll need to install the **cert-manager**, which provides certificates for the webhook server used by the Milvus Operator.\n\n\n### 1. Install cert-manager\n\nMilvus Operator requires a [cert-manager](https://cert-manager.io/docs/installation/supported-releases/) to manage certificates for secure communication. Make sure you install **cert-manager version 1.1.3** or later. To install it, run the following command:\n\n```\n$ kubectl apply -f https://github.com/jetstack/cert-manager/releases/download/v1.5.3/cert-manager.yaml\n```\n\nAfter the installation, verify that the cert-manager pods are running by executing:\n\n```\n$ kubectl get pods -n cert-manager\n```\n\n\n\n\n\n### 2. Install the Milvus Operator\n\nOnce the cert-manager is up and running, you can install the Milvus Operator. Run the following command to deploy it using `kubectl`:\n\n```\n$ kubectl apply -f https://raw.githubusercontent.com/zilliztech/milvus-operator/main/deploy/manifests/deployment.yaml\n```\n\nYou can check if the Milvus Operator pod is running using the following command: \n\n```\n$ kubectl get pods -n milvus-operator\n```\n\n\n\n\n### 3. Deploy Milvus Cluster\n\nOnce the Milvus Operator pod is running, you can deploy a Milvus cluster with the operator. The following command deploys a Milvus cluster with its components and dependencies in separate pods using default configurations:\n\n```\n$ kubectl apply -f https://raw.githubusercontent.com/zilliztech/milvus-operator/main/config/samples/milvus_cluster_default.yaml\n```\n\n\n\n\n\nTo customize the Milvus settings, you will need to replace the YAML file with your own configuration YAML file. In addition to manually editing or creating the file, you can use the Milvus Sizing Tool to adjust the configurations and then download the corresponding YAML file.\n\nTo customize Milvus settings, you must replace the default YAML file with your own configuration. You can either manually edit or create this file, tailoring it to your specific requirements. \n\nAlternatively, you can use the [**Milvus Sizing Tool**](https://milvus.io/tools/sizing) for a more streamlined approach. This tool allows you to adjust various settings, such as resource allocation and storage options, and then download the corresponding YAML file with your desired configurations. This ensures that your Milvus deployment is optimized for your specific use case.\n\n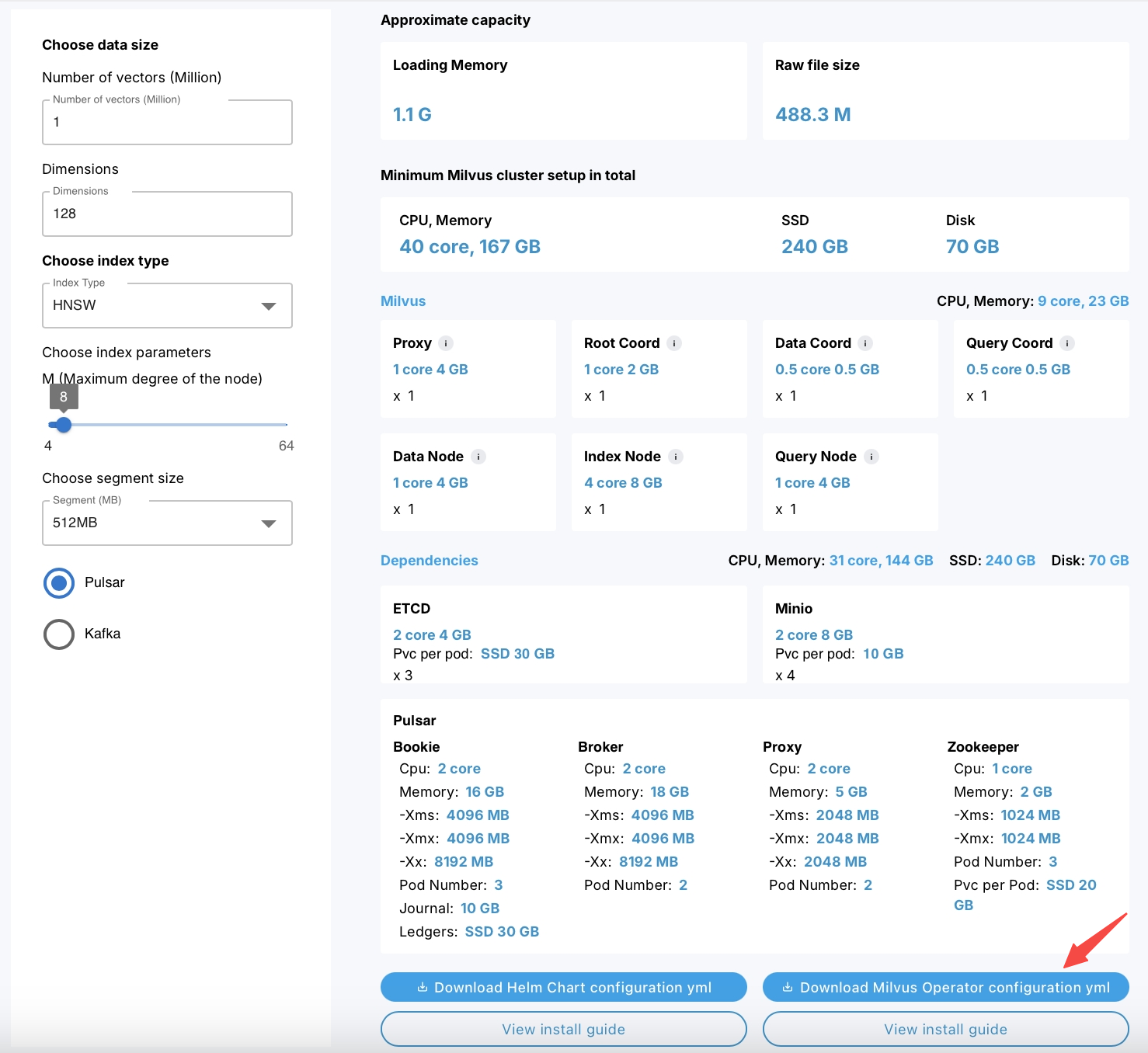\n\nFigure: Milvus sizing tool \n\nIt may take some time to finish the deployment. You can check the status of your Milvus cluster via the command:\n\n```\n$ kubectl get milvus my-release\n```\n\n\n\n\nOnce your Milvus cluster is ready, all pods in the Milvus cluster should be running or completed:\n\n```\n$ kubectl get pods\n```\n\n\n## Step 4: Accessing Your Milvus Cluster\n\nOnce your Milvus cluster is deployed, you need to access it by forwarding a local port to the Milvus service port. Follow these steps to retrieve the service port and set up port forwarding.\n\n\n#### **1. Get the Service Port**\n\nFirst, identify the service port by using the following command. Replace `\u003cYOUR_MILVUS_PROXY_POD\u003e` with the name of your Milvus proxy pod, which typically starts with `my-release-milvus-proxy-`:\n\n```\n$ kubectl get pod \u003cYOUR_MILVUS_PROXY_POD\u003e --template ='{{(index (index .spec.containers 0).ports 0).containerPort}}{{\"\\n\"}}'\n```\n\nThis command will return the port number that your Milvus service is using.\n\n\n#### **2. Forward the Port**\n\nTo access your Milvus cluster locally, forward a local port to the service port using the following command. Replace `\u003cYOUR_LOCAL_PORT\u003e` with the local port you want to use and `\u003cYOUR_SERVICE_PORT\u003e` with the service port retrieved in the previous step:\n\n```\n$ kubectl port-forward --address 0.0.0.0 service/my-release-milvus \u003cYOUR_LOCAL_PORT\u003e:\u003cYOUR_SERVICE_PORT\u003e\n```\n\nThis command allows port-forwarding to listen on all IP addresses of the host machine. If you only need the service to listen on `localhost`, you can omit the `--address 0.0.0.0` option.\n\nOnce the port-forwarding is set up, you can access your Milvus cluster via the specified local port for further operations or integrations.\n\n\n## Step 5: Connecting to Milvus Using Python SDK\n\nWith your Milvus cluster up and running, you can now interact with it using any Milvus SDK. In this example, we'll use [PyMilvus](https://zilliz.com/blog/what-is-pymilvus), Milvus’s **Python SDK,** to connect to the cluster and perform basic operations.\n\n\n### 1. Install PyMilvus\n\nTo interact with Milvus via Python, you need to install the `pymilvus` package:\n\n```\n$ pip install pymilvus\n```\n\n\n### 2. Connect to Milvus\n\nThe following is a sample Python script that connects to your Milvus cluster and demonstrates how to perform basic operations such as creating a collection.\n\n```\nfrom pymilvus import MilvusClient\n```\n\n```\n# Connect to the Milvus server\nclient = MilvusClient(uri=\"http://localhost:\u003cYOUR_LOCAL_PORT\u003e\")\n```\n\n```\n# Create a collection\ncollection_name = \"example_collection\"\nif client.has_collection(collection_name):\n client.drop_collection(collection_name)\nclient.create_collection(\n collection_name=collection_name,\n dimension=768, # The vectors we will use in this demo has 768 dimensions\n)\n```\n\n\n#### Explanation:\n\n- Connect to Milvus: The script connects to the Milvus server running on `localhost` using the local port you set up in Step 4.\n\n- Create a Collection: It checks if a collection named `example_collection` already exists, drops it if so, and then creates a new collection with vectors of 768 dimensions.\n\nThis script establishes a connection to the Milvus cluster and creates a collection, serving as a starting point for more complex operations like inserting vectors and performing similarity searches.\n\n\n## Conclusion\n\nDeploying Milvus in a distributed setup on Kubernetes unlocks powerful capabilities for managing large-scale vector data, enabling seamless scalability and high-performance AI-driven applications. Following this guide, you've learned how to set up Milvus using the Milvus Operator, making the process streamlined and efficient.\n\nAs you continue to explore Milvus, consider scaling your cluster to meet growing demands or deploying it on cloud platforms such as Amazon EKS, Google Cloud, or Microsoft Azure. For enhanced management and monitoring, tools like [**Milvus Backup**](https://milvus.io/docs/milvus_backup_overview.md), [**Birdwatcher**](https://milvus.io/docs/birdwatcher_overview.md), and [**Attu**](https://github.com/zilliztech/attu) offer valuable support for maintaining the health and performance of your deployments.\n\nYou're now ready to harness the full potential of Milvus on Kubernetes—happy deploying! 🚀\n\n\n## Further Resources\n\n- [Milvus Documentation](https://milvus.io/docs/overview.md)\n\n- [Milvus Lite vs. Standalone vs. Distributed: Which Mode is Right for You? ](https://zilliz.com/blog/choose-the-right-milvus-deployment-mode-ai-applications)\n\n- [Supercharging Vector Search: Milvus on GPUs with NVIDIA RAPIDS cuVS](https://zilliz.com/blog/milvus-on-gpu-with-nvidia-rapids-cuvs)\n\n- [What is RAG? ](https://zilliz.com/learn/Retrieval-Augmented-Generation)\n\n- [Generative AI Resource Hub | Zilliz](https://zilliz.com/learn/generative-ai)\n\n- [Top Performing AI Models for Your GenAI Apps | Zilliz](https://zilliz.com/ai-models)\n","title":"Deploying Milvus on Kubernetes: A Step-by-Step Guide for Kubernetes Users","metaData":{}},{"id":"how-to-deploy-open-source-milvus-vector-database-on-amazon-eks.md","author":"AWS","desc":"A step-by-step guide on deploying the Milvus vector database on AWS using managed services such as Amazon EKS, S3, MSK, and ELB.","tags":["Engineering"],"recommend":true,"canonicalUrl":"https://milvus.io/blog/how-to-deploy-open-source-milvus-vector-database-on-amazon-eks.md","date":"2024-08-09T00:00:00.000Z","cover":"https://assets.zilliz.com/Getting_started_with_Milvus_cluster_and_K8s_1_34b2c81802.png","href":"/blog/how-to-deploy-open-source-milvus-vector-database-on-amazon-eks.md","content":"\n\n_This post was originally published on the [_AWS website_](https://aws.amazon.com/cn/blogs/china/build-open-source-vector-database-milvus-based-on-amazon-eks/) and is translated, edited, and reposted here with permission._ \n\n\n## An Overview of Vector Embeddings and Vector Databases \n\nThe rise of [Generative AI (GenAI)](https://zilliz.com/learn/generative-ai), particularly large language models ([LLMs](https://zilliz.com/glossary/large-language-models-(llms))), has significantly boosted interest in [vector databases](https://zilliz.com/learn/what-is-vector-database), establishing them as an essential component within the GenAI ecosystem. As a result, vector databases are being adopted in increasing [use cases](https://milvus.io/use-cases). \n\nAn [IDC Report](https://venturebeat.com/data-infrastructure/report-80-of-global-datasphere-will-be-unstructured-by-2025/) predicts that by 2025, over 80% of business data will be unstructured, existing in formats such as text, images, audio, and videos. Understanding, processing, storing, and querying this vast amount of [unstructured data](https://zilliz.com/learn/introduction-to-unstructured-data) at scale presents a significant challenge. The common practice in GenAI and deep learning is to transform unstructured data into vector embeddings, store, and index them in a vector database like [Milvus](https://milvus.io/intro) or [Zilliz Cloud](https://zilliz.com/cloud) (the fully managed Milvus) for [vector similarity](https://zilliz.com/learn/vector-similarity-search) or semantic similarity searches. \n\nBut what exactly are [vector embeddings](https://zilliz.com/glossary/vector-embeddings)? Simply put, they are numerical representations of floating-point numbers in a high-dimensional space. The [distance between two vectors](https://zilliz.com/blog/similarity-metrics-for-vector-search) indicates their relevance: the closer they are, the more relevant they are to each other, and vice versa. This means that similar vectors correspond to similar original data, which differs from traditional keyword or exact searches. \n\n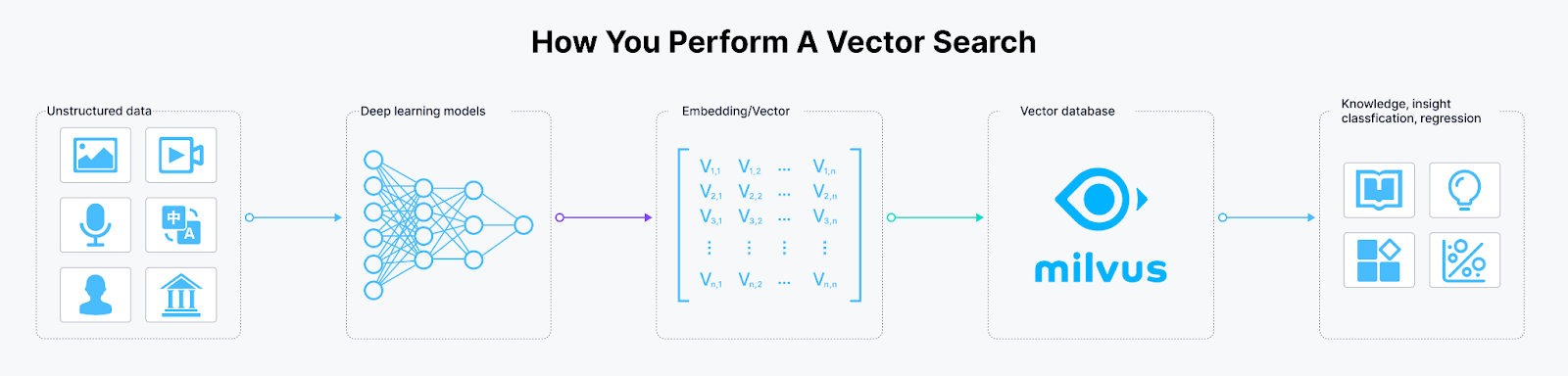\n\n_Figure 1: How to perform a vector similarity search_ \n\nThe ability to store, index, and search vector embeddings is the core functionality of vector databases. Currently, mainstream vector databases fall into two categories. The first category extends existing relational database products, such as Amazon OpenSearch Service with the [KNN](https://zilliz.com/blog/k-nearest-neighbor-algorithm-for-machine-learning) plugin and Amazon RDS for [PostgreSQL](https://zilliz.com/comparison/milvus-vs-pgvector) with the pgvector extension. The second category comprises specialized vector database products, including well-known examples like Milvus, Zilliz Cloud (the fully managed Milvus), [Pinecone](https://zilliz.com/comparison/pinecone-vs-zilliz-vs-milvus), [Weaviate](https://zilliz.com/comparison/milvus-vs-weaviate), [Qdrant](https://zilliz.com/comparison/milvus-vs-qdrant), and [Chroma](https://zilliz.com/blog/milvus-vs-chroma). \n\nEmbedding techniques and vector databases have broad applications across various [AI-driven use cases](https://zilliz.com/vector-database-use-cases), including image similarity search, video deduplication and analysis, natural language processing, recommendation systems, targeted advertising, personalized search, intelligent customer service, and fraud detection.\n\n[Milvus](https://milvus.io/docs/quickstart.md) is one of the most popular open-source options among the numerous vector databases. This post introduces Milvus and explores the practice of deploying Milvus on AWS EKS. \n\n\n## What is Milvus? \n\n[Milvus](https://milvus.io/intro) is a highly flexible, reliable, and blazing-fast cloud-native, open-source vector database. It powers vector similarity search and AI applications and strives to make vector databases accessible to every organization. Milvus can store, index, and manage a billion+ vector embeddings generated by deep neural networks and other machine learning (ML) models.\n\nMilvus was released under the [open-source Apache License 2.0](https://github.com/milvus-io/milvus/blob/master/LICENSE) in October 2019. It is currently a graduate project under [LF AI \u0026 Data Foundation](https://lfaidata.foundation/). At the time of writing this blog, Milvus had reached more than [50 million Docker pull](https://hub.docker.com/r/milvusdb/milvus) downloads and was used by [many customers](https://milvus.io/), such as NVIDIA, AT\u0026T, IBM, eBay, Shopee, and Walmart.\n\n\n### Milvus Key Features\n\nAs a cloud-native vector database, Milvus boasts the following key features: \n\n- High performance and millisecond search on billion-scale vector datasets.\n\n- Multi-language support and toolchain.\n\n- Horizontal scalability and high reliability even in the event of a disruption.\n\n- [Hybrid search](https://zilliz.com/blog/a-review-of-hybrid-search-in-milvus), achieved by pairing scalar filtering with vector similarity search.\n\n\n### Milvus Architecture \n\nMilvus follows the principle of separating data flow and control flow. The system breaks down into four levels, as shown in the diagram:\n\n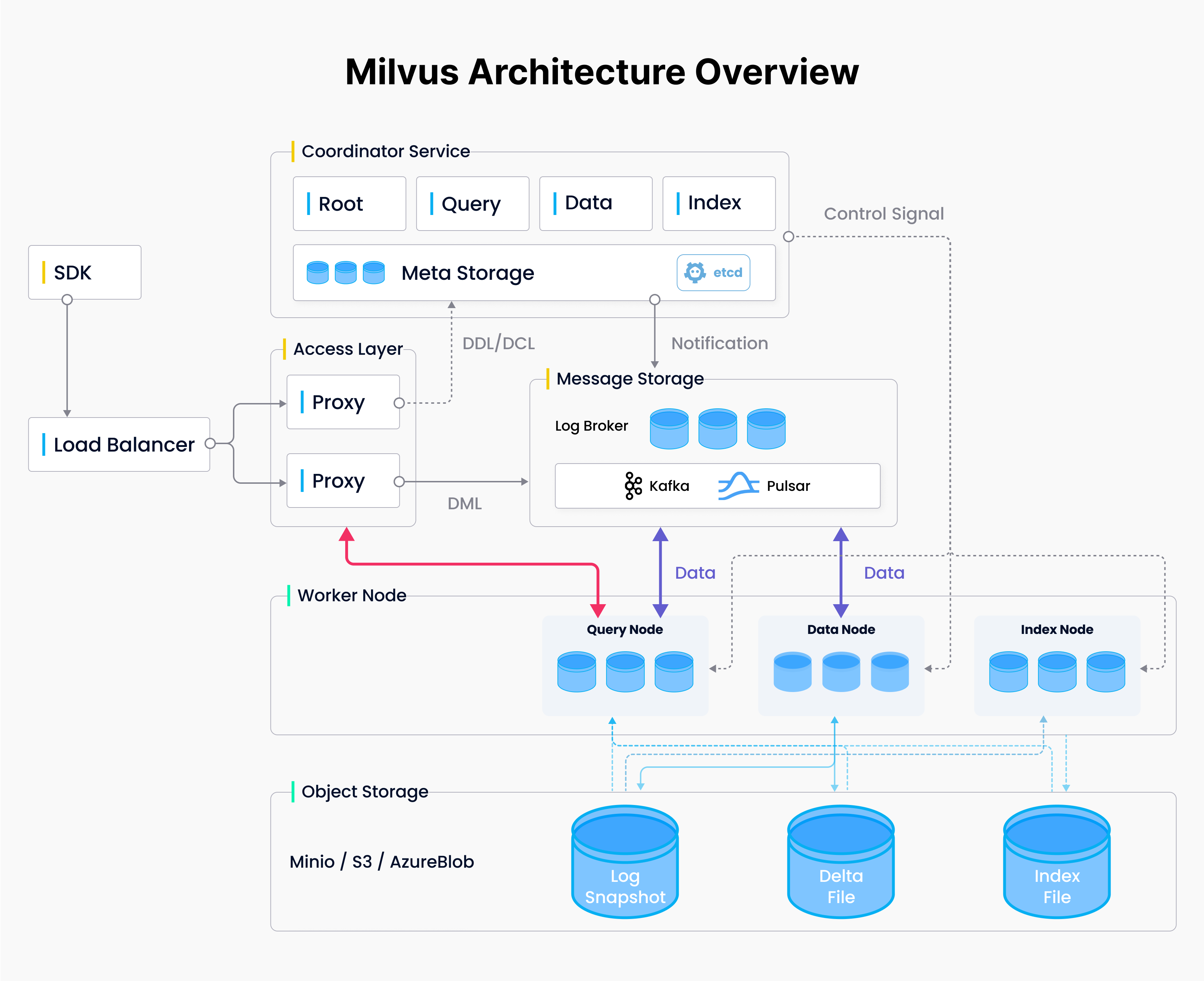\n\n_Figure 2 Milvus Architecture_ \n\n- **Access layer:** The access layer is composed of a group of stateless proxies and serves as the system's front layer and endpoint to users.\n\n- **Coordinator service:** The coordinator service assigns tasks to the worker nodes.\n\n- **Worker nodes:** The worker nodes are dumb executors that follow instructions from the coordinator service and execute user-triggered DML/DDL commands. \n\n- **Storage:** Storage is responsible for data persistence. It comprises a meta storage, log broker, and object storage.\n\n\n### Milvus Deployment Options\n\nMilvus supports three running modes: [Milvus Lite, Standalone, and Distributed](https://milvus.io/docs/install-overview.md). \n\n- **Milvus Lite** is a Python library that can be imported into local applications. As a lightweight version of Milvus, it is ideal for quick prototyping in Jupyter Notebooks or running on smart devices with limited resources.\n\n- **Milvus Standalone i**s a single-machine server deployment. If you have a production workload but prefer not to use Kubernetes, running Milvus Standalone on a single machine with sufficient memory is a good option.\n\n- **Milvus Distributed** can be deployed on Kubernetes clusters. It supports larger datasets, higher availability, and scalability, and is more suitable for production environments.\n\nMilvus is designed from the start to support Kubernetes, and can be easily deployed on AWS. We can use Amazon Elastic Kubernetes Service (Amazon EKS) as the managed Kubernetes, Amazon S3 as the Object Storage, Amazon Managed Streaming for Apache Kafka (Amazon MSK) as the Message storage, and Amazon Elastic Load Balancing (Amazon ELB) as the Load Balancer to build a reliable, elastic Milvus database cluster.\n\nNext, we'll provide step-by-step guidance on deploying a Milvus cluster using EKS and other services. \n\n\n## Deploying Milvus on AWS EKS \n\n### Prerequisites\n\nWe’ll use AWS CLI to create an EKS cluster and deploy a Milvus database. The following prerequisites are required:\n\n- A PC/Mac or Amazon EC2 instance with[ AWS CLI](https://docs.aws.amazon.com/cli/latest/userguide/getting-started-install.html) installed and configured with appropriate permissions. The AWS CLI tools are installed by default if you use Amazon Linux 2 or Amazon Linux 2023.\n\n- [EKS tools installed](https://docs.aws.amazon.com/eks/latest/userguide/getting-started.html), including Helm, Kubectl, eksctl, etc.\n\n- An Amazon S3 bucket.\n\n- An Amazon MSK instance.\n\n\n### Considerations when creating MSK\n\n- The latest stable version of Milvus (v2.3.13) depends on Kafka's `autoCreateTopics` feature. So when creating MSK, we need to use a custom configuration and change the `auto.create.topics.enable` property from the default `false` to `true`. In addition, to increase the message throughput of MSK, it is recommended that the values of `message.max.bytes` and `replica.fetch.max.bytes` be increased. See [Custom MSK configurations](https://docs.aws.amazon.com/msk/latest/developerguide/msk-configuration-properties.html) for details.\n\n```\nauto.create.topics.enable=true\nmessage.max.bytes=10485880\nreplica.fetch.max.bytes=20971760\n```\n\n- Milvus does not support MSK's IAM role-based authentication. So, when creating MSK, enable `SASL/SCRAM authentication` option in the security configuration, and configure `username` and `password` in the AWS Secrets Manager. See [Sign-in credentials authentication with AWS Secrets Manager](https://docs.aws.amazon.com/msk/latest/developerguide/msk-password.html) for details.\n\n\n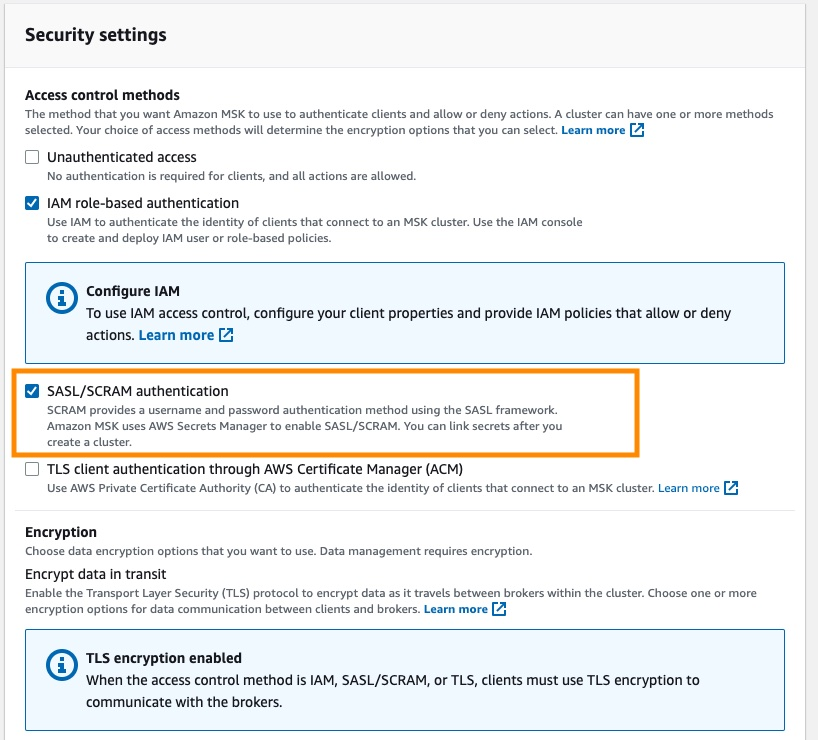\n\n_Figure 3: Security settings: enable SASL/SCRAM authentication_\n\n\n- We need to enable access to the MSK security group from the EKS cluster’s security group or IP address range. \n\n\n### Creating an EKS Cluster\n\nThere are many ways to create an EKS cluster, such as via the console, CloudFormation, eksctl, etc. This post will show how to create an EKS cluster using eksctl.\n\n`eksctl` is a simple command-line tool for creating and managing Kubernetes clusters on Amazon EKS. It provides the fastest and easiest way to create a new cluster with nodes for Amazon EKS. See eksctl’s [website](https://eksctl.io/) for more information. \n\n1. First, create an `eks_cluster.yaml` file with the following code snippet. Replace `cluster-name` with your cluster name, replace `region-code` with the AWS region where you want to create the cluster and replace `private-subnet-idx` with your private subnets. \nNote: This configuration file creates an EKS cluster in an existing VPC by specifying private subnets. If you want to create a new VPC, remove the VPC and subnets configuration, and then the `eksctl` will automatically create a new one.\n\n```\napiVersion: eksctl.io/v1alpha5\nkind: ClusterConfig\n\nmetadata:\n name: \u003ccluster-name\u003e\n region: \u003cregion-code\u003e\n version: \"1.26\"\n\niam:\n withOIDC: true\n\n serviceAccounts:\n - metadata:\n name: aws-load-balancer-controller\n namespace: kube-system\n wellKnownPolicies:\n awsLoadBalancerController: true\n - metadata:\n name: milvus-s3-access-sa\n # if no namespace is set, \"default\" will be used;\n # the namespace will be created if it doesn't exist already\n namespace: milvus\n labels: {aws-usage: \"milvus\"}\n attachPolicyARNs:\n - \"arn:aws:iam::aws:policy/AmazonS3FullAccess\"\n\n# Use existed VPC to create EKS.\n# If you don't config vpc subnets, eksctl will automatically create a brand new VPC\nvpc:\n subnets:\n private:\n us-west-2a: { id: \u003cprivate-subnet-id1\u003e }\n us-west-2b: { id: \u003cprivate-subnet-id2\u003e }\n us-west-2c: { id: \u003cprivate-subnet-id3\u003e }\n\nmanagedNodeGroups:\n - name: ng-1-milvus\n labels: { role: milvus }\n instanceType: m6i.2xlarge\n desiredCapacity: 3\n privateNetworking: true\n \naddons:\n- name: vpc-cni # no version is specified so it deploys the default version\n attachPolicyARNs:\n - arn:aws:iam::aws:policy/AmazonEKS_CNI_Policy\n- name: coredns\n version: latest # auto discovers the latest available\n- name: kube-proxy\n version: latest\n- name: aws-ebs-csi-driver\n wellKnownPolicies: # add IAM and service account\n ebsCSIController: true\n```\n\n\n2. Then, run the `eksctl` command to create the EKS cluster. \n\n```\neksctl create cluster -f eks_cluster.yaml\n```\n\n\nThis command will create the following resources:\n\n- An EKS cluster with the specified version.\n\n- A managed node group with three m6i.2xlarge EC2 instances.\n\n- An [IAM OIDC identity provider](https://docs.aws.amazon.com/en_us/eks/latest/userguide/enable-iam-roles-for-service-accounts.html) and a ServiceAccount called `aws-load-balancer-controller`, which we will use later when installing the **AWS Load Balancer Controller**.\n\n- A namespace `milvus` and a ServiceAccount `milvus-s3-access-sa` within this namespace. This namespace will be used later when configuring S3 as the object storage for Milvus.\n\n Note: For simplicity, the `milvus-s3-access-sa` here is granted full S3 access permissions. In production deployments, it's recommended to follow the principle of least privilege and only grant access to the specific S3 bucket used for Milvus.\n\n- Multiple add-ons, where `vpc-cni`, `coredns`, `kube-proxy` are core add-ons required by EKS. `aws-ebs-csi-driver` is the AWS EBS CSI driver that allows EKS clusters to manage the lifecycle of Amazon EBS volumes.\n\n\nNow, we just need to wait for the cluster creation to complete. \n\nWait for the cluster creation to complete. During the cluster creation process, the `kubeconfig` file will be automatically created or updated. You can also manually update it by running the following command. Make sure to replace `region-code` with the AWS region where your cluster is being created, and replace `cluster-name` with the name of your cluster.\n\n```\naws eks update-kubeconfig --region \u003cregion-code\u003e --name \u003ccluster-name\u003e\n```\n\nOnce the cluster is created, you can view nodes by running:\n\n```\nkubectl get nodes -A -o wide\n```\n\n\n3. Create a `ebs-sc` StorageClass configured with GP3 as the storage type, and set it as the default StorageClass. Milvus uses etcd as its Meta Storage and needs this StorageClass to create and manage PVCs. \n\n```\ncat \u003c\u003cEOF | kubectl apply -f -\napiVersion: storage.k8s.io/v1\nkind: StorageClass\nmetadata:\n name: ebs-sc\n annotations:\n storageclass.kubernetes.io/is-default-class: \"true\"\nprovisioner: ebs.csi.aws.com\nvolumeBindingMode: WaitForFirstConsumer\nparameters:\n type: gp3\nEOF\n```\n\nThen, set the original `gp2` StorageClass to non-default:\n\n```\nkubectl patch storageclass gp2 -p '{\"metadata\": {\"annotations\":{\"storageclass.kubernetes.io/is-default-class\":\"false\"}}}'\n```\n\n\n4. Install the AWS Load Balancer Controller. We will use this controller later for the Milvus Service and Attu Ingress, so let's install it beforehand. \n\n- First, add the `eks-charts` repo and update it. \n\n```\nhelm repo add eks https://aws.github.io/eks-charts\nhelm repo update\n```\n\n\n- Next, install the AWS Load Balancer Controller. Replace `cluster-name` with your cluster name. The ServiceAccount named `aws-load-balancer-controller` was already created when we created the EKS cluster in previous steps. \n\n```\nhelm install aws-load-balancer-controller eks/aws-load-balancer-controller \\\n -n kube-system \\\n --set clusterName=\u003ccluster-name\u003e \\\n --set serviceAccount.create=false \\\n --set serviceAccount.name=aws-load-balancer-controller\n```\n\n\n- Verify if the controller was installed successfully.\n\n```\nkubectl get deployment -n kube-system aws-load-balancer-controller\n```\n\n\n- The output should look like:\n\n```\nNAME READY UP-TO-DATE AVAILABLE AGE\naws-load-balancer-controller 2/2 2 2 12m\n```\n\n\n\n### Deploying a Milvus Cluster\n\nMilvus supports multiple deployment methods, such as Operator and Helm. Operator is simpler, but Helm is more direct and flexible. We’ll use Helm to deploy Milvus in this example. \n\nWhen deploying Milvus with Helm, you can customize the configuration via the `values.yaml` file. Click [values.yaml](https://raw.githubusercontent.com/milvus-io/milvus-helm/master/charts/milvus/values.yaml) to view all the options. By default, Milvus creates in-cluster minio and pulsar as the Object Storage and Message Storage, respectively. We will make some configuration changes to make it more suitable for production. \n\n1. First, add the Milvus Helm repo and update it.\n\n```\nhelm repo add milvus https://zilliztech.github.io/milvus-helm/\nhelm repo update\n```\n\n\n2. Create a `milvus_cluster.yaml` file with the following code snippet. This code snippet customizes Milvus's configuration, such as configuring Amazon S3 as the object storage and Amazon MSK as the message queue. We’ll provide detailed explanations and configuration guidance later. \n\n```\n#####################################\n# Section 1\n#\n# Configure S3 as the Object Storage\n#####################################\n\n# Service account\n# - this service account are used by External S3 access\nserviceAccount:\n create: false\n name: milvus-s3-access-sa\n\n# Close in-cluster minio\nminio:\n enabled: false\n\n# External S3\n# - these configs are only used when `externalS3.enabled` is true\nexternalS3:\n enabled: true\n host: \"s3.\u003cregion-code\u003e.amazonaws.com\"\n port: \"443\"\n useSSL: true\n bucketName: \"\u003cbucket-name\u003e\"\n rootPath: \"\u003croot-path\u003e\"\n useIAM: true\n cloudProvider: \"aws\"\n iamEndpoint: \"\"\n\n#####################################\n# Section 2\n#\n# Configure MSK as the Message Storage\n#####################################\n\n# Close in-cluster pulsar\npulsar:\n enabled: false\n\n# External kafka\n# - these configs are only used when `externalKafka.enabled` is true\nexternalKafka:\n enabled: true\n brokerList: \"\u003cbroker-list\u003e\"\n securityProtocol: SASL_SSL\n sasl:\n mechanisms: SCRAM-SHA-512\n username: \"\u003cusername\u003e\"\n password: \"\u003cpassword\u003e\"\n \n#####################################\n# Section 3\n#\n# Expose the Milvus service to be accessed from outside the cluster (LoadBalancer service).\n# or access it from within the cluster (ClusterIP service). Set the service type and the port to serve it.\n#####################################\nservice:\n type: LoadBalancer\n port: 19530\n annotations:\n service.beta.kubernetes.io/aws-load-balancer-type: external #AWS Load Balancer Controller fulfills services that has this annotation\n service.beta.kubernetes.io/aws-load-balancer-name : milvus-service #User defined name given to AWS Network Load Balancer\n service.beta.kubernetes.io/aws-load-balancer-scheme: internal # internal or internet-facing, later allowing for public access via internet\n service.beta.kubernetes.io/aws-load-balancer-nlb-target-type: ip #The Pod IPs should be used as the target IPs (rather than the node IPs)\n \n#####################################\n# Section 4\n#\n# Installing Attu the Milvus management GUI\n#####################################\nattu:\n enabled: true\n name: attu\n ingress:\n enabled: true\n annotations:\n kubernetes.io/ingress.class: alb # Annotation: set ALB ingress type\n alb.ingress.kubernetes.io/scheme: internet-facing #Places the load balancer on public subnets\n alb.ingress.kubernetes.io/target-type: ip #The Pod IPs should be used as the target IPs (rather than the node IPs)\n alb.ingress.kubernetes.io/group.name: attu # Groups multiple Ingress resources\n hosts:\n -\n \n#####################################\n# Section 5\n#\n# HA deployment of Milvus Core Components\n#####################################\nrootCoordinator:\n replicas: 2\n activeStandby:\n enabled: true # Enable active-standby when you set multiple replicas for root coordinator\n resources:\n limits:\n cpu: 1\n memory: 2Gi\nindexCoordinator:\n replicas: 2\n activeStandby:\n enabled: true # Enable active-standby when you set multiple replicas for index coordinator\n resources:\n limits:\n cpu: \"0.5\"\n memory: 0.5Gi\nqueryCoordinator:\n replicas: 2\n activeStandby:\n enabled: true # Enable active-standby when you set multiple replicas for query coordinator\n resources:\n limits:\n cpu: \"0.5\"\n memory: 0.5Gi\ndataCoordinator:\n replicas: 2\n activeStandby:\n enabled: true # Enable active-standby when you set multiple replicas for data coordinator\n resources:\n limits:\n cpu: \"0.5\"\n memory: 0.5Gi\nproxy:\n replicas: 2\n resources:\n limits:\n cpu: 1\n memory: 4Gi\n\n#####################################\n# Section 6\n#\n# Milvus Resource Allocation\n#####################################\nqueryNode:\n replicas: 1\n resources:\n limits:\n cpu: 2\n memory: 8Gi\ndataNode:\n replicas: 1\n resources:\n limits:\n cpu: 1\n memory: 4Gi\nindexNode:\n replicas: 1\n resources:\n limits:\n cpu: 4\n memory: 8Gi\n```\n\n\nThe code contains six sections. Follow the following instructions to change the corresponding configurations.\n\n**Section 1**: Configure S3 as Object Storage. The serviceAccount grants Milvus access to S3 (in this case, it is `milvus-s3-access-sa`, which was created when we created the EKS cluster). Make sure to replace `\u003cregion-code\u003e` with the AWS region where your cluster is located. Replace `\u003cbucket-name\u003e` with the name of your S3 bucket and `\u003croot-path\u003e` with the prefix for the S3 bucket (this field can be left empty).\n\n**Section 2**: Configure MSK as Message Storage. Replace `\u003cbroker-list\u003e` with the endpoint addresses corresponding to the SASL/SCRAM authentication type of MSK. Replace `\u003cusername\u003e` and `\u003cpassword\u003e` with the MSK account username and password. You can get the `\u003cbroker-list\u003e` from MSK client information, as shown in the image below. \n\n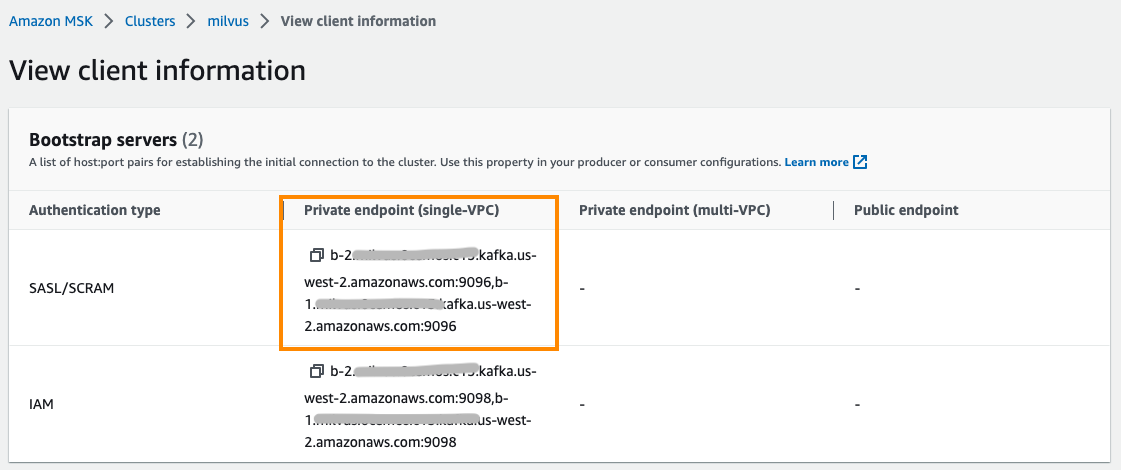\n\n_Figure 4: Configure MSK as the Message Storage of Milvus_ \n\n**Section 3:** Expose Milvus service and enable access from outside the cluster. Milvus endpoint used ClusterIP type service by default, which is only accessible within the EKS cluster. If needed, you can change it to LoadBalancer type to allow access from outside the EKS cluster. The LoadBalancer type Service uses Amazon NLB as the load balancer. According to security best practices, `aws-load-balancer-scheme` is configured as internal mode by default here, which means only intranet access to Milvus is allowed. Click to [view the NLB configuration instructions](https://docs.aws.amazon.com/eks/latest/userguide/network-load-balancing.html). \n\n**Section 4:** Install and configure [Attu](https://github.com/zilliztech/attu), an open-source milvus administration tool. It has an intuitive GUI that allows you to easily interact with Milvus. We enable Attu, configure ingress using AWS ALB, and set it to `internet-facing` type so that Attu can be accessed via the Internet. Click [this document](https://docs.aws.amazon.com/eks/latest/userguide/alb-ingress.html) for the guide to ALB configuration.\n\n**Section 5:** Enable HA deployment of Milvus Core Components. Milvus contains multiple independent and decoupled components. For example, the coordinator service acts as the control layer, handling coordination for the Root, Query, Data, and Index components. The Proxy in the access layer serves as the database access endpoint. These components default to only 1 pod replica. Deploying multiple replicas of these service components is especially necessary to improve Milvus availability.\n\n**Note:** The multi-replica deployment of the Root, Query, Data, and Index coordinator components requires the `activeStandby` option enabled.\n\n**Section 6:** Adjust resource allocation for Milvus components to meet your workloads' requirements. The Milvus website also provides a [sizing tool](https://milvus.io/tools/sizing/) to generate configuration suggestions based on data volume, vector dimensions, index types, etc. It can also generate a Helm configuration file with just one click. The following configuration is the suggestion given by the tool for 1 million 1024 dimensions vectors and HNSW index type. \n\n1. Use Helm to create Milvus (deployed in namespace `milvus`). Note: You can replace `\u003cdemo\u003e` with a custom name. \n\n```\nhelm install \u003cdemo\u003e milvus/milvus -n milvus -f milvus_cluster.yaml\n```\n\n2. Run the following command to check the deployment status. \n\n```\nkubectl get deployment -n milvus\n```\n\n\nThe following output shows that Milvus components are all AVAILABLE, and coordination components have multiple replicas enabled.\n\n```\nNAME READY UP-TO-DATE AVAILABLE AGE\ndemo-milvus-attu 1/1 1 1 5m27s\ndemo-milvus-datacoord 2/2 2 2 5m27s\ndemo-milvus-datanode 1/1 1 1 5m27s\ndemo-milvus-indexcoord 2/2 2 2 5m27s\ndemo-milvus-indexnode 1/1 1 1 5m27s\ndemo-milvus-proxy 2/2 2 2 5m27s\ndemo-milvus-querycoord 2/2 2 2 5m27s\ndemo-milvus-querynode 1/1 1 1 5m27s\ndemo-milvus-rootcoord 2/2 2 2 5m27s\n```\n\n\n\n### Accessing and Managing Milvus\n\nSo far, we have successfully deployed the Milvus vector database. Now, we can access Milvus through endpoints. Milvus exposes endpoints via Kubernetes services. Attu exposes endpoints via Kubernetes Ingress.\n\n\n#### **Accessing Milvus endpoints**\n\nRun the following command to get service endpoints: \n\n```\nkubectl get svc -n milvus\n```\n\n\nYou can view several services. Milvus supports two ports, port `19530` and port `9091`:\n\n- Port `19530` is for gRPC and RESTful API. It is the default port when you connect to a Milvus server with different Milvus SDKs or HTTP clients.\n- Port `9091` is a management port for metrics collection, pprof profiling, and health probes within Kubernetes.\n\nThe `demo-milvus` service provides a database access endpoint, which is used to establish a connection from clients. It uses NLB as the service load balancer. You can get the service endpoint from the `EXTERNAL-IP` column. \n\n```\nNAME TYPE CLUSTER-IP EXTERNAL-IP PORT(S) AGE\ndemo-etcd ClusterIP 172.20.103.138 \u003cnone\u003e 2379/TCP,2380/TCP 62m\ndemo-etcd-headless ClusterIP None \u003cnone\u003e 2379/TCP,2380/TCP 62m\ndemo-milvus LoadBalancer 172.20.219.33 milvus-nlb-xxxx.elb.us-west-2.amazonaws.com 19530:31201/TCP,9091:31088/TCP 62m\ndemo-milvus-datacoord ClusterIP 172.20.214.106 \u003cnone\u003e 13333/TCP,9091/TCP 62m\ndemo-milvus-datanode ClusterIP None \u003cnone\u003e 9091/TCP 62m\ndemo-milvus-indexcoord ClusterIP 172.20.106.51 \u003cnone\u003e 31000/TCP,9091/TCP 62m\ndemo-milvus-indexnode ClusterIP None \u003cnone\u003e 9091/TCP 62m\ndemo-milvus-querycoord ClusterIP 172.20.136.213 \u003cnone\u003e 19531/TCP,9091/TCP 62m\ndemo-milvus-querynode ClusterIP None \u003cnone\u003e 9091/TCP 62m\ndemo-milvus-rootcoord ClusterIP 172.20.173.98 \u003cnone\u003e 53100/TCP,9091/TCP 62m\n```\n\n#### **Managing Milvus using Attu**\n\nAs described before, we have installed Attu to manage Milvus. Run the following command to get the endpoint:\n\n```\nkubectl get ingress -n milvus\n```\n\nYou can see an Ingress called `demo-milvus-attu`, where the `ADDRESS` column is the access URL. \n\n```\nNAME \tCLASS\tHOSTS ADDRESS PORTS AGE\ndemo-milvus-attu \u003cnone\u003e * \tk8s-attu-xxxx.us-west-2.elb.amazonaws.com 80 \t27s\n```\n\n\nOpen the Ingress address in a browser and see the following page. Click **Connect** to log in. \n\n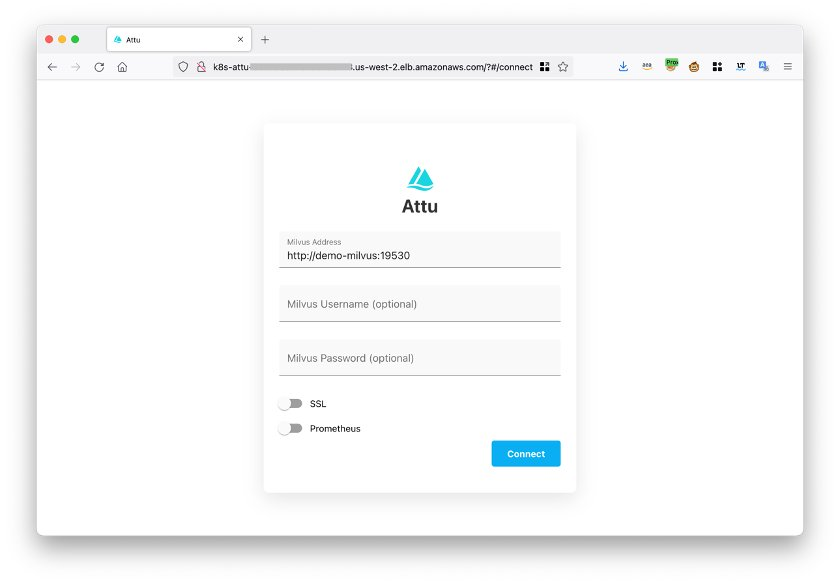\n\n_Figure 5: Log in to your Attu account_ \n\nAfter logging in, you can manage Milvus databases through Attu.\n\n\n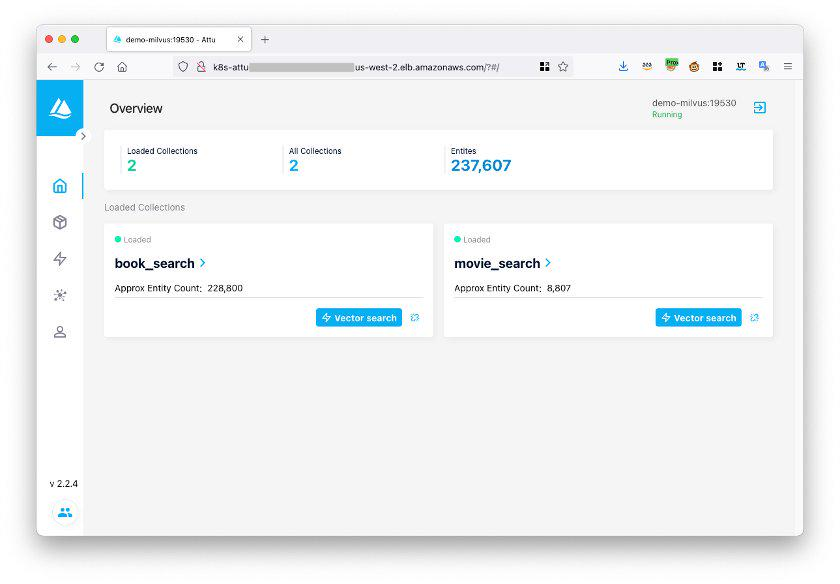\n\nFigure 6: The Attu interface \n\n\n## Testing the Milvus vector database\n\nWe will use the Milvus [example code](https://milvus.io/docs/example_code.md) to test if the Milvus database is working properly. First, download the `hello_milvus.py` example code using the following command: \n\n```\nwget https://raw.githubusercontent.com/milvus-io/pymilvus/master/examples/hello_milvus.py\n```\n\nModify the host in the example code to the Milvus service endpoint. \n\n```\nprint(fmt.format(\"start connecting to Milvus\"))\nconnections.connect(\"default\", host=\"milvus-nlb-xxx.elb.us-west-2.amazonaws.com\", port=\"19530\")\n```\n\n\nRun the code:\n\n```\npython3 hello_milvus.py\n```\n\nIf the system returns the following result, then it indicates that Milvus is running normally.\n\n```\n=== start connecting to Milvus ===\nDoes collection hello_milvus exist in Milvus: False\n=== Create collection `hello_milvus` ===\n=== Start inserting entities ===\nNumber of entities in Milvus: 3000\n=== Start Creating index IVF_FLAT ===\n=== Start loading ===\n```\n\n## Conclusion\n\nThis post introduces [Milvus](https://milvus.io/intro), one of the most popular open-source vector databases, and provides a guide on deploying Milvus on AWS using managed services such as Amazon EKS, S3, MSK, and ELB to achieve greater elasticity and reliability.\n\nAs a core component of various GenAI systems, particularly Retrieval Augmented Generation (RAG), Milvus supports and integrates with a variety of mainstream GenAI models and frameworks, including Amazon Sagemaker, PyTorch, HuggingFace, LlamaIndex, and LangChain. Start your GenAI innovation journey with Milvus today!\n\n\n## References\n\n- [Amazon EKS User Guide](https://docs.aws.amazon.com/eks/latest/userguide/getting-started.html)\n- [Milvus Official Website](https://milvus.io/)\n- [Milvus GitHub Repository](https://github.com/milvus-io/milvus)\n- [eksctl Official Website](https://eksctl.io/)","title":"How to Deploy the Open-Source Milvus Vector Database on Amazon EKS","metaData":{}},{"id":"introducing-pymilvus-integrations-with-embedding-models.md","author":"Stephen Batifol","tags":["Engineering"],"recommend":true,"canonicalUrl":"https://milvus.io/blog/introducing-pymilvus-integrations-with-embedding-models.md","date":"2024-06-05T00:00:00.000Z","cover":"https://assets.zilliz.com/Getting_started_with_Milvus_cluster_and_K8s_1_34b2c81802.png","href":"/blog/introducing-pymilvus-integrations-with-embedding-models.md","content":"\n[Milvus](https://milvus.io/intro) is an open-source vector database designed specifically for AI applications. Whether you're working on machine learning, deep learning, or any other AI-related project, Milvus offers a robust and efficient way to handle large-scale vector data.\n\nNow, with the [model module integration](https://milvus.io/docs/embeddings.md) in PyMilvus, the Python SDK for Milvus, it's even easier to add Embedding and Reranking models. This integration simplifies transforming your data into searchable vectors or reranking results for more accurate outcomes, such as in [Retrieval Augmented Generation (RAG)](https://zilliz.com/learn/Retrieval-Augmented-Generation).\n\nIn this blog, we will review dense embedding models, sparse embedding models, and re-rankers and demonstrate how to use them in practice using [Milvus Lite](https://milvus.io/blog/introducing-milvus-lite.md), a lightweight version of Milvus that can run locally in your Python applications. \n\n\n## Dense vs Sparse Embeddings \n\nBefore we walk you through how to use our integrations, let’s look at two main categories of vector embeddings. \n\n[Vector Embeddings](https://zilliz.com/glossary/vector-embeddings) generally fall into two main categories: [**Dense Embeddings** and **Sparse Embeddings**](https://zilliz.com/learn/sparse-and-dense-embeddings).\n\n- Dense Embeddings are high-dimensional vectors in which most or all elements are non-zero, making them ideal for encoding text semantics or fuzzy meaning.\n\n- Sparse Embeddings are high-dimensional vectors with many zero elements, better suited for encoding exact or adjacent concepts.\n\nMilvus supports both types of embeddings and offers hybrid search. [Hybrid Search](https://zilliz.com/blog/hybrid-search-with-milvus) allows you to conduct searches across various vector fields within the same collection. These vectors can represent different facets of data, use diverse embedding models, or employ distinct data processing methods, combining the results using re-rankers.\n\n## How to Use Our Embedding and Reranking Integrations\n\nIn the following sections, we’ll demonstrate three practical examples of using our integrations to generate embeddings and conduct vector searches. \n\n### Example 1: Use the Default Embedding Function to Generate Dense Vectors \n\nYou must install the `pymilvus` client with the `model` package to use embedding and reranking functions with Milvus. \n\n```\npip install \"pymilvus[model]\"\n```\n\nThis step will install [Milvus Lite](https://milvus.io/docs/quickstart.md), allowing you to run Milvus locally within your Python application. It also includes the model subpackage, which includes all utilities for Embedding and reranking.\n\nThe model subpackage supports various embedding models, including those from OpenAI, [Sentence Transformers](https://zilliz.com/learn/Sentence-Transformers-for-Long-Form-Text), [BGE-M3](https://zilliz.com/learn/bge-m3-and-splade-two-machine-learning-models-for-generating-sparse-embeddings), BM25, [SPLADE](https://zilliz.com/learn/bge-m3-and-splade-two-machine-learning-models-for-generating-sparse-embeddings), and Jina AI pre-trained models.\n\nThis example uses the `DefaultEmbeddingFunction`, based on the `all-MiniLM-L6-v2` Sentence Transformer model for simplicity. The model is about 70MB and will be downloaded during the first use:\n\n```\nfrom pymilvus import model\n\n# This will download \"all-MiniLM-L6-v2\", a lightweight model.\nef = model.DefaultEmbeddingFunction()\n\n# Data from which embeddings are to be generated\ndocs = [\n \"Artificial intelligence was founded as an academic discipline in 1956.\",\n \"Alan Turing was the first person to conduct substantial research in AI.\",\n \"Born in Maida Vale, London, Turing was raised in southern England.\",\n]\n\nembeddings = ef.encode_documents(docs)\n\nprint(\"Embeddings:\", embeddings)\n# Print dimension and shape of embeddings\nprint(\"Dim:\", ef.dim, embeddings[0].shape)\n```\n\nThe expected output should be something like the following: \n\n```\nEmbeddings: [array([-3.09392996e-02, -1.80662833e-02, 1.34775648e-02, 2.77156215e-02,\n -4.86349640e-03, -3.12581174e-02, -3.55921760e-02, 5.76934684e-03,\n 2.80773244e-03, 1.35783911e-01, 3.59678417e-02, 6.17732145e-02,\n...\n -4.61330153e-02, -4.85207550e-02, 3.13997865e-02, 7.82178566e-02,\n -4.75336798e-02, 5.21207601e-02, 9.04406682e-02, -5.36676683e-02],\n dtype=float32)]\nDim: 384 (384,)\n```\n\n\n### Example 2: Generate Sparse Vectors Using The BM25 Model\n\nBM25 is a well-known method that uses word occurrence frequencies to determine the relevance between queries and documents. In this example, we’ll show how to use `BM25EmbeddingFunction` to generate sparse embeddings for queries and documents.\n\nIn BM25, it's important to calculate the statistics in your documents to obtain the IDF (Inverse Document Frequency), which can represent the patterns in your documents. The IDF measures how much information a word provides, whether it's common or rare across all documents.\n\n```\nfrom pymilvus.model.sparse import BM25EmbeddingFunction\n\n# 1. Prepare a small corpus to search\ndocs = [\n \"Artificial intelligence was founded as an academic discipline in 1956.\",\n \"Alan Turing was the first person to conduct substantial research in AI.\",\n \"Born in Maida Vale, London, Turing was raised in southern England.\",\n]\nquery = \"Where was Turing born?\"\nbm25_ef = BM25EmbeddingFunction()\n\n# 2. Fit the corpus to get BM25 model parameters on your documents.\nbm25_ef.fit(docs)\n\n# 3. Store the fitted parameters to expedite future processing.\nbm25_ef.save(\"bm25_params.json\")\n\n# 4. Load the saved params\nnew_bm25_ef = BM25EmbeddingFunction()\nnew_bm25_ef.load(\"bm25_params.json\")\n\ndocs_embeddings = new_bm25_ef.encode_documents(docs)\nquery_embeddings = new_bm25_ef.encode_queries([query])\nprint(\"Dim:\", new_bm25_ef.dim, list(docs_embeddings)[0].shape)\n```\n\n### Example 3: Using a ReRanker \n\nA search system aims to find the most relevant results quickly and efficiently. Traditionally, methods like BM25 or TF-IDF have been used to rank search results based on keyword matching. Recent methods, such as embedding-based cosine similarity, are straightforward but can sometimes miss the subtleties of language and, most importantly, the interaction between documents and a query's intent.\n\nThis is where using a [re-ranker](https://zilliz.com/learn/optimize-rag-with-rerankers-the-role-and-tradeoffs) helps. A re-ranker is an advanced AI model that takes the initial set of results from a search—often provided by an embeddings/token-based search—and re-evaluates them to ensure they align more closely with the user's intent. It looks beyond the surface-level matching of terms to consider the deeper interaction between the search query and the content of the documents.\n\nFor this example, we’ll use the [Jina AI Reranker](https://milvus.io/docs/integrate_with_jina.md).\n\n\n\n```\nfrom pymilvus.model.reranker import JinaRerankFunction\n\njina_api_key = \"\u003cYOUR_JINA_API_KEY\u003e\"\n\nrf = JinaRerankFunction(\"jina-reranker-v1-base-en\", jina_api_key)\n\nquery = \"What event in 1956 marked the official birth of artificial intelligence as a discipline?\"\n\ndocuments = [\n \"In 1950, Alan Turing published his seminal paper, 'Computing Machinery and Intelligence,' proposing the Turing Test as a criterion of intelligence, a foundational concept in the philosophy and development of artificial intelligence.\",\n \"The Dartmouth Conference in 1956 is considered the birthplace of artificial intelligence as a field; here, John McCarthy and others coined the term 'artificial intelligence' and laid out its basic goals.\",\n \"In 1951, British mathematician and computer scientist Alan Turing also developed the first program designed to play chess, demonstrating an early example of AI in game strategy.\",\n \"The invention of the Logic Theorist by Allen Newell, Herbert A. Simon, and Cliff Shaw in 1955 marked the creation of the first true AI program, which was capable of solving logic problems, akin to proving mathematical theorems.\"\n]\n\nresults = rf(query, documents)\n\nfor result in results:\n print(f\"Index: {result.index}\")\n print(f\"Score: {result.score:.6f}\")\n print(f\"Text: {result.text}\\n\")\n```\n\nThe expected output is similar to the following:\n\n```\nIndex: 1\nScore: 0.937096\nText: The Dartmouth Conference in 1956 is considered the birthplace of artificial intelligence as a field; here, John McCarthy and others coined the term 'artificial intelligence' and laid out its basic goals.\n\nIndex: 3\nScore: 0.354210\nText: The invention of the Logic Theorist by Allen Newell, Herbert A. Simon, and Cliff Shaw in 1955 marked the creation of the first true AI program, which was capable of solving logic problems, akin to proving mathematical theorems.\n\nIndex: 0\nScore: 0.349866\nText: In 1950, Alan Turing published his seminal paper, 'Computing Machinery and Intelligence,' proposing the Turing Test as a criterion of intelligence, a foundational concept in the philosophy and development of artificial intelligence.\n\nIndex: 2\nScore: 0.272896\nText: In 1951, British mathematician and computer scientist Alan Turing also developed the first program designed to play chess, demonstrating an early example of AI in game strategy.\n```\n\n## Star Us On GitHub and Join Our Discord!\n\nIf you liked this blog post, consider starring Milvus on [GitHub](https://github.com/milvus-io/milvus), and feel free to join our [Discord](https://discord.gg/FG6hMJStWu)! 💙\n","title":"Introducing PyMilvus Integration with Embedding Models","metaData":{}},{"id":"introducing-milvus-lite.md","author":"Jiang Chen","tags":["News"],"recommend":true,"canonicalUrl":"https://milvus.io/blog/introducing-milvus-lite.md","date":"2024-05-30T00:00:00.000Z","cover":"https://assets.zilliz.com/introducing_Milvus_Lite_76ed4baf75.jpeg","href":"/blog/introducing-milvus-lite.md","content":"\n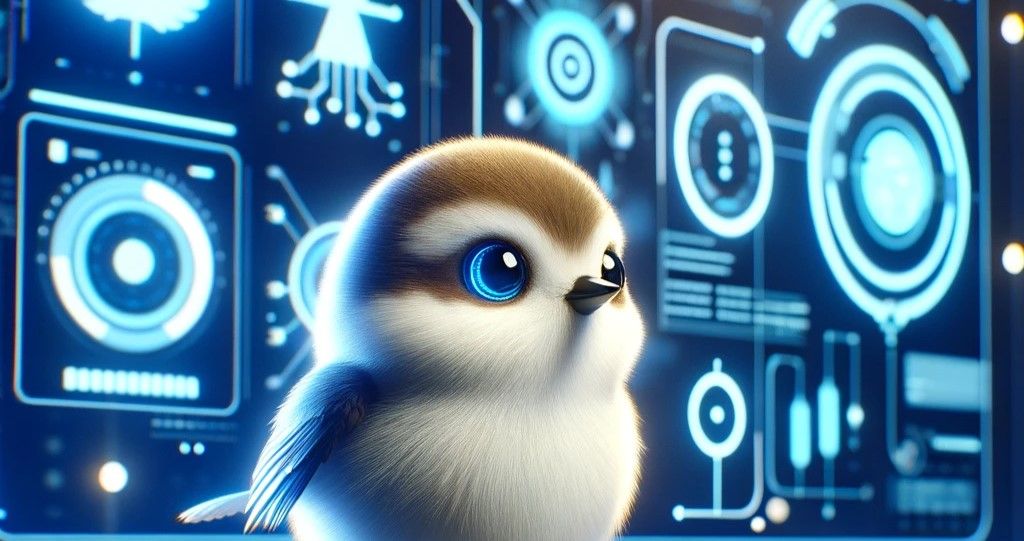\n\nWe are excited to introduce [Milvus Lite](https://milvus.io/docs/milvus_lite.md), a lightweight vector database that runs locally within your Python application. Based on the popular open-source [Milvus](https://milvus.io/intro) vector database, Milvus Lite reuses the core components for vector indexing and query parsing while removing elements designed for high scalability in distributed systems. This design makes a compact and efficient solution ideal for environments with limited computing resources, such as laptops, Jupyter Notebooks, and mobile or edge devices.\n\nMilvus Lite integrates with various AI development stacks like LangChain and LlamaIndex, enabling its use as a vector store in Retrieval Augmented Generation (RAG) pipelines without the need for server setup. Simply run `pip install pymilvus` (version 2.4.3 or above) to incorporate it into your AI application as a Python library.\n\nMilvus Lite shares the Milvus API, ensuring that your client-side code works for both small-scale local deployments and Milvus servers deployed on Docker or Kubernetes with billions of vectors.\n\n\u003ciframe style=\"border-radius:12px\" src=\"https://open.spotify.com/embed/episode/5bMcZgPgPVxSuoi1M2vn1p?utm_source=generator\" width=\"100%\" height=\"152\" frameBorder=\"0\" allowfullscreen=\"\" allow=\"autoplay; clipboard-write; encrypted-media; fullscreen; picture-in-picture\" loading=\"lazy\"\u003e\u003c/iframe\u003e\n\n## Why We Built Milvus Lite\n\nMany AI applications require vector similarity search for unstructured data, including text, images, voices, and videos, for applications such as chatbots and shopping assistants. Vector databases are crafted for storing and searching vector embeddings and are a crucial part of the AI development stack, particularly for generative AI use cases like [Retrieval Augmented Generation (RAG)](https://zilliz.com/learn/Retrieval-Augmented-Generation).\n\nDespite the availability of numerous vector search solutions, an easy-to-start option that also works for large-scale production deployments was missing. As the creators of Milvus, we designed Milvus Lite to help AI developers build applications faster while ensuring a consistent experience across various deployment options, including Milvus on Kubernetes, Docker, and managed cloud services.\n\nMilvus Lite is a crucial addition to our suite of offerings within the Milvus ecosystem. It provides developers with a versatile tool that supports every stage of their development journey. From prototyping to production environments and from edge computing to large-scale deployments, Milvus is now the only vector database that covers use cases of any size and all stages of development.\n\n## How Milvus Lite Works \n\nMilvus Lite supports all the basic operations available in Milvus, such as creating collections and inserting, searching, and deleting vectors. It will soon support advanced features like hybrid search. Milvus Lite loads data into memory for efficient searches and persists it as an SQLite file.\n\nMilvus Lite is included in the [Python SDK of Milvus](https://github.com/milvus-io/pymilvus) and can be deployed with a simple `pip install pymilvus`. The following code snippet demonstrates how to set up a vector database with Milvus Lite by specifying a local file name and then creating a new collection. For those familiar with the Milvus API, the only difference is that the `uri` refers to a local file name instead of a network endpoint, e.g., `\"milvus_demo.db\"` instead of `\"http://localhost:19530\"` for a Milvus server. Everything else remains the same. Milvus Lite also supports storing raw text and other labels as metadata, using a dynamic or explicitly defined schema, as shown below.\n\n```\nfrom pymilvus import MilvusClient\n\nclient = MilvusClient(\"milvus_demo.db\")\n# This collection can take input with mandatory fields named \"id\", \"vector\" and\n# any other fields as \"dynamic schema\". You can also define the schema explicitly.\nclient.create_collection(\n collection_name=\"demo_collection\",\n dimension=384 # Dimension for vectors.\n)\n```\nFor scalability, an AI application developed with Milvus Lite can easily transition to using Milvus deployed on Docker or Kubernetes by simply specifying the `uri` with the server endpoint.\n\n\n## Integration with AI Development Stack\n\nIn addition to introducing Milvus Lite to make vector search easy to start with, Milvus also integrates with many frameworks and providers of the AI development stack, including [LangChain](https://python.langchain.com/v0.2/docs/integrations/vectorstores/milvus/), [LlamaIndex](https://docs.llamaindex.ai/en/stable/examples/vector_stores/MilvusIndexDemo/), [Haystack](https://haystack.deepset.ai/integrations/milvus-document-store), [Voyage AI](https://blog.voyageai.com/2024/05/30/semantic-search-with-milvus-lite-and-voyage-ai/), [Ragas](https://milvus.io/docs/integrate_with_ragas.md), [Jina AI](https://jina.ai/news/implementing-a-chat-history-rag-with-jina-ai-and-milvus-lite/), [DSPy](https://dspy-docs.vercel.app/docs/deep-dive/retrieval_models_clients/MilvusRM), [BentoML](https://www.bentoml.com/blog/building-a-rag-app-with-bentocloud-and-milvus-lite), [WhyHow](https://chiajy.medium.com/70873c7576f1), [Relari AI](https://blog.relari.ai/case-study-using-synthetic-data-to-benchmark-rag-systems-be324904ace1), [Airbyte](https://docs.airbyte.com/integrations/destinations/milvus), [HuggingFace](https://milvus.io/docs/integrate_with_hugging-face.md) and [MemGPT](https://memgpt.readme.io/docs/storage#milvus). Thanks to their extensive tooling and services, these integrations simplify the development of AI applications with vector search capability.\n\nAnd this is just the beginning—many more exciting integrations are coming soon! Stay tuned! \n\n\n## More Resources and Examples\n\nExplore [Milvus quickstart documentation](https://milvus.io/docs/quickstart.md) for detailed guides and code examples on using Milvus Lite to build AI applications like Retrieval-Augmented Generation ([RAG](https://github.com/milvus-io/bootcamp/blob/master/bootcamp/tutorials/quickstart/build_RAG_with_milvus.ipynb)) and [image search](https://github.com/milvus-io/bootcamp/blob/master/bootcamp/tutorials/quickstart/image_search_with_milvus.ipynb).\n\nMilvus Lite is an open-source project, and we welcome your contributions. Check out our [Contributing Guide](https://github.com/milvus-io/milvus-lite/blob/main/CONTRIBUTING.md) to get started. You can also report bugs or request features by filing an issue on the [Milvus Lite GitHub](https://github.com/milvus-io/milvus-lite) repository.\n","title":"Introducing Milvus Lite: Start Building a GenAI Application in Seconds","metaData":{}},{"id":"optimize-vector-databases-enhance-rag-driven-generative-ai.md","author":"Cathy Zhang, Dr. Malini Bhandaru","desc":"In this article, you’ll learn more about vector databases and their benchmarking frameworks, datasets to tackle different aspects, and the tools used for performance analysis — everything you need to start optimizing vector databases.","tags":["Engineering"],"recommend":true,"canonicalUrl":"https://medium.com/intel-tech/optimize-vector-databases-enhance-rag-driven-generative-ai-90c10416cb9c","date":"2024-05-13T00:00:00.000Z","cover":"https://assets.zilliz.com/Optimize_Vector_Databases_Enhance_RAG_Driven_Generative_AI_6e3b370f25.png","href":"/blog/optimize-vector-databases-enhance-rag-driven-generative-ai.md","content":"\n*This post was originally published on [Intel's Medium Channel](https://medium.com/intel-tech/optimize-vector-databases-enhance-rag-driven-generative-ai-90c10416cb9c) and is reposted here with permission.*\n\n\n\u003cbr\u003e\n\nTwo methods to optimize your vector database when using RAG\n\n\n\n\n\nPhoto by [Ilya Pavlov](https://unsplash.com/@ilyapavlov?utm_content=creditCopyText\\\u0026utm_medium=referral\\\u0026utm_source=unsplash) on [Unsplash](https://unsplash.com/photos/monitor-showing-java-programming-OqtafYT5kTw?utm_content=creditCopyText\\\u0026utm_medium=referral\\\u0026utm_source=unsplash)\n\n\n\nBy Cathy Zhang and Dr. Malini Bhandaru\nContributors: Lin Yang and Changyan Liu\n\n\nGenerative AI (GenAI) models, which are seeing exponential adoption in our daily lives, are being improved by [retrieval-augmented generation (RAG)](https://www.techtarget.com/searchenterpriseai/definition/retrieval-augmented-generation), a technique used to enhance response accuracy and reliability by fetching facts from external sources. RAG helps a regular [large language model (LLM)](https://www.techtarget.com/whatis/definition/large-language-model-LLM) understand context and reduce [hallucinations](https://en.wikipedia.org/wiki/Hallucination_\\(artificial_intelligence\\)) by leveraging a giant database of unstructured data stored as vectors — a mathematical presentation that helps capture context and relationships between data.\n\n\nRAG helps to retrieve more contextual information and thus generate better responses, but the vector databases they rely on are getting ever larger to provide rich content to draw upon. Just as trillion-parameter LLMs are on the horizon, vector databases of billions of vectors are not far behind. As optimization engineers, we were curious to see if we could make vector databases more performant, load data faster, and create indices faster to ensure retrieval speed even as new data is added. Doing so would not only result in reduced user wait time, but also make RAG-based AI solutions a little more sustainable.\n\n\nIn this article, you’ll learn more about vector databases and their benchmarking frameworks, datasets to tackle different aspects, and the tools used for performance analysis — everything you need to start optimizing vector databases. We will also share our optimization achievements on two popular vector database solutions to inspire you on your optimization journey of performance and sustainability impact.\n\n\n\n\n## Understanding Vector Databases\n\n\nUnlike traditional relational or non-relational databases where data is stored in a structured manner, a vector database contains a mathematical representation of individual data items, called a vector, constructed using an embedding or transformation function. The vector commonly represents features or semantic meanings and can be short or long. Vector databases do vector retrieval by similarity search using a distance metric (where closer means the results are more similar) such as [Euclidean, dot product, or cosine similarity](https://www.pinecone.io/learn/vector-similarity/).\n\n\nTo accelerate the retrieval process, the vector data is organized using an indexing mechanism. Examples of these organization methods include flat structures, [inverted file (IVF),](https://arxiv.org/abs/2002.09094) [Hierarchical Navigable Small Worlds (HNSW),](https://arxiv.org/abs/1603.09320) and [locality-sensitive hashing (LSH)](https://en.wikipedia.org/wiki/Locality-sensitive_hashing), among others. Each of these methods contributes to the efficiency and effectiveness of retrieving similar vectors when needed.\n\n\nLet’s examine how you would use a vector database in a GenAI system. Figure 1 illustrates both the loading of data into a vector database and using it in the context of a GenAI application. When you input your prompt, it undergoes a transformation process identical to the one used to generate vectors in the database. This transformed vector prompt is then used to retrieve similar vectors from the vector database. These retrieved items essentially serve as conversational memory, furnishing contextual history for prompts, akin to how LLMs operate. This feature proves particularly advantageous in natural language processing, computer vision, recommendation systems, and other domains requiring semantic comprehension and data matching. Your initial prompt is subsequently “merged” with the retrieved elements, supplying context, and assisting the LLM in formulating responses based on the provided context rather than solely relying on its original training data.\n\n\n\n\n\nFigure 1. A RAG application architecture.\n\n\nVectors are stored and indexed for speedy retrieval. Vector databases come in two main flavors, traditional databases that have been extended to store vectors, and purpose-built vector databases. Some examples of traditional databases that provide vector support are [Redis](https://redis.io/), [pgvector](https://github.com/pgvector/pgvector), [Elasticsearch](https://www.elastic.co/elasticsearch), and [OpenSearch](https://opensearch.org/). Examples of purpose-built vector databases include proprietary solutions [Zilliz](https://zilliz.com/) and [Pinecone](https://www.pinecone.io/), and open source projects [Milvus](https://milvus.io/), [Weaviate](https://weaviate.io/), [Qdrant](https://qdrant.tech/), [Faiss](https://github.com/facebookresearch/faiss), and [Chroma](https://www.trychroma.com/). You can learn more about vector databases on GitHub via [LangChain ](https://github.com/langchain-ai/langchain/tree/master/libs/langchain/langchain/vectorstores)and [OpenAI Cookbook](https://github.com/openai/openai-cookbook/tree/main/examples/vector_databases).\n\n\nWe’ll take a closer look at one from each category, Milvus and Redis.\n\n\n\n\n## Improving Performance\n\n\nBefore diving into the optimizations, let’s review how vector databases are evaluated, some evaluation frameworks, and available performance analysis tools.\n\n\n\n\n### Performance Metrics\n\n\nLet’s look at key metrics that can help you measure vector database performance.\n\n\n- **Load latency** measures the time required to load data into the vector database’s memory and build an index. An index is a data structure used to efficiently organize and retrieve vector data based on its similarity or distance. Types of [in-memory indices](https://milvus.io/docs/index.md#In-memory-Index) include [flat index](https://thedataquarry.com/posts/vector-db-3/#flat-indexes), [IVF\\_FLAT](https://supabase.com/docs/guides/ai/vector-indexes/ivf-indexes), [IVF\\_PQ, HNSW](https://towardsdatascience.com/ivfpq-hnsw-for-billion-scale-similarity-search-89ff2f89d90e), [scalable nearest neighbors (ScaNN),](https://github.com/google-research/google-research/tree/master/scann)and [DiskANN](https://milvus.io/docs/disk_index.md).\n- **Recall** is the proportion of true matches, or relevant items, found in the [Top K](https://redis.io/docs/data-types/probabilistic/top-k/) results retrieved by the search algorithm. Higher recall values indicate better retrieval of relevant items.\n- **Queries per second (QPS)** is the rate at which the vector database can process incoming queries. Higher QPS values imply better query processing capability and system throughput.\n\n\n\n\n### Benchmarking Frameworks\n\n\n\n\n\nFigure 2. The vector database benchmarking framework.\n\n\nBenchmarking a vector database requires a vector database server and clients. In our performance tests, we used two popular open source tools.\n\n\n- [**VectorDBBench**](https://github.com/zilliztech/VectorDBBench/tree/main)**:** Developed and open sourced by Zilliz, VectorDBBench helps test different vector databases with different index types and provides a convenient web interface.\n- [**vector-db-benchmark**](https://github.com/qdrant/vector-db-benchmark/tree/master)**:** Developed and open sourced by Qdrant, vector-db-benchmark helps test several typical vector databases for the [HNSW](https://www.datastax.com/guides/hierarchical-navigable-small-worlds) index type. It runs tests through the command line and provides a [Docker Compose](https://docs.docker.com/compose/) __file to simplify starting server components.\n\n\n\n\n\nFigure 3. An example vector-db-benchmark command used to run the benchmark test.\n\n\nBut the benchmark framework is only part of the equation. We need data that exercises different aspects of the vector database solution itself, such as its ability to handle large volumes of data, various vector sizes, and speed of retrieval.With that, let’s look at some available public datasets.\n\n\n\n\n### Open Datasets to Exercise Vector Databases\n\n\nLarge datasets are good candidates to test load latency and resource allocation. Some datasets have high dimensional data and are good for testing speed of computing similarity.\n\n\nDatasets range from a dimension of 25 to a dimension of 2048. The [LAION](https://laion.ai/) dataset, an open image collection, has been used for training very large visual and language deep-neural models like stable diffusion generative models. OpenAI’s dataset of 5M vectors, each with a dimension of 1536, was created by VectorDBBench by running OpenAI on [raw data](https://huggingface.co/datasets/allenai/c4). Given each vector element is of type FLOAT, to save the vectors alone, approximately 29 GB (5M \\* 1536 \\* 4) of memory is needed, plus a similar amount extra to hold indices and other metadata for a total of 58 GB of memory for testing. When using the vector-db-benchmark tool, ensure adequate disk storage to save results.\n\n\nTo test for load latency, we needed a large collection of vectors, which [deep-image-96-angular](https://docs.hippo.transwarp.io/docs/performance-dataset) offers. To test performance of index generation and similarity computation, high dimensional vectors provide more stress. To this end we chose the 500K dataset of 1536 dimension vectors.\n\n\n\n\n### Performance Tools\n\n\nWe’ve covered ways to stress the system to identify metrics of interest, but let’s examine what’s happening at a lower level: How busy is the computing unit, memory consumption, waits on locks, and more? These provide clues to databasebehavior, particularly useful in identifying problem areas.\n\n\nThe Linux [top](https://www.redhat.com/sysadmin/interpret-top-output) utility provides system-performance information. However, the [perf](https://perf.wiki.kernel.org/index.php/Main_Page) tool in Linux provides a deeper set of insights. To learn more, we also recommend reading [Linux perf examples](https://www.brendangregg.com/perf.html) and the [Intel top-down microarchitecture analysis method](https://www.intel.com/content/www/us/en/docs/vtune-profiler/cookbook/2023-0/top-down-microarchitecture-analysis-method.html). Yet another tool is the [Intel® vTune™ Profiler](https://www.intel.com/content/www/us/en/developer/tools/oneapi/vtune-profiler.html), which is useful when optimizing not just application but also system performance and configuration for a variety of workloads spanning HPC, cloud, IoT, media, storage, and more.\n\n\n\n\n## Milvus Vector Database Optimizations\n\n\nLet’s walk through some examples of how we attempted to improve the performance of the Milvus vector database.\n\n\n\n\n### Reducing Memory Movement Overhead in Datanode Buffer Write\n\n\nMilvus’s write path proxies write data into a log broker via _MsgStream_. The data nodes then consume the data, converting and storing it into segments. Segments will merge the newly inserted data. The merge logic allocates a new buffer to hold/move both the old data and the new data to be inserted and then returns the new buffer as old data for the next data merge. This results in the old data getting successively larger, which in turn makes data movement slower. Perf profiles showed a high overhead for this logic.\n\n\n\n\n\nFigure 4. Merging and moving data in the vector database generates a high-performance overhead.\n\n\nWe changed the _merge buffer_ logic to directly append the new data to be inserted into the old data, avoiding allocating a new buffer and moving the large old data. Perf profiles confirm that there is no overhead to this logic. The microcode metrics _metric\\_CPU operating frequency_ and _metric\\_CPU utilization_ indicate an improvement that is consistent with the system not having to wait for the long memory movement anymore. Load latency improved by more than 60 percent. The improvement is captured on [GitHub](https://github.com/milvus-io/milvus/pull/26839).\n\n\n\n\n\nFigure 5. With less copying we see a performance improvement of more than 50 percent in load latency.\n\n\n\n\n### Inverted Index Building with Reduced Memory Allocation Overhead\n\n\nThe Milvus search engine, [Knowhere](https://milvus.io/docs/knowhere.md), employs the [Elkan k-means algorithm](https://www.vlfeat.org/api/kmeans-fundamentals.html#kmeans-elkan) to train cluster data for creating [inverted file (IVF) indices](https://milvus.io/docs/v1.1.1/index.md). Each round of data training defines an iteration count. The larger the count, the better the training results. However, it also implies that the Elkan algorithm will be called more frequently.\n\n\nThe Elkan algorithm handles memory allocation and deallocation each time it’s executed. Specifically, it allocates memory to store half the size of symmetric matrix data, excluding the diagonal elements. In Knowhere, the symmetric matrix dimension used by the Elkan algorithm is set to 1024, resulting in a memory size of approximately 2 MB. This means for each training round Elkan repeatedly allocates and deallocates 2 MB memory.\n\n\nPerf profiling data indicated frequent large memory allocation activity. In fact, it triggered [virtual memory area (VMA)](https://www.oreilly.com/library/view/linux-device-drivers/9781785280009/4759692f-43fb-4066-86b2-76a90f0707a2.xhtml)allocation, physical page allocation, page map setup, and updating of memory cgroup statistics in the kernel. This pattern of large memory allocation/deallocation activity can, in some situations, also aggravate memory fragmentation. This is a significant tax.\n\n\nThe _IndexFlatElkan_ structure is specifically designed and constructed to support the Elkan algorithm. Each data training process will have an _IndexFlatElkan_ instance initialized. To mitigate the performance impact resulting from frequent memory allocation and deallocation in the Elkan algorithm, we refactored the code logic, moving the memory management outside of the Elkan algorithm function up into the construction process of _IndexFlatElkan_. This enables memory allocation to occur only once during the initialization phase while serving all subsequent Elkan algorithm function calls from the current data training process and helps to improve load latency by around 3 percent. Find the [Knowhere patch here](https://github.com/zilliztech/knowhere/pull/280).\n\n\n\n\n## Redis Vector Search Acceleration through Software Prefetch\n\n\nRedis, a popular traditional in-memory key-value data store, recently began supporting vector search. To go beyond a typical key-value store, it offers extensibility modules; the [RediSearch](https://github.com/RediSearch/RediSearch) module facilitates the storage and search of vectors directly within Redis.\n\n\nFor vector similarity search, Redis supports two algorithms, namely brute force and HNSW. The HNSW algorithm is specifically crafted for efficiently locating approximate nearest neighbors in high-dimensional spaces. It uses a priority queue named _candidate\\_set_ to manage all vector candidates for distance computing.\n\n\nEach vector candidate encompasses substantial metadata in addition to the vector data. As a result, when loading a candidate from memory it can cause data cache misses, which incur processing delays. Our optimization introduces software prefetching to proactively load the next candidate while processing the current one. This enhancement has resulted in a 2 to 3 percent throughput improvement for vector similarity searches in a single instance Redis setup. The patch is in the process of being upstreamed.\n\n\n\n\n## GCC Default Behavior Change to Prevent Mixed Assembly Code Penalties\n\n\nTo drive maximum performance, frequently used sections of code are often handwritten in assembly. However, when different segments of code are written either by different people or at different points in time, the instructions used may come from incompatible assembly instruction sets such as [Intel® Advanced Vector Extensions 512 (Intel® AVX-512)](https://www.intel.com/content/www/us/en/architecture-and-technology/avx-512-overview.html) and [Streaming SIMD Extensions (SSE)](https://en.wikipedia.org/wiki/Streaming_SIMD_Extensions). If not compiled appropriately, the mixed code results in a performance penalty. [Learn more about mixing Intel AVX and SSE instructions here](https://www.intel.com/content/dam/develop/external/us/en/documents/11mc12-avoiding-2bavx-sse-2btransition-2bpenalties-2brh-2bfinal-809104.pdf).\n\n\nYou can easily determine if you’re using mixed-mode assembly code and have not compiled the code with _VZEROUPPER_, incurring the performance penalty. It can be observed through a perf command like _sudo perf stat -e ‘assists.sse\\_avx\\_mix/event/event=0xc1,umask=0x10/’ \\\u003cworkload\u003e_. If your OS doesn’t have support for the event, use _cpu/event=0xc1,umask=0x10,name=assists\\_sse\\_avx\\_mix/_.\n\n\nThe Clang compiler by default inserts _VZEROUPPER_, avoiding any mixed mode penalty. But the GCC compiler only inserted _VZEROUPPER_ when the -O2 or -O3 compiler flags were specified. We contacted the GCC team and explained the issue and they now, by default, correctly handle mixed mode assembly code.\n\n\n\n## Start Optimizing Your Vector Databases\n\n\nVector databases are playing an integral role in GenAI, and they are growing ever larger to generate higher-quality responses. With respect to optimization, AI applications are no different from other software applications in that they reveal their secrets when one employs standard performance analysis tools along with benchmark frameworks and stress input.\n\nUsing these tools, we uncovered performance traps pertaining to unnecessary memory allocation, failing to prefetch instructions, and using incorrect compiler options. Based on our findings, we upstreamed enhancements to Milvus, Knowhere, Redis, and the GCC compiler to help make AI a little more performant and sustainable. Vector databases are an important class of applications worthy of your optimization efforts. We hope this article helps you get started.\n","title":"Optimize Vector Databases, Enhance RAG-Driven Generative AI","metaData":{}},{"id":"getting-started-with-milvus-cluster-and-k8s.md","author":"Stephen Batifol","desc":"Through this tutorial, you'll learn the basics of setting up Milvus with Helm, creating a collection, and performing data ingestion and similarity searches.","tags":["Engineering"],"recommend":true,"canonicalUrl":"https://milvus.io/blog/getting-started-with-milvus-and-k8s.md","date":"2024-04-03T00:00:00.000Z","cover":"https://assets.zilliz.com/Getting_started_with_Milvus_cluster_and_K8s_1_34b2c81802.png","href":"/blog/getting-started-with-milvus-cluster-and-k8s.md","content":"\n\n## Introduction\n\nMilvus is a distributed vector database that aims to store, index and manage massive embedding vectors. Its ability to efficiently index and search through trillions of vectors makes Milvus a go-to choice for AI and machine learning workloads.\n\nKubernetes (K8s), on the other hand, excels in managing and scaling containerized applications. It provides features like auto-scaling, self-healing, and load balancing, which are crucial for maintaining high availability and performance in production environments.\n\n\n## Why Use Them Together? \n\nK8s can automatically scale the Milvus clusters based on the workload. As your data grows or the number of queries increases, K8s can spin up more Milvus instances to handle the load, ensuring your applications remain responsive.\n\nOne of the standout features of K8s is its horizontal scaling, which makes expanding your Milvus cluster a breeze. As your dataset grows, K8s effortlessly accommodates this growth, making it a straightforward and efficient solution.\n\nIn addition, the ability to handle queries also scales horizontally with K8s. As the query load increases, K8s can deploy more Milvus instances to handle the increased similarity search queries, ensuring low latency responses even under heavy loads.\n\n\n## Prerequisites \u0026 Setting Up K8s\n\n### Prerequisites\n\n- **Docker** - Ensure Docker is installed on your system.\n\n- **Kubernetes** - Have a Kubernetes cluster ready. You can use `minikube` for local development or a cloud provider's Kubernetes service for production environments.\n\n- **Helm** - Install Helm, a package manager for Kubernetes, to help you manage Kubernetes applications, you can check our documentation to see how to do that \u003chttps://milvus.io/docs/install_cluster-helm.md\u003e\n\n- **Kubectl** - Install `kubectl`, a command-line tool for interacting with Kubernetes clusters, to deploy applications, inspect and manage cluster resources, and view logs.\n\n### Setting Up K8s\n\nAfter installing everything needed to run a K8s cluster, and if you used `minikube`, start your cluster with: \n\n```\nminikube start\n```\n\nCheck the status of your K8s cluster with:\n\n```\nkubectl cluster-info\n```\n\n### Deploying Milvus on K8s\n\nFor this deployment, we're opting for Milvus in cluster-mode to leverage its full distributed capabilities. We'll be using Helm, to streamline the installation process.\n\n**1. Helm Installation Command** \n\n```\nhelm install my-milvus milvus/milvus --set pulsar.enabled=false --set kafka.enabled=true\n```\n\nThis command installs Milvus on your K8s cluster with Kafka enabled and Pulsar disabled. Kafka serves as the messaging system within Milvus, handling data streaming between different components. Disabling Pulsar and enabling Kafka tailors the deployment to our specific messaging preferences and requirements.\n\n**2. Port Forwarding**\n\nTo access Milvus from your local machine, create a port forward: `kubectl port-forward svc/my-milvus 27017:19530`.\n\nThis command maps port `19530` from the Milvus service `svc/my-milvus` to the same port on your local machine, allowing you to connect to Milvus using local tools. If you leave the local port unspecified (as in `:19530`), K8s will allocate an available port, making it dynamic. Ensure you note the allocated local port if you choose this method.\n\n**3. Verifying the Deployment:**\n\n```\nkubectl get pods \n\nNAME READY STATUS RESTARTS AGE\nmy-milvus-datacoord-595b996bd4-zprpd 1/1 Running 0 85m\nmy-milvus-datanode-d9d555785-47nkt 1/1 Running 0 85m\nmy-milvus-etcd-0 1/1 Running 0 84m\nmy-milvus-etcd-1 1/1 Running 0 85m\nmy-milvus-etcd-2 1/1 Running 0 85m\nmy-milvus-indexcoord-65bc68968c-6jg6q 1/1 Running 0 85m\nmy-milvus-indexnode-54586f55d-z9vx4 1/1 Running 0 85m\nmy-milvus-kafka-0 1/1 Running 0 85m\nmy-milvus-kafka-1 1/1 Running 0 85m\nmy-milvus-kafka-2 1/1 Running 0 85m\nmy-milvus-minio-0 1/1 Running 0 96m\nmy-milvus-minio-1 1/1 Running 0 96m\nmy-milvus-minio-2 1/1 Running 0 96m\nmy-milvus-minio-3 1/1 Running 0 96m\nmy-milvus-proxy-76bb7d497f-sqwvd 1/1 Running 0 85m\nmy-milvus-querycoord-6f4c7b7598-b6twj 1/1 Running 0 85m\nmy-milvus-querynode-677bdf485b-ktc6m 1/1 Running 0 85m\nmy-milvus-rootcoord-7498fddfd8-v5zw8 1/1 Running 0 85m\nmy-milvus-zookeeper-0 1/1 Running 0 85m\nmy-milvus-zookeeper-1 1/1 Running 0 85m\nmy-milvus-zookeeper-2 1/1 Running 0 85m\n```\n\n\nYou should see a list of pods similar to the output above, all in the Running state. This indicates that your Milvus cluster is operational. Specifically, look for the 1/1 under the `READY` column, which signifies that each pod is fully ready and running. If any pods are not in the Running state, you may need to investigate further to ensure a successful deployment.\n\nWith your Milvus cluster deployed and all components confirmed running, you're now ready to proceed to data ingestion and indexing. This will involve connecting to your Milvus instance, creating collections, and inserting vectors for search and retrieval.\n\n\n## Data Ingestion and Indexing\n\nTo start ingesting and indexing data in our Milvus cluster, we'll use the pymilvus SDK. There are two installation options:\n\n- Basic SDK: `pip install pymilvus`\n\n- For rich text embeddings and advanced models: `pip install pymilvus[model]`\n\nTime to insert data in our cluster, we’ll be using `pymilvus`, you can either install the SDK only with `pip install pymilvus` or if you want to extract rich text embeddings, you can also use `PyMilvus Models` by installing `pip install pymilvus[model]`. \n\n### Connecting and Creating a Collection:\n\nFirst, connect to your Milvus instance using the port you forwarded earlier. Ensure the URI matches the local port assigned by K8s:\n\n```\nfrom pymilvus import MilvusClient\n\nclient = MilvusClient(\n uri=\"http://127.0.0.1:52070\",\n )\n\nclient.create_collection(collection_name=\"quick_setup\", dimension=5)\n```\n\nThe `dimension=5` parameter defines the vector size for this collection, essential for the vector search capabilities.\n\n\n### Insert Data\n\nHere's how to insert an initial set of data, where each vector represents an item, and the color field adds a descriptive attribute:\n\n```\ndata=[\n {\"id\": 0, \"vector\": [0.3580376395471989, -0.6023495712049978, 0.18414012509913835, -0.26286205330961354, 0.9029438446296592], \"color\": \"pink_8682\"},\n {\"id\": 1, \"vector\": [0.19886812562848388, 0.06023560599112088, 0.6976963061752597, 0.2614474506242501, 0.838729485096104], \"color\": \"red_7025\"},\n {\"id\": 2, \"vector\": [0.43742130801983836, -0.5597502546264526, 0.6457887650909682, 0.7894058910881185, 0.20785793220625592], \"color\": \"orange_6781\"},\n {\"id\": 3, \"vector\": [0.3172005263489739, 0.9719044792798428, -0.36981146090600725, -0.4860894583077995, 0.95791889146345], \"color\": \"pink_9298\"},\n {\"id\": 4, \"vector\": [0.4452349528804562, -0.8757026943054742, 0.8220779437047674, 0.46406290649483184, 0.30337481143159106], \"color\": \"red_4794\"},\n {\"id\": 5, \"vector\": [0.985825131989184, -0.8144651566660419, 0.6299267002202009, 0.1206906911183383, -0.1446277761879955], \"color\": \"yellow_4222\"},\n {\"id\": 6, \"vector\": [0.8371977790571115, -0.015764369584852833, -0.31062937026679327, -0.562666951622192, -0.8984947637863987], \"color\": \"red_9392\"},\n {\"id\": 7, \"vector\": [-0.33445148015177995, -0.2567135004164067, 0.8987539745369246, 0.9402995886420709, 0.5378064918413052], \"color\": \"grey_8510\"},\n {\"id\": 8, \"vector\": [0.39524717779832685, 0.4000257286739164, -0.5890507376891594, -0.8650502298996872, -0.6140360785406336], \"color\": \"white_9381\"},\n {\"id\": 9, \"vector\": [0.5718280481994695, 0.24070317428066512, -0.3737913482606834, -0.06726932177492717, -0.6980531615588608], \"color\": \"purple_4976\"}\n]\n\nres = client.insert(\n collection_name=\"quick_setup\",\n data=data\n)\n\nprint(res)\n```\n\n\nThe provided code assumes that you have created a collection in the Quick Setup manner. As shown in the above code,\n\nThe data to insert is organized into a list of dictionaries, where each dictionary represents a data record, termed as an entity.\n\nEach dictionary contains a non-schema-defined field named color.\n\nEach dictionary contains the keys corresponding to both pre-defined and dynamic fields.\n\n\n### Insert Even More Data\n\n```\ncolors = [\"green\", \"blue\", \"yellow\", \"red\", \"black\", \"white\", \"purple\", \"pink\", \"orange\", \"brown\", \"grey\"]\ndata = [ {\n \"id\": i, \n \"vector\": [ random.uniform(-1, 1) for _ in range(5) ], \n \"color\": f\"{random.choice(colors)}_{str(random.randint(1000, 9999))}\" \n} for i in range(1000) ]\n\nres = client.insert(\n collection_name=\"quick_setup\",\n data=data[10:]\n)\n\nprint(res)\n```\n\n\n## Similarity Search\n\nAfter populating the collection, you can perform a similarity search to find vectors close to a query vector. The value of the query_vectors variable is a list containing a sub-list of floats. The sub-list represents a vector embedding of 5 dimensions.\n\n```\nquery_vectors = [\n [0.041732933, 0.013779674, -0.027564144, -0.013061441, 0.009748648]\n]\n\nres = client.search(\n collection_name=\"quick_setup\", # target collection\n data=query_vectors, # query vectors\n limit=3, # number of returned entities\n)\n\nprint(res)\n```\n\n\nThis query searches for the top 3 vectors most similar to our query vector, demonstrating Milvus's powerful search capabilities.\n\n\n## Uninstall Milvus from K8s\n\nOnce you are done with this tutorial, feel free to uninstall Milvus from your K8s cluster with:`helm uninstall my-milvus`.\n\nThis command will remove all Milvus components deployed in the `my-milvus` release, freeing up cluster resources.\n\n## Conclusion\n\n- Deploying Milvus on a Kubernetes cluster showcases the scalability and flexibility of vector databases in handling AI and machine learning workloads. Through this tutorial, you've learned the basics of setting up Milvus with Helm, creating a collection, and performing data ingestion and similarity searches.\n\n- Installing Milvus on a Kubernetes cluster with Helm should be straightforward. To go deeper into scaling Milvus clusters for larger datasets or more intensive workloads, our documentation offers detailed guidance \u003chttps://milvus.io/docs/scaleout.md\u003e\n\nFeel free to check out the code on [Github](https://github.com/stephen37/K8s-tutorial-milvus), check out [Milvus](https://github.com/milvus-io/milvus), experiment with different configurations and use cases, and share your experiences with the community by joining our [Discord](https://discord.gg/FG6hMJStWu).\n","title":"Getting started with Milvus cluster and K8s","metaData":{}},{"id":"milvus-2-4-nvidia-cagra-gpu-index-multivector-search-sparse-vector-support.md","author":"Fendy Feng","desc":"We are happy to announce the launch of Milvus 2.4, a major advancement in enhancing search capabilities for large-scale datasets.","metaTitle":"Milvus 2.4 Supports Multi-vector Search, Sparse Vector, CAGRA, and More!","tags":["Engineering"],"recommend":true,"canonicalUrl":"https://milvus.io/blog/milvus-2-4-nvidia-cagra-gpu-index-multivector-search-sparse-vector-support.md","date":"2024-03-20T00:00:00.000Z","cover":"https://assets.zilliz.com/What_is_new_in_Milvus_2_4_1_c580220be3.png","href":"/blog/milvus-2-4-nvidia-cagra-gpu-index-multivector-search-sparse-vector-support.md","content":"\nWe are happy to announce the launch of Milvus 2.4, a major advancement in enhancing search capabilities for large-scale datasets. This latest release adds new features, such as support for the GPU-based CAGRA index, beta support for [sparse embeddings](https://zilliz.com/learn/sparse-and-dense-embeddings), group search, and various other improvements in search capabilities. These developments reinforce our commitment to the community by offering developers like you a powerful and efficient tool for handling and querying vector data. Let's jump into the key benefits of Milvus 2.4 together.\n\n\n## Enabled Multi-vector Search for Simplified Multimodal Searches\n\nMilvus 2.4 provides multivector search capability, allowing simultaneous search and reranking of different vector types within the same Milvus system. This feature streamlines multimodal searches, significantly enhancing recall rates and enabling developers to effortlessly manage intricate AI applications with varied data types. Additionally, this functionality simplifies the integration and fine-tuning of custom reranking models, aiding in the creation of advanced search functions like precise [recommender systems](https://zilliz.com/vector-database-use-cases/recommender-system) that utilize insights from multidimensional data.\n\n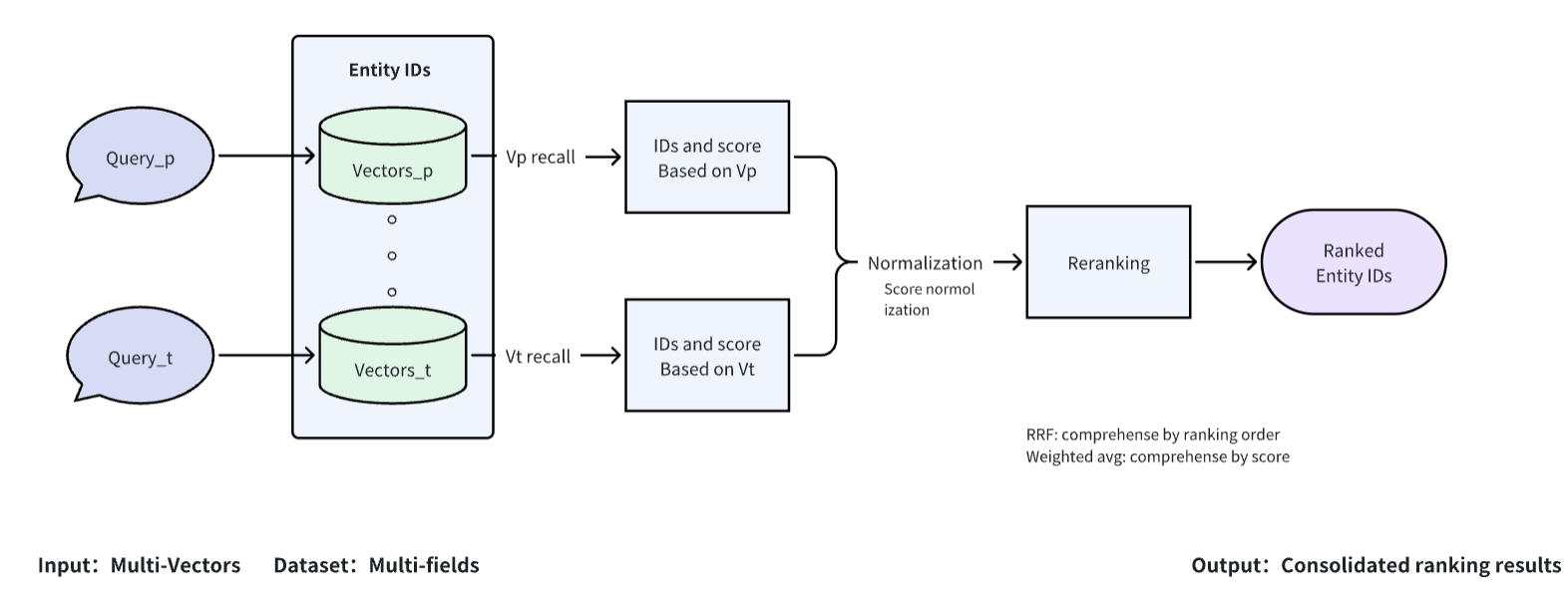\n\nMultivector support in Milvus has two components: \n\n1. The ability to store/query multiple vectors for a single entity within a collection, which is a more natural way to organize data \n\n2. The ability to build/optimize a reranking algorithm by leveraging the prebuilt reranking algorithms in Milvus\n\nBesides being a highly [requested feature](https://github.com/milvus-io/milvus/issues/25639), we built this capability because the industry is moving towards multimodal models with the release of GPT-4 and Claude 3. Reranking is a commonly used technique to further improve query performance in search. We aimed to make it easy for developers to build and optimize their rerankers within the Milvus ecosystem.\n\n\n## Grouping Search Support for Enhanced Compute Efficiency\n\nGrouping Search is another often [requested feature](https://github.com/milvus-io/milvus/issues/25343) we added to Milvus 2.4. It integrates a group-by operation designed for fields of types BOOL, INT, or VARCHAR, filling a crucial efficiency gap in executing large-scale grouping queries. \n\nTraditionally, developers relied on extensive Top-K searches followed by manual post-processing to distill group-specific results, a compute-intensive and code-heavy method. Grouping Search refines this process by efficiently linking query outcomes to aggregate group identifiers like document or video names, streamlining the handling of segmented entities within larger datasets.\n\nMilvus distinguishes its Grouping Search with an iterator-based implementation, offering a marked improvement in computational efficiency over similar technologies. This choice ensures superior performance scalability, particularly in production environments where compute resource optimization is paramount. By reducing data traversal and computation overhead, Milvus supports more efficient query processing, significantly reducing response times and operational costs compared to other vector databases. \n\nGrouping Search bolsters Milvus's capability to manage high-volume, complex queries and aligns with high-performance computing practices for robust data management solutions.\n\n## Beta Support for Sparse Vector Embeddings\n\n[Sparse embeddings](https://zilliz.com/learn/sparse-and-dense-embeddings) represent a paradigm shift from traditional dense vector approaches, catering to the nuances of semantic similarity rather than mere keyword frequency. This distinction allows for a more nuanced search capability, aligning closely with the semantic content of the query and the documents. Sparse vector models, particularly useful in information retrieval and natural language processing, offer powerful out-of-domain search capabilities and interpretability compared to their dense counterparts.\n\nIn Milvus 2.4, we have expanded the Hybrid Search to include sparse embeddings generated by advanced neural models like SPLADEv2 or statistical models such as BM25. In Milvus, sparse vectors are treated on par with dense vectors, enabling the creation of collections with sparse vector fields, data insertion, index building, and performing similarity searches. Notably, sparse embeddings in Milvus support the [Inner Product](https://zilliz.com/blog/similarity-metrics-for-vector-search#Inner-Product) (IP) distance metric, which is advantageous given their high-dimensional nature, making other metrics less effective. This functionality also supports data types with a dimension as an unsigned 32-bit integer and a 32-bit float for the value, thus facilitating a broad spectrum of applications, from nuanced text searches to elaborate [information retrieval](https://zilliz.com/learn/information-retrieval-metrics) systems.\n\nWith this new feature, Milvus allows for hybrid search methodologies that meld keyword and embedding-based techniques, offering a seamless transition for users moving from keyword-centric search frameworks seeking a comprehensive, low-maintenance solution.\n\nWe are labeling this feature as “Beta” to continue our performance testing of the feature and gather feedback from the community. The general availability (GA) of sparse vector support is anticipated with the release of Milvus 3.0.\n\n\n## CAGRA Index Support for Advanced GPU-Accelerated Graph Indexing\n\nDeveloped by NVIDIA, [CAGRA](https://arxiv.org/abs/2308.15136) (Cuda Anns GRAph-based) is a GPU-based graph indexing technology that significantly surpasses traditional CPU-based methods like the HNSW index in efficiency and performance, especially in high-throughput environments.\n\nWith the introduction of the CAGRA Index, Milvus 2.4 provides enhanced GPU-accelerated graph indexing capability. This enhancement is ideal for building similarity search applications requiring minimal latency. Additionally, Milvus 2.4 integrates a brute-force search with the CAGRA index to achieve maximum recall rates in applications. For detailed insights, explore the [introduction blog on CAGRA](https://zilliz.com/blog/Milvus-introduces-GPU-index-CAGRA).\n\n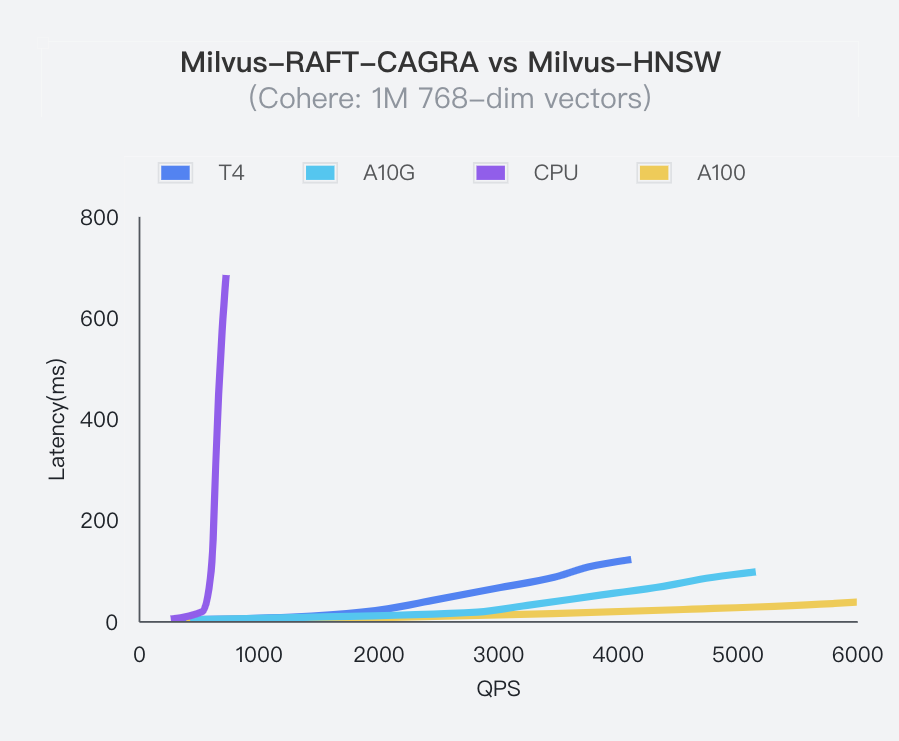\n\n## Additional Enhancements and Features\n\nMilvus 2.4 also includes other key enhancements, such as Regular Expression support for enhanced substring matching in [metadata filtering](https://zilliz.com/blog/metadata-filtering-with-zilliz-cloud-pipelines), a new scalar inverted index for efficient scalar data type filtering, and a Change Data Capture tool for monitoring and replicating changes in Milvus collections. These updates collectively enhance Milvus's performance and versatility, making it a comprehensive solution for complex data operations.\n\nFor more details, see [Milvus 2.4 documentation](https://milvus.io/docs/release_notes.md). \n\n\n## Stay Connected!\n\nExcited to learn more about Milvus 2.4? [Join our upcoming webinar](https://zilliz.com/event/unlocking-advanced-search-capabilities-milvus) with James Luan, Zilliz’s VP of Engineering, for an in-depth discussion on the capabilities of this latest release. If you have questions or feedback, join our [Discord channel](https://discord.com/invite/8uyFbECzPX) to engage with our engineers and community members. Don’t forget to follow us on [Twitter](https://twitter.com/milvusio) or [LinkedIn](https://www.linkedin.com/company/the-milvus-project) for the latest news and updates about Milvus.\n","title":"Unveiling Milvus 2.4: Multi-vector Search, Sparse Vector, CAGRA Index, and More!","metaData":{}},{"id":"milvus-supports-apache-parquet-file-supports.md","author":"Cai Zhang, Fendy Feng","desc":"By embracing Apache Parquet, users can streamline their data import processes and enjoy substantial storage and computation cost savings.","metaTitle":"Milvus Supports Imports of Apache Parquet Files","tags":["Engineering"],"recommend":true,"canonicalUrl":"https://milvus.io/blog/milvus-supports-apache-parquet-file-supports.md","date":"2024-03-08T00:00:00.000Z","cover":"https://assets.zilliz.com/Milvus_Supports_the_Imports_of_Parquet_Files_3288e755b8.png","href":"/blog/milvus-supports-apache-parquet-file-supports.md","content":"\n[Milvus](https://zilliz.com/what-is-milvus), the highly scalable vector database renowned for its ability to handle vast datasets, takes a significant step forward by introducing Parquet file support in [version 2.3.4](https://zilliz.com/blog/what-is-new-in-milvus-2-3-4). By embracing Apache Parquet, users can streamline their data import processes and enjoy substantial savings in storage and computation costs.\n\nIn our latest post, we explore Parquet's advantages and the benefits it brings to Milvus users. We discuss the motivation behind integrating this feature and provide a step-by-step guide on seamlessly importing Parquet files into Milvus, unlocking new possibilities for efficient data management and analysis.\n\n## What Is Apache Parquet? \n\n[Apache Parquet](https://parquet.apache.org/) is a popular open-source column-oriented data file format designed to enhance the efficiency of storing and processing large-scale datasets. In contrast to traditional row-oriented data formats like CSV or JSON, Parquet stores data by column, offering more efficient data compression and encoding schemes. This approach translates to improved performance, reduced storage requirements, and enhanced processing power, making it ideal for handling complex data in bulk. \n\n## How Milvus Users Benefit from the Support for Parquet File Imports\n\nMilvus extends support for Parquet file imports, providing users with optimized experiences and various advantages, including lowered storage and computation expenses, streamlined data management, and a simplified importing process.\n\n### Optimized Storage Efficiency and Streamlined Data Management \n\nParquet provides flexible compression options and efficient encoding schemes catering to different data types, ensuring optimal storage efficiency. This flexibility is particularly valuable in cloud environments where every ounce of storage savings directly correlates to tangible cost reductions. With this new feature in Milvus, users can effortlessly consolidate all their diverse data into a single file, streamlining data management and enhancing the overall user experience. This feature is particularly beneficial for users working with variable-length Array data types, who can now enjoy a simplified data import process. \n\n### Improved Query Performance \n\nParquet's columnar storage design and advanced compression methods significantly enhance query performance. When conducting queries, users can focus solely on the pertinent data without scanning through the irrelevant data. This selective column reading minimizes CPU usage, resulting in faster query times. \n\n### Broad Language Compatibility\n\nParquet is available in multiple languages such as Java, C++, and Python and is compatible with a large number of data processing tools. With the support of Parquet files, Milvus users using different SDKs can seamlessly generate Parquet files for parsing within the database. \n\n## How to Import Parquet Files into Milvus \n\nIf your data is already in Parquet file format, importing is easy. Upload the Parquet file to an object storage system such as MinIO, and you're ready to import. \n\nThe code snippet below is an example of importing Parquet files into Milvus.\n\n\n```\nremote_files = []\ntry:\n print(\"Prepare upload files\")\n minio_client = Minio(endpoint=MINIO_ADDRESS, access_key=MINIO_ACCESS_KEY, secret_key=MINIO_SECRET_KEY,\n secure=False)\n found = minio_client.bucket_exists(bucket_name)\n if not found:\n minio_client.make_bucket(bucket_name)\n print(\"MinIO bucket '{}' doesn't exist\".format(bucket_name))\n return False, []\n\n # set your remote data path\n remote_data_path = \"milvus_bulkinsert\"\n\n def upload_file(f: str):\n file_name = os.path.basename(f)\n minio_file_path = os.path.join(remote_data_path, \"parquet\", file_name)\n minio_client.fput_object(bucket_name, minio_file_path, f)\n print(\"Upload file '{}' to '{}'\".format(f, minio_file_path))\n remote_files.append(minio_file_path)\n\n upload_file(data_file)\n\nexcept S3Error as e:\n print(\"Failed to connect MinIO server {}, error: {}\".format(MINIO_ADDRESS, e))\n return False, []\n\nprint(\"Successfully upload files: {}\".format(remote_files))\nreturn True, remote_files\n```\n\nIf your data is not Parquet files or has dynamic fields, you can leverage BulkWriter, our data format conversion tool, to help you generate Parquet files. BulkWriter has now embraced Parquet as its default output data format, ensuring a more intuitive experience for developers. \n\nThe code snippet below is an example of using BulkWriter to generate Parquet files. \n\n```\nimport numpy as np\nimport json\n\nfrom pymilvus import (\n RemoteBulkWriter,\n BulkFileType,\n)\n\nremote_writer = RemoteBulkWriter(\n schema=your_collection_schema,\n remote_path=\"your_remote_data_path\",\n connect_param=RemoteBulkWriter.ConnectParam(\n endpoint=YOUR_MINIO_ADDRESS,\n access_key=YOUR_MINIO_ACCESS_KEY,\n secret_key=YOUR_MINIO_SECRET_KEY,\n bucket_name=\"a-bucket\",\n ),\n file_type=BulkFileType.PARQUET,\n)\n\n# append your data\nbatch_count = 10000\nfor i in range(batch_count):\n row = {\n \"id\": i,\n \"bool\": True if i % 5 == 0 else False,\n \"int8\": i % 128,\n \"int16\": i % 1000,\n \"int32\": i % 100000,\n \"int64\": i,\n \"float\": i / 3,\n \"double\": i / 7,\n \"varchar\": f\"varchar_{i}\",\n \"json\": {\"dummy\": i, \"ok\": f\"name_{i}\"},\n \"vector\": gen_binary_vector() if bin_vec else gen_float_vector(),\n f\"dynamic_{i}\": i,\n }\n remote_writer.append_row(row)\n\n# append rows by numpy type\nfor i in range(batch_count):\n remote_writer.append_row({\n \"id\": np.int64(i + batch_count),\n \"bool\": True if i % 3 == 0 else False,\n \"int8\": np.int8(i % 128),\n \"int16\": np.int16(i % 1000),\n \"int32\": np.int32(i % 100000),\n \"int64\": np.int64(i),\n \"float\": np.float32(i / 3),\n \"double\": np.float64(i / 7),\n \"varchar\": f\"varchar_{i}\",\n \"json\": json.dumps({\"dummy\": i, \"ok\": f\"name_{i}\"}),\n \"vector\": gen_binary_vector() if bin_vec else gen_float_vector(),\n f\"dynamic_{i}\": i,\n })\n\nprint(f\"{remote_writer.total_row_count} rows appends\")\nprint(f\"{remote_writer.buffer_row_count} rows in buffer not flushed\")\nprint(\"Generate data files...\")\nremote_writer.commit()\nprint(f\"Data files have been uploaded: {remote_writer.batch_files}\")\nremote_files = remote_writer.batch_files\n```\n\nThen, you can start to import your Parquet files into Milvus. \n\n```\nremote_files = [remote_file_path]\ntask_id = utility.do_bulk_insert(collection_name=collection_name,\n files=remote_files)\n\ntask_ids = [task_id] \nstates = wait_tasks_to_state(task_ids, BulkInsertState.ImportCompleted)\ncomplete_count = 0\nfor state in states:\n if state.state == BulkInsertState.ImportCompleted:\n complete_count = complete_count + 1\n ```\n\nNow, your data is seamlessly integrated into Milvus.\n\n## What's Next?\n\nAs Milvus continues to support ever-growing data volumes, the challenge arises in managing sizable imports, particularly when Parquet files surpass 10GB. To tackle this challenge, we plan to segregate the import data into scalar and vector columns, creating two Parquet files per import to alleviate the I/O pressure. For datasets exceeding several hundred gigabytes, we recommend importing the data multiple times. \n\n","title":"Milvus Supports Imports of Apache Parquet Files for Enhanced Data Processing Efficiency","metaData":{}},{"id":"what-milvus-version-to-start-with.md","author":"Chris Churilo","desc":"A comprehensive guide to the features and capabilities of each Milvus version to make an informed decision for your vector search projects.","tags":["Engineering"],"recommend":true,"canonicalUrl":"https://milvus.io/blog/what-milvus-version-to-start-with.md","date":"2024-02-19T00:00:00.000Z","cover":"https://assets.zilliz.com/which_milvus_to_start_4a4250e314.jpeg","href":"/blog/what-milvus-version-to-start-with.md","content":"\n# Introduction to the Milvus versions\n\nSelecting the appropriate Milvus version is foremost to the success of any project leveraging vector search technology. With different Milvus versions tailored to varying requirements, understanding the importance of selecting the correct version is crucial for achieving the desired results.\n\nThe right Milvus version can help a developer to learn and prototype quickly or help optimize resource utilization, streamline development efforts, and ensure compatibility with existing infrastructure and tools. Ultimately, it is about maintaining developer productivity and improving efficiency, reliability, and user satisfaction.\n\n\n## Available Milvus versions \n\nThree versions of Milvus are available for developers, and all are open source. The three versions are Milvus Lite, Milvus Standalone, and Milvus Cluster, which differ in features and how users plan to use Milvus in the short and long term. So, let's explore these individually.\n\n\n## Milvus Lite\n\nAs the name suggests, Milvus Lite is a lightweight version that integrates seamlessly with Google Colab and Jupyter Notebook. It is packaged as a single binary with no additional dependencies, making it easy to install and run on your machine or embed in Python applications. Additionally, Milvus Lite includes a CLI-based Milvus standalone server, providing flexibility for running it directly on your machine. Whether you embed it within your Python code or utilize it as a standalone server is entirely up to your preference and specific application requirements.\n\n\n### Features and Capabilities\n\nMilvus Lite includes all core Milvus vector search features. \n\n- **Search Capabilities**: Supports top-k, range, and hybrid searches, including metadata filtering, to cater to diverse search requirements.\n\n- **Index Types and Similarity Metrics**: Offers support for 11 index types and five similarity metrics, providing flexibility and customization options for your specific use case.\n\n- **Data Processing**: Enables batch (Apache Parquet, Arrays, JSON) and stream processing, with seamless integration through connectors for Airbyte, Apache Kafka, and Apache Spark.\n\n- **CRUD Operations**: Offers full CRUD support (create, read, update/upsert, delete), empowering users with comprehensive data management capabilities.\n\n\n### Applications and limitations\n\nMilvus Lite is ideal for rapid prototyping and local development, offering support for quick setup and experimentation with small-scale datasets on your machine. However, its limitations become apparent when transitioning to production environments with larger datasets and more demanding infrastructure requirements. As such, while Milvus Lite is an excellent tool for initial exploration and testing, it may not be suitable for deploying applications in high-volume or production-ready settings.\n\n\n### Available Resources\n\n- [Documentation](https://milvus.io/docs/milvus_lite.md)\n\n- [Github Repository](https://github.com/milvus-io/milvus-lite/)\n\n- [Google Colab Example](https://github.com/milvus-io/milvus-lite/tree/main/examples)\n\n- [Getting Started Video](https://www.youtube.com/watch?v=IgJdrGiB5ZY)\n\n\n## Milvus Standalone\n\nMilvus offers two operational modes: Standalone and Cluster. Both modes are identical in core vector database features and differ in data size support and scalability requirements. This distinction allows you to select the mode that best aligns with your dataset size, traffic volume, and other infrastructure requirements for production. \n\nMilvus Standalone is a mode of operation for the Milvus vector database system where it operates independently as a single instance without any clustering or distributed setup. Milvus runs on a single server or machine in this mode, providing functionalities such as indexing and searching for vectors. It is suitable for situations where the data and traffic volume scale is relatively small and does not require the distributed capabilities provided by a clustered setup.\n\n\n### Features and Capabilities\n\n- **High Performance**: Conduct vector searches on massive datasets (billions or more) with exceptional speed and efficiency.\n\n- **Search Capabilities**: Supports top-k, range, and hybrid searches, including metadata filtering, to cater to diverse search requirements.\n\n- **Index Types and Similarity Metrics**: Offers support for 11 index types and 5 similarity metrics, providing flexibility and customization options for your specific use case.\n\n- **Data Processing**: Enables both batch (Apache Parquet, Arrays, Json) and stream processing, with seamless integration through connectors for Airbyte, Apache Kafka, and Apache Spark.\n\n- **Scalability**: Achieve dynamic scalability with component-level scaling, allowing for seamless scaling up and down based on demand. Milvus can autoscale at a component level, optimizing resource allocation for enhanced efficiency.\n\n- **Multi-Tenancy**: Supports multi-tenancy with the capability to manage up to 10,000 collections/partitions in a cluster, providing efficient resource utilization and isolation for different users or applications.\n\n- **CRUD Operations**: Offers full CRUD support (create, read, update/upsert, delete), empowering users with comprehensive data management capabilities.\n\n\n### Essential components:\n\n- Milvus: The core functional component.\n\n- etcd: The metadata engine responsible for accessing and storing metadata from Milvus' internal components, including proxies, index nodes, and more.\n\n- MinIO: The storage engine responsible for data persistence within Milvus.\n\n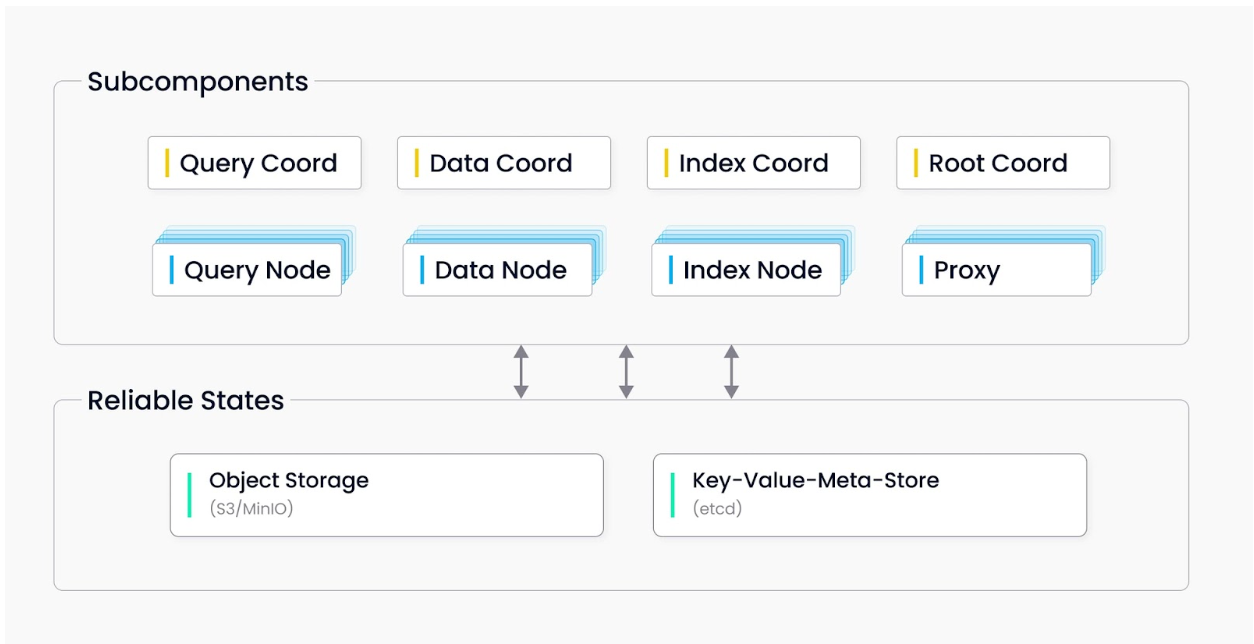\n\nFigure 1: Milvus Standalone Architecture\n\n\n### Available Resources\n\n- Documentation\n\n - [Environment Checklist for Milvus with Docker Compose](https://milvus.io/docs/prerequisite-docker.md)\n\n - [Install Milvus Standalone with Docker](https://milvus.io/docs/install_standalone-docker.md)\n\n- [Github Repository](https://github.com/milvus-io/milvus)\n\n\n## Milvus Cluster\n\nMilvus Cluster is a mode of operation for the Milvus vector database system where it operates and is distributed across multiple nodes or servers. In this mode, Milvus instances are clustered together to form a unified system that can handle larger volumes of data and higher traffic loads compared to a standalone setup. Milvus Cluster offers scalability, fault tolerance, and load balancing features, making it suitable for scenarios that need to handle big data and serve many concurrent queries efficiently.\n\n\n### Features and Capabilities\n\n- Inherits all features available in Milvus Standalone, including high-performance vector search, support for multiple index types and similarity metrics, and seamless integration with batch and stream processing frameworks.\n\n- Offers unparalleled availability, performance, and cost optimization by leveraging distributed computing and load balancing across multiple nodes.\n\n- Enables deploying and scaling secure, enterprise-grade workloads with lower total costs by efficiently utilizing resources across the cluster and optimizing resource allocation based on workload demands.\n\n\n### Essential components:\n\nMilvus Cluster includes eight microservice components and three third-party dependencies. All microservices can be deployed on Kubernetes independently from each other.\n\n\n#### Microservice components\n\n- Root coord\n\n- Proxy\n\n- Query coord\n\n- Query node\n\n- Index coord\n\n- Index node\n\n- Data coord\n\n- Data node\n\n\n#### Third-party dependencies\n\n- etcd: Stores metadata for various components in the cluster.\n\n- MinIO: Responsible for data persistence of large files in the cluster, such as index and binary log files.\n\n- Pulsar: Manages logs of recent mutation operations, outputs streaming log, and provides log publish-subscribe services.\n\n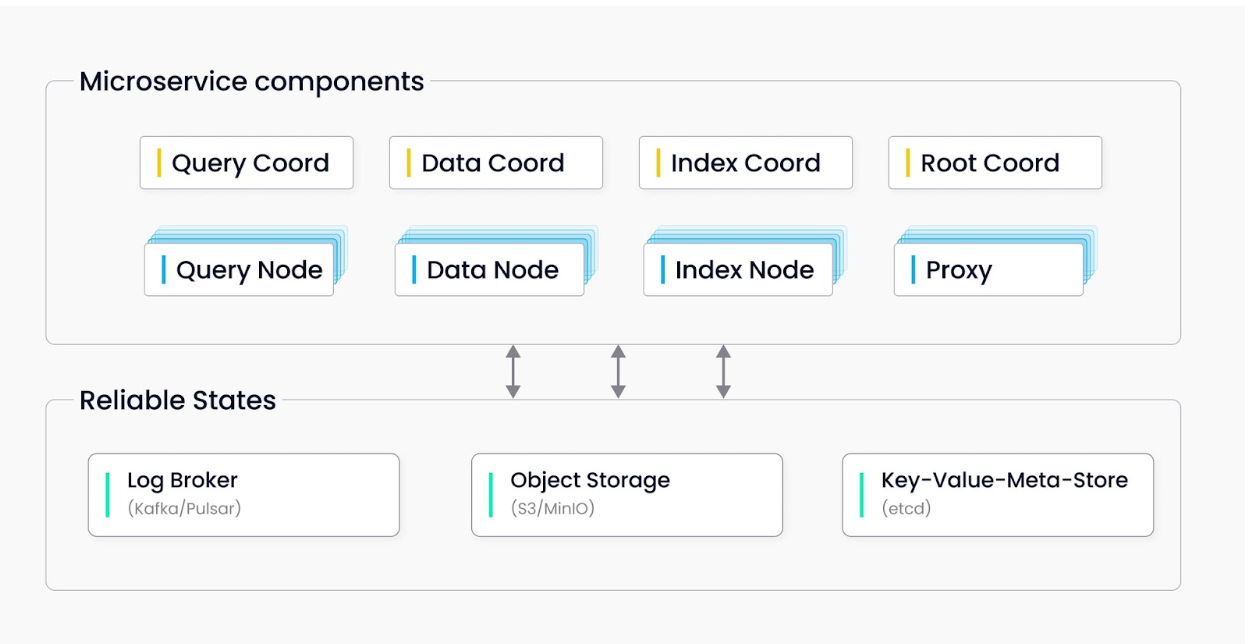\n\nFigure 2: Milvus Cluster Architecture\n\n\n#### Available Resources\n\n- [Documentation](https://milvus.io/docs/install_cluster-milvusoperator.md) | How to get started\n\n - [Install Milvus Cluster with Milvus Operator](https://milvus.io/docs/install_cluster-milvusoperator.md)\n\n - [Install Milvus Cluster with Helm](https://milvus.io/docs/install_cluster-helm.md)\n\n - [How to scale a Milvus Cluster](https://milvus.io/docs/scaleout.md)\n\n- [Github Repository](https://github.com/milvus-io/milvus)\n\n\n## Making the Decision on which Milvus version to use\n\nWhen deciding which version of Milvus to use for your project, you must consider factors such as your dataset size, traffic volume, scalability requirements, and production environment constraints. Milvus Lite is perfect for prototyping on your laptop. Milvus Standalone offers high performance and flexibility for conducting vector searches on your datasets, making it suitable for smaller-scale deployments, CI/CD, and offline deployments when you have no Kubernetes support.. And finally, Milvus Cluster provides unparalleled availability, scalability, and cost optimization for enterprise-grade workloads, making it the preferred choice for large-scale, highly available production environments.\n\nThere is another version that is a hassle-free version, and that is a managed version of Milvus called [Zilliz Cloud](https://cloud.zilliz.com/signup). \n\nUltimately, the Milvus version will depend on your specific use case, infrastructure requirements, and long-term goals. By carefully evaluating these factors and understanding the features and capabilities of each version, you can make an informed decision that aligns with your project's needs and objectives. Whether you choose Milvus Standalone or Milvus Cluster, you can leverage the power of vector databases to enhance the performance and efficiency of your AI applications.\n","title":"What Milvus version to start with","metaData":{}},{"id":"top-10-keywords-dominates-milvus-community-in-2023.md","author":"Jack Li, Fendy Feng","desc":"This post explores the heart of the community by analyzing chat histories and revealing the top 10 keywords in discussions.","metaTitle":"Top 10 Keywords Dominating the Milvus Community in 2023","tags":["Engineering"],"recommend":true,"canonicalUrl":"https://milvus.io/blog/top-10-keywords-dominates-milvus-community-in-2023.md","date":"2024-01-21T00:00:00.000Z","cover":"https://assets.zilliz.com/Top_10_Keywords_in_the_Milvus_Community_20240116_111204_1_f65b17a8ea.png","href":"/blog/top-10-keywords-dominates-milvus-community-in-2023.md","content":"\nAs we conclude 2023, let's review the Milvus community's remarkable journey: boasting [25,000 GitHub Stars](https://github.com/milvus-io/milvus), the launch of [Milvus 2.3.0](https://milvus.io/blog/unveiling-milvus-2-3-milestone-release-offering-support-for-gpu-arm64-cdc-and-other-features.md), and exceeding 10 million [Docker image](https://hub.docker.com/r/milvusdb/milvus) downloads. This post explores the heart of the community by analyzing chat histories and revealing the top 10 keywords in discussions.\n\n\n\u003ciframe class=\"video-player\" src=\"https://www.youtube.com/embed/o5uMdNLioQ0?list=PLPg7_faNDlT5Fb8WN8r1PzzQTNzdechnS\" title=\"Mastering Milvus: Turbocharge Your Vector Database with Optimization Secrets!\" frameborder=\"0\" allow=\"accelerometer; autoplay; clipboard-write; encrypted-media; gyroscope; picture-in-picture; web-share\" allowfullscreen\u003e\u003c/iframe\u003e\n\n## #1 Version — The rise of AIGC drives rapid Milvus iteration\n\nSurprisingly, \"Version\" emerged as the most discussed keyword in 2023. This revelation is rooted in the year's AI wave, with vector databases as a critical infrastructure to tackle challenges in AIGC applications' hallucination issues.\n\nThe enthusiasm around vector databases drives Milvus into a stage of swift iteration. The community witnessed the release of Twenty versions in 2023 alone, accommodating the demands of AIGC developers flooding the community with inquiries about choosing the optimal version of Milvus for various applications. For users navigating these updates, we recommend embracing the latest version for enhanced features and performance. \n\nIf you are interested in Milvus's release planning, refer to the [Milvus Roadmap](https://wiki.lfaidata.foundation/display/MIL/Milvus+Long+Term+Roadmap+and+Time+schedule) page on the official website. \n\n\n## #2 Search — beyond Vector Search\n\n\"Search\" takes second place, reflecting its fundamental role in database operations. Milvus supports various search capabilities, from Top-K ANN search to scalar filtered search and range search. The imminent release of Milvus 3.0 (Beta) promises keyword search (sparse embeddings), which many RAG app developers eagerly await.\n\nCommunity discussions about searching focus on performance, capabilities, and principles. Users often ask questions about attribute filtering, setting index threshold values, and addressing latency concerns. Resources like [query and search documentation](https://milvus.io/docs/v2.0.x/search.md), [Milvus Enhancement Proposals (MEPs)](https://wiki.lfaidata.foundation/pages/viewpage.action?pageId=43287103), and Discord discussions have become the go-to references for unraveling the intricacies of searching within Milvus.\n\n\n## #3 Memory — trade-offs between performance and accuracy for minimized memory overhead\n\n“Memory” also took center stage in community discussions over the past year. As a distinctive data type, vectors inherently have high dimensions. Storing vectors in memory is a common practice for optimal performance, but the escalating data volume limits available memory. Milvus optimizes memory usage by adopting techniques like [MMap](https://zilliz.com/blog/milvus-introduced-mmap-for-redefined-data-management-increased-storage-capability) and DiskANN.\n\nHowever, achieving low memory usage, excellent performance, and high accuracy simultaneously in a database system remains complex, necessitating trade-offs between performance and accuracy to minimize memory overhead.\n\nIn the case of Artificial Intelligence Generated Content (AIGC), developers usually prioritize fast responses and result accuracy over stringent performance requirements. Milvus’s addition of MMap and DiskANN minimizes memory usage while maximizing data processing and result accuracy, striking a balance that aligns with the practical needs of AIGC applications. \n\n\n## #4 Insert — smooth sailing through data insertion\n\nEfficient data insertion is a crucial concern for developers, sparking frequent discussions on optimizing insertion speed within the Milvus community. Milvus excels in the efficient insertion of streaming data and the building of indexes, thanks to its adept separation of streaming and batch data. This capability sets it apart as a highly performant solution compared to other vector database providers, such as Pinecone.\n\nHere are some valuable insights and recommendations about data insertions: \n\n- **Batch Insertion:** Opt for batch over single-row insertion for enhanced efficiency. Notably, insertion from files surpasses batch insertion in speed. When handling large datasets exceeding ten million records, consider using the `bulk_insert` interface for a streamlined and accelerated import process.\n\n- **Strategic `flush()` Usage:** Rather than invoking the `flush()` interface after each batch, make a single call after completing all data insertion. Excessive use of the `flush()` interface between batches can lead to the generation of fragmented segment files, placing a considerable compaction burden on the system.\n\n- **Primary Key Deduplication:** Milvus does not perform primary key deduplication when using the `insert` interface for data insertion. If you need to deduplicate primary keys, we recommend you deploy the `upsert` interface. However, the insertion performance of `upsert `is lower than that of `insert`, owing to an additional internal query operation. \n\n\n## #5 Configuration — decoding the parameter maze\n\nMilvus is a distributed vector database that integrates many third-party components like object storage, message queues, and Etcd. Users grappled with adjusting parameters and understanding their impact on Milvus's performance, making \"Configuration\" a frequently discussed topic.\n\nAmong all the questions about configurations, \"which parameters to adjust\" is arguably the most challenging aspect, as the parameters vary in different situations. For instance, optimizing search performance parameters differs from optimizing insertion performance parameters and relies heavily on practical experience.\n\nOnce users identify \"which parameters to adjust,\" the subsequent questions of \"how to adjust\" become more manageable. For specific procedures, refer to our documentation [Configure Milvus](https://milvus.io/docs/configure-helm.md). The great news is that Milvus has supported dynamic parameter adjustments since version 2.3.0, eliminating the need for restarts for changes to take effect. For specific procedures, refer to [Configure Milvus on the Fly](https://milvus.io/docs/dynamic_config.md). \n\n\n## #6 Logs — navigating the troubleshooting compass\n\n\"Logs\" serve as the troubleshooter's compass. Users sought guidance in the community on exporting Milvus logs, adjusting log levels, and integrating with systems like Grafana’s Loki. Here are some suggestions about Milvus logs. \n\n- **How to view and export Milvus logs:** You can easily export Milvus logs with the one-click script [export-milvus-log.sh](https://github.com/milvus-io/milvus/tree/master/deployments/export-log) which is available on the GitHub repository. \n\n- **Log level:** Milvus has multiple log levels to accommodate diverse use cases. The info level is enough for most cases, and the debug level is for debugging. An excess of Milvus logs may signal misconfigured log levels.\n\n- **We recommend integrating Milvus logs with a log collection system** like Loki for streamlined log retrieval in future troubleshooting.\n\n\n## #7 Cluster — scaling for production environments\n\nGiven Milvus's identity as a distributed vector database, the term \"cluster\" is a frequent topic of discussion in the community. Conversations revolve around scaling data in a cluster, data migration, and data backup and synchronization. \n\nIn production environments, robust scalability and high availability are standard requirements for distributed database systems. Milvus's storage-computation separation architecture allows seamless data scalability by expanding resources for computation and storage nodes, accommodating limitless data scales. Milvus also provides high availability with a multi-replica architecture and robust backup and syncing capabilities. For more information, refer to [Coordinator HA](https://milvus.io/docs/coordinator_ha.md#Coordinator-HA).\n\n\n## #8 Documentation — the gateway to understanding Milvus\n\n\"Documentation\" is another frequently raised keyword in community discussions, often tied to questions about whether there is any documentation page for a specific feature and where to find it. \n\nServing as the gateway to understanding Milvus, around 80% of community inquiries find answers in the [official documentation](https://milvus.io/docs). We recommend you read our documentation before using Milvus or encountering any problems. In addition, you can explore code examples in various SDK repositories for insights into using Milvus. \n\n\n## #9 Deployment — simplifying the Milvus journey\n\nSimple deployment remains the Milvus team’s ongoing goal. To fulfill this commitment, we introduced [Milvus Lite](https://milvus.io/docs/milvus_lite.md#Get-Started-with-Milvus-Lite), a lightweight alternative to Milvus that is fully functional but has no K8s or Docker dependencies. \n\nWe further streamlined deployment by introducing the lighter [NATS](https://zilliz.com/blog/optimizing-data-communication-milvus-embraces-nats-messaging) messaging solution and consolidating node components. Responding to user feedback, we're gearing up to release a standalone version without dependencies, with ongoing efforts to enhance features and simplify deployment operations. The rapid iteration of Milvus showcases the community's continuous commitment to the continued refinement of the deployment process.\n\n\n## #10 Deletion — unraveling the impact\n\nThe prevalent discussions on \"deletion\" revolve around unchanged data counts after deletion, the continued retrievability of deleted data, and the failure of disk space recovery after deletion.\n\nMilvus 2.3 introduces the `count(*)` expression to address delayed entity count updates. The persistence of deleted data in queries is probably due to the inappropriate use of [data consistency models](https://zilliz.com/blog/understand-consistency-models-for-vector-databases). Disk space recovery failure concerns prompt insights into redesigning Milvus's garbage collection mechanism, which sets a waiting period before the complete deletion of data. This approach allows a time window for potential recovery.\n\n\n## Conclusion\n\nThe top 10 keywords offer a glimpse into the vibrant discussions within the Milvus community. As Milvus continues to evolve, the community remains an invaluable resource for developers seeking solutions, sharing experiences, and contributing to advancing vector databases in the era of AI. \n\nJoin this exciting journey by joining our [Discord channel](https://discord.com/invite/8uyFbECzPX) in 2024. There, you can engage with our brilliant engineers and connect with like-minded Milvus enthusiasts. Also, attend the [Milvus Community Lunch and Learn](https://discord.com/invite/RjNbk8RR4f) every Tuesday from 12:00 to 12:30 PM PST. Share your thoughts, questions, and feedback, as every contribution adds to the collaborative spirit propelling Milvus forward. Your active participation is not just welcomed; it's appreciated. Let's innovate together!\n","title":"Unveiling the Top 10 Keywords Dominating the Milvus Community in 2023","metaData":{}},{"id":"milvus-2-3-4-faster-searches-expanded-data-support-improved-monitoring-and-more.md","author":"Ken Zhang, Fendy Feng","tags":["News"],"desc":"introducing Milvus 2.3.4 new features and improvements","recommend":true,"canonicalUrl":"https://milvus.io/blog/milvus-2-3-4-faster-searches-expanded-data-support-improved-monitoring-and-more.md","date":"2024-01-12T00:00:00.000Z","cover":"https://assets.zilliz.com/What_is_new_in_Milvus_2_3_4_1847b0fa8a.png","href":"/blog/milvus-2-3-4-faster-searches-expanded-data-support-improved-monitoring-and-more.md","content":"\n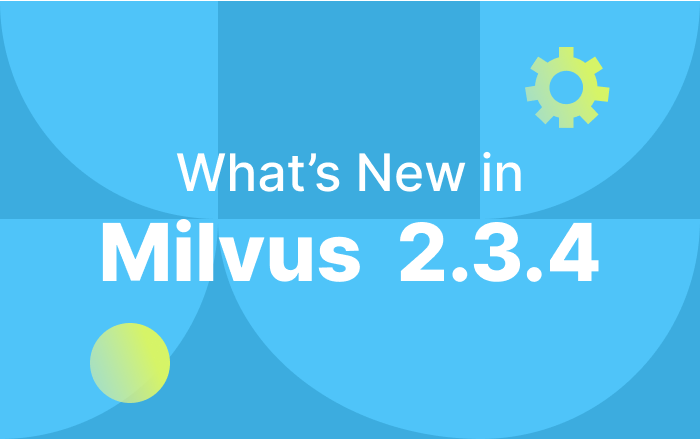\n\n\nWe are excited to unveil the latest release of Milvus 2.3.4. This update introduces a suite of features and enhancements meticulously crafted to optimize performance, boost efficiency, and deliver a seamless user experience. In this blog post, we'll delve into the highlights of Milvus 2.3.4. \n\n## Access logs for improved monitoring\n\nMilvus now supports access logs, offering invaluable insights into interactions with external interfaces. These logs record method names, user requests, response times, error codes, and other interaction information, empowering developers and system administrators to conduct performance analysis, security auditing, and efficient troubleshooting. \n\n**_Note:_** _Currently, access logs only support gRPC interactions. However, our commitment to improvement continues, and future versions will extend this capability to include RESTful request logs._ \n\nFor more detailed information, refer to [Configure Access Logs](https://milvus.io/docs/configure_access_logs.md).\n\n## Parquet file imports for enhanced data processing efficiency\n\nMilvus 2.3.4 now supports importing Parquet files, a widely embraced columnar storage format designed to enhance the efficiency of storing and processing large-scale datasets. This addition gives users increased flexibility and efficiency in their data processing endeavors. By eliminating the need for laborious data format conversions, users managing substantial datasets in the Parquet format will experience a streamlined data import process, significantly reducing the time from initial data preparation to subsequent vector retrieval.\n\nFurthermore, our data format conversion tool, BulkWriter, has now embraced Parquet as its default output data format, ensuring a more intuitive experience for developers. \n\n## Binlog index on growing segments for faster searches\n\nMilvus now leverages a binlog index on growing segments, resulting in up to tenfold faster searches in growing segments. This enhancement significantly boosts search efficiency and supports advanced indices like IVF or Fast Scan, improving the overall user experience.\n\n## Support for up to 10,000 collections/partitions\n\nLike tables and partitions in relational databases, collections and partitions are the core units for storing and managing vector data in Milvus. Responding to users' evolving needs for nuanced data organization, Milvus 2.3.4 now supports up to 10,000 collections/partitions in a cluster, a significant jump from the previous limit of 4,096. This enhancement benefits diverse use cases, such as knowledge base management and multi-tenant environments. The expanded support for collections/partitions stems from refinements to the time tick mechanism, goroutine management, and memory usage.\n\n **_Note:_** _The recommended limit for the number of collections/partitions is 10,000, as exceeding this limit may impact failure recovery and resource usage._\n\n## Other enhancements \n\nIn addition to the features above, Milvus 2.3.4 includes various improvements and bug fixes. These include reduced memory usage during data retrieval and variable-length data handling, refined error messaging, accelerated loading speed, and improved query shard balance. These collective enhancements contribute to a smoother and more efficient overall user experience.\n\nFor a comprehensive overview of all the changes introduced in Milvus 2.3.4, refer to our [Release Notes](https://milvus.io/docs/release_notes.md#v234).\n\n## Stay connected!\n\nIf you have questions or feedback about Milvus, join our [Discord channel](https://discord.com/invite/8uyFbECzPX) to engage with our engineers and the community directly or join our [Milvus Community Lunch and Learn](https://discord.com/invite/RjNbk8RR4f) Every Tuesday from 12-12:30 PM PST. You’re also welcome to follow us on [Twitter](https://twitter.com/milvusio) or [LinkedIn](https://www.linkedin.com/company/the-milvus-project) for the latest news and updates about Milvus.\n","title":"Milvus 2.3.4: Faster Searches, Expanded Data Support, Improved Monitoring, and More","metaData":{}},{"id":"milvus-in-2023-unprecedented-vector-database-amidst-tech-buzz.md","author":"James Luan","desc":"Reflecting on the entire vector database industry, with a special focus on Milvus—a standout product in this landscape.","tags":[],"recommend":true,"canonicalUrl":"https://thenewstack.io/milvus-in-2023-open-source-vector-database-year-in-review/","date":"2024-01-05T00:00:00.000Z","cover":"https://assets.zilliz.com/Milvus_in_2023_An_Atypical_Vector_DB_Amidst_Tech_Buzz_1_1151400765.png","href":"/blog/milvus-in-2023-unprecedented-vector-database-amidst-tech-buzz.md","content":"\n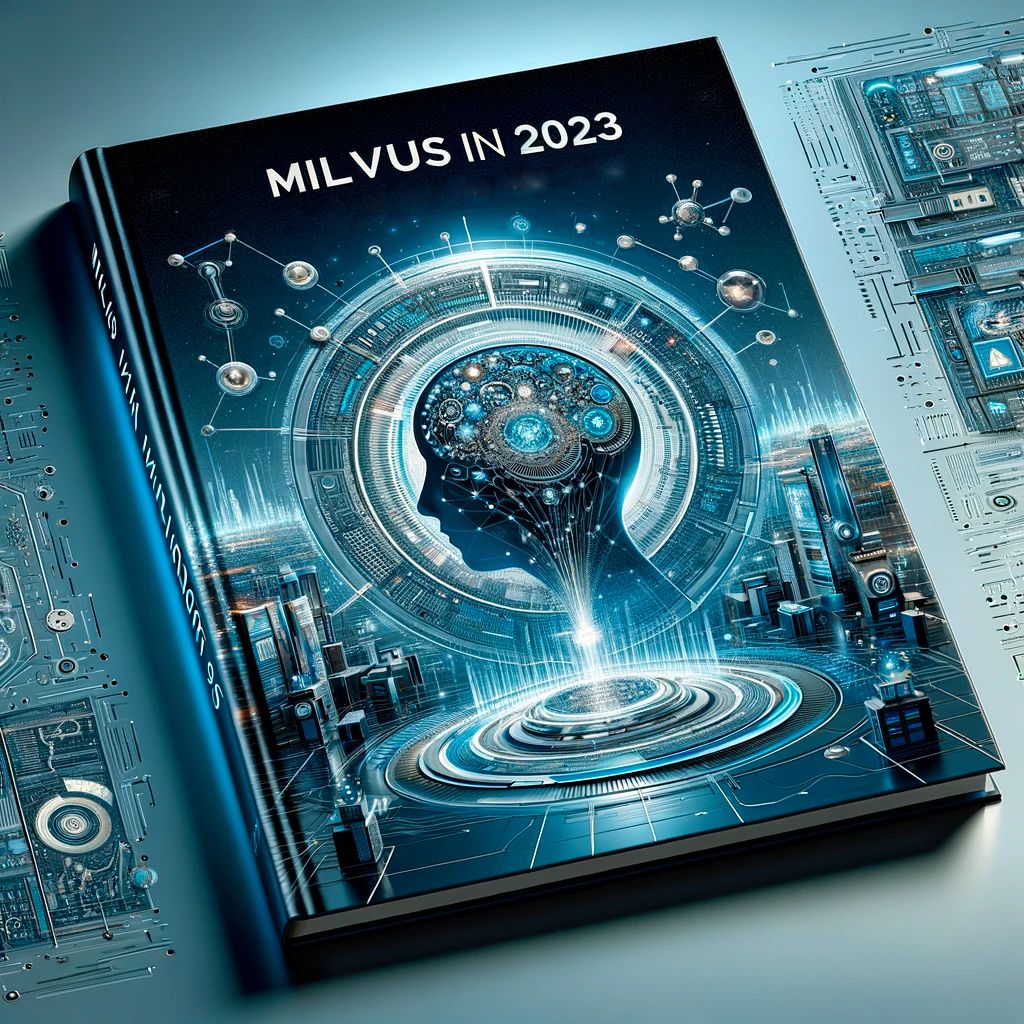\n# Milvus in 2023: An Unprecedented Vector Database Amidst Tech Buzz\n\n\n*This post was written by James Luan with the help of ChatGPT. James primarily wrote prompts and reviewed and polished the AI-generated content.*\n\n## 2023: the year of AI \n\n2023 marks a pivotal turning point in artificial intelligence (AI). [Large Language Models (LLMs)](https://zilliz.com/glossary/large-language-models-\\(llms\\)) have taken center stage, garnering widespread recognition for their exceptional natural language processing capabilities. This surge in popularity has substantially expanded the possibilities of machine learning applications, enabling developers to construct more intelligent and interactive applications.\n\n\nAmidst this revolution, [vector databases](https://zilliz.com/learn/what-is-vector-database) have emerged as a crucial component, acting as the long-term memory for LLMs. The rise of [Retrieval-Augmented Generation (RAG)](https://zilliz.com/use-cases/llm-retrieval-augmented-generation) models, intelligent agents, and multimodal retrieval apps has demonstrated the vast potential of vector databases in enhancing multimodal data retrieval efficiency, reducing hallucinations in LLMs, and supplementing domain knowledge.\n\n\nThe LLM evolution has also catalyzed significant advancements in embedding technologies. According to the [Massive Text Embedding Benchmark (MTEB) Leaderboard](https://huggingface.co/spaces/mteb/leaderboard) on HuggingFace, leading embedding models such as UAE, VoyageAI, CohereV3, and Bge were all released in 2023. These advancements have bolstered the vector retrieval effectiveness of various vector search technologies like Milvus, providing more precise and efficient data processing capabilities for AI applications.\n\n\nHowever, with the growing popularity of vector databases, debates arose about the necessity of specialized solutions. Tens of startups have entered the vector database arena. Many traditional relational and NoSQL databases have started treating vectors as a significant data type, and many claim to be capable of substituting specialized vector databases in every situation. \n\n\nAs we enter 2024, it's an sensible moment to reflect on the entire vector database industry, with a special focus on Milvus—a standout product in this landscape. \n\n\n\n\n## Milvus in 2023: numbers don't lie\n\n\nFirst launched in 2019, [Milvus](https://zilliz.com/what-is-milvus) has pioneered the concept of vector databases and consistently maintained a reputation for high reliability, scalability, search quality, and performance. In 2023, Milvus achieved impressive results and underwent significant shifts, primarily driven by the rapid advancement of LLMs and the boom of AIGC applications. Here are some key figures that best represent Milvus's progress in 2023. \n\n\n\n\n### ZERO downtime during rolling upgrades\n\n\nFor those new to vector databases, their primary focus centers on functionality rather than operational maintenance. Many application developers also pay less attention to stability in their vector databases than transactional databases since their applications are often in the early stages of exploration. However, stability becomes indispensable if you aim to deploy your AIGC application in a production environment and achieve the best user experience. \n\n\nMilvus distinguishes itself by prioritizing not just functionality but also operational stability. We added rolling upgrades to Milvus starting from version 2.2.3. After continuous refinement, this feature can ensure zero downtime during upgrades without interrupting business processes. \n\n\n\n\n### 3x performance improvement in production environments\n\n\nBoosting vector search performance needs to be a primary goal for vector databases. Many vector search solutions chose to base their solution on adapting the [HNSW](https://zilliz.com/learn/hierarchical-navigable-small-worlds-HNSW) algorithm to get to market quickly; unfortunately, this leads to them facing significant challenges in real-world production environments, especially with highly filtered searches (over 90%) and frequent data deletions. Milvus considers performance from the get-go and excels in optimizing performance during any phase of development, especially in production environments, achieving a threefold improvement in search performance, especially in filtered search and streaming insert/search situations.\n\n\nTo further assist the vector database community, we introduced [VectorDBBench](https://github.com/zilliztech/VectorDBBench), an open-source benchmarking tool, last year. This tool is vital for early evaluations of vector databases across different conditions. Unlike traditional evaluation methods, VectorDBBench assesses databases using real-world data, including super large datasets or those closely resembling data from actual embedding models, providing users with more insightful information for informed decision-making.\n\n\n\n\n### 5% recall improvement on the Beir dataset\n\n\nWhile [dense embeddings](https://zilliz.com/learn/sparse-and-dense-embeddings) have proven effective in vector search, they must catch up when searching for names, objects, abbreviations, and short query contexts. In response to their limitations, Milvus has introduced a hybrid query approach that integrates dense embeddings with [sparse embeddings](https://zilliz.com/learn/sparse-and-dense-embeddings) to enhance the quality of search results. The synergy of this hybrid solution with a reranking model has resulted in a substantial 5% improvement in the recall rate on the Beir dataset, as validated by our tests.\n\n\nGoing beyond improvements in search quality, Milvus has also unveiled a graph-based retrieval solution tailored for sparse embeddings, surpassing the performance of conventional search algorithms like WAND.\n\n\nAt the 2023 NeurIPS BigANN competition, Zihao Wang, a talented engineer at Zilliz, presented [Pyanns](https://big-ann-benchmarks.com/neurips23.html#winners), a search algorithm that demonstrated significant superiority over other entries in the sparse embedding search track. This breakthrough solution is a precursor to our sparse embedding search algorithms for production environments.\n\n\n\n\n### 10x memory saving on large datasets\n\n\n[Retrieval Augmented Generation](https://zilliz.com/use-cases/llm-retrieval-augmented-generation) (RAG) was the most popular use case for vector databases in 2023. However, the increase in vector data volumes with RAG applications presents a storage challenge for these applications. This challenge is especially true when the volume of transformed vectors exceeds that of the original document chunks, potentially escalating memory usage costs. For example, after dividing documents into chunks, the size of a 1536-dimensional float32 vector (roughly 3kb) transformed from a 500-token chunk (about 1kb) is greater than the 500-token chunk. \n\n\nMilvus is the first open-source vector database to support disk-based indexing, bringing about a remarkable 5x memory saving. By the close of 2023, we introduced [Milvus 2.3.4](https://milvus.io/docs/release_notes.md#v234), enabling the capability to load scalar and vector data/indexes onto the disk using memory-mapped files ([MMap](https://zilliz.com/blog/milvus-introduced-mmap-for-redefined-data-management-increased-storage-capability)). This advancement offers more than a 10x reduction in memory usage compared to traditional in-memory indexing. \n\n\n\n\n### 20 Milvus releases\n\n\nIn 2023, Milvus underwent a transformative journey marked by significant milestones. Over the year, we launched 20 releases, a testament to the dedication of over 300 community developers and the realization of our commitment to a user-driven approach in development.\n\n\nTo illustrate, Milvus 2.2.9 introduced [dynamic schema](https://zilliz.com/blog/what-is-dynamic-schema), marking a crucial shift from prioritizing performance to enhancing usability. Building on this, [Milvus 2.3](https://milvus.io/blog/unveiling-milvus-2-3-milestone-release-offering-support-for-gpu-arm64-cdc-and-other-features.md) introduced critical features such as Upsert, [Range Search](https://zilliz.com/blog/unlock-advanced-recommendation-engines-with-milvus-new-range-search), Cosine metrics, and more, all driven by our user community's specific needs and feedback. This iterative development process underscores our commitment to continually aligning Milvus with the evolving requirements of our users.\n\n\n\n\n### 1,000,000 tenants in a Single Custer \n\n\nImplementing multi-tenancy is crucial for developing RAG systems, AI agents, and other LLM applications, meeting the heightened user demands for data isolation. For B2C businesses, tenant numbers can skyrocket into the millions, making physical isolation of user data impractical (as an example, it's unlikely that anyone would create millions of tables in a relational database). Milvus introduced the Partition Key feature, allowing for efficient, logical isolation and data filtering based on partition keys, which is handy at a large scale.\n\n\nConversely, B2B enterprises, accustomed to dealing with tens of thousands of tenants, benefit from a more nuanced strategy involving physical resource isolation. The latest Milvus 2.3.4 brings enhanced memory management, coroutine handling, and CPU optimization, making creating tens of thousands of tables within a single cluster easier. This enhancement also accommodates the needs of B2B businesses with enhanced efficiency and control.\n\n\n\n\n### 10,000,000 Docker image pulls\n\n\nAs 2023 drew to a close, Milvus reached an impressive milestone with [10 million Docker pull](https://hub.docker.com/r/milvusdb/milvus) downloads. This accomplishment signals the increasing fascination of the developer community with Milvus and emphasizes its rising significance within the vector database domain. \n\n\nAs the world's first cloud-native vector database, Milvus boasts seamless integration with Kubernetes and the broader container ecosystem. Gazing into the future, one can't help but ponder the next focal point in the ever-evolving vector database landscape. Could it be the rise of Serverless services?\n\n\n\n\n### 10 billion entities in a single collection\n\n\nWhile scalability might not currently steal the spotlight in the AI phenomenon, it certainly plays a pivotal role, far from being a mere sideshow. Milvus vector database can seamlessly scale out to accommodate billions of vector data without breaking a sweat. Take a look at one of our LLM customers, for example. Milvus effortlessly helped this customer store, process, and retrieve an astounding 10 billion data points. But how do you balance the cost and performance when dealing with such a massive volume of data? Rest assured, Mivus has various capabilities to help you address that challenge and elevate your experience. \n\n\n\n\n## Beyond the numbers: the new insights into vector databases \n\n\nBeyond the numerical milestones, 2023 has enriched us with valuable insights. We've delved into the intricacies of the vector database landscape, moving beyond mere statistics to grasp the subtle nuances and evolving dynamics of vector search technology.\n\n\n\n\n### LLM apps are still in the early stages. \n\n\nReflecting on the early days of the mobile internet boom, many developers created simple apps like flashlights or weather forecasts, which eventually were integrated into smartphone operating systems. Last year, most AI Native applications, like AutoGPT, which rapidly hit 100,000 stars on GitHub, didn't deliver practical value but only represented meaningful experiments. For vector database applications, the current use cases may just be the first wave of AI Native transformations, and I eagerly anticipate more killer use cases to emerge.\n\n\n\n\n### Vector databases go toward diversification. \n\n\nSimilar to the evolution of databases into categories like OLTP, OLAP, and NoSQL, vector databases show a clear trend toward diversification. Departing from the conventional focus on online services, offline analysis has gained significant traction. Another notable instance of this shift is the introduction of [GPTCache](https://zilliz.com/blog/building-llm-apps-100x-faster-responses-drastic-cost-reduction-using-gptcache), an open-sourced semantic cache released in 2023. It enhances the efficiency and speed of GPT-based applications by storing and retrieving responses generated by language models.\n\n\nWe are hopeful and excited to witness even more diversified applications and system designs in vector databases in the coming year.\n\n\n\n\n### Vector operations are becoming more complicated. \n\n\nWhile supporting [Approximate Nearest Neighbor (ANN)](https://zilliz.com/glossary/anns) search is a defining feature of vector databases, it doesn't stand alone. The common belief that merely keeping Nearest Neighbour Search is sufficient to classify a database as a vector or AI native database oversimplifies the intricacies of vector operations. Beyond the basic capabilities of hybrid scalar filtering and vector search, databases tailored for AI native applications should support more sophisticated semantic capabilities like NN Filtering, KNN Join, and cluster querying. \n\n\n\n\n### Elastic scalability is essential for AI native applications. \n\n\nThe exponential growth of AI applications, exemplified by ChatGPT amassing over 100 million monthly active users in two months, surpasses any prior business trajectory. Swiftly scaling from 1 million to 1 billion data points becomes paramount once businesses hit their stride in growth. AI application developers benefit from the pay-as-you-go service model set by LLM providers, leading to substantial reductions in operational costs. Similarly, storing data that aligns with this pricing model proves advantageous for developers, allowing them to channel more attention toward core business. \n\n\nUnlike Language Models (LLMs) and various other technological systems, vector databases operate in a stateful manner, demanding persistent data storage for their functionality. Consequently, when selecting vector databases, it is crucial to prioritize elasticity and scalability. This prioritization ensures alignment with the dynamic demands of evolving AI applications, highlighting the need for seamless adaptability to changing workloads.\n\n\n\n\n### Leveraging machine learning in vector databases can yield extraordinary results. \n\n\nIn 2023, our substantial investment in the AI4DB (AI for Database) projects yielded remarkable success. As part of our endeavors, we introduced two pivotal capabilities to [Zilliz Cloud](https://zilliz.com/cloud), the fully managed Milvus solution: 1) AutoIndex, an auto-parameter-tuning index rooted in machine learning, and 2) a data partitioning strategy based on data clustering. Both innovations played a crucial role in significantly enhancing the search performance of Zilliz Cloud.\n\n\n\n\n### Open source vs. closed source\n\n\nClosed-source LLMs like OpenAI's GPT series and Claude currently take the lead, placing the open-source community disadvantaged due to the absence of comparable computational and data resources.\n\n\nHowever, within vector databases, open source will eventually become the favored choice for users. Opting for open source introduces many advantages, including more diverse use cases, expedited iteration, and cultivating a more robust ecosystem. Furthermore, database systems are so intricate that they cannot afford the opacity often associated with LLMs. Users must thoroughly understand the database before choosing the most reasonable approach for its utilization. Moreover, the transparency ingrained in open source empowers users to possess the liberty and the control to customize the database according to their needs.\n\n\n\n\n## Epilogue - And a new beginning!\n\n\nAs 2023 swiftly passes amidst transformative changes, the story of vector databases is just beginning. Our journey with the Milvus vector database is about something other than getting lost in the hype of AIGC. Instead, we focus on meticulously developing our product, identifying and nurturing application use cases that align with our strengths, and unwaveringly serving our users. Our commitment to open source aims to bridge the gap between us and our users, allowing them to sense our dedication and craftsmanship, even from a distance.\n\n\n2023 also saw many AI startups being founded and getting their first funding rounds. It is exciting to see the innovation from these developers, and it reminds me of why I got into VectorDB development in the first place. 2024 will be a year for all these innovative applications to gain real traction, attracting not just funding but real paying customers. Customer revenue will bring different requirements for these developers, as building a fully scalable solution with little to no downtime is paramount. \n\n\nLet's make extraordinary things happen in 2024!\n","title":"Milvus in 2023: An Unprecedented Vector Database Amidst Tech Buzz","metaData":{}},{"id":"efficient-vector-similarity-search-recommender-workflows-using-milvus-nvidia-merlin.md","author":"Burcin Bozkaya","desc":"An introduction to NVIDIA Merlin and Milvus integration in building recommender systems and benchmarking its performance in various scenarios.","tags":["Engineering"],"recommend":true,"canonicalUrl":"https://zilliz.com/blog/efficient-vector-similarity-search-recommender-workflows-using-milvus-nvidia-merlin","date":"2023-12-15T00:00:00.000Z","cover":"https://assets.zilliz.com/nvidia_4921837ca6.png","href":"/blog/efficient-vector-similarity-search-recommender-workflows-using-milvus-nvidia-merlin.md","content":"\n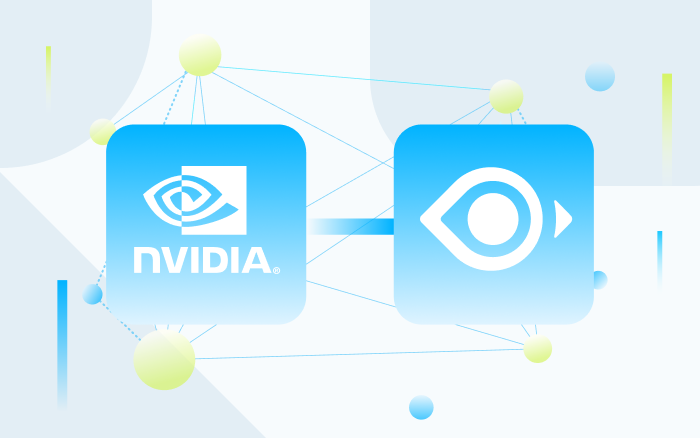\n\n\n*This post was first published on [NVIDIA Merlin’s Medium channel](https://medium.com/nvidia-merlin/efficient-vector-similarity-search-in-recommender-workflows-using-milvus-with-nvidia-merlin-84d568290ee4) and edited and reposted here with permission. It was jointly written by [Burcin Bozkaya](https://medium.com/u/743df9db1666?source=post_page-----84d568290ee4--------------------------------) and [William Hicks](https://medium.com/u/279d4c25a145?source=post_page-----84d568290ee4--------------------------------) from NVIDIA and [Filip Haltmayer](https://medium.com/u/3e8a3c67a8a5?source=post_page-----84d568290ee4--------------------------------) and [Li Liu](https://github.com/liliu-z) from Zilliz.*\n\n## Introduction\n\nModern recommender systems (Recsys) consist of training/inference pipelines involving multiple stages of data ingestion, data preprocessing, model training, and hyperparameter-tuning for retrieval, filtering, ranking, and scoring relevant items. An essential component of a recommender system pipeline is the retrieval or discovery of things that are most relevant to a user, particularly in the presence of large item catalogs. This step typically involves an [approximate nearest neighbor (ANN)](https://zilliz.com/glossary/anns) search over an indexed database of low-dimensional vector representations (i.e., embeddings) of product and user attributes created from deep learning models that train on interactions between users and products/services.\n\n \n\n[NVIDIA Merlin](https://github.com/NVIDIA-Merlin), an open-source framework developed for training end-to-end models to make recommendations at any scale, integrates with an efficient [vector database](https://zilliz.com/learn/what-is-vector-database) index and search framework. One such framework that has gained much recent attention is [Milvus](https://zilliz.com/what-is-milvus), an open-source vector database created by [Zilliz](https://zilliz.com/). It offers fast index and query capabilities. Milvus recently added [GPU acceleration support](https://zilliz.com/blog/getting-started-with-gpu-powered-milvus-unlocking-10x-higher-performance) that uses NVIDIA GPUs to sustain AI workflows. GPU acceleration support is great news because an accelerated vector search library makes fast concurrent queries possible, positively impacting the latency requirements in today’s recommender systems, where developers expect many concurrent requests. Milvus has over 5M docker pulls, ~23k stars on GitHub (as of September 2023), over 5,000 Enterprise customers, and a core component of many applications (see use [cases](https://medium.com/vector-database/tagged/use-cases-of-milvus)).\n\n \n\nThis blog demonstrates how Milvus works with the Merlin Recsys framework at training and inference time. We show how Milvus complements Merlin in the item retrieval stage with a highly efficient top-k vector embedding search and how it can be used with NVIDIA Triton Inference Server (TIS) at inference time (see Figure 1). **Our benchmark results show an impressive 37x to 91x speedup with GPU-accelerated Milvus that uses NVIDIA RAFT with the vector embeddings generated by Merlin Models.** The code we use to show Merlin-Milvus integration and detailed benchmark results, along with the [library](https://github.com/zilliztech/VectorDBBench) that facilitated our benchmark study, are available here.\n\n\n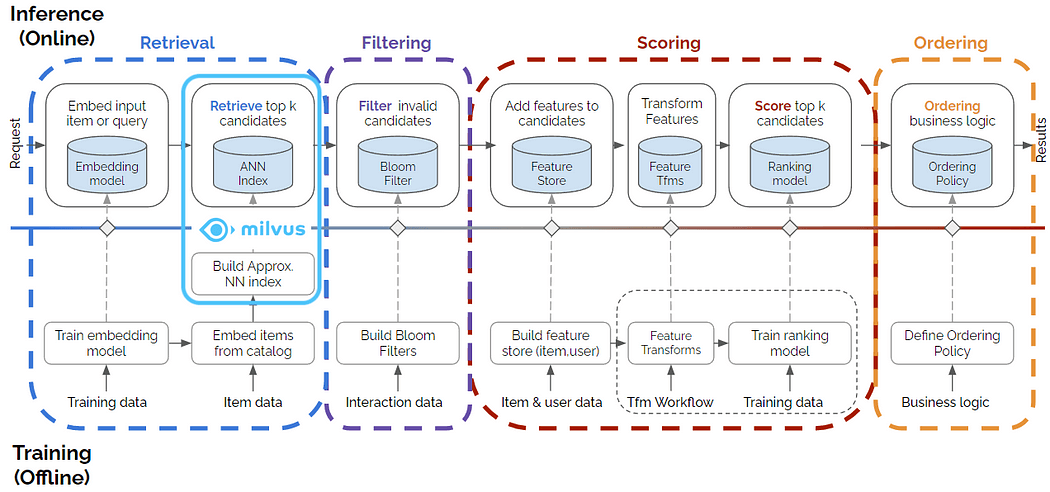\n\n*Figure 1. Multistage recommender system with Milvus framework contributing to the retrieval stage. Source for the original multistage figure: this [blog post](https://medium.com/nvidia-merlin/recommender-systems-not-just-recommender-models-485c161c755e).*\n\n## The challenges facing recommenders\n\nGiven the multistage nature of recommenders and the availability of various components and libraries they integrated, a significant challenge is integrating all components seamlessly in an end-to-end pipeline. We aim to show that integration can be done with less effort in our example notebooks.\n\nAnother challenge of recommender workflows is accelerating certain pipeline parts. While known to play a huge role in training large neural networks, GPUs are only recent additions to vector databases and ANN search. With an increasing size of e-commerce product inventories or streaming media databases and the number of users using these services, CPUs must provide the required performance to serve millions of users in performant Recsys workflows. GPU acceleration in other pipeline parts has become necessary to address this challenge. The solution in this blog addresses this challenge by showing that ANN search is efficient when using GPUs.\n\n## Tech stacks for the solution\n\nLet’s start by first reviewing some of the fundamentals needed to conduct our work.\n\n- NVIDIA [Merlin](https://github.com/NVIDIA-Merlin/Merlin): an open-source library with high-level APIs accelerating recommenders on NVIDIA GPUs.\n \n- [NVTabular](https://github.com/NVIDIA-Merlin/NVTabular): for pre-processing the input tabular data and feature engineering.\n \n- [Merlin Models](https://github.com/NVIDIA-Merlin/models): for training deep learning models, and to learn, in this case, user and item embedding vectors from user interaction data.\n \n- [Merlin Systems](https://github.com/NVIDIA-Merlin/systems): for combining a TensorFlow-based recommendation model with other elements (e.g., feature store, ANN search with Milvus) to be served with TIS.\n \n- [Triton Inference Server](https://github.com/triton-inference-server/server): for the inference stage where a user feature vector is passed, and product recommendations are generated.\n \n- Containerization: all of the above is available via container(s) NVIDIA provides in the [NGC catalog](https://catalog.ngc.nvidia.com/). We used the Merlin TensorFlow 23.06 container available [here](https://catalog.ngc.nvidia.com/orgs/nvidia/teams/merlin/containers/merlin-tensorflow).\n \n- [Milvus 2.3](https://github.com/milvus-io/milvus/releases/tag/v2.3.0): for conducting GPU-accelerated vector indexing and querying.\n \n- [Milvus 2.2.11](https://github.com/milvus-io/milvus/releases): same as above, but for doing it on CPU.\n \n- [Pymilvus SDK](https://zilliz.com/product/integrations/python): for connecting to the Milvus server, creating vector database indexes, and running queries via a Python interface.\n \n- [Feast](https://github.com/feast-dev/feast): for saving and retrieving user and item attributes in an (open source) feature store as part of our end-to-end RecSys pipeline.\n \n\nSeveral underlying libraries and frameworks are also used under the hood. For example, Merlin relies on other NVIDIA libraries, such as cuDF and Dask, both available under [RAPIDS cuDF](https://github.com/rapidsai/cudf). Likewise, Milvus relies on [NVIDIA RAFT](https://github.com/rapidsai/raft) for primitives on GPU acceleration and modified libraries such as [HNSW](https://zilliz.com/learn/hierarchical-navigable-small-worlds-HNSW) and [FAISS](https://zilliz.com/blog/set-up-with-facebook-ai-similarity-search-faiss) for search.\n\n## Understanding vector databases and Milvus\n\n\n[Approximate nearest neighbor (ANN)](https://zilliz.com/glossary/anns) is a functionality that relational databases cannot handle. Relational DBs are designed to handle tabular data with predefined structures and directly comparable values. Relational database indexes rely on this to compare data and create structures that take advantage of knowing if each value is less than or greater than the other. Embedding vectors cannot be directly compared to one another in this fashion, as we need to know what each value in the vector represents. They cannot say if one vector is necessarily less than the other. The only thing that we can do is calculate the distance between the two vectors. If the distance between two vectors is small, we can assume that the features they represent are similar, and if it is large, we can assume that the data they represent are more different. However, these efficient indexes come at a cost; computing the distance between two vectors is computationally expensive, and vector indexes are not readily adaptable and sometimes not modifiable. Due to these two limitations, integrating these indexes is more complex in relational databases, which is why [purpose-built vector databases](https://zilliz.com/blog/what-is-a-real-vector-database) are needed.\n\n[Milvus](https://zilliz.com/what-is-milvus) was created to solve the problems that relational databases hit with vectors and was designed from the ground up to handle these embedding vectors and their indexes at a large scale. To fulfill the cloud-native badge, Milvus separates computing and storage and different computing tasks — querying, data wrangling, and indexing. Users can scale each database part to handle other use cases, whether data insert-heavy or search-heavy. If there is a large influx of insertion requests, the user can temporarily scale the index nodes horizontally and vertically to handle the ingestion. Likewise, if no data is being ingested, but there are many searches, the user can reduce the index nodes and instead scale up the query nodes for more throughput. This system design (see Figure 2) required us to think in a parallel computing mindset, resulting in a compute-optimized system with many doors open for further optimizations.\n\n\n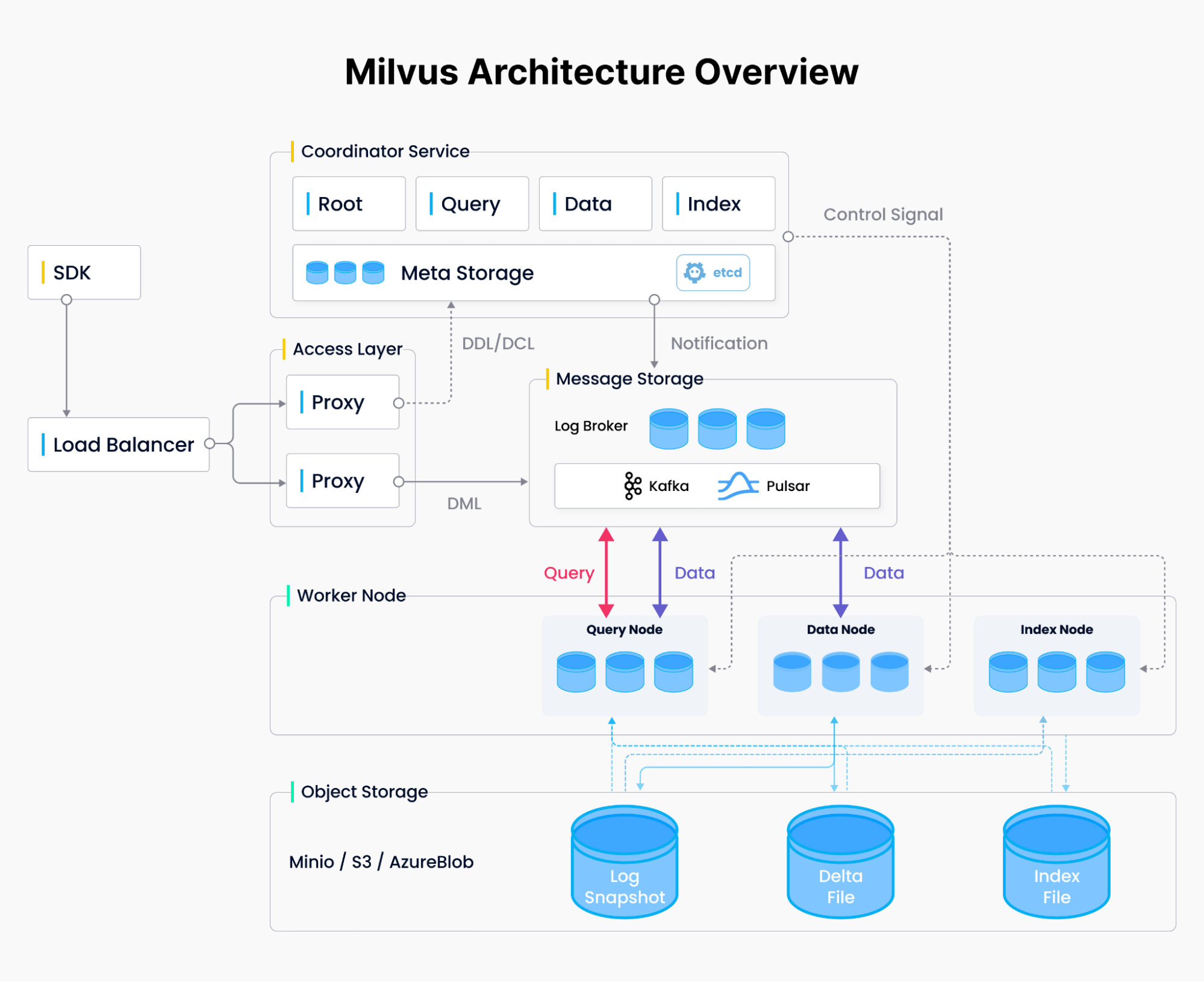\n\n*Figure 2. Milvus system design*\n\nMilvus also uses many state-of-the-art indexing libraries to give users as much customization for their system as possible. It improves them by adding the ability to handle CRUD operations, streamed data, and filtering. Later on, we will discuss how these indexes differ and what the pros and cons of each are.\n\n## Example solution: integration of Milvus and Merlin\n\nThe example solution we present here demonstrates the integration of Milvus with Merlin at the item retrieval stage (when the k most relevant items are retrieved through an ANN search). We use a real-life dataset from a [RecSys challenge](https://www.kaggle.com/datasets/chadgostopp/recsys-challenge-2015), described below. We train a Two-Tower deep learning model that learns vector embeddings for users and items. This section also provides the blueprint of our benchmarking work, including the metrics we collect and the range of parameters we use.\n\nOur approach involves:\n\n- Data ingestion and preprocessing\n \n- Two-Tower deep learning model training\n \n- Milvus index building\n \n- Milvus similarity search\n \n\nWe briefly describe each step and refer the reader to our [notebooks](https://github.com/bbozkaya/merlin-milvus/tree/main/notebooks) for details.\n\n### Dataset\n\nYOOCHOOSE GmbH provides the dataset we use in this integration and benchmark study for the [RecSys 2015 challenge](https://www.kaggle.com/datasets/chadgostopp/recsys-challenge-2015) and is available on Kaggle. It contains user click/buy events from a European online retailer with attributes such as a session ID, timestamp, item ID associated with click/buy, and item category, available in the file yoochoose-clicks.dat. The sessions are independent, and there is no hint of returning users, so we treat each session as belonging to a distinct user. The dataset has 9,249,729 unique sessions (users) and 52,739 unique items.\n\n### Data ingestion and preprocessing\n\nThe tool we use for data preprocessing is [NVTabular](https://github.com/NVIDIA-Merlin/NVTabular), a GPU-accelerated, highly scalable feature engineering and preprocessing component of Merlin. We use NVTabular to read data into GPU memory, rearrange features as necessary, export to parquet files, and create a train-validation split for training. This results in 7,305,761 unique users and 49,008 unique items to train on. We also categorize each column and its values into integer values. The dataset is now ready for training with the Two-Tower model.\n\n### Model training\n\nWe use the [Two-Tower](https://github.com/NVIDIA-Merlin/models/blob/main/examples/05-Retrieval-Model.ipynb) deep learning model to train and generate user and item embeddings, later used in vector indexing and querying. After training the model, we can extract the learned user and item embeddings.\n\n \n\nThe following two steps are optional: a [DLRM](https://arxiv.org/abs/1906.00091) model trained to rank the retrieved items for recommendation and a feature store used (in this case, [Feast](https://github.com/feast-dev/feast)) to store and retrieve user and item features. We include them for the completeness of the multi-stage workflow.\n\n \n\nFinally, we export the user and item embeddings to parquet files, which can later be reloaded to create a Milvus vector index.\n\n### Building and querying the Milvus index\n\nMilvus facilitates vector indexing and similarity search via a “server” launched on the inference machine. In our notebook #2, we set this up by pip-installing the Milvus server and Pymilvus, then starting the server with its default listening port. Next, we demonstrate building a simple index (IVF_FLAT) and querying against it using the functions `setup_milvus` and `query_milvus`, respectively.\n\n## Benchmarking\n\nWe have designed two benchmarks to demonstrate the case for using a fast and efficient vector indexing/search library such as Milvus.\n\n1. Using Milvus to build vector indexes with the two sets of embeddings we generated: 1) user embeddings for 7.3M unique users, split as 85% train set (for indexing) and 15% test set (for querying), and 2) item embeddings for 49K products (with a 50–50 train-test split). This benchmark is done independently for each vector dataset, and results are reported separately.\n \n2. Using Milvus to build a vector index for the 49K item embeddings dataset and querying the 7.3M unique users against this index for similarity search.\n \n\nIn these benchmarks, we used IVFPQ and HNSW indexing algorithms executed on GPU and CPU, along with various combinations of parameters. Details are available [here](https://github.com/bbozkaya/merlin-milvus/tree/main/results).\n\nThe search quality-throughput tradeoff is an important performance consideration, especially in a production environment. Milvus allows complete control over indexing parameters to explore this tradeoff for a given use case to achieve better search results with ground truth. This may mean increased computational cost in the form of reduced throughput rate or queries per second (QPS). We measure the quality of the ANN search with a recall metric and provide QPS-recall curves that demonstrate the tradeoff. One can then decide on an acceptable level of search quality given the compute resources or latency/throughput requirements of the business case.\n\nAlso, note the query batch size (nq) used in our benchmarks. This is useful in workflows where multiple simultaneous requests are sent to inference (e.g., offline recommendations requested and sent to a list of email recipients or online recommendations created by pooling concurrent requests arriving and processing them all at once). Depending on the use case, TIS can also help process these requests in batches.\n\n### Results\n\nWe now report the results for the three sets of benchmarks on both CPU and GPU, using HNSW (CPU only) and IVF_PQ (CPU and GPU) index types implemented by Milvus.\n\n#### Items vs. Items vector similarity search\n\nWith this smallest dataset, each run for a given parameter combination takes 50% of the item vectors as query vectors and queries the top 100 similar vectors from the rest. HNSW and IVF_PQ produce high recall with the parameter settings tested, in the range 0.958–1.0 and 0.665–0.997, respectively. This result suggests that HNSW performs better w.r.t. recall, but IVF_PQ with small nlist settings produces highly comparable recall. We should also note that the recall values can vary greatly depending on the indexing and querying parameters. The values we report have been obtained after preliminary experimentation with general parameter ranges and zooming further into a select subset.\n\nThe total time to execute all queries on CPU with HNSW for a given parameter combination ranges between 5.22 and 5.33 sec.s (faster as m gets larger, relatively unchanged with ef) and with IVF_PQ between 13.67 and 14.67 sec.s (slower as nlist and nprobe get larger). GPU acceleration does have a noticeable effect, as seen in Figure 3.\n\nFigure 3 shows the recall-throughput trade-off over all runs completed on CPU and GPU with this small dataset using IVF_PQ. We find that GPU provides a speedup of 4x to 15x across all parameter combinations tested (larger speedup as nprobe gets larger). This is calculated by taking the ratio of QPS from GPU over QPS from CPU runs for each parameter combination. Overall, this set presents a little challenge for CPU or GPU and shows prospects for further speedup with the larger datasets, as discussed below.\n\n\n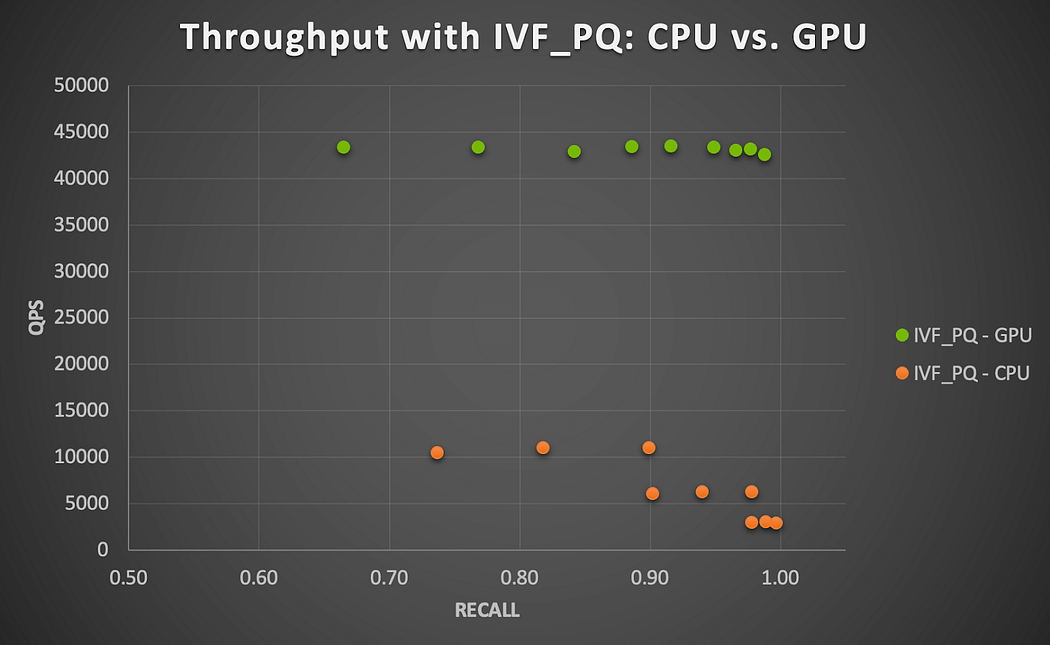\n\n*Figure 3. GPU speedup with Milvus IVF_PQ algorithm running on NVIDIA A100 GPU (item-item similarity search)*\n\n\n#### Users vs. Users vector similarity search\n\nWith the much larger second dataset (7.3M users), we set aside 85% (~6.2M) of the vectors as “train” (the set of vectors to be indexed), and the remaining 15% (~1.1M) “test” or query vector set. HNSW and IVF_PQ perform exceptionally well in this case, with recall values of 0.884–1.0 and 0.922–0.999, respectively. They are, however, computationally much more demanding, especially with IVF_PQ on the CPU. The total time to execute all queries on CPU with HNSW ranges from 279.89 to 295.56 sec.s and with IVF_PQ from 3082.67 to 10932.33 sec.s. Note that these query times are cumulative for 1.1M vectors queried, so one can say that a single query against the index is still very fast.\n\nHowever, CPU-based querying may not be viable if the inference server expects many thousands of concurrent requests to run queries against an inventory of millions of items.\n\nThe A100 GPU delivers a blazing speedup of 37x to 91x (averaging 76.1x) across all parameter combinations with IVF_PQ in terms of throughput (QPS), shown in Figure 4. This is consistent with what we observed with the small dataset, which suggests the GPU performance scales reasonably well using Milvus with millions of embedding vectors.\n\n\n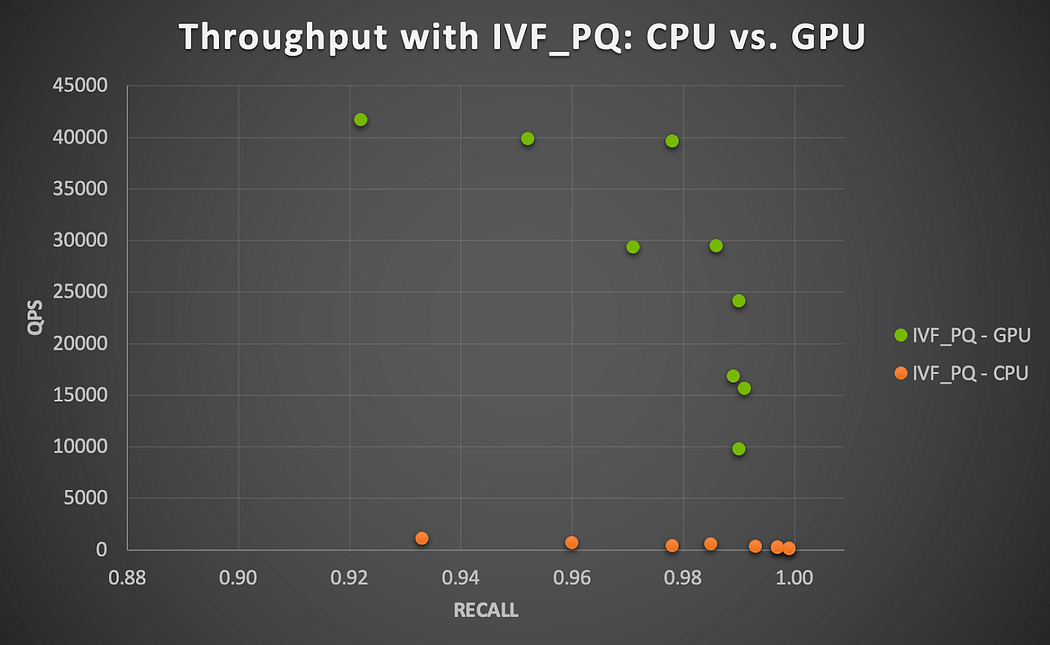\n\n*Figure 4. GPU speedup with Milvus IVF_PQ algorithm running on NVIDIA A100 GPU (user-user similarity search)*\n \n\nThe following detailed Figure 5 shows the recall-QPS tradeoff for all parameter combinations tested on CPU and GPU with IVF_PQ. Each point set (top for GPU, bottom for CPU) on this chart depicts the tradeoff faced when changing vector indexing/query parameters towards achieving higher recall at the expense of lower throughput. Note the considerable loss of QPS in the GPU case as one tries to achieve higher recall levels.\n\n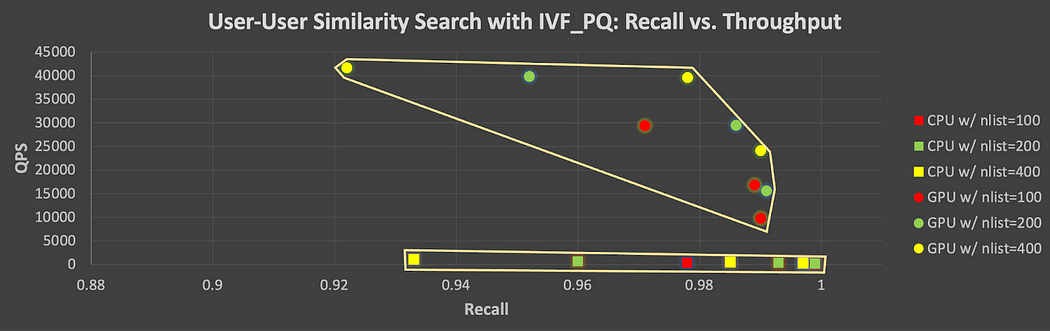\n\n*Figure 5. Recall-Throughput tradeoff for all parameter combinations tested on CPU and GPU with IVF_PQ (users vs. users)*\n\n#### Users vs. Items vector similarity search\n\nFinally, we consider another realistic use case where user vectors are queried against item vectors (as demonstrated in Notebook 01 above). In this case, 49K item vectors are indexed, and 7.3M user vectors are each queried for the top 100 most similar items.\n\nThis is where things get interesting because querying 7.3M in batches of 1000 against an index of 49K items appears time-consuming on the CPU for both HNSW and IVF_PQ. GPU seems to handle this case better (see Figure 6). The highest accuracy levels by IVF_PQ on CPU when nlist = 100 are computed in about 86 minutes on average but vary significantly as the nprobe value increases (51 min. when nprobe = 5 vs. 128 min. when nprobe = 20). The NVIDIA A100 GPU speeds up the performance considerably by a factor 4x to 17x (higher speedups as nprobe gets larger). Remember that the IVF_PQ algorithm, through its quantization technique, also reduces memory footprint and provides a computationally viable ANN search solution combined with the GPU acceleration.\n\n \n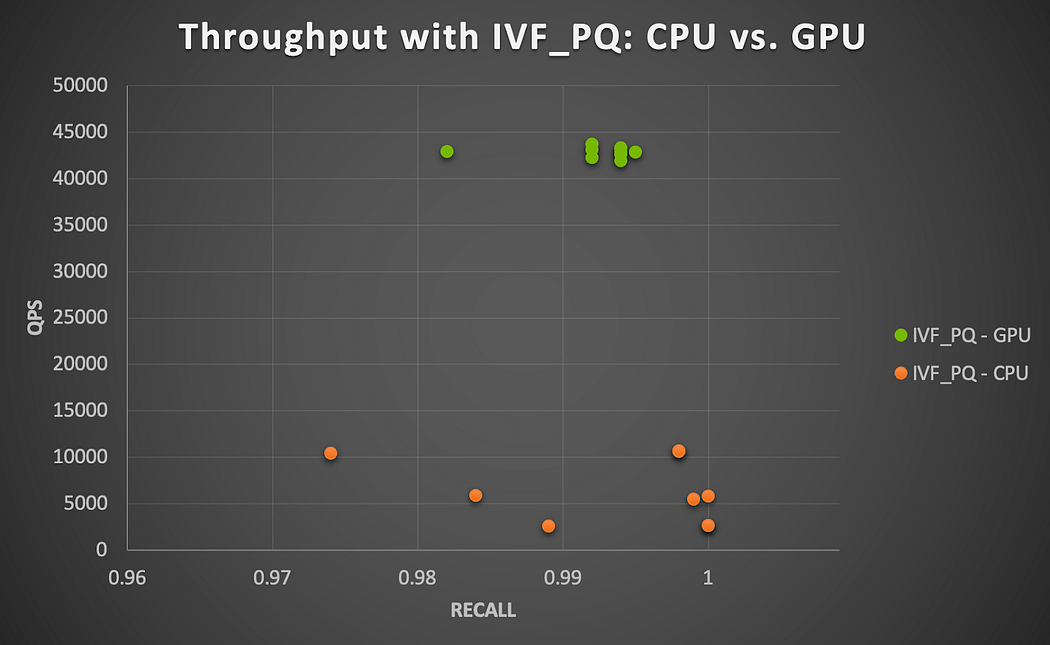 \n\n*Figure 6. GPU speedup with Milvus IVF_PQ algorithm running on NVIDIA A100 GPU (user-item similarity search)*\n\nSimilar to Figure 5, the recall-throughput trade-off is shown in Figure 7 for all parameter combinations tested with IVF_PQ. Here, one can still see how one may need to slightly give up some accuracy on ANN search in favor of increased throughput, though the differences are much less noticeable, especially in the case of GPU runs. This suggests that one can expect relatively consistently high levels of computational performance with the GPU while still achieving high recall.\n\n\n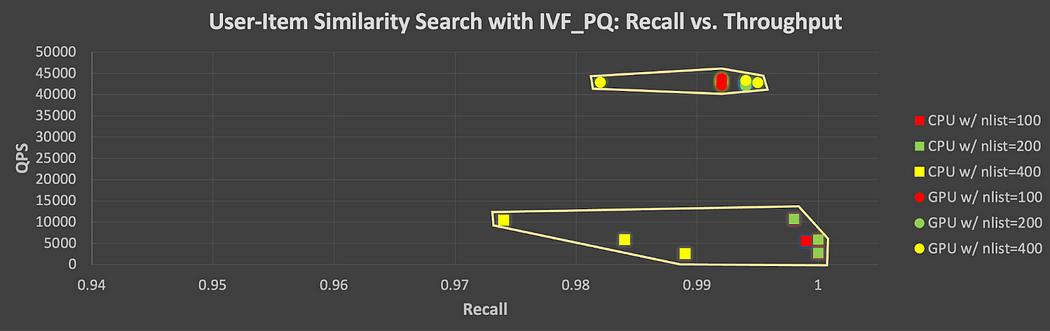\n\n*Figure 7. Recall-Throughput tradeoff for all parameter combinations tested on CPU and GPU with IVF_PQ (users vs. items)*\n\n## Conclusion\n\nWe'd happily share a few concluding remarks if you've made it this far. We want to remind you that modern Recsys' complexity and multi-stage nature necessitate performance and efficiency at every step. Hopefully, this blog has given you compelling reasons to consider using two critical features in your RecSys pipelines:\n\n- NVIDIA Merlin's Merlin Systems library allows you to easily plug in [Milvus](https://github.com/milvus-io/milvus/tree/2.3.0), an efficient GPU-accelerated vector search engine.\n \n- Use GPU to accelerate computations for vector database indexing, and ANN search with technology such as [RAPIDS RAFT](https://github.com/rapidsai/raft).\n\n\n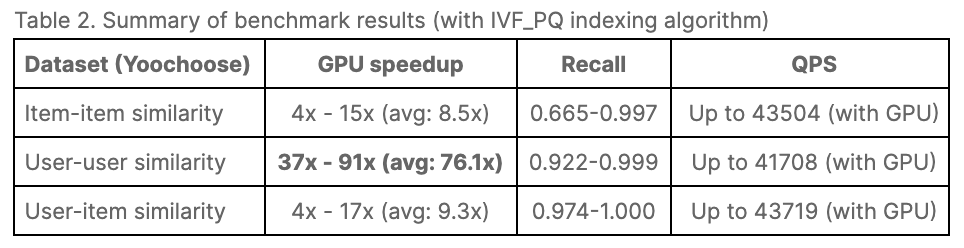\n\n\nThese findings suggest that the Merlin-Milvus integration presented is highly performant and much less complex than other options for training and inference. Also, both frameworks are actively developed, and many new features (e.g., new GPU-accelerated vector database indexes by Milvus) are added in every release. The fact that vector similarity search is a crucial component in various workflows, such as computer vision, large language modeling, and recommender systems, makes this effort all the more worthwhile.\n\nIn closing, we would like to thank all those from Zilliz/Milvus and Merlin and the RAFT teams who contributed to the effort in producing this work and the blog post. Looking forward to hearing from you, should you have a chance to implement Merlin and Milvus in your recsys or other workflows.","title":"Efficient Vector Similarity Search in Recommender Workflows Using Milvus with NVIDIA Merlin","metaData":{}},{"id":"how-to-get-the-right-vector-embeddings.md","author":"Yujian Tang","desc":"A comprehensive introduction to vector embeddings and how to generate them with popular open-source models.","tags":["Engineering"],"recommend":true,"canonicalUrl":"https://zilliz.com/blog/how-to-get-the-right-vector-embeddings","date":"2023-12-08T00:00:00.000Z","cover":"https://assets.zilliz.com/How_to_Get_the_Right_Vector_Embedding_d9ebcacbbb.png","href":"/blog/how-to-get-the-right-vector-embeddings.md","content":"\n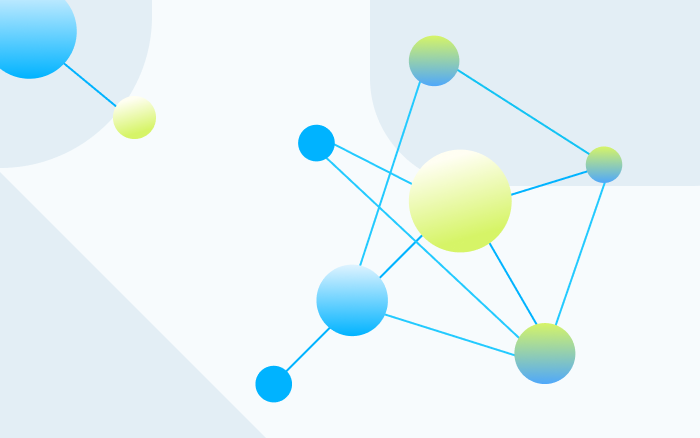\n\n\n*This article was originally published in [The New Stack](https://thenewstack.io/how-to-get-the-right-vector-embeddings/) and is reposted here with permission.*\n\n**A comprehensive introduction to vector embeddings and how to generate them with popular open source models.**\n\n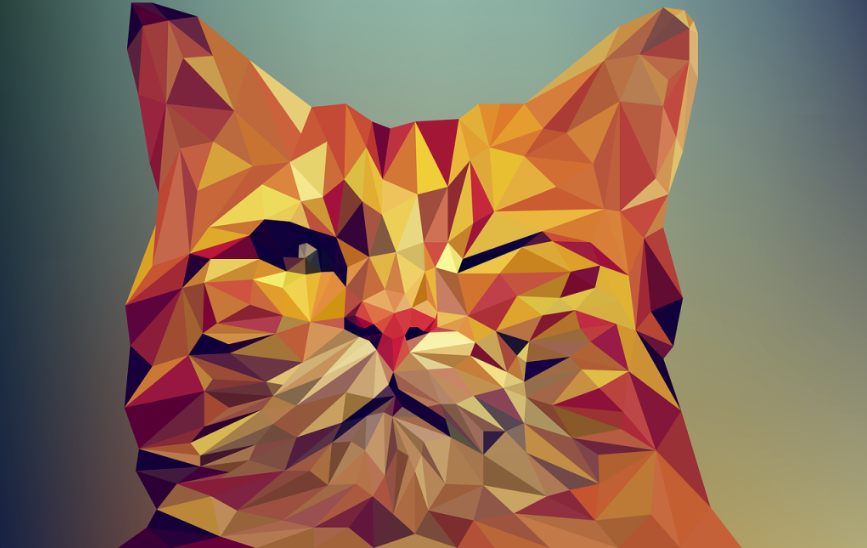\n\n\nVector embeddings are critical when working with [semantic similarity](https://zilliz.com/blog/vector-similarity-search). However, a vector is simply a series of numbers; a vector embedding is a series of numbers representing input data. Using vector embeddings, we can structure [unstructured data](https://zilliz.com/blog/introduction-to-unstructured-data) or work with any type of data by converting it into a series of numbers. This approach allows us to perform mathematical operations on the input data, rather than relying on qualitative comparisons.\n\nVector embeddings are influential for many tasks, particularly for [semantic search](https://zilliz.com/glossary/semantic-search). However, it is crucial to obtain the appropriate vector embeddings before using them. For instance, if you use an image model to vectorize text, or vice versa, you will probably get poor results.\n\nIn this post, we will learn what vector embeddings mean, how to generate the right vector embeddings for your applications using different models and how to make the best use of vector embeddings with vector databases like [Milvus](https://milvus.io/) and [Zilliz Cloud](https://zilliz.com/).\n\n## How are vector embeddings created?\n\n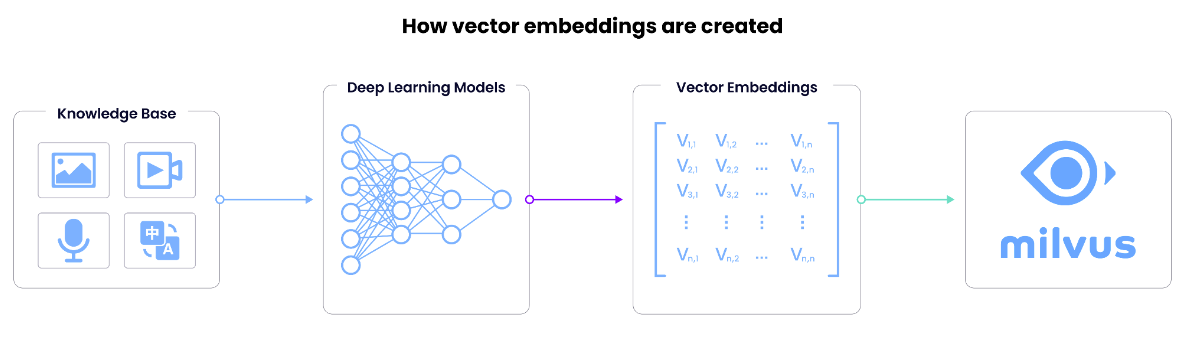\n\n\nNow that we understand the importance of vector embeddings, let’s learn how they work. A vector embedding is the internal representation of input data in a deep learning model, also known as embedding models or a deep neural network. So, how do we extract this information?\n\nWe obtain vectors by removing the last layer and taking the output from the second-to-last layer. The last layer of a neural network usually outputs the model’s prediction, so we take the output of the second-to-last layer. The vector embedding is the data fed to a neural network’s predictive layer.\n\nThe dimensionality of a vector embedding is equivalent to the size of the second-to-last layer in the model and, thus, interchangeable with the vector’s size or length. Common vector dimensionalities include 384 (generated by Sentence Transformers Mini-LM), 768 (by Sentence Transformers MPNet), 1,536 (by OpenAI) and 2,048 (by ResNet-50).\n\n\n## What does a vector embedding mean?\n\nSomeone once asked me about the meaning of each dimension in a vector embedding. The short answer is nothing. A single dimension in a vector embedding does not mean anything, as it is too abstract to determine its meaning. However, when we take all dimensions together, they provide the semantic meaning of the input data.\n\nThe dimensions of the vector are high-level, abstract representations of different attributes. The represented attributes depend on the training data and the model itself. Text and image models generate different embeddings because they’re trained for fundamentally different data types. Even different text models generate different embeddings. Sometimes they differ in size; other times, they differ in the attributes they represent. For instance, a model trained on legal data will learn different things than one trained on health-care data. I explored this topic in my post [comparing vector embeddings](https://zilliz.com/blog/comparing-different-vector-embeddings).\n\n## Generate the right vector embeddings\n\nHow do you obtain the proper vector embeddings? It all starts with identifying the type of data you wish to embed. This section covers embedding five different types of data: images, text, audio, videos and multimodal data. All models we introduce here are open source and come from Hugging Face or PyTorch.\n\n### Image embeddings\n\nImage recognition took off in 2012 after AlexNet hit the scene. Since then, the field of computer vision has witnessed numerous advancements. The latest notable image recognition model is ResNet-50, a 50-layer deep residual network based on the former ResNet-34 architecture.\n\nResidual neural networks (ResNet) solve the vanishing gradient problem in deep convolutional neural networks using shortcut connections. These connections allow the output from earlier layers to go to later layers directly without passing through all the intermediate layers, thus avoiding the vanishing gradient problem. This design makes ResNet less complex than VGGNet (Visual Geometry Group), a previously top-performing convolutional neural network.\n\nI recommend two ResNet-50 implementations as examples: [ResNet 50 on Hugging Face](https://huggingface.co/microsoft/resnet-50) and [ResNet 50 on PyTorch Hub](https://pytorch.org/vision/main/models/generated/torchvision.models.resnet50.html). While the networks are the same, the process of obtaining embeddings differs.\n\nThe code sample below demonstrates how to use PyTorch to obtain vector embeddings. First, we load the model from PyTorch Hub. Next, we remove the last layer and call `.eval()` to instruct the model to behave like it’s running for inference. Then, the `embed` function generates the vector embedding.\n\n\n```\n# Load the embedding model with the last layer removed\nmodel = torch.hub.load('pytorch/vision:v0.10.0', 'resnet50', pretrained=True) model = torch.nn.Sequential(*(list(model.children())[:-1]))\nmodel.eval()\n\n\ndef embed(data):\nwith torch.no_grad():\noutput = model(torch.stack(data[0])).squeeze()\nreturn output\n```\n\n\nHuggingFace uses a slightly different setup. The code below demonstrates how to obtain a vector embedding from Hugging Face. First, we need a feature extractor and model from the `transformers` library. We will use the feature extractor to get inputs for the model and use the model to obtain outputs and extract the last hidden state.\n\n\n```\n# Load model directly\nfrom transformers import AutoFeatureExtractor, AutoModelForImageClassification\n\n\nextractor = AutoFeatureExtractor.from_pretrained(\"microsoft/resnet-50\")\nmodel = AutoModelForImageClassification.from_pretrained(\"microsoft/resnet-50\")\n\n\nfrom PIL import Image\n\n\nimage = Image.open(\"\u003cimage path\u003e\")\n# image = Resize(size=(256, 256))(image)\n\n\ninputs = extractor(images=image, return_tensors=\"pt\")\n# print(inputs)\n\n\noutputs = model(**inputs)\nvector_embeddings = outputs[1][-1].squeeze()\n```\n\n\n### Text embeddings\n\nEngineers and researchers have been experimenting with natural language and AI since the invention of AI. Some of the earliest experiments include:\n\n- ELIZA, the first AI therapist chatbot.\n- John Searle’s Chinese Room, a thought experiment that examines whether the ability to translate between Chinese and English requires an understanding of the language.\n- Rule-based translations between English and Russian.\n\nAI’s operation on natural language has evolved significantly from its rule-based embeddings. Starting with primary neural networks, we added recurrence relations through RNNs to keep track of steps in time. From there, we used transformers to solve the sequence transduction problem.\n\nTransformers consist of an encoder, which encodes an input into a matrix representing the state, an attention matrix and a decoder. The decoder decodes the state and attention matrix to predict the correct next token to finish the output sequence. GPT-3, the most popular language model to date, comprises strict decoders. They encode the input and predict the right next token(s).\n\nHere are two models from the `sentence-transformers` library by Hugging Face that you can use in addition to OpenAI’s embeddings:\n\n- [MiniLM-L6-v2](https://huggingface.co/sentence-transformers/all-MiniLM-L6-v2): a 384-dimensional model\n- [MPNet-Base-V2](https://huggingface.co/sentence-transformers/all-mpnet-base-v2): a 768-dimensional model\n\nYou can access embeddings from both models in the same way.\n\n\n```\nfrom sentence_transformers import SentenceTransformer\n\n\nmodel = SentenceTransformer(\"\u003cmodel-name\u003e\")\nvector_embeddings = model.encode(“\u003cinput\u003e”)\n```\n\n\n### Multimodal embeddings\n\nMultimodal models are less well-developed than image or text models. They often relate images to text.\n\nThe most useful open source example is [CLIP VIT](https://huggingface.co/openai/clip-vit-large-patch14), an image-to-text model. You can access CLIP VIT’s embeddings in the same way as you would an image model, as shown in the code below.\n\n\n```\n# Load model directly\nfrom transformers import AutoProcessor, AutoModelForZeroShotImageClassification\n\n\nprocessor = AutoProcessor.from_pretrained(\"openai/clip-vit-large-patch14\")\nmodel = AutoModelForZeroShotImageClassification.from_pretrained(\"openai/clip-vit-large-patch14\")\nfrom PIL import Image\n\n\nimage = Image.open(\"\u003cimage path\u003e\")\n# image = Resize(size=(256, 256))(image)\n\n\ninputs = extractor(images=image, return_tensors=\"pt\")\n# print(inputs)\n\n\noutputs = model(**inputs)\nvector_embeddings = outputs[1][-1].squeeze()\n```\n\n\n### Audio embeddings\n\nAI for audio has received less attention than AI for text or images. The most common use case for audio is speech-to-text for industries such as call centers, medical technology and accessibility. One popular open source model for speech-to-text is [Whisper from OpenAI](https://huggingface.co/openai/whisper-large-v2). The code below shows how to obtain vector embeddings from the speech-to-text model. \n\n\n```\nimport torch\nfrom transformers import AutoFeatureExtractor, WhisperModel\nfrom datasets import load_dataset\n\n\nmodel = WhisperModel.from_pretrained(\"openai/whisper-base\")\nfeature_extractor = AutoFeatureExtractor.from_pretrained(\"openai/whisper-base\")\nds = load_dataset(\"hf-internal-testing/librispeech_asr_dummy\", \"clean\", split=\"validation\")\ninputs = feature_extractor(ds[0][\"audio\"][\"array\"], return_tensors=\"pt\")\ninput_features = inputs.input_features\ndecoder_input_ids = torch.tensor([[1, 1]]) * model.config.decoder_start_token_id\nvector_embedding = model(input_features, decoder_input_ids=decoder_input_ids).last_hidden_state\n```\n\n\n### Video embeddings\n\nVideo embeddings are more complex than audio or image embeddings. A multimodal approach is necessary when working with videos, as they include synchronized audio and images. One popular video model is the [multimodal perceiver](https://huggingface.co/deepmind/multimodal-perceiver) from DeepMind. This [notebook tutorial](https://github.com/NielsRogge/Transformers-Tutorials/blob/master/Perceiver/Perceiver_for_Multimodal_Autoencoding.ipynb) shows how to use the model to classify a video.\n\nTo get the embeddings of the input, use `outputs[1][-1].squeeze()` from the code shown in the notebook instead of deleting the outputs. I highlight this code snippet in the `autoencode` function.\n\n\n```\ndef autoencode_video(images, audio):\n # only create entire video once as inputs\n inputs = {'image': torch.from_numpy(np.moveaxis(images, -1, 2)).float().to(device),\n 'audio': torch.from_numpy(audio).to(device),\n 'label': torch.zeros((images.shape[0], 700)).to(device)}\n nchunks = 128\n reconstruction = {}\n for chunk_idx in tqdm(range(nchunks)):\n image_chunk_size = np.prod(images.shape[1:-1]) // nchunks\n audio_chunk_size = audio.shape[1] // SAMPLES_PER_PATCH // nchunks\n subsampling = {\n 'image': torch.arange(\n image_chunk_size * chunk_idx, image_chunk_size * (chunk_idx + 1)),\n 'audio': torch.arange(\n audio_chunk_size * chunk_idx, audio_chunk_size * (chunk_idx + 1)),\n 'label': None,\n }\n # forward pass\n with torch.no_grad():\n outputs = model(inputs=inputs, subsampled_output_points=subsampling)\n\n\n output = {k:v.cpu() for k,v in outputs.logits.items()}\n reconstruction['label'] = output['label']\n if 'image' not in reconstruction:\n reconstruction['image'] = output['image']\n reconstruction['audio'] = output['audio']\n else:\n reconstruction['image'] = torch.cat(\n [reconstruction['image'], output['image']], dim=1)\n reconstruction['audio'] = torch.cat(\n [reconstruction['audio'], output['audio']], dim=1)\n vector_embeddings = outputs[1][-1].squeeze()\n# finally, reshape image and audio modalities back to original shape\n reconstruction['image'] = torch.reshape(reconstruction['image'], images.shape)\n reconstruction['audio'] = torch.reshape(reconstruction['audio'], audio.shape)\n return reconstruction\n\n\n return None\n```\n\n\n## Storing, indexing, and searching vector embeddings with vector databases\n\nNow that we understand what vector embeddings are and how to generate them using various powerful embedding models, the next question is how to store and take advantage of them. Vector databases are the answer.\n\nVector databases like [Milvus](https://zilliz.com/what-is-milvus) and [Zilliz Cloud](https://zilliz.com/cloud) are purposely built for storing, indexing, and searching across massive datasets of unstructured data through vector embeddings. They are also one of the most critical infrastructures for various AI stacks.\n\nVector databases usually use the [Approximate Nearest Neighbor (ANN)](https://zilliz.com/glossary/anns) algorithm to calculate the spatial distance between the query vector and vectors stored in the database. The closer the two vectors are located, the more relevant they are. Then the algorithm finds the top k nearest neighbors and delivers them to the user.\n\nVector databases are popular in use cases such as [LLM retrieval augmented generation](https://zilliz.com/use-cases/llm-retrieval-augmented-generation) (RAG), question and answer systems, recommender systems, semantic searches, and image, video and audio similarity searches.\n\nTo learn more about vector embeddings, unstructured data, and vector databases, consider starting with the [Vector Database 101](https://zilliz.com/blog?tag=39\u0026page=1) series.\n\n## Summary\n\nVectors are a powerful tool for working with unstructured data. Using vectors, we can mathematically compare different pieces of unstructured data based on semantic similarity. Choosing the right vector-embedding model is critical for building a vector search engine for any application.\n\nIn this post, we learned that vector embeddings are the internal representation of input data in a neural network. As a result, they depend highly on the network architecture and the data used to train the model. Different data types (such as images, text, and audio) require specific models. Fortunately, many pretrained open source models are available for use. In this post, we covered models for the five most common types of data: images, text, multimodal, audio, and video. In addition, if you want to make the best use of vector embeddings, vector databases are the most popular tool.","title":"How to Get the Right Vector Embeddings","metaData":{}},{"id":"how-to-migrate-data-to-milvus-seamlessly.md","author":"Wenhui Zhang","desc":"A comprehensive guide on migrating your data from Elasticsearch, FAISS, and older Milvus 1.x to Milvus 2.x versions.","tags":["Engineering"],"recommend":true,"canonicalUrl":"https://zilliz.com/blog/how-to-migrate-data-to-milvus-seamlessly-comprehensive-guide","date":"2023-12-01T00:00:00.000Z","cover":"https://assets.zilliz.com/How_to_Migrate_Your_Data_to_Milvus_with_Ease_485dcb8b22.png","href":"/blog/how-to-migrate-data-to-milvus-seamlessly.md","content":"\n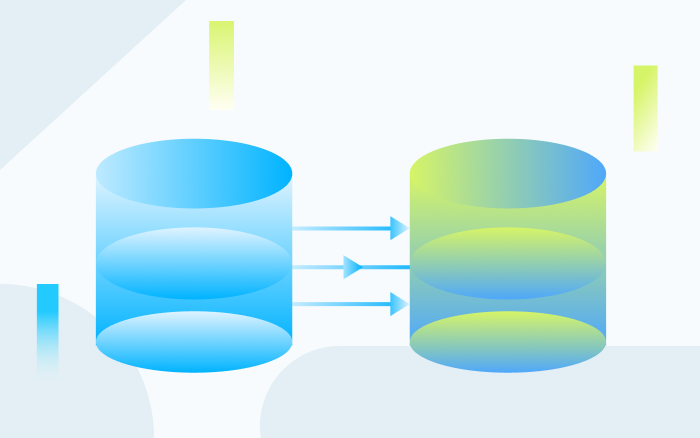\n\n\n[Milvus](https://milvus.io/) is a robust open-source vector database for [similarity search](https://zilliz.com/learn/vector-similarity-search) that can store, process, and retrieve billions and even trillions of vector data with minimal latency. It is also highly scalable, reliable, cloud-native, and feature-rich. [The newest release of Milvus](https://milvus.io/blog/unveiling-milvus-2-3-milestone-release-offering-support-for-gpu-arm64-cdc-and-other-features.md) introduces even more exciting features and improvements, including [GPU support](https://zilliz.com/blog/getting-started-with-gpu-powered-milvus-unlocking-10x-higher-performance) for over 10x faster performance and MMap for greater storage capacity on a single machine.\n\nAs of September 2023, Milvus has earned almost 23,000 stars on GitHub and has tens of thousands of users from diverse industries with varying needs. It is becoming even more popular as Generative AI technology like [ChatGPT](https://zilliz.com/learn/ChatGPT-Vector-Database-Prompt-as-code) becomes more prevalent. It is an essential component of various AI stacks, especially the [retrieval augmented generation](https://zilliz.com/use-cases/llm-retrieval-augmented-generation) framework, which addresses the hallucination problem of large language models.\n\nTo meet the growing demand from new users who want to migrate to Milvus and existing users who wish to upgrade to the latest Milvus versions, we developed [Milvus Migration](https://github.com/zilliztech/milvus-migration). In this blog, we'll explore the features of Milvus Migration and guide you through quickly transitioning your data to Milvus from Milvus 1.x, [FAISS](https://zilliz.com/blog/set-up-with-facebook-ai-similarity-search-faiss), and [Elasticsearch 7.0](https://zilliz.com/comparison/elastic-vs-milvus) and beyond.\n\n## Milvus Migration, a powerful data migration tool\n\n[Milvus Migration](https://github.com/zilliztech/milvus-migration) is a data migration tool written in Go. It enables users to move their data seamlessly from older versions of Milvus (1.x), FAISS, and Elasticsearch 7.0 and beyond to Milvus 2.x versions.\n\nThe diagram below demonstrates how we built Milvus Migration and how it works.\n\n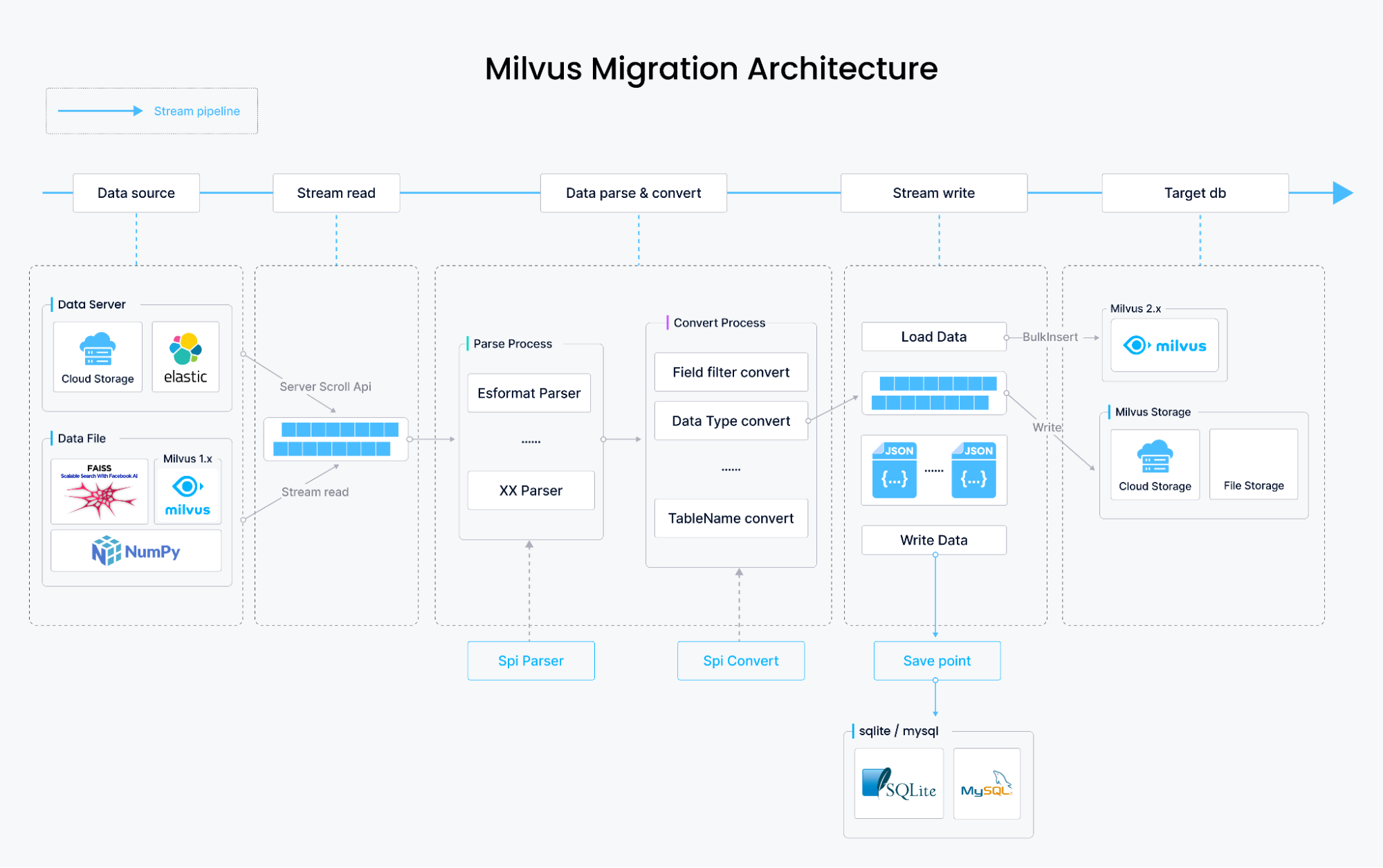\n\n\n### How Milvus Migration migrates data\n\n#### From Milvus 1.x and FAISS to Milvus 2.x\n\nThe data migration from Milvus 1.x and FAISS involves parsing the content of the original data files, transforming them into the data storage format of Milvus 2.x, and writing the data using Milvus SDK's `bulkInsert`. This entire process is stream-based, theoretically limited only by disk space, and stores data files on your local disk, S3, OSS, GCP, or Minio.\n\n#### From Elasticsearch to Milvus 2.x\n\nIn the Elasticsearch data migration, data retrieval is different. Data is not obtained from files but sequentially fetched using Elasticsearch's scroll API. The data is then parsed and transformed into Milvus 2.x storage format, followed by writing it using `bulkInsert`. Besides migrating `dense_vector` type vectors stored in Elasticsearch, Milvus Migration also supports migrating other field types, including long, integer, short, boolean, keyword, text, and double.\n\n### Milvus Migration feature set\n\nMilvus Migration simplifies the migration process through its robust feature set:\n\n- **Supported Data Sources:**\n\n\t- Milvus 1.x to Milvus 2.x\n\n\t- Elasticsearch 7.0 and beyond to Milvus 2.x\n\n\t- FAISS to Milvus 2.x\n\n \n\n- **Multiple Interaction Modes:**\n\n\t- Command-line interface (CLI) using the Cobra framework\n\n\t- Restful API with a built-in Swagger UI\n\n\t- Integration as a Go module in other tools\n\n \n\n- **Versatile File Format Support:**\n\n\t- Local files\n\n\t- Amazon S3\n\n\t- Object Storage Service (OSS)\n\n\t- Google Cloud Platform (GCP)\n\n \n\n- **Flexible Elasticsearch Integration:**\n\n\t- Migration of `dense_vector` type vectors from Elasticsearch\n\n\t- Support for migrating other field types such as long, integer, short, boolean, keyword, text, and double\n \n\n### Interface definitions\n\nMilvus Migration provides the following key interfaces:\n\n- `/start`: Initiates a migration job (equivalent to a combination of dump and load, currently only supports ES migration).\n \n- `/dump`: Initiates a dump job (writes source data into the target storage medium).\n \n- `/load`: Initiates a load job (writes data from the target storage medium into Milvus 2.x).\n \n- `/get_job`: Allows users to view job execution results. (For more details, refer to [the project's server.go](https://github.com/zilliztech/milvus-migration/blob/main/server/server.go))\n \n\n \n\nNext, let's use some example data to explore how to use Milvus Migration in this section. You can find these examples [here](https://github.com/zilliztech/milvus-migration#migration-examples-migrationyaml-details) on GitHub.\n\n## Migration from Elasticsearch to Milvus 2.x\n\n1. Prepare Elasticsearch Data\n \n\nTo [migrate Elasticsearch](https://zilliz.com/blog/elasticsearch-cloud-vs-zilliz) data, you should already set up your own Elasticsearch server. You should store vector data in the `dense_vector` field and index them with other fields. The index mappings are as shown below.\n\n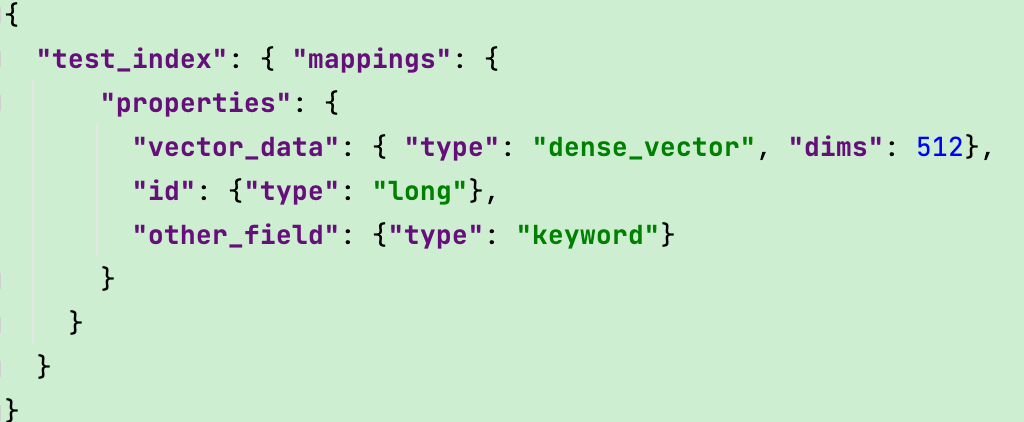\n\n\n\n2. Compile and Build\n \n\nFirst, download the Milvus Migration’s [source code from GitHub](https://github.com/zilliztech/milvus-migration). Then, run the following commands to compile it.\n\n\n```\ngo get\ngo build\n```\n\nThis step will generate an executable file named `milvus-migration`.\n\n \n\n3. Configure `migration.yaml`\n \n\nBefore starting the migration, you must prepare a configuration file named `migration.yaml` that includes information about the data source, target, and other relevant settings. Here's an example configuration:\n\n\n\n```\n# Configuration for Elasticsearch to Milvus 2.x migration\n\n\ndumper:\n worker:\n workMode: Elasticsearch\n reader:\n bufferSize: 2500\nmeta:\n mode: config\n index: test_index\n fields:\n - name: id\n pk: true\n type: long\n - name: other_field\n maxLen: 60\n type: keyword\n - name: data\n type: dense_vector\n dims: 512\n milvus:\n collection: \"rename_index_test\"\n closeDynamicField: false\n consistencyLevel: Eventually\n shardNum: 1\n\n\nsource:\n es:\n urls:\n - http://localhost:9200\n username: xxx\n password: xxx\n\n\ntarget:\n mode: remote\n remote:\n outputDir: outputPath/migration/test1\n cloud: aws\n region: us-west-2\n bucket: xxx\n useIAM: true\n checkBucket: false\n milvus2x:\n endpoint: {yourMilvusAddress}:{port}\n username: ******\n password: ******\n```\n \n\nFor a more detailed explanation of the configuration file, refer to [this page](https://github.com/zilliztech/milvus-migration/blob/main/README_ES.md#elasticsearch-to-milvus-2x-migrationyaml-example) on GitHub.\n\n \n\n4. Execute the migration job\n \n\nNow that you have configured your `migration.yaml` file, you can start the migration task by running the following command:\n\n\n```\n./milvus-migration start --config=/{YourConfigFilePath}/migration.yaml\n```\n\n \n\nObserve the log output. When you see logs similar to the following, it means the migration was successful.\n\n \n```\n[task/load_base_task.go:94] [\"[LoadTasker] Dec Task Processing--------------\u003e\"] [Count=0] [fileName=testfiles/output/zwh/migration/test_mul_field4/data_1_1.json] [taskId=442665677354739304][task/load_base_task.go:76] [\"[LoadTasker] Progress Task ---------------\u003e\"] [fileName=testfiles/output/zwh/migration/test_mul_field4/data_1_1.json] [taskId=442665677354739304][dbclient/cus_field_milvus2x.go:86] [\"[Milvus2x] begin to ShowCollectionRows\"][loader/cus_milvus2x_loader.go:66] [\"[Loader] Static: \"] [collection=test_mul_field4_rename1] [beforeCount=50000] [afterCount=100000] [increase=50000][loader/cus_milvus2x_loader.go:66] [\"[Loader] Static Total\"] [\"Total Collections\"=1] [beforeTotalCount=50000] [afterTotalCount=100000] [totalIncrease=50000][migration/es_starter.go:25] [\"[Starter] migration ES to Milvus finish!!!\"] [Cost=80.009174459][starter/starter.go:106] [\"[Starter] Migration Success!\"] [Cost=80.00928425][cleaner/remote_cleaner.go:27] [\"[Remote Cleaner] Begin to clean files\"] [bucket=a-bucket] [rootPath=testfiles/output/zwh/migration][cmd/start.go:32] [\"[Cleaner] clean file success!\"]\n```\n\n\n \n\nIn addition to the command-line approach, Milvus Migration also supports migration using Restful API.\n\nTo use the Restful API, start the API server using the following command:\n\n\n```\n./milvus-migration server run -p 8080\n```\n\nOnce the service runs, you can initiate the migration by calling the API.\n\n\n```\ncurl -XPOST http://localhost:8080/api/v1/start\n```\n\n \n\nWhen the migration is complete, you can use [Attu](https://zilliz.com/attu), an all-in-one vector database administration tool, to view the total number of successful rows migrated and perform other collection-related operations.\n\n\n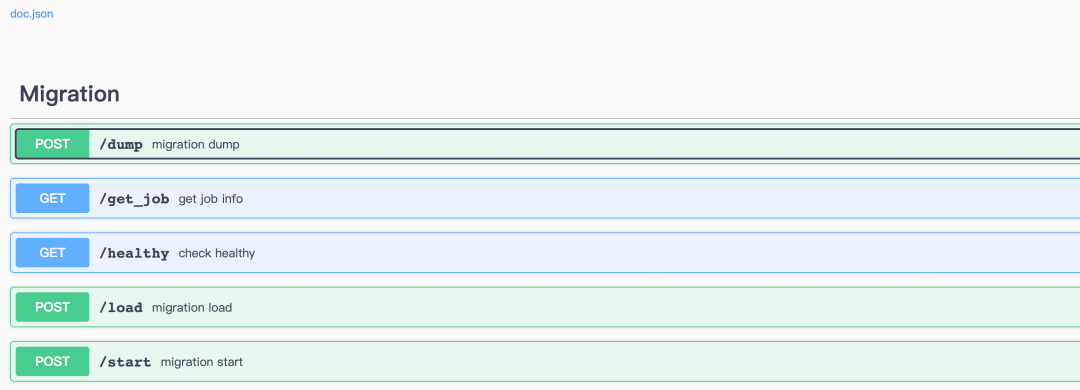\n\n\n\n## Migration from Milvus 1.x to Milvus 2.x\n\n1. Prepare Milvus 1.x Data\n \n\nTo help you quickly experience the migration process, we’ve put 10,000 Milvus 1.x [test data](https://github.com/zilliztech/milvus-migration/blob/main/README_1X.md) records in the source code of Milvus Migration. However, in real cases, you must export your own `meta.json` file from your Milvus 1.x instance before starting the migration process.\n\n- You can export the data with the following command.\n \n\n```\n./milvus-migration export -m \"user:password@tcp(adderss)/milvus?charset=utf8mb4\u0026parseTime=True\u0026loc=Local\" -o outputDir\n```\n\n \n\nMake sure to:\n\n- Replace the placeholders with your actual MySQL credentials.\n \n- Stop the Milvus 1.x server or halt data writes before performing this export.\n \n- Copy the Milvus `tables` folder and the `meta.json` file to the same directory.\n \n\n**Note:** If you use Milvus 2.x on [Zilliz Cloud](https://zilliz.com/cloud) (the fully managed service of Milvus), you can start the migration using Cloud Console.\n\n2. Compile and Build\n \n\nFirst, download the Milvus Migration’s [source code from GitHub](https://github.com/zilliztech/milvus-migration). Then, run the following commands to compile it.\n\n\n```\ngo get\ngo build\n```\n\n\nThis step will generate an executable file named `milvus-migration`.\n\n3. Configure `migration.yaml`\n \n\nPrepare a `migration.yaml` configuration file, specifying details about the source, target, and other relevant settings. Here's an example configuration:\n\n\n```\n# Configuration for Milvus 1.x to Milvus 2.x migration\n\n\ndumper:\n worker:\n limit: 2\n workMode: milvus1x\n reader:\n bufferSize: 1024\n writer:\n bufferSize: 1024\nloader:\n worker:\n limit: 16\nmeta:\n mode: local\n localFile: /outputDir/test/meta.json\n\n\nsource:\n mode: local\n local:\n tablesDir: /db/tables/\n\n\ntarget:\n mode: remote\n remote:\n outputDir: \"migration/test/xx\"\n ak: xxxx\n sk: xxxx\n cloud: aws\n endpoint: 0.0.0.0:9000\n region: ap-southeast-1\n bucket: a-bucket\n useIAM: false\n useSSL: false\n checkBucket: true\n milvus2x:\n endpoint: localhost:19530\n username: xxxxx\n password: xxxxx\n```\n\n\n \n\nFor a more detailed explanation of the configuration file, refer to [this page](https://github.com/zilliztech/milvus-migration/blob/main/README_1X.md) on GitHub.\n\n \n\n4. Execute Migration Job\n \n\nYou must execute the `dump` and `load` commands separately to finish the migration. These commands convert the data and import it into Milvus 2.x.\n\n**Note:** We’ll simplify this step and enable users to finish migration using just one command shortly. Stay tuned.\n\n \n**Dump Command:**\n\n```\n./milvus-migration dump --config=/{YourConfigFilePath}/migration.yaml\n```\n \n\n**Load Command:**\n\n\n```\n./milvus-migration load --config=/{YourConfigFilePath}/migration.yaml\n```\n\n \n\nAfter the migration, the generated collection in Milvus 2.x will contain two fields: `id` and `data`. You can view more details using [Attu](https://zilliz.com/attu), an all-in-one vector database administration tool.\n\n## Migration from FAISS to Milvus 2.x\n\n1. Prepare FAISS Data\n \n\nTo migrate Elasticsearch data, you should have your own FAISS data ready. To help you quickly experience the migration process, we’ve put some [FAISS test data](https://github.com/zilliztech/milvus-migration/blob/main/README_FAISS.md) in the source code of Milvus Migration.\n\n2. Compile and Build\n \n\nFirst, download the Milvus Migration’s [source code from GitHub](https://github.com/zilliztech/milvus-migration). Then, run the following commands to compile it.\n\n\n```\ngo get\ngo build\n```\n\nThis step will generate an executable file named `milvus-migration`.\n\n \n\n3. Configure `migration.yaml`\n \n\nPrepare a `migration.yaml` configuration file for FAISS migration, specifying details about the source, target, and other relevant settings. Here's an example configuration:\n\n```\n# Configuration for FAISS to Milvus 2.x migration\n\n\ndumper:\n worker:\n limit: 2\n workMode: FAISS\n reader:\n bufferSize: 1024\n writer:\n bufferSize: 1024\nloader:\n worker:\n limit: 2\nsource:\n mode: local\n local:\n FAISSFile: ./testfiles/FAISS/FAISS_ivf_flat.index\n\n\ntarget:\n create:\n collection:\n name: test1w\n shardsNums: 2\n dim: 256\n metricType: L2\n mode: remote\n remote:\n outputDir: testfiles/output/\n cloud: aws\n endpoint: 0.0.0.0:9000\n region: ap-southeast-1\n bucket: a-bucket\n ak: minioadmin\n sk: minioadmin\n useIAM: false\n useSSL: false\n checkBucket: true\n milvus2x:\n endpoint: localhost:19530\n username: xxxxx\n password: xxxxx\n```\n \n\nFor a more detailed explanation of the configuration file, refer to [this page](https://github.com/zilliztech/milvus-migration/blob/main/README_FAISS.md) on GitHub.\n\n \n\n4. Execute Migration Job\n \n\nLike Milvus 1.x to Milvus 2.x migration, FAISS migration requires executing both the `dump` and `load` commands. These commands convert the data and import it into Milvus 2.x.\n\n**Note:** We’ll simplify this step and enable users to finish migration using just one command shortly. Stay tuned.\n\n**Dump Command:**\n```\n./milvus-migration dump --config=/{YourConfigFilePath}/migration.yaml\n```\n\n**Load Command:**\n\n```\n./milvus-migration load --config=/{YourConfigFilePath}/migration.yaml\n```\n\n\n\n \n\nYou can view more details using [Attu](https://zilliz.com/attu), an all-in-one vector database administration tool.\n\n## Stay tuned for future migration plans\n\nIn the future, we’ll support migration from more data sources and add more migration features, including:\n\n- Support migration from Redis to Milvus.\n \n- Support migration from MongoDB to Milvus.\n \n- Support resumable migration.\n \n- Simplify migration commands by merging the dump and load processes into one.\n \n- Support migration from other mainstream data sources to Milvus.\n \n\n## Conclusion\n\nMilvus 2.3, the latest release of Milvus, brings exciting new features and performance improvements that cater to the growing needs of data management. Migrating your data to Milvus 2.x can unlock these benefits, and the Milvus Migration project makes the migration process streamlined and easy. Give it a try, and you won't be disappointed.\n\n***Note:** The information in this blog is based on the state of the Milvus and [Milvus Migration](https://github.com/zilliztech/milvus-migration) projects as of September 2023. Check the official [Milvus documentation](https://milvus.io/docs) for the most up-to-date information and instructions.*","title":"How to Migrate Your Data to Milvus Seamlessly: A Comprehensive Guide","metaData":{}},{"id":"milvus-embraces-nats-messaging.md","author":"Zhen Ye","desc":"Introducing the integration of NATS and Milvus, exploring its features, setup and migration process, and performance testing results.","tags":["Engineering"],"recommend":true,"canonicalUrl":"https://zilliz.com/blog/optimizing-data-communication-milvus-embraces-nats-messaging","date":"2023-11-24T00:00:00.000Z","cover":"https://assets.zilliz.com/Exploring_NATS_878f48c848.png","href":"/blog/milvus-embraces-nats-messaging.md","content":"\n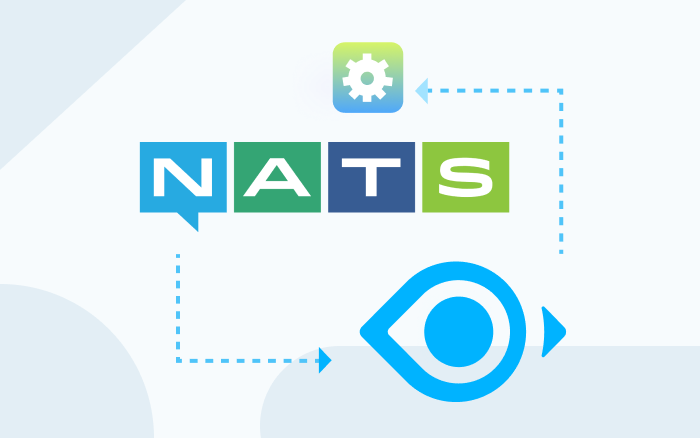\n\nIn the intricate tapestry of data processing, seamless communication is the thread that binds operations together. [Milvus](https://zilliz.com/what-is-milvus), the trailblazing [open-source vector database](https://zilliz.com/cloud), has embarked on a transformative journey with its latest feature: NATS messaging integration. In this comprehensive blog post, we'll unravel the intricacies of this integration, exploring its core features, setup process, migration benefits, and how it stacks up against its predecessor, RocksMQ.\n\n## Understanding the role of message queues in Milvus\n\nIn Milvus’ cloud-native architecture, the message queue, or Log Broker, holds pivotal importance. It’s the backbone ensuring persistent data streams, synchronization, event notifications, and data integrity during system recoveries. Traditionally, RocksMQ was the most straightforward choice in Milvus Standalone mode, especially when compared with Pulsar and Kafka, but its limitations became evident with extensive data and complex scenarios.\n\nMilvus 2.3 introduces NATS, a single-node MQ implementation, redefining how to manage data streams. Unlike its predecessors, NATS liberates Milvus users from performance constraints, delivering a seamless experience in handling substantial data volumes.\n\n## What is NATS?\n\nNATS is a distributed system connectivity technology implemented in Go. It supports various communication modes like Request-Reply and Publish-Subscribe across systems, provides data persistence through JetStream, and offers distributed capabilities through built-in RAFT. You can refer to the [NATS official website](https://nats.io/) for a more detailed understanding of NATS.\n\nIn Milvus 2.3 Standalone mode, NATS, JetStream, and PubSub provide Milvus with robust MQ capabilities.\n\n## Enabling NATS\n\nMilvus 2.3 offers a new control option, `mq.type`, which allows users to specify the type of MQ they want to use. To enable NATS, set `mq.type=natsmq`. If you see logs similar to the ones below after you initiate Milvus instances, you have successfully enabled NATS as the message queue.\n\n \n```\n[INFO] [dependency/factory.go:83] [\"try to init mq\"] [standalone=true] [mqType=natsmq]\n```\n \n\n## Configuring NATS for Milvus\n\nNATS customization options include specifying the listening port, JetStream storage directory, maximum payload size, and initialization timeout. Fine-tuning these settings ensures optimal performance and reliability.\n\n\n```\nnatsmq:\nserver: # server side configuration for natsmq.\nport: 4222 # 4222 by default, Port for nats server listening.\nstoreDir: /var/lib/milvus/nats # /var/lib/milvus/nats by default, directory to use for JetStream storage of nats.\nmaxFileStore: 17179869184 # (B) 16GB by default, Maximum size of the 'file' storage.\nmaxPayload: 8388608 # (B) 8MB by default, Maximum number of bytes in a message payload.\nmaxPending: 67108864 # (B) 64MB by default, Maximum number of bytes buffered for a connection Applies to client connections.\ninitializeTimeout: 4000 # (ms) 4s by default, waiting for initialization of natsmq finished.\nmonitor:\ntrace: false # false by default, If true enable protocol trace log messages.\ndebug: false # false by default, If true enable debug log messages.\nlogTime: true # true by default, If set to false, log without timestamps.\nlogFile: /tmp/milvus/logs/nats.log # /tmp/milvus/logs/nats.log by default, Log file path relative to .. of milvus binary if use relative path.\nlogSizeLimit: 536870912 # (B) 512MB by default, Size in bytes after the log file rolls over to a new one.\nretention:\nmaxAge: 4320 # (min) 3 days by default, Maximum age of any message in the P-channel.\nmaxBytes: # (B) None by default, How many bytes the single P-channel may contain. Removing oldest messages if the P-channel exceeds this size.\nmaxMsgs: # None by default, How many message the single P-channel may contain. Removing oldest messages if the P-channel exceeds this limit.\n```\n \n\n**Note:**\n\n- You must specify `server.port` for NATS server listening. If there is a port conflict, Milvus cannot start. Set `server.port=-1` to randomly select a port.\n \n- `storeDir` specifies the directory for JetStream storage. We recommend storing the directory in a high-performant solid-state drive (SSD) for better read/write throughput of Milvus.\n \n- `maxFileStore` sets the upper limit of JetStream storage size. Exceeding this limit will prevent further data writing.\n \n- `maxPayload` limits individual message size. You should keep it above 5MB to avoid any write rejections.\n \n- `initializeTimeout`controls NATS server startup timeout.\n \n- `monitor` configures NATS’ independent logs.\n \n- `retention` controls the retention mechanism of NATS messages.\n \n\nFor more information, refer to [NATS official documentation](https://docs.nats.io/running-a-nats-service/configuration).\n\n## Migrating from RocksMQ to NATS\n\nMigrating from RocksMQ to NATS is a seamless process involving steps like stopping write operations, flushing data, modifying configurations, and verifying the migration through Milvus logs.\n\n1. Before initiating the migration, stop all write operations in Milvus.\n \n2. Execute the `FlushALL` operation in Milvus and wait for its completion. This step ensures that all pending data is flushed and the system is ready for shutdown.\n \n3. Modify the Milvus configuration file by setting `mq.type=natsmq` and adjusting relevant options under the `natsmq` section.\n \n4. Start the Milvus 2.3.\n \n5. Back up and clean the original data stored in the `rocksmq.path` directory. (Optional)\n \n\n## NATS vs. RocksMQ: A Performance Showdown\n\n### Pub/Sub Performance Testing\n\n- **Testing Platform:** M1 Pro Chip / Memory: 16GB\n \n- **Testing Scenario:** Subscribing and publishing random data packets to a topic repeatedly until the last published result is received.\n \n- **Results:**\n \n - For smaller data packets (\u003c 64kb), RocksMQ outperforms NATS regarding memory, CPU, and response speed.\n \n - For larger data packets (\u003e 64kb), NATS outshines RocksMQ, offering much faster response times.\n \n\n \n\n| Test Type | MQ | op count | cost per op | Memory cost | CPU Total Time | Storage cost |\n| ------------------- | ------- | -------- | ---------------- | ----------- | -------------- | ------------ |\n| 5MB\\*100 Pub/Sub | NATS | 50 | 1.650328186 s/op | 4.29 GB | 85.58 | 25G |\n| 5MB\\*100 Pub/Sub | RocksMQ | 50 | 2.475595131 s/op | 1.18 GB | 81.42 | 19G |\n| 1MB\\*500 Pub/Sub | NATS | 50 | 2.248722593 s/op | 2.60 GB | 96.50 | 25G |\n| 1MB\\*500 Pub/Sub | RocksMQ | 50 | 2.554614279 s/op | 614.9 MB | 80.19 | 19G |\n| 64KB\\*10000 Pub/Sub | NATS | 50 | 2.133345262 s/op | 3.29 GB | 97.59 | 31G |\n| 64KB\\*10000 Pub/Sub | RocksMQ | 50 | 3.253778195 s/op | 331.2 MB | 134.6 | 24G |\n| 1KB\\*50000 Pub/Sub | NATS | 50 | 2.629391004 s/op | 635.1 MB | 179.67 | 2.6G |\n| 1KB\\*50000 Pub/Sub | RocksMQ | 50 | 0.897638581 s/op | 232.3 MB | 60.42 | 521M |\n\n \n\nTable 1: Pub/Sub performance testing results\n\n### Milvus Integration Testing\n\n**Data size:** 100M\n\n**Result:** In extensive testing with a 100 million vectors dataset, NATS showcased lower vector search and query latency.\n\n| Metrics | RocksMQ (ms) | NATS (ms) |\n| --------------------------------------- | ------------ | --------- |\n| Average vector search latency | 23.55 | 20.17 |\n| Vector search requests per second (RPS) | 2.95 | 3.07 |\n| Average query latency | 7.2 | 6.74 |\n| Query requests per second (RPS) | 1.47 | 1.54 |\n\nTable 2: Milvus integration testing results with 100m dataset\n\n \n\n**Dataset: \u003c100M**\n\n**Result:** For datasets smaller than 100M, NATS and RocksMQ show similar performance.\n\n## Conclusion: Empowering Milvus with NATS messaging\n\nThe integration of NATS within Milvus marks a significant stride in data processing. Whether delving into real-time analytics, machine learning applications, or any data-intensive venture, NATS empowers your projects with efficiency, reliability, and speed. As the data landscape evolves, having a robust messaging system like NATS within Milvus ensures seamless, reliable, and high-performing data communication.","title":"Optimizing Data Communication: Milvus Embraces NATS Messaging","metaData":{}},{"id":"revealing-milvus-2-3-2-and-milvus-2-3-3.md","author":"Fendy Feng, Owen Jiao","desc":"Today, we are thrilled to announce the release of Milvus 2.3.2 and 2.3.3! These updates bring many exciting features, optimizations, and improvements, enhancing system performance, flexibility, and overall user experience.","tags":["News"],"recommend":true,"canonicalUrl":null,"date":"2023-11-20T00:00:00.000Z","cover":"https://assets.zilliz.com/What_s_New_in_Milvus_2_3_2_and_2_3_3_d3d0db03c3.png","href":"/blog/revealing-milvus-2-3-2-and-milvus-2-3-3.md","content":"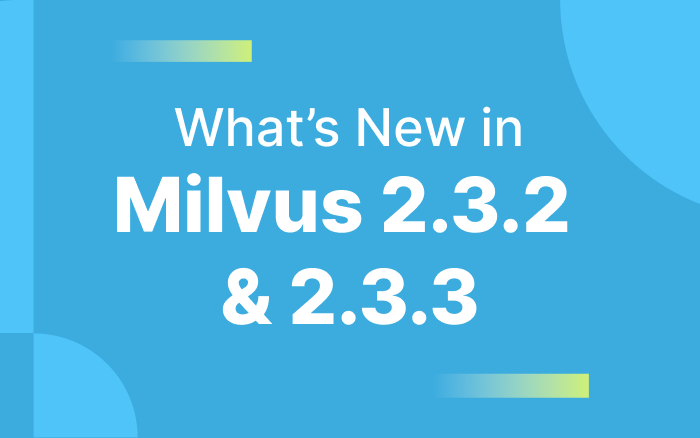\n\nIn the ever-evolving landscape of vector search technologies, Milvus remains at the forefront, pushing boundaries and setting new standards. Today, we are thrilled to announce the release of Milvus 2.3.2 and 2.3.3! These updates bring many exciting features, optimizations, and improvements, enhancing system performance, flexibility, and overall user experience.\n\n## Support for Array data types - making search results more accurate and relevant\n\nAdding the Array data type support is a pivotal enhancement for Milvus, particularly in query filtering scenarios like intersection and union. This addition ensures that search results are not only more accurate but also more relevant. In practical terms, for instance, within the e-commerce sector, product tags stored as string Arrays allow consumers to perform advanced searches, filtering out irrelevant results.\n\nDive into our comprehensive [documentation](https://milvus.io/docs/array_data_type.md) for an in-depth guide on leveraging Array types in Milvus.\n\n## Support for complex delete expressions - improving your data management\n\nIn previous versions, Milvus supported primary key deletion expressions, providing a stable and streamlined architecture. With Milvus 2.3.2 or 2.3.3, users can employ complex delete expressions, facilitating sophisticated data management tasks such as rolling cleanup of old data or GDPR compliance-driven data deletion based on user IDs.\n\nNote: Ensure you’ve loaded collections before utilizing complex expressions. Additionally, it's important to note that the deletion process does not guarantee atomicity.\n\n## TiKV integration - scalable metadata storage with stability\n\nPreviously relying on Etcd for metadata storage, Milvus faced limited capacity and scalability challenges in metadata storage. To address these problems, Milvus added TiKV, an open-source key-value store, as one more option for metadata storage. TiKV offers enhanced scalability, stability, and efficiency, making it an ideal solution for Milvus's evolving requirements. Starting from Milvus 2.3.2, users can seamlessly transition to TiKV for their metadata storage by modifying the configuration.\n\n## Support for FP16 vector type - embracing machine learning efficiency\n\nMilvus 2.3.2 and later versions now support the FP16 vector type at the interface level. FP16, or 16-bit floating point, is a data format widely used in deep learning and machine learning, providing efficient representation and calculation of numerical values. While full support for FP16 is underway, various indexes in the indexing layer require converting FP16 to FP32 during construction.\n\nWe will fully support FP16, BF16, and int8 data types in later versions of Milvus. Stay tuned.\n\n## Significant improvement in the rolling upgrade experience - seamless transition for users\n\nRolling upgrade is a critical feature for distributed systems, enabling system upgrades without disrupting business services or experiencing downtimes. In the latest Milvus releases, we’ve strengthened Milvus’s rolling upgrade feature, ensuring a more streamlined and efficient transition for users’ upgrading from version 2.2.15 to 2.3.3 and all later versions. The community has also invested in extensive testing and optimizations, reducing query impact during the upgrade to less than 5 minutes, providing users with a hassle-free experience.\n\n## Performance optimization\n\nIn addition to introducing new features, we’ve significantly optimized the performance of Milvus in the latest two releases.\n\n- Minimized data copy operations for optimized data loading\n \n- Simplified large-capacity inserts using batch varchar reading\n \n- Removed unnecessary offset checks during data padding to improve recall phase performance.\n \n- Addressed high CPU consumption issues in scenarios with substantial data insertions\n \n\nThese optimizations collectively contribute to a faster and more efficient Milvus experience. Check out our monitoring dashboard for a quick glance at how Milvus improved its performance.\n\n \n\n## Incompatible changes\n\n- Permanently deleted TimeTravel-related code.\n \n- Deprecated support for MySQL as the metadata store.\n \n\nRefer to the [Milvus release notes](https://milvus.io/docs/release_notes.md) for more detailed information about all the new features and enhancements.\n\n## Conclusion\n\nWith the latest Milvus 2.3.2 and 2.3.3 releases, we're committed to providing a robust, feature-rich, high-performance database solution. Explore these new features, take advantage of the optimizations, and join us on this exciting journey as we evolve Milvus to meet the demands of modern data management. Download the latest version now and experience the future of data storage with Milvus!\n\n## Let’s keep in touch!\n\nIf you have questions or feedback about Milvus, join our [Discord channel](https://discord.com/invite/8uyFbECzPX) to engage with our engineers and the community directly or join our [Milvus Community Lunch and Learn](https://discord.com/invite/RjNbk8RR4f) Every Tuesday from 12-12:30 PM PST. You’re also welcome to follow us on [Twitter](https://twitter.com/milvusio) or [LinkedIn](https://www.linkedin.com/company/the-milvus-project) for the latest news and updates about Milvus.","title":"Revealing Milvus 2.3.2 \u0026 2.3.3: Support for Array Data Types, Complex Delete, TiKV Integration, and More","metaData":{}},{"id":"milvus-introduced-mmap-for-redefined-data-management-increased-storage-capability.md","author":"Yang Cen","desc":"The Milvus MMap feature empowers users to handle more data within limited memory, striking a delicate balance between performance, cost, and system limits.","tags":["Engineering"],"recommend":true,"canonicalUrl":"https://zilliz.com/blog/milvus-introduced-mmap-for-redefined-data-management-increased-storage-capability","date":"2023-11-15T00:00:00.000Z","cover":"https://assets.zilliz.com/Exploring_M_Map_5086d652bd.png","href":"/blog/milvus-introduced-mmap-for-redefined-data-management-increased-storage-capability.md","content":"\n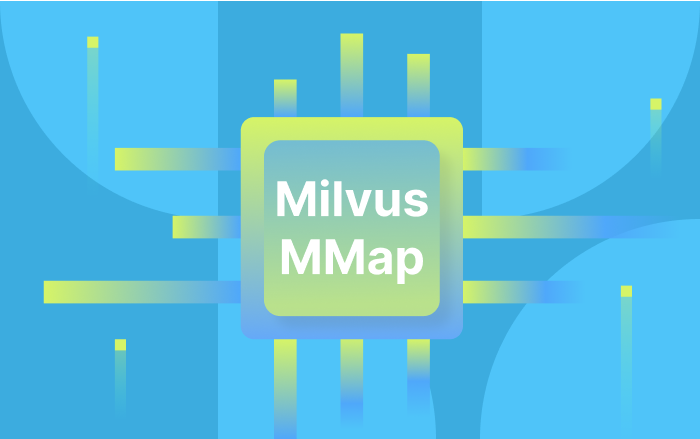\n\n[Milvus](https://zilliz.com/what-is-milvus) is the fastest solution in open-source [vector databases](https://zilliz.com/blog/what-is-a-real-vector-database), catering to users with intensive performance requirements. However, the diversity of user needs mirrors the data they work with. Some prioritize budget-friendly solutions and expansive storage over sheer speed. Understanding this spectrum of demands, Milvus introduces the MMap feature, redefining how we handle large data volumes while promising cost efficiency without sacrificing functionality.\n\n## What is MMap?\n\nMMap, short for memory-mapped files, bridges the gap between files and memory within operating systems. This technology allows Milvus to map large files directly into the system's memory space, transforming files into contiguous memory blocks. This integration eliminates the need for explicit read or write operations, fundamentally changing how Milvus manages data. It ensures seamless access and efficient storage for large files or situations where users need to access files randomly.\n\n## Who benefits from MMap?\n\nVector databases demand substantial memory capacity due to the storage requirements of vector data. With the MMap feature, processing more data within limited memory becomes a reality. However, this increased capability comes at a performance cost. The system intelligently manages memory, evicting some data based on load and usage. This eviction allows Milvus to process more data within the same memory capacity.\n\nDuring our tests, we observed that with ample memory, all data resides in memory after a warm-up period, preserving system performance. However, as data volume grows, performance gradually decreases. **Therefore, we recommend the MMap feature for users less sensitive to performance fluctuations.**\n\n## Enabling MMap in Milvus: a simple configuration\n\nEnabling MMap in Milvus is remarkably straightforward. All you need to do is modify the `milvus.yaml` file: add the `mmapDirPath` item under the `queryNode` configuration and set a valid path as its value.\n\n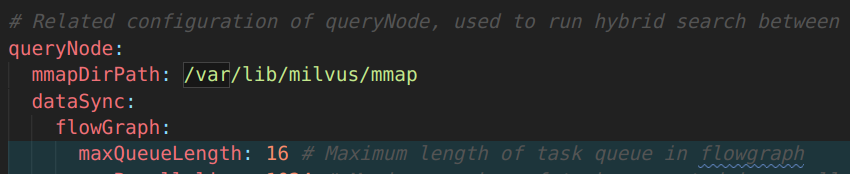\n\n## Striking the balance: performance, storage, and system limits\n\nData access patterns significantly impact performance. Milvus's MMap feature optimizes data access based on locality. MMap enables Milvus to write scalar data directly to the disk for sequentially accessed data segments. Variable-length data such as strings undergoes flattening and is indexed using an offsets array in memory. This approach ensures data access locality and eliminates the overhead of storing each variable-length data separately. Optimizations for vector indexes are meticulous. MMap is selectively employed for vector data while retaining adjacency lists in memory, conserving significant memory without compromising performance.\n\nIn addition, MMap maximizes data processing by minimizing memory usage. Unlike previous Milvus versions where QueryNode copied entire datasets, MMap adopts a streamlined, copy-free streaming process during development. This optimization drastically reduces memory overhead.\n\n**Our internal testing results show that Milvus can efficiently handle double the data volume when enabling MMap.**\n\n## The road ahead: continuous innovation and user-centric enhancements\n\nWhile the MMap feature is in its beta phase, Milvus's team is committed to continuous improvement. Future updates will refine the system's memory usage, enabling Milvus to support even more extensive data volumes on a single node. Users can anticipate more granular control over the MMap feature, enabling dynamic changes to collections and advanced field loading modes. These enhancements provide unprecedented flexibility, allowing users to tailor their data processing strategies to specific requirements.\n\n## Conclusion: redefining data processing excellence with Milvus MMap\n\nMilvus 2.3's MMap feature marks a significant leap in data processing technology. By striking a delicate balance between performance, cost, and system limits, Milvus empowers users to handle vast amounts of data efficiently and cost-effectively. As Milvus continues to evolve, it remains at the forefront of innovative solutions, redefining the boundaries of what's achievable in data management.\n\nStay tuned for more groundbreaking developments as Milvus continues its journey toward unparalleled data processing excellence.\n","title":"Milvus Introduced MMap for Redefined Data Management and Increased Storage Capability","metaData":{}},{"id":"comparing-vector-database-vector-search-plugin-vector-search-libraries.md","author":"Frank Liu","desc":"In this post, we’ll continue to explore the intricate realm of vector search, comparing vector databases, vector search plugins, and vector search libraries.","tags":["Engineering"],"recommend":true,"canonicalUrl":"https://zilliz.com/learn/comparing-vector-database-vector-search-library-and-vector-search-plugin","date":"2023-11-09T00:00:00.000Z","cover":"https://assets.zilliz.com/Vector_Databases_vs_Vector_Search_Plugins_vs_Vector_Search_Libraries_74def521ad.png","href":"/blog/comparing-vector-database-vector-search-plugin-vector-search-libraries.md","content":"\n\n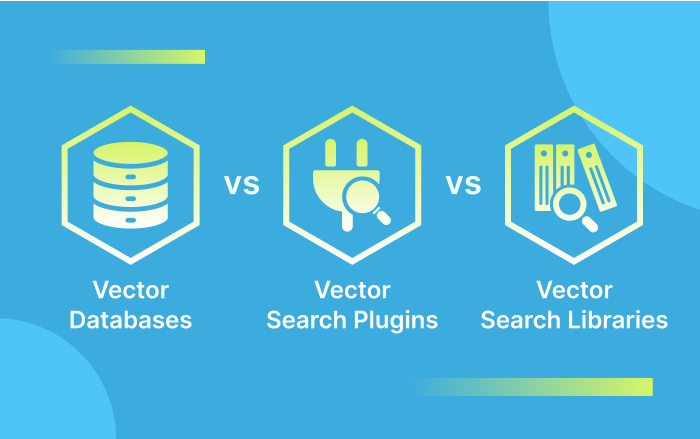\n\nHey there - welcome back to Vector Database 101!\n\nThe surge in [ChatGPT](https://zilliz.com/learn/ChatGPT-Vector-Database-Prompt-as-code) and other large language models (LLMs) has driven the growth of vector search technologies, featuring specialized vector databases like [Milvus](https://zilliz.com/what-is-milvus) and [Zilliz Cloud](https://zilliz.com/cloud) alongside libraries such as [FAISS](https://zilliz.com/blog/set-up-with-facebook-ai-similarity-search-faiss) and integrated vector search plugins within conventional databases.\n\n\nIn our [previous series post](https://zilliz.com/learn/what-is-vector-database), we delved into the fundamentals of vector databases. In this post, we’ll continue to explore the intricate realm of vector search, comparing vector databases, vector search plugins, and vector search libraries.\n\n\n## What is vector search?\n\n\n[Vector search](https://zilliz.com/learn/vector-similarity-search), also known as vector similarity search, is a technique for retrieving the top-k results that are most similar or semantically related to a given query vector among an extensive collection of dense vector data. Before conducting similarity searches, we leverage neural networks to transform [unstructured data](https://zilliz.com/learn/introduction-to-unstructured-data), such as text, images, videos, and audio, into high-dimensional numerical vectors called embedding vectors. After generating embedding vectors, vector search engines compare the spatial distance between the input query vector and the vectors in the vector stores. The closer they are in space, the more similar they are.\n\n\nMultiple vector search technologies are available in the market, including machine learning libraries like Python's NumPy, vector search libraries like FAISS, vector search plugins built on traditional databases, and specialized vector databases like Milvus and Zilliz Cloud.\n\n\n\n\n## Vector databases vs. vector search libraries\n\n\n[Specialized vector databases](https://zilliz.com/blog/what-is-a-real-vector-database) are not the only stack for similarity searches. Before the advent of vector databases, many vector searching libraries, such as FAISS, ScaNN, and HNSW, were used for vector retrieval.\n\n\nVector search libraries can help you quickly build a high-performance prototype vector search system. Taking FAISS as an example, it is open-source and developed by Meta for efficient similarity search and dense vector clustering. FAISS can handle vector collections of any size, even those that cannot be fully loaded into memory. Additionally, FAISS offers tools for evaluation and parameter tuning. Even though written in C++, FAISS provides a Python/NumPy interface.\n\n\nHowever, vector search libraries are merely lightweight ANN libraries rather than managed solutions, and they have limited functionality. If your dataset is small and limited, these libraries can be sufficient for unstructured data processing, even for systems running in production. However, as dataset sizes increase and more users are onboarded, the scale problem becomes increasingly difficult to solve. Moreover, they don’t allow any modifications to their index data and cannot be queried during data import.\n\n\nBy contrast, vector databases are a more optimal solution for unstructured data storage and retrieval. They can store and query millions or even billions of vectors while providing real-time responses simultaneously; they’re highly scalable to meet users’ growing business needs.\n\n\nIn addition, vector databases like Milvus have much more user-friendly features for structured/semi-structured data: cloud-nativity, multi-tenancy, scalability, etc. These features will become clear as we dive deeper into this tutorial.\n\n\nThey also operate in a totally different layer of abstraction from vector search libraries - vector databases are full-fledged services, while ANN libraries are meant to be integrated into the application that you’re developing. In this sense, ANN libraries are one of the many components that vector databases are built on top of, similar to how Elasticsearch is built on top of Apache Lucene.\n\n\nTo give an example of why this abstraction is so important, let’s look at inserting a new unstructured data element into a vector database. This is super easy in Milvus:\n\n\n```\nfrom pymilvus import Collectioncollection = Collection('book')mr = collection.insert(data)\n```\n\n\nIt’s really as easy as that - 3 lines of code. With a library such as FAISS or ScaNN, there is, unfortunately, no easy way of doing this without manually re-creating the entire index at certain checkpoints. Even if you could, vector search libraries still lack scalability and multi-tenancy, two of the most important vector database features.\n\n\n## Vector databases vs. vector search plugins for traditional databases\n\n\nGreat, now that we’ve established the difference between vector search libraries and vector databases, let’s take a look at how vector databases differ from **vector search plugins**.\n\n\nAn increasing number of traditional relational databases, and search systems such as Clickhouse and [Elasticsearch](https://zilliz.com/blog/elasticsearch-cloud-vs-zilliz) are including built-in vector search plugins. Elasticsearch 8.0, for example, includes vector insertion and ANN search functionality that can be called via restful API endpoints. The problem with vector search plugins should be clear as night and day - **these solutions do not take a full-stack approach to embedding management and vector search**. Instead, these plugins are meant to be enhancements on top of existing architectures, thereby making them limited and unoptimized. Developing an unstructured data application atop a traditional database would be like trying to fit lithium batteries and electric motors inside the frame of a gas-powered car - not a great idea!\n\n\nTo illustrate why this is, let’s go back to the list of features that a vector database should implement (from the first section). Vector search plugins are missing two of these features - tunability and user-friendly APIs/SDKs. I’ll continue to use Elasticsearch’s ANN engine as an example; other vector search plugins operate very similarly so I won’t go too much further into detail. Elasticsearch supports vector storage via the `dense_vector` data field type and allows for querying via the `knnsearch endpoint`:\n\n\n```json\nPUT index\n{\n\"mappings\": {\n \"properties\": {\n \"image-vector\": {\n \"type\": \"dense_vector\",\n \"dims\": 128,\n \"index\": true,\n \"similarity\": \"l2_norm\"\n }\n }\n}\n}\n\n\nPUT index/_doc\n{\n\"image-vector\": [0.12, 1.34, ...]\n}\n```\n\n\n```json\nGET index/_knn_search\n{\n\"knn\": {\n \"field\": \"image-vector\",\n \"query_vector\": [-0.5, 9.4, ...],\n \"k\": 10,\n \"num_candidates\": 100\n}\n}\n```\n\n\nElasticsearch's ANN plugin supports only one indexing algorithm: Hierarchical Navigable Small Worlds, also known as HNSW (I like to think that the creator was ahead of Marvel when it came to popularizing the multiverse). On top of that, only L2/Euclidean distance is supported as a distance metric. This is an okay start, but let's compare it to Milvus, a full-fledged vector database. Using `pymilvus`:\n\n\n```python\n\u003e\u003e\u003e field1 = FieldSchema(name='id', dtype=DataType.INT64, description='int64', is_primary=True)\n\u003e\u003e\u003e field2 = FieldSchema(name='embedding', dtype=DataType.FLOAT_VECTOR, description='embedding', dim=128, is_primary=False)\n\u003e\u003e\u003e schema = CollectionSchema(fields=[field1, field2], description='hello world collection')\n\u003e\u003e\u003e collection = Collection(name='my_collection', data=None, schema=schema)\n\u003e\u003e\u003e index_params = {\n 'index_type': 'IVF_FLAT',\n 'params': {'nlist': 1024},\n \"metric_type\": 'L2'}\n\u003e\u003e\u003e collection.create_index('embedding', index_params)\n```\n\n\n```python\n\u003e\u003e\u003e search_param = {\n 'data': vector,\n 'anns_field': 'embedding',\n 'param': {'metric_type': 'L2', 'params': {'nprobe': 16}},\n 'limit': 10,\n 'expr': 'id_field \u003e 0'\n }\n\u003e\u003e\u003e results = collection.search(**search_param)\n```\n\n\nWhile both [Elasticsearch and Milvus](https://zilliz.com/comparison/elastic-vs-milvus) have methods for creating indexes, inserting embedding vectors, and performing nearest neighbor search, it’s clear from these examples that Milvus has a more intuitive vector search API (better user-facing API) and broader vector index + distance metric support (better tunability). Milvus also plans to support more vector indices and allow for querying via SQL-like statements in the future, further improving both tunability and usability.\n\n\nWe just blew through quite a bit of content. This section was admittedly fairly long, so for those of you who skimmed it, here’s a quick tl;dr: Milvus is better than vector search plugins because Milvus was built from the ground-up as a vector database, allowing for a richer set of features and an architecture more suited towards unstructured data.\n\n\n## How to choose from different vector search technologies?\n\n\nNot all vector databases are created equal; each possesses unique traits that cater to specific applications. Vector search libraries and plugins are user-friendly and ideal for handling small-scale production environments with millions of vectors. If your data size is small and you just require basic vector search functionality, these technologies are sufficient for your business.\n\n\nHowever, a specialized vector database should be your top choice for data-intensive businesses dealing with hundreds of millions of vectors and demanding real-time responses. Milvus, for instance, effortlessly manages billions of vectors, offering lightning-fast query speeds and rich functionality. Moreover, fully managed solutions like Zilliz prove even more advantageous, liberating you from operational challenges and enabling an exclusive focus on your core business activities.\n\n\n## Take another look at the Vector Database 101 courses\n\n\n1. [Introduction to Unstructured Data](https://zilliz.com/blog/introduction-to-unstructured-data)\n2. [What is a Vector Database?](https://zilliz.com/learn/what-is-vector-database)\n3. [Comparing Vector Databases, Vector Search Libraries, and Vector Search Plugins](https://zilliz.com/learn/comparing-vector-database-vector-search-library-and-vector-search-plugin)\n4. [Introduction to Milvus](https://zilliz.com/blog/introduction-to-milvus-vector-database)\n5. [Milvus Quickstart](https://zilliz.com/blog/milvus-vector-database-quickstart)\n6. [Introduction to Vector Similarity Search](https://zilliz.com/blog/vector-similarity-search)\n7. [Vector Index Basics and the Inverted File Index](https://zilliz.com/blog/vector-index)\n8. [Scalar Quantization and Product Quantization](https://zilliz.com/blog/scalar-quantization-and-product-quantization)\n9. [Hierarchical Navigable Small Worlds (HNSW)](https://zilliz.com/blog/hierarchical-navigable-small-worlds-HNSW)\n10. [Approximate Nearest Neighbors Oh Yeah (ANNOY)](https://zilliz.com/learn/approximate-nearest-neighbor-oh-yeah-ANNOY)\n11. [Choosing the Right Vector Index for Your Project](https://zilliz.com/learn/choosing-right-vector-index-for-your-project)\n12. [DiskANN and the Vamana Algorithm](https://zilliz.com/learn/DiskANN-and-the-Vamana-Algorithm)\n","title":"Comparing Vector Databases, Vector Search Libraries, and Vector Search Plugins\n","metaData":{}},{"id":"Ingesting-Chaos-MLOps-Behind-Handling-Unstructured-Data-Reliably-at-Scale-for-RAG.md","author":"David Garnitz","tags":["News"],"recommend":true,"desc":"With technologies like VectorFlow and Milvus, the team can efficiently test across different environments while complying with privacy and security requirements.","canonicalUrl":"https://milvus.io/blog/Ingesting-Chaos-MLOps-Behind-Handling-Unstructured-Data-Reliably-at-Scale-for-RAG.md","date":"2023-10-16T00:00:00.000Z","cover":"https://assets.zilliz.com/Ingesting_Chaos_20231017_110103_54fe2009cb.png","href":"/blog/Ingesting-Chaos-MLOps-Behind-Handling-Unstructured-Data-Reliably-at-Scale-for-RAG.md","content":"\n\n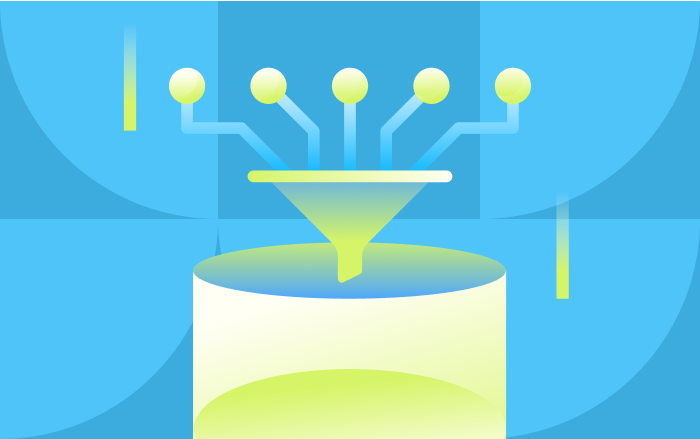\n\n\nData is being generated faster than ever before in every form imaginable. This data is the gasoline that will power a new wave of artificial intelligence applications, but these productivity enhancement engines need help ingesting this fuel. The wide range of scenarios and edge cases surrounding unstructured data makes it challenging to use in production AI systems.\n\nFor starters, there are a vast number of data sources. These export data in various file formats, each with its eccentricities. For example, how you process a PDF varies greatly depending on where it comes from. Ingesting a PDF for a securities litigation case will likely focus on textual data. In contrast, a system design specification for a rocket engineer will be full of diagrams that require visual processing. The lack of a defined schema in unstructured data further adds complexity. Even when the challenge of processing the data is overcome, the issue of ingesting it at scale remains. Files can vary significantly in size, which changes how they are processed. You can quickly process a 1MB upload on an API over HTTP, but reading in dozens of GBs from a single file requires streaming and a dedicated worker. \n\nOvercoming these traditional data engineering challenges is table stakes for connecting raw data to [LLMs](https://zilliz.com/glossary/large-language-models-(llms)) via [vector databases](https://zilliz.com/learn/what-is-vector-database) like [Milvus](https://github.com/milvus-io/milvus). However, emerging use cases such as performing semantic similarity searches with the help of a vector database require new processing steps like chunking the source data, orchestrating metadata for hybrid searches, picking the suitable vector embedding model, and tuning the search parameters to determine what data to feed to the LLM. These workflows are so new that there are no established best practices for developers to follow. Instead, they must experiment to find the correct configuration and use case for their data. To speed up this process, using a vector embedding pipeline to handle the data ingestion into the vector database is invaluable. \n\nA vector embedding pipeline like [VectorFlow](https://github.com/dgarnitz/vectorflow) will connect your raw data to your vector database, including chunking, metadata orchestration, embedding, and upload. VectorFlow enables engineering teams to focus on the core application logic, experimenting with the different retrieval parameters generated from the embedding model, the chunking strategy, the metadata fields, and aspects of the search to see what performs best. \n\nIn our work helping engineering teams move their [retrieval augmented generation (RAG)](https://zilliz.com/use-cases/llm-retrieval-augmented-generation) systems from prototype to production, we have observed the following approach to be successful in testing the different parameters of a RAG search pipeline:\n\n1. Use a small set of the data you are familiar with for speed of iteration, like a few PDFs where they have relevant chunks for the search queries.\n2. Make a standard set of questions and answers about that subset of the data. For example, after reading the PDFs, write a list of questions and have your team agree on the answers.\n3. Create an automated evaluation system that scores how the retrieval does on each question. One way to do this is to take the answer from the RAG system and run it back through the LLM with a prompt that asks if this RAG result answers the question given the correct answer. This should be a “yes” or “no” answer. For example, if you have 25 questions on your documents, and the system gets 20 correct, you can use this to benchmark against other approaches. \n4. Ensure you use a different LLM for the evaluation than you used to encode the vector embeddings stored in the database. The evaluation LLM is typically a decoder-type of a model like GPT-4. One thing to remember is the cost of these evaluations when run repeatedly. Open-source models like Llama2 70B or the Deci AI LLM 6B, which can run on a single, smaller GPU, have roughly the same performance at a fraction of the cost.\n5. Run each test multiple times and average the score to smooth out the stochasticity of the LLM.\n\nHolding every option constant except one, you can quickly determine which parameters work best for your use case. A vector embedding pipeline like VectorFlow makes this especially easy on the ingestion side, where you can quickly try out different chunking strategies, chunk lengths, chunk overlaps, and open-source embedding models to see what leads to the best results. This is especially useful when your dataset has various file types and data sources that require custom logic. \n\nOnce the team knows what works for its use case, the vector embedding pipeline enables them to quickly move to production without having to redesign the system to consider things like reliability and monitoring. With technologies like VectorFlow and [Milvus](https://zilliz.com/what-is-milvus), which are open-source and platform-agnostic, the team can efficiently test across different environments while complying with privacy and security requirements. \n\n\n\n","title":"Ingesting Chaos: The MLOps Behind Handling Unstructured Data Reliably at Scale for RAG \n","metaData":{}},{"id":"why-and-when-you-need-a-purpose-built-vector-database.md","author":"James Luan","tags":["Engineering"],"desc":"This post provides an overview of vector search and its functioning, compare different vector search technologies, and explain why opting for a purpose-built vector database is crucial.","recommend":true,"canonicalUrl":"https://www.aiacceleratorinstitute.com/why-and-when-do-you-need-a-purpose-built-vector-database/","date":"2023-08-29T00:00:00.000Z","cover":"https://assets.zilliz.com/Why_you_need_a_real_vector_database2_20230830_075505_1_4b32582c87.png","href":"/blog/why-and-when-you-need-a-purpose-built-vector-database.md","content":"\n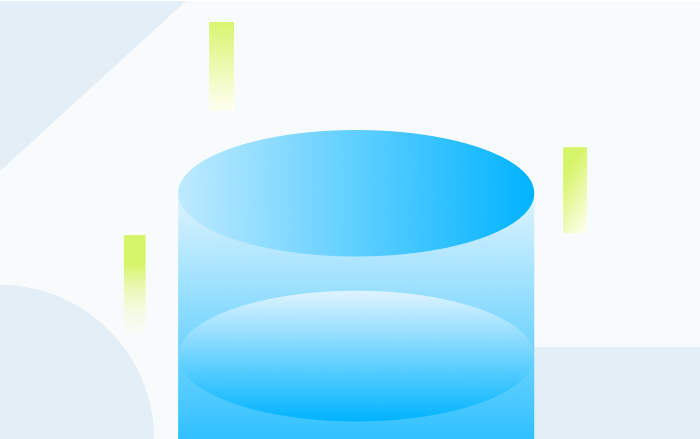\n\n*This article was originally published on [AIAI](https://www.aiacceleratorinstitute.com/why-and-when-do-you-need-a-purpose-built-vector-database/) and is reposted here with permission.*\n\n\nThe increasing popularity of [ChatGPT](https://zilliz.com/learn/ChatGPT-Vector-Database-Prompt-as-code) and other large language models (LLMs) has fueled the rise of vector search technologies, including purpose-built vector databases such as [Milvus](https://milvus.io/docs/overview.md) and [Zilliz Cloud](https://zilliz.com/cloud), vector search libraries such as [FAISS](https://zilliz.com/blog/set-up-with-facebook-ai-similarity-search-faiss), and vector search plugins integrated with traditional databases. However, choosing the best solution for your needs can be challenging. Like choosing between a high-end restaurant and a fast-food chain, selecting the right vector search technology depends on your needs and expectations.\n\nIn this post, I will provide an overview of vector search and its functioning, compare different vector search technologies, and explain why opting for a purpose-built vector database is crucial.\n\n## What is vector search, and how does it work?\n\n[Vector search](https://zilliz.com/blog/vector-similarity-search), also known as vector similarity search, is a technique for retrieving the top-k results that are most similar or semantically related to a given query vector among an extensive collection of dense vector data.\n\nBefore conducting similarity searches, we leverage neural networks to transform [unstructured data](https://zilliz.com/blog/introduction-to-unstructured-data), such as text, images, videos, and audio, into high-dimensional numerical vectors called embedding vectors. For example, we can use the pre-trained ResNet-50 convolutional neural network to transform a bird image into a collection of embeddings with 2,048 dimensions. Here, we list the first three and last three vector elements: `[0.1392, 0.3572, 0.1988, ..., 0.2888, 0.6611, 0.2909]`.\n\n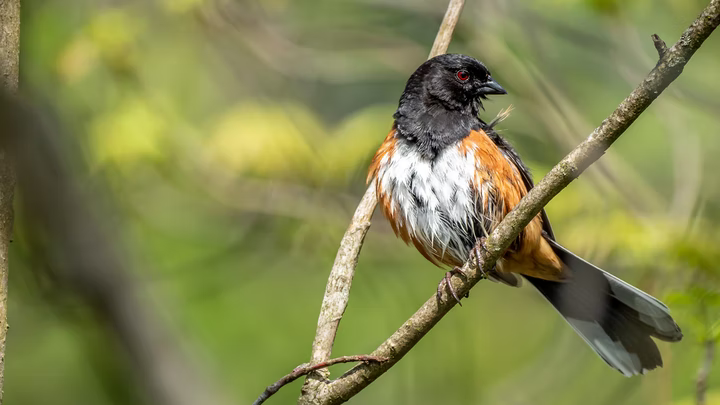\n\nAfter generating embedding vectors, vector search engines compare the spatial distance between the input query vector and the vectors in the vector stores. The closer they are in space, the more similar they are. \n\n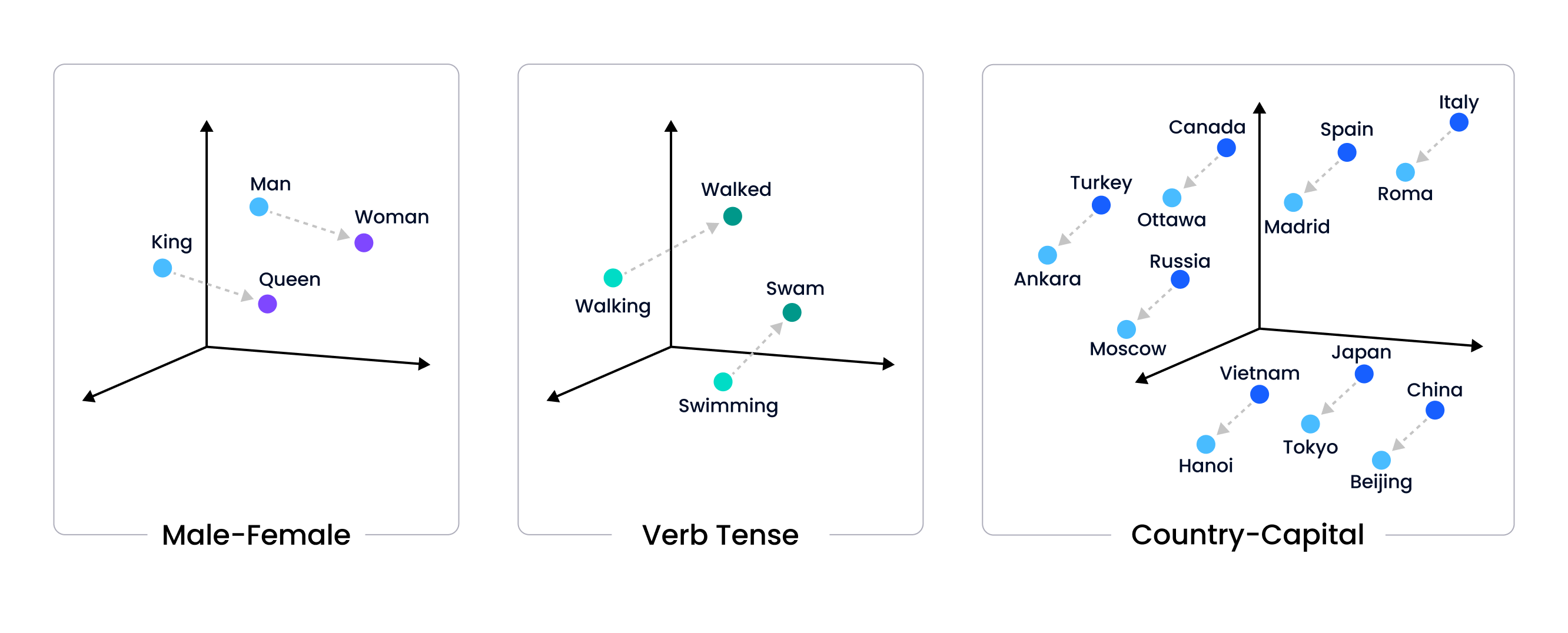\n\n## Popular vector search technologies \n\nMultiple vector search technologies are available in the market, including machine learning libraries like Python's NumPy, vector search libraries like FAISS, vector search plugins built on traditional databases, and specialized vector databases like Milvus and Zilliz Cloud. \n\n### Machine learning libraries \n\nUsing machine learning libraries is the easiest way to implement vector searches. For instance, we can use Python's NumPy to implement a nearest neighbor algorithm in less than 20 lines of code.\n\n```\nimport numpy as np\n\n# Function to calculate euclidean distance\ndef euclidean_distance(a, b):\nreturn np.linalg.norm(a - b)\n\n# Function to perform knn\ndef knn(data, target, k):\n# Calculate distances between target and all points in the data\ndistances = [euclidean_distance(d, target) for d in data]\n# Combine distances with data indices\ndistances = np.array(list(zip(distances, np.arange(len(data)))))\n\n# Sort by distance\nsorted_distances = distances[distances[:, 0].argsort()]\n\n# Get the top k closest indices\nclosest_k_indices = sorted_distances[:k, 1].astype(int)\n\n# Return the top k closest vectors\nreturn data[closest_k_indices]\n```\n\nWe can generate 100 two-dimensional vectors and find the nearest neighbor to the vector [0.5, 0.5].\n\n```\n# Define some 2D vectors\ndata = np.random.rand(100, 2)\n\n# Define a target vector\ntarget = np.array([0.5, 0.5])\n\n# Define k\nk = 3\n\n# Perform knn\nclosest_vectors = knn(data, target, k)\n\n# Print the result\nprint(\"The closest vectors are:\")\nprint(closest_vectors)\n```\n\nMachine learning libraries, such as Python's NumPy, offer great flexibility at a low cost. However, they do have some limitations. For instance, they can only handle a small amount of data and do not ensure data persistence.\n\nI only recommend using NumPy or other machine learning libraries for vector search when:\n\n- You need quick prototyping.\n- You don't care about data persistence.\n- Your data size is under one million, and you do not require scalar filtering.\n- You do not need high performance.\n\n### Vector search libraries\n\nVector search libraries can help you quickly build a high-performance prototype vector search system. FAISS is a typical example. It is open-source and developed by Meta for efficient similarity search and dense vector clustering. FAISS can handle vector collections of any size, even those that cannot be fully loaded into memory. Additionally, FAISS offers tools for evaluation and parameter tuning. Even though written in C++, FAISS provides a Python/NumPy interface.\n\nBelow is the code for an example vector search based on FAISS:\n\n```\nimport numpy as np\nimport faiss\n\n# Generate some example data\ndimension = 64 # dimension of the vector space\ndatabase_size = 10000 # size of the database\nquery_size = 100 # number of queries to perform\nnp.random.seed(123) # make the random numbers predictable\n\n# Generating vectors to index in the database (db_vectors)\ndb_vectors = np.random.random((database_size, dimension)).astype('float32')\n\n# Generating vectors for query (query_vectors)\nquery_vectors = np.random.random((query_size, dimension)).astype('float32')\n\n# Building the index\nindex = faiss.IndexFlatL2(dimension) # using the L2 distance metric\nprint(index.is_trained) # should return True\n\n# Adding vectors to the index\nindex.add(db_vectors)\nprint(index.ntotal) # should return database_size (10000)\n\n# Perform a search\nk = 4 # we want to see 4 nearest neighbors\ndistances, indices = index.search(query_vectors, k)\n\n# Print the results\nprint(\"Indices of nearest neighbors: \\n\", indices)\nprint(\"\\nL2 distances to the nearest neighbors: \\n\", distances)\n```\n\nVector search libraries such as FAISS are easy to use and fast enough to handle small-scale production environments with millions of vectors. You can enhance their query performance by utilizing quantization and GPUs and reducing data dimensions.\n\nHowever, these libraries have some limitations when used in production. For example, FAISS does not support real-time data addition and deletion, remote calls, multiple languages, scalar filtering, scalability, or disaster recovery.\n\n### Different types of vector databases \n\nVector databases have emerged to address the limitations of the libraries above, providing a more comprehensive and practical solution for production applications.\n\nFour types of vector databases are available on the battlefield:\n\n- Existing relational or columnar databases that incorporate a vector search plugin. PG Vector is an example. \n- Traditional inverted index search engines with support for dense vector indexing. [ElasticSearch](https://zilliz.com/comparison/elastic-vs-milvus) is an example.\n- Lightweight vector databases built on vector search libraries. Chroma is an example.\n- **Purpose-built vector databases**. This type of database is specifically designed and optimized for vector searching from the bottom up. Purpose-built vector databases typically offer more advanced features, including distributed computing, disaster recovery, and data persistence. [Milvus](https://zilliz.com/what-is-milvus) is a primary example.\n\nNot all vector databases are created equal. Each stack has unique advantages and limitations, making them more or less suitable for different applications. \n\nI prefer specialized vector databases over other solutions because they are the most efficient and convenient option, offering numerous unique benefits. In the following sections, I will use Milvus as an example to explain the reasons for my preference. \n\n## Key benefits of purpose-built vector databases\n\n[Milvus](https://milvus.io/) is an open-source, distributed, purpose-built vector database that can store, index, manage, and retrieve billions of embedding vectors. It is also one of the most popular vector databases for [LLM retrieval augmented generation](https://zilliz.com/use-cases/llm-retrieval-augmented-generation). As an exemplary instance of purpose-built vector databases, Milvus shares many unique advantages with its counterparts.\n\n### Data Persistence and Cost-Effective Storage\n\nWhile preventing data loss is the minimum requirement for a database, many single-machine and lightweight vector databases do not prioritize data reliability. By contrast, purpose-built distributed vector databases like [Milvus](https://zilliz.com/what-is-milvus) prioritize system resilience, scalability, and data persistence by separating storage and computation. \n\nMoreover, most vector databases that utilize approximate nearest neighbor (ANN) indexes need a lot of memory to perform vector searching, as they load ANN indexes purely into memory. However, Milvus supports disk indexes, making storage over ten times more cost-effective than in-memory indexes.\n\n### Optimal Query Performance \n\nA specialized vector database provides optimal query performance compared to other vector search options. For example, Milvus is ten times faster at handling queries than vector search plugins. Milvus uses the [ANN algorithm](https://zilliz.com/glossary/anns) instead of the KNN brutal search algorithm for faster vector searching. Additionally, it shards its indexes, reducing the time it takes to construct an index as the data volume increases. This approach enables Milvus to easily handle billions of vectors with real-time data additions and deletions. In contrast, other vector search add-ons are only suitable for scenarios with fewer than tens of millions of data and infrequent additions and deletions.\n\nMilvus also supports GPU acceleration. Internal testing shows that GPU-accelerated vector indexing can achieve 10,000+ QPS when searching tens of millions of data, which is at least ten times faster than traditional CPU indexing for single-machine query performance.\n\n### System Reliability \n\nMany applications use vector databases for online queries that require low query latency and high throughput. These applications demand single-machine failover at the minute level, and some even require cross-region disaster recovery for critical scenarios. Traditional replication strategies based on Raft/Paxos suffer from serious resource waste and need help to pre-shard the data, leading to poor reliability. In contrast, Milvus has a distributed architecture that leverages K8s message queues for high availability, reducing recovery time and saving resources. \n\n### Operability and Observability\n\nTo better serve enterprise users, vector databases must offer a range of enterprise-level features for better operability and observability. Milvus supports multiple deployment methods, including K8s Operator and Helm chart, docker-compose, and pip install, making it accessible to users with different needs. Milvus also provides a monitoring and alarm system based on Grafana, Prometheus, and Loki, improving its observability. With a distributed cloud-native architecture, Milvus is the industry's first vector database to support multi-tenant isolation, RBAC, quota limiting, and rolling upgrades. All of these approaches make managing and monitoring Milvus much simpler.\n\n## Getting started with Milvus in 3 simple steps within 10 minutes \n\nBuilding a vector database is a complex task, but using one is as simple as using Numpy and FAISS. Even students unfamiliar with AI can implement vector search based on Milvus in just ten minutes. To experience highly scalable and high-performance vector search services, follow these three steps:\n\n- Deploy Milvus on your server with the help of the [Milvus deployment document](https://milvus.io/docs/install_standalone-docker.md).\n- Implement vector search with just 50 lines of code by referring to the [Hello Milvus document](https://milvus.io/docs/example_code.md).\n- Explore the [example documents of Towhee](https://github.com/towhee-io/examples/) to gain insight into popular [use cases of vector databases](https://zilliz.com/use-cases).\n","title":"Why and When Do You Need a Purpose-Built Vector Database?","metaData":{}},{"id":"unveiling-milvus-2-3-milestone-release-offering-support-for-gpu-arm64-cdc-and-other-features.md","author":"Owen Jiao, Fendy Feng","desc":"Milvus 2.3 is a milestone release with numerous highly anticipated features, including support for GPU, Arm64, upsert, change data capture, ScaNN index, and range search. It also introduces improved query performance, more robust load balancing and scheduling, and better observability and operability.","tags":["News"],"recommend":true,"canonicalUrl":"https://milvus.io/blog/unveiling-milvus-2-3-milestone-release-offering-support-for-gpu-arm64-cdc-and-other-features.md","date":"2023-08-28T00:00:00.000Z","cover":"https://assets.zilliz.com/Milvus_2_3_Milvus_io_2e3b0eb55c.jpeg","href":"/blog/unveiling-milvus-2-3-milestone-release-offering-support-for-gpu-arm64-cdc-and-other-features.md","content":"\n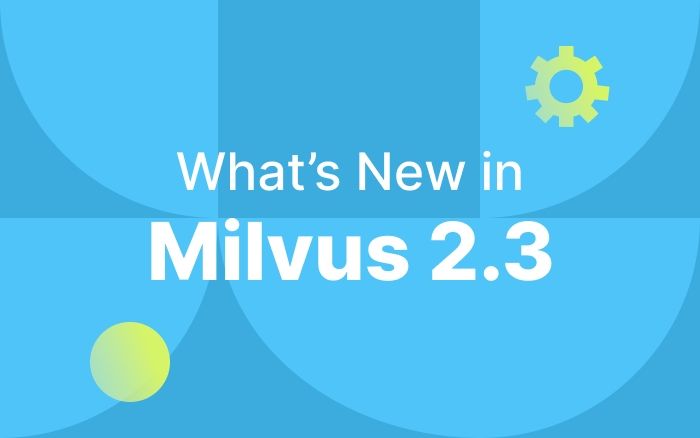\n\nExciting news! After eight months of concerted effort, we're thrilled to announce the release of Milvus 2.3, a milestone version that brings numerous highly anticipated features, including support for GPU, Arm64, upsert, change data capture, ScaNN index, and MMap technology. Milvus 2.3 also introduces improved query performance, more robust load balancing and scheduling, and better observability and operability. \n\nJoin me to look at these new features and enhancements and learn how you can benefit from this release. \n\n## Support for GPU index that leads to 3-10 times faster in QPS \n\nGPU index is a highly anticipated feature in the Milvus community. Thanks to a great collaboration with the Nvidia engineers, Milvus 2.3 has supported GPU indexing with the robust RAFT algorithm added to Knowhere, the Milvus index engine. With GPU support, Milvus 2.3 is more than three times faster in QPS than older versions using the CPU HNSW index and almost ten times faster for specific datasets that require heavy computation. \n\n## Arm64 support to accommodate growing user demand\n\nArm CPUs are becoming increasingly popular among cloud providers and developers. To meet this growing demand, Milvus now provides Docker images for the ARM64 architecture. With this new CPU support, MacOS users can build their applications with Milvus more seamlessly. \n\n## Upsert support for better user experience \n\nMilvus 2.3 introduces a notable enhancement by supporting the upsert operation. This new functionality allows users to update or insert data seamlessly and empowers them to perform both operations in a single request through the Upsert interface. This feature streamlines data management and brings efficiency to the table.\n\n**Note**:\n- The upsert feature does not apply to auto-increment IDs.\n- Upsert is implemented as a combination of `delete` and `insert`, which may result in some performance loss. We recommend using `insert` if you use Milvus in write-heavy scenarios.\n\n## Range search for more accurate results \nMilvus 2.3 allows users to specify the distance between the input vector and the vectors stored in Milvus during a query. Milvus then returns all matching results within the set range. Below is an example of specifying the search distance using the range search feature.\n\n```\n// add radius and range_filter to params in search_params\nsearch_params = {\"params\": {\"nprobe\": 10, \"radius\": 10, \"range_filter\" : 20}, \"metric_type\": \"L2\"}\nres = collection.search(\nvectors, \"float_vector\", search_params, topK,\n\"int64 \u003e 100\", output_fields=[\"int64\", \"float\"]\n)\n```\n\nIn this example, the user requires Milvus to return vectors within a distance of 10 to 20 units from the input vector.\n\n**Note**: Different distance metrics vary in how they calculate distances, resulting in distinct value ranges and sorting strategies. Therefore, it is essential to understand their characteristics before using the range search feature.\n\n## ScaNN index for faster query speed \n\nMilvus 2.3 now supports the ScaNN index, an open-source [approximate nearest neighbor (ANN)](https://zilliz.com/glossary/anns) index developed by Google. ScaNN index has demonstrated superior performance in various benchmarks, outperforming HNSW by around 20% and being approximately seven times faster than IVFFlat. With the support for the ScaNN index, Milvus achieves much faster query speed compared to older versions.\n\n## Growing index for stable and better query performance \n\nMilvus includes two categories of data: indexed data and streaming data. Milvus can use indexes to search indexed data quickly but can only brutely search streaming data row by row, which can impact performance. Milvus 2.3 introduces the Growing Index, which automatically creates real-time indexes for streaming data to improve query performance.\n\n## Iterator for data retrieval in batches \n\nIn Milvus 2.3, Pymilvus has introduced an iterator interface that allows users to retrieve more than 16,384 entities in a search or range search. This feature is handy when users need to export tens of thousands or even more vectors in batches.\n\n## Support for MMap for increased capacity\n\nMMap is a UNIX system call used to map files and other objects into memory. Milvus 2.3 supports MMap, which enables users to load data onto local disks and map it to memory, thereby increasing single-machine capacity.\n\nOur testing results indicate that using MMap technology, Milvus can double its data capacity while limiting performance degradation to within 20%. This approach significantly reduces overall costs, making it particularly beneficial for users on a tight budget who do not mind compromising performance.\n\n## CDC support for higher system availability \n\nChange Data Capture (CDC) is a commonly used feature in database systems that captures and replicates data changes to a designated destination. With the CDC feature, Milvus 2.3 enables users to synchronize data across data centers, back up incremental data, and seamlessly migrate data, making the system more available. \n\nIn addition to the features above, Milvus 2.3 introduces a count interface to accurately calculate the number of rows of data stored in a collection in real-time, supports the Cosine metric to measure vector distance, and more operations on JSON arrays. For more features and detailed information, refer to [Milvus 2.3 release notes](https://milvus.io/docs/release_notes.md). \n\n## Enhancements and bug fixes \n\nIn addition to new features, Milvus 2.3 includes many improvements and bug fixes for older versions.\n\n### Improved performance for data filtering\n\nMilvus performs scalar filtering before vector searching in hybrid scalar and vector data queries to achieve more accurate results. However, the indexing performance may decline if the user has filtered out too much data after scalar filtering. In Milvus 2.3, we optimized the filtering strategy of HNSW to address this issue, resulting in improved query performance.\n\n### Increased multi-core CPU usage \n\nApproximate nearest search (ANN) is a computationally intensive task that requires massive CPU resources. In previous releases, Milvus could only utilize around 70% of the available multi-core CPU resources. However, with the latest release, Milvus has overcome this limitation and can fully utilize all available multi-core CPU resources, resulting in improved query performance and reduced resource waste.\n\n### Refactored QueryNode\n\nQueryNode is a crucial component in Milvus that is responsible for vector searching. However, in older versions, QueryNode had complex states, duplicate message queues, an unorganized code structure, and non-intuitive error messages.\n\nIn Milvus 2.3, we've upgraded QueryNode by introducing a stateless code structure and removing the message queue for deleting data. These updates result in less resource waste and faster and more stable vector searching.\n\n### Enhanced message queues based on NATS\n\nWe built Milvus on a log-based architecture, and in previous versions, we used Pulsar and Kafka as the core log brokers. However, this combination faced three key challenges:\n\n- It was unstable in multi-topic situations.\n- It consumed resources when idle and struggled to deduplicate messages.\n- Pulsar and Kafka are closely tied to the Java ecosystem, so their community rarely maintains and updates their Go SDKs.\n\nTo address these problems, we have combined NATS and Bookeeper as our new log broker for Milvus, which fits users' needs better.\n\n### Optimized load balancer\n\nMilvus 2.3 has adopted a more flexible load-balancing algorithm based on the system's real loads. This optimized algorithm lets users quickly detect node failures and unbalanced loads and adjust schedulings accordingly. According to our testing results, Milvus 2.3 can detect faults, unbalanced load, abnormal node status, and other events within seconds and make adjustments promptly.\n\nFor more information about Milvus 2.3, refer to [Milvus 2.3 release notes](https://milvus.io/docs/release_notes.md). \n\n## Tool upgrades\n\nWe have also upgraded Birdwatcher and Attu, two valuable tools for operating and maintaining Milvus, along with Milvus 2.3.\n\n### Birdwatcher update \n\nWe've upgraded [Birdwatcher](https://github.com/milvus-io/birdwatcher), the debug tool of Milvus, introducing numerous features and enhancements, including: \n- RESTful API for seamless integration with other diagnostic systems.\n- PProf command support to facilitate integration with the Go pprof tool.\n- Storage usage analysis capabilities.\n- Efficient log analysis functionality.\n- Support for viewing and modifying configurations in etcd.\n\n### Attu update \n\nWe’ve launched a brand-new interface for [Attu](https://zilliz.com/attu), an all-in-one vector database administration tool. The new interface has a more straightforward design and is easier to understand.\n\n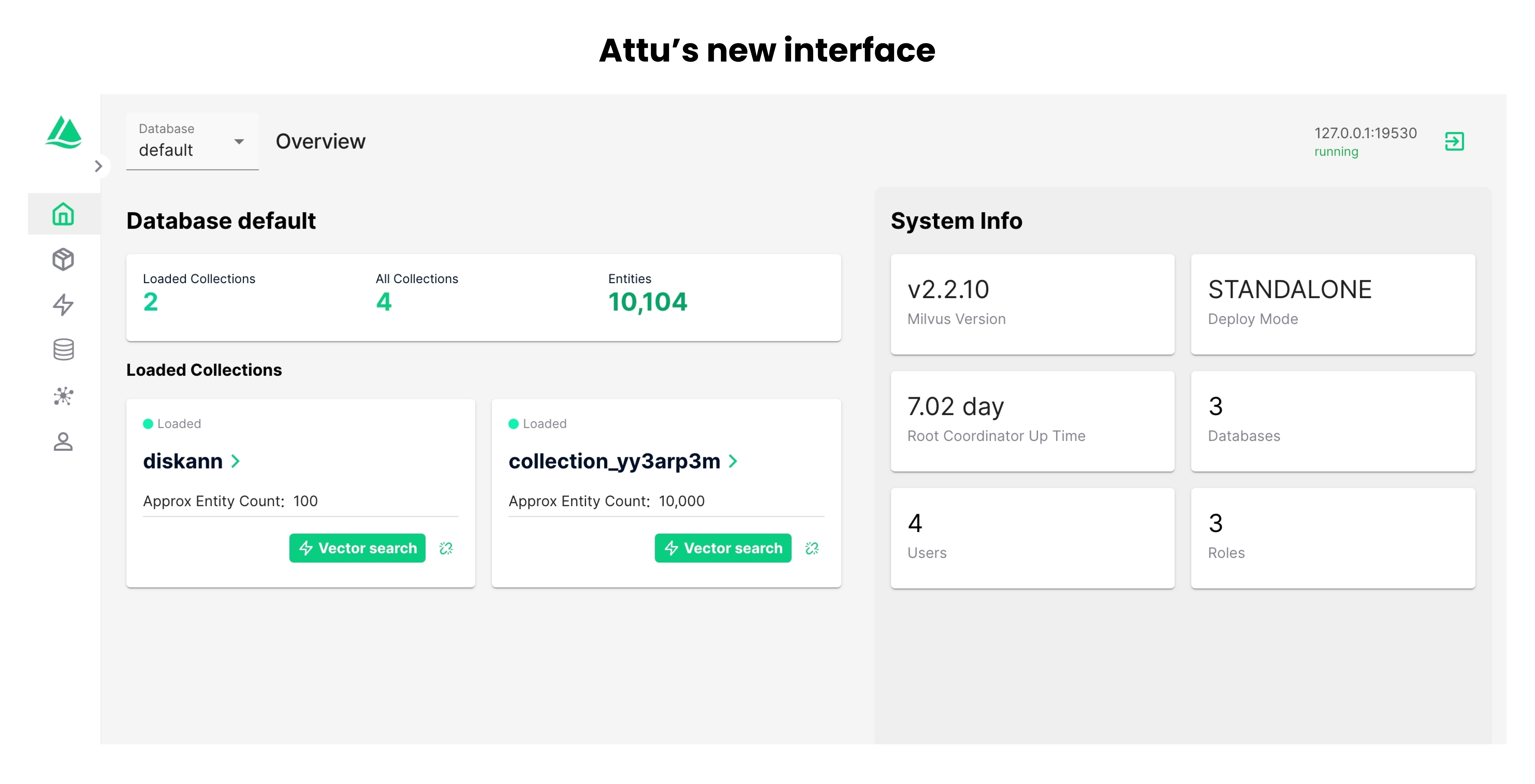\n\nFor more details, refer to [Milvus 2.3 release notes](https://milvus.io/docs/release_notes.md). \n\n## Let’s keep in touch!\n\nIf you have questions or feedback about Milvus, please don't hesitate to contact us through [Twitter](https://twitter.com/milvusio) or [LinkedIn](https://www.linkedin.com/company/the-milvus-project). You're also welcome to join our [Slack channel](https://milvus.io/slack/) to chat with our engineers and the community directly or check out our [Tuesday office hours](https://us02web.zoom.us/meeting/register/tZ0pcO6vrzsuEtVAuGTpNdb6lGnsPBzGfQ1T#/registration)!\n","title":"Unveiling Milvus 2.3: A Milestone Release Offering Support for GPU, Arm64, CDC, and Many Other Highly Anticipated Features","metaData":{}},{"id":"milvus-2-2-12-easier-access-faster-vector-search-speeds-better-user-experience.md","author":"Owen Jiao, Fendy Feng","tags":["News"],"recommend":true,"canonicalUrl":"https://milvus.io/blog/milvus-2-2-12-easier-access-faster-vector-search-speeds-better-user-experience.md","date":"2023-07-28T00:00:00.000Z","cover":"https://assets.zilliz.com/What_s_New_in_2_2_12_20230720_143424_7d19280738.png","href":"/blog/milvus-2-2-12-easier-access-faster-vector-search-speeds-better-user-experience.md","content":"\n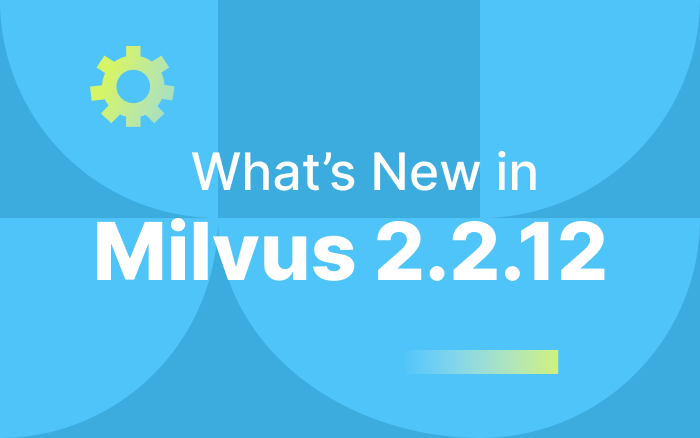\n\nWe are thrilled to announce the latest release of Milvus 2.2.12. This update includes multiple new features, such as support for RESTful API, `json_contains` function, and vector retrieval during ANN searches in response to user feedback. We have also streamlined the user experience, enhanced the vector searching speeds, and resolved many issues. Let's delve into what we can expect from Milvus 2.2.12.\n\n## Support for RESTful API\n\nMilvus 2.2.12 now supports RESTful API, which enables users to access Milvus without installing a client, making client-server operations effortless. Additionally, deploying Milvus has become more convenient because the Milvus SDK and RESTful API share the same port number. \n\n**Note**: We still recommend using the SDK to deploy Milvus for advanced operations or if your business is latency sensitive. \n\n## Vector retrieval during ANN searches\n\nIn earlier versions, Milvus did not allow vector retrieval during approximate nearest neighbor (ANN) searches to prioritize performance and memory usage. As a result, retrieving raw vectors had to be split into two steps: performing the ANN search and then querying the raw vectors based on their IDs. This approach increased development costs and made it harder for users to deploy and adopt Milvus.\n\nWith Milvus 2.2.12, users can retrieve raw vectors during ANN searches by setting the vector field as an output field and querying in HNSW-, DiskANN-, or IVF-FLAT-indexed collections. In addition, users can expect a much faster vector retrieval speed. \n\n## Support for operations on JSON arrays\n\nWe recently added support for JSON in Milvus 2.2.8. Since then, users have sent numerous requests to support additional JSON arrays operations, such as inclusion, exclusion, intersection, union, difference, and more. In Milvus 2.2.12, we've prioritized supporting the `json_contains` function to enable the inclusion operation. We will continue to add support for other operators in future versions.\n\n## Enhancements and bug fixes \n\nIn addition to introducing new features, Milvus 2.2.12 has improved its vector search performance with reduced overhead, making it easier to handle extensive topk searches. Moreover, it enhances the write performance in partition-key-enabled and multi-partition situations and optimizes CPU usage for large machines. \nThis update addresses various issues: excessive disk usage, stuck compaction, infrequent data deletions, and bulk insertion failures. For further information, please refer to the [Milvus 2.2.12 Release Notes](https://milvus.io/docs/release_notes.md#2212).\n\n## Let's keep in touch!\n\nIf you have questions or feedback about Milvus, please don't hesitate to contact us through [Twitter](https://twitter.com/milvusio) or [LinkedIn](https://www.linkedin.com/company/the-milvus-project). You're also welcome to join our [Slack channel](https://milvus.io/slack/) to chat with our engineers and the community directly or check out our [Tuesday office hours](https://us02web.zoom.us/meeting/register/tZ0pcO6vrzsuEtVAuGTpNdb6lGnsPBzGfQ1T#/registration)!\n","title":"Milvus 2.2.12: Easier Access, Faster Vector Search Speeds, and Better User Experience \n","metaData":{}},{"id":"milvus-2210-and-2211-enhanced-stability-and-better-user-experience.md","author":"Fendy Feng, Owen Jiao","tags":["News"],"desc":"introducing new features and improvements of Milvus 2.2.10 and 2.2.11","recommend":true,"metaTitle":"Milvus 2.2.10 \u0026 2.2.11 Enhanced System Stability and User Experience","canonicalUrl":"https://milvus.io/blog/milvus-2210-and-2211-enhanced-stability-and-better-user-experience.md","date":"2023-07-06T00:00:00.000Z","cover":"https://assets.zilliz.com/What_s_New_in_Milvus_2_2_10_and_2_2_11_5018946465.png","href":"/blog/milvus-2210-and-2211-enhanced-stability-and-better-user-experience.md","content":"\n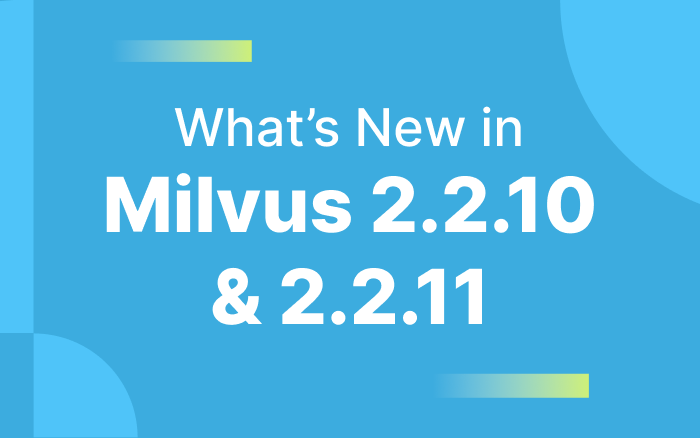\n\n\nGreetings, Milvus fans! We're excited to announce that we have just released Milvus 2.2.10 and 2.2.11, two minor updates primarily focusing on bug fixes and overall performance improvement. You can expect a more stable system and a better user experience with the two updates. Let’s take a quick look at what is new in these two releases. \n\n## Milvus 2.2.10\n\nMilvus 2.2.10 has fixed occasional system crashes, accelerated loading and indexing, reduced memory usage in data nodes, and made many other improvements. Below are some notable changes: \n\n- Replaced the old CGO payload writer with a new one written in pure Go, reducing memory usage in data nodes.\n- Added `go-api/v2` to the `milvus-proto` file to prevent confusion with different `milvus-proto` versions. \n- Upgraded Gin from version 1.9.0 to 1.9.1 to fix a bug in the `Context.FileAttachment` function.\n- Added role-based access control (RBAC) for the FlushAll and Database APIs.\n- Fixed a random crash caused by the AWS S3 SDK.\n- Improved the loading and indexing speeds. \n\nFor more details, see [Milvus 2.2.10 Release Notes](https://milvus.io/docs/release_notes.md#2210). \n\n## Milvus 2.2.11\n\nMilvus 2.2.11 has resolved various issues to improve the system's stability. It has also improved its performance in monitoring, logging, rate limiting, and interception of cross-cluster requests. See below for the highlights of this update. \n\n- Added an interceptor to the Milvus GRPC server to prevent any issues with Cross-Cluster routing.\n- Added error codes to the minio chunk manager to make diagnosing and fixing errors easier. \n- Utilized a singleton coroutine pool to avoid wasting coroutines and maximize the use of resources. \n- Reduced the disk usage for RocksMq to one-tenth of its original level by enabling zstd compression.\n- Fixed occasional QueryNode panic during loading.\n- Rectified the read request throttling issue caused by miscalculating queue length twice.\n- Fixed issues with GetObject returning null values on MacOS.\n- Fixed a crash caused by incorrect use of the noexcept modifier.\n\nFor more details, see [Milvus 2.2.11 Release Notes](https://milvus.io/docs/release_notes.md#2211). \n\n## Let’s keep in touch!\n\nIf you have questions or feedback about Milvus, please don't hesitate to contact us through [Twitter](https://twitter.com/milvusio) or [LinkedIn](https://www.linkedin.com/company/the-milvus-project). You're also welcome to join our [Slack channel](https://milvus.io/slack/) to chat with our engineers and the community directly or check out our [Tuesday office hours](https://us02web.zoom.us/meeting/register/tZ0pcO6vrzsuEtVAuGTpNdb6lGnsPBzGfQ1T#/registration)!\n\n","title":"Milvus 2.2.10 \u0026 2.2.11: Minor Updates for Enhanced System Stability and User Experience","metaData":{}},{"id":"milvus-surpasses-20000-GitHub-stars.md","author":"Yujian Tang","tags":["News"],"recommend":true,"canonicalUrl":"https://milvus.io/blog/milvus-surpasses-20000-github-stars.md","date":"2023-06-29T00:00:00.000Z","cover":"https://assets.zilliz.com/Milvus_hit_20_000_stars_284ed694d2.png","href":"/blog/milvus-surpasses-20000-GitHub-stars.md","content":"\n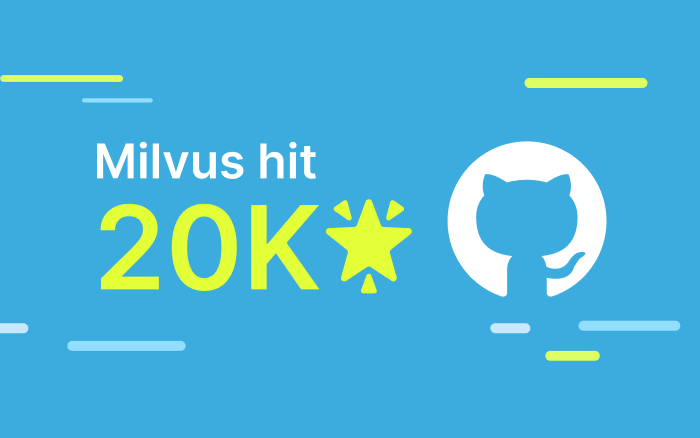\n\nLet's break out the confetti and celebrate! \n\n[Milvus](https://github.com/milvus-io/milvus) has achieved a stellar milestone - surpassing 20,000 stars on GitHub! We couldn't have achieved it without the support and feedback of our loyal fans and community members. Thank you so much. \n\nTo share the joy, we've put together a list of critical topics from the broader Milvus community, including:\n \n- Six prominent projects that utilize Milvus\n- Five integrations with other open-source projects\n- Five well-known use cases of Milvus\n- Five excellent upcoming features\n\n## Six LLM projects that utilize Milvus\n\n### PaperGPT\n\n[PaperGPT](http://papergpt.bio) is a search tool for biomedical papers powered by an LLM and a vector database. It leverages the PubMed database and technologies like SentenceTransformers and Zilliz Cloud for efficient search. \n\n### NoticeAI\n\nNoticeAI helps companies automate their customer support process. It utilizes Milvus and LangChain to track customer support questions, update the knowledge base, and decide whether or not to involve a human.\n\n### Search.anything.io \n\n[Search Anything](http://search.anything.io) leverages LLMs and Milvus to provide a better search experience. It takes a text description from a user and finds the corresponding images on the web.\n\n### IkuStudies\n\n[IkuStudies](https://ikustudies.xyz/) is a project allowing users to search through homophones in 28 languages. It uses Milvus as the vector store to find similarities between the words. \n\n### AssistLink AI\n\n[AssistLink AI](https://www.linkedin.com/company/assistlink/about/) is a Seattle-based startup that uses Milvus and LLaMa 65bn to store variables for a Government Assistance system.\n\n### OSS Chat\n\n[OSS Chat](http://osschat.io) allows you to chat with open-source software. It uses Milvus to inject domain knowledge about open-source projects and uses [GPTCache](https://zilliz.com/blog/Caching-LLM-Queries-for-performance-improvements) to cache frequently asked questions to reduce LLM costs. \n\n## Five AI projects Milvus integrates with\n\n### LlamaIndex \n\n[LlamaIndex](https://github.com/jerryjliu/llama_index) is a data framework for LLM applications that provides data connectors to link external data sources with an LLM. LlamaIndex enables you to inject your private data or domain-specific knowledge directly into your LLM application. \n\n### LangChain/LangChainJS\n\n[LangChain](https://github.com/hwchase17/langchain) is a library designed to accelerate LLM application development, offering features such as prompt management, chains, data-augmented generation, memory, and more. \n\n### ChatGPT Retrieval Plugin \n\nThe [ChatGPT Retrieval Plugin](https://github.com/openai/chatgpt-retrieval-plugin) from OpenAI enables semantic search and retrieval of personal or organizational documents using natural language queries, allowing developers to deploy their plugin and register it with ChatGPT. \n\n### Haystack \n\n[Haystack](https://github.com/deepset-ai/haystack) is an end-to-end NLP framework for building NLP applications powered by LLMs, transformer models, vector search, and other technologies for tasks such as question answering, semantic document search, and building complex decision-making. \n\n### GPTCache\n\nLLM API calls can be both expensive and slow. [GPTCache](https://github.com/zilliztech/gptcache) can remedy both problems by providing a semantic cache for LLM queries. \n\n## Five well-known use cases of Milvus\n\n### LLM augmentation\n\nLLMs or LLM applications have three major problems: lack of domain-specific data, outdated data, and high costs. Milvus can solve all three of these problems by allowing you to inject external data and serve as a cache for frequent queries.\n\n### Anomaly detection\n\nAnomalous data is significantly different from the rest in a given set. Vector databases like Milvus are beneficial for conducting similarity searches, making it easier to identify such anomalous data. \n\n### Recommender systems\n\nA recommender system recommends people items similar to what they already enjoy. It is a classic example of Milvus accelerating this process by performing similarity searches. \n\n### Semantic search\n\nKeyword search doesn’t do it when you want to search text to find things with similar meanings. That’s where semantic search comes in and where Milvus can help. Milvus allows you to compare the intentions behind the text, not just the words themselves.\n\n### Automatic data labeling\n\nGot labeled data and need to mark more? By storing the vector representations in Milvus, you can automatically detect the most similar data points in your new data and apply the appropriate labels.\n\n## Five awesome upcoming features\n\n### NVIDIA GPU support\n\nGPU support is coming in Milvus 2.3 and is already available in the most [recent beta release](https://github.com/milvus-io/milvus/releases/tag/v2.3.0-beta)! \n\n### Delete by Expression\n\nYou can already perform scalar/metadata filtering via boolean expressions in Milvus. The Delete by Expression feature is also coming.\n\n### Change Data Capture (CDC)\n\nCDC is a technique to capture and track changes made to your Milvus instance in real-time.\n\n### Range Search\n\nRange search, also known in some circles as epsilon search, will allow you to find all vectors within a certain distance of your query vector.\n\n### Fast Scan\n\n4-bit quantization and FastScan were added to FAISS recently and will also be coming to Milvus.\n\n## Looking forward to Milvus reaching even greater heights!\n\nWe would like to give our most enormous thanks to our users, community members, ecosystem partners, and stargazers for your continued support, feedback, and contribution. Looking forward to Milvus reaching even greater heights! \n\n\n","title":"A Stellar Milestone: Milvus Surpasses 20,000 Stars on GitHub","metaData":{}},{"id":"conversational-memory-in-langchain.md","author":"Yujian Tang","tags":["Engineering"],"recommend":true,"canonicalUrl":"https://milvus.io/blog/conversational-memory-in-langchain.md","date":"2023-06-06T00:00:00.000Z","cover":"https://assets.zilliz.com/Conversational_Memory_in_Lang_Chain_7c1b4b7ba9.png","href":"/blog/conversational-memory-in-langchain.md","content":"\n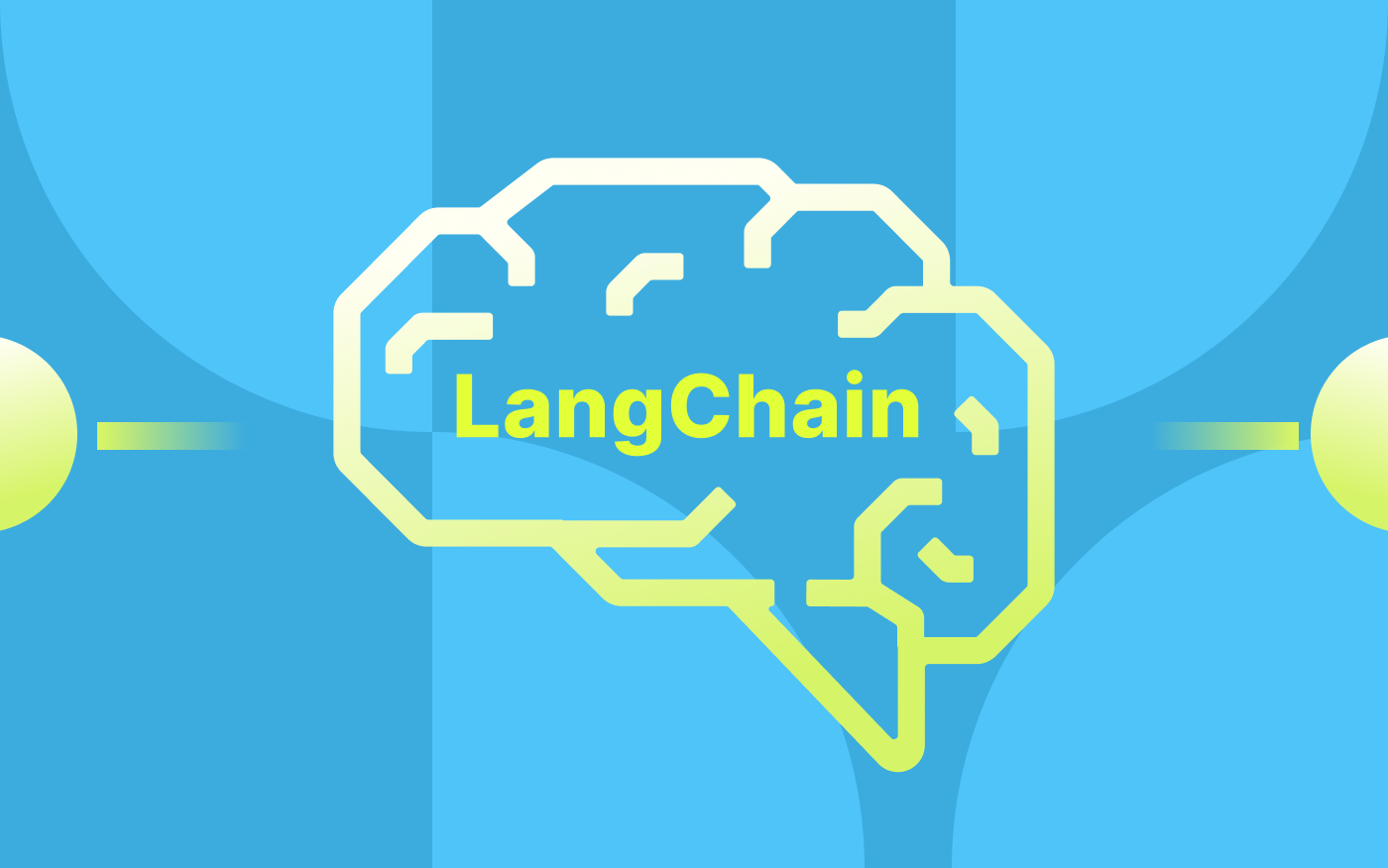\n\n\nLangChain is a robust framework for building LLM applications. However, with that power comes quite a bit of complexity. LangChain provides many ways to prompt an LLM and essential features like conversational memory. Conversational memory offers context for the LLM to remember your chat.\n\nIn this post, we look at how to use conversational memory with LangChain and Milvus. To follow along, you need to `pip` install four libraries and an OpenAI API key. The four libraries you need can be installed by running `pip install langchain milvus pymilvus python-dotenv`. Or executing the first block in the [CoLab Notebook](https://colab.research.google.com/drive/11p-u8nKqrQYePlXR0HiSrUapmKLD0QN9?usp=sharing) for this article.\n\nIn this post, we’ll learn about:\n- Conversational Memory with LangChain\n- Setting Up Conversation Context\n- Prompting the Conversational Memory with LangChain\n- LangChain Conversational Memory Summary\n\n## Conversational Memory with LangChain\n\nIn the default state, you interact with an LLM through single prompts. Adding memory for context, or “conversational memory” means you no longer have to send everything through one prompt. LangChain offers the ability to store the conversation you’ve already had with an LLM to retrieve that information later.\n\nTo set up persistent conversational memory with a vector store, we need six modules from LangChain. First, we must get the `OpenAIEmbeddings` and the `OpenAI` LLM. We also need `VectorStoreRetrieverMemory` and the LangChain version of `Milvus` to use a vector store backend. Then we need `ConversationChain` and `PromptTemplate` to save our conversation and query it.\n\nThe `os`, `dotenv`, and `openai` libraries are mainly for operational purposes. We use them to load and use the OpenAI API key. The final setup step is to spin up a local [Milvus Lite](https://milvus.io/docs/milvus_lite.md) instance. We do this through using the `default_server` from the Milvus Python package.\n\n```\nfrom langchain.embeddings.openai import OpenAIEmbeddings\nfrom langchain.llms import OpenAI\nfrom langchain.memory import VectorStoreRetrieverMemory\nfrom langchain.chains import ConversationChain\nfrom langchain.prompts import PromptTemplate\nfrom langchain.vectorstores import Milvus\nembeddings = OpenAIEmbeddings()\n\n\nimport os\nfrom dotenv import load_dotenv\nimport openai\nload_dotenv()\nopenai.api_key = os.getenv(\"OPENAI_API_KEY\")\n\n\nfrom milvus import default_server\ndefault_server.start()\n```\n\n## Setting Up Conversation Context\n\nNow that we have all our prerequisites set up, we can proceed to create our conversational memory. Our first step is to create a connection to the Milvus server using LangChain. Next, we use an empty dictionary to create our LangChain Milvus collection. In addition, we pass in the embeddings we created above and the connection details for the Milvus Lite server.\n\nTo use the vector database for conversational memory, we need to instantiate it as a retriever. We only retrieve the top 1 result for this case, setting `k=1`. The last conversational memory setup step is using the `VectorStoreRetrieverMemory` object as our conversational memory through the retriever and vector database connection we just set up. \n\nTo use our conversational memory, it has to have some context in it. So let’s give the memory some context. For this example, we give five pieces of information. Let’s store my favorite snack (chocolate), sport (swimming), beer (Guinness), dessert (cheesecake), and musician (Taylor Swift). Each entry is saved to the memory through the `save_context` function.\n\n```\nvectordb = Milvus.from_documents(\n {},\n embeddings,\n connection_args={\"host\": \"127.0.0.1\", \"port\": default_server.listen_port})\nretriever = Milvus.as_retriever(vectordb, search_kwargs=dict(k=1))\nmemory = VectorStoreRetrieverMemory(retriever=retriever)\nabout_me = [\n {\"input\": \"My favorite snack is chocolate\",\n \"output\": \"Nice\"},\n {\"input\": \"My favorite sport is swimming\",\n \"output\": \"Cool\"},\n {\"input\": \"My favorite beer is Guinness\",\n \"output\": \"Great\"},\n {\"input\": \"My favorite dessert is cheesecake\",\n \"output\": \"Good to know\"},\n {\"input\": \"My favorite musician is Taylor Swift\",\n \"output\": \"Same\"}\n]\nfor example in about_me:\n memory.save_context({\"input\": example[\"input\"]}, {\"output\": example[\"output\"]})\n```\n\n## Prompting the Conversational Memory with LangChain\n\nIt’s time to look at how we can use our conversational memory. Let’s start by connecting to the OpenAI LLM through LangChain. We use a temperature of 0 to indicate that we don’t want our LLM to be creative. \n\nNext, we create a template. We tell the LLM that it is engaged in a friendly conversation with a human and inserts two variables. The `history` variable provides the context from the conversational memory. The `input` variable provides the current input. We use the `PromptTemplate` object to insert these variables.\n\nWe use the `ConversationChain` object to combine our prompt, LLM, and memory. Now we are ready to check the memory of our conversation by giving it some prompts. We start by telling the LLM that our name is Gary, the main rival in the Pokemon series (everything else in the conversational memory is a fact about me).\n\n```\nllm = OpenAI(temperature=0) # Can be any valid LLM\n_DEFAULT_TEMPLATE = \"\"\"The following is a friendly conversation between a human and an AI. The AI is talkative and provides lots of specific details from its context. If the AI does not know the answer to a question, it truthfully says it does not know.\n\n\nRelevant pieces of previous conversation:\n{history}\n\n\n(You do not need to use these pieces of information if not relevant)\n\n\nCurrent conversation:\nHuman: {input}\nAI:\"\"\"\nPROMPT = PromptTemplate(\n input_variables=[\"history\", \"input\"], template=_DEFAULT_TEMPLATE\n)\nconversation_with_summary = ConversationChain(\n llm=llm,\n prompt=PROMPT,\n memory=memory,\n verbose=True\n)\nconversation_with_summary.predict(input=\"Hi, my name is Gary, what's up?\")\n```\n\nThe image below shows what an expected response from the LLM could look like. In this example, it has responded by saying its name is “AI”.\n\n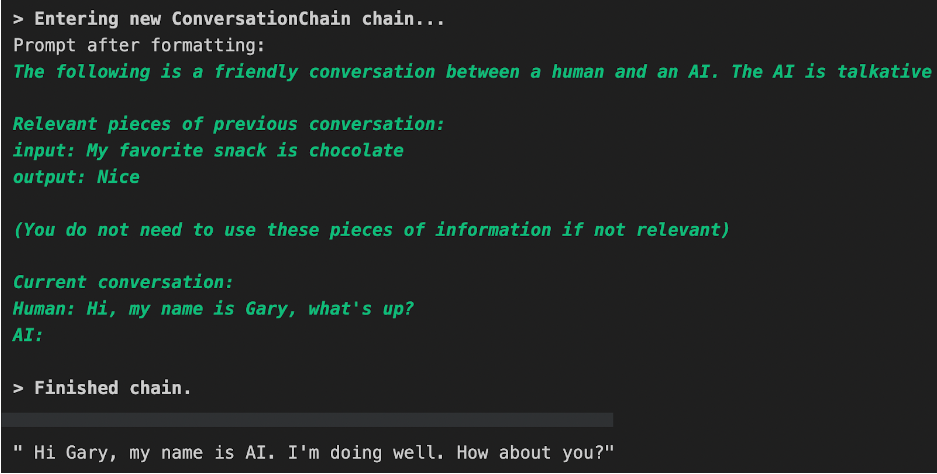\n\nNow let’s test out the memory so far. We use the `ConversationChain` object we created earlier and query for my favorite musician.\n\n```\nconversation_with_summary.predict(input=\"who is my favorite musician?\")\n```\n\nThe image below shows an expected response from the Conversation Chain. Since we used the verbose option, it also shows us the relevant conversation. We can see that it returns that my favorite artist is Taylor Swift, as expected.\n\n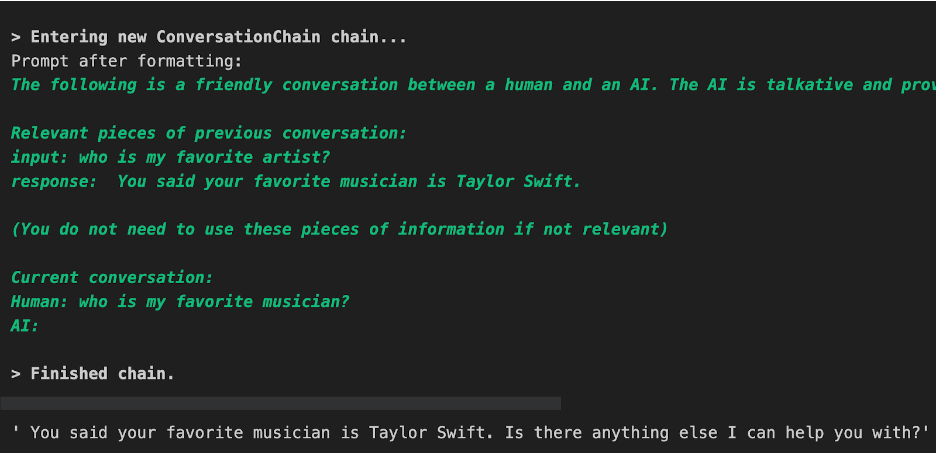\n\nNext, let’s check for my favorite dessert - cheesecake.\n\n```\nconversation_with_summary.predict(input=\"Whats my favorite dessert?\")\n```\n\nWhen we query for my favorite dessert, we can see that the Conversation Chain once again picks the correct information from Milvus. It finds that my favorite dessert is cheesecake, as I told it earlier.\n\n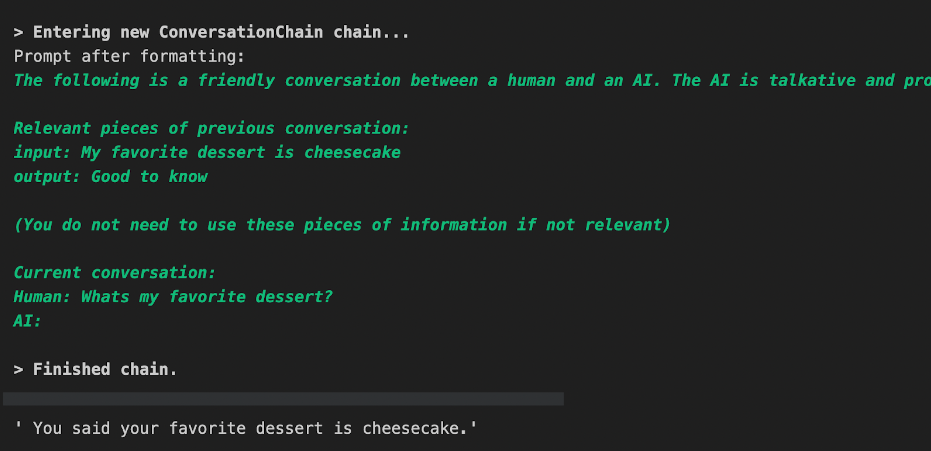\n\nNow that we’ve confirmed that we can query for the information we gave earlier, let’s check for one more thing - the information we provided at the beginning of our conversation. We started our conversation by telling the AI that our name was Gary. \n\n```\nconversation_with_summary.predict(input=\"What's my name?\")\n```\n\nOur final check yields that the conversation chain stored the bit about our name in our vector store conversational memory. It returns that we said our name is Gary.\n\n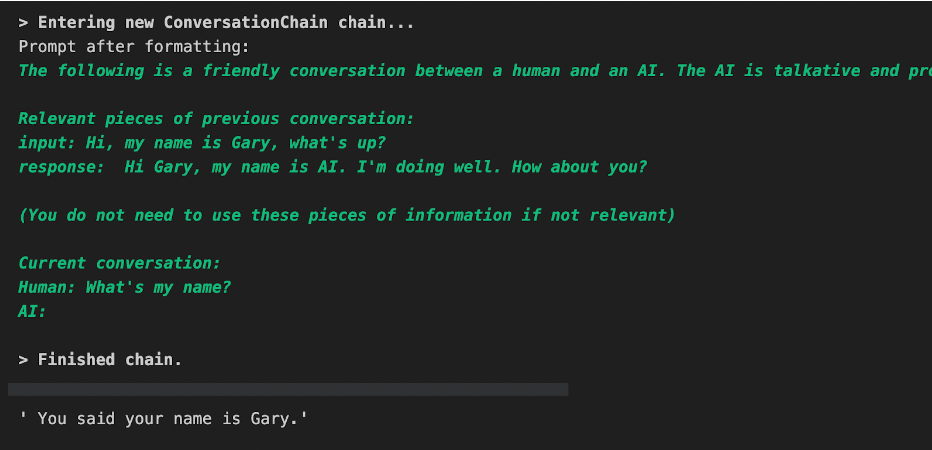\n\n\n## LangChain Conversational Memory Summary\n\nIn this tutorial, we learned how to use conversational memory in LangChain. LangChain offers access to vector store backends like Milvus for persistent conversational memory. We can use conversational memory by injecting history into our prompts and saving historical context in the `ConversationChain` object. \n\nFor this example tutorial, we gave the Conversation Chain five facts about me and pretended to be the main rival in Pokemon, Gary. Then, we pinged the Conversation Chain with questions about the a priori knowledge we stored - my favorite musician and dessert. It answered both of these questions correctly and surfaced the relevant entries. Finally, we asked it about our name as given at the beginning of the conversation, and it correctly returned that we said our name was “Gary.”","title":"Conversational Memory in LangChain\n","metaData":{}},{"id":"milvus-229-highly-anticipated-release-with-optimal-user-experience.md","author":"Owen Jiao, Fendy Feng","tags":["News"],"recommend":true,"canonicalUrl":"https://milvus.io/blog/milvus-229-highly-anticipated-release-with-optimal-user-experience.md","date":"2023-06-06T00:00:00.000Z","cover":"https://assets.zilliz.com/What_s_New_in_2_2_9_858e54a2d3.png","href":"/blog/milvus-229-highly-anticipated-release-with-optimal-user-experience.md","content":"\n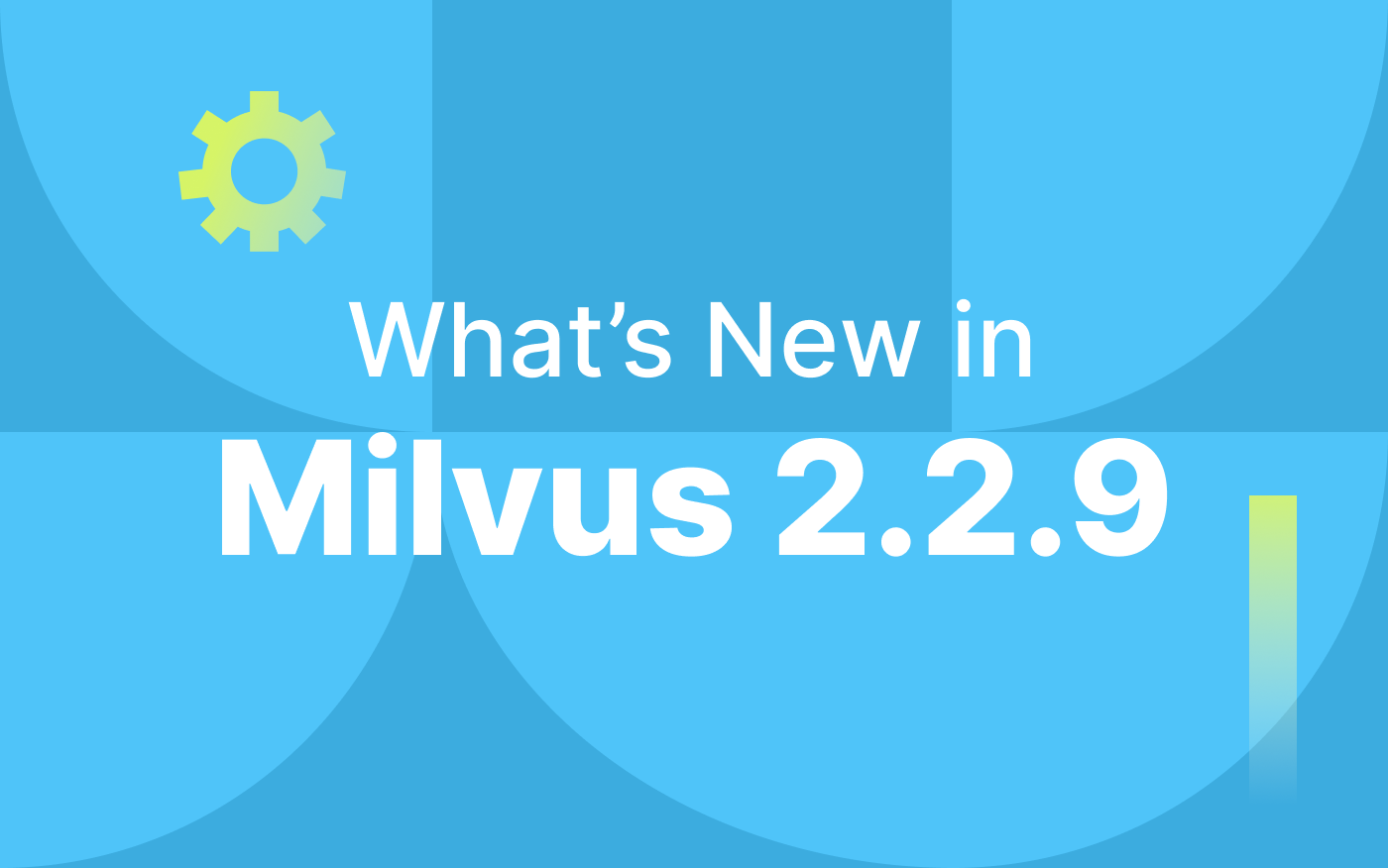\n\nWe are thrilled to announce the arrival of Milvus 2.2.9, a highly anticipated release that marks a significant milestone for the team and the community. This release offers many exciting features, including long-awaited support for JSON data types, dynamic schema, and partition keys, ensuring an optimized user experience and streamlined development workflow. Additionally, this release incorporates numerous enhancements and bug fixes. Join us in exploring Milvus 2.2.9 and discovering why this release is so exciting.\n\n## Optimized user experience with JSON support\n\nMilvus has introduced highly anticipated support for the JSON data type, allowing for the seamless storage of JSON data alongside the metadata of vectors within users' collections. With this enhancement, users can efficiently insert JSON data in bulk and perform advanced querying and filtering based on their JSON fields' contents. Furthermore, users can leverage expressions and perform operations tailored to their dataset's JSON fields, construct queries, and apply filters based on the content and structure of their JSON fields, allowing them to extract relevant information and manipulate data better. \n\nIn the future, the Milvus team will add indexes for fields within the JSON type, further optimizing the performance of mixed scalar and vector queries. So stay tuned for exciting developments ahead! \n\n## Added flexibility with support for dynamic schema\n\nWith support for JSON data, Milvus 2.2.9 now provides dynamic schema functionality through a simplified software development kit (SDK).\n\nStarting with Milvus 2.2.9, the Milvus SDK includes a high-level API that automatically fills dynamic fields into the hidden JSON field of the collection, allowing users to concentrate solely on their business fields.\n\n## Better data separation and enhanced search efficiency with Partition Key\n\nMilvus 2.2.9 enhances its partitioning capabilities by introducing the Partition Key feature. It allows user-specific columns as primary keys for partitioning, eliminating the need for additional APIs such as `loadPartition` and `releasePartition`. This new feature also removes the limit on the number of partitions, leading to more efficient resource utilization. \n\n## Support for Alibaba Cloud OSS\n\nMilvus 2.2.9 now supports Alibaba Cloud Object Storage Service (OSS). Alibaba Cloud users can easily configure the `cloudProvider` to Alibaba Cloud and take advantage of seamless integration for efficient storage and retrieval of vector data in the cloud.\n\nIn addition to the previously mentioned features, Milvus 2.2.9 offers database support in Role-Based Access Control (RBAC), introduces connection management, and includes multiple enhancements and bug fixes. For more information, refer to [Milvus 2.2.9 Release Notes](https://milvus.io/docs/release_notes.md).\n\n## Let’s keep in touch!\n\nIf you have questions or feedback about Milvus, please don't hesitate to contact us through [Twitter](https://twitter.com/milvusio) or [LinkedIn](https://www.linkedin.com/company/the-milvus-project). You're also welcome to join our [Slack channel](https://milvus.io/slack/) to chat with our engineers and the community directly or check out our [Tuesday office hours](https://us02web.zoom.us/meeting/register/tZ0pcO6vrzsuEtVAuGTpNdb6lGnsPBzGfQ1T#/registration)!","title":"Milvus 2.2.9: A Highly Anticipated Release with Optimal User Experience\n","metaData":{}},{"id":"introducing-milvus-lite-lightweight-version-of-milvus.md","author":"Fendy Feng","desc":"Experience the speed and efficiency of Milvus Lite, the lightweight variant of the renowned Milvus vector database for lightning-fast similarity search.","tags":["News"],"recommend":true,"canonicalUrl":"https://milvus.io/blog/introducing-milvus-lite-lightweight-version-of-milvus.md","date":"2023-05-23T00:00:00.000Z","cover":"https://assets.zilliz.com/introducing_Milvus_Lite_7c0d0a1174.jpeg","href":"/blog/introducing-milvus-lite-lightweight-version-of-milvus.md","content":"\n***Important Note***\n\n_We upgraded Milvus Lite in June 2024, enabling AI developers to build applications faster while ensuring a consistent experience across various deployment options, including Milvus on Kurbernetes, Docker, and managed cloud services. Milvus Lite also integrates with various AI frameworks and technologies, streamlining the development of AI applications with vector search capabilities. For more information, see the following references:_ \n\n- _Milvus Lite launch blog: [https://milvus.io/blog/introducing-milvus-lite.md](https://milvus.io/blog/introducing-milvus-lite.md)_\n\n- _Milvus Lite documentation: [https://milvus.io/docs/quickstart.md](https://milvus.io/docs/quickstart.md)_\n\n- _Milvus Lite GitHub repository: [https://github.com/milvus-io/milvus-lite](https://github.com/milvus-io/milvus-lite)_\n\n\n\n\u003cbr\u003e\n\n\n[Milvus](https://github.com/milvus-io/milvus) is an open-source vector database purpose-built to index, store, and query embedding vectors generated by deep neural networks and other machine learning (ML) models at billions of scales. It has become a popular choice for many companies, researchers, and developers who must perform similarity searches on large-scale datasets. \n\nHowever, some users may find the full version of Milvus too heavy or complex. To address this problem, [Bin Ji](https://github.com/matrixji), one of the most active contributors in the Milvus community, built [Milvus Lite](https://github.com/milvus-io/milvus-lite), a lightweight version of Milvus. \n\n## What is Milvus Lite?\n\nAs previously mentioned, [Milvus Lite](https://github.com/milvus-io/milvus-lite) is a simplified alternative to Milvus that offers so many advantages and benefits. \n\n- You can integrate it into your Python application without adding extra weight. \n- It is self-contained and does not require any other dependencies, thanks to the standalone Milvus' ability to work with embedded Etcd and local storage. \n- You can import it as a Python library and use it as a command-line interface (CLI)-based standalone server. \n- It works smoothly with Google Colab and Jupyter Notebook.\n- You can safely migrate your work and write code to other Milvus instances (standalone, clustered, and fully-managed versions) without any risk of losing data. \n\n## When should you use Milvus Lite?\n\nSpecifically, Milvus Lite is most helpful in the following situations:\n- When you prefer to use Milvus without container techniques and tools like [Milvus Operator](https://milvus.io/docs/install_standalone-operator.md), [Helm](https://milvus.io/docs/install_standalone-helm.md), or [Docker Compose](https://milvus.io/docs/install_standalone-docker.md).\n- When you don't require virtual machines or containers for using Milvus.\n- When you want to incorporate Milvus features into your Python applications.\n- When you want to spin up a Milvus instance in Colab or Notebook for a quick experiment. \n\n**Note**: We do not recommend using Milvus Lite in any production environment or if you require high performance, strong availability, or high scalability. Instead, consider using [Milvus clusters](https://github.com/milvus-io/milvus) or [fully-managed Milvus on Zilliz Cloud](https://zilliz.com/cloud) for production.\n\n## How to get started with Milvus Lite?\n\nNow, let’s take a look at how to install, configure, and use Milvus Lite. \n### Prerequisites \n\nTo use Milvus Lite, please ensure you have completed the following requirements:\n- Installed Python 3.7 or a later version.\n- Using one of the verified operating systems listed below:\n - Ubuntu \u003e= 18.04 (x86_64)\n - CentOS \u003e= 7.0 (x86_64)\n - MacOS \u003e= 11.0 (Apple Silicon)\n\n**Notes**: \n\n1. Milvus Lite uses `manylinux2014` as the base image, making it compatible with most Linux distributions for Linux users. \n2. Running Milvus Lite on Windows is also possible, although this has yet to be fully verified.\n\n### Install Milvus Lite\n\nMilvus Lite is available on PyPI so you can install it via `pip`.\n\n```\n$ python3 -m pip install milvus\n```\n\nYou can also install it with PyMilvus as follows:\n\n```\n$ python3 -m pip install milvus[client]\n```\n\n### Use and start Milvus Lite\n\nDownload the [example notebook](https://github.com/milvus-io/milvus-lite/tree/main/examples) from our project repository's example folder. You have two options for using Milvus Lite: either import it as a Python library or run it as a standalone server on your machine using the CLI.\n\n- To start Milvus Lite as a Python module, execute the following commands:\n\n```\nfrom milvus import default_server\nfrom pymilvus import connections, utility\n\n# Start your milvus server\ndefault_server.start()\n\n# Now you can connect with localhost and the given port\n# Port is defined by default_server.listen_port\nconnections.connect(host='127.0.0.1', port=default_server.listen_port)\n\n# Check if the server is ready.\nprint(utility.get_server_version())\n\n# Stop your milvus server\ndefault_server.stop()\n```\n\n- To suspend or stop Milvus Lite, use the `with` statement. \n\n```\nfrom milvus import default_server\n\nwith default_server:\n # Milvus Lite has already started, use default_server here.\n connections.connect(host='127.0.0.1', port=default_server.listen_port)\n```\n\n- To start Milvus Lite as a CLI-based standalone server, run the following command: \n\n```\nmilvus-server\n```\n\nAfter you start Milvus Lite, you can use PyMilvus or other tools you prefer to connect to the standalone server.\n\n### Start Milvus Lite in a debug mode\n\n- To run Milvus Lite in a debug mode as a Python Module, execute the following commands: \n\n```\nfrom milvus import debug_server, MilvusServer\n\ndebug_server.run()\n\n# Or you can create a MilvusServer by yourself\n# server = MilvusServer(debug=True)\n```\n\n- To run the standalone server in a debug mode, execute the following command:\n\n```\nmilvus-server --debug\n```\n\n### Persist data and logs\n\n- To create a local directory for Milvus Lite that will contain all relevant data and logs, execute the following commands:\n\n```\nfrom milvus import default_server\n\nwith default_server:\n default_server.set_base_dir('milvus_data')\n```\n\n- To persist all data and logs generated by the standalone server on your local drive, run the following command: \n\n```\n$ milvus-server --data milvus_data\n```\n\n### Configure Milvus Lite\n\nConfiguring Milvus Lite is similar to setting up Milvus instances using Python APIs or CLI.\n\n- To configure Milvus Lite using Python APIs, use the `config.set` API of a `MilvusServer` instance for both the basic and extra settings: \n\n```\nfrom milvus import default_server\n\nwith default_server:\n default_server.config.set('system_Log_level', 'info')\n default_server.config.set('proxy_port', 19531)\n default_server.config.set('dataCoord.segment.maxSize', 1024)\n```\n\n- To configure Milvus Lite using CLI, run the following command for basic settings:\n\n```\n$ milvus-server --system-log-level info\n$ milvus-server --proxy-port 19531\n```\n\n- Or, run the following for extra configurations.\n\n```\n$ milvus-server --extra-config dataCoord.segment.maxSize=1024\n```\n\nAll configurable items are in the `config.yaml` template shipped with the Milvus package. \n\nFor more technical details on how to install and configure Milvus Lite, see our [documentation](https://milvus.io/docs/milvus_lite.md#Prerequisites). \n\n## Summary \n\nMilvus Lite is an excellent choice for those seeking the capabilities of Milvus in a compact format. Whether you are a researcher, developer, or data scientist, it's worth exploring this option.\n\nMilvus Lite is also a beautiful addition to the open-source community, showcasing the extraordinary work of its contributors. Thanks to Bin Ji's efforts, Milvus is now available to more users. We cannot wait to see the innovative ideas that Bin Ji and other members of the Milvus community will bring forth in the future.\n\n## Let’s keep in touch!\n\nIf you encounter problems installing or using Milvus Lite, you can [file an issue here](https://github.com/milvus-io/milvus-lite/issues/new) or contact us through [Twitter](https://twitter.com/milvusio) or [LinkedIn](https://www.linkedin.com/company/the-milvus-project). You're also welcome to join our [Slack channel](https://milvus.io/slack/) to chat with our engineers and the entire community, or check out [our Tuesday office hours](https://us02web.zoom.us/meeting/register/tZ0pcO6vrzsuEtVAuGTpNdb6lGnsPBzGfQ1T#/registration)!\n","title":"Introducing Milvus Lite: the Lightweight Version of Milvus\n","metaData":{}},{"id":"Milvus-2-2-8-better-query-performance-20-higher-throughputs.md","author":"Fendy Feng","tags":["News"],"recommend":true,"canonicalUrl":"https://milvus.io/blog/Milvus-2-2-8-better-query-performance-20-higher-throughputs.md","date":"2023-05-12T00:00:00.000Z","cover":"https://assets.zilliz.com/What_s_New_in_2_2_8_f4dd6de0f2.png","href":"/blog/Milvus-2-2-8-better-query-performance-20-higher-throughputs.md","content":"\n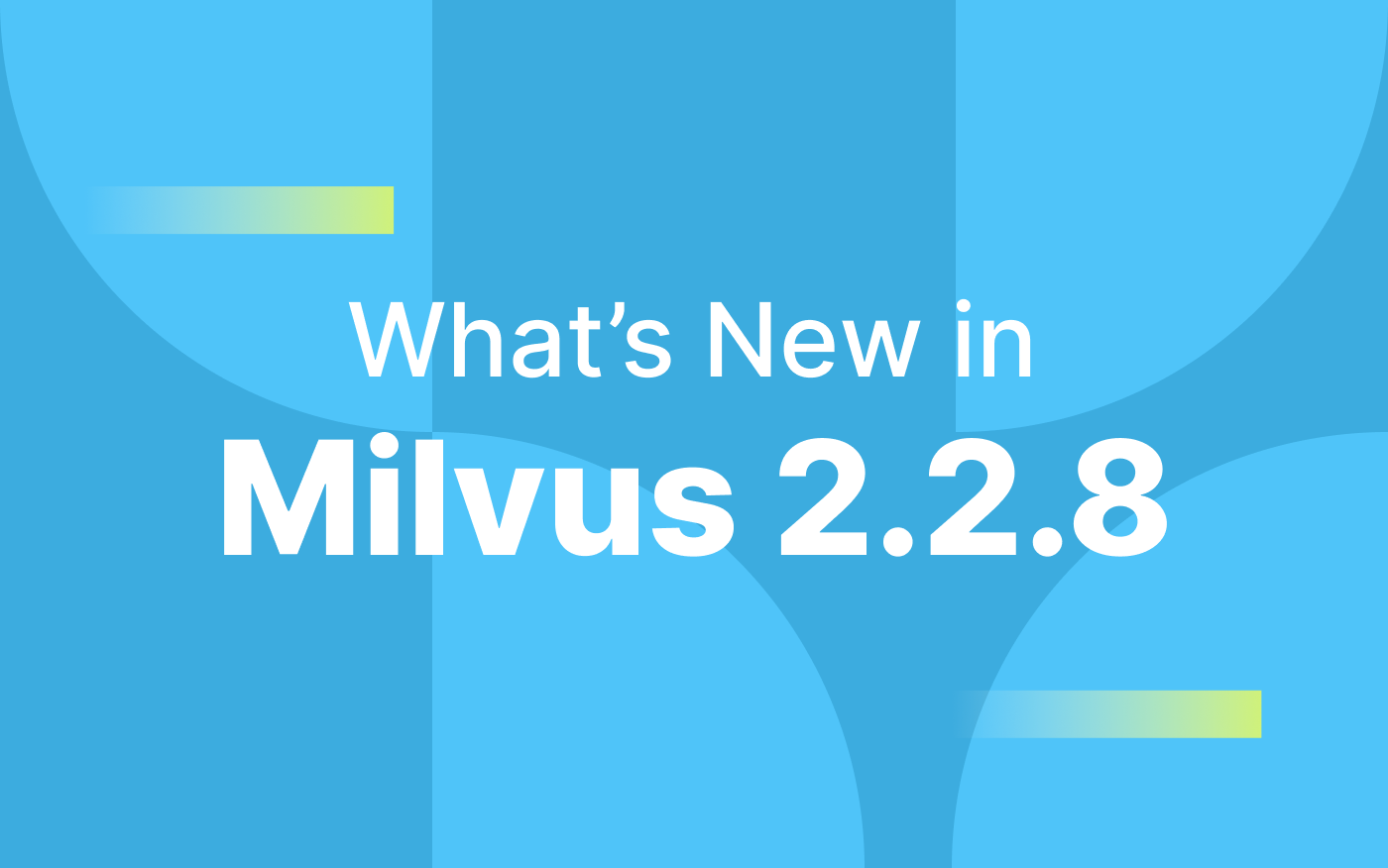\n\n\nWe are excited to announce our latest release of Milvus 2.2.8. This release includes numerous improvements and bug fixes from previous versions, resulting in better querying performance, resource-saving, and higher throughputs. Let's take a look at what's new in this release together. \n\n## Reduced peak memory consumption during collection loading\n\nTo perform queries, Milvus needs to load data and indexes into memory. However, during the loading process, multiple memory copies can cause the peak memory usage to increase up to three to four times higher than during actual runtime. The latest version of Milvus 2.2.8 effectively addresses this issue and optimizes memory usage.\n\n## Expanded querying scenarios with QueryNode supporting plugins\n\nQueryNode now supports plugins in the latest Milvus 2.2.8. You can easily specify the path of the plugin file with the `queryNode.soPath` configuration. Then, Milvus can load the plugin at runtime and expand the available querying scenarios. Refer to the [Go plugin documentation](https://pkg.go.dev/plugin), if you need guidance on developing plugins. \n\n## Optimized querying performance with enhanced compaction algorithm\n\nThe compaction algorithm determines the speed at which the segments can converge, directly affecting the querying performance. With the recent improvements to the compaction algorithm, the convergence efficiency has dramatically improved, resulting in faster queries. \n\n## Better resource saving and querying performance with reduced collection shards\n\nMilvus is a massively parallel processing (MPP) system, which means that the number of collection shards impacts Milvus’ efficiency in writing and querying. In older versions, a collection had two shards by default, which resulted in excellent writing performance but compromised querying performance and resource cost. With the new Milvus 2.2.8 update, the default collection shards have been reduced to one, allowing users to save more resources and perform better queries. Most users in the community have less than 10 million data volumes, and one shard is sufficient to achieve good writing performance.\n\n**Note**: This upgrade does not affect collections created before this release. \n\n## 20% throughput increase with an improved query grouping algorithm \nMilvus has an efficient query grouping algorithm that combines multiple query requests in the queue into one for faster execution, significantly improving throughput. In the latest release, we make additional enhancements to this algorithm, increasing Milvus' throughput by at least 20%.\n\nIn addition to the mentioned improvements, Milvus 2.2.8 also fixes various bugs. For more details, see [Milvus Release Notes](https://milvus.io/docs/release_notes.md). \n\n## Let’s keep in touch!\nIf you have questions or feedback about Milvus, please don't hesitate to contact us through [Twitter](https://twitter.com/milvusio) or [LinkedIn](https://www.linkedin.com/company/the-milvus-project). You're also welcome to join our [Slack channel](https://milvus.io/slack/) to chat with our engineers and the entire community directly or check out our [Tuesday office hours](https://us02web.zoom.us/meeting/register/tZ0pcO6vrzsuEtVAuGTpNdb6lGnsPBzGfQ1T#/registration)!\n","title":"Milvus 2.2.8: Better Query Performance, 20% Higher Throughput \n","metaData":{}},{"id":"milvus-at-its-best-exploring-2-2-to-2-2-6.md","author":"Fendy Feng","tags":["News"],"desc":"what is new with Milvus 2.2 to 2.2.6","recommend":true,"canonicalUrl":"https://milvus.io/blog/milvus-at-its-best-exploring-2-2-to-2-2-6.md","date":"2023-04-22T00:00:00.000Z","cover":"https://assets.zilliz.com/explore_milvus_latest_versions_48a4138d02.png","href":"/blog/milvus-at-its-best-exploring-2-2-to-2-2-6.md","content":"\n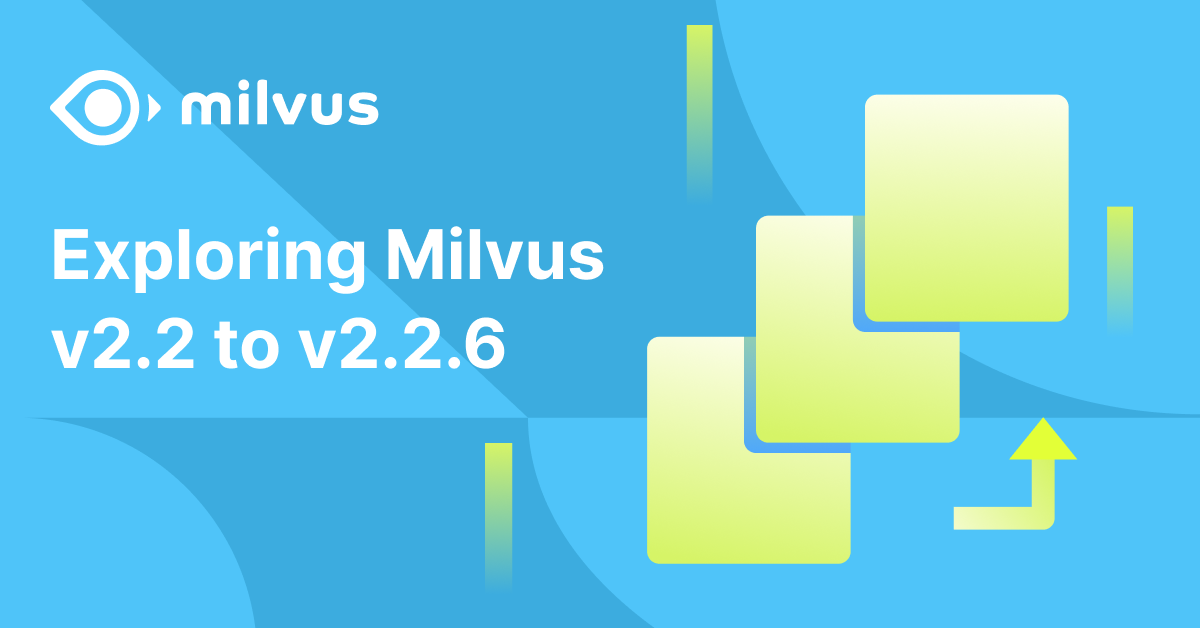\n\nWelcome back, Milvus followers! We know it's been a while since we last shared our updates on this cutting-edge open-source vector database. But fear not, because we’re here to catch you up on all the exciting developments that have taken place since last August.\n\nIn this blog post, we'll take you through the latest Milvus releases, spanning from version 2.2 to version 2.2.6. We have much to cover, including new features, improvements, bug fixes, and optimizations. So, fasten your seatbelts, and let’s dive in!\n\n## Milvus v2.2: a major release with enhanced stability, faster search speed, and flexible scalability\n\nMilvus v2.2 is a significant release that introduces seven brand-new features and numerous breakthrough improvements to previous versions. Let's take a closer look at some of the highlights:\n\n* **Bulk Inserts of Entities from Files**: With this feature, you can upload a batch of entities in one or multiple files directly to Milvus with just a few lines of code, saving you time and effort.\n* **Query Result Pagination**: To avoid massive search and query results returning in a single remote procedure call (RPC), Milvus v2.2 allows you to configure offset and filter results with keywords in searches and queries. \n* **Role-Based Access Control (RBAC)**: Milvus v2.2 now supports RBAC, allowing you to control access to your Milvus instance by managing users, roles, and permissions. \n* **Quotas and Limits**: Quotas and limits is a new mechanism in Milvus v2.2 that protects the database system from out-of-memory (OOM) errors and crashes during sudden traffic surges. With this feature, you can control ingestion, search, and memory usage.\n* **Time to Live (TTL) at a Collection Level**: In previous releases, Milvus only allowed you to configure TTL for your clusters. However, Milvus v2.2 now supports configuring TTL at the collection level. Configuring TTL for a specific collection and entities in that collection will automatically expire after the TTL ends. This configuration provides more fine-grained control over data retention.\n* **Disk-Based Approximate Nearest Neighbor Search (ANNS) Indexes (Beta)**: Milvus v2.2 introduces support for DiskANN, an SSD-resident, and Vamana graph-based ANNS algorithm. This support allows for direct searching on large-scale datasets, which can significantly reduce memory usage, by up to 10 times.\n* **Data Backup (Beta)**: Milvus v2.2 provides [a brand new tool](https://github.com/zilliztech/milvus-backup) for backing up and restoring your Milvus data properly, either through a command line or an API server. \n\nIn addition to the new features mentioned above, Milvus v2.2 includes fixes for five bugs and multiple improvements to enhance Milvus' stability, observability, and performance. For more details, see [Milvus v2.2 Release Notes](https://milvus.io/docs/release_notes.md#v220). \n\n## Milvus v2.2.1 \u0026 v2.2.2: minor releases with issues fixed\n\nMilvus v2.2.1 and v2.2.2 are minor releases focusing on fixing critical issues in older versions and introducing new features. Here are some highlights:\n\n### Milvus v2.2.1\n\n* Supports Pulsa tenant and authentication\n* Supports transport layer security (TLS) in the etcd config source\n* Improves search performance by over 30%\n* Optimizes the scheduler and increases the probability of merge tasks\n* Fixes multiple bugs, including term filtering failures on indexed scalar fields and IndexNode panic upon failures to create an index\n\n### Milvus v2.2.2\n\n* Fixes the issue that the proxy doesn't update the cache of shard leaders\n* Fixes the issue that the loaded info is not cleaned for released collections/partitions\n* Fixes the issue that the load count is not cleared on time\n\nFor more details, see [Milvus v2.2.1 Release Notes](https://milvus.io/docs/release_notes.md#v221) and [Milvus v2.2.2 Release Notes](https://milvus.io/docs/release_notes.md#v222). \n\n## Milvus v2.2.3: more secure, stable, and available \n\nMilvus v2.2.3 is a release that focuses on enhancing the system’s security, stability, and availability. In addition, it introduces two important features:\n\n* **Rolling upgrade**: This feature allows Milvus to respond to incoming requests during the upgrade process, which was impossible in previous releases. Rolling upgrades ensure the system remains available and responsive to user requests even during upgrades.\n\n* **Coordinator high availability (HA)**: This feature enables Milvus coordinators to work in an active-standby mode, reducing the risk of single-point failures. Even in unexpected disasters, the recovery time is reduced to at most 30 seconds. \n\nIn addition to these new features, Milvus v2.2.3 includes numerous improvements and bug fixes, including enhanced bulk insert performance, reduced memory usage, optimized monitoring metrics, and improved meta-storage performance. For more details, see [Milvus v2.2.3 Release Notes](https://milvus.io/docs/release_notes.md#v223). \n\n## Milvus v2.2.4: faster, more reliable and resource saving\n\nMilvus v2.2.4 is a minor update to Milvus v2.2. It introduces four new features and several enhancements, resulting in faster performance, improved reliability, and reduced resource consumption. The highlights of this release include:\n\n* **Resource grouping**: Milvus now supports grouping QueryNodes into other resource groups, allowing for complete isolation of access to physical resources in different groups. \n* **Collection renaming**: The Collection-renaming API allows users to change the name of a collection, providing more flexibility in managing collections and improving usability.\n* **Support for Google Cloud Storage**\n* **New option in search and query APIs**: This new feature allows users to skip search on all growing segments, offering better search performance in scenarios where the search is performed concurrently with data insertion. \n\nFor more information, see [Milvus v2.2.4 Release Notes](https://milvus.io/docs/release_notes.md#v224). \n\n## Milvus v2.2.5: NOT RECOMMENDED\n\nMilvus v2.2.5 has several critical issues, and therefore, we do not recommend using this release. We sincerely apologize for any inconvenience caused by them. However, these issues have been addressed in Milvus v2.2.6.\n\n## Milvus v2.2.6: resolves critical issues from v2.2.5 \n\nMilvus v2.2.6 has successfully addressed the critical issues discovered in v2.2.5, including problems with recycling dirty binlog data and the DataCoord GC failure. If you currently use v2.2.5, please upgrade it to ensure optimal performance and stability.\n\nCritical issues fixed include: \n\n* DataCoord GC failure\n* Override of passed index parameters \n* System delay caused by RootCoord message backlog\n* Inaccuracy of metric RootCoordInsertChannelTimeTick \n* Possible timestamp stop\n* Occasional coordinator role self-destruction during the restart process \n* Checkpoints falling behind due to abnormal exit of garbage collection \n\nFor more details, see [Milvus v2.2.6 Release Notes](https://milvus.io/docs/release_notes.md#v226). \n\n## Summary \n\nIn conclusion, the latest Milvus releases from v2.2 to v2.2.6 have delivered many exciting updates and improvements. From new features to bug fixes and optimizations, Milvus continues to meet its commitments to provide cutting-edge solutions and empower applications in various domains. Stay tuned for more exciting updates and innovations from the Milvus community. \n\n","title":"Milvus at Its Best: Exploring v2.2 to v2.2.6\n","metaData":{}},{"id":"dynamically-change-log-levels-in-the-milvus-vector-database.md","author":"Enwei Jiao","desc":"Learn how to adjust log level in Milvus without restarting the service.","tags":["Engineering"],"canonicalUrl":"https://milvus.io/blog/dynamically-change-log-levels-in-the-milvus-vector-database.md","date":"2022-09-21T00:00:00.000Z","cover":"https://assets.zilliz.com/Dynamically_Change_Log_Levels_in_the_Milvus_Vector_Database_58e31c66cc.png","href":"/blog/dynamically-change-log-levels-in-the-milvus-vector-database.md","content":"\n\n\n\u003e This article is written by [Enwei Jiao](https://github.com/jiaoew1991) and translated by [Angela Ni](https://www.linkedin.com/in/yiyun-n-2aa713163/).\n\nTo prevent an over-output of logs from affecting disk and system performance, Milvus by default outputs logs at the `info` level while running. However, sometimes logs at the `info` level are not sufficient enough to help us efficiently identify bugs and issues. What's worse, in some cases, changing the log level and restarting the service might lead to the failure of reproducing the issues, making troubleshooting all the more difficult. Consequently, the support for changing log levels dynamically in the Milvus vector database is urgently needed.\n\nThis article aims to introduce the mechanism behind that enables changing log levels dynamically and provide instructions on how to do so in the Milvus vector database.\n\n**Jump to:**\n- [Mechanism](#Mechanism)\n- [How to dynamically change log levels](#How-to-dynamically-change-log-levels)\n\n## Mechanism\n\nThe Milvus vector database adopts the [zap](https://github.com/uber-go/zap) logger open sourced by Uber. As one the most powerful log components in the Go language ecosystem, zap incorporates an [http_handler.go](https://github.com/uber-go/zap/blob/master/http_handler.go) module so that you can view the current log level and dynamically change the log level via an HTTP interface.\n\nMilvus listens the HTTP service provided by the `9091` port. Therefore, you can access the `9091` port to take advantage such features as performance debugging, metrics, health checks. Similarly, the `9091` port is reused to enable dynamic log level modification and a `/log/level` path is also added to the port. See the[ log interface PR](https://github.com/milvus-io/milvus/pull/18430) for more information.\n\n## How to dynamically change log levels\n\nThis section provides instructions on how to dynamically change log levels without the need the restarting the running Milvus service.\n\n### Prerequisite\n\nEnsure that you can access the `9091` port of Milvus components.\n\n### Change the log level\n\nSuppose the IP address of the Milvus proxy is `192.168.48.12`. \n\nYou can first run `$ curl -X GET 192.168.48.12:9091/log/level` to check the current log level of the proxy.\n\nThen you can make adjustments by specifying the log level. Log level options include: \n\n- `debug`\n\n- `info`\n\n- `warn`\n\n- `error`\n\n- `dpanic`\n\n- `panic`\n\n- `fatal`\n\nThe following example code changes the log level from the default log level from `info` to `error`. \n\n```Python\n$ curl -X PUT 192.168.48.12:9091/log/level -d level=error\n```\n\n\n\n\n\n\n\n\n\n\n\n","title":"Dynamically Change Log Levels in the Milvus Vector Database","metaData":{}},{"id":"understanding-consistency-levels-in-the-milvus-vector-database-2.md","author":"Jiquan Long","desc":"An anatomy of the mechanism behind tunable consistency levels in the Milvus vector database.","tags":["Engineering"],"canonicalUrl":"https://milvus.io/blog/understanding-consistency-levels-in-the-milvus-vector-database-2.md","date":"2022-09-13T00:00:00.000Z","cover":"https://assets.zilliz.com/1280_X1280_0e0d4bc107.png","href":"/blog/understanding-consistency-levels-in-the-milvus-vector-database-2.md","content":"\n\n\n\u003e This article is written by [Jiquan Long](https://github.com/longjiquan) and transcreated by [Angela Ni](https://www.linkedin.com/in/yiyun-n-2aa713163/).\n\nIn the [previous blog](https://milvus.io/blog/understanding-consistency-levels-in-the-milvus-vector-database.md) about consistency, we have explained what is the connotation of consistency in a distributed vector database, covered the four levels of consistency - strong, bounded staleness, session, and eventual supported in the Milvus vector database, and explained the best-suited application scenario of each consistency level. \n\nIn this post, we will continue to examine the mechanism behind that enables users of the Milvus vector database to flexibly choose the ideal consistency level for various application scenarios. We will also provide the basic tutorial on how to tune consistency level in the Milvus vector database.\n\n**Jump to:**\n\n- [The underlying time tick mechanism](#The-underlying-time-tick-mechanism)\n- [Guarantee timestamp](#Guarantee-timestamp)\n- [Consistency levels](#Consistency-levels)\n- [How to tune consistency level in Milvus?](#How-to-tune-consistency-level-in-Milvus)\n\n## The underlying time tick mechanism\n\nMilvus uses the time tick mechanism to ensure different levels of consistency when a vector search or query is conducted. Time Tick is the watermark of Milvus which acts like a clock in Milvus and signifies at which point of time is the Milvus system in. Whenever there is a data manipulation language (DML) request sent to the Milvus vector database, it assigns a timestamp to the request. As shown in the figure below, when new data are inserted into the message queue for instance, Milvus not only marks a timestamp on these inserted data, but also inserts time ticks at a regular interval. \n\n\n\nLet's take `syncTs1` in the figure above as an example. When downstream consumers like query nodes see `syncTs1`, the consumer components understand that all data which are inserted earlier than `syncTs1` has been consumed. In other words, the data insertion requests whose timestamp values are smaller than `syncTs1` will no longer appear in the message queue.\n\n## Guarantee Timestamp\n\nAs mentioned in the previous section, downstream consumer components like query nodes continuously obtains messages of data insertion requests and time tick from the message queue. Every time a time tick is consumed, the query node will mark this consumed time tick as the serviceable time - `ServiceTime` and all data inserted before `ServiceTime` are visible to the query node.\n\nIn addition to `ServiceTime`, Milvus also adopts a type of timestamp - guarantee timestamp (`GuaranteeTS`) to satisfy the need for various levels of consistency and availability by different users. This means that users of the Milvus vector datbase can specify `GuaranteeTs` in order to inform query nodes that all the data before `GuaranteeTs` should be visible and involved when a search or query is conducted.\n\nThere are usually two scenarios when the query node executes a search request in the Milvus vector database.\n\n### Scenario 1: Execute search request immediately\n\nAs shown in the figure below, if `GuaranteeTs` is smaller than `ServiceTime`, query nodes can execute the search request immediately.\n\n\n\n### Scenario 2: Wait till \"ServiceTime \u003e GuaranteeTs\"\n\nIf `GuaranteeTs` is greater than `ServiceTime`, query nodes must continue to consume time tick from the message queue. Search requests cannot be executed until `ServiceTime` is greater than `GuaranteeTs`.\n\n\n\n## Consistency Levels\n\nTherefore, the `GuaranteeTs` is configurable in the search request to achieve the level of consistency specified by you. A `GuaranteeTs` with a large value ensures [strong consistency](https://milvus.io/blog/understanding-consistency-levels-in-the-milvus-vector-database.md#Strong) at the cost of a high search latency. And a `GuaranteeTs` with a small value reduces search latency but the data visibility is compromised.\n\n`GuaranteeTs` in Milvus is a hybrid timestamp format. And the user has no idea of the [TSO](https://github.com/milvus-io/milvus/blob/master/docs/design_docs/20211214-milvus_hybrid_ts.md) inside Milvus. Therefore, Specifying the value of`GuaranteeTs` is a much too complicated task for users. To save the trouble for users and provide an optimal user experience, Milvus only requires the users to choose the specific consistency level, and the Milvus vector database will automatically handle the `GuaranteeTs` value for users. That is to say, the Milvus user only needs to choose from the four consistency levels: `Strong`, `Bounded`, `Session`, and `Eventually`. And each of the consistency level corresponds to a certain `GuaranteeTs` value.\n\nThe figure below illustrates the `GuaranteeTs` for each of the four levels of consistency in the Milvus vector database.\n\n\n\nThe Milvus vector database supports four levels of consistency:\n\n- `CONSISTENCY_STRONG`: `GuaranteeTs` is set to the same value as the latest system timestamp, and query nodes wait until the service time proceeds to the latest system timestamp to process the search or query request. \n\n- `CONSISTENCY_EVENTUALLY`: `GuaranteeTs` is set to a value insignificantly smaller than the latest system timestamp in order to skip the consistency check. Query nodes search immediately on the existing data view. \n\n- `CONSISTENCY_BOUNDED`: `GuaranteeTs` is set to a value relatively smaller than the latest system timestamp, and query nodes search on a tolerably less updated data view. \n\n- `CONSISTENCY_SESSION`: The client uses the timestamp of the last write operation as the `GuaranteeTs` so that each client can at least retrieve the data inserted by itself. \n\n## How to tune consistency level in Milvus?\n\nMilvus supports tuning the consistency level when [creating a collection](https://milvus.io/docs/v2.1.x/create_collection.md) or conducting a [search](https://milvus.io/docs/v2.1.x/search.md) or [query](https://milvus.io/docs/v2.1.x/query.md). \n\n### Conduct a vector similarity search\n\nTo conduct a vector similarity search with the level of consistency you want, simply set the value for the parameter `consistency_level` as either `Strong`, `Bounded`, `Session`, or `Eventually`. If you do not set the value for the parameter `consistency_level`, the consistency level will be `Bounded` by default. The example conducts a vector similarity search with `Strong` consistency. \n\n```\nresults = collection.search(\n data=[[0.1, 0.2]], \n anns_field=\"book_intro\", \n param=search_params, \n limit=10, \n expr=None,\n consistency_level=\"Strong\"\n)\n```\n\n### Conduct a vector query\n\nSimilar to conducting a vector similarity search, you can specify the value for the parameter `consistency_level` when conducting a vector query. The example conducts a vector query with `Strong` consistency. \n\n```\nres = collection.query(\n expr = \"book_id in [2,4,6,8]\", \n output_fields = [\"book_id\", \"book_intro\"],\n consistency_level=\"Strong\"\n)\n```\n\n## What's next\n\nWith the official release of Milvus 2.1, we have prepared a series of blogs introducing the new features. Read more in this blog series:\n\n- [How to Use String Data to Empower Your Similarity Search Applications](https://milvus.io/blog/2022-08-08-How-to-use-string-data-to-empower-your-similarity-search-applications.md)\n- [Using Embedded Milvus to Instantly Install and Run Milvus with Python](https://milvus.io/blog/embedded-milvus.md)\n- [Increase Your Vector Database Read Throughput with In-Memory Replicas](https://milvus.io/blog/in-memory-replicas.md)\n- [Understanding Consistency Level in the Milvus Vector Database](https://milvus.io/blog/understanding-consistency-levels-in-the-milvus-vector-database.md)\n- [Understanding Consistency Level in the Milvus Vector Database (Part II)](https://milvus.io/blog/understanding-consistency-levels-in-the-milvus-vector-database-2.md)\n- [How Does the Milvus Vector Database Ensure Data Security?](https://milvus.io/blog/data-security.md)\n\n\n\n","title":"Understanding Consistency Level in the Milvus Vector Database - Part II","metaData":{}},{"id":"data-security.md","author":"Angela Ni","desc":"Learn about user authentication and encryption in transit in Milvus.","tags":["Engineering"],"canonicalUrl":"https://milvus.io/blog/data-security.md","date":"2022-09-05T00:00:00.000Z","cover":"https://assets.zilliz.com/Security_192e35a790.png","href":"/blog/data-security.md","content":"\n\n\nIn full consideration of your data security, user authentication and transport layer security (TLS) connection are now officially available in Milvus 2.1. Without user authentication, anyone can access all data in your vector database with SDK. However, starting from Milvus 2.1, only those with a valid username and password can access the Milvus vector database. In addition, in Milvus 2.1 data security is further protected by TLS, which ensures secure communications in a computer network. \n\nThis article aims to analyze how Milvus the vector database ensures data security with user authentication and TLS connection and explain how you can utilize these two features as a user who wants to ensure data security when using the vector database.\n\n\n**Jump to:**\n\n- [What is database security and why is it important?](#What-is-database-security-and-why-is-it-important)\n- [How does the Milvus vector database ensure data security?](#How-does-the-Milvus-vector-database-ensure-data-security)\n - [User authentication](#User-authentication)\n - [TLS connection](#TLS-connection)\n\n## What is database security and why is it important?\n\nDatabase security refers to the measures taken to ensure that all data in the database are safe and kept confidential. Recent data breach and data leak cases at [Twitter, Marriott, and Texas Department of Insurance, etc](https://firewalltimes.com/recent-data-breaches/) makes us all the more vigilant to the issue of data security. All these cases constantly remind us that companies and businesses can suffer from severe loss if the data are not well protected and the databases they use are secure. \n\n## How does the Milvus vector database ensure data security?\n\nIn the current release of 2.1, the Milvus vector database attempts to ensure database security via authentication and encryption. More specifically, on the access level, Milvus supports basic user authentication to control who can access the database. Meanwhile, on the database level, Milvus adopts the transport layer security (TLS) encryption protocol to protect data communication.\n\n### User authentication\n\nThe basic user authentication feature in Milvus supports accessing the vector database using a username and password for the sake of data security. This means clients can only access the Milvus instance upon providing an authenticated username and password. \n\n#### The authentication workflow in the Milvus vector database\n\nAll gRPC requests are handled by the Milvus proxy, hence authentication is completed by the proxy. The workflow of logging in with the credentials to connect to the Milvus instance is as follows. \n\n1. Create credentials for each Milvus instance and the encrypted passwords are stored in etcd. Milvus uses [bcrypt](https://golang.org/x/crypto/bcrypt) for encryption as it implements Provos and Mazières's [adaptive hashing algorithm](http://www.usenix.org/event/usenix99/provos/provos.pdf).\n2. On the client side, SDK sends ciphertext when connecting to the Milvus service. The base64 ciphertext (\u003cusername\u003e:\u003cpassword\u003e) is attached to the metadata with the key `authorization`.\n3. The Milvus proxy intercepts the request and verifies the credentials.\n4. Credentials are cached locally in the proxy.\n \n\n\n \nWhen the credentials are updated, the system workflow in Milvus is as follows\n1. Root coord is in charge of the credentials when insert, query, delete APIs are called.\n2. When you update the credentials because you forget the password for instance, the new password is persisted in etcd. Then all the old credentials in the proxy's local cache are invalidated.\n3. The authentication interceptor looks for the records from local cache first. If the credentials in the cache is not correct, the RPC call to fetch the most updated record from root coord will be triggered. And the credentials in the local cache are updated accordingly. \n\n\n\n \n \n#### How to manage user authentication in the Milvus vector database\n\nTo enable authentication, you need to first set `common.security.authorizationEnabled` to `true` when configuring Milvus in the `milvus.yaml` file.\n\nOnce enabled, a root user will be created for the Milvus instance. This root user can use the initial password of `Milvus` to connect to the Milvus vector database. \n\n```\nfrom pymilvus import connections\nconnections.connect(\n alias='default',\n host='localhost',\n port='19530',\n user='root_user',\n password='Milvus',\n)\n```\n\nWe highly recommend changing the password of the root user when starting Milvus for the first time.\n\nThen root user can further create more new users for authenticated access by running the following command to create new users.\n\n```\nfrom pymilvus import utility\nutility.create_credential('user', 'password', using='default') \n```\n\nThere are two things to remember when creating new users:\n\n1. As for the new username, it can not exceed 32 characters in length and must start with a letter. Only underscores, letters, or numbers are allowed in the username. For example a username of \"2abc!\" is not accepted.\n\n2. As for the password, its length should be 6-256 characters.\n\nOnce the new credential is set up, the new user can connect to the Milvus instance with the username and password.\n\n```\nfrom pymilvus import connections\nconnections.connect(\n alias='default',\n host='localhost',\n port='19530',\n user='user',\n password='password',\n)\n```\n\nLike all authentication processes, you do not have to worry if you forget the password. The password for an existing user can be reset with the following command.\n\n```\nfrom pymilvus import utility\nutility.reset_password('user', 'new_password', using='default')\n```\n\nRead the [Milvus documentation](https://milvus.io/docs/v2.1.x/authenticate.md) to learn more about user authentication.\n\n### TLS connection\n\nTransport layer security (TLS) is a type of authentication protocol to provide communications security in a computer network. TLS uses certificates to provide authentication services between two or more communicating parties.\n\n#### How to enable TLS in the Milvus vector database\n\nTo enable TLS in Milvus, you need to first run the following command to perpare two files for generating the certificate: a default OpenSSL configuration file named `openssl.cnf` and a file named `gen.sh` used to generate relevant certificates.\n\n```\nmkdir cert \u0026\u0026 cd cert\ntouch openssl.cnf gen.sh\n```\n\nThen you can simply copy and paste the configuration we provide [here](https://milvus.io/docs/v2.1.x/tls.md#Create-files) to the two files. Or you can also make modifications based on our configuration to better suit your application.\n\nWhen the two files are ready, you can run the `gen.sh` file to create nine certificate files. Likewise, you can also modify the configurations in the nine certificate files to suit your need.\n\n```\nchmod +x gen.sh\n./gen.sh\n```\n\nThere is one final step before you can connect to the Milvus service with TLS. You have to set `tlsEnabled` to `true` and configure the file paths of `server.pem`, `server.key`, and `ca.pem` for the server in `config/milvus.yaml`. The code below is an example.\n\n```\ntls:\n serverPemPath: configs/cert/server.pem\n serverKeyPath: configs/cert/server.key\n caPemPath: configs/cert/ca.pem\n\ncommon:\n security:\n tlsEnabled: true\n```\n\nThen you are all set and can connect to the Milvus service with TLS as long as you specify the file paths of `client.pem`, `client.key`, and `ca.pem` for the client when using the Milvus connection SDK. The code below is also an example.\n\n```\nfrom pymilvus import connections\n\n_HOST = '127.0.0.1'\n_PORT = '19530'\n\nprint(f\"\\nCreate connection...\")\nconnections.connect(host=_HOST, port=_PORT, secure=True, client_pem_path=\"cert/client.pem\",\n client_key_path=\"cert/client.key\",\n ca_pem_path=\"cert/ca.pem\", server_name=\"localhost\")\nprint(f\"\\nList connections:\")\nprint(connections.list_connections())\n```\n \n## What's next\n\nWith the official release of Milvus 2.1, we have prepared a series of blogs introducing the new features. Read more in this blog series:\n\n- [How to Use String Data to Empower Your Similarity Search Applications](https://milvus.io/blog/2022-08-08-How-to-use-string-data-to-empower-your-similarity-search-applications.md)\n- [Using Embedded Milvus to Instantly Install and Run Milvus with Python](https://milvus.io/blog/embedded-milvus.md)\n- [Increase Your Vector Database Read Throughput with In-Memory Replicas](https://milvus.io/blog/in-memory-replicas.md)\n- [Understanding Consistency Level in the Milvus Vector Database](https://milvus.io/blog/understanding-consistency-levels-in-the-milvus-vector-database.md)\n- [Understanding Consistency Level in the Milvus Vector Database (Part II)](https://milvus.io/blog/understanding-consistency-levels-in-the-milvus-vector-database-2.md)\n- [How Does the Milvus Vector Database Ensure Data Security?](https://milvus.io/blog/data-security.md)\n\n\n","title":"How Does the Milvus Vector Database Ensure Data Security?","metaData":{}},{"id":"understanding-consistency-levels-in-the-milvus-vector-database.md","author":"Chenglong Li","desc":"Learn about the four levels of consistency - strong, bounded staleness, session, and eventual supported in the Milvus vector database.","tags":["Engineering"],"canonicalUrl":"https://milvus.io/blog/understanding-consistency-levels-in-the-milvus-vector-database.md","date":"2022-08-29T00:00:00.000Z","cover":"https://assets.zilliz.com/1280_X1280_0e0d4bc107.png","href":"/blog/understanding-consistency-levels-in-the-milvus-vector-database.md","content":"\n\n\n\u003e This article is written by [Chenglong Li](https://github.com/JackLCL) and transcreated by [Angela Ni](https://www.linkedin.com/in/yiyun-n-2aa713163/).\n\nHave you ever wondered why sometimes the data you have deleted from the Mlivus vector database still appear in the search results? \n\nA very likely reason is that you have not set the appropriate consistency level for your application. Consistency level in a distributed vector database is critical as it determines at which point a particular data write can be read by the system. \n\nTherefore, this article aims to demystify the concept of consistency and delve into the levels of consistency supported by the Milvus vector database.\n\n\n**Jump to:**\n- [What is consistency](#What-is-consistency)\n- [Four levels of consistency in the Milvus vector database](#Four-levels-of-consistency-in-the-Milvus-vector-database)\n - [Strong](#Strong)\n - [Bounded staleness](#Bounded-staleness)\n - [Session](#Session)\n - [Eventual](#Eventual)\n\n\n## What is consistency\n\nBefore getting started, we need to first clarify the connotation of consistency in this article as the word \"consistency\" is an overloaded term in the computing industry. Consistency in a distributed database specifically refers to the property that ensures every node or replica has the same view of data when writing or reading data at a given time. Therefore, here we are talking about consistency as in the [CAP theorem](https://en.wikipedia.org/wiki/CAP_theorem). \n\nFor serving massive online businesses in the modern world, multiple replicas are commonly adopted. For instance, online e-commerce giant Amazon replicates its orders or SKU data across multiple data centers, zones, or even countries to ensure high system availability in the event of a system crash or failure. This poses a challenge to the system - data consistency across multiple replicas. Without consistency, it is very likely that the deleted item in your Amazon cart reappears, causing very bad user experience. \n\nHence, we need different data consistency levels for different applications. And luckily, Milvus, a database for AI, offers flexibility in consistency level and you can set the consistency level that best suits your application.\n\n\n### Consistency in the Milvus vector database\n\nThe concept of consistency level was first introduced with the release of Milvus 2.0. The 1.0 version of Milvus was not a distributed vector database so we did not involve tunable levels of consistency then. Milvus 1.0 flushes data every second, meaning that new data are almost immediately visible upon their insertion and Milvus reads the most updated data view at the exact time point when a vector similarity search or query request comes. \n\nHowever, Milvus was refactored in its 2.0 version and [Milvus 2.0 is a distributed vector database](https://milvus.io/blog/deep-dive-1-milvus-architecture-overview.md) based on a pub-sub mechanism. The [PACELC](https://en.wikipedia.org/wiki/PACELC_theorem) theorem points out that a distributed system must trade off among consistency, availability, and latency. Furthermore, different levels of consistency serve for different scenarios. Therefore, the concept of consistency was introduced in [Milvus 2.0](https://milvus.io/blog/2022-1-25-annoucing-general-availability-of-milvus-2-0.md) and it supports tuning levels of consistency.\n\n## Four levels of consistency in the Milvus vector database\n\nMilvus supports four levels of consistency: strong, bounded staleness, session, and eventual. And a Milvus user can specify the consistency level when [creating a collection](https://milvus.io/docs/v2.1.x/create_collection.md) or conducting a [vector similarity search](https://milvus.io/docs/v2.1.x/search.md) or [query](https://milvus.io/docs/v2.1.x/query.md). This section will continue to explain how these four levels of consistency are different and which scenario are they best suited for. \n\n### Strong\n\nStrong is the highest and the most strict level of consistency. It ensures that users can read the latest version of data. \n\n\n\nAccording to the PACELC theorem, if the consistency level is set to strong, the latency will increase. Therefore, we recommend choosing strong consistency during functional testings to ensure the accuracy of the test results. And strong consistency is also best suited for applications that have strict demand for data consistency at the cost of search speed. An example can be an online financial system dealing with order payments and billing.\n\n### Bounded staleness\n\nBounded staleness, as its name suggests, allows data inconsistency during a certain period of time. However, generally, the data are always globally consistent out of that period of time.\n\n\n\nBounded staleness is suitable for scenarios that needs to control search latency and can accept sporadic data invisibility. For instance, in recommender systems like video recommendation engines, data invisibility once in a while has really small impact on the overall recall rate, but can significantly boost the performance of the recommender system. An example can be an app for tracking the status of your online orders.\n\n### Session\n\nSession ensures that all data writes can be immediately perceived in reads during the same session. In other words, when you write data via one client, the newly inserted data instantaneously become searchable. \n\n\n\nWe recommend choosing session as the consistency level for those scenarios where the demand of data consistency in the same session is high. An example can be deleting the data of a book entry from the library system, and after confirmation of the deletion and refreshing the page (a different session), the book should no longer be visible in search results.\n\n### Eventual\n\nThere is no guaranteed order of reads and writes, and replicas eventually converge to the same state given that no further write operations are done. Under eventual consistency, replicas start working on read requests with the latest updated values. Eventual consistency is the weakest level among the four. \n\n\n\nHowever, according to the PACELC theorem, search latency can be tremendously shortened upon sacrificing consistency. Therefore, eventual consistency is best suited for scenarios that do not have high demand for data consistency but requires blazing-fast search performance. An example can be retrieving reviews and ratings of Amazon products with eventual consistency. \n\n## Endnote\n\nSo going back to the question raised at the beginning of this article, deleted data are still returned as search results because the user has not chosen the proper level of consistency. The default value for consistency level is bounded staleness (`Bounded`) in the Milvus vector database. Therefore, the data read might lag behind and Milvus might happen to read the data view before you conducted delete operations during a similarity search or query. However, this issue is simple to solve. All you need to do is [tune the consistency level](https://milvus.io/docs/v2.1.x/tune_consistency.md) when creating a collection or conducting vector similarity search or query. Simple!\n\nIn the next post, we will unveil the mechanism behind and explain how the Milvus vector database achieves different levels of consistency. Stay tuned!\n\n## What's next\n\nWith the official release of Milvus 2.1, we have prepared a series of blogs introducing the new features. Read more in this blog series:\n\n- [How to Use String Data to Empower Your Similarity Search Applications](https://milvus.io/blog/2022-08-08-How-to-use-string-data-to-empower-your-similarity-search-applications.md)\n- [Using Embedded Milvus to Instantly Install and Run Milvus with Python](https://milvus.io/blog/embedded-milvus.md)\n- [Increase Your Vector Database Read Throughput with In-Memory Replicas](https://milvus.io/blog/in-memory-replicas.md)\n- [Understanding Consistency Level in the Milvus Vector Database](https://milvus.io/blog/understanding-consistency-levels-in-the-milvus-vector-database.md)\n- [Understanding Consistency Level in the Milvus Vector Database (Part II)](https://milvus.io/blog/understanding-consistency-levels-in-the-milvus-vector-database-2.md)\n- [How Does the Milvus Vector Database Ensure Data Security?](https://milvus.io/blog/data-security.md)\n\n\n","title":"Understanding Consistency Level in the Milvus Vector Database","metaData":{}},{"id":"in-memory-replicas.md","author":"Congqi Xia","desc":"Use in-memory replicas to enhance read throughput and the utilization of hardware resources.","tags":["Engineering"],"canonicalUrl":"https://milvus.io/blog/in-memory-replicas.md","date":"2022-08-22T00:00:00.000Z","cover":"https://assets.zilliz.com/in_memory_replica_af1fa21d61.png","href":"/blog/in-memory-replicas.md","content":"\n\n\n\n\u003e This article is co-authored by [Congqi Xia](https://github.com/congqixia) and [Angela Ni](https://www.linkedin.com/in/yiyun-n-2aa713163/).\n\n\nWith its official release, Milvus 2.1 comes with many new features to provide convenience and a better user experience. Though the concept of in-memory replica is nothing new to the world of distributed databases, it is a critical feature that can help you boost system performance and enhance system availability in an effortless way. Therefore, this post sets out to explain what in-memory replica is and why it is important, and then introduces how to enable this new feature in Milvus, a vector database for AI.\n\n**Jump to:**\n\n- [Concepts related to in-memory replica](#Concepts-related-to-in-memory-replica)\n\n- [What is in-memory replica?](#What-is-in-memory-replica)\n\n- [Why are in-memory replicas important?](#Why-are-in-memory-replicas-important)\n\n- [Enable in-memory replicas in the Milvus vector database](#Enable-in-memory-replicas-in-the-Milvus-vector-database)\n\n\n\n## Concepts related to in-memory replica\n\nBefore getting to know what in-memory replica is and why it is important, we need to first understand a few relevant concepts including replica group, shard replica, streaming replica, historical replica, and shard leader. The image below is an illustration of these concepts.\n\n\n\n### Replica group\n\nA replica group consists of multiple [query nodes](https://milvus.io/docs/v2.1.x/four_layers.md#Query-node) that are responsible for handling historical data and replicas.\n\n### Shard replica\n\nA shard replica consists of a streaming replica and a historical replica, both belonging to the same [shard](https://milvus.io/blog/deep-dive-1-milvus-architecture-overview.md#Shard) (i.e DML channel). Multiple shard replicas make up a replica group. And the exact number of shard replicas in a replica group is determined by the number of shards in a specified collection.\n\n### Streaming replica\n\nA streaming replica contains all the [growing segments](https://milvus.io/docs/v2.1.x/glossary.md#Segment) from the same DML channel. Technically speaking, a streaming replica should be served by only one query node in one replica.\n\n### Historical replica\n\nA historical replica contains all the sealed segments from the same DML channel. The sealed segments of one historical replica can be distributed on several query nodes within the same replica group.\n\n### Shard leader\n\nA shard leader is the query node serving the streaming replica in a shard replica.\n\n\n\n## What is in-memory replica?\n\nEnabling in-memory replicas allows you to load data in a collection on multiple query nodes so that you can leverage extra CPU and memory resources. This feature is very useful if you have a relatively small dataset but want to increase read throughput and enhance the utilization of hardware resources.\n\nThe Milvus vector database holds one replica for each segment in memory for now. However, with in-memory replicas, you can have multiple replications of a segment on different query nodes. This means if one query node is conducting a search on a segment, an incoming new search request can be assigned to another idle query node as this query node has a replication of exactly the same segment. \n\nIn addition, if we have multiple in-memory replicas, we can better cope with the situation in which a query node crashes. Before, we have to wait for the segment to be reloaded in order to continue and search on another query node. However, with in-memory replication, the search request can be resent to a new query node immediately without having to reload the data again.\n\n\n\n\n\n## Why are in-memory replicas important?\n\nOne of the most significant benefits of enabling in-memory replicas is the increase in overall QPS (query per second) and throughput. Furthermore, multiple segment replicas can be maintained and the system is more resilient in the face of a failover.\n\n\n\n## Enable in-memory replicas in the Milvus vector database\n\nEnabling the new feature of in-memory replicas is effortless in the Milvus vector database. All you need to do is simply specify the number of replicas you want when loading a collection (ie. calling `collection.load()`). \n\nIn the following example tutorial, we suppose you have already [created a collection](https://milvus.io/docs/v2.1.x/create_collection.md) named \"book\" and [inserted data](https://milvus.io/docs/v2.1.x/insert_data.md) into it. Then you can run the following command to create two replicas when [loading](https://milvus.io/docs/v2.1.x/load_collection.md) a book collection.\n\n```\nfrom pymilvus import Collection\ncollection = Collection(\"book\") # Get an existing collection.\ncollection.load(replica_number=2) # load collection as 2 replicas\n```\n\nYou can flexibly modify the number of replicas in the example code above to best suit your application scenario. Then you can directly conduct a vector similarity [search](https://milvus.io/docs/v2.1.x/search.md) or [query](https://milvus.io/docs/v2.1.x/query.md) on multiple replicas without running any extra commands. However, it should be noted that the maximum number of replicas allowed is limited by the total amount of usable memory to run the query nodes. If the number of replicas you specify exceeds the limitations of usable memory, an error will be returned during data load. \n\nYou can also check the information of the in-memory replicas you created by running `collection.get_replicas()`. The information of replica groups and the corresponding query nodes and shards will be returned. The following is an example of the output. \n\n```\nReplica groups:\n- Group: \u003cgroup_id:435309823872729305\u003e, \u003cgroup_nodes:(21, 20)\u003e, \u003cshards:[Shard: \u003cchannel_name:milvus-zong-rootcoord-dml_27_435367661874184193v0\u003e, \u003cshard_leader:21\u003e, \u003cshard_nodes:[21]\u003e, Shard: \u003cchannel_name:milvus-zong-rootcoord-dml_28_435367661874184193v1\u003e, \u003cshard_leader:20\u003e, \u003cshard_nodes:[20, 21]\u003e]\u003e\n- Group: \u003cgroup_id:435309823872729304\u003e, \u003cgroup_nodes:(25,)\u003e, \u003cshards:[Shard: \u003cchannel_name:milvus-zong-rootcoord-dml_28_435367661874184193v1\u003e, \u003cshard_leader:25\u003e, \u003cshard_nodes:[25]\u003e, Shard: \u003cchannel_name:milvus-zong-rootcoord-dml_27_435367661874184193v0\u003e, \u003cshard_leader:25\u003e, \u003cshard_nodes:[25]\u003e]\u003e\n```\n\n## What's next\n\nWith the official release of Milvus 2.1, we have prepared a series of blogs introducing the new features. Read more in this blog series:\n\n- [How to Use String Data to Empower Your Similarity Search Applications](https://milvus.io/blog/2022-08-08-How-to-use-string-data-to-empower-your-similarity-search-applications.md)\n- [Using Embedded Milvus to Instantly Install and Run Milvus with Python](https://milvus.io/blog/embedded-milvus.md)\n- [Increase Your Vector Database Read Throughput with In-Memory Replicas](https://milvus.io/blog/in-memory-replicas.md)\n- [Understanding Consistency Level in the Milvus Vector Database](https://milvus.io/blog/understanding-consistency-levels-in-the-milvus-vector-database.md)\n- [Understanding Consistency Level in the Milvus Vector Database (Part II)](https://milvus.io/blog/understanding-consistency-levels-in-the-milvus-vector-database-2.md)\n- [How Does the Milvus Vector Database Ensure Data Security?](https://milvus.io/blog/data-security.md)\n","title":"Increase Your Vector Database Read Throughput with In-Memory Replicas","metaData":{}},{"id":"2022-08-16-Benchmark-Quick-Setup.md","author":"Yanliang Qiao","desc":"Follow our step-by-step guide to perform a Milvus 2.1 benchmark by yourself.","tags":["Engineering"],"canonicalUrl":"https://milvus.io/blog/2022-08-16-A-Quick-Guide-to-Benchmarking-Milvus-2-1.md","date":"2022-08-16T00:00:00.000Z","cover":"https://assets.zilliz.com/Benchmark_Quick_Setup_58cc8eed5b.png","href":"/blog/2022-08-16-Benchmark-Quick-Setup.md","content":"\n\n\nRecently, we have updated the [benchmark report of Milvus 2.1](https://milvus.io/docs/v2.1.x/benchmark.md). Tests with a dataset of 1 million vectors have proved that QPS can be dramatically increased by merging small-[nq](https://milvus.io/docs/v2.1.x/benchmark.md#Terminology) queries.\n\nHere are some simple scripts for you to easily reproduce the tests.\n\n## Procedures\n\n1. Deploy a Milvus standalone or cluster. In this case, the IP address of the Milvus server is 10.100.31.105.\n\n2. Deploy a client. In this case, we use Ubuntu 18.04 and Python 3.8.13 for the deployment. Run the following code to install PyMilvus 2.1.1.\n\n```\npip install pymilvus==2.1.1\n```\n\n3. Download and copy the following files to the same working directory as the client. In this case, the working directory is `/go_ben`.\n\n - [`collection_prepare.py`](https://github.com/milvus-io/milvus-tools/blob/main/benchmark/collection_prepare.py)\n\n - [`go_benchmark.py`](https://github.com/milvus-io/milvus-tools/blob/main/benchmark/go_benchmark.py)\n\n - [`benchmark`](https://github.com/milvus-io/milvus-tools/blob/main/benchmark/benchmark) (for Ubuntu) or [`benchmark-mac`](https://github.com/milvus-io/milvus-tools/blob/main/benchmark/benchmark-mac) (for macOS)\n \n **Note:**\n \n - `benchmark` and `benchmark-mac` are executable files developed and compiled using Go SDK 2.1.1. They are only used to conduct a concurrent search. \n \n - For Ubuntu users, please download `benchmark`; for macOS users, please download `benchmark-mac`.\n \n - Executable permissions are required to access `benchmark` or `benchmark-mac`. \n \n - Mac users need to trust the `benchmark-mac` file by configuring Security \u0026 Privacy in System Preferences.\n \n - Settings on concurrent search can be found and modified in the `go_benchmark.py` source code.\n \n\n4. Create a collection and insert vector data.\n\n```\nroot@milvus-pytest:/go_ben# python collection_prepare.py 10.100.31.105 \n```\n\n5. Open `/tmp/collection_prepare.log` to check the running result.\n\n```\n...\n08/11/2022 17:33:34 PM - INFO - Build index costs 263.626\n08/11/2022 17:33:54 PM - INFO - Collection prepared completed\n```\n\n6. Call `benchmark` (or `benchmark-mac` on macOS) to conduct a concurrent search.\n\n```\nroot@milvus-pytest:/go_ben# python go_benchmark.py 10.100.31.105 ./benchmark\n[write_json_file] Remove file(search_vector_file.json).\n[write_json_file] Write json file:search_vector_file.json done.\nParams of go_benchmark: ['./benchmark', 'locust', '-u', '10.100.31.105:19530', '-q', 'search_vector_file.json', '-s', '{\\n \"collection_name\": \"random_1m\",\\n \"partition_names\": [],\\n \"fieldName\": \"embedding\",\\n \"index_type\": \"HNSW\",\\n \"metric_type\": \"L2\",\\n \"params\": {\\n \"sp_value\": 64,\\n \"dim\": 128\\n },\\n \"limit\": 1,\\n \"expr\": null,\\n \"output_fields\": [],\\n \"timeout\": 600\\n}', '-p', '10', '-f', 'json', '-t', '60', '-i', '20', '-l', 'go_log_file.log']\n[2022-08-11 11:37:39.811][ INFO] - Name # reqs # fails | Avg Min Max Median | req/s failures/s (benchmark_run.go:212:sample)\n[2022-08-11 11:37:39.811][ INFO] - go search 9665 0(0.00%) | 20.679 6.499 81.761 12.810 | 483.25 0.00 (benchmark_run.go:213:sample)\n[2022-08-11 11:37:59.811][ INFO] - Name # reqs # fails | Avg Min Max Median | req/s failures/s (benchmark_run.go:212:sample)\n[2022-08-11 11:37:59.811][ INFO] - go search 19448 0(0.00%) | 20.443 6.549 78.121 13.401 | 489.22 0.00 (benchmark_run.go:213:sample)\n[2022-08-11 11:38:19.811][ INFO] - Name # reqs # fails | Avg Min Max Median | req/s failures/s (benchmark_run.go:212:sample)\n[2022-08-11 11:38:19.811][ INFO] - go search 29170 0(0.00%) | 20.568 6.398 76.887 12.828 | 486.15 0.00 (benchmark_run.go:213:sample)\n[2022-08-11 11:38:19.811][ DEBUG] - go search run finished, parallel: 10(benchmark_run.go:95:benchmark)\n[2022-08-11 11:38:19.811][ INFO] - Name # reqs # fails | Avg Min Max Median | req/s failures/s (benchmark_run.go:159:samplingLoop)\n[2022-08-11 11:38:19.811][ INFO] - go search 29180 0(0.00%) | 20.560 6.398 81.761 13.014 | 486.25 0.00 (benchmark_run.go:160:samplingLoop)\nResult of go_benchmark: {'response': True, 'err_code': 0, 'err_message': ''} \n```\n\n7. Open the `go_log_file.log` file under the current directory to check the detailed search log. The following is the search information you can find in the search log.\n - reqs: number of search requests from the moment when concurrency happens to the current moment (the current time-span)\n \n - fails: number of failed requests as a percentage of reqs in the current time-span\n \n - Avg: average request response time in the current time-span (unit: milliseconds)\n \n - Min: minimum request response time in the current time-span (unit: milliseconds)\n \n - Max: maximum request response time in the current time-span (unit: milliseconds)\n \n - Median: median request response time in the current time-span (unit: milliseconds)\n \n - req/s: number of requests per second, i.e. QPS\n \n - failures/s: average number of failed requests per second in the current time-span\n\n## Downloading Scripts and Executable Files\n\n - [collection_prepare.py](https://github.com/milvus-io/milvus-tools/blob/main/benchmark/collection_prepare.py)\n\n - [go_benchmark.py](https://github.com/milvus-io/milvus-tools/blob/main/benchmark/go_benchmark.py)\n\n - [benchmark](https://github.com/milvus-io/milvus-tools/blob/main/benchmark/benchmark) for Ubuntu\n \n - [benchmark-mac](https://github.com/milvus-io/milvus-tools/blob/main/benchmark/benchmark-mac) for macOS\n \n\n## What's next\n\nWith the official release of Milvus 2.1, we have prepared a series of blogs introducing the new features. Read more in this blog series:\n\n- [How to Use String Data to Empower Your Similarity Search Applications](https://milvus.io/blog/2022-08-08-How-to-use-string-data-to-empower-your-similarity-search-applications.md)\n- [Using Embedded Milvus to Instantly Install and Run Milvus with Python](https://milvus.io/blog/embedded-milvus.md)\n- [Increase Your Vector Database Read Throughput with In-Memory Replicas](https://milvus.io/blog/in-memory-replicas.md)\n- [Understanding Consistency Level in the Milvus Vector Database](https://milvus.io/blog/understanding-consistency-levels-in-the-milvus-vector-database.md)\n- [How Does the Milvus Vector Database Ensure Data Security?](https://milvus.io/blog/data-security.md)\n\n","title":"A Quick Guide to Benchmarking Milvus 2.1","metaData":{}},{"id":"embedded-milvus.md","author":"Alex Gao","desc":"A Python user-friendly Milvus version that makes installation more flexible.","tags":["Engineering"],"canonicalUrl":"https://milvus.io/blog/embedded-milvus.md","date":"2022-08-15T00:00:00.000Z","cover":"https://assets.zilliz.com/embeddded_milvus_1_8132468cac.png","href":"/blog/embedded-milvus.md","content":"\n\n\n\n\u003e This article is co-authored by [Alex Gao](https://github.com/soothing-rain/) and [Angela Ni](https://www.linkedin.com/in/yiyun-n-2aa713163/).\n\nMilvus is an open-source vector database for AI applications. It provides a variety of installation methods including building from source code, and installing Milvus with Docker Compose/Helm/APT/YUM/Ansible. Users can choose one of the installation methods depending on their operating systems and preferences. However, there are many data scientists and AI engineers in the Milvus community who work with Python and yearn for a much simpler installation method than the currently available ones.\n\nTherefore, we released embedded Milvus, a Python user-friendly version, along with Milvus 2.1 to empower more Python developers in our community. This article introduces what embedded Milvus is and provides instructions on how to install and use it.\n\n**Jump to:**\n\n- [An overview of embedded Milvus](#An-overview-of-embedded-Milvus)\n - [When to use embedded Milvus?](#When-to-use-embedded-Milvus)\n - [A comparison of different modes of Milvus](#A-comparison-of-different-modes-of-Milvus)\n- [How to install embedded Milvus](#How-to-install-embedded-Milvus)\n- [Start and stop embedded Milvus](#Start-and-stop-embedded-Milvus)\n\n## An overview of embedded Milvus\n\n[Embedded Milvus](https://github.com/milvus-io/embd-milvus) enables you to quickly install and use Milvus with Python. It can quickly bring up a Milvus instance and allows you to start and stop the Milvus service whenever you wish to. All data and logs are persisted even if you stop embedded Milvus. \n\nEmbedded Milvus itself does not have any internal dependencies and do not require pre-installing and running any third-party dependencies like etcd, MinIO, Pulsar, etc.\n\nEverything you do with embedded Milvus, and every piece of code you write for it can be safely migrated to other Milvus modes - standalone, cluster, cloud version, etc. This reflects one of the most distinctive features of embedded Milvus - **\"Write once, run anywhere\"**.\n\n### When to use embedded Milvus?\n\nEmbedded Milvus and [PyMilvus](https://milvus.io/docs/v2.1.x/install-pymilvus.md) are constructed for different purposes. You may consider choosing embedded Milvus in the following scenarios:\n\n- You want to use Milvus without installing Milvus in any of the ways provided [here](https://milvus.io/docs/v2.1.x/install_standalone-docker.md).\n\n- You want to use Milvus without keeping a long-running Milvus process in your machine.\n\n- You want to quickly use Milvus without starting a separate Milvus process and other required components like etcd, MinIO, Pulsar, etc.\n\nIt is suggested that you should **NOT** use embedded Milvus:\n\n- In a production environment. (_To use Milvus for production, consider Milvus cluster or [Zilliz cloud](https://zilliz.com/cloud), a fully managed Milvus service._)\n\n- If you have a high demand for performance. (_Comparatively speaking, embedded Milvus might not provide the best performance._)\n\n### A comparison of different modes of Milvus\n\nThe table below compares several modes of Milvus: standalone, cluster, embedded Milvus, and the Zilliz Cloud, a fully managed Milvus service.\n\n\n\n## How to install embedded Milvus?\n\nBefore installing embedded Milvus, you need to first ensure that you have installed Python 3.6 or later. Embedded Milvus supports the following operating systems: \n\n- Ubuntu 18.04\n\n- Mac x86_64 \u003e= 10.4\n\n- Mac M1 \u003e= 11.0\n\nIf the requirements are met, you can run `$ python3 -m pip install milvus` to install embedded Milvus. You can also add the version in the command to install a specific version of embedded Milvus. For instance, if you want to install the 2.1.0 version, run `$ python3 -m pip install milvus==2.1.0`. And later when new version of embedded Milvus is released, you can also run `$ python3 -m pip install --upgrade milvus` to upgrade embedded Milvus to the latest version.\n\nIf you are an old user of Milvus who has already installed PyMilvus before and wants to install embedded Milvus, you can run `$ python3 -m pip install --no-deps milvus`.\n\nAfter running the installation command, you need to create a data folder for embedded Milvus under `/var/bin/e-milvus` by running the following command:\n\n```\nsudo mkdir -p /var/bin/e-milvus\nsudo chmod -R 777 /var/bin/e-milvus\n```\n\n## Start and stop embedded Milvus\n\nWhen the installation is successful, you can start the service.\n\nIf you are running embedded Milvus for the first time you need to import Milvus and set up embedded Milvus first.\n\n```\n$ python3\nPython 3.9.10 (main, Jan 15 2022, 11:40:53)\n[Clang 13.0.0 (clang-1300.0.29.3)] on darwin\nType \"help\", \"copyright\", \"credits\" or \"license\" for more information.\n\u003e\u003e\u003e import milvus\n\u003e\u003e\u003e milvus.before()\nplease do the following if you have not already done so:\n1. install required dependencies: bash /var/bin/e-milvus/lib/install_deps.sh\n2. export LD_PRELOAD=/SOME_PATH/embd-milvus.so\n3. export LD_LIBRARY_PATH=$LD_LIBRARY_PATH:/usr/lib:/usr/local/lib:/var/bin/e-milvus/lib/\n\u003e\u003e\u003e\n```\n\nIf you have successfully started embedded Milvus before and comes back to restart it, you can directly run `milvus.start()` after importing Milvus.\n\n```\n$ python3\nPython 3.9.10 (main, Jan 15 2022, 11:40:53)\n[Clang 13.0.0 (clang-1300.0.29.3)] on darwinType \"help\", \"copyright\", \"credits\" or \"license\" for more information.\n\u003e\u003e\u003e import milvus\n\u003e\u003e\u003e milvus.start()\n\u003e\u003e\u003e\n```\n\nYou will see the following output if you have successfully started the embedded Milvus service.\n\n```\n---Milvus Proxy successfully initialized and ready to serve!---\n```\n\nAfter the service starts, you can start another terminal window and run the example code of \"[Hello Milvus](https://github.com/milvus-io/embd-milvus/blob/main/milvus/examples/hello_milvus.py)\" to play around with embedded Milvus!\n\n```\n# Download hello_milvus script\n$ wget https://raw.githubusercontent.com/milvus-io/pymilvus/v2.1.0/examples/hello_milvus.py\n# Run Hello Milvus \n$ python3 hello_milvus.py\n```\n\nWhen you are done with using embedded Milvus, we recommend stopping it gracefully and clean up the environment variables by run the following command or press Ctrl-D.\n\n```\n\u003e\u003e\u003e milvus.stop()\nif you need to clean up the environment variables, run:\nexport LD_PRELOAD=\nexport LD_LIBRARY_PATH=\n\u003e\u003e\u003e\n\u003e\u003e\u003e exit()\n```\n\n## What's next\n\nWith the official release of Milvus 2.1, we have prepared a series of blogs introducing the new features. Read more in this blog series:\n\n- [How to Use String Data to Empower Your Similarity Search Applications](https://milvus.io/blog/2022-08-08-How-to-use-string-data-to-empower-your-similarity-search-applications.md)\n- [Using Embedded Milvus to Instantly Install and Run Milvus with Python](https://milvus.io/blog/embedded-milvus.md)\n- [Increase Your Vector Database Read Throughput with In-Memory Replicas](https://milvus.io/blog/in-memory-replicas.md)\n- [Understanding Consistency Level in the Milvus Vector Database](https://milvus.io/blog/understanding-consistency-levels-in-the-milvus-vector-database.md)\n- [Understanding Consistency Level in the Milvus Vector Database (Part II)](https://milvus.io/blog/understanding-consistency-levels-in-the-milvus-vector-database-2.md)\n- [How Does the Milvus Vector Database Ensure Data Security?](https://milvus.io/blog/data-security.md)\n","title":"Using Embedded Milvus to Instantly Install and Run Milvus with Python","metaData":{}},{"id":"2022-08-08-How-to-use-string-data-to-empower-your-similarity-search-applications.md","author":"Xi Ge","desc":"Use string data to streamline the process of building your own similarity search applications.","tags":["Engineering"],"canonicalUrl":"https://milvus.io/blog/2022-08-08-How-to-use-string-data-to-empower-your-similarity-search-applications.md","date":"2022-08-08T00:00:00.000Z","cover":"https://assets.zilliz.com/string_6129ce83e6.png","href":"/blog/2022-08-08-How-to-use-string-data-to-empower-your-similarity-search-applications.md","content":"\n\n\nMilvus 2.1 comes with [some significant updates](https://milvus.io/blog/2022-08-05-whats-new-in-milvus-2-1.md) which make working with Milvus a lot easier. One of them is the support of string data type. Right now Milvus [supports data types](https://milvus.io/docs/v2.1.x/schema.md#Supported-data-type) including strings, vectors, Boolean, integers, floating-point numbers, and more. \n\nThis article presents an introduction to the support of string data type. Read and learn what you can do with it and how to use it.\n\n**Jump to:**\n- [What can you do with string data?](#What-can-you-do-with-string-data)\n- [How to manage string data in Milvus 2.1?](#How-to-manage-string-data-in-Milvus-21)\n - [Create a collection](#Create-a-collection)\n - [Insert and delete data](#Insert-data)\n - [Build an index](#Build-an-index)\n - [Hybrid search](#Hybrid-search)\n - [String expressions](#String-expressions)\n\n# What can you do with string data?\n\nThe support of string data type has been one of the functions most expected by users. It both streamlines the process of building an application with the Milvus vector database and accelerates the speed of similarity search and vector query, largely increasing the efficiency and reducing the maintenance cost of whatever application you are working on.\n\nSpecifically, Milvus 2.1 supports VARCHAR data type, which stores character strings of varying length. With the support of VARCHAR data type, you can:\n\n1. Directly manage string data without the help of an external relational database.\n\nThe support of VARCHAR data type enables you to skip the step of converting strings into other data types when inserting data into Milvus. Let's say you're working on a book search system for your own online bookstore. You are creating a book dataset and want to identify the books with their names. While in previous versions where Milvus does not support the string data type, before inserting data into MIilvus, you may need to first transform the strings (the names of the books) into book IDs with the help of a relational database like MySQL. Right now, as string data type is supported, you can simply create a string field and directly enter the book names instead of their ID numbers.\n\nThe convenience also goes to the search and query process. Imagine there is a client whose favourite book is *Hello Milvus*. You want to search in the system for similar books and recommend them to the client. In previous versions of Milvus, the system will only return you book IDs and you need to take an extra step to check the corresponding book information in a relational database. But in Milvus 2.1, you can directly get the book names as you have already created a string field with book names in it.\n\nIn a word, the support of string data type saves you the effort to turn to other tools to manage string data, which greatly simplifies the development process.\n\n2. Accelerate the speed of [hybrid search](https://milvus.io/docs/v2.1.x/hybridsearch.md) and [vector query](https://milvus.io/docs/v2.1.x/query.md) through attribute filtering.\n\nLike other scalar data types, VARCHAR can be used for attribute filtering in hybrid search and vector query through Boolean expression. It is particularly worth mentioning that Milvus 2.1 adds the operator `like`, which enables you to perform prefix matching. Also, you can perform exact matching using the operator `==`.\n\nBesides, a MARISA-trie based inverted index is supported to accelerate hybrid search and query. Continue to read and find out all the string expressions you may want to know to perform attribute filtering with string data.\n\n# How to manage string data in Milvus 2.1?\n\nNow we know the string data type is extremely useful, but when exactly do we need to use this data type in building our own applications? In the following, you will see some code examples of scenarios that may involve string data, which will give you a better understanding of how to manage VARCHAR data in Milvus 2.1.\n\n## Create a collection\n\nLet's follow the previous example. You are still working on the book recommender system and want to create a book collection with a primary key field called `book_name`, into which you will insert string data. In this case, you can set the data type as `DataType.VARCHAR`when setting the field schema, as shown in the example below. \n\nNote that when creating a VARCHAR field, it is necessary to specify the maximum character length via the parameter `max_length` whose value can range from 1 to 65,535. In this example, we set the maximum length as 200.\n\n```Python\nfrom pymilvus import CollectionSchema, FieldSchema, DataType\nbook_id = FieldSchema(\n name=\"book_id\", \n dtype=DataType.INT64, \n)\nbook_name = FieldSchema( \n name=\"book_name\", \n dtype=DataType.VARCHAR, \n max_length=200, \n is_primary=True, \n)\nword_count = FieldSchema(\n name=\"word_count\", \n dtype=DataType.INT64, \n)\nbook_intro = FieldSchema(\n name=\"book_intro\", \n dtype=DataType.FLOAT_VECTOR, \n dim=2\n)\nschema = CollectionSchema(\n fields=[book_id, word_count, book_intro], \n description=\"Test book search\"\n)\ncollection_name = \"book\"\n```\n\n## Insert data\n\nNow that the collection is created, we can insert data into it. In the following example, we insert 2,000 rows of randomly generated string data.\n\n```Python\nimport random\ndata = [\n [i for i in range(2000)],\n [\"book_\" + str(i) for i in range(2000)],\n [i for i in range(10000, 12000)],\n [[random.random() for _ in range(2)] for _ in range(2000)],\n]\n```\n\n## Delete data\n\nSuppose two books, named `book_0` and `book_1`, are no longer available in your store, so you want to delete the relevant information from your database. In this case, you can use the term expression `in` to filter the entities to delete, as shown in the example below.\n\nRemember that Milvus only supports deleting entities with clearly specified primary keys, so before running the following code, make sure that you have set the `book_name` field as the primary key field.\n\n```Python\nexpr = \"book_name in [\\\"book_0\\\", \\\"book_1\\\"]\" \nfrom pymilvus import Collection\ncollection = Collection(\"book\") \ncollection.delete(expr)\n```\n\n## Build an Index\n\nMilvus 2.1 supports building scalar indexes, which will greatly accelerate the filtering of string fields. Unlike building a vector index, you don't have to prepare parameters before building a scalar index. Milvus temporarily only supports the dictionary tree (MARISA-trie) index, so the index type of VARCHAR type field is MARISA-trie by default.\n\nYou can specify the index name when building it. If not specified, the default value of `index_name` is `\"_default_idx_\"`. In the example below, we named the index `scalar_index`.\n\n```Python\nfrom pymilvus import Collection\ncollection = Collection(\"book\") \ncollection.create_index(\n field_name=\"book_name\", \n index_name=\"scalar_index\",\n)\n```\n\n## Hybrid search\n\nBy specifying boolean expressions, you can filter the string fields during a vector similarity search. \n\nFor example, if you are searching for books whose intro are most similar to Hello Milvus but only want to get the books whose names start with 'book_2', you can use the operator `like`to perform a prefix match and get the targeted books, as shown in the example below. \n\n```Python\nsearch_param = {\n \"data\": [[0.1, 0.2]],\n \"anns_field\": \"book_intro\",\n \"param\": {\"metric_type\": \"L2\", \"params\": {\"nprobe\": 10}},\n \"limit\": 2,\n \"expr\": \"book_name like \\\"Hello%\\\"\",\n}\nres = collection.search(**search_param)\n```\n\n## String expressions\n\nApart from the newly added operator `like`, other operators, which are already supported in previous versions of Milvus, can also be used for string field filtering. Below are some examples of commonly used [string expressions](https://milvus.io/docs/v2.1.x/boolean.md), where `A` represents a field of type VARCHAR. Remember that all the string expressions below can be logically combined using logical operators, such as AND, OR, and NOT.\n\n### Set operations\n\nYou can use `in` and `not in` to realize set operations, such as `A in [\"str1\", \"str2\"]`.\n\n### Compare two string fields\n\nYou can use relational operators to compare the values of two string fields. Such relational operators include `==`, `!=`, `\u003e`, `\u003e=`, `\u003c`, `\u003c=`. For more information, see [Relational operators](https://milvus.io/docs/v2.1.x/boolean.md#Relational-operators).\n\nNote that string fields can only be compared with other string fields instead of fields of other data types. For example, a field of type VARCHAR cannot be compared with a field of type Boolean or of type integer.\n\n### Compare a field with a constant value\n\nYou can use `==` or `!=` to verify if the value of a field is equal to a constant value. \n\n### Filter fields with a single range\n\nYou can use `\u003e`, `\u003e=`, `\u003c`, `\u003c=` to filter string fields with a single range, such as `A \u003e \"str1\"`. \n\n### Prefix matching\n\nAs mentioned earlier, Milvus 2.1 adds the operator `like` for prefix matching, such as `A like \"prefix%\"`. \n\n## What's next\n\nWith the official release of Milvus 2.1, we have prepared a series of blogs introducing the new features. Read more in this blog series:\n\n- [How to Use String Data to Empower Your Similarity Search Applications](https://milvus.io/blog/2022-08-08-How-to-use-string-data-to-empower-your-similarity-search-applications.md)\n- [Using Embedded Milvus to Instantly Install and Run Milvus with Python](https://milvus.io/blog/embedded-milvus.md)\n- [Increase Your Vector Database Read Throughput with In-Memory Replicas](https://milvus.io/blog/in-memory-replicas.md)\n- [Understanding Consistency Level in the Milvus Vector Database](https://milvus.io/blog/understanding-consistency-levels-in-the-milvus-vector-database.md)\n- [Understanding Consistency Level in the Milvus Vector Database (Part II)](https://milvus.io/blog/understanding-consistency-levels-in-the-milvus-vector-database-2.md)\n- [How Does the Milvus Vector Database Ensure Data Security?](https://milvus.io/blog/data-security.md)\n","title":"How to Use String Data to Empower Your Similarity Search Applications","metaData":{}},{"id":"2022-08-05-whats-new-in-milvus-2-1.md","author":"Xiaofan Luan","desc":"Milvus, the open-source vector database, now has performance and usability improvements that users have long been anticipating.","canonicalUrl":"https://milvus.io/blog/2022-08-05-whats-new-in-milvus-2-1.md","date":"2022-08-05T00:00:00.000Z","cover":"https://assets.zilliz.com/What_s_New_in_2_1_2_a0660df2a5.png","tags":["News"],"href":"/blog/2022-08-05-whats-new-in-milvus-2-1.md","content":"\n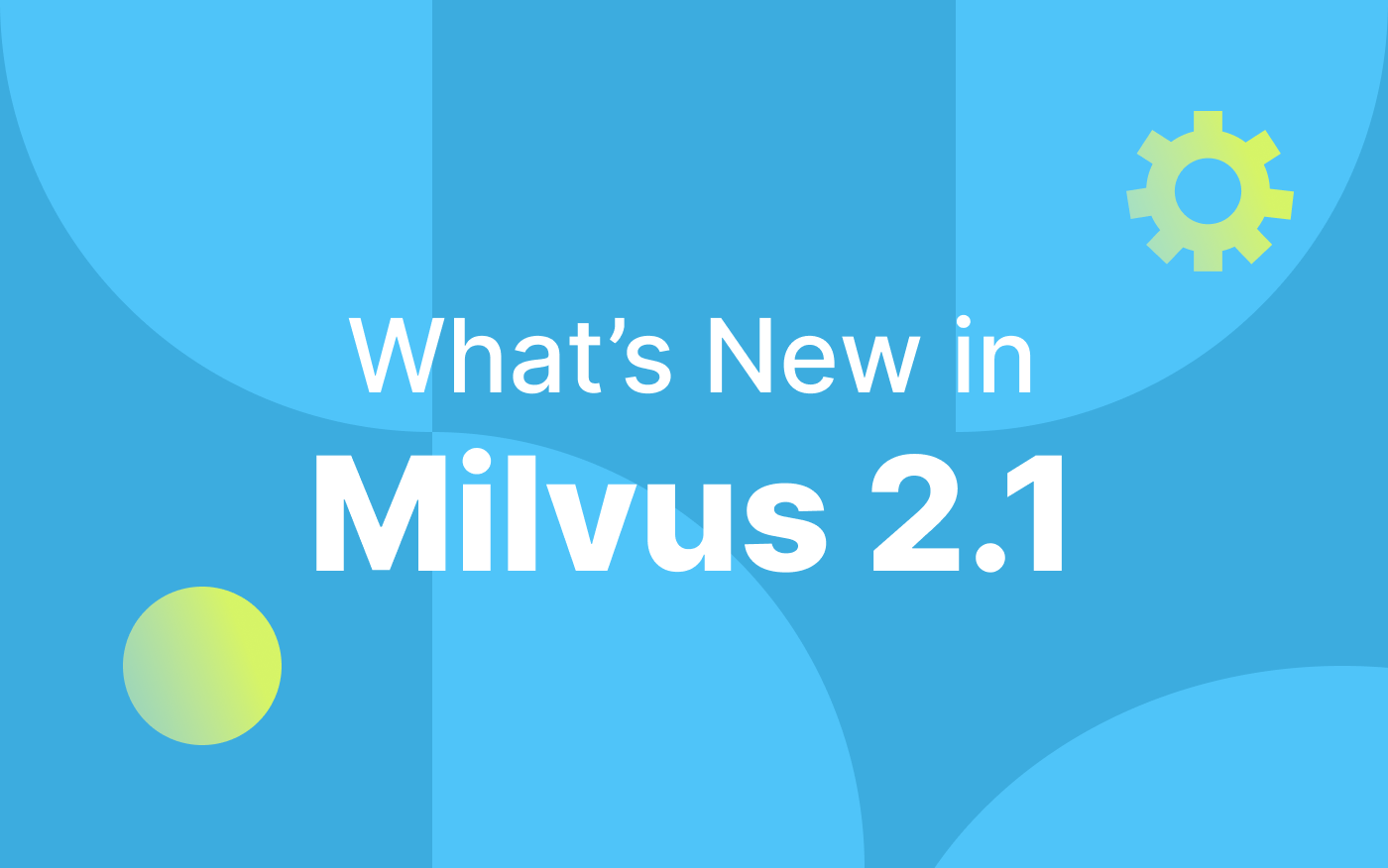\n\nWe are very glad to announce the\n[release](https://milvus.io/docs/v2.1.x/release_notes.md) of Milvus 2.1\nis now live after six months of hard work by all of our Milvus community\ncontributors. This major iteration of the popular vector database\nemphasizes **performance** and **usability**, two most important\nkeywords of our focus. We added support for strings, Kafka message\nqueue, and embedded Milvus, as well as a number of improvements in\nperformance, scalability, security, and observability. Milvus 2.1 is an\nexciting update that will bridge the \"last mile\" from the algorithm\nengineer's laptop to production-level vector similarity search\nservices.\n\n# Performance - More than a 3.2x boost\n\n## 5ms-level latency\n\nMilvus already supports approximate nearest neighbor (ANN) search, a\nsubstantial leap from the traditional KNN method. However, problems of\nthroughput and latency continue to challenge users who need to deal with\nbillion-scale vector data retrieval scenarios.\n\nIn Milvus 2.1, there is a new routing protocol that no longer relies on\nmessage queues in the retrieval link, significantly reducing retrieval\nlatency for small datasets. Our test results show that Milvus now brings\nits latency level down to 5ms, which meets the requirements of critical\nonline links such as similarity search and recommendation.\n\n## Concurrency control\n\nMilvus 2.1 fine-tunes its concurrency model by introducing a new cost\nevaluation model and concurrency scheduler. It now provides concurrency\ncontrol, which ensures that there will not be a large number of\nconcurrent requests competing for CPU and cache resources, nor will the\nCPU be under-utilized because there are not enough requests. The new,\nintelligent scheduler layer in Milvus 2.1 also merges small-nq queries\nthat have consistent request parameters, delivering an amazing 3.2x\nperformance boost in scenarios with small-nq and high query concurrency.\n\n## In-memory replicas\n\nMilvus 2.1 brings in-memory replicas that improve scalability and\navailability for small datasets. Similar to the read-only replicas in\ntraditional databases, in-memory replicas can scale horizontally by\nadding machines when the read QPS is high. In vector retrieval for small\ndatasets, a recommendation system often needs to provide QPS that\nexceeds the performance limit of a single machine. Now in these\nscenarios, the system's throughput can be significantly improved by\nloading multiple replicas in the memory. In the future, we will also\nintroduce a hedged read mechanism based on in-memory replicas, which\nwill quickly request other functional copies in case the system needs to\nrecover from failures and makes full use of memory redundancy to improve\nthe system's overall availability.\n\n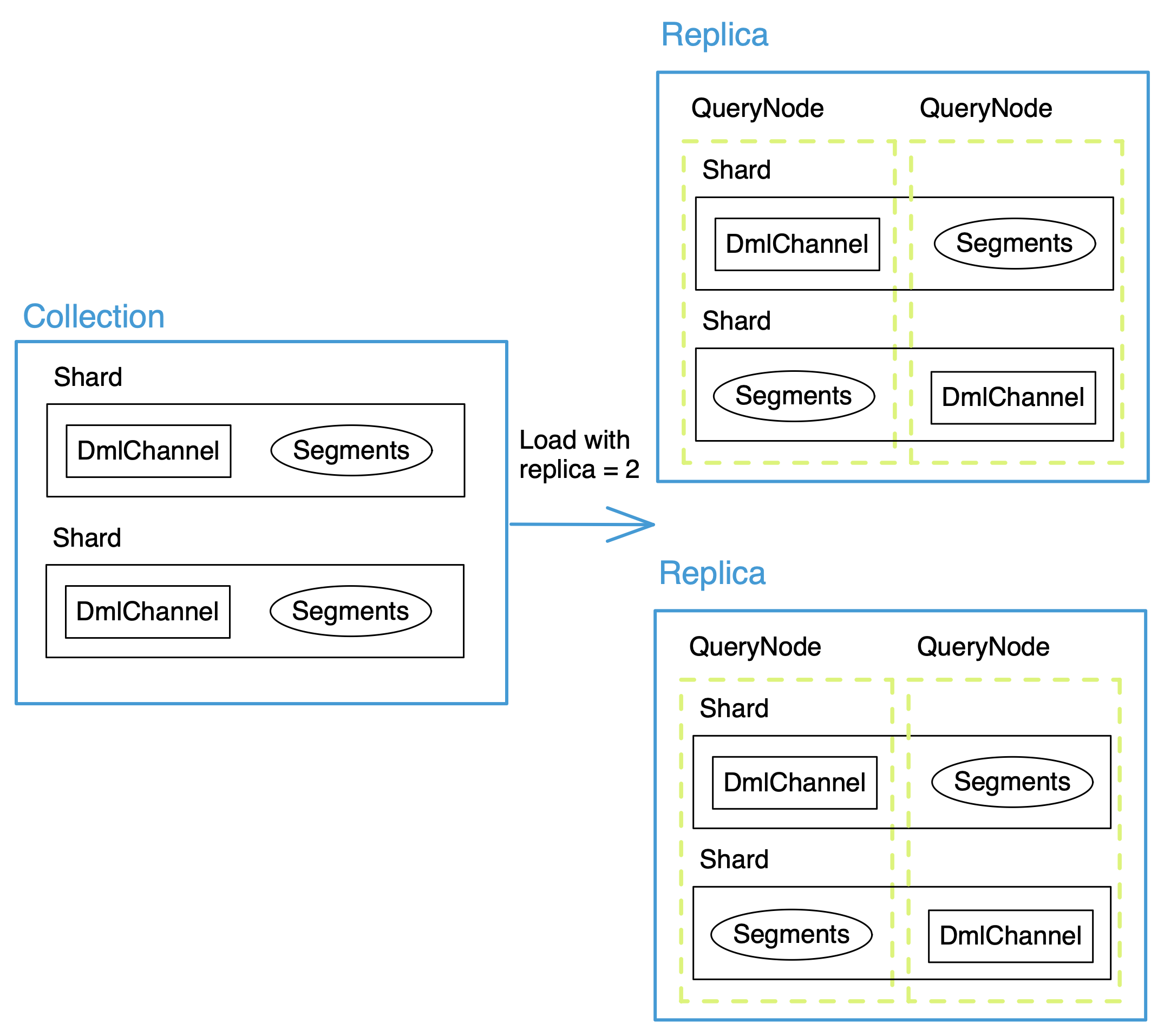\n\n## Faster data loading\n\nThe last performance boost comes from data loading. Milvus 2.1 now\ncompresses [binary\nlogs](https://milvus.io/docs/v2.1.x/glossary.md#Log-snapshot) with\nZstandard (zstd), which significantly reduces data size in the object\nand message stores as well as network overhead during data loading. In\naddition, goroutine pools are now introduced so that Milvus can load\nsegments concurrently with memory footprints controlled and minimize the\ntime required to recover from failures and to load data.\n\nThe complete benchmark results of Milvus 2.1 will be released on our\nwebsite soon. Stay tuned.\n\n## String and scalar index support\n\nWith 2.1, Milvus now supports variable-length string (VARCHAR) as a\nscalar data type. VARCHAR can be used as the primary key that can be\nreturned as output, and can also act as attribute filters. [Attribute\nfiltering](https://milvus.io/docs/v2.1.x/hybridsearch.md) is one of the\nmost popular functions Milvus users need. If you often find yourself\nwanting to \"find products most similar to a user in a $200 - $300\nprice range\", or \"find articles that have the keyword 'vector\ndatabase' and are related to cloud-native topics\", you'll love Milvus\n2.1.\n\nMilvus 2.1 also supports scalar inverted index to improve filtering\nspeed based on\n[succinct](https://www.cs.le.ac.uk/people/ond1/XMLcomp/confersWEA06_LOUDS.pdf)\n[MARISA-Tries](https://github.com/s-yata/marisa-trie) as the data\nstructure. All the data can now be loaded into memory with a very low\nfootprint, which allows much quicker comparison, filtering and prefix\nmatching on strings. Our test results show that the memory requirement\nof MARISA-trie is only 10% of that of Python dictionaries to load all\nthe data into memory and provide query capabilities.\n\n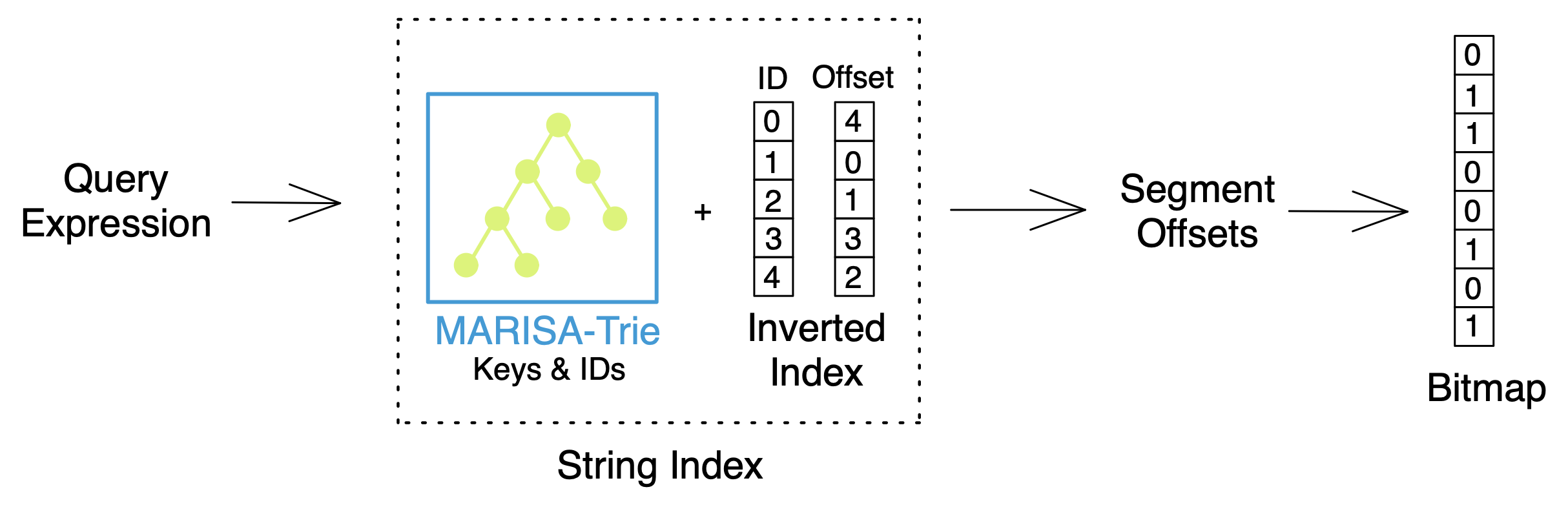\n\nIn the future, Milvus will continue focusing on scalar query-related\ndevelopments, support more scalar index types and query operators, and\nprovide disk-based scalar query capabilities, all as part of an ongoing\neffort to reduce storage and usage cost of scalar data.\n\n# Usability improvements\n\n## Kafka support\n\nOur community has long been requesting support for [Apache\nKafka](https://kafka.apache.org) as the [message\nstorage](https://milvus.io/docs/v2.1.x/deploy_pulsar.md) in Milvus.\nMilvus 2.1 now offers you the option to use\n[Pulsar](https://pulsar.apache.org) or Kafka as the message storage\nbased on user configurations, thanks to the abstraction and\nencapsulation design of Milvus and the Go Kafka SDK contributed by\nConfluent.\n\n## Production-ready Java SDK\n\nWith Milvus 2.1, our [Java\nSDK](https://github.com/milvus-io/milvus-sdk-java) is now officially\nreleased. The Java SDK has the exact same capabilities as the Python\nSDK, with even better concurrency performance. In the next step, our\ncommunity contributors will gradually improve documentation and use\ncases for the Java SDK, and help push Go and RESTful SDKs into the\nproduction-ready stage, too.\n\n## Observability and maintainability\n\nMilvus 2.1 adds important monitoring\n[metrics](https://milvus.io/docs/v2.1.x/metrics_dashboard.md) such as\nvector insertion counts, search latency/throughput, node memory\noverhead, and CPU overhead. Plus, the new version also significantly\noptimizes log keeping by adjusting log levels and reducing useless log\nprinting.\n\n## Embedded Milvus\n\nMilvus has greatly simplified the deployment of large-scale massive\nvector data retrieval services, but for scientists who want to validate\nalgorithms on a smaller scale, Docker or K8s is still too unnecessarily\ncomplicated. With the introduction of [embedded\nMilvus](https://github.com/milvus-io/embd-milvus), you can now install\nMilvus using pip, just like with Pyrocksb and Pysqlite. Embedded Milvus\nsupports all the functionalities of both the cluster and standalone\nversions, allowing you to easily switch from your laptop to a\ndistributed production environment without changing a single line of\ncode. Algorithm engineers will have a much better experience when\nbuilding a prototype with Milvus.\n\n# Try out-of-the-box vector search now\n\nMoreover, Milvus 2.1 also has some great improvements in stability and\nscalability, and we look forward to your use and feedbacks.\n\n## What's next\n\n- See the detailed [Release\n Notes](https://milvus.io/docs/v2.1.x/release_notes.md) for all the\n changes in Milvus 2.1\n- [Install](https://milvus.io/docs/v2.1.x/install_standalone-docker.md)\n Milvus 2.1 and try out the new features\n- Join our [Slack community](https://slack.milvus.io/) and discuss the\n new features with thousands of Milvus users around the world\n- Follow us on [Twitter](https://twitter.com/milvusio) and\n [LinkedIn](https://www.linkedin.com/company/the-milvus-project) to\n get updates once our blogs on specific new features are out\n\n\u003e Edited by [Songxian Jiang](https://github.com/songxianj)\n","title":"What's new in Milvus 2.1 - Towards simplicity and speed","metaData":{}},{"id":"intelligent-wardrobe-customization-system.md","author":"Yiyun Ni","desc":"Using similarity search technology to unlock the potential of unstructured data, even like wardrobes and its components!","tags":["Engineering"],"canonicalUrl":"https://milvus.io/blog/intelligent-wardrobe-customization-system.md","date":"2022-07-08T00:00:00.000Z","cover":"https://assets.zilliz.com/Frame_1282_edc1fb7d99.png","href":"/blog/intelligent-wardrobe-customization-system.md","content":"\n\n\nIf you are looking for a wardrobe to fit perfectly into your bedroom or fitting room, I bet most people will think of the made-to-measure ones. However, not everyone's budget can stretch that far. Then what about those ready-made ones? The problem with this type of wardrobe is that they are very likely to fall short of your expectation as they are not flexible enough to cater to your unique storage needs. Plus, when searching online, it is rather difficult to summarize the particular type of wardrobe you are looking for with keywords. Very likely, the keyword you type in the search box (eg. A wardrobe with a jewellery tray) might be very different from how it is defined in the search engine (eg. A wardrobe with [pullout tray with insert](https://www.ikea.com/us/en/p/komplement-pull-out-tray-with-insert-black-brown-s79249366/)). \n\nBut thanks to emerging technologies, there is a solution! IKEA, the furniture retail conglomerate, provides a popular design tool [PAX wardrobe](https://www.ikea.com/us/en/rooms/bedroom/how-to/how-to-design-your-perfect-pax-wardrobe-pub8b76dda0) that allows users to choose from a number of ready-made wardrobes and customize the color, size, and interior design of the wardrobes. Whether you need hanging space, multiple shelves or internal drawers, this intelligent wardrobe customization system can always cater to your needs. \n\nTo find or build your ideal wardrobe using this smart wardrobe design system, you need to:\n1. Specify the basic requirements - the shape (normal, L-shaped, or U-shaped), length and depth of the wardrobe.\n2. Specify your storage need and the interior organization of the wardrobe (eg. Hanging space, a pullout pants rack, etc is needed).\n3. Add or remove parts of the wardrobe like drawers or shelves.\n\nThen your design is completed. Simple and easy!\n\n\n\n\n\n\nA very critical component that makes such a wardrobe design system possible is the [vector database](https://zilliz.com/learn/what-is-vector-database). Therefore, this article aims to introduce the workflow and similarity search solutions used to build an intelligent wardrobe customization system powered by vector similarity search.\n\nJump to:\n- [System overview](#System-overview)\n- [Data flow](#Data-flow)\n- [System demo](#System-demo)\n\n\n## System Overview\nIn order to deliver such a smart wardrobe customization tool, we need to first define the business logic and understand item attributes and user journey. Wardrobes along with its components like drawers, trays, racks, are all unstructured data. Therefore, the second step is to leverage AI algorithms and rules, prior knowledge, item description, and more, to convert those unstructured data into a type of data that can be understood by computers - vectors!\n\n\n\n\nWith the generated vectors, we need powerful vector databases and search engines to process them.\n\n\n\n\nThe customization tool leverages some of the most popular search engines and databases: Elasticsearch, [Milvus](https://milvus.io/), and PostgreSQL.\n\n### Why Milvus?\nA wardrobe component contains highly complex information, such as color, shape, and interior organization, etc. However, the traditional way of keeping wardrobe data in a relational database is far from enough. A popular way is to use embedding techniques to convert wardrobes into vectors. Therefore, we need to look for a new type of database specifically designed for vector storage and similarity search. After probing into several popular solutions, the [Milvus](https://github.com/milvus-io/milvus) vector database is selected for its excellent performance, stability, compatibility, and ease-of-use. The chart below is a comparison of several popular vector search solutions.\n\n\n\n\n### System workflow\n\n\n\n\nElasticsearch is used for a coarse filtering by the wardrobe size, color, etc. Then the filtered results go through Milvus the vector database for a similarity search and the results are ranked based on their distance/similarity to the query vector. Finally, the results are consolidated and further refined based on business insights.\n\n## Data flow \nThe wardrobe customization system is very similar to traditional search engines and recommender systems. It contains three parts: \n- Offline data preparation including data definition and generation.\n- Online services including recall and ranking.\n- Data post-processing based on business logic.\n\n\n\n\n### Offline data flow \n1. Define data using business insight.\n2. Use prior knowledge to define how to combine different components and form them into a wardrobe.\n3. Recognize feature labels of the wardrobes and encode the features into Elasticsearch data in `.json` file.\n4. Prepare recall data by encoding unstructured data into vectors.\n5. Use Milvus the vector database to rank the recalled results obtained in the previous step.\n\n\n\n\n### Online data flow\n1. Receive query request from users and collect user profiles.\n2. Understand user query by identifying their requirements for the wardrobe.\n3. Coarse search using Elasticsearch.\n4. Score and rank the results obtained from coarse search based on the calculation of vector similarity in Milvus.\n5. Post-process and organize the results on the back-end platform to generate the final results.\n\n\n\n\n### Data post-processing\nThe business logic varies among each company. You can add a final touch to the results by applying your company's business logic.\n\n## System demo\nNow let's see how the system we build actually works.\n\nThe user interface (UI) displays the possibility of different combinations of wardrobe components. \n\nEach component is labelled by its feature (size, color, etc.) and stored in Elasticsearch (ES). When storing the labels in ES, there are four main data fields to be filled out: ID, tags, storage path, and other support fields. ES and the labelled data are used for granular recall and attribute filtering.\n\n\n\nThen different AI algorithms are used to encode a wardrobe into a set of vectors. The vector sets are stored in Milvus for similarity search and ranking. This step returns more refined and accurate results.\n\n\n\nElasticsearch, Milvus, and other system components altogether form the customization design platform as a whole. During recall, the domain-specific language (DSL) in Elasticsearch and Milvus is as follows.\n\n\n\n\n## Looking for more resources?\nLearn how the Milvus vector database can power more AI applications:\n- [How Short Video Platform Likee Removes Duplicate Videos with Milvus](https://milvus.io/blog/2022-06-23-How-Short-video-Platform-Likee-Removes-Duplicate-Videos-with-Milvus.md)\n- [Zhentu - The Photo Fraud Detector Based on Milvus](https://milvus.io/blog/2022-06-20-Zhentu-the-Photo-Fraud-Detector-Based-on-Milvus.md)\n","title":"Building an Intelligent Wardrobe Customization System Powered by Milvus Vector Database","metaData":{}},{"id":"2022-06-23-How-Short-video-Platform-Likee-Removes-Duplicate-Videos-with-Milvus.md","author":"Xinyang Guo, Baoyu Han","desc":"Learn how Likee uses Milvus to identify duplicate videos in milliseconds.","tags":["Scenarios"],"canonicalUrl":"https://milvus.io/blog/2022-06-23-How-Short-video-Platform-Likee-Removes-Duplicate-Videos-with-Milvus.md","date":"2022-06-23T00:00:00.000Z","cover":"https://assets.zilliz.com/How_Short_video_Platform_Likee_Removes_Duplicate_Videos_with_Milvus_07bd75ec82.png","href":"/blog/2022-06-23-How-Short-video-Platform-Likee-Removes-Duplicate-Videos-with-Milvus.md","content":"\n\n\n\u003e This article is written by Xinyang Guo and Baoyu Han, engineers at BIGO, and translated by [Rosie Zhang](https://www.linkedin.cn/incareer/in/rosie-zhang-694528149).\n\n[BIGO Technology](https://www.bigo.sg/) (BIGO) is one of the fastest-growing Singapore technology companies. Powered by artificial intelligence technology, BIGO's video-based products and services have gained immense popularity worldwide, with over 400 million users in more than 150 countries. These include [Bigo Live](https://www.bigo.tv/bigo_intro/en.html?hk=true) (live streaming) and [Likee](https://likee.video/) (short-form video). \n\nLikee is a global short video creation platform where users can share their moments, express themselves, and connect with the world. To increase user experience and recommend higher-quality content to users, Likee needs to weed out duplicate videos from a tremendous amount of videos generated by users every day, which poses no straightforward task. \n\nThis blog presents how BIGO uses [Milvus](https://milvus.io), an open-source vector database, to effectively remove duplicate videos.\n\n**Jump to:**\n- [Overview](#Overview)\n- [Video deduplication workflow](#Video-deduplication-workflow)\n- [System Architecture](#System-architecture)\n- [Using Milvus to power similarity search](#Using-Milvus-vector-database-to-power-similarity-search)\n\n# Overview\nMilvus is an open-source vector database featuring ultra-fast vector search. Powered by Milvus, Likee is able to complete a search within 200ms while ensuring a high recall rate. Meanwhile, by [scaling Milvus horizontally](https://milvus.io/docs/v2.0.x/scaleout.md#Scale-a-Milvus-Cluster), Likee successfully increases the throughput of vector queries, further improving its efficiency.\n\n# Video deduplication workflow\nHow does Likee identify duplicate videos? Every time a query video is input into Likee's system, it will be cut into 15-20 frames and each frame will be converted to a feature vector. Then Likee searches in a database of 700 million vectors to find the top K most similar vectors. Each one of the top K vectors corresponds to a video in the database. Likee further conducts refined searches to get the final results and determine the videos to be removed.\n\n# System architecture\nLet's take a closer look at how Likee's video de-duplication system works using Milvus. As is shown in the diagram below, new videos uploaded to Likee will be written to Kafka, a data storage system, in real-time and consumed by Kafka consumers. The feature vectors of these videos are extracted through deep learning models, where unstructured data (video) is converted into feature vectors. These feature vectors will be packaged by the system and sent to the similarity auditor.\n\n\n\nThe feature vectors extracted will be indexed by Milvus and stored in Ceph, before being [loaded by the Milvus query node](https://milvus.io/blog/deep-dive-5-real-time-query.md) for further search. The corresponding video IDs of these feature vectors will also be stored simultaneously in TiDB or Pika according to the actual needs.\n\n### Using Milvus vector database to power similarity search\nWhen searching for similar vectors, billions of existing data, along with large amounts of new data generated every day, pose great challenges to the functionality of the vector search engine. After thorough analysis, Likee eventually chose Milvus, a distributed vector search engine of high performance and high recall rate, to conduct vector similarity search.\n\nAs is shown in the diagram below, the procedure of a similarity search goes as follows:\n\n1. First, Milvus performs a batch search to recall the top 100 similar vectors for each of the multiple feature vectors extracted from a new video. Each similar vector is bound to its corresponding video ID. \n\n2. Second, by comparing the video IDs, Milvus removes the duplicate videos and retrieves the feature vectors of the remaining videos from TiDB or Pika. \n\n3. Finally, Milvus calculates and scores the similarity between each set of the feature vectors retrieved and the feature vectors of the query video. The video ID with the highest score is returned as the result. Thus the video similarity search is concluded.\n\n\n\nAs a high-performance vector search engine, Milvus has done an extraordinary job in Likee's video de-duplication system, greatly fueling the growth of BIGO's short-video business. In terms of video businesses, there are many other scenarios where Milvus can be applied to, such as illegal content blocking or personalized video recommendation. Both BIGO and Milvus are looking forward to future cooperation in more areas.","title":"How Short-video Platform Likee Removes Duplicate Videos with Milvus","metaData":{}},{"id":"scalable-and-blazing-fast-similarity-search-with-milvus-vector-database.md","author":"Dipanjan Sarkar","desc":"Store, index, manage and search trillions of document vectors in milliseconds!","tags":["Engineering"],"canonicalUrl":"https://milvus.io/blog/scalable_and_blazing_fast_similarity_search_with_milvus_vector_database.md","date":"2022-06-21T00:00:00.000Z","cover":"https://assets.zilliz.com/69eba74e_4a9a_4c38_a2d9_2cde283e8a1d_e265515238.png","href":"/blog/scalable-and-blazing-fast-similarity-search-with-milvus-vector-database.md","content":"\n\n\n## Introduction\n\nIn this article, we will cover some interesting aspects relevant to vector databases and similarity search at scale. In today’s rapidly evolving world, we see new technology, new businesses, new data sources and consequently we will need to keep using new ways to store, manage and leverage this data for insights. Structured, tabular data has been stored in relational databases for decades, and Business Intelligence thrives on analyzing and extracting insights from such data. However, considering the current data landscape, “over 80–90% of data is unstructured information like text, video, audio, web server logs, social media, and more”. Organizations have been leveraging the power of machine learning and deep learning to try and extract insights from such data as traditional query-based methods may not be enough or even possible. There is a huge, untapped potential to extract valuable insights from such data and we are only getting started!\n\n\u003e “Since most of the world’s data is unstructured, an ability to analyze and act on it presents a big opportunity.” — Mikey Shulman, Head of ML, Kensho\n\nUnstructured data, as the name suggests, does not have an implicit structure, like a table of rows and columns (hence called tabular or structured data). Unlike structured data, there is no easy way to store the contents of unstructured data within a relational database. There are three main challenges with leveraging unstructured data for insights:\n\n- **Storage:** Regular relational databases are good for holding structured data. While you can use NoSQL databases to store such data, it becomes an additional overhead to process such data to extract the right representations to power AI applications at scale\n- **Representation:** Computers don’t understand text or images like we do. They only understand numbers and we need to covert unstructed data into some useful numeric representation, typically vectors or embeddings.\n- **Querying:** You can’t query unstructured data directly based on definite conditional statements like SQL for structured data. Imagine, a simple example of you trying to search for similar shoes given a photo of your favorite pair of shoes! You can’t use raw pixel values for search, neither can you represent structured features like shoe shape, size, style, color and more. Now imagine having to do this for millions of shoes!\n\nHence, in order for computers to understand, process and represent unstructured data, we typically convert them into dense vectors, often called embeddings.\n\n\n\nThere exist a variety of methodologies especially leveraging deep learning, including convolutional neural networks (CNNs) for visual data like images and Transformers for text data which can be used to transform such unstructured data into embeddings. [Zilliz](https://zilliz.com/) has [an excellent article covering different embedding techiques](https://zilliz.com/learn/embedding-generation)!\n\nNow storing these embedding vectors is not enough. One also needs to be able to query and find out similar vectors. Why do you ask? A majority of real-world applications are powered by vector similarity search for AI based solutions. This includes visual (image) search in Google, recommendations systems in Netflix or Amazon, text search engines in Google, multi-modal search, data de-duplication and many more!\n\nStoring, managing and querying vectors at scale is not a simple task. You need specialized tools for this and vector databases are the most effective tool for the job! In this article we will cover the following aspects:\n\n- [Vectors and Vector Similarity Search](#Vectors-and-Vector-Similarity-Search)\n- [What is a Vector Database?](#What-is-a-Vector-Database)\n- [Milvus — The World’s Most Advanced Vector Database](#Milvus—The-World-s-Most-Advanced-Vector-Database)\n- [Performing visual image search with Milvus — A use-case blueprint](#Performing-visual-image-search-with-Milvus—A-use-case-blueprint)\n\nLet’s get started!\n\n## Vectors and Vector Similarity Search\n\nEarlier, we established the necessity of representing unstructured data like images and text as vectors, since computers can only understand numbers. We typically leverage AI models, to be more specific deep learning models to convert unstructured data into numeric vectors which can be read in by machines. Typically these vectors are basically a list of floating point numbers which collectively represents the underlying item (image, text etc.).\n\n### Understanding Vectors\n\nConsidering the field of natural language processing (NLP) we have many word embedding models like [Word2Vec, GloVe and FastText](https://towardsdatascience.com/understanding-feature-engineering-part-4-deep-learning-methods-for-text-data-96c44370bbfa) which can help represent words as numeric vectors. With advancements over time, we have seen the rise of [Transformer](https://arxiv.org/abs/1706.03762) models like [BERT](https://jalammar.github.io/illustrated-bert/) which can be leveraged to learn contextual embedding vectors and better representations for entire sentences and paragraphs.\n\nSimilarly for the field of computer vision we have models like [Convolutional Neural Networks (CNNs)](https://proceedings.neurips.cc/paper/2012/file/c399862d3b9d6b76c8436e924a68c45b-Paper.pdf) which can help in learning representations from visual data such as images and videos. With the rise of Transformers, we also have [Vision Transformers](https://arxiv.org/abs/2010.11929) which can perform better than regular CNNs.\n\n\n\nThe advantage with such vectors is that we can leverage them for solving real-world problems such as visual search, where you typically upload a photo and get search results including visually similar images. Google has this as a very popular feature in their search engine as depicted in the following example.\n\n\n\nSuch applications are powered with data vectors and vector similarity search. If you consider two points in an X-Y cartesian coordinate space. The distance between two points can be computed as a simple euclidean distance depicted by the following equation.\n\n\n\nNow imagine each data point is a vector having D-dimensions, you could still use euclidean distance or even other distance metrics like hamming or cosine distance to find out how close the two data points are to each other. This can help build a notion of closeness or similarity which could be used as a quantifiable metric to find similar items given a reference item using their vectors.\n\n### Understanding Vector Similarity Search\n\nVector similarity search, often known as nearest neighbor (NN) search, is basically the process of computing pairwise similarity (or distances) between a reference item (for which we want to find similar items) and a collection of existing items (typically in a database) and returning the top ‘k’ nearest neighbors which are the top ‘k’ most similar items. The key component to compute this similarity is the similarity metric which can be euclidean distance, inner product, cosine distance, hamming distance, etc. The smaller the distance, the more similar are the vectors.\n\nThe challenge with exact nearest neighbor (NN) search is scalability. You need to compute N-distances (assuming N existing items) everytime to get similar items. This can be super slow especially if you don’t store and index the data somewhere (like a vector database!). To speed up computation, we typically leverage approximate nearest neighbor search which is often called ANN search which ends up storing the vectors into an index. The index helps in storing these vectors in an intelligent way to enable quick retrieval of ‘approximately’ similar neighbors for a reference query item. Typical ANN indexing methodologies include:\n\n- **Vector Transformations:** This includes adding additional transformations to the vectors like dimension reduction (e.g PCA \\ t-SNE), rotation and so on\n- **Vector Encoding:** This includes applying techniques based on data structures like Locality Sensitive Hashing (LSH), Quantization, Trees etc. which can help in faster retrieval of similar items\n- **Non-Exhaustive Search Methods:** This is mostly used to prevent exhaustive search and includes methods like neighborhood graphs, inverted indices etc.\n\nThis establishes the case that to build any vector similarity search application, you need a database which can help you with efficient storing, indexing and querying (search) at scale. Enter vector databases!\n\n## What is a Vector Database?\n\nGiven that we now understand how vectors can be used to represent unstructured data and how vector search works, we can combine the two concepts together to build a vector database.\n\nVector databases are scalable data platforms to store, index and query across embedding vectors which are generated from unstructured data (images, text etc.) using deep learning models.\n\nHandling a massive numbers of vectors for similarity search (even with indices) can be super expensive. Despite this, the best and most advanced vector databases should allow you to insert, index and search across millions or billions of target vectors, in addition to specifying an indexing algorithm and similarity metric of your choice.\n\nVector databases mainly should satisfy the following key requirements considering a robust database management system to be used in the enterprise:\n\n1. **Scalable:** Vector databases should be able to index and run approximate nearest neighbor search for billions of embedding vectors\n2. **Reliable:** Vector databases should be able to handle internal faults without data loss and with minimal operational impact, i.e be fault-tolerant\n3. **Fast:** Query and write speeds are important for vector databases. For platforms such as Snapchat and Instagram, which can have hundreds or thousands of new images uploaded per second, speed becomes an incredibly important factor.\n\nVector databases don’t just store data vectors. They are also responsible for using efficient data structures to index these vectors for fast retrieval and supporting CRUD (create, read, update and delete) operations. Vector databases should also ideally support attribute filtering which is filtering based on metadata fields which are usually scalar fields. A simple example would be retrieving similar shoes based on the image vectors for a specific brand. Here brand would be the attribute based on which filtering would be done.\n\n\n\nThe figure above showcases how [Milvus](https://milvus.io/), the vector database we will talk about shortly, uses attribute filtering. [Milvus](https://milvus.io/) introduces the concept of a bitmask to the filtering mechanism to keep similar vectors with a bitmask of 1 based on satisfying specific attribute filters. More details on this [here](https://zilliz.com/learn/attribute-filtering).\n\n## Milvus — The World’s Most Advanced Vector Database\n\n[Milvus](https://milvus.io/) is an open-source vector database management platform built specifically for massive-scale vector data and streamlining machine learning operations (MLOps).\n\n\n\n\n[Zilliz](https://zilliz.com/), is the organization behind building [Milvus](https://milvus.io/), the world’s most advanced vector database, to accelerate the development of next generation data fabric. Milvus is currently a graduation project at the [LF AI \u0026 Data Foundation](https://lfaidata.foundation/) and focuses on managing massive unstructured datasets for storage and search. The platform’s efficiency and reliability simplifies the process of deploying AI models and MLOps at scale. Milvus has broad applications spanning drug discovery, computer vision, recommendation systems, chatbots, and much more.\n\n### Key Features of Milvus\n\nMilvus is packed with useful features and capabilities, such as:\n\n- **Blazing search speeds on a trillion vector datasets:** Average latency of vector search and retrieval has been measured in milliseconds on a trillion vector datasets.\n- **Simplified unstructured data management:** Milvus has rich APIs designed for data science workflows.\n- **Reliable, always on vector database:** Milvus’ built-in replication and failover/failback features ensure data and applications can maintain business continuity always.\n- **Highly scalable and elastic:** Component-level scalability makes it possible to scale up and down on demand.\n- **Hybrid search:** In addition to vectors, Milvus supports data types such as Boolean, String, integers, floating-point numbers, and more. Milvus pairs scalar filtering with powerful vector similarity search (as seen in the shoe similarity example earlier).\n- **Unified Lambda structure:** Milvus combines stream and batch processing for data storage to balance timeliness and efficiency.\n- **[Time Travel](https://milvus.io/docs/v2.0.x/timetravel_ref.md):** Milvus maintains a timeline for all data insert and delete operations. It allows users to specify timestamps in a search to retrieve a data view at a specified point in time.\n- **Community supported \u0026 Industry recognized:** With over 1,000 enterprise users, 10.5K+ stars on [GitHub](https://github.com/milvus-io/milvus), and an active open-source community, you’re not alone when you use Milvus. As a graduate project under the [LF AI \u0026 Data Foundation](https://lfaidata.foundation/), Milvus has institutional support.\n\n### Existing Approaches to Vector Data Management and Search\n\nA common way to build an AI system powered by vector similarity search is to pair algorithms like Approximate Nearest Neighbor Search (ANNS) with open-source libraries such as:\n\n- **[Facebook AI Similarity Search (FAISS)](https://ai.facebook.com/tools/faiss/):** This framework enables efficient similarity search and clustering of dense vectors. It contains algorithms that search in sets of vectors of any size, up to ones that possibly do not fit in RAM. It supports indexing capabilities like inverted multi-index and product quantization\n- **[Spotify’s Annoy (Approximate Nearest Neighbors Oh Yeah)](https://github.com/spotify/annoy):** This framework uses [random projections](http://en.wikipedia.org/wiki/Locality-sensitive_hashing#Random_projection) and builds up a tree to enable ANNS at scale for dense vectors\n- **[Google’s ScaNN (Scalable Nearest Neighbors)](https://github.com/google-research/google-research/tree/master/scann):** This framework performs efficient vector similarity search at scale. Consists of implementations, which includes search space pruning and quantization for Maximum Inner Product Search (MIPS)\n\nWhile each of these libraries are useful in their own way, due to several limitations, these algorithm-library combinations are not equivalent to a full-fledged vector data management system like Milvus. We will discuss some of these limitations now.\n\n### Limitations of Existing Approaches\n\nExisting approaches used for managing vector data as discussed in the previous section has the following limitations:\n\n1. **Flexibility:** Existing systems typically store all data in main memory, hence they cannot be run in distributed mode across multiple machines easily and are not well-suited for handling massive datasets\n2. **Dynamic data handling:** Data is often assumed to be static once fed into existing systems, complicating processing for dynamic data and making near real-time search impossible\n3. **Advanced query processing:** Most tools do not support advanced query processing (e.g., attribute filtering, hybrid search and multi-vector queries), which is essential for building real-world similarity search engines supporting advanced filtering.\n4. **Heterogeneous computing optimizations:** Few platforms offer optimizations for heterogenous system architectures on both CPUs and GPUs (excluding FAISS), leading to efficiency losses.\n\n[Milvus](https://milvus.io/) attempts to overcome all of these limitations and we will discuss this in detail in the next section.\n\n### The Milvus Advantage —Understanding Knowhere\n\n[Milvus](https://milvus.io/) tries to tackle and successfully solve the limitations of existing systems build on top of inefficient vector data management and similarity search algorithms in the following ways:\n\n- It enhances flexibility by offering support for a variety of application interfaces (including SDKs in Python, Java, Go, C++ and RESTful APIs)\n- It supports multiple vector index types (e.g., quantization-based indexes and graph-based indexes), and advanced query processing\n- Milvus handles dynamic vector data using a log-structured merge-tree (LSM tree), keeping data insertions and deletions efficient and searches humming along in real time\n- Milvus also provides optimizations for heterogeneous computing architectures on modern CPUs and GPUs, allowing developers to adjust systems for specific scenarios, datasets, and application environments\n\nKnowhere, the vector execution engine of Milvus, is an operation interface for accessing services in the upper layers of the system and vector similarity search libraries like Faiss, Hnswlib, Annoy in the lower layers of the system. In addition, Knowhere is also in charge of heterogeneous computing. Knowhere controls on which hardware (eg. CPU or GPU) to execute index building and search requests. This is how Knowhere gets its name — knowing where to execute the operations. More types of hardware including DPU and TPU will be supported in future releases.\n\n\n\nComputation in Milvus mainly involves vector and scalar operations. Knowhere only handles the operations on vectors in Milvus. The figure above illustrates the Knowhere architecture in Milvus. The bottom-most layer is the system hardware. The third-party index libraries are on top of the hardware. Then Knowhere interacts with the index node and query node on the top via CGO. Knowhere not only further extends the functions of Faiss but also optimizes the performance and has several advantages including support for BitsetView, support for more similarity metrics, support for AVX512 instruction set, automatic SIMD-instruction selection and other performance optimizations. Details can be found [here](https://milvus.io/blog/deep-dive-8-knowhere.md).\n\n### Milvus Architecture\n\nThe following figure showcases the overall architecture of the Milvus platform. Milvus separates data flow from control flow, and is divided into four layers that are independent in terms of scalability and disaster recovery.\n\n\n\n- **Access layer:** The access layer is composed of a group of stateless proxies and serves as the front layer of the system and endpoint to users.\n- **Coordinator service:** The coordinator service is responsible for cluster topology node management, load balancing, timestamp generation, data declaration, and data management\n- **Worker nodes:** The worker, or execution, node executes instructions issued by the coordinator service and the data manipulation language (DML) commands initiated by the proxy. A worker node in Milvus is similar to a data node in [Hadoop](https://hadoop.apache.org/), or a region server in HBase\n- **Storage:** This is the cornerstone of Milvus, responsible for data persistence. The storage layer is comprised of **meta store**, **log broker** and **object storage**\n\nDo check out more details about the architecture [here](https://milvus.io/docs/v2.0.x/four_layers.md)!\n\n## Performing visual image search with Milvus — A use-case blueprint\n\nOpen-source vector databases like Milvus makes it possible for any business to create their own visual image search system with a minimum number of steps. Developers can use pre-trained AI models to convert their own image datasets into vectors, and then leverage Milvus to enable searching for similar products by image. Let’s look at the following blueprint of how to design and build such a system.\n\n\n\nIn this workflow we can use an open-source framework like [towhee](https://github.com/towhee-io/towhee) to leverage a pre-trained model like ResNet-50 and extract vectors from images, store and index these vectors with ease in Milvus and also store a mapping of image IDs to the actual pictures in a MySQL database. Once the data is indexed we can upload any new image with ease and perform image search at scale using Milvus. The following figure shows a sample visual image search.\n\n\n\nDo check out the detailed [tutorial](https://github.com/milvus-io/bootcamp/tree/master/solutions/reverse_image_search/quick_deploy) which has been open-sourced on GitHub thanks to Milvus.\n\n## Conclusion\n\nWe’ve covered a fair amount of ground in this article. We started with challenges in representing unstrucutured data, leveraging vectors and vector similarity search at scale with Milvus, an open-source vector database. We discussed about details on how Milvus is structured and the key components powering it and a blueprint of how to solve a real-world problem, visual image search with Milvus. Do give it a try and start solving your own real-world problems with [Milvus](https://milvus.io/)!\n\nLiked this article? Do [reach out to me](https://www.linkedin.com/in/dipanzan/) to discuss more on it or give feedback!\n\n## About the author\n\nDipanjan (DJ) Sarkar is a Data Science Lead, Google Developer Expert — Machine Learning, Author, Consultant and AI Advisor. Connect: http://bit.ly/djs_linkedin\n\n","title":"Scalable and Blazing Fast Similarity Search with Milvus Vector Database","metaData":{}},{"id":"2022-06-20-Zhentu-the-Photo-Fraud-Detector-Based-on-Milvus.md","author":"Yan Shi, Minwei Tang","desc":"How is Zhentu's detection system built with Milvus as its vector search engine?","tags":["Scenarios"],"canonicalUrl":"https://milvus.io/blog/2022-06-20-Zhentu-the-Photo-Fraud-Detector-Based-on-Milvus.md","date":"2022-06-20T00:00:00.000Z","cover":"https://assets.zilliz.com/zhentu_0ae11c98ee.png","href":"/blog/2022-06-20-Zhentu-the-Photo-Fraud-Detector-Based-on-Milvus.md","content":"\n\n\n\u003e This article is written by Yan Shi and Minwei Tang, senior algorithm engineers at BestPay, and translated by [Rosie Zhang](https://www.linkedin.cn/incareer/in/rosie-zhang-694528149).\n\nIn recent years, as e-commerce and online transactions become commonplace throughout the world, e-commerce fraud also flourished. By using computer-generated photos instead of real ones to pass identity verification on online business platforms, fraudsters create massive fake accounts and cash in on businesses' special offers (e.g. membership gifts, coupons, tokens), which brings irretrievable losses to both consumers and businesses.\n\nTraditional risk control methods are no longer effective in the face of a substantial amount of data. To solve the problem, [BestPay](https://www.bestpay.com.cn) created a photo fraud detector, namely Zhentu (meaning detecting images in Chinese), based on deep learning (DL) and digital image processing (DIP) technologies. Zhentu is applicable to various scenarios involving image recognition, with one important offshoot being the identification of fake business licenses. If the business license photo submitted by a user is very similar to another photo already existing in a platform's photo library, it is likely that the user has stolen the photo somewhere or has forged a license for fraudulent purposes.\n\nTraditional algorithms for measuring image similarity, such as [PSNR](https://en.wikipedia.org/wiki/Peak_signal-to-noise_ratio) and ORB, are slow and inaccurate, only applicable to offline tasks. Deep learning, on the other hand, is capable of processing large-scale image data in real-time and is the ultimate method for matching similar images. With the joint efforts of BestPay’s R\u0026D team and [the Milvus community](https://milvus.io/), a photo fraud detection system is developed as part of Zhentu. It functions by converting massive amounts of image data into feature vectors through deep learning models and inserting them into [Milvus](https://milvus.io/), a vector search engine. With Milvus, the detection system is able to index trillions of vectors and efficiently retrieve similar photos among tens of millions of images.\n\n**Jump to:**\n- [An overview of Zhentu](#an-overview-of-zhentu)\n- [System structure](#system-structure)\n- [**Deployment**](#deployment)\n- [**Real-world performance**](#real-world-performance)\n- [**Reference**](#reference)\n- [**About BestPay**](#about-bestpay)\n\n## An overview of Zhentu\n\nZhentu is BestPay’s self-designed multimedia visual risk control product deeply integrated with machine learning (ML) and neural network image recognition technologies. Its built-in algorithm can accurately identify fraudsters during user authentication and respond at the millisecond level. With its industry-leading technology and innovative solution, Zhentu has won five patents and two software copyrights. It is now being used in a number of banks and financial institutions to help identify potential risks in advance.\n\n## System structure\n\nBestPay currently has over 10 million business license photos, and the actual volume is still growing exponentially as the business grows. In order to quickly retrieve similar photos from such a large database, Zhentu has chosen Milvus as the feature vector similarity calculation engine. The general structure of the photo fraud detection system is shown in the diagram below.\n\n\n\nThe procedure can be divided into four steps:\n\n1. Image pre-processing. Pre-processing, including noise reduction, noise removal, and contrast enhancement, ensures both the integrity of the original information and the removal of useless information from the image signal.\n\n2. Feature vector extraction. A specially trained deep learning model is used to extract the feature vectors of the image. Converting images into vectors for further similarity search is a routine operation.\n\n3. Normalization. Normalizing the extracted feature vectors helps to improve the efficiency of the subsequent processing.\n\n4. Vector search with Milvus. Inserting the normalized feature vectors into Milvus database for vector similarity search.\n\n## **Deployment**\n\nHere is a brief description of how Zhentu's photo fraud detection system is deployed.\n\n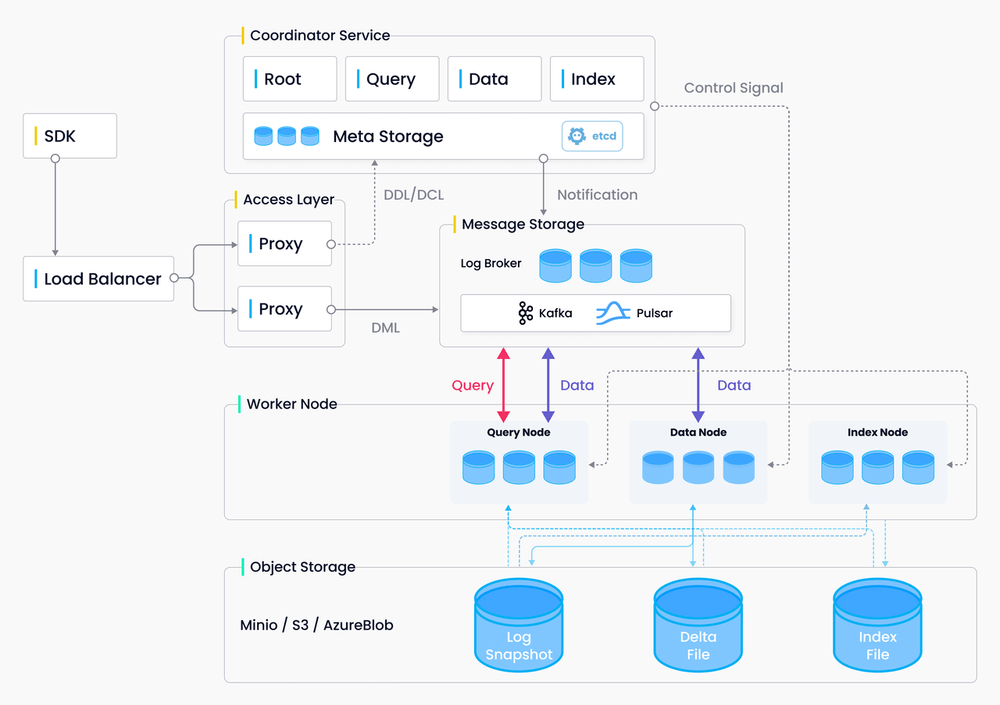\n\nWe deployed our [Milvus cluster on Kubernetes](https://milvus.io/docs/v2.0.x/install_cluster-helm.md) to ensure high availability and real-time synchronization of cloud services. The general steps are as follows:\n\n1. View available resources. Run the command `kubectl describe nodes` to see the resources that the Kubernetes cluster can allocate to the created cases.\n\n2. Allocate resources. Run the command `kubect`` -- apply xxx.yaml` to allocate memory and CPU resources for Milvus cluster components using Helm.\n\n3. Apply the new configuration. Run the command `helm upgrade my-release milvus/milvus --reuse-values -fresources.yaml`.\n\n4. Apply the new configuration to the Milvus cluster. The cluster deployed in this way not only allows us to adjust system capacity according to different business needs, but also better meets the high-performance requirements for massive vector data retrieval.\n\nYou can [configure Milvus](https://milvus.io/docs/v2.0.x/configure-docker.md) to optimize search performance for different types of data from different business scenarios, as shown in the following two examples.\n\nIn [building the vector index](https://milvus.io/docs/v2.0.x/build_index.md), we parameterize the index according to the actual scenario of the system as follows:\n\n```Python\nindex = {\"index_type\": \"IVF_PQ\", \"params\": {\"nlist\": 2048}, \"metric_type\": \"IP\"}\n```\n\n[IVF_PQ](https://milvus.io/docs/v2.0.x/index.md#IVF_PQ) performs IVF index clustering before quantizing the product of vectors. It features high-speed disk query and very low memory consumption, which meets the needs of the real-world application of Zhentu.\n\nBesides, we set the optimal search parameters as follows:\n\n```Python\nsearch_params = {\"metric_type\": \"IP\", \"params\": {\"nprobe\": 32}}\n```\n\nAs the vectors are already normalized before input into Milvus, the inner product (IP) is chosen to calculate the distance between two vectors. Experiments have proved that the recall rate is raised by about 15% using IP than using the Euclidean distance (L2). \n\nThe above examples show that we can test and set Milvus' parameters according to different business scenarios and performance requirements. \n\nIn addition, Milvus not only integrates different index libraries, but also supports different index types and similarity calculation methods. Milvus also provides official SDKs in multiple languages and rich APIs for insertion, querying, etc., allowing our front-end business groups to use the SDKs to call on the risk control center.\n\n## **Real-world performance**\n\nSo far, the photo fraud detection system has been running steadily, helping businesses to identify potential fraudsters. In 2021, it detected over 20,000 fake licenses throughout the year. In terms of query speed, a single vector query among tens of millions of vectors takes less than 1 second, and the average time of batch query is less than 0.08 seconds. Milvus' high-performance search meets businesses' needs for both accuracy and concurrency.\n\n## **Reference**\n\nAglave P, Kolkure V S. Implementation of High Performance Feature Extraction Method Using Oriented Fast and Rotated Brief Algorithm[J]. Int. J. Res. Eng. Technol, 2015, 4: 394-397. \n\n## **About BestPay**\n\nChina Telecom BestPay Co., Ltd is a wholly owned subsidiary of China Telecom. It operates the payment and finance businesses. BestPay is committed to using cutting-edge technologies such as big data, artificial intelligence and cloud computing to empower business innovation, providing intelligent products, risk control solutions and other services. Up to January 2016, the app called BestPay has attracted over 200 million users and become the third largest payment platform operator in China, closely following Alipay and WeChat Payment.\n","title":"Zhentu - the Photo Fraud Detector Based on Milvus","metaData":{}},{"id":"raft-or-not.md","author":"Xiaofan Luan","desc":"Why consensus-based replication algorithm is not the silver bullet for achieving data consistency in distributed databases?","tags":["Engineering"],"canonicalUrl":"https://milvus.io/blog/raft-or-not.md","date":"2022-05-16T00:00:00.000Z","cover":"https://assets.zilliz.com/Tech_Modify_5_e18025ffbc.png","href":"/blog/raft-or-not.md","content":"\n\n\n\u003e This article was written by [Xiaofan Luan](https://github.com/xiaofan-luan) and transcreated by [Angela Ni](https://www.linkedin.com/in/yiyun-n-2aa713163/).\n\nConsensus-based replication is a widely-adopted strategy in many cloud-native, distributed databases. However, it has certain shortcomings and is definitely not the silver bullet.\n\nThis post aims to first explain the concepts of replication, consistency, and consensus in a cloud-native and distributed database, then clarify why consensus-based algorithms like Paxos and Raft are not the silver bullet, and finally propose a [solution to consensus-based replication](#a-log-replication-strategy-for-cloud-native-and-distributed-database).\n\n**Jump to:**\n- [Understanding replication, consistency, and consensus](#Understanding-replication-consistency-and-consensus)\n- [Consensus-based replication](#Consensus-based-replication)\n- [A log replication strategy for cloud-native and distributed database](#A-log-replication-strategy-for-cloud-native-and-distributed-database)\n- [Summary](#Summary)\n\n\n## Understanding replication, consistency, and consensus\n\nBefore going deep into the pros and cons of Paxos and Raft, and proposing a best suited log replication strategy, we need to first demystify concepts of replication, consistency, and consensus.\n\nNote that this article mainly focuses on the synchronization of incremental data/log. Therefore, when talking about data/log replication, only replicating incremental data, not historical data, is referred to.\n\n### Replication\n\nReplication is the process of making multiple copies of data and storing them in different disks, processes, machines, clusters, etc, for the purpose of increasing data reliability and accelerating data queries. Since in replication, data are copied and stored at multiple locations, data are more reliable in the face of recovering from disk failures, physical machine failures, or cluster errors. In addition, multiple replicas of data can boost the performance of a distributed database by greatly speeding up queries.\n\nThere are various modes of replication, such as synchronous/asynchronous replication, replication with strong/eventual consistency, leader-follower/decentralized replication. The choice of replication mode has an effect on system availability and consistency. Therefore, as proposed in the famous [CAP theorem](https://medium.com/analytics-vidhya/cap-theorem-in-distributed-system-and-its-tradeoffs-d8d981ecf37e), a system architect needs to trade off between consistency and availability when network partition is inevitable.\n\n### Consistency\n\nIn short, consistency in a distributed database refers to the property that ensures every node or replica has the same view of data when writing or reading data at a given time. For a full list of consistency levels, read the doc [here](https://docs.microsoft.com/en-us/azure/cosmos-db/consistency-levels).\n\nTo clarify, here we are talking about consistency as in the CAP theorem, not ACID (atomicity, consistency, isolation, durability). Consistency in CAP theorem refers to each node in the system having the same data while consistency in ACID refers to a single node enforcing the same rules on every potential commit.\n\nGenerally, OLTP (online transaction processing) databases require strong consistency or linearizability to ensure that:\n- Each read can access the latest inserted data.\n- If a new value is returned after a read, all following reads, regardless on the same or different clients, must return the new value.\n\nThe essence of linearizability is to guarantee the recency of multiple data replicas - once a new value is written or read, all subsequent reads can view the new value until the value is later overwritten. A distributed system providing linearizability can save users the trouble of keeping an eye on multiple replicas, and can guarantee the atomicity and order or each operation.\n\n### Consensus\n\nThe concept of consensus is introduced to distributed systems as users are eager to see distributed systems work in the same way as standalone systems.\n\nTo put it simple, consensus is a general agreement on value. For instance, Steve and Frank wanted to grab something to eat. Steve suggested having sandwiches. Frank agreed to Steve's suggestion and both of them are had sandwiches. They reached a consensus. More specifically, a value (sandwiches) proposed by one of them is agreed upon by both, and both of them take actions based on the value. Similarly, consensus in a distributed system means when a process propose a value, all the rest processes in the system agree on and act upon this value. \n\n\n\n\n## Consensus-based replication\n\nThe earliest consensus-based algorithms were proposed along with [viewstamped replication](https://pmg.csail.mit.edu/papers/vr.pdf) in 1988. In 1989, Leslie Lamport proposed [Paxos](https://lamport.azurewebsites.net/pubs/paxos-simple.pdf), a consensus-based algorithm.\n\nIn recent years, we witness another prevalent consensus-based algorithm in the industry - [Raft](https://raft.github.io/). It has been adopted by many mainstream NewSQL databases like CockroachDB, TiDB, OceanBase, etc.\n\nNotably, a distributed system does not necessarily support linearizability even if it adopts consensus-based replication. However, linearizability is the prerequisite for building ACID distributed database.\n\nWhen designing a database system, the commit order of logs and state machines should be taken into consideration. Extra caution is also needed to maintain the leader lease of Paxos or Raft and prevent a split-brain under network partition.\n\n\n\n\n### Pros and cons\n\nIndeed, Raft, ZAB, and [quorum-based log protocol](https://aws.amazon.com/blogs/database/amazon-aurora-under-the-hood-quorum-and-correlated-failure/) in Aurora are all Paxos variations. Consensus-based replication has the following advantages:\n\n1. Though consensus-based replication focus more on consistency and network partition in the CAP theorem, it provides relatively better availability compared to traditional leader-follower replication. \n2. Raft is a breakthrough that greatly simplified consensus-based algorithms. And there are many open-source Raft libraries on GitHub (Eg. [sofa-jraft](https://github.com/sofastack/sofa-jraft)).\n3. The performance of consensus-based replication can satisfy most of the applications and businesses. With the coverage of high-performance SSD and gigabyte NICs (network interface card), the burden of synchronizing multiple replicas is relieved, making Paxos and Raft algorithms the mainstream in the industry.\n\nOne misconception is that consensus-based replication is the silver bullet to achieving data consistency in a distributed database. However, this is not the truth. The challenges in availability, complexity, and performance faced by consensus-based algorithm blocks it from being the perfect solution.\n\n1. Compromised availability\nOptimized Paxos or Raft algorithm has strong dependency on the leader replica, which comes with a weak ability to fight against grey failure. In consensus-based replication, a new election of leader replica will not take place until the leader node does not respond for a long time. Therefore, consensus-based replication is incapable of handling situations where the leader node is slow or a thrashing occurs.\n\n2. High complexity\nThough there are already many extended algorithms based on Paxos and Raft, the emergence of [Multi-Raft](http://www.vldb.org/pvldb/vol13/p3072-huang.pdf) and [Parallel Raft](https://www.vldb.org/pvldb/vol11/p1849-cao.pdf) requires more considerations and tests on synchronization between logs and state machines. \n\n3. Compromised performance\nIn a cloud-native era, local storage is replaced by shared storage solutions like EBS and S3 to ensure data reliability and consistency. As a result, consensus-based replication is no longer a must for distributed systems. What's more, consensus-based replication comes with the problem of data redundancy as both the solution and EBS has multiple replicas.\n\nFor multi-datacenter and multi-cloud replication, the pursuit for consistency compromises not only availability but also [latency](https://en.wikipedia.org/wiki/PACELC_theorem), resulting in a decline in performance. Therefore, linearizability is not a must for multi-datacenter disaster tolerance in most of the applications. \n\n## A log replication strategy for cloud-native and distributed database\n\nUndeniably, consensus-based algorithms like Raft and Paxos are still the mainstream algorithms adopted by many OLTP databases. However, by observing the examples of [PacificA](https://www.microsoft.com/en-us/research/publication/pacifica-replication-in-log-based-distributed-storage-systems/) protocol, [Socrates](https://www.microsoft.com/en-us/research/uploads/prod/2019/05/socrates.pdf) and [Rockset](https://rockset.com/), we can see the trend is shifting. \n\nThere are two major principles for a solution that can best serve a cloud-native, distributed database.\n\n### 1. Replication as a service\n\nA separate microservice dedicated to data synchronization is needed. The synchronization module and storage module should no longer be tightly coupled within the same process.\n\nFor instance, Socrates decouples storage, log, and computing. There is one dedicated log service (XLog service in the middle of the figure below).\n\n\n\n\nXLog service is an individual service. Data persistence is achieved with the help of low-latency storage. The landing zone in Socrates is in charge of keeping three replicas at an accelerated speed.\n\n\n\n\nThe leader node distributes logs to log broker asynchronously, and flushes data to Xstore. Local SSD cache can accelerate data read. Once data flush is successful, buffers in the landing zone can be cleaned. Obviously, all log data are divided into three layers - landing zone, local SSD, and XStore.\n\n### 2. Russian doll principle\n\nOne way to design a system is to follow the Russian doll principle: each layer is complete and perfectly suited to what the layer does so that other layers may be built on-top or around it. \n\nWhen designing a cloud-native database, we need to cleverly leverage other third-party services to reduce the complexity of system architecture.\n\nIt seems like we cannot get around with Paxos to avoid single point failure. However, we can still greatly simplify log replication by handing leader election over to Raft or Paxos services based on [Chubby](https://research.google.com/archive/chubby-osdi06.pdf), [Zk](https://github.com/bloomreach/zk-replicator), and [etcd](https://etcd.io/).\n\nFor instance, [Rockset](https://rockset.com/) architecture follows the Russian doll principle and uses Kafka/Kineses for distributed logs, S3 for storage, and local SSD cache for improving query performance. \n\n\n\n\n### The Milvus approach\n\nTunable consistency in Milvus is in fact similar to follower reads in consensus-based replication. Follower read feature refers to using follower replica to undertake data read tasks under the premise of strong consistency. The purpose is to enhance cluster throughput and reduce the load on leader. The mechanism behind follower read feature is inquiring the commit index of the latest log and providing query service until all data in the commit index are applied to state machines.\n\nHowever, the design of Milvus did not adopt the follower strategy. In other words, Milvus does not inquire commit index every time it receives a query request. Instead, Milvus adopts a mechanism like the watermark in [Flink](https://flink.apache.org/), which notifies query node the location of commit index at a regular interval. The reason for such a mechanism is that Milvus users usually do not have high demand for data consistency, and they can accept a compromise in data visibility for better system performance. \n\nIn addition, Milvus also adopts multiple microservices and separates storage from computing. In the [Milvus architecture](https://milvus.io/blog/deep-dive-1-milvus-architecture-overview.md#A-bare-bones-skeleton-of-the-Milvus-architecture), S3, MinIo, and Azure Blob are used for storage.\n\n\n\n\n## Summary\n\nNowadays, an increasing number of cloud-native databases are making log replication an individual service. By doing so, the cost of adding read-only replicas and heterogeneous replication can be reduced. Using multiple microservices enables quick utilization of mature cloud-based infrastructure, which is impossible for traditional databases. An individual log service can rely on consensus-based replication, but it can also follow the Russian doll strategy to adopt various consistency protocols together with Paxos or Raft to achieve linearizability.\n\n## References\n\n- Lamport L. Paxos made simple[J]. ACM SIGACT News (Distributed Computing Column) 32, 4 (Whole Number 121, December 2001), 2001: 51-58.\n- Ongaro D, Ousterhout J. In search of an understandable consensus algorithm[C]//2014 USENIX Annual Technical Conference (Usenix ATC 14). 2014: 305-319.\n- Oki B M, Liskov B H. Viewstamped replication: A new primary copy method to support highly-available distributed systems[C]//Proceedings of the seventh annual ACM Symposium on Principles of distributed computing. 1988: 8-17.\n- Lin W, Yang M, Zhang L, et al. PacificA: Replication in log-based distributed storage systems[J]. 2008.\n- Verbitski A, Gupta A, Saha D, et al. Amazon aurora: On avoiding distributed consensus for i/os, commits, and membership changes[C]//Proceedings of the 2018 International Conference on Management of Data. 2018: 789-796.\n- Antonopoulos P, Budovski A, Diaconu C, et al. Socrates: The new sql server in the cloud[C]//Proceedings of the 2019 International Conference on Management of Data. 2019: 1743-1756.\n","title":"Raft or not? The Best Solution to Data Consistency in Cloud-native Databases","metaData":{}},{"id":"deep-dive-8-knowhere.md","author":"Yudong Cai","desc":"And no, it's not Faiss.","tags":["Engineering"],"canonicalUrl":"https://milvus.io/blog/deep-dive-8-knowhere.md","date":"2022-05-10T00:00:00.000Z","cover":"https://assets.zilliz.com/Deep_Dive_8_6919720d59.png","href":"/blog/deep-dive-8-knowhere.md","content":"\n\n\n\u003e This article is written by [Yudong Cai](https://github.com/cydrain) and translated by [Angela Ni](https://www.linkedin.com/in/yiyun-n-2aa713163/).\n\nAs the core vector execution engine, Knowhere to Milvus is what an engine is to a sports car. This post introduces what Knowhere is, how it is different from Faiss, and how the code of Knowhere is structured.\n\n**Jump to:**\n- [The concept of Knowhere](#The-concept-of-Knowhere)\n- [Knowhere in the Milvus architecture](#Knowhere-in-the-Milvus-architecture)\n- [Knowhere Vs Faiss](#Knowhere-Vs-Faiss)\n- [Understanding the Knowhere code](#Understanding-the-Knowhere-code)\n- [Adding indexes to Knowhere](#Adding-indexes-to-Knowhere)\n\n## The concept of Knowhere\nNarrowly speaking, Knowhere is an operation interface for accessing services in the upper layers of the system and vector similarity search libraries like [Faiss](https://github.com/facebookresearch/faiss), [Hnswlib](https://github.com/nmslib/hnswlib), [Annoy](https://github.com/spotify/annoy) in the lower layers of the system. In addition, Knowhere is also in charge of heterogeneous computing. More specifically, Knowhere controls on which hardware (eg. CPU or GPU) to execute index building and search requests. This is how Knowhere gets its name - knowing where to execute the operations. More types of hardware including DPU and TPU will be supported in future releases.\n\nIn a broader sense, Knowhere also incorporates other third-party index libraries like Faiss. Therefore, as a whole, Knowhere is recognized as the core vector computation engine in the Milvus vector database.\n\nFrom the concept of Knowhere, we can see that it only processes data computing tasks, while those tasks like sharding, load balance, disaster recovery are beyond the work scope of Knowhere.\n\nStarting from Milvus 2.0.1, [Knowhere](https://github.com/milvus-io/knowhere) (in the broader sense) becomes independent from the Milvus project.\n\n## Knowhere in the Milvus architecture\n\n\n\n\n\nComputation in Milvus mainly involves vector and scalar operations. Knowhere only handles the operations on vectors in Milvus. The figure above illustrates the Knowhere architecture in Milvus.\n\nThe bottom-most layer is the system hardware. The third-party index libraries are on top of the hardware. Then Knowhere interacts with the index node and query node on the top via CGO. \n\nThis article talks about Knowhere in its broader sense, as marked within the blue frame in the architecture illustration.\n\n## Knowhere Vs Faiss\n\nKnowhere not only further extends the functions of Faiss but also optimizes the performance. More specifically, Knowhere has the following advantages.\n\n### 1. Support for BitsetView\n\nInitially, bitset was introduced in Milvus for the purpose of \"soft deletion\". A soft-deleted vector still exists in the database but will not be computed during a vector similarity search or query. Each bit in the bitset corresponds to an indexed vector. If a vector is marked as \"1\" in the bitset, it means this vector is soft-deleted and will not be involved during a vector search.\n\nThe bitset parameters are added to all the exposed Faiss index query APIs in Knowhere, including CPU and GPU indexes. \n\nLearn more about [how bitset enables the versatility of vector search](https://milvus.io/blog/2022-2-14-bitset.md).\n\n### 2. Support for more similarity metrics for indexing binary vectors\n\nApart from [Hamming](https://milvus.io/docs/v2.0.x/metric.md#Hamming-distance), Knowhere also supports [Jaccard](https://milvus.io/docs/v2.0.x/metric.md#Jaccard-distance), [Tanimoto](https://milvus.io/docs/v2.0.x/metric.md#Tanimoto-distance), [Superstructure](https://milvus.io/docs/v2.0.x/metric.md#Superstructure), [Substructure](https://milvus.io/docs/v2.0.x/metric.md#Substructure). Jaccard and Tanimoto can be used to measure the similarity between two sample sets while Superstructure and Substructure can be used to measure the similarity of chemical structures.\n\n### 3. Support for AVX512 instruction set\n\nFaiss itself supports multiple instruction sets including [AArch64](https://en.wikipedia.org/wiki/AArch64), [SSE4.2](https://en.wikipedia.org/wiki/SSE4#SSE4.2), [AVX2](https://en.wikipedia.org/wiki/Advanced_Vector_Extensions). Knowhere further extends the supported instruction sets by adding [AVX512](https://en.wikipedia.org/wiki/AVX-512), which can [improve the performance of index building and query by 20% to 30%](https://milvus.io/blog/milvus-performance-AVX-512-vs-AVX2.md) compared to AVX2.\n\n### 4. Automatic SIMD-instruction selection\n\nKnowhere is designed to work well on a wide spectrum of CPU processors (both on-premises and cloud platforms) with different SIMD instructions (e.g., SIMD SSE, AVX, AVX2, and AVX512). Thus the challenge is, given a single piece of software binary (i.e., Milvus), how to make it automatically invoke the suitable SIMD instructions on any CPU processor? Faiss does not support automatic SIMD-instruction selection and users need to manually specify the SIMD flag (e.g., “-msse4”) during compilation. However, Knowhere is built by refactoring the codebase of Faiss. Common functions (e.g., similarity computing) that rely on SIMD accelerations are factored out. Then for each function, four versions (i.e., SSE, AVX, AVX2, AVX512) are implemented and each put into a separate source file. Then the source files are further compiled individually with the corresponding SIMD flag. Therefore, at runtime, Knowhere can automatically choose the best suited SIMD instructions based on the current CPU flags and then link the right function pointers using hooking. \n\n### 5. Other performance optimization\nRead [Milvus: A Purpose-Built Vector Data Management System](https://www.cs.purdue.edu/homes/csjgwang/pubs/SIGMOD21_Milvus.pdf) for more about Knowhere's performance optimization.\n\n## Understanding the Knowhere code\n\nAs mentioned in the first section, Knowhere only handles vector search operations. Therefore, Knowhere only processes the vector field of an entity (currently, only one vector field for entities in a collection is supported). Index building and vector similarity search are also targeted at the vector field in a segment. To have a better understanding of the data model, read the blog [here](https://milvus.io/blog/deep-dive-1-milvus-architecture-overview.md#Data-Model).\n\n\n\n\n### Index\n\nIndex is a type of independent data structure from the original vector data. Indexing requires four steps: create an index, train data, insert data and build an index.\n\nFor some of the AI applications, dataset training is an individual process from vector search. In this type of application, data from datasets are first trained and then inserted into a vector database like Milvus for similarity search. Open datasets like sift1M and sift1B provides data for training and testing. However, in Knowhere, data for training and searching are mixed together. That is to say, Knowhere trains all the data in a segment and then inserts all the trained data and builds an index for them.\n\n### Knowhere code structure\n\nDataObj is the base class of all data structure in Knowhere. `Size()` is the only virtual method in DataObj. The Index class inherits from DataObj with a field named \"size_\". The Index class also has two virtual methods - `Serialize()` and `Load()`. The VecIndex class derived from Index is the virtual base class for all vector indexes. VecIndex provides methods including `Train()`, `Query()`, `GetStatistics()`, and `ClearStatistics()`.\n\n\n\n\n\nOther index types are listed on the right in the figure above. \n\n- The Faiss index has two sub classes: FaissBaseIndex for all indexes on float point vectors, and FaissBaseBinaryIndex for all indexes on binary vectors.\n- GPUIndex is the base class for all Faiss GPU indexes.\n- OffsetBaseIndex is the base class for all self-developed indexes. Only vector ID is stored in the index file. As a result, an index file size for 128-dimensional vectors can be reduced by 2 orders of magnitude. We recommend taking the original vectors into consideration as well when using this type of index for vector similarity search.\n\n\n\n\n\nTechnically speaking, [IDMAP](https://github.com/facebookresearch/faiss/wiki/Guidelines-to-choose-an-index#then-flat) is not an index, but rather used for brute-force search. When vectors are inserted to the vector database, no data training and index building is required. Searches will be conducted directly on the inserted vector data.\n\nHowever, for the sake of code consistency, IDMAP also inherits from the VecIndex class with all its virtual interfaces. The usage of IDMAP is the same as other indexes.\n\n\n\n\n\nThe IVF (inverted file) indexes are the most frequently used. The IVF class is derived from VecIndex and FaissBaseIndex, and further extends to IVFSQ and IVFPQ. GPUIVF is derived from GPUIndex and IVF. Then GPUIVF further extends to GPUIVFSQ and GPUIVFPQ.\n\nIVFSQHybrid is a class for self-developed hybrid index that is executed by coarse quantize on GPU. And search in the bucket is executed on CPU. This type of index can reduce the occurrence of memory copy between CPU and GPU by leveraging the computing power of GPU. IVFSQHybrid has the same recall rate as GPUIVFSQ but comes with a better performance.\n\nThe base class structure for binary indexes are relatively simpler. BinaryIDMAP and BinaryIVF are derived from FaissBaseBinaryIndex and VecIndex.\n\n\n\n\n\nCurrently, only two types of third-party indexes are supported apart from Faiss: tree-based index Annoy, and graph-based index HNSW. These two common and frequently used third-party indexes are both derived from VecIndex. \n\n## Adding indexes to Knowhere\n\nIf you want to add new indexes to Knowhere, you can refer to existing indexes first:\n- To add quantization-based index, refer to IVF_FLAT. \n- To add graph-based index, refer to HNSW. \n- To add tree-based index, refer to Annoy.\n\nAfter referring to the existing index, you can follow the steps below to add a new index to Knowhere.\n1. Add the name of the new index in `IndexEnum`. The data type is string.\n2. Add data validation check on the new index in the file `ConfAdapter.cpp`. The validation check is mainly to validate the parameters for data training and query.\n3. Create a new file for the new index. The base class of the new index should include `VecIndex`, and the necessary virtual interface of `VecIndex`.\n4. Add the index building logic for new index in `VecIndexFactory::CreateVecIndex()`.\n5. Add unit test under the `unittest` directory.\n\n\n## About the Deep Dive Series\n\nWith the [official announcement of general availability](https://milvus.io/blog/2022-1-25-annoucing-general-availability-of-milvus-2-0.md) of Milvus 2.0, we orchestrated this Milvus Deep Dive blog series to provide an in-depth interpretation of the Milvus architecture and source code. Topics covered in this blog series include:\n\n- [Milvus architecture overview](https://milvus.io/blog/deep-dive-1-milvus-architecture-overview.md)\n- [APIs and Python SDKs](https://milvus.io/blog/deep-dive-2-milvus-sdk-and-api.md)\n- [Data processing](https://milvus.io/blog/deep-dive-3-data-processing.md)\n- [Data management](https://milvus.io/blog/deep-dive-4-data-insertion-and-data-persistence.md)\n- [Real-time query](https://milvus.io/blog/deep-dive-5-real-time-query.md)\n- [Scalar execution engine](https://milvus.io/blog/deep-dive-7-query-expression.md)\n- [QA system](https://milvus.io/blog/deep-dive-6-oss-qa.md)\n- [Vector execution engine](https://milvus.io/blog/deep-dive-8-knowhere.md)\n","title":"What Powers Similarity Search in Milvus Vector Database?","metaData":{}},{"id":"deep-dive-7-query-expression.md","author":"Milvus","desc":"A vector query is the process of retrieving vectors via scalar filtering.","tags":["Engineering"],"canonicalUrl":"https://milvus.io/blog/deep-dive-7-query-expression.md","date":"2022-05-05T00:00:00.000Z","cover":"https://assets.zilliz.com/Deep_Dive_7_baae830823.png","href":"/blog/deep-dive-7-query-expression.md","content":"\n\n\n\u003e This article is transcreated by [Angela Ni](https://www.linkedin.com/in/yiyun-n-2aa713163/).\n\nA [vector query](https://milvus.io/docs/v2.0.x/query.md) in Milvus is the process of retrieving vectors via scalar filtering based on boolean expression. With scalar filtering, users can limit their query results with certain conditions applied on attributes of data. For instance, if a user queries for films released during 1990-2010 and with scores higher than 8.5, only films whose attributes (release year and score) fulfill the condition.\n\nThis post aims to examine how a query is completed in Milvus from the input of a query expression to query plan generation and query execution. \n\n**Jump to:**\n- [Query expression](#Query-expression)\n- [Plan AST generation](#Plan-AST-generation)\n- [Query execution](#Query-execution)\n\n## Query expression\n\nExpression of query with attribute filtering in Milvus adopts the EBNF(Extended Backus–Naur form) syntax. The image below is the expression rules in Milvus.\n\n\n\nLogical expressions can be created using the combination of binary logical operators, unary logical operators, logical expressions, and single expressions. Since EBNF syntax is itself recursive, a logical expression can be the outcome of the combination or part of a bigger logical expression. A logical expression can contain many sub-logical expressions. The same rule applies in Milvus. If a user needs to filter the attributes of the results with many conditions, the user can create his own set of filtering conditions by combining different logical operators and expressions.\n\n\n\nThe image above shows part of the [Boolean expression rules](https://milvus.io/docs/v2.0.x/boolean.md) in Milvus. Unary logical operators can be added to an expression. Currently Milvus only supports the unary logical operator \"not\", which indicates that the system needs to take the vectors whose scalar field values do not satisfy the calculation results. Binary logical operators include \"and\" and \"or\". Single expressions include term expressions and compare expressions.\n\nBasic arithmetic calculation like addition, subtraction, multiplication, and division is also supported during a query in Milvus. The following image demonstrates the precedence of the operations. Operators are listed from top to bottom in descending precedence.\n\n\n\n### How a query expression on certain films is processed in Milvus?\n\nSuppose there is an abundance of film data stored in Milvus and the user wants to query certain films. As an example, each film data stored in Milvus has the following five fields: film ID, release year, film type, score, and poster. In this example, the data type of film ID and release year is int64, while film scores are float point data. Also, film posters are stored in the format of float-point vectors, and film type in the format of string data. Notably, support for string data type is a new feature in Milvus 2.1.\n\nFor instance, if a user want to query the movies with scores higher than 8.5. The films should also be released during a decade before 2000 to a decade after 2000 or their types should be either comedy or action movie, the user need to input the following predicate expression: `score \u003e 8.5 \u0026\u0026 (2000 - 10 \u003c release_year \u003c 2000 + 10 || type in [comedy,action])`.\n\nUpon receiving the query expression, the system will execute it in the following precedence:\n\n1. Query for films with scores higher than 8.5. The query results are called \"result1\".\n2. Calculate 2000 - 10 to get \"result2\" (1990).\n3. Calculate 2000 + 10 to get \"result3\" (2010).\n4. Query for films with the value of `release_year` greater than \"result2\" and smaller than \"result3\". That is to say, the system needs to query for films released between 1990 and 2010. The query results are called \"result4\".\n5. Query for films that are either comedies or action movies. The query results are called \"result5\".\n6. Combine \"result4\" and \"result5\" to obtain films that are either released between 1990 and 2010 or belong to the category of comedy or action movie. The results are called \"result6\".\n7. Take the common part in \"result1\" and \"result6\" to obtain the final results satisfying all the conditions.\n\n\n\n## Plan AST generation\n\nMilvus leverages the open-source tool [ANTLR](https://www.antlr.org/) (ANother Tool for Language Recognition) for plan AST (abstract syntax tree) generation. ANTLR is a powerful parser generator for reading, processing, executing, or translating structure text or binary files. More specifically, ANTLR can generate a parser for building and walking parse trees based on pre-defined syntax or rules. The following image is an example in which the input expression is \"SP=100;\". LEXER, the built-in language recognition functionality in ANTLR, generates four tokens for the input expression - \"SP\", \"=\", \"100\", and \";\". Then the tool will further parse the four tokens to generate the corresponding parse tree.\n\n\n\nThe walker mechanism is a crucial part in the ANTLR tool. It is designed to walk through all the parse trees to examine whether each node obeys the syntax rules, or to detect certain sensitive words. Some of the relevant APIs are listed in the image below. Since ANTLR starts from the root node and goes down all the way through each sub-node to the bottom, there is no need to differentiate the order of how to walk through the parse tree. \n\n\n\nMilvus generates the PlanAST for query in a similar way to the ANTLR. However, using ANTLR requires redefining rather complicated syntax rules. Therefore, Milvus adopts one of the most prevalent rules - Boolean expression rules, and depends on the [Expr](https://github.com/antonmedv/expr) package open sourced on GitHub to query and parse the syntax of query expressions.\n\nDuring a query with attribute filtering, Milvus will generate a primitive unsolved plan tree using ant-parser, the parsing method provided by Expr, upon receiving the query expression. The primitive plan tree we will get is a simple binary tree. Then the plan tree is fine-tuned by Expr and the built-in optimizer in Milvus. The optimizer in Milvus is quite similar to the aforementioned walker mechanism. Since the plan tree optimization functionality provided by Expr is pretty sophisticated, the burden of the Milvus built-in optimizer is alleviated to a great extent. Ultimately, the analyzer analyzes the optimized plan tree in a recursive way to generate a plan AST in the structure of [protocol buffers](https://developers.google.com/protocol-buffers) (protobuf).\n\n\n\n## Query execution\n\nQuery execution is at root the execution of the plan AST generated in the previous steps.\n\nIn Milvus, a plan AST is defined in a proto structure. The image below is a message with the protobuf structure. There are six types of expressions, among which binary expression and unary expression can further have binary logical expression and unary logical expression.\n\n\n\n\n\nThe image below is a UML image of the query expression. It demonstrates the basic class and derivative class of each expression. Each class comes with a method to accept visitor parameters. This is a typical visitor design pattern. Milvus uses this pattern to execute the plan AST as its biggest advantage is that users do not have to do anything to the primitive expressions but can directly access one of the methods in the patterns to modify certain query expression class and relevant elements.\n\n\n\nWhen executing a plan AST, Milvus first receives a proto-type plan node. Then a segcore-type plan node is obtained via the internal C++ proto parser. Upon obtaining the two types of plan nodes, Milvus accepts a series of class access and then modifies and executes in the internal structure of the plan nodes. Finally, Milvus searches through all the execution plan nodes to obtain the filtered results. The final results are output in the format of a bitmask. A bitmask is an array of bit numbers (\"0\" and \"1\"). Those data satisfying filter conditions are marked as \"1\" in the bitmask, while those do not meet the requirements are marked as \"0\" in the bitmask.\n\n\n\n## About the Deep Dive Series\n\nWith the [official announcement of general availability](https://milvus.io/blog/2022-1-25-annoucing-general-availability-of-milvus-2-0.md) of Milvus 2.0, we orchestrated this Milvus Deep Dive blog series to provide an in-depth interpretation of the Milvus architecture and source code. Topics covered in this blog series include:\n\n- [Milvus architecture overview](https://milvus.io/blog/deep-dive-1-milvus-architecture-overview.md)\n- [APIs and Python SDKs](https://milvus.io/blog/deep-dive-2-milvus-sdk-and-api.md)\n- [Data processing](https://milvus.io/blog/deep-dive-3-data-processing.md)\n- [Data management](https://milvus.io/blog/deep-dive-4-data-insertion-and-data-persistence.md)\n- [Real-time query](https://milvus.io/blog/deep-dive-5-real-time-query.md)\n- [Scalar execution engine](https://milvus.io/blog/deep-dive-7-query-expression.md)\n- [QA system](https://milvus.io/blog/deep-dive-6-oss-qa.md)\n- [Vector execution engine](https://milvus.io/blog/deep-dive-8-knowhere.md)\n\n\n\n\n","title":"How Does the Database Understand and Execute Your Query?","metaData":{}},{"id":"deep-dive-6-oss-qa.md","author":"Wenxing Zhu","desc":"Quality assurance is a process of determining whether a product or service meets certain requirements.","tags":["Engineering"],"canonicalUrl":"https://milvus.io/blog/deep-dive-6-oss-qa.md","date":"2022-04-25T00:00:00.000Z","cover":"https://assets.zilliz.com/Deep_Dive_6_c2cd44801d.png","href":"/blog/deep-dive-6-oss-qa.md","content":"\n\n\n\u003e This article is written by [Wenxing Zhu](https://github.com/zhuwenxing) and transcreated by [Angela Ni](https://www.linkedin.com/in/yiyun-n-2aa713163/).\n\nQuality assurance (QA) is a systematic process of determining whether a product or service meets certain requirements. A QA system is an indispensable part of the R\u0026D process because, as its name suggests, it ensures that quality of the product.\n\nThis post introduces the QA framework adopted in developing the Milvus vector database, aiming to provide a guideline for contributing developers and users to participate in the process. It will also cover the major test modules in Milvus as well as methods and tools that can be leveraged to improve the efficiency of QA testings.\n\n**Jump to:**\n- [A general introduction to the Milvus QA system](#A-general-introduction-to-the-Milvus-QA-system)\n- [Test modules in Milvus](#Test-modules-in-Milvus)\n- [Tools and methods for better QA efficiency](#Tools-and-methods-for-better-QA-efficiency)\n\n## A general introduction to the Milvus QA system\n\nThe [system architecture](https://milvus.io/blog/deep-dive-1-milvus-architecture-overview.md) is critical to conducting QA testings. The more a QA engineer is familiar with the system, the more likely he or she is going to come up with a reasonable and efficient testing plan. \n\n\n\nMilvus 2.0 adopts a [cloud-native, distributed, and layered architecture](https://milvus.io/blog/deep-dive-1-milvus-architecture-overview.md#A-cloud-native-first-approach), with SDK being the [main entrance for data](https://milvus.io/blog/deep-dive-2-milvus-sdk-and-api.md) to flow in Milvus. The Milvus users leverage the SDK very frequently, hence functional testing on the SDK side is much needed. Also, function tests on SDK can help detect the internal issues that might exist within the Milvus system. Apart from function tests, other types of tests will also be conducted on the vector database, including unit tests, deployment tests, reliability tests, stability tests, and performance tests. \n\nA cloud-native and distributed architecture brings both convenience and challenges to QA testings. Unlike systems that are deployed and run locally, a Milvus instance deployed and run on a Kubernetes cluster can ensure that software testing is carried out under the same circumstance as software development. However, the downside is that the complexity of distributed architecture brings more uncertainties that can make QA testing of the system even harder and strenuous. For instance, Milvus 2.0 uses microservices of different components, and this leads to an increased number of [services and nodes](https://milvus.io/blog/deep-dive-1-milvus-architecture-overview.md#A-bare-bones-skeleton-of-the-Milvus-architecture), and a greater possibility of a system error. Consequently, a more sophisticated and comprehensive QA plan is needed for better testing efficiency.\n\n### QA testings and issue management\n\nQA in Milvus involves both conducting tests and managing issues emerged during software development.\n\n#### QA testings\n\nMilvus conducts different types of QA testing according to Milvus features and user needs in order of priority as shown in the image below. \n\n\n\nQA testings are conducted on the following aspects in Milvus in the following priority:\n\n1. **Function**: Verify if the functions and features work as originally designed.\n2. **Deployment**: Check if a user can deploy, reinstall, and upgrade both Mivus standalone version and Milvus cluster with different methods (Docker Compose, Helm, APT or YUM, etc.). \n3. **Performance**: Test the performance of data insertion, indexing, vector search and query in Milvus.\n4. **Stability**: Check if Milvus can run stably for 5-10 days under a normal level of workload.\n5. **Reliability**: Test if Milvus can still partly function if certain system error occurs. \n6. **Configuration**: Verify if Milvus works as expected under certain configuration.\n7. **Compatibility**: Test if Milvus is compatible with different types of hardware or software.\n\n#### Issue management\n\nMany issues may emerge during software development. The author of the templated issues can be QA engineers themselves or Milvus users from the open-source community. The QA team is responsible for figuring out the issues.\n\n\n\nWhen an [issue](https://github.com/milvus-io/milvus/issues) is created, it will go through triage first. During triage, new issues will be examined to ensure that sufficient details of the issues are provided. If the issue is confirmed, it will be accepted by the developers and they will try to fix the issues. Once development is done, the issue author needs to verify if it is fixed. If yes, the issue will be ultimately closed.\n\n### When is QA needed?\n\nOne common misconception is that QA and development are independent from each other. However, the truth is to ensure the quality of the system, efforts are needed from both developers and QA engineers. Therefore, QA needs to be involved throughout the whole lifecycle.\n\n\n\nAs shown in the figure above, a complete software R\u0026D lifecycle includes three stages. \n\nDuring the initial stage, the developers publish design documentation while QA engineers come up with test plans, define release criteria, and assign QA tasks. Developers and QA engineers need to be familiar with both the design doc and test plan so that a mutual understanding of the objective of the release (in terms of features, performance, stability, bug convergence, etc.) is shared among the two teams. \n\nDuring R\u0026D, development and QA testings interact frequently to develop and verify features and functions, and fix bugs and issues reported by the open-source [community](https://slack.milvus.io/) as well.\n\nDuring the final stage, if the release criteria is met, a new Docker image of the new Milvus version will be released. A release note focusing on new features and fixed bugs and a release tag is needed for the official release. Then the QA team will also publish a testing report on this release.\n\n## Test modules in Milvus\n\nThere are several test modules in Milvus and this section will explain each module in detail.\n\n### Unit test\n\n\n\nUnit tests can help identify software bugs at an early stage and provide a verification criteria for code restructuring. According to the Milvus pull request (PR) acceptance criteria, the [coverage](https://app.codecov.io/gh/milvus-io/milvus/) of code unit test should be 80%.\n\n### Function test\n\nFunction tests in Milvus are mainly organized around [PyMilvus](https://github.com/milvus-io/pymilvus) and SDKs. The main purpose of function tests are to verify if the interfaces can work as designed. Function tests have two facets:\n\n- Test if SDKs can return expected results when correct parameters are passed.\n- Test if SDKs can handle errors and return reasonable error messages when incorrect parameters are passed.\n\nThe figure below depicts the current framework for function tests which is based on the mainstream [pytest](https://pytest.org/) framework. This framework adds a wrapper to PyMilvus and empowers testing with an automated testing interface.\n\n\n\nConsidering a shared testing method is needed and some functions need to be reused, the above testing framework is adopted, rather than using the PyMilvus interface directly. A \"check\" module is also included in the framework to bring convenience to the verification of expected and actual values.\n\nAs many as 2,700 function test cases are incorporated into the `tests/python_client/testcases` directory, fully covering almost all the PyMilvus interfaces. These function tests strictly supervise the quality of each PR.\n\n### Deployment test\n\nMilvus comes in two modes: [standalone](https://milvus.io/docs/v2.0.x/install_standalone-docker.md) and [cluster](https://milvus.io/docs/v2.0.x/install_cluster-docker.md). And there are two major ways to deploy Milvus: using Docker Compose or Helm. And after deploying Milvus, users can also restart or upgrade the Milvus service. There are two main categories of deployment test: restart test and upgrade test.\n\nRestart test refers to the process of testing data persistence, i.e. whether data are still available after a restart. Upgrade test refers to the process of testing data compatibility to prevent situations where incompatible formats of data are inserted into Milvus. Both the two types of deployment tests share the same workflow as illustrated in the image below.\n\n\n\nIn a restart test, the two deployments uses the same docker image. However in an upgrade test, the first deployment uses a docker image of a previous version while the second deployment uses a docker image of a later version. The test results and data are saved in the `Volumes` file or [persistent volume claim](https://kubernetes.io/docs/concepts/storage/persistent-volumes/) (PVC).\n\nWhen running the first test, multiple collections are created and different operations are made to each of the collection. When running the second test, the main focus will be on verifying if the created collections are still available for CRUD operations, and if new collections can be further created.\n\n### Reliability test\n\nTestings on the reliability of cloud-native distributed system usually adopt a chaos engineering method whose purpose is to nip errors and system failures in the bud. In other words, in an chaos engineering test, we purposefully create system failures to identify issues in pressure tests and fix system failures before they really start to do hazards. During a chaos test in Milvus, we choose [Chaos Mesh](https://chaos-mesh.org/) as the tool to create a chaos. There are several types of failures that needs to be created:\n\n- **Pod kill**: a simulation of the scenario where nodes are down.\n- **Pod failure**: Test if one of the worker node pods fails whether the whole system can still continue to work.\n- **Memory stress**: a simulation of heavy memory and CPU resources consumption from the work nodes.\n- **Network partition**: Since Milvus [separates storage from computing](https://milvus.io/docs/v2.0.x/four_layers.md), the system relies heavily on the communication between various components. A simulation of the scenario where the communication between different pods are partitioned is needed to test the interdependency of different Milvus components.\n\n\n\nThe figure above demonstrates the reliability test framework in Milvus that can automate chaos tests. The workflow of a reliability test is as follows:\n\n1. Initialize a Milvus cluster by reading the deployment configurations.\n2. When the cluster is ready, run `test_e2e.py` to test if the Milvus features are available.\n3. Run `hello_milvus.py` to test data persistence. Create a collection named \"hello_milvus\" for data insertion, flush, index building, vector search and query. This collection will not be released or dropped during the test.\n4. Create a monitoring object which will start six threads executing create, insert, flush, index, search and query operations.\n\n```\ncheckers = {\n Op.create: CreateChecker(),\n Op.insert: InsertFlushChecker(),\n Op.flush: InsertFlushChecker(flush=True),\n Op.index: IndexChecker(),\n Op.search: SearchChecker(),\n Op.query: QueryChecker()\n}\n```\n\n5. Make the first assertion - all operations are successful as expected.\n6. Introduce a system failure to Milvus by using Chaos Mesh to parse the yaml file which defines the failure. A failure can be killing the query node every five seconds for instance.\n7. Make the second assertion while introducing a system failure - Judge whether the returned results of the operations in Milvus during a system failure matches the expectation.\n8. Eliminate the failure via Chaos Mesh.\n9. When the Milvus service is recovered (meaning all pods are ready), make the third assertion - all operations are successful as expected.\n10. Run `test_e2e.py` to test if the Milvus features are available. Some of the operations during the chaos might be blocked due to the third assertion. And even after the chaos is eliminated, some operations might continue to be blocked, hampering the third assertion from being successful as expected. This step aims to facilitate the third assertion and serves as a standard for checking if the Milvus service has recovered.\n11. Run `hello_milvus.py`, load the created collection, and conduct CRUP operations on the collection. Then, check if the data existing before the system failure are still available after failure recovery. \n12. Collect logs.\n\n### Stability and performance test\n\nThe figure below describes the purposes, test scenarios, and metrics of stability and performance test.\n\n| | Stability test | Performance test |\n|------------|--------------------------------------------------------------------------------------------------------------------------------------------------------------------------------------------------------------------------------------------------------------------------------------------------------------------------------------------------------------------------------------------------|-------------------------------------------------------------------------------------------------------------------------------------------------------------------------------------------------------------------------------------|\n| Purposes | - Ensure that Milvus can work smoothly for a fixed period of time under normal workload. \u003cbr\u003e - Make sure resources are consumed stably when the Milvus service starts. | - Test the performance of alll Milvus interfaces. \u003cbr\u003e - Find the optimal configuration with the help of performance tests. \u003cbr\u003e - Serve as the benchmark for future releases. \u003cbr\u003e - Find the bottleneck that hampers a better performance. |\n| Scenarios | - Offline read-intensive scenario where data are barely updated after insertion and the percentage of processing each type of request is: search request 90%, insert request 5%, others 5%. \u003cbr\u003e - Online write-intensive scenario where data are inserted and searched simultaneously and the percentage of processing each type of request is: insert request 50%, search request 40%, others 10%. | - Data insertion \u003cbr\u003e - Index building \u003cbr\u003e - Vector search |\n| Metrics | - Memory usage \u003cbr\u003e - CPU consumption \u003cbr\u003e - IO latency \u003cbr\u003e - The status of Milvus pods \u003cbr\u003e - Response time of the Milvus service \u003cbr\u003e etc. | - Data throughput during data insertion \u003cbr\u003e - The time it takes to build an index \u003cbr\u003e - Response time during a vector search \u003cbr\u003e - Query per second (QPS) \u003cbr\u003e - Request per second \u003cbr\u003e - Recall rate \u003cbr\u003e etc. |\n\nBoth stability test and performance test share the same set of workflow:\n\n\n\n1. Parse and update configurations, and define metrics. The `server-configmap` corresponds to the configuration of Milvus standalone or cluster while `client-configmap` corresponds to the test case configurations.\n2. Configure the server and the client.\n3. Data preparation\n4. Request interaction between the server and the client.\n5. Report and display metrics.\n\n## Tools and methods for better QA efficiency\n\nFrom the module testing section, we can see that the procedure for most of the testings are in fact almost the same, mainly involving modifying Milvus server and client configurations, and passing API parameters. When there are multiple configurations, the more varied the combination of different configurations, the more testing scenarios these experiments and tests can cover. As a result, the reuse of codes and procedures is all the more critical to the process of enhancing testing efficiency.\n\n### SDK test framework\n\n\n\nTo accelerate the testing process, we can add an `API_request` wrapper to the original testing framework, and set it as something similar to the API gateway. This API gateway will be in charge of collecting all API requests and then pass them to Milvus to collectively receive responses. These responses will be passed back to the client afterwards. Such a design makes capturing certain log information like parameters, and returned results much more easier. In addition, the checker component in the SDK test framework can verify and examine the results from Milvus. And all checking methods can be defining within this checker component.\n\nWith the SDK test framework, some crucial initialization processes can be wrapped into one single function. By doing so, large chunks of tedious codes can be eliminated.\n\nIt is also noteworthy that each individual test case is related to its unique collection to ensure data isolation.\n\nWhen executing test cases,`pytest-xdist`, the pytest extension, can be leveraged to execute all individual test cases in parallel, greatly boosting the efficiency.\n\n### GitHub action\n\n\n\n[GitHub Action](https://docs.github.com/en/actions) is also adopted to improve QA efficiency for its following characteristics:\n\n- It is a native CI/CD tool deeply integrated with GitHub.\n- It comes with a uniformly configured machine environment and pre-installed common software development tools including Docker, Docker Compose, etc.\n- It supports multiple operating systems and versions including Ubuntu, MacOs, Windows-server, etc.\n- It has a marketplace that offers rich extensions and out-of-box functions.\n- Its matrix supports concurrent jobs, and reusing the same test flow to improve efficiency \n\nApart from the characteristics above, another reason for adopting GitHub action is that deployment tests and reliability tests require independent and isolated environment. And GitHub Action is ideal for daily inspection checks on small-scale datasets.\n\n### Tools for benchmark tests\n\nTo make QA tests more efficient, a number of tools are used.\n\n\n\n- [Argo](https://argoproj.github.io/): a set of open-source tools for Kubernetes to run workflows and manage clusters by scheduling tasks. It can also enable running multiple tasks in parallel.\n- [Kubernetes dashboard](https://kubernetes.io/docs/tasks/access-application-cluster/web-ui-dashboard/): a web-based Kubernetes user interface for visualizing `server-configmap` and `client-configmap`.\n- [NAS](https://en.wikipedia.org/wiki/Network-attached_storage): Network attached storage (NAS) is a file-level computer data storage server for keeping common ANN-benchmark datasets.\n- [InfluxDB](https://www.influxdata.com/) and [MongoDB](https://www.mongodb.com/): Databases for saving results of benchmark tests.\n- [Grafana](https://grafana.com/): An open-source analytics and monitoring solution for monitoring server resource metrics and client performance metrics.\n- [Redash](https://redash.io/): A service that helps visualize your data and create charts for benchmark tests.\n\n## About the Deep Dive Series\n\nWith the [official announcement of general availability](https://milvus.io/blog/2022-1-25-annoucing-general-availability-of-milvus-2-0.md) of Milvus 2.0, we orchestrated this Milvus Deep Dive blog series to provide an in-depth interpretation of the Milvus architecture and source code. Topics covered in this blog series include:\n\n- [Milvus architecture overview](https://milvus.io/blog/deep-dive-1-milvus-architecture-overview.md)\n- [APIs and Python SDKs](https://milvus.io/blog/deep-dive-2-milvus-sdk-and-api.md)\n- [Data processing](https://milvus.io/blog/deep-dive-3-data-processing.md)\n- [Data management](https://milvus.io/blog/deep-dive-4-data-insertion-and-data-persistence.md)\n- [Real-time query](https://milvus.io/blog/deep-dive-5-real-time-query.md)\n- [Scalar execution engine](https://milvus.io/blog/deep-dive-7-query-expression.md)\n- [QA system](https://milvus.io/blog/deep-dive-6-oss-qa.md)\n- [Vector execution engine](https://milvus.io/blog/deep-dive-8-knowhere.md)\n","title":"Open Source Software (OSS) Quality Assurance - A Milvus Case Study","metaData":{}},{"id":"2022-4-12-milvus-10k-stars.md","author":"The Milvus Community","desc":"We recently hit an incredible milestone in the journey of vector database - over 10K stars on the GitHub repo of Milvus.","tags":["News"],"canonicalUrl":"https://milvus.io/blog/2022-4-12-milvus-10k-stars.md","date":"2022-04-12T00:00:00.000Z","cover":"https://assets.zilliz.com/20220516_163732_d40acac221.png","href":"/blog/2022-4-12-milvus-10k-stars.md","content":"\n\n\nWe recently hit an incredible milestone in the journey of vector database - over 10K stars on the [GitHub repo of Milvus](https://github.com/milvus-io/milvus). Thanks to each and every one of you who has supported us by giving us a star ⭐️.\n\nIt has been a wonderful 2.5 years since Milvus became an open-source project. When we first started, very few people understood exactly what a vector database is, let alone talking about how it would enable and accelerate AI adoption in our daily lives. Milvus vector database carries our vision of defining and building the next-generation database for AI. We combine SOTA algorithms with cloud technologies in the Milvus project so developers could build AI applications at ease with Milvus vector database.\n\n\n\n\u003cdiv align=\"center\"\u003eClick \u003ca href=\"https://www.youtube.com/watch?v=zIAX_oPI2Jk\u0026ab_channel=Milvus-VectorDatabase\"\u003ehere\u003c/a\u003e to watch the full video of Milvus repository development visualization.\u003c/div\u003e\n\n\u003cbr/\u003e\n\n\nThere are many [brilliant projects](https://github.com/milvus-io/milvus) that laid the foundation for Milvus, and we are thankful to stand on the shoulders of the giants. Without them Milvus wouldn't have existed. \n\nWe are also incredibly grateful to our awesome community, who has helped open an exciting chapter for this new type of database. Before we look forward, we want to take a moment to reflect on this journey, gaze at our milestones and acknowledge and thank our developers:\n\n- We joined LF AI \u0026 Data Foundation at [incubation](https://lfaidata.foundation/blog/2020/04/02/milvus-joins-lf-ai-as-new-incubation-project/) level, finding our home alongside many other great open source AI and data projects. Now Milvus is already a [graduation project](https://lfaidata.foundation/blog/2021/06/23/lf-ai-data-foundation-announces-graduation-of-milvus-project/). \n- We delivered two major releases: [Milvus 1.0](https://milvus.io/docs/v1.0.0/announcement.md) and [Milvus 2.0](https://milvus.io/docs/v2.0.x/comparison.md). \n- A SIGMOD paper was published: [Milvus: A Purpose-Built Vector Data Management System](https://dl.acm.org/doi/abs/10.1145/3448016.3457550).\n- Currently, we have more than 10,000 community members and over 220 contributors worldwide. \n- There are over [1000 enterprise users](https://milvus.io/) who adopted Milvus. Among them, there are many industry leaders. \n\n\n\nAll of this is only made possible by every single person who contributes. Thank you for your pull requests, issue reports, emails, meetings, and sharing your knowledge of Milvus. You all are playing a valuable part in Milvus' progress. \n\n## Special event\n\nFor this special milestone, we have prepared a small thank-you gift – [a downloadable desktop wallpaper](https://assets.zilliz.com/10_K_stars_2b2e0e0b36.png). Hope you enjoy it! \n\nIt'd be nice if you help [spread the word](https://twitter.com/milvusio)! ❤️\n\n","title":"A Small Step for Milvus - 10,000 Stars","metaData":{}},{"id":"deep-dive-5-real-time-query.md","author":"Xi Ge","desc":"Learn about the underlying mechanism of real-time query in Milvus.","tags":["Engineering"],"canonicalUrl":"https://milvus.io/blog/deep-dive-5-real-time-query.md","date":"2022-04-11T00:00:00.000Z","cover":"https://assets.zilliz.com/deep_dive_5_5e9175c7f7.png","href":"/blog/deep-dive-5-real-time-query.md","content":"\n\n\n\u003e This article is written by [Xi Ge](https://github.com/xige-16) and transcreated by [Angela Ni](https://www.linkedin.com/in/yiyun-n-2aa713163/).\n\nIn the previous post, we have talked about [data insertion and data persistence](https://milvus.io/blog/deep-dive-4-data-insertion-and-data-persistence.md) in Milvus. In this article, we will continue to explain how [different components](https://milvus.io/blog/deep-dive-1-milvus-architecture-overview.md) in Milvus interact with each other to complete real-time data query.\n\n*Some useful resources before getting started are listed below. We recommend reading them first to better understand the topic in this post.*\n- [Deep dive into the Milvus architecture](https://milvus.io/blog/deep-dive-1-milvus-architecture-overview.md)\n- [Milvus data model](https://milvus.io/blog/deep-dive-1-milvus-architecture-overview.md#Data-Model)\n- [The role and function of each Milvus component](https://milvus.io/docs/v2.0.x/four_layers.md)\n- [Data processing in Milvus](https://milvus.io/blog/deep-dive-3-data-processing.md)\n- [Data insertion and data persistence in Milvus](https://milvus.io/blog/deep-dive-4-data-insertion-and-data-persistence.md)\n\n## Load data to query node\n\nBefore a query is executed, the data has to be loaded to the query nodes first.\n\nThere are two types of data that are loaded to query node: streaming data from [log broker](https://milvus.io/docs/v2.0.x/four_layers.md#Log-broker), and historical data from [object storage](https://milvus.io/docs/v2.0.x/four_layers.md#Object-storage) (also called persistent storage below).\n\n\n\nData coord is in charge of handling streaming data that are continuously inserted into Milvus. When a Milvus user calls `collection.load()` to load a collection, query coord will inquire the data coord to learn which segments have been persisted in storage and their corresponding checkpoints. A checkpoint is a mark to signify that persisted segments before the checkpoints are consumed while those after the checkpoint are not.\n\nThen, the query coord outputs allocation strategy based on the information from the data coord: either by segment or by channel. The segment allocator is responsible for allocating segments in persistent storage (batch data) to different query nodes. For instance, in the image above, the segment allocator allocates segment 1 and 3 (S1, S3) to query node 1, and segment 2 and 4 (S2, S4) to query node 2. The channel allocator assigns different query nodes to watch multiple data manipulation [channels](https://milvus.io/docs/v2.0.x/data_processing.md#Data-insertion) (DMChannels) in the log broker. For instance, in the image above, the channel allocator assigns query node 1 to watch channel 1 (Ch1), and query node 2 to watch channel 2 (Ch2).\n\nWith the allocation strategy, each query node loads segment data and watch the channels accordingly. In query node 1 in the image, historical data (batch data), are loaded via the allocated S1 and S3 from persistent storage. In the meanwhile, query node 1 loads incremental data (streaming data) by subscribing to channel 1 in log broker. \n\n## Data management in query node\n\nA query node needs to manage both historical and incremental data. Historical data are stored in [sealed segments](https://milvus.io/blog/deep-dive-4-data-insertion-and-data-persistence.md#Sealed-segment) while incremental data are stored in [growing segments](https://milvus.io/blog/deep-dive-4-data-insertion-and-data-persistence.md#Growing-segment).\n\n### Historical data management\n\nThere are mainly two considerations for historical data management: load balance and query node failover.\n\n\n\nFor instance, as shown in the illustration, query node 4 has been allocated more sealed segments than the rest of the query nodes. Very likely, this will make query node 4 the bottleneck that slows down the whole query process. To solve this issue, the system needs to allocate several segments in query node 4 to other query nodes. This is called load balance.\n\n\n\nAnother possible situation is illustrated in the image above. One of the nodes, query node 4, is suddenly down. In this case, the load (segments allocated to query node 4) needs to be transferred to other working query nodes to ensure the accuracy of query results.\n\n### Incremental data management\n\nQuery node watches DMChannels to receive incremental data. Flowgraph is introduced in this process. It first filters all the data insertion messages. This is to ensure that only data in a specified partition is loaded. Each collection in Milvus has a corresponding channel, which is shared by all partitions in that collection. Therefore, a flowgraph is needed for filtering inserted data if a Milvus user only needs to load data in a certain partition. Otherwise, data in all partitions in the collection will be loaded to query node. \n\nAfter being filtered, the incremental data are inserted into growing segments, and further passed on to server time nodes.\n\n\n\nDuring data insertion, each insertion message is assigned a timestamp. In the DMChannel shown in the image above, data are are inserted in order, from left to right. The timestamp for the first insertion message is 1; the second, 2; and the third, 6. The fourth message marked in red is not an insertion message, but rather a timetick message. This is to signify that inserted data whose timestamps are smaller than this timetick are already in log broker. In other words, data inserted after this timetick message should all have timestamps whose values are bigger than this timetick. For instance, in the image above, when query node perceives that the current timetick is 5, it means all insertion messages whose timestamp value is less than 5 are all loaded to query node. \n\nThe server time node provides an updated `tsafe` value every time it receives a timetick from the insert node. `tsafe` means safety time, and all data inserted before this point of time can be queried. Take an example, if `tsafe` = 9, inserted data with timestamps smaller than 9 can all be queried. \n\n## Real-time query in Milvus\n\nReal-time query in Milvus is enabled by query messages. Query messages are inserted into log broker by proxy. Then query nodes obtain query messages by watching the query channel in log broker.\n\n### Query message\n\n\n\nA query message includes the following crucial information about a query:\n- `msgID`: Message ID, the ID of the query message assigned by the system.\n- `collectionID`: The ID of the collection to query (if specified by user).\n- `execPlan`: The execution plan is mainly used for attribute filtering in a query. \n- `service_ts`: Service timestamp will be updated together with `tsafe` mentioned above. Service timestamp signifies at which point is the service in. All data inserted before `service_ts` are available for query.\n- `travel_ts`: Travel timestamp specifies a range of time in the past. And the query will be conducted on data existing in the time period specified by `travel_ts`. \n- `guarantee_ts`: Guarantee timestamp specifies a period of time after which the query needs to be conducted. Query will only be conducted when `service_ts` \u003e `guarantee_ts`.\n\n### Real-time query\n\n\n\nWhen a query message is received, Milvus first judges if the current service time, `service_ts`, is larger than the guarantee timestamp, `guarantee_ts`, in the query message. If yes, the query will be executed. Query will be conducted in parallel on both historical data and incremental data. Since there can be an overlap of data between streaming data and batch data , an action called \"local reduce\" is needed to filter out the redundant query results. \n\nHowever, if the current service time is smaller than the guarantee timestamp in a newly inserted query message, the query message will become an unsolved message and wait to be processed till the service time becomes bigger than the guarantee timestamp.\n\nQuery results are ultimately pushed to the result channel. Proxy obtains the query results from that channel. Likewise, proxy will conduct a \"global reduce\" as well because it receives results from multiple query nodes and query results might be repetitive.\n\nTo ensure that the proxy has received all query results before returning them to the SDK, result message will also keep a record of information including searched sealed segments, searched DMChannels, and global sealed segments (all segments on all query nodes). The system can conclude that the proxy has received all query results only if both of the following conditions are met:\n\n- The union of all searched sealed segments recorded in all result messages is larger than global sealed segments,\n- All DMChannels in the collection are queried.\n\nUltimately, proxy returns the final results after \"global reduce\" to the Milvus SDK.\n\n## About the Deep Dive Series\n\nWith the [official announcement of general availability](https://milvus.io/blog/2022-1-25-annoucing-general-availability-of-milvus-2-0.md) of Milvus 2.0, we orchestrated this Milvus Deep Dive blog series to provide an in-depth interpretation of the Milvus architecture and source code. Topics covered in this blog series include:\n\n- [Milvus architecture overview](https://milvus.io/blog/deep-dive-1-milvus-architecture-overview.md)\n- [APIs and Python SDKs](https://milvus.io/blog/deep-dive-2-milvus-sdk-and-api.md)\n- [Data processing](https://milvus.io/blog/deep-dive-3-data-processing.md)\n- [Data management](https://milvus.io/blog/deep-dive-4-data-insertion-and-data-persistence.md)\n- [Real-time query](https://milvus.io/blog/deep-dive-5-real-time-query.md)\n- [Scalar execution engine](https://milvus.io/blog/deep-dive-7-query-expression.md)\n- [QA system](https://milvus.io/blog/deep-dive-6-oss-qa.md)\n- [Vector execution engine](https://milvus.io/blog/deep-dive-8-knowhere.md)\n","title":"Using the Milvus Vector Database for Real-Time Query","metaData":{}},{"id":"deep-dive-4-data-insertion-and-data-persistence.md","author":"Bingyi Sun","desc":"Learn about the mechanism behind data insertion and data persistence in Milvus vector database.","tags":["Engineering"],"canonicalUrl":"https://milvus.io/blog/deep-dive-4-data-insertion-and-data-persistence.md","date":"2022-04-06T00:00:00.000Z","cover":"https://assets.zilliz.com/Deep_Dive_4_812021d715.png","href":"/blog/deep-dive-4-data-insertion-and-data-persistence.md","content":"\n\n\n\u003e This article is written by [Bingyi Sun](https://github.com/sunby) and transcreated by [Angela Ni](https://www.linkedin.com/in/yiyun-n-2aa713163/).\n\nIn the previous post in the Deep Dive series, we have introduced [how data is processed in Milvus](https://milvus.io/blog/deep-dive-3-data-processing.md), the world's most advanced vector database. In this article, we will continue to examine the components involved in data insertion, illustrate the data model in detail, and explain how data persistence is achieved in Milvus.\n\nJump to:\n- [Milvus architecture recap](#Milvus-architecture-recap)\n- [The portal of data insertion requests](#The-portal-of-data-insertion-requests)\n- [Data coord and data node](#Data-coord-and-data-node)\n- [Root coord and Time Tick](#Root-coord-and-Time-Tick)\n- [Data organization: collection, partition, shard (channel), segment](#Data-organization-collection-partition-shard-channel-segment)\n- [Data allocation: when and how](#Data-allocation-when-and-how)\n- [Binlog file structure and data persistence](#Binlog-file-structure-and-data-persistence)\n\n## Milvus architecture recap\n\n\n\nSDK sends data requests to proxy, the portal, via load balancer. Then the proxy interacts with coordinator service to write DDL (data definition language) and DML (data manipulation language) requests into message storage.\n\nWorker nodes, including query node, data node, and index node, consume the requests from message storage. More specifically, the query node is in charge of data query; the data node is responsible for data insertion and data persistence; and the index node mainly deals with index building and query acceleration. \n\nThe bottom layer is object storage, which mainly leverages MinIO, S3, and AzureBlob for storing logs, delta binlogs, and index files.\n\n## The portal of data insertion requests\n\n\n\nProxy serves as a portal of data insertion requests. \n\n1. Initially, proxy accepts data insertion requests from SDKs, and allocates those requests into several buckets using hash algorithm.\n2. Then the proxy requests data coord to assign segments, the smallest unit in Milvus for data storage. \n3. Afterwards, the proxy inserts information of the requested segments into message store so that these information will not be lost. \n\n## Data coord and data node\n\nThe main function of data coord is to manage channel and segment allocation while the main function of data node is to consume and persist inserted data.\n\n\n\n### Function\n\nData coord serves in the following aspects:\n\n- **Allocate segment space**\nData coord allocates space in growing segments to the proxy so that the proxy can use free space in segments to insert data.\n\n- **Record segment allocation and the expiry time of the allocated space in the segment**\nThe space within each segment allocated by the data coord is not permanent, therefore, the data coord also needs to keep a record of the expiry time of each segment allocation.\n\n- **Automatically flush segment data**\nIf the segment is full, the data coord automatically triggers data flush.\n\n- **Allocate channels to data nodes**\nA collection can have multiple [vchannels](https://milvus.io/docs/v2.0.x/glossary.md#VChannel). Data coord determines which vchannels are consumed by which data nodes.\n\nData node serves in the following aspects:\n\n- **Consume data**\nData node consumes data from the channels allocated by data coord and creates a sequence for the data.\n\n- **Data persistence**\nCache inserted data in memory and auto-flush those inserted data to disk when data volume reach a certain threshold.\n\n### Workflow\n\n\n\nAs shown in the image above, the collection has four vchannels (V1, V2, V3, and V4) and there are two data nodes. It is very likely that data coord will assign one data node to consume data from V1 and V2, and the other data node from V3 and V4. One single vchannel cannot be assigned to multiple data nodes and this is to prevent repetition of data consumption, which will otherwise cause the same batch of data being inserted into the same segment repetitively.\n \n## Root coord and Time Tick\n\nRoot coord manages TSO (timestamp Oracle), and publishes time tick messages globally. Each data insertion request has a timestamp assigned by root coord. Time Tick is the cornerstone of Milvus which acts like a clock in Milvus and signifies at which point of time is the Milvus system in.\n\nWhen data are written in Milvus, each data insertion request carries a timestamp. During data consumption, each time data node consumes data whose timestamps are within a certain range. \n\n\n\nThe image above is the process of data insertion. The value of the timestamps are represented by numbers 1,2,6,5,7,8. The data are written into the system by two proxies: p1 and p2. During data consumption, if the current time of the Time Tick is 5, data nodes can only read data 1 and 2. Then during the second read, if the current time of the Time Tick becomes 9, data 6,7,8 can be read by data node.\n\n## Data organization: collection, partition, shard (channel), segment\n\n\n\nRead this [article](https://milvus.io/blog/deep-dive-1-milvus-architecture-overview.md#Data-Model) first to understand the data model in Milvus and the concepts of collection, shard, partition, and segment.\n\nIn summary, the largest data unit in Milvus is a collection which can be likened to a table in a relational database. A collection can have multiple shards (each corresponding to a channel) and multiple partitions within each shard. As shown in the illustration above, channels (shards) are the vertical bars while the partitions are the horizontal ones. At each intersection is the concept of segment, the smallest unit for data allocation. In Milvus, indexes are built on segments. During a query, the Milvus system also balances query loads in different query nodes and this process is conducted based on the unit of segments. Segments contain several [binlogs](https://milvus.io/docs/v2.0.x/glossary.md#Binlog), and when the segment data are consumed, a binlog file will be generated.\n\n### Segment\n\nThere are three types of segments with different status in Milvus: growing, sealed, and flushed segment.\n\n#### Growing segment\n\nA growing segment is a newly created segment that can be allocated to the proxy for data insertion. The internal space of a segment can be used, allocated, or free.\n\n\n\n- Used: this part of space of a growing segment has been consumed by data node.\n- Allocated: this part of space of a growing segment has been requested by the proxy and allocated by data coord. Allocated space will expire after a certain period time.\n- Free: this part of space of a growing segment has not been used. The value of free space equals the overall space of the segment subtracted by the value of used and allocated space. So the free space of a segment increases as the allocated space expires.\n\n#### Sealed segment\n\nA sealed segment is a closed segment that can no longer be allocated to the proxy for data insertion.\n\n\n\nA growing segment is sealed in the following circumstances: \n\n- If the used space in a growing segment reaches 75% of the total space, the segment will be sealed.\n- Flush() is manually called by a Milvus user to persist all data in a collection.\n- Growing segments that are not sealed after a long period of time will be sealed as too many growing segments cause data nodes to over-consume memory.\n\n#### Flushed segment \n\nA flushed segment is a segment that has already been written into disk. Flush refers to storing segment data to object storage for the sake of data persistence. A segment can only be flushed when the allocated space in a sealed segment expires. When flushed, the sealed segment turns into a flushed segment.\n\n\n\n### Channel\n\nA channel is allocated :\n\n- When data node starts or shuts down; or\n- When segment space allocated is requested by proxy.\n\nThen there are several strategies of channel allocation. Milvus supports 2 of the strategies:\n\n1. Consistent hashing\n\n\n\nThe default strategy in Milvus. This strategy leverages the hashing technique to assign each channel a position on the ring, then searches in a clock-wise direction to find the nearest data node to a channel. Thus, in the illustration above, channel 1 is allocated to data node 2, while channel 2 is allocated to data node 3.\n\nHowever, one problem with this strategy is that the increase or decrease in the number of data nodes (eg. A new data node starts or a data node suddenly shuts down) can affect the process of channel allocation. To solve this issue, data coord monitors the status of data nodes via etcd so that data coord can be immediately notified if there is any change in the status of data nodes. Then data coord further determines to which data node to allocate the channels properly.\n\n2. Load balancing \n\nThe second strategy is to allocate channels of the same collection to different data nodes, ensuring the channels are evenly allocated. The purpose of this strategy is to achieve load balance.\n\n## Data allocation: when and how\n\n\n\nThe process of data allocation starts from the client. It first sends data insertion requests with a timestamp `t1` to proxy. Then the proxy sends a request to data coord for segment allocation. \n\nUpon receiving the segment allocation request, the data coord checks segment status and allocates segment. If the current space of the created segments is sufficient for the newly inserted rows of data, the data coord allocates those created segments. However, if the space available in current segments is not sufficient, the data coord will allocate a new segment. The data coord can return one or more segments upon each request. In the meantime, the data coord also saves the allocated segment in meta server for data persistence. \n\nSubsequently, the data coord returns the information of the allocated segment (including segment ID, number of rows, expiry time `t2`, etc.) to the proxy. The proxy sends such information of the allocated segment to message store so that these information are properly recorded. Note that the value of `t1` must be smaller than that of `t2`. The default value of `t2` is 2,000 millisecond and it can be changed by configuring the parameter `segment.assignmentExpiration` in the `data_coord.yaml` file. \n\n## Binlog file structure and data persistence\n\n\n\nData node subscribes to the message store because data insertion requests are kept in the message store and the data nodes can thus consume insert messages. The data nodes first place insert requests in an insert buffer, and as the requests accumulate, they will be flushed to object storage after reaching a threshold. \n\n### Binlog file structure\n\n\n\nThe binlog file structure in Milvus is similar to that in MySQL. Binlog is used to serve two functions: data recovery and index building. \n\nA binlog contains many [events](https://github.com/milvus-io/milvus/blob/master/docs/developer_guides/chap08_binlog.md#event-format). Each event has an event header and event data. \n\nMetadata including binlog creation time, write node ID, event length, and NextPosition (offset of the next event), etc. are written in the event header. \n\nEvent data can be divided into two parts: fixed and variable.\n\n\n\nThe fixed part in the event data of an `INSERT_EVENT` contains `StartTimestamp`, `EndTimestamp`, and `reserved`.\n\nThe variable part in fact stores inserted data. The insert data are sequenced into the format of parquet and stored in this file.\n\n ### Data persistence\n\nIf there are multiple columns in schema, Milvus will store binlogs in columns. \n\n\n\nAs illustrated in the image above, the first column is primary key binlog. The second one is timestamp column. The rest are the columns defined in schema. The file path of binlogs in MinIO is also indicated in the image above. \n\n## About the Deep Dive Series\n\nWith the [official announcement of general availability](https://milvus.io/blog/2022-1-25-annoucing-general-availability-of-milvus-2-0.md) of Milvus 2.0, we orchestrated this Milvus Deep Dive blog series to provide an in-depth interpretation of the Milvus architecture and source code. Topics covered in this blog series include:\n\n- [Milvus architecture overview](https://milvus.io/blog/deep-dive-1-milvus-architecture-overview.md)\n- [APIs and Python SDKs](https://milvus.io/blog/deep-dive-2-milvus-sdk-and-api.md)\n- [Data processing](https://milvus.io/blog/deep-dive-3-data-processing.md)\n- [Data management](https://milvus.io/blog/deep-dive-4-data-insertion-and-data-persistence.md)\n- [Real-time query](https://milvus.io/blog/deep-dive-5-real-time-query.md)\n- [Scalar execution engine](https://milvus.io/blog/deep-dive-7-query-expression.md)\n- [QA system](https://milvus.io/blog/deep-dive-6-oss-qa.md)\n- [Vector execution engine](https://milvus.io/blog/deep-dive-8-knowhere.md)\n","title":"Data Insertion and Data Persistence in a Vector Database","metaData":{}},{"id":"deep-dive-3-data-processing.md","author":"Zhenshan Cao","desc":"Milvus provides a data management infrastructure essential for production AI applications. This article unveils the intricacies of data processing inside.","tags":["Engineering"],"canonicalUrl":"https://milvus.io/blog/deep-dive-3-data-processing.md","date":"2022-03-28T00:00:00.000Z","cover":"https://assets.zilliz.com/How_Is_Data_Processed_in_a_Vector_Database_9fb236bc01.png","href":"/blog/deep-dive-3-data-processing.md","content":"\n\n\n\u003e This article is written by [Zhenshan Cao](https://github.com/czs007) and transcreated by [Angela Ni](https://www.linkedin.com/in/yiyun-n-2aa713163/).\n\nIn the previous two posts in this blog series, we have already covered the [system architecture](https://milvus.io/blog/deep-dive-1-milvus-architecture-overview.md) of Milvus, the world's most advanced vector database, and its [Python SDK and API](https://milvus.io/blog/deep-dive-2-milvus-sdk-and-api.md). \n\nThis post mainly aims to help you understand how data is processed in Milvus by going deep into the Milvus system and examining the interaction between the data processing components.\n\n*Some useful resources before getting started are listed below. We recommend reading them first to better understand the topic in this post.*\n\n- [Deep dive into the Milvus architecture](https://milvus.io/blog/deep-dive-1-milvus-architecture-overview.md)\n- [Milvus data model](https://milvus.io/blog/deep-dive-1-milvus-architecture-overview.md#Data-Model)\n- [The role and function of each Milvus component](https://milvus.io/docs/v2.0.x/four_layers.md)\n- [Data processing in Milvus](https://milvus.io/docs/v2.0.x/data_processing.md)\n\n\n## MsgStream interface\n\n[MsgStream interface](https://github.com/milvus-io/milvus/blob/ca129d4308cc7221bb900b3722dea9b256e514f9/docs/developer_guides/chap04_message_stream.md) is crucial to data processing in Milvus. When `Start()` is called, the coroutine in the background writes data into the [log broker](https://milvus.io/blog/deep-dive-1-milvus-architecture-overview.md#Log-as-data) or reads data from there. When `Close()` is called, the coroutine stops.\n\n\n\nThe MsgStream can serve as a producer and a consumer. The `AsProducer(channels []string)` interface defines MsgStream as a producer while the `AsConsumer(channels []string, subNamestring)`defines it as a consumer. The parameter `channels` is shared in both interfaces and it is used to define which (physical) channels to writes data into or read data from.\n\n\u003e The number of shards in a collection can be specified when a collection is created. Each shard corresponds to a [virtual channel (vchannel)](https://milvus.io/docs/v2.0.x/glossary.md#VChannel). Therefore, a collection can have multiple vchannels. Milvus assigns each vchannel in the log broker a [physical channel (pchannel)](https://milvus.io/docs/v2.0.x/glossary.md#PChannel). \n\n\n\n\n`Produce()` in the MsgStream interface in charge of writing data into the pchannels in log broker. Data can be written in two ways:\n- Single write: entities are written into different shards (vchannel) by the hash values of primary keys. Then these entities flow into corresponding pchannels in the log broker.\n- Broadcast write: entities are written into all of the pchannels specified by the parameter `channels`.\n\n`Consume()` is a type of blocking API. If there is no data available in the specified pchannel, the coroutine will be blocked when `Consume()` is called in MsgStream interface. On the other hand, `Chan()` is a non-blocking API, which means that the coroutine reads and processes data only if there is existing data in the specified pchannel. Otherwise, the coroutine can process other tasks and will not be blocked when there is no data available.\n\n`Seek()` is method for failure recovery. When a new node is started, the data consumption record can be obtained and data consumption can resume from where it was interrupted by calling `Seek()`.\n\n## Write data\n\nThe data written into different vchannels (shards) can either be insert message or delete message. These vchannels can also be called DmChannels (data manipulation channels).\n\nDifferent collections may share the same pchannels in the log broker. One collection can have multiple shards and hence multiple corresponding vchannels. The entities in the same collection consequently flow into multiple corresponding pchannels in the log broker. As a result, the benefit of sharing pchannels is an increased volume of throughput enabled by high concurrency of the log broker. \n\nWhen a collection is created, not only the number of shards is specified, but also the mapping between vchannels and pchannels in the log broker is decided.\n\n \n\nAs shown in the illustration above, in the write path, proxies write data into the log broker via the `AsProducer()` interface of the MsgStream. Then data nodes consume the data, then convert and store the consumed data in object storage. The storage path is a type of meta information which will be recorded in etcd by data coordinators.\n\n### Flowgraph\n\nSince different collections may share the same pchannels in the log broker, when consuming data, data nodes or query nodes need to judge to which collection the data in a pchannel belongs. To solve this problem, we introduced flowgraph in Milvus. It is mainly in charge of filtering data in a shared pchannel by collection IDs. So, we can say that each flowgraph handles the data stream in a corresponding shard (vchannel) in a collection.\n\n \n\n### MsgStream creation\n\nWhen writing data, MsgStream object is created in the following two scenarios:\n- When the proxy receives a data insertion request, it first tries to obtain the mapping between vchannels and pchannels via root coordinator (root coord). Then the proxy creates an MsgStream object.\n\n \n\n- When data node starts and reads the meta information of channels in etcd, the MsgStream object is created. \n\n \n\n## Read data\n\n\n\nThe general workflow of reading data is illustrated in the image above. Query requests are broadcast via DqRequestChannel to query nodes. The query nodes execute the query tasks in parallel. The query results from query nodes go through gRPC and proxy aggregate the results and returns them to the client.\n\nTo take a closer look at the data reading process, we can see that the proxy writes query requests into DqRequestChannel. Query nodes then consume message by subscribing to DqRequestChannel. Each message in the DqRequestChannel is broadcast so that all subscribed query nodes can receive the message.\n\nWhen query nodes receive query requests, they conduct a local query on both batch data stored in sealed segments and streaming data that are dynamically inserted into Milvus and stored in growing segments. Afterwards, query nodes need to aggregate the query results in both [sealed and growing segments](https://milvus.io/docs/v2.0.x/glossary.md#Segment). These aggregated results are passed on to proxy via gRPC.\n\nThe proxy collects all the results from multiple query nodes and then aggregate them to obtain the final results. Then the proxy returns the final query results to the client. Since each query request and its corresponding query results are labelled by the same unique requestID, proxy can figure out which query results correspond to which query request.\n\n### Flowgraph\n\n\n\nSimilar to the write path, flowgraphs are also introduced in the read path. Milvus implements the unified Lambda architecture, which integrates the processing of the incremental and historical data. Therefore, query nodes need to obtain real-time streaming data as well. Similarly, flowgraphs in read path filters and differentiates data from different collections.\n\n### MsgStream creation\n\n\n\nWhen reading data, the MsgStream object is created in the following scenario:\n- In Milvus, data cannot be read unless they are loaded. When the proxy receives a data load request, it sends the request to query coordinator which decides the way of assigning shards to different query nodes. The assigning information (i.e. The names of vchannels and the mapping between vchannels and their corresponding pchannels) is sent to query nodes via method call or RPC (remote procedure call). Subsequently, the query nodes create corresponding MsgStream objects to consume data. \n\n## DDL operations\nDDL stands for data definition language. DDL operations on metadata can be categorized into write requests and read requests. However, these two types of requests are treated equally during metadata processing. \n\nRead requests on metadata include:\n- Query collection schema\n- Query indexing information\nAnd more\n\nWrite requests include:\n- Create a collection\n- Drop a collection\n- Build an index\n- Drop an index\nAnd more\n\nDDL requests are sent to the proxy from the client, and the proxy further passes on these requests in the received order to the root coord which assigns a timestamp for each DDL request and conducts dynamic checks on the requests. Proxy handles each request in a serial manner, meaning one DDL request at a time. The proxy will not process the next request until it completes processing the previous request and receive results from the root coord. \n\n\n\nAs shown in the illustration above, there are `K` DDL requests in the Root coord task queue. The DDL requests in the task queue are arranged in the order they are received by the root coord. So, `ddl1` is the first sent to root coord, and `ddlK` is the last one in this batch. The root coord processes the requests one by one in the time order.\n\nIn a distributed system, the communication between the proxies and the root coord is enabled by gRPC. The root coord keeps a record of the maximum timestamp value of the executed tasks to ensure that all DDL requests are processed in time order.\n\nSuppose there are two independent proxies, proxy 1 and proxy 2. They both send DDL requests to the same root coord. However, one problem is that earlier requests are not necessarily sent to the root coord before those requests received by another proxy later. For instance, in the image above, when `DDL_K-1` is sent to the root coord from proxy 1, `DDL_K` from proxy 2 has already been accepted and executed by the root coord. As recorded by the root coord, the maximum timestamp value of the executed tasks at this point is `K`. So in order not to interrupt the time order, the request `DDL_K-1` will be rejected by the root coord's task queue . However, if proxy 2 sends the request `DDL_K+5` to the root coord at this point, the request will be accepted to the task queue and will be executed later according to its timestamp value.\n\n## Indexing\n\n### Building an index\n\nUpon receiving index building requests from the client, the proxy first carries out static checks on the requests and sends them to the root coord. Then the root coord persists these index building requests into meta storage (etcd) and sends the requests to the index coordinator (index coord).\n\n\n\nAs illustrated above, when the index coord receives index building requests from the root coord, it first persists the task in etcd for meta store. The initial status of the index building task is `Unissued`. The index coord maintains a record of the task load of each index node, and sends to inbound tasks to a less loaded index node. Upon completion of the task, the index node writes the status of the task, either `Finished` or `Failed` into meta storage, which is etcd in Milvus. Then the index coord will understand if the index building task succeeds or fails by looking up in etcd. If the task fails due to limited system resources or dropout of the index node, the index coord will re-trigger the whole process and assign the same task to another index node.\n\n### Dropping an index\n\nIn addition, the index coord is also in charge of the requests of dropping indexes. \n\n\n\nWhen the root coord receives a request of dropping an index from the client, it first marks the index as \"dropped\", and returns the result to the client while notifying the index coord. Then the index coord filters all indexing tasks with the `IndexID` and those tasks matching the condition are dropped.\n\nThe background coroutine of the index coord will gradually delete all indexing tasks marked as \"dropped\" from object storage (MinIO and S3). This process involves the recycleIndexFiles interface. When all corresponding index files are deleted, the meta information of the deleted indexing tasks are removed from meta storage (etcd).\n\n## About the Deep Dive Series\n\nWith the [official announcement of general availability](https://milvus.io/blog/2022-1-25-annoucing-general-availability-of-milvus-2-0.md) of Milvus 2.0, we orchestrated this Milvus Deep Dive blog series to provide an in-depth interpretation of the Milvus architecture and source code. Topics covered in this blog series include:\n\n- [Milvus architecture overview](https://milvus.io/blog/deep-dive-1-milvus-architecture-overview.md)\n- [APIs and Python SDKs](https://milvus.io/blog/deep-dive-2-milvus-sdk-and-api.md)\n- [Data processing](https://milvus.io/blog/deep-dive-3-data-processing.md)\n- [Data management](https://milvus.io/blog/deep-dive-4-data-insertion-and-data-persistence.md)\n- [Real-time query](https://milvus.io/blog/deep-dive-5-real-time-query.md)\n- [Scalar execution engine](https://milvus.io/blog/deep-dive-7-query-expression.md)\n- [QA system](https://milvus.io/blog/deep-dive-6-oss-qa.md)\n- [Vector execution engine](https://milvus.io/blog/deep-dive-8-knowhere.md)\n\n\n\n","title":"How Is Data Processed in a Vector Database?","metaData":{}},{"id":"deep-dive-2-milvus-sdk-and-api.md","author":"Xuan Yang","desc":"Learn how SDKs interact with Milvus and why ORM-style API helps you better manage Milvus.","tags":["Engineering"],"canonicalUrl":"https://milvus.io/blog/deep-dive-2-milvus-sdk-and-api.md","date":"2022-03-21T00:00:00.000Z","cover":"https://assets.zilliz.com/20220322_175856_e8e7bea7dc.png","href":"/blog/deep-dive-2-milvus-sdk-and-api.md","content":"\n\n\nBy [Xuan Yang](https://github.com/XuanYang-cn)\n\n## Background\n\nThe following illustration depicts the interaction between SDKs and Milvus through gRPC. Imagine that Milvus is a black box. Protocol Buffers are used to define the interfaces of the server, and the structure of the information they carry. Therefore, all operations in the black box Milvus is defined by Protocol API.\n\n\n\n## Milvus Protocol API\n\nMilvus Protocol API consists of `milvus.proto`, `common.proto`, and `schema.proto`, which are Protocol Buffers files suffixed with `.proto`. To ensure proper operation, SDKs must interact with Milvus with these Protocol Buffers files.\n\n### milvus.proto\n\n`milvus.proto` is the vital component of Milvus Protocol API because it defines the `MilvusService`, which further defines all RPC interfaces of Milvus.\n\nThe following code sample shows the interface `CreatePartitionRequest`. It has two major string-type parameters `collection_name` and `partition_name`, based on which you can start a partition creation request.\n\n\n\nCheck an example of Protocol in [PyMilvus GitHub Repository](https://github.com/milvus-io/milvus-proto/blob/44f59db22b27cc55e4168c8e53b6e781c010a713/proto/milvus.proto) on line 19.\n\n\n\nYou can find the definition of `CreatePartitionRequest` here.\n\n\n\nContributors who wish to develop a feature of Milvus or an SDK in a different programming language are welcome to find all interfaces Milvus offers via RPC.\n\n### common.proto\n\n`common.proto` defines the common types of information, including `ErrorCode`, and `Status`. \n\n\n\n### schema.proto\n\n`schema.proto` defines the schema in the parameters. The following code sample is an example of `CollectionSchema`.\n\n\n\n`milvus.proto`, `common.proto`, and `schema.proto` together constitutes the API of Milvus, representing all operations that can be called via RPC.\n\nIf you dig into the source code and observe carefully, you will find that when interfaces like `create_index` are called, they actually call multiple RPC interfaces such as `describe_collection` and `describe_index`. Many of the outward interface of Milvus is a combination of multiple RPC interfaces.\n\nHaving understood the behaviors of RPC, you can then develop new features for Milvus through combination. You are more than welcome to use your imagination and creativeness and contribute to Milvus community.\n\n## PyMilvus 2.0\n\n### Object-relational mapping (ORM)\n\nTo put it in a nutshell, Object-relational mapping (ORM) refers to that when you operate on a local object, such operations will affect the corresponding object on server. PyMilvus ORM-style API features the following characteristics:\n\n1. It operates directly on objects.\n2. It isolates service logic and data access details.\n3. It hides the complexity of implementation, and you can run the same scripts across different Milvus instances regardless of their deployment approaches or implementation.\n\n### ORM-style API\n\nOne of the essence of ORM-style API lies in the control of Milvus connection. For example, you can specify aliases for multiple Milvus servers, and connect to or disconnect from them merely with their aliases. You can even delete the local server address, and control certain objects via specific connection precisely.\n\n\n\nAnother feature of ORM-style API is that, after abstraction, all operations can be performed directly on objects, including collection, partition, and index.\n\nYou can abstract a collection object by getting an existing one or creating a new one. You can also assign a Milvus connection to specific objects using connection alias, so that you can operate on these objects locally.\n\nTo create a partition object, you can either create it with its parent collection object, or you can do it just like when you create a collection object. These methods can be employed on an index object as well.\n\nIn the case that these partition or index objects exist, you can get them through their parent collection object.\n\n## About the Deep Dive Series\n\nWith the [official announcement of general availability](https://milvus.io/blog/2022-1-25-annoucing-general-availability-of-milvus-2-0.md) of Milvus 2.0, we orchestrated this Milvus Deep Dive blog series to provide an in-depth interpretation of the Milvus architecture and source code. Topics covered in this blog series include:\n\n- [Milvus architecture overview](https://milvus.io/blog/deep-dive-1-milvus-architecture-overview.md)\n- [APIs and Python SDKs](https://milvus.io/blog/deep-dive-2-milvus-sdk-and-api.md)\n- [Data processing](https://milvus.io/blog/deep-dive-3-data-processing.md)\n- [Data management](https://milvus.io/blog/deep-dive-4-data-insertion-and-data-persistence.md)\n- [Real-time query](https://milvus.io/blog/deep-dive-5-real-time-query.md)\n- [Scalar execution engine](https://milvus.io/blog/deep-dive-7-query-expression.md)\n- [QA system](https://milvus.io/blog/deep-dive-6-oss-qa.md)\n- [Vector execution engine](https://milvus.io/blog/deep-dive-8-knowhere.md)\n","title":"An Introduction to Milvus Python SDK and API","metaData":{}},{"id":"deep-dive-1-milvus-architecture-overview.md","author":"Xiaofan Luan","desc":"The first one in a blog series to take a closer look at the thought process and design principles behind the building of the most popular open-source vector database.","tags":["Engineering"],"canonicalUrl":"https://milvus.io/blog/deep-dive-1-milvus-architecture-overview.md","date":"2022-03-14T00:00:00.000Z","cover":"https://assets.zilliz.com/20220705_102717_dd4124dee3.png","href":"/blog/deep-dive-1-milvus-architecture-overview.md","content":"\n\n\n\u003e This article is written by Xiaofan Luan and transcreated by Angela Ni and Claire Yu.\n\nAccording to [statistics](https://mitsloan.mit.edu/ideas-made-to-matter/tapping-power-unstructured-data), about 80%-90% of the world's data is unstructured. Fueled by the rapid growth of the Internet, an explosion of unstructured data is expected in the coming years. Consequently, companies are in urgent need of a powerful database that can help them better handle and understand such kind of data. However, developing a database is always easier said than done. This article aims to share the thinking process and design principles of building Milvus, an open-source, cloud-native vector database for scalable similarity search. This article also explains the Milvus architecture in detail.\n\nJump to:\n- [Unstructured data requires a complete basic software stack](#Unstructured-data-requires-a-complete-basic-software-stack)\n - [Vectors and scalars](#Vectors-and-scalars)\n - [From vector search engine to vector database](#From-vector-search-engine-to-vector-database)\n - [A cloud-native first approach](#A-cloud-native-first-approach)\n- [The design principles of Milvus 2.0](#The-design-principles-of-Milvus-20)\n - [Log as data](#Log-as-data)\n - [Duality of table and log](#Duality-of-table-and-log)\n - [Log persistency](#Log-persistency)\n- [Building a vector database for scalable similarity search](#Building-a-vector-database-for-scalable-similarity-search)\n - [Standalone and cluster](#Standalone-and-cluster)\n - [A bare-bones skeleton of the Milvus architecture](#A-bare-bones-skeleton-of-the-Milvus-architecture)\n - [Data model](#Data-Model)\n\n## Unstructured data requires a complete basic software stack \n\nAs the Internet grew and evolved, unstructured data became more and more common, including emails, papers, IoT sensor data, Facebook photos, protein structures, and much more. In order for computers to understand and process unstructured data, these are converted into vectors using [embedding techniques](https://zilliz.com/learn/embedding-generation). \n\nMilvus stores and indexes these vectors, and analyzes the correlation between two vectors by calculating their similarity distance. If the two embedding vectors are very similar, it means that the original data sources are similar as well.\n\n\n\n### Vectors and scalars\n\nA scalar is a quantity that is described only in one measurement - magnitude. A scalar can be represented as a number. For instance, a car is traveling at the speed of 80 km/h. Here, the speed (80km/h) is a scalar. Meanwhile, a vector is a quantity that is described in at least two measurements - magnitude and direction. If a car is traveling towards west at the speed of 80 km/h, here the velocity (80 km/h west) is a vector. The image below is an example of common scalars and vectors.\n\n\n\nSince most of the important data have more than one attribute, we can understand these data better if we convert them into vectors. One common way for us to manipulate vector data is to calculate the distance between vectors using [metrics](https://milvus.io/docs/v2.0.x/metric.md) such as Euclidean distance, inner product, Tanimoto distance, Hamming distance, etc. The closer the distance, the more similar the vectors are. To query a massive vector dataset efficiently, we can organize vector data by building indexes on them. After the dataset is indexed, queries can be routed to clusters, or subsets of data, that are most likely to contain vectors similar to an input query. \n\nTo learn more about the indexes, refer to [Vector Index](https://milvus.io/docs/v2.0.x/index.md).\n\n### From vector search engine to vector database\n\nFrom the very beginning, Milvus 2.0 is designed to serve not only as a search engine, but more importantly, as a powerful vector database.\n\nOne way to help you understand the difference here is by drawing an analogy between [InnoDB](https://dev.mysql.com/doc/refman/5.7/en/innodb-introduction.html) and [MySQL](https://www.mysql.com/), or [Lucene](https://lucene.apache.org/) and [Elasticsearch](https://www.elastic.co/).\n\nJust like MySQL and Elasticsearch, Milvus is also built on top of open-source libraries such as [Faiss](https://github.com/facebookresearch/faiss), [HNSW](https://github.com/nmslib/hnswlib), [Annoy](https://github.com/spotify/annoy), which focus on providing search functionalities and ensuring search performance. However, it would be unfair to degrade Milvus to merely a layer atop Faiss as it stores, retrieves, analyzes vectors, and, just as with any other database, also provides a standard interface for CRUD operations. In addition, Milvus also boasts features including:\n- Sharding and partitioning\n- Replication\n- Disaster recovery\n- Load balance\n- Query parser or optimizer\n\n\n\nFor a more comprehensive understanding of what a vector database is, read the blog [here](https://zilliz.com/learn/what-is-vector-database).\n\n### A cloud-native first approach\n\n\n\n#### From shared nothing, to shared storage, then to shared something\n\nTraditional databases used to adopt a \"shared nothing\" architecture in which nodes in the distributed systems are independent but connected by a network. No memory or storage are shared among the nodes. However, [Snowflake](https://docs.snowflake.com/en/user-guide/intro-key-concepts.html) revolutionized the industry by introducing a \"shared storage\" architecture in which compute (query processing) is separated from storage (database storage). With a shared storage architecture, databases can achieve greater availability, scalability, and a reduction of data duplication. Inspired by Snowflake, many companies started to leverage cloud-based infrastructure for data persistence while using local storage for caching. This type of database architecture is called \"shared something\" and has become the mainstream architecture in most applications today. \n\nApart from the \"shared something\" architecture, Milvus supports flexible scaling of each component by using Kubernetes to manage its execution engine and separating read, write and other services with microservices.\n\n#### Database as a service (DBaaS)\n\nDatabase as a service is a hot trend as many users not only care about regular database functionalities but also yearn for more varied services. This means that apart from the traditional CRUD operations, our database has to enrich the type of services it can provide, such as database management, data transport, charging, visualization, etc.\n\n#### Synergy with the broader open-source ecosystem\n\nAnother trend in database development is leveraging the synergy between the database and other cloud-native infrastructure. In the case of Milvus, it relies on some open-source systems. For instance, Milvus uses [etcd](https://etcd.io/) for storing metadata. It also adopts message queue, a type of asynchronous service-to-service communication used in microservices architecture, which can help export incremental data.\n\nIn the future, we hope to build Milvus on top of AI infrastructures such as [Spark](https://spark.apache.org/) or [Tensorflow](https://www.tensorflow.org/), and integrate Milvus with streaming engines so that we can better support unified stream and batch processing to meet the various needs of Milvus users.\n\n## The design principles of Milvus 2.0\n\nAs our next-generation cloud-native vector database, Milvus 2.0 is built around the following three principles.\n\n### Log as data\n\nA log in a database serially records all the changes made to data. As shown in the figure below, from left to right are \"old data\" and \"new data\". And the logs are in time order. Milvus has a global timer mechanism assigning one globally unique and auto-incremental timestamp.\n\n\n\nIn Milvus 2.0, the log broker serves as the system's backbone: all data insert and update operations must go through the log broker, and worker nodes execute CRUD operations by subscribing to and consuming logs.\n\n### Duality of table and log\n\nBoth the table and the log are data, and they are but just two different forms. Tables are bounded data while logs are unbounded. Logs can be converted into tables. In the case of Milvus, it aggregates logs using a processing window from TimeTick. Based on log sequence, multiple logs are aggregated into one small file called log snapshot. Then these log snapshots are combined to form a segment, which can be used individually for load balance.\n\n### Log persistency\n\nLog persistency is one of the tricky issues faced by many databases. The storage of logs in a distributed system usually depends on replication algorithms.\n\nUnlike databases such as [Aurora](https://aws.amazon.com/rds/aurora/), [HBase](https://hbase.apache.org/), [Cockroach DB](https://www.cockroachlabs.com/), and [TiDB](https://en.pingcap.com/), Milvus takes a ground-breaking approach and introduces a publish-subscribe (pub/sub) system for log storage and persistency. A pub/sub system is analogous to the message queue in [Kafka](https://kafka.apache.org/) or [Pulsar](https://pulsar.apache.org/). All nodes within the system can consume the logs. In Milvus, this kind of system is called a log broker. Thanks to the log broker, logs are decoupled from the server, ensuring that Milvus is itself stateless and better positioned to quickly recover from system failure. \n\n\n\n## Building a vector database for scalable similarity search\n\nBuilt on top of popular vector search libraries including Faiss, ANNOY, HNSW, and more, Milvus was designed for similarity search on dense vector datasets containing millions, billions, or even trillions of vectors. \n\n### Standalone and cluster\n\nMilvus offers two ways of deployment - standalone or cluster. In Milvus standalone, since all nodes are deployed together, we can see Milvus as one single process. Currently, Milvus standalone relies on MinIO and etcd for data persistence and metadata storage. In future releases, we hope to eliminate these two third-party dependencies to ensure the simplicity of the Milvus system. Milvus cluster includes eight microservice components and three third-party dependencies: MinIO, etcd, and Pulsar. Pulsar serves as the log broker and provides log pub/sub services.\n\n\n\n### A bare-bones skeleton of the Milvus architecture\n\nMilvus separates data flow from control flow, and is divided into four layers that are independent in terms of scalability and disaster recovery.\n\n\n\n#### Access layer\n\nThe access layer acts as the system's face, exposing the endpoint of the client connection to the outside world. It is responsible for processing client connections, carrying out static verification, basic dynamic checks for user requests, forwarding requests, and gathering and returning results to the client. The proxy itself is stateless and provides unified access addresses and services to the outside world through load balancing components (Nginx, Kubernetess Ingress, NodePort, and LVS). Milvus uses a massively parallel processing (MPP) architecture, where proxies return results gathered from worker nodes after global aggregation and post-processing.\n\n#### Coordinator service \n\nThe coordinator service is the system's brain, responsible for cluster topology node management, load balancing, timestamp generation, data declaration, and data management. For a detailed explanation of the function of each coordinator service, read the [Milvus technical documentation](https://milvus.io/docs/v2.0.x/four_layers.md#Coordinator-service).\n\n#### Worker nodes\n\nThe worker, or execution, node acts as the limbs of the system, executing instructions issued by the coordinator service and the data manipulation language (DML) commands initiated by the proxy. A worker node in Milvus is similar to a data node in [Hadoop](https://hadoop.apache.org/), or a region server in HBase. Each type of worker node corresponds to a coord service. For a detailed explanation of the function of each worker node, read the [Milvus technical documentation](https://milvus.io/docs/v2.0.x/four_layers.md#Worker-nodes).\n\n#### Storage\n\nStorage is the cornerstone of Milvus, responsible for data persistence. The storage layer is divided into three parts:\n\n- **Meta store:** Responsible for storing snapshots of meta data such as collection schema, node status, message consumption checkpoints, etc. Milvus relies on etcd for these functions and Etcd also assumes the responsibility of service registration and health checks. \n- **Log broker:** A pub/sub system that supports playback and is responsible for streaming data persistence, reliable asynchronous query execution, event notifications, and returning query results. When nodes are performing downtime recovery, the log broker ensures the integrity of incremental data through log broker playback. Milvus cluster uses Pulsar as its log broker, while the standalone mode uses RocksDB. Streaming storage services such as Kafka and Pravega can also be used as log brokers.\n- **Object storage:** Stores snapshot files of logs, scalar/vector index files, and intermediate query processing results. Milvus supports [AWS S3](https://aws.amazon.com/s3/) and [Azure Blob](https://azure.microsoft.com/en-us/services/storage/blobs/), as well as [MinIO](https://min.io/), a lightweight, open-source object storage service. Due to the high access latency and billing per query of object storage services, Milvus will soon support memory/SSD-based cache pools and hot/cold data separation to improve performance and reduce costs.\n\n### Data Model\n\nThe data model organizes the data in a database. In Milvus, all data are organized by collection, shard, partition, segment, and entity.\n\n\n\n#### Collection\n\nA collection in Milvus can be likened to a table in a relational storage system. Collection is the biggest data unit in Milvus.\n\n#### Shard\n\nTo take full advantage of the parallel computing power of clusters when writing data, collections in Milvus must spread data writing operations to different nodes. By default, a single collection contains two shards. Depending on your dataset volume, you can have more shards in a collection. Milvus uses a master key hashing method for sharding.\n\n#### Partition\n\nThere are also multiple partitions in a shard. A partition in Milvus refers to a set of data marked with the same label in a collection. Common partitioning methods including partitioning by date, gender, user age, and more. Creating partitions can benefit the query process as tremendous data can be filtered by partition tag.\n\nIn comparison, sharding is more of scaling capabilities when writing data, while partitioning is more of enhancing system performance when reading data.\n\n\n\n#### Segments\n\nWithin each partition, there are multiple small segments. A segment is the smallest unit for system scheduling in Milvus. There are two types of segments, growing and sealed. Growing segments are subscribed by query nodes. The Milvus user keeps writing data into growing segments. When the size of a growing segment reaches an upper limit (512 MB by default), the system will not allow writing extra data into this growing segment, hence sealing this segment. Indexes are built on sealed segments.\n\nTo access data in real time, the system reads data in both growing segments and sealed segments. \n\n#### Entity\n\nEach segment contains massive amount of entities. An entity in Milvus is equivalent to a row in a traditional database. Each entity has a unique primary key field, which can also be automatically generated. Entities must also contain timestamp (ts), and vector field - the core of Milvus.\n\n## About the Deep Dive Series\n\nWith the [official announcement of general availability](https://milvus.io/blog/2022-1-25-annoucing-general-availability-of-milvus-2-0.md) of Milvus 2.0, we orchestrated this Milvus Deep Dive blog series to provide an in-depth interpretation of the Milvus architecture and source code. Topics covered in this blog series include:\n\n- [Milvus architecture overview](https://milvus.io/blog/deep-dive-1-milvus-architecture-overview.md)\n- [APIs and Python SDKs](https://milvus.io/blog/deep-dive-2-milvus-sdk-and-api.md)\n- [Data processing](https://milvus.io/blog/deep-dive-3-data-processing.md)\n- [Data management](https://milvus.io/blog/deep-dive-4-data-insertion-and-data-persistence.md)\n- [Real-time query](https://milvus.io/blog/deep-dive-5-real-time-query.md)\n- [Scalar execution engine](https://milvus.io/blog/deep-dive-7-query-expression.md)\n- [QA system](https://milvus.io/blog/deep-dive-6-oss-qa.md)\n- [Vector execution engine](https://milvus.io/blog/deep-dive-8-knowhere.md)\n","title":"Building a Vector Database for Scalable Similarity Search","metaData":{}},{"id":"2022-03-10-manage-your-milvus-vector-database-with-one-click-simplicity.md","author":"Zhen Chen","desc":"Attu - a GUI tool for Milvus 2.0.","tags":["Engineering"],"canonicalUrl":"https://zilliz.com/blog/manage-your-milvus-vector-database-with-one-click-simplicity","date":"2022-03-10T00:00:00.000Z","cover":"https://assets.zilliz.com/Attu_3ff9a76156.png","href":"/blog/2022-03-10-manage-your-milvus-vector-database-with-one-click-simplicity.md","content":"\n\n\nDraft by [Zhen Chen](https://github.com/czhen-zilliz) and transcreation by [Lichen Wang](https://github.com/LocoRichard).\n\n\u003cp style=\"font-size: 12px;color: #4c5a67\"\u003eClick \u003ca href=\"https://zilliz.com/blog/manage-your-milvus-vector-database-with-one-click-simplicity\"\u003ehere\u003c/a\u003e to check the original post.\u003c/p\u003e \n\nIn the face of rapidly growing demand for unstructured data processing, Milvus 2.0 stands out. It is an AI-oriented vector database system designed for massive production scenarios. Apart from all these Milvus SDKs and Milvus CLI, a command-line interface for Milvus, is there a tool that allows users to operate Milvus more intuitively? The anwer is YES. Zilliz has announced a graphical user interface - Attu - specifically for Milvus. In this article, we would like to show you step by step how to perform a vector similarity search with Attu.\n\n is an island on the west edge of Aleutian Islands. This uninhabited realm symbolizes an adventurous spirit.\")\n\nIn comparison with Milvus CLI which brings the uttermost simplicity of usage, Attu features more:\n- Installers for Windows OS, macOS, and Linux OS;\n- Intuitive GUI for easier usage of Milvus;\n- Coverage of major functionalities of Milvus;\n- Plugins for expansion of customized functionalities;\n- Complete system topology information for easier understanding and administration of Milvus instance.\n\n## Installation\n\nYou can find the newest release of Attu at [GitHub](https://github.com/zilliztech/attu/releases). Attu offers executable installers for different operating systems. It is an open-source project and welcomes contribution from everyone.\n\n\n\nYou can also install Attu via Docker.\n\n```shell\ndocker run -p 8000:3000 -e HOST_URL=http://{ attu IP }:8000 -e MILVUS_URL={milvus server IP}:19530 zilliz/attu:latest\n```\n\n`attu IP` is the IP address of the environment where Attu runs, and `milvus server IP` is IP address of the environment where Milvus runs.\n\nHaving installed Attu successfully, you can input the Milvus IP and Port in the interface to start Attu.\n\n\n\n## Feature overview\n\n\n\nAttu interface consists of **Overview** page, **Collection** page, **Vector Search** page, and **System View** page, corresponding to the four icons on the left-side navigation pane respectively.\n\nThe **Overview** page shows the loaded collections. While the **Collection** page lists all the collections and indicates if they are loaded or released.\n\n\n\nThe **Vector Search** and **System View** pages are plugins of Attu. The concepts and usage of the plugins will be introduced in the final part of the blog.\n\nYou can perform vector similarity search in **Vector Search** page.\n\n\n\nIn **System View** page, you can check the topological structure of Milvus.\n\n\n\nYou can also check the detailed information of each node by clicking the node.\n\n\n\n## Demonstration\n\nLet's explore Attu with a test dataset.\n\nCheck our [GitHub repo](https://github.com/zilliztech/attu/tree/main/examples) for the dataset used in the following test.\n\nFirst, create a collection named test with the following four fields:\n- Field Name: id, primary key field\n- Field Name: vector, vector field, float vector, Dimension: 128\n- Field Name: brand, scalar field, Int64\n- Field Name: color, scalar field, Int64\n\n\n\nLoad the collection for search after it was successfully created.\n\n\n\nYou can now check the newly created collection in the **Overview** page.\n\n\n\nImport the test dataset into Milvus.\n\n\n\n\n\n\n\nClick the collection name in Overview or Collection page to enter query interface to check the imported data.\n\nAdd filter, specify the expression `id != 0`, click **Apply Filter**, and click **Query**.\n\n\n\nYou will find all fifty entries of entities are imported successfully.\n\n\n\nLet's try vector similarity search.\n\nCopy one vector from the `search_vectors.csv` and paste it in **Vector Value** field. Choose the collection and field. Click **Search**.\n\n\n\nYou can then check the search result. Without compiling any scripts, you can search with Milvus easily.\n\n\n\nFinally, let's check the **System View** page.\n\nWith Metrics API encapsulated in Milvus Node.js SDK, you can check the system status, node relations, and node status.\n\nAs an exclusive feature of Attu, System Overview page includes a complete system topological graph. By clicking on each node, you can check its status (refresh every 10 seconds).\n\n\n\nClick on each node to enter the **Node List View**. You can check all child nodes of a coord node. By sorting, you can identify the nodes with high CPU or memory usage quickly, and locate the problem with the system.\n\n\n\n## What's more\n\nAs mentioned earlier, the **Vector Search** and **System View** pages are plugins of Attu. We encourage users to develop their own plugins in Attu to suit their application scenarios. In the source code, there is folder built specifically for plugin codes.\n\n\n\nYou can refer to any of the plugin to learn how to build a plugin. By setting the following config file, you can add the plugin to Attu.\n\n\n\nYou can see [Attu GitHub Repo](https://github.com/zilliztech/attu/tree/main/doc) and [Milvus Technical Document](https://milvus.io/docs/v2.0.x/attu.md) for detailed instruction.\n\nAttu is an open-source project. All contributions are welcome. You can also [file an issue](https://github.com/zilliztech/attu/issues) if you had any problem with Attu.\n\nWe sincerely hope that Attu can bring you a better user experience with Milvus. And if you like Attu, or have some feedbacks about the usage, you can complete this [Attu User Survey](https://wenjuan.feishu.cn/m/cfm?t=suw4QnODU1ui-ok7r) to help us optimize Attu for a better user experience.\n","title":"Manage Your Milvus Vector Database with One-click Simplicity","metaData":{}},{"id":"2022-02-28-how-milvus-balances-query-load-across-nodes.md","author":"Xi Ge","desc":"Milvus 2.0 supports automatic load balance across query nodes.","tags":["Engineering"],"canonicalUrl":"https://milvus.io/blog/2022-02-28-how-milvus-balances-query-load-across-nodes.md","date":"2022-02-28T00:00:00.000Z","cover":"https://assets.zilliz.com/Load_balance_b2f35a5577.png","href":"/blog/2022-02-28-how-milvus-balances-query-load-across-nodes.md","content":"\n\n\nBy [Xi Ge](https://github.com/xige-16).\n\nIn previous blog articles, we have successively introduced the Deletion, Bitset, and Compaction functions in Milvus 2.0. To culminate this series, we would like to share the design behind Load Balance, a vital function in the distributed cluster of Milvus.\n\n\n## Implementation\n\nWhereas the number and size of segments buffered in query nodes differ, the search performance across the query nodes may also vary. The worst case could happen when a few query nodes are exhausted searching on a large amount of data, but newly created query nodes remain idle because no segment is distributed to them, causing a massive waste of CPU resources and a huge drop in search performance.\n\nTo avoid such circumstances, the query coordinator (query coord) is programmed to distribute segments evenly to each query node according to the RAM usage of the nodes. Therefore, CPU resources are consumed equally across the nodes, thereby significantly improving search performance.\n\n### Trigger automatic load balance\n\nAccording to the default value of the configuration `queryCoord.balanceIntervalSeconds`, the query coord checks the RAM usage (in percentage) of all query nodes every 60 seconds. If either of the following conditions is satisfied, the query coord starts to balance the query load across the query node:\n\n1. RAM usage of any query node in the cluster is larger than `queryCoord.overloadedMemoryThresholdPercentage` (default: 90);\n2. Or the absolute value of any two query nodes' RAM usage difference is larger than `queryCoord.memoryUsageMaxDifferencePercentage` (default: 30).\n\nAfter the segments are transferred from the source query node to the destination query node, they should also satisfy both the following conditions:\n\n1. RAM usage of the destination query node is no larger than `queryCoord.overloadedMemoryThresholdPercentage` (default: 90);\n2. The absolute value of the source and destination query nodes' RAM usage difference after load balancing is less than that before load balancing.\n\nWith the above conditions satisfied, the query coord proceeds to balance the query load across the nodes.\n\n## Load balance\n\nWhen load balance is triggered, the query coord first loads the target segment(s) to the destination query node. Both query nodes return search results from the target segment(s) at any search request at this point to guarantee the completeness of the result.\n\nAfter the destination query node successfully loads the target segment, the query coord publishes a `sealedSegmentChangeInfo` to the Query Channel. As shown below, `onlineNodeID` and `onlineSegmentIDs` indicate the query node that loads the segment and the segment loaded respectively, and `offlineNodeID` and `offlineSegmentIDs` indicate the query node that needs to release the segment and the segment to release respectively.\n\n\n\nHaving received the `sealedSegmentChangeInfo`, the source query node then releases the target segment. \n\n\n\nThe whole process succeeds when the source query node releases the target segment. By completing that, the query load is set balanced across the query nodes, meaning the RAM usage of all query nodes is no larger than `queryCoord.overloadedMemoryThresholdPercentage`, and the absolute value of the source and destination query nodes' RAM usage difference after load balancing is less than that before load balancing.\n\n## What's next?\n\nIn the 2.0 new feature series blog, we aim to explain the design of the new features. Read more in this blog series!\n- [How Milvus Deletes Streaming Data in a Distributed Cluster](https://milvus.io/blog/2022-02-07-how-milvus-deletes-streaming-data-in-distributed-cluster.md)\n- [How to Compact Data in Milvus?](https://milvus.io/blog/2022-2-21-compact.md)\n- [How Milvus Balances Query Load across Nodes?](https://milvus.io/blog/2022-02-28-how-milvus-balances-query-load-across-nodes.md)\n- [How Bitset Enables the Versatility of Vector Similarity Search](https://milvus.io/blog/2022-2-14-bitset.md) \n\nThis is the finale of the Milvus 2.0 new feature blog series. Following this series, we are planning a new series of Milvus [Deep Dive](https://milvus.io/blog/deep-dive-1-milvus-architecture-overview.md), which introduces the basic architecture of Milvus 2.0. Please stay tuned.\n","title":"How Milvus Balances Query Load across Nodes?","metaData":{}},{"id":"2022-2-21-compact.md","author":"Bingyi Sun","desc":"Compaction is a new feature released in Milvus 2.0 that helps you save storage space.","tags":["Engineering"],"canonicalUrl":"https://milvus.io/blog/2022-2-21-compact.md","date":"2022-02-21T00:00:00.000Z","cover":"https://assets.zilliz.com/Compact_173a08ec1c.png","href":"/blog/2022-2-21-compact.md","content":"\n\n\nBy [Bingyi Sun](https://github.com/sunby) and [Angela Ni](https://www.linkedin.com/in/yiyun-n-2aa713163/).\n\nWith the [official release](https://milvus.io/blog/2022-1-25-annoucing-general-availability-of-milvus-2-0.md) of Milvus 2.0 GA, a list of new features are supported. Among those, compaction is one of the new features that can help you save storage space.\n\nCompaction refers to the process of merging small segments into large ones and clean logically deleted data. In other words, compaction reduces usage of disk space by purging the deleted or expired entities in binlogs. It is a background task that is triggered by [data coord](https://milvus.io/docs/v2.0.x/four_layers.md#Data-coordinator-data-coord) and executed by [data node](https://milvus.io/docs/v2.0.x/four_layers.md#Data-node) in Milvus.\n\nThis article dissects the concept and implementation of compaction in Milvus.\n\n## What is compaction?\n\nBefore going deep into the details of how to implement compaction in Milvus 2.0, it is critical to figure out what compaction is in Milvus.\n\nMore often than not, as a Milvus user you might have been bothered by the increasing usage of hard disk space. Another issue is that a segment with less than 1,024 rows is not indexed and only supports brute-force search to process queries. Small segments caused by auto-flush or user-invoked flush might hamper query efficiency.\n\nTherefore, to solve the two issues mentioned above and help reduce disk usage and improve query efficiency, Milvus supports compaction.\n\nDatabases like [LevelDB](https://github.com/google/leveldb) and [RocksDB](http://rocksdb.org/) append data to sorted strings tables (SSTables). The average disk reads per query increase with the number of SSTables, leading to inefficient queries. To reduce read amplification and release hard drive space, these databases compact SSTables into one. Compaction processes run in the background automatically. \n\nSimilarly, Milvus appends inserted and deleted data to [binlogs](https://github.com/milvus-io/milvus/blob/master/docs/developer_guides/chap08_binlog.md). As the number of binlogs increases, more hard disk space is used. To release hard disk space, Milvus compacts binlogs of deleted and inserted data. If an entity is inserted but later deleted, it no longer exists in the binlogs that records data insertion or deletion once compacted. In addition, Milvus also compacts [segments](https://milvus.io/docs/v2.0.x/glossary.md#Segment) - data files automatically created by Milvus for holding inserted data.\n\n## How to configure compaction?\n\nConfiguration of compaction in Milvus mainly involves two parameters: `dataCoord.enableCompaction` and `common.retentionDuration`. \n\n`dataCoord.enableCompaction` specifies whether to enable compaction. Its default value is `true`. \n\n`common.retentionDuration` specifies a period when compaction does not run. Its unit is second. When you compact data, all deleted entities will be made unavailable for search with Time Travel. Therefore, if you plan to search with Time Travel, you have to specify a period of time during which compaction does not run and does not affect deleted data. To ensure accurate results of searches with Time Travel, Milvus retains data operated in a period specified by `common.retentionDuration`. That is, data operated in this period will not be compacted. For more details, see [Search with Time Travel](https://milvus.io/docs/v2.0.x/timetravel.md).\n\nCompaction is enabled in Milvus by default. If you disabled compaction but later want to manually enable it, you can follow the steps below:\n\n1. Call the `collection.compact()` method to trigger a global compaction process manually. However, please be noted that this operation might take a long time.\n2. After calling the method, a compaction ID is returned. View the compaction status by calling the `collection.get_compaction_state()` method.\n\nAfter compaction is enabled, it runs in the background automatically. Since the compaction process might take a long time, compaction requests are processed asynchronously to save time.\n\n## How to implement compaction?\n\nIn Milvus, you can either implement compaction manually or automatically.\n\nManual compaction of binlogs or segments does not require meeting any trigger conditions. Therefore, if you manually invoke compaction, the binlogs or segments will be compacted no matter what.\n\nHowever, if you want to enable automatic compaction, certain compaction trigger conditions need to be met in order for the system to compact your segments or binlogs.\n\nGenerally there are two types of objects that can be compacted in Milvus: binglogs and segments.\n\n\n## Binlog compaction\n\nA binlog is a binary log, or a smaller unit in segment, that records and handles the updates and changes made to data in the Milvus vector database. Data from a segment is persisted in multiple binlogs. Binlog compaction involves two types of binlogs in Milvus: insert binlogs and delta binlogs.\n\nDelta binlogs are generated when data is deleted while insert binlogs are generated under the following three circumstances.\n\n- As inserted data is being appended, the segment reaches the upper limit of size and is automatically flushed to the disk.\n- DataCoord automatically flushes segments that stay unsealed for a long time.\n- Some APIs like `collection.num_entities`, `collection.load()`, and more automatically invoke flush to write segments to disk.\n\nTherefore, binlog compaction, as its name suggests, refers to compacting binlogs within a segment. More specifically, during binlog compaction, all delta binlogs and insert binlogs that are not retained are compacted.\n\n\n\nWhen a segment is flushed to disk, or when Milvus requests global compaction as compaction has not run for a long time, at least one of the following two conditions need to be met to trigger automatic compaction:\n\n1. Rows in delta binlogs are more than 20% of the total rows.\n2. The size of delta binlogs exceeds 10 MB.\n\n\n## Segment compaction\n\nA [segment](https://milvus.io/docs/v2.0.x/glossary.md#Segment) is a data file automatically created by Milvus for holding inserted data. There are two types of segments in Milvus: growing segment and sealed segment. \n\nA growing segment keeps receiving the newly inserted data until it is sealed. A sealed segment no longer receives any new data, and will be flushed to the object storage, leaving new data to be inserted into a newly created growing segment. \n\nTherefore, segment compaction refers to compacting multiple sealed segments. More specifically, during segment compaction, small segments are compacted into bigger ones.\n\n\n\nEach segment generated after compaction cannot exceed the upper limit of a segment size, which is 512 MB by default. Read [system configurations](https://milvus.io/docs/v2.0.x/system_configuration.md) to learn how to modify the upper limit of a segment size.\n\nWhen a segment flushes to disk, or when Milvus requests global compaction as compaction has not run for a long time, the following condition needs to be met to trigger automatic compaction:\n\n- Segments smaller than 0.5 * `MaxSegmentSize` is more than 10.\n\n## What's next?\n\nWhat's next after learning the basics of compaction in Milvus? Currently, not all parameters for configuring compaction are in the `milvus.yaml` file, and plan generation strategies are relatively basic. Come and contribute to Milvus, [the open-source project](https://github.com/milvus-io) if you are interested!\n\nAlso, in the 2.0 new feature series blog, we aim to explain the design of the new features. Read more in this blog series!\n- [How Milvus Deletes Streaming Data in a Distributed Cluster](https://milvus.io/blog/2022-02-07-how-milvus-deletes-streaming-data-in-distributed-cluster.md)\n- [How to Compact Data in Milvus?](https://milvus.io/blog/2022-2-21-compact.md)\n- [How Milvus Balances Query Load across Nodes?](https://milvus.io/blog/2022-02-28-how-milvus-balances-query-load-across-nodes.md)\n- [How Bitset Enables the Versatility of Vector Similarity Search](https://milvus.io/blog/2022-2-14-bitset.md) \n\n## About the author\n\nBingyi Sun, Senior Software Engineer of the Milvus project, achieved his Master's degree in software engineering at Shanghai Jiao Tong University. He is mainly responsible for developing storage related components in Milvus 2.0. His area of interest is database and distributed systems. He is great fan of open source projects and a gourmet who enjoys playing video games and reading in his spare time.\n","title":"How to Compact Data in Milvus?","metaData":{}},{"id":"2022-2-14-bitset.md","author":"Yudong Cai","desc":"Learn how bitset is used to enable several important features in Milvus.","tags":["Engineering"],"canonicalURL":"https://milvus.io/blog/2022-2-14-bitset.md","date":"2022-02-14T00:00:00.000Z","cover":"https://assets.zilliz.com/Bitset_cd54487e7b.png","href":"/blog/2022-2-14-bitset.md","content":"\n\n\nBy [Yudong Cai](https://github.com/cydrain) and [Angela Ni](https://www.linkedin.com/in/yiyun-n-2aa713163/).\n\nVarious [new essential features](https://milvus.io/blog/2022-1-27-milvus-2-0-a-glimpse-at-new-features.md) of a vector database are provided together with the [release](https://milvus.io/blog/2022-1-25-annoucing-general-availability-of-milvus-2-0.md) of Milvus 2.0. Among the new features, [Time Travel](https://milvus.io/docs/v2.0.x/timetravel_ref.md), attribute filtering, and [delete operations](https://milvus.io/blog/2022-02-07-how-milvus-deletes-streaming-data-in-distributed-cluster.md) are correlated as these three features are achieved by one common mechanism - bitset.\n\nTherefore, this article aims to clarify the concept of bitset in Milvus and explain how it works to support delete operations, Time Travel, and attribute filtering with three examples.\n\n## What is bitset?\n\nA bitset is an array of bit numbers (\"0\" and \"1\") that can be used to represent certain data information. With bitsets, you can store certain types of data compactly and efficiently as opposed to store them in Ints, floats, or chars. Bitsets work on [boolean logic](https://milvus.io/docs/v2.0.x/boolean.md), according to which the value of an output is either valid or invalid, usually denoted by \"1\" and \"0\" respectively. \"1\" stands for valid, and \"0\" for invalid. Since bitsets are highly efficient and can save storage, they can also be used to achieve many features such as attribute filtering, delete operations, Time Travel, and more.\n\nStarting from version 0.7.0, the concept of bitset has been introduced in Milvus to enable the [delete function](https://milvus.io/blog/deleting-data-in-milvus.md). More specifically, bitset is used to mark if each row in the segment is deleted. Deleted entities are marked with \"1\" in the corresponding bitset, and as a result, the deleted entities will not be computed during a search or query.\n\nIn the Milvus 2.0 version, the application of bitset is extended to enable more features, like attribute filtering and Time Travel. The general principle in a bitset remains the same. That is, if an entity is marked with \"1\" in the corresponding bitset, the entity will be ignored during a search or query. Bitsets are used to enable 3 features in Milvus:\n\n- Attribute filtering\n- Data deletion\n- Query with Time Travel\n\n## How does bitset work in Milvus?\n\nThe examples below are used to illustrate how bitset works in Milvus.\n\n### Prerequisites\n\nSuppose there is a segment with eight entities and a series of data manipulation language (DML) events happens in the order shown in the figure below.\n\n- Four of the entities, whose `primary_keys` are \\[1, 2, 3, 4] respectively, are inserted when the timestamp `ts` equals 100. \n- The remaining four entities, whose `primary_keys` are \\[5, 6, 7, 8], are inserted when the timestamp `ts` equals 200. \n- Entities whose `primary_keys` are \\[7, 8] are deleted when the timestamp `ts` equals 300. \n- Only entities, whose `primary_keys` are \\[1, 3, 5, 7], satisfy the conditions of attribute filtering. \n\n\n\n### Case one\n\nSuppose the value a user sets for `time_travel` is 150. In other words, the user conducts a query on the data stored in Milvus when `ts` = 150. The bitset generation process is illustrated by Figure 1. \n\nDuring the initial filtering stage, the result of the `filter_bitset` should be \\[1, 0, 1, 0, 1, 0, 1, 0] as entities \\[1, 3, 5, 7] are valid filtering results and marked as \"1\" in the bitset. However, entities \\[4, 5, 6, 7] were not even inserted to the vector database when `ts` equals 150. Therefore, these four entities should be marked as \"0\" regardless of the filtering condition. Now the bitset result should be \\[1, 0, 1, 0, 0, 0, 0, 0]. Since in Milvus, the general principle of bitset computing is that entities marked with \"1\" in the bitset are ignored during a search or query, the bitset result after Time Travel and attribute filtering needs to be flipped in order to be combined with the deletion bitmap. The flipped result of `filter_bitset` should be \\[0, 1, 0, 1, 1, 1, 1, 1].\n\nAs for the deletion bitset `del_bitset`, the initial value should be \\[0, 0, 0, 0, 0, 0, 1, 1]. However, entities 7 and 8 are not deleted until `ts` is 300. Therefore, when `ts` is 150, entities 7 and 8 are still valid. As a result, the `del_bitset` value after Time Travel should be \\[0, 0, 0, 0, 0, 0, 0, 0]. \n\nNow we have two bitsets after Time Travel and attribute filtering: `filter_bitset` \\[0, 1, 0, 1, 1, 1, 1, 1] and `del_bitset` \\[0, 0, 0, 0, 0, 0, 0, 0]. Combine these two bitsets with the \"OR\" binary logic operator. The ultimate value of `result_bitset` is \\[0, 1, 0, 1, 1, 1, 1, 1]. That is to say, only entities 1 and 3 will be computed in the following search or query stage.\n\n\n\n\n### Case two\n\nSuppose the value the user sets for `time_travel` is 250. In other words, the user conducts a query on the data stored in Milvus when `ts` = 250. The bitset generation process is illustrated by Figure 2. \n\nLike in case one, the resultant `filter_bitset` of the initial attribute filtering stage should be \\[1, 0, 1, 0, 1, 0, 1, 0]. \n\nAll entities \\[1, 2, 3, 4, 5, 6, 7, 8] are inserted to the vector database when `ts`= 250. Therefore, the previous result of `filter_bitset` remains the same. Again, we need to flip the result of the `filter_bitset`, and we will get \\[0, 1, 0, 1, 0, 1, 0, 1].\n\nAs for the deletion bitset `del_bitset`, the initial value should be \\[0, 0, 0, 0, 0, 0, 1, 1]. However, entities 7 and 8 were not deleted until `ts` is 300. Therefore, when `ts` is 250, entities 7 and 8 are still valid. As a result, the `del_bitset` value after Time Travel should be \\[0, 0, 0, 0, 0, 0, 0, 0]. \n\nNow we have two bitsets after Time Travel and attribute filtering: `filter_bitset` \\[0, 1, 0, 1, 0, 1, 0, 1] and `del_bitset` \\[0, 0, 0, 0, 0, 0, 0, 0]. Combine these two bitsets with the \"OR\" binary logic operator. The ultimate value of `result_bitset` is \\[0, 1, 0, 1, 0, 1, 0, 1]. That is to say, only entities \\[1, 3, 5, 7] will be computed in the following search or query stage.\n\n\n\n### Case three\n\nSuppose the value the user sets for `time_travel` is 350. In other words, the user conducts a query on the data stored in Milvus when `ts` = 350. The bitset generation process is illustrated by Figure 3. \n\nSame as case one and two, the resultant `filter_bitset` of the initial attribute filtering stage is \\[0, 1, 0, 1, 0, 1, 0, 1]. \n\nAll entities \\[1, 2, 3, 4, 5, 6, 7, 8] are inserted to the vector database when `ts`= 350. Therefore, the final flipped result of the `filter_bitset` is \\[0, 1, 0, 1, 0, 1, 0, 1], the same as in case two.\n\nAs for the deletion bitset `del_bitset`, since entities 7 and 8 are already deleted when `ts`=350, therefore, the result of `del_bitset` should be \\[0, 0, 0, 0, 0, 0, 1, 1].\n\nNow we have two bitsets after Time Travel and attribute filtering: `filter_bitset` \\[0, 1, 0, 1, 0, 1, 0, 1] and `del_bitset` \\[0, 0, 0, 0, 0, 0, 1, 1]. Combine these two bitsets with the \"OR\" binary logic operator. The ultimate value of `result_bitset` is \\[0, 1, 0, 1, 0, 1, 1, 1]. That is to say, only entities \\[1, 3, 5] will be computed in the following search or query stage.\n\n\n\n## What's next?\n\nIn the 2.0 new feature series blog, we aim to explain the design of the new features. Read more in this blog series!\n\n- [How Milvus Deletes Streaming Data in a Distributed Cluster](https://milvus.io/blog/2022-02-07-how-milvus-deletes-streaming-data-in-distributed-cluster.md)\n- [How to Compact Data in Milvus?](https://milvus.io/blog/2022-2-21-compact.md)\n- [How Milvus Balances Query Load across Nodes?](https://milvus.io/blog/2022-02-28-how-milvus-balances-query-load-across-nodes.md)\n- [How Bitset Enables the Versatility of Vector Similarity Search](https://milvus.io/blog/2022-2-14-bitset.md) \n","title":"How Bitset Enables the Versatility of Vector Similarity Search","metaData":{}},{"id":"2022-02-07-how-milvus-deletes-streaming-data-in-distributed-cluster.md","author":"Lichen Wang","desc":"The cardinal design behind the deletion function in Milvus 2.0, the world's most advanced vector database.","date":"2022-02-07T00:00:00.000Z","cover":"https://assets.zilliz.com/Delete_9f40bbfa94.png","tags":["Engineering"],"href":"/blog/2022-02-07-how-milvus-deletes-streaming-data-in-distributed-cluster.md","content":"\n# How Milvus Deletes Streaming Data in a Distributed Cluster\n\nFeaturing unified batch-and-stream processing and cloud-native architecture, Milvus 2.0 poses a greater challenge than its predecessor did during the development of the DELETE function. Thanks to its advanced storage-computation disaggregation design and the flexible publication/subscription mechanism, we are proud to announce that we made it happen. In Milvus 2.0, you can delete an entity in a given collection with its primary key so that the deleted entity will no longer be listed in the result of a search or a query.\n\nPlease note that the DELETE operation in Milvus refers to logical deletion, whereas physical data cleanup occurs during the Data Compaction. Logical deletion not only greatly boosts the search performance constrained by the I/O speed, but also facilitates data recovery. Logically deleted data can still be retrieved with the help of the Time Travel function.\n\n## Usage\n\nLet's try out the DELETE function in Milvus 2.0 first. (The following example uses PyMilvus 2.0.0 on Milvus 2.0.0).\n\n```python\nfrom pymilvus import connections, utility, Collection, DataType, FieldSchema, CollectionSchema\n# Connect to Milvus\nconnections.connect(\n alias=\"default\", \n host='x.x.x.x', \n port='19530'\n)\n# Create a collection with Strong Consistency level\npk_field = FieldSchema(\n name=\"id\", \n dtype=DataType.INT64, \n is_primary=True, \n)\nvector_field = FieldSchema(\n name=\"vector\", \n dtype=DataType.FLOAT_VECTOR, \n dim=2\n)\nschema = CollectionSchema(\n fields=[pk_field, vector_field], \n description=\"Test delete\"\n)\ncollection_name = \"test_delete\"\ncollection = Collection(\n name=collection_name, \n schema=schema, \n using='default', \n shards_num=2,\n consistency_level=\"Strong\"\n)\n# Insert randomly generated vectors\nimport random\ndata = [\n [i for i in range(100)],\n [[random.random() for _ in range(2)] for _ in range(100)],\n]\ncollection.insert(data)\n# Query to make sure the entities to delete exist\ncollection.load()\nexpr = \"id in [2,4,6,8,10]\"\npre_del_res = collection.query(\n expr,\n output_fields = [\"id\", \"vector\"]\n)\nprint(pre_del_res)\n# Delete the entities with the previous expression\ncollection.delete(expr)\n# Query again to check if the deleted entities exist\npost_del_res = collection.query(\n expr,\n output_fields = [\"id\", \"vector\"]\n)\nprint(post_del_res)\n```\n\n## Implementation\n\nIn a Milvus instance, a data node is mainly responsible for packing streaming data (logs in log broker) as historical data (log snapshots) and automatically flushing them to object storage. A query node executes search requests on full data, i.e. both streaming data and historical data.\n\nTo make the most of the data writing capacity of parallel nodes in a cluster, Milvus adopts a sharding strategy based on primary key hashing to distribute writing operations evenly to different worker nodes. That is to say, proxy will route the Data Manipulation Language (DML) messages (i.e. requests) of an entity to the same data node and query node. These messages are published through the DML-Channel and consumed by the data node and query node separately to provide search and query services together.\n\n### Data node\n\nHaving received data INSERT messages, the data node inserts the data in a growing segment, which is a new segment created to receive streaming data in memory. If either the data row count or the duration of the growing segment reaches the threshold, the data node seals it to prevent any incoming data. The data node then flushes the sealed segment, which contains the historical data, to the object storage. Meanwhile, the data node generates a bloom filter based on the primary keys of the new data, and flushed it to the object storage together with the sealed segment, saving the bloom filter as a part of the statistics binary log (binlog), which contains the statistical information of the segment.\n\n\u003e A bloom filter is a probabilistic data structure that consists of a long binary vector and a series of random mapping functions. It can be used to test whether an element is a member of a set, but might return false positive matches. —— Wikipedia\n\nWhen data DELETE messages come in, data node buffers all bloom filters in the corresponding shard, and matches them with the primary keys provided in the messages to retrieve all segments (from both growing and sealed ones) that possibly include the entities to delete. Having pinpointed the corresponding segments, data node buffers them in memory to generate the Delta binlogs to record the delete operations, and then flushes those binlogs together with the segments back to the object storage.\n\n\n\nSince one shard is only assigned with one DML-Channel, extra query nodes added to the cluster will not be able to subscribe to the DML-Channel. To ensure that all query nodes can receive the DELETE messages, data nodes filter the DELETE messages from the DML-Channel, and forward them to Delta-Channel to notify all query nodes of the delete operations.\n\n### Query node\n\nWhen loading a collection from object storage, the query node first obtains each shard's checkpoint, which marks the DML operations since the last flush operation. Based on the checkpoint, the query node loads all sealed segments together with their Delta binlog and bloom filters. With all data loaded, the query node then subscribes to DML-Channel, Delta-Channel, and Query-Channel.\n\nIf more data INSERT messages come after the collection is loaded to memory, query node first pinpoints the growing segments according to the messages, and updates corresponding bloom filters in memory for query purposes only. Those query-dedicated bloom filters will not be flushed to object storage after the query is finished.\n\n\n\nAs mentioned above, only a certain number of query nodes can receive DELETE messages from the DML-Channel, meaning only they can execute the DELETE requests in growing segments. For those query nodes which have subscribed to the DML-Channel, they first filter the DELETE messages in the growing segments, locate the entities by matching the provided primary keys with those query-dedicated bloom filters of the growing segments, and then record the delete operations in the corresponding segments. \n\nQuery nodes that cannot subscribe to the DML-Channel are only allowed to process search or query requests on sealed segments because they can only subscribe to the Delta-Channel, and receive the DELETE messages forwarded by data nodes. Having collected all DELETE messages in the sealed segments from Delta-Channel, the query nodes locate the entities by matching the provided primary keys with the bloom filters of the sealed segments, and then record the delete operations in the corresponding segments.\n\nEventually, in a search or query, the query nodes generate a bitset based on the delete records to omit the deleted entities, and search among the remaining entities from all segments, regardless of the segment status. Last but not least, the consistency level affects the visibility of the deleted data. Under Strong Consistency Level (as shown in the previous code sample), the deleted entities are immediately invisible after deletion. While Bounded Consistency Level is adopted, there will be several seconds of latency before the deleted entities become invisible.\n\n## What's next?\n\nIn the 2.0 new feature series blog, we aim to explain the design of the new features. Read more in this blog series!\n- [How Milvus Deletes Streaming Data in a Distributed Cluster](https://milvus.io/blog/2022-02-07-how-milvus-deletes-streaming-data-in-distributed-cluster.md)\n- [How to Compact Data in Milvus?](https://milvus.io/blog/2022-2-21-compact.md)\n- [How Milvus Balances Query Load across Nodes?](https://milvus.io/blog/2022-02-28-how-milvus-balances-query-load-across-nodes.md)\n- [How Bitset Enables the Versatility of Vector Similarity Search](https://milvus.io/blog/2022-2-14-bitset.md) \n","title":"How Milvus Deletes Streaming Data in a Distributed Cluster","metaData":{}},{"id":"2022-1-27-milvus-2-0-a-glimpse-at-new-features.md","author":"Yanliang Qiao","desc":"Check out the Newest Features of Milvus 2.0.","date":"2022-01-27T00:00:00.000Z","cover":"https://assets.zilliz.com/New_features_in_Milvus_2_0_93a87a7a8a.png","tags":["Engineering"],"href":"/blog/2022-1-27-milvus-2-0-a-glimpse-at-new-features.md","content":"\n# Milvus 2.0: A Glimpse at New Features\n\n\nIt has been half a year since the first release candidate of Milvus 2.0. Now we are proud to announce the general availability of the Milvus 2.0. Please follow me step by step to catch a glimpse at some of the new features that Milvus supports.\n\n\n## Entity deletion\n\nMilvus 2.0 supports entity deletion, allowing users to delete vectors based on the primary keys (IDs) of the vectors. They won't be worried about the expired or invalid data anymore. Let's try it.\n\n1. Connect to Milvus, create a new collection, and insert 300 rows of randomly-generated 128-dimensional vectors.\n\n```python\nfrom pymilvus import connections, utility, Collection, DataType, FieldSchema, CollectionSchema\n# connect to milvus\nhost = 'x.x.x.x'\nconnections.add_connection(default={\"host\": host, \"port\": 19530})\nconnections.connect(alias='default')\n# create a collection with customized primary field: id_field\ndim = 128\nid_field = FieldSchema(name=\"cus_id\", dtype=DataType.INT64, is_primary=True)\nage_field = FieldSchema(name=\"age\", dtype=DataType.INT64, description=\"age\")\nembedding_field = FieldSchema(name=\"embedding\", dtype=DataType.FLOAT_VECTOR, dim=dim)\nschema = CollectionSchema(fields=[id_field, age_field, embedding_field],\n auto_id=False, description=\"hello MilMil\")\ncollection_name = \"hello_milmil\"\ncollection = Collection(name=collection_name, schema=schema)\nimport random\n# insert data with customized ids\nnb = 300\nids = [i for i in range(nb)]\nages = [random.randint(20, 40) for i in range(nb)]\nembeddings = [[random.random() for _ in range(dim)] for _ in range(nb)]\nentities = [ids, ages, embeddings]\nins_res = collection.insert(entities)\nprint(f\"insert entities primary keys: {ins_res.primary_keys}\")\n```\n```\ninsert entities primary keys: [0, 1, 2, 3, 4, 5, 6, 7, 8, 9, 10, 11, 12, 13, 14, 15, 16, 17, 18, 19, 20, 21, 22, 23, 24, 25, 26, 27, 28, 29, 30, 31, 32, 33, 34, 35, 36, 37, 38, 39, 40, 41, 42, 43, 44, 45, 46, 47, 48, 49, 50, 51, 52, 53, 54, 55, 56, 57, 58, 59, 60, 61, 62, 63, 64, 65, 66, 67, 68, 69, 70, 71, 72, 73, 74, 75, 76, 77, 78, 79, 80, 81, 82, 83, 84, 85, 86, 87, 88, 89, 90, 91, 92, 93, 94, 95, 96, 97, 98, 99, 100, 101, 102, 103, 104, 105, 106, 107, 108, 109, 110, 111, 112, 113, 114, 115, 116, 117, 118, 119, 120, 121, 122, 123, 124, 125, 126, 127, 128, 129, 130, 131, 132, 133, 134, 135, 136, 137, 138, 139, 140, 141, 142, 143, 144, 145, 146, 147, 148, 149, 150, 151, 152, 153, 154, 155, 156, 157, 158, 159, 160, 161, 162, 163, 164, 165, 166, 167, 168, 169, 170, 171, 172, 173, 174, 175, 176, 177, 178, 179, 180, 181, 182, 183, 184, 185, 186, 187, 188, 189, 190, 191, 192, 193, 194, 195, 196, 197, 198, 199, 200, 201, 202, 203, 204, 205, 206, 207, 208, 209, 210, 211, 212, 213, 214, 215, 216, 217, 218, 219, 220, 221, 222, 223, 224, 225, 226, 227, 228, 229, 230, 231, 232, 233, 234, 235, 236, 237, 238, 239, 240, 241, 242, 243, 244, 245, 246, 247, 248, 249, 250, 251, 252, 253, 254, 255, 256, 257, 258, 259, 260, 261, 262, 263, 264, 265, 266, 267, 268, 269, 270, 271, 272, 273, 274, 275, 276, 277, 278, 279, 280, 281, 282, 283, 284, 285, 286, 287, 288, 289, 290, 291, 292, 293, 294, 295, 296, 297, 298, 299]\n```\n\n2. Before proceeding to deletion, check the entities you want to delete exist by search or query, and do it twice to make sure the result is reliable.\n\n```python\n# search\nnq = 10\nsearch_vec = [[random.random() for _ in range(dim)] for _ in range(nq)]\nsearch_params = {\"metric_type\": \"L2\", \"params\": {\"nprobe\": 16}}\nlimit = 3\n# search 2 times to verify the vector persists\nfor i in range(2):\n results = collection.search(search_vec, embedding_field.name, search_params, limit)\n ids = results[0].ids\n print(f\"search result ids: {ids}\")\n expr = f\"cus_id in {ids}\"\n # query to verify the ids exist\n query_res = collection.query(expr)\n print(f\"query results: {query_res}\")\n```\n```\nsearch result ids: [76, 2, 246]\nquery results: [{'cus_id': 246}, {'cus_id': 2}, {'cus_id': 76}]\nsearch result ids: [76, 2, 246]\nquery results: [{'cus_id': 246}, {'cus_id': 2}, {'cus_id': 76}]\n```\n\n3. Delete the entity with the cus_id of 76, and then search and query for this entity.\n\n```python\nprint(f\"trying to delete one vector: id={ids[0]}\")\ncollection.delete(expr=f\"cus_id in {[ids[0]]}\")\nresults = collection.search(search_vec, embedding_field.name, search_params, limit)\nids = results[0].ids\nprint(f\"after deleted: search result ids: {ids}\")\nexpr = f\"cus_id in {ids}\"\n# query to verify the id exists\nquery_res = collection.query(expr)\nprint(f\"after deleted: query res: {query_res}\")\nprint(\"completed\")\n```\n```\ntrying to delete one vector: id=76\nafter deleted: search result ids: [76, 2, 246]\nafter deleted: query res: [{'cus_id': 246}, {'cus_id': 2}, {'cus_id': 76}]\ncompleted\n```\n\n\nWhy is the deleted entity still retrievable? If you have checked the source code of Milvus, you will find that the deletion within Milvus is asynchronous and logical, which means that entities won't be physically deleted. Instead, they will be attached with a \"deleted\" mark so that no search or query requests will retrieve them. In addition, Milvus searches under Bounded Staleness consistency level by default. Therefore, the deleted entities are still retrievable before the data is synchronized in data node and query node. Try search or query the deleted entity after a few seconds, you will then find it is no longer in the result. \n\n\n```python\nexpr = f\"cus_id in {[76, 2, 246]}\"\n# query to verify the id exists\nquery_res = collection.query(expr)\nprint(f\"after deleted: query res: {query_res}\")\nprint(\"completed\")\n```\n```\nafter deleted: query res: [{'cus_id': 246}, {'cus_id': 2}]\ncompleted\n```\n\n## Consistency level\n\nThe above experiment shows us how the consistency level influences the immediate visibility of the newly deleted data.\nUsers can adjust the consistency level for Milvus flexibly to adapt it to various service scenarios. Milvus 2.0 supports four levels of consistency:\n- `CONSISTENCY_STRONG`: `GuaranteeTs` is set as identical to the newest system timestamp, and query nodes wait until the service time proceeds to the newest system timestamp, and then process the search or query request.\n- `CONSISTENCY_EVENTUALLY`: `GuaranteeTs` is set insignificantly smaller than the newest system timestamp to skip the consistency check. Query nodes search immediately on the existing data view.\n- `CONSISTENCY_BOUNDED`: `GuaranteeTs` is set relatively smaller than the newest system timestamp, and query nodes search on a tolerable, less updated data view.\n- `CONSISTENCY_SESSION`: The client uses the timestamp of the last write operation as the `GuaranteeTs`, so that each client can at least retrieve the data inserted by itself. \n\n\nIn the previous RC release, Milvus adopts Strong as the default consistency. However, taking account of the fact that most users are less demanding about consistency than performance, Milvus changes the default consistency as Bounded Staleness, which can balance their requirements to a greater extent. In the future, we will further optimize the configuration of the GuaranteeTs, which can be achieved only during collection creation in current release. For more information about `GuaranteeTs`, see [Guarantee Timestamp in Search Requests](https://github.com/milvus-io/milvus/blob/master/docs/developer_guides/how-guarantee-ts-works.md). \n\nWill lower consistency lead to better performance? You can never find the answer until you try it.\n\n4. Modify the code above to record the search latency.\n\n\n```python\nfor i in range(5):\n start = time.time()\n results = collection.search(search_vec, embedding_field.name, search_params, limit)\n end = time.time()\n print(f\"search latency: {round(end-start, 4)}\")\n ids = results[0].ids\n print(f\"search result ids: {ids}\")\n```\n\n\n5. Search with the identical data scale and parameters except that `consistency_level` is set as `CONSISTENCY_STRONG`.\n\n\n```python\ncollection_name = \"hello_milmil_consist_strong\"\ncollection = Collection(name=collection_name, schema=schema,\n consistency_level=CONSISTENCY_STRONG)\n```\n```\nsearch latency: 0.3293\nsearch latency: 0.1949\nsearch latency: 0.1998\nsearch latency: 0.2016\nsearch latency: 0.198\ncompleted\n```\n\n\n6. Search in a collection with `consistency_level` set as `CONSISTENCY_BOUNDED`.\n\n\n```python\ncollection_name = \"hello_milmil_consist_bounded\"\ncollection = Collection(name=collection_name, schema=schema,\n consistency_level=CONSISTENCY_BOUNDED)\n```\n```\nsearch latency: 0.0144\nsearch latency: 0.0104\nsearch latency: 0.0107\nsearch latency: 0.0104\nsearch latency: 0.0102\ncompleted\n```\n\n7. Clearly, average search latency in `CONSISTENCY_BOUNDED` collection is 200ms shorter than that in `CONSISTENCY_STRONG` collection.\n\n\nAre the deleted entities immediately invisible if the consistency level is set as Strong? The answer is Yes. You can still try this on your own.\n\n## Handoff\nWorking with streaming dataset, many users are used to building an index and loading the collection before inserting data into it. In previous releases of Milvus, users have to load collection manually after the index building to replace the raw data with the index, which is slow and laborious. The handoff feature allows Milvus 2.0 to automatically load indexed segment to replace the streaming data that reaches certain thresholds of indexing, greatly improving the search performance.\n\n\n8. Build index and load the collection before inserting more entities.\n\n```python\n# index\nindex_params = {\"index_type\": \"IVF_SQ8\", \"metric_type\": \"L2\", \"params\": {\"nlist\": 64}}\ncollection.create_index(field_name=embedding_field.name, index_params=index_params)\n# load\ncollection.load()\n```\n\n\n9. Insert 50,000 rows of entities 200 times (same batches of vectors are used for the sake of convenience, but this will not affect the result).\n\n```python\nimport random\n# insert data with customized ids\nnb = 50000\nids = [i for i in range(nb)]\nages = [random.randint(20, 40) for i in range(nb)]\nembeddings = [[random.random() for _ in range(dim)] for _ in range(nb)]\nentities = [ids, ages, embeddings]\nfor i in range(200):\n ins_res = collection.insert(entities)\n print(f\"insert entities primary keys: {ins_res.primary_keys}\")\n```\n\n\n10. Check the loading segment information in query node during and after the inserting. \n\n```python\n# did this in another python console\nutility.get_query_segment_info(\"hello_milmil_handoff\")\n```\n\n11. You will find that all sealed segments loaded to query node are indexed.\n\n```\n[segmentID: 430640405514551298\ncollectionID: 430640403705757697\npartitionID: 430640403705757698\nmem_size: 394463520\nnum_rows: 747090\nindex_name: \"_default_idx\"\nindexID: 430640403745079297\nnodeID: 7\nstate: Sealed\n, segmentID: 430640405514551297\ncollectionID: 430640403705757697\npartitionID: 430640403705757698\nmem_size: 397536480\nnum_rows: 752910\nindex_name: \"_default_idx\"\nindexID: 430640403745079297\nnodeID: 7\nstate: Sealed\n...\n```\n\n## What's more\n\nIn addition to the above functionalities, new features such as Data Compaction, Dynamic Load Balance, and more are introduced into Milvus 2.0. Please enjoy your exploratory journey with Milvus!\n\nIn the near future, we will share with you a series of blogs introducing the design of the new features in Milvus 2.0.\n- [Deletion](https://milvus.io/blog/2022-02-07-how-milvus-deletes-streaming-data-in-distributed-cluster.md)\n- [Data Compaction](https://milvus.io/blog/2022-2-21-compact.md)\n- [Dynamic Load Balance](https://milvus.io/blog/2022-02-28-how-milvus-balances-query-load-across-nodes.md)\n- [Bitset](https://milvus.io/blog/2022-2-14-bitset.md) \n\nFind us on:\n- [GitHub](https://github.com/milvus-io/milvus)\n- [Milvus.io](https://milvus.io/)\n- [Slack Channel](https://slack.milvus.io/)\n","title":"Milvus 2.0 - A Glimpse at New Features","metaData":{}},{"id":"2022-1-25-annoucing-general-availability-of-milvus-2-0.md","author":"Xiaofan Luan","desc":"An easy way to handle massive high-dimensional data","date":"2022-01-25T00:00:00.000Z","cover":"https://assets.zilliz.com/Milvus_2_0_GA_4308a0f552.png","tags":["News"],"href":"/blog/2022-1-25-annoucing-general-availability-of-milvus-2-0.md","content":"\nDear Members and Friends of the Milvus Community:\n\nToday, six months after the first Release Candidate (RC) was made public, we are thrilled to announce that Milvus 2.0 is [General Available (GA)](https://milvus.io/docs/v2.0.x/release_notes.md#v200) and production ready! It's been a long journey, and we thank everyone – community contributors, users, and the LF AI \u0026 Data Foundation – along the way who helped us make this happen.\n\nThe ability to handle billions of high dimensional data is a big deal for AI systems these days, and for good reasons:\n 1. Unstructured data occupy dominant volumes compared to traditional structured data.\n 2. Data freshness has never been more important. Data scientists are eager for timely data solutions rather than the traditional T+1 compromise.\n 3. Cost and performance have become even more critical, and yet there still exists a big gap between current solutions and real world use cases.\nHence, Milvus 2.0. Milvus is a database that helps handle high dimensional data at scale. It is designed for cloud with the ability to run everywhere. If you've been following our RC releases, you know we've spent great effort on making Milvus more stable and easier to deploy and maintain. \n\n## Milvus 2.0 GA now offers\n\n**Entity deletion**\n\nAs a database, Milvus now supports [deleting entities by primary key](https://milvus.io/docs/v2.0.x/delete_data.md) and will support deleting entities by expression later on.\n\n**Automatic load balance**\n\nMilvus now supports plugin load balance policy to balance the load of each query node and data node. Thanks to the disaggregation of computation and storage, the balance will be done in just a couple of minutes.\n\n**Handoff** \n\nOnce growing segments are sealed through flush, handoff tasks replace growing segments with indexed historical segments to improve search performance.\n\n**Data compaction**\n\nData compaction is a background task to merge small segments into large ones and clean logical deleted data. \n\n**Support embedded etcd and local data storage**\n\nUnder Milvus standalone mode, we can remove etcd/MinIO dependency with just a few configurations. Local data storage can also be used as a local cache to avoid loading all data into main memory.\n\n**Multi language SDKs**\n\nIn addition to [PyMilvus](https://github.com/milvus-io/pymilvus), [Node.js](https://github.com/milvus-io/milvus-sdk-node), [Java](https://github.com/milvus-io/milvus-sdk-java) and [Go](https://github.com/milvus-io/milvus-sdk-go) SDKs are now ready-to-use.\n\n**Milvus K8s Operator**\n\n[Milvus Operator](https://milvus.io/docs/v2.0.x/install_cluster-milvusoperator.md) provides an easy solution to deploy and manage a full Milvus service stack, including both Milvus components and its relevant dependencies (e.g. etcd, Pulsar and MinIO), to the target [Kubernetes](https://kubernetes.io/) clusters in a scalable and highly available manner.\n\n**Tools that help to manage Milvus**\n\nWe have [Zilliz](https://zilliz.com/) to thank for the fantastic contribution of management tools. We now have [Attu](https://milvus.io/docs/v2.0.x/attu.md), which allows us to interact with Milvus via an intuitive GUI, and [Milvus_CLI](https://milvus.io/docs/v2.0.x/cli_overview.md), a command-line tool for managing Milvus.\n\nThanks to all 212 contributors, the community finished 6718 commits during the last 6 months, and tons of stability and performance issues have been closed. We'll open our stability and performance benchmark report soon after the 2.0 GA release. \n\n## What's next?\n\n**Functionality**\n\nString type support will be the next killer features for Milvus 2.1. We will also bring in time to live (TTL) mechanism and basic ACL management to better satisfy user needs.\n\n**Availability**\n\nWe are working on refactoring the query coord scheduling mechanism to support multi memory replicas for each segment. With multiple active replicas, Milvus can support faster failover and speculative execution to shorten the downtime to within a couple of seconds.\n\n**Performance**\n\nPerformance benchmark results will soon be offered on our websites. The following releases are anticipated to see an impressive performance improvement. Our target is to halve the search latency under smaller datasets and double the system throughput.\n\n**Ease of use**\n\nMilvus is designed to run anywhere. We will support Milvus on MacOS (Both M1 and X86) and on ARM servers in the next few small releases. We will also offer embedded PyMilvus so you can simply `pip install` Milvus without complex environment setup.\n\n**Community governance**\n\nWe will refine the membership rules and clarify the requirements and responsibilities of contributor roles. A mentorship program is also under development; for anyone who is interested in cloud-native database, vector search, and/or community governance, feel free to contact us.\n\nWe’re really excited about the latest Milvus GA release! As always, we are happy to hear your feedback. If you encounter any problems, don't hesitate to contact us on [GitHub](https://github.com/milvus-io/milvus) or via [Slack](http://milvusio.slack.com/).\n\n\u003cbr/\u003e\n\nBest regards,\n\nXiaofan Luan \n\nMilvus Project Maintainer\n\n\u003cbr/\u003e\n\n\n\u003e *Edited by [Claire Yu](https://github.com/claireyuw).*\n","title":"Announcing General Availability of Milvus 2.0","metaData":{}},{"id":"2022-01-20-story-of-smartnews.md","author":"Milvus","desc":"Learn about the story of SmartNews, both a Milvus user and contributor.","date":"2022-01-20T00:00:00.000Z","cover":"https://assets.zilliz.com/Smartnews_user_to_contributor_f219e6e008.png","tags":["Scenarios"],"href":"/blog/2022-01-20-story-of-smartnews.md","content":"\nThis article is translated by [Angela Ni](https://www.linkedin.com/in/yiyun-n-2aa713163/).\n\n\nInformation is everywhere in our lives. Meta (formerly known as Facebook), Instagram, Twitter, and other social media platforms make information streams all the more ubiquitous. Therefore, engines dealing with such information streams have become a must-have in most system architecture. However, as a user of social media platforms and relevant apps, I bet you must have been bothered by duplicate articles, news, memes, and more. Exposure to duplicate content hampers the process of information retrieval and leads to bad user experience.\n\n\nFor a product dealing with information streams, it is a high priority for the developers to find a flexible data processor that can be integrated seamlessly into the system architecture to deduplicate identical news or advertisements.\n\n[SmartNews](https://www.smartnews.com/en/), valued at [2 billion US dollars](https://techcrunch.com/2021/09/15/news-aggregator-smartnews-raises-230-million-valuing-its-business-at-2-billion/), is the most highly-valued news app company in the US. Noticeably, it used to be a user of Milvus, an open-source vector database, but later transformed into an active contributor to the Milvus project.\n\nThis article shares the story of SmartNews and tells why it decided to make contributions to the Milvus project.\n\n## An overview of SmartNews\n\nSmartNews, founded in 2012, is headquartered in Tokyo, Japan. The news app developed by SmartNews has always been [top-rated](https://www.businessinsider.com/guides/smartnews-free-news-app-2018-9) in the Japanese market. SmartNews is the [fastest growing](https://about.smartnews.com/en/2019/06/12/smartnews-builds-global-momentum-with-over-500-us-growth-new-executives-and-three-new-offices/) news app and also boasts [high user viscosity](https://about.smartnews.com/en/2018/07/21/smartnews-reaches-more-than-10-million-monthly-active-users-in-the-united-states-and-japan/) in the US market. According to the statistics from [APP Annie](https://www.appannie.com/en/), the monthly average session duration of SmartNews ranked first among all news apps by the end of July, 2021, greater than the accumulated session duration of AppleNews and Google News.\n\nWith the rapid growth of user base and viscosity, SmartNews has to face more challenges in terms of recommendation mechanism and AI algorithm. Such challenges include utilizing massive discrete features in large-scale machine learning (ML), accelerating unstructured data query with vector similarity search, and more.\n\nAt the beginning of 2021, the dynamic Ad algorithm team at SmartNews sent a request to AI infrastructure team that the functions of recalling and querying advertisements need to be optimized. After two months of research, AI infrastructure engineer Shu decided to use Milvus, an open-source vector database that supports multiple indexes and similarity metrics and online data updates. Milvus is trusted by more than a thousand organizations worldwide.\n\n## Advertisement recommendation powered by vector similarity search\n\nThe open-source vector database Milvus is adopted in the SmartNews Ad system to match and recommend dynamic ads from a 10-milllion-scale dataset to its users. By doing so, SmartNews can create a mapping relationship between two previously unmatchable datasets - user data and advertisement data. In the second quarter of 2021, Shu managed to deploy Milvus 1.0 on Kubernetes. Learn more about how to [deploy Milvus](https://milvus.io/docs).\n\n\n\nAfter the successful deployment of Milvus 1.0, the first project to use Milvus was the advertisement recall project initiated by the Ad team at SmartNews. During the initial stage, the advertisement dataset was on a million scale. Meanwhile, the P99 latency was strictly controlled within less than 10 milliseconds.\n\nIn June, 2021, Shu and his colleagues in the algorithm team applied Milvus to more business scenarios and attempted data aggregation and online data/index update in real time.\n\nBy now, Milvus, the open-source vector database has been used in various business scenarios at SmartNews, including ad recommendation.\n\n## **From a user to an active contributor**\n\nWhile integrating Milvus into the Smartnews product architecture, Shu and other developers came up with requests of functions such as hot reload, item TTL (time-to-live), item update/replace, and more. These are also functions desired by many users in the Milvus community. Therefore, Dennis Zhao, head of the AI infrastructure team at SmartNews decided to develop and contribute the hot reload function to the community. Dennis believed that \"SmartNews team has been benefiting from the Milvus community, therefore, we are more than willing to contribute if we have something to share with the community.\"\n\nData reload supports code editing while running the code. With the help of data reload, developers no longer need to stop at a breakpoint or restart the application. Instead, they can edit the code directly and see the result in real time.\n\nIn late July, Yusup, engineer at SmartNews proposed an idea of using [collection alias](https://milvus.io/docs/v2.0.x/collection_alias.md#Collection-Alias) to achieve hot reload.\n\nCreating collection alias refers to specifying alias names for a collection. A collection can have multiple aliases. However, an alias corresponds to a maximum of one collection. Simply draw an analogy between a collection and a locker. A locker, like a collection, has its own number and position, which will always remain unchanged. However, you can always put in and draw out different things from the locker. Similarly, the name of the collection is fixed but the data in the collection is dynamic. You can always insert or delete vectors in a collection, as data deletion is supported in the Milvus [pre-GA version](https://milvus.io/docs/v2.0.x/release_notes.md#v200-PreGA).\n\nIn the case of SmartNews advertisement business, nearly 100 million vectors are inserted or updated as new dynamic ad vectors are generated. There are several solutions to this:\n\n- Solution 1: delete old data first and insert new ones.\n- Solution 2: create a new collection for new data.\n- Solution 3: use collection alias.\n\nFor solution 1, one of the most straightforward shortcoming is that it is extremely time-consuming, especially when the dataset to be updated is tremendous. It generally takes hours to update a dataset on a 100-million-scale.\n\nAs for solution 2, the problem is that the new collection is not immediately available for search. That is to say, a collection is not searchable during load. Plus, Milvus does not allow two collections to use the same collection name. Switching to a new collection would always require users to manually modify the client side code. That is to say, users have to revise the value of the parameter `collection_name` every time they need to switch between collections.\n\nSolution 3 would be the silver bullet. You only need to insert the new data in a new collection and use collection alias. By doing so, you only need to swap the collection alias every time you need to switch the collection to conduct the search. You do not need extra efforts to revise the code. This solution saves you the troubles mentioned in the previous two solutions.\n\nYusup started from this request and helped the whole SmartNews team understand the Milvus architecture. After one and half months, the Milvus project received a PR about hot reload from Yusup. And later, this function is officially available along with the release of Milvus 2.0.0-RC7.\n\nCurrently, the AI infrastructure team is taking the lead to deploy Milvus 2.0 and migrate all data gradually from Milvus 1.0 to 2.0.\n\n\n\n\nSupport for collection alias can greatly improve user experience, especially for those large Internet companies with great volumes of user requests. Chenglong Li, data engineer from the Milvus community, who helped build the bridge between Milvus and Smartnews, said, \"The collection alias function arises from the real business request of SmartNews, a Milvus user. And SmartNews contributed the code to the Milvus community. This act of reciprocity is a great example of the open-source spirit: from the community and for the community. We hope to see more contributors like SmartNews and jointly build a more prosperous Milvus community.\"\n\n\"Currently, part of the ad business is adopting Milvus as the offline vector database. The official release of Mivus 2.0 is approaching, and we hope that we can use Milvus to build more reliable systems and provide real-time services for more business scenarios.\" said Dennis.\n\n\u003e Update: Milvus 2.0 is now general available! [Learn more](2022-1-25-annoucing-general-availability-of-milvus-2-0.md)\n","title":"The Story of SmartNews - from a Milvus User to an Active Contributor","metaData":{}},{"id":"2022-01-07-year-in-review.md","author":"Xiaofan Luan","desc":"Learn what Milvus community has achieved and what's in store for year 2022.","date":"2022-01-07T00:00:00.000Z","cover":"https://assets.zilliz.com/Year_in_review_6deaee3a96.png","tags":["Events"],"href":"/blog/2022-01-07-year-in-review.md","content":"\n2021 was an amazing year for Milvus as an open-source project. I want to take a moment to thank all the contributors and users of Milvus, as well as partners for contributing to such an outstanding year.\n\n**One of the most impressive moments of this year for me is the release of Milvus 2.0. Before we started this project, only a few community members believed that we could deliver the most advanced vector database in the world , but now I am proud to say that Milvus 2.0 GA is production-ready.**\n\nWe’re already working on a new and exciting set of challenges for 2022, but I thought it would be fun to celebrate a couple of the big steps we took last year. Here are a few:\n\n\n## Community Growth\n\nFirst, here’s a summary of community statistics from GitHub and Slack. By the end of December 2021:\n\n- **Contributors** have increased from 121 in December 2020 to 209 in December 2021 (up 172%)\n\n- **Stars** have increased from 4828 in December 2020 to 9090 in December 2021 (up 188%)\n\n- **Forks** have increased from 756 in December 2020 to 1383 in December 2021 (up 182%)\n\n- **Slack members** have increased from 541 in December 2020 to 1233 in December 2021 (up 227%)\n\n\n\n\n## Community Governance and Advocacy\n\nWhen Milvus first went open source in Oct. 2019, we had a relatively small team and a small community, so naturally the project was mainly governed by a few core team members. But since then the community had grown significantly, we realized that we needed a better system to run the project so we could welcome new contributors more efficiently. \n\nAs a result, we have appointed 5 new maintainers in 2021 to keep track of the ongoing work and reported issues to make sure that they get reviewed and merged in a timely manner. The GitHub IDs of the five maintainers are @xiaofan-luan; @congqixia; @scsven; @czs007; @yanliang567. Please feel free to contact these maintainers if you need help with your PRs.\n\nWe've also launched the [Milvus Advocate Program](https://milvus.io/community/milvus_advocate.md), and we welcome more people to join us to share your experiences, offer help to community members and gain recognition in return.\n\n\n\n\n(Image: Milvus GitHub contributors, made with dynamicwebpaige's [project](https://github.com/dynamicwebpaige/nanowrimo-2021/blob/main/15_VS_Code_contributors.ipynb) )\n\n\n## Milvus Project Announcements and Milestones\n\n1. **Number of version releases:14**\n\n- [Milvus 1.0 release](https://milvus.io/blog/Whats-Inside-Milvus-1.0.md)\n- [Milvus 2.0 release RC](https://milvus.io/blog/milvus2.0-redefining-vector-database.md) \n- [Milvus 2.0 release PreGA](https://milvus.io/docs/v2.0.x/release_notes.md#v200-PreGA)\n\n1. **Milvus v2.0.0 GA supported SDKs**\n\n- PyMilvus (Available)\n\n- Go SDK (Available)\n\n- Java SDK (Available)\n\n- Node.js SDK (Available)\n\n- C++ SDK (Developing)\n\n3. **New Milvus tools launched:**\n\n- [Milvus_CLI](https://github.com/zilliztech/milvus_cli#community) (Milvus Command Line)\n- [Attu](https://github.com/zilliztech/attu) (Milvus Management GUI)\n- [Milvus K8s Operator](https://github.com/milvus-io/milvus-operator)\n\n4. **[Milvus became a graduation project of LF AI \u0026 Data Foundation.](https://lfaidata.foundation/blog/2021/06/23/lf-ai-data-foundation-announces-graduation-of-milvus-project/)**\n\n5. **[Milvus: A Purpose-Built Vector Data Management System published in SIGMOD'2021.](https://www.cs.purdue.edu/homes/csjgwang/pubs/SIGMOD21_Milvus.pdf))**\n\n6. **[Milvus Community Forum launched.](https://discuss.milvus.io/)**\n\n## Community Events\n\nWe have hosted and joined many events this year so our global community members can meet (mostly virtually) despite the current Covid-19 situation. In total, we have attended 21 conferences and hosted:\n\n- 6 Technical Meetings\n- 7 Milvus Office Hours\n- 34 Webinars\n- 3 Offline Meetups\n\nWe are planning for more events in 2022. If you want to join the events near you, please check the [Events and Meetup](https://discuss.milvus.io/c/events-and-meetups/13) category in our community forum to see the upcoming events and their locations. If you'd like to be our speaker or host for the future events, please contact us at [community@milvus.io](mailto:community@milvus.io).\n\n## Looking Ahead to 2022- Roadmap \u0026 Announcement\n\n**Community:**\n\n1. Improve Milvus Project Membership to attract/elect more maintainers and committers to build the community together.\n2. Launch Mentorship Program to offer more help to newcomers who want to join the community and contribute.\n3. Improve community document governance, including **Technical documents, user guides and community documents**. In 2022, hopefully our community members can complete a Milvus Handbook together so people can learn how to use Milvus better.\n4. Strengthen the cooperation and interaction with other open source communities, including upstream AI communities and communities like Kubernetes, MinIO, etcd and Pulsar which Milvus relies on.\n5. Become more community-driven by having more regular SIG meetings. Besides the sig-pymilvus that is currently running, our plan is to have more SIGs in 2022.\n\n\n**Milvus Project:**\n\n1. Performance tuning\n\nExcellent performance has always been an important reason why users choose Milvus. In 2022, we plan to start a performance optimization project to increase throughput and delay by at least twice. We also plan to introduce memory replicas to improve throughput and system stability under small data set, and support GPU to accelerate index building and online serving.\n\n2. Functionality\n\nMilvus 2.0 has already supported functionalities such as vector/scalar hybrid search, entity deletion and time travel. We plan to support the following features in the next two major releases:\n\n- Support for richer data types: String, Blob, Geospatial, etc. \n- Role-based access control\n- Primary key deduplication\n- Support for range search on vectors (search where distance \u003c 0.8)\n- Restful API support, and other language SDKs\n\n3. Ease of use\n\nIn the coming year we plan to develop several tools to help better deploy and manage Milvus.\n\n- Milvus up: A deployment component that helps users to bring up Milvus in an offline environment without K8s cluster. It also helps to deploy monitoring, tracing and other Milvus development.\n\n- Attu — We'll keep improving Attu as our cluster management system. We are planing to add functionalities such as health diagnosis and index optimization.\n\n- Milvus DM: Data migration tool for migrating vectors from other database or files to Milvus. We'll first support FAISS, HNSW, Milvus 1.0/2.0, then other databases such as MySQL and Elasticsearch.\n\n## About the author\n\nXiaofan Luan, partner and Engineering Director of Zilliz, and Technical Advisory Committee member of LF AI \u0026 Data Foundation. He worked successively in the Oracle US headquarters and Hedvig, a software defined storage startup. He joined Alibaba Cloud Database team and was in charge of the development of NoSQL database HBase and Lindorm. Luan obtained his master's degree in Electronic Computer Engineering from Cornell University.\n","title":"Milvus in 2021 - Year in Review","metaData":{}},{"id":"2021-12-31-get-started-with-milvus-cli.md","author":"Zhuanghong Chen and Zhen Chen","desc":"This article introduces Milvus_CLI and helps you complete common tasks.","recommend":true,"canonicalUrl":"https://zilliz.com/blog/get-started-with-milvus-cli","date":"2021-12-31T00:00:00.000Z","cover":"https://assets.zilliz.com/CLI_9a10de4fcc.png","tags":["Engineering"],"href":"/blog/2021-12-31-get-started-with-milvus-cli.md","content":"\nIn the age of information explosion, we are producing voice, images, videos, and other unstructured data all the time. How do we efficiently analyze this massive amount of data? The emergence of neural networks enables unstructured data to be embedded as vectors, and the Milvus database is a basic data service software, which helps complete the storage, search, and analysis of vector data.\n\nBut how can we use the Milvus vector database quickly?\n\nSome users have complained that APIs are hard to memorize and hope there could be simple command lines to operate the Milvus database.\n\nWe're thrilled to introduce Milvus_CLI, a command-line tool dedicated to the Milvus vector database.\n\nMilvus_CLI is a convenient database CLI for Milvus, supporting database connection, data import, data export, and vector calculation using interactive commands in shells. The latest version of Milvus_CLI has the following features.\n\n- All platforms supported, including Windows, Mac, and Linux\n\n- Online and offline installation with pip supported\n\n- Portable, can be used anywhere\n\n- Built on the Milvus SDK for Python\n\n- Help docs included\n\n- Auto-complete supported\n\n## Installation\n\nYou can install Milvus_CLI either online or offline.\n\n### Install Milvus_CLI online\n\nRun the following command to install Milvus_CLI online with pip. Python 3.8 or later is required.\n\n```\npip install milvus-cli\n```\n\n### Install Milvus_CLI offline\n\nTo install Milvus_CLI offline, [download](https://github.com/milvus-io/milvus_cli/releases) the latest tarball from the release page first.\n\n\n\nAfter the tarball is downloaded, run the following command to install Milvus_CLI.\n\n```\npip install milvus_cli-\u003cversion\u003e.tar.gz\n```\n\nAfter Milvus_CLI is installed, run `milvus_cli`. The `milvus_cli \u003e` prompt that appears indicates that the command line is ready.\n\n\n\nIf you're using a Mac with the M1 chip or a PC without a Python environment, you can choose to use a portable application instead. To accomplish this, [download](https://github.com/milvus-io/milvus_cli/releases) a file on the release page corresponding to your OS, run `chmod +x` on the file to make it executable, and run `./` on the file to run it.\n\n#### **Example**\n\nThe following example makes `milvus_cli-v0.1.8-fix2-macOS` executable and runs it.\n\n```\nsudo chmod +x milvus_cli-v0.1.8-fix2-macOS\n./milvus_cli-v0.1.8-fix2-macOS\n```\n\n## Usage\n\n### Connect to Milvus\n\nBefore connecting to Milvus, ensure that Milvus is installed on your server. See [Install Milvus Standalone](https://milvus.io/docs/v2.0.x/install_standalone-docker.md) or [Install Milvus Cluster](https://milvus.io/docs/v2.0.x/install_cluster-docker.md) for more information.\n\nIf Milvus is installed on your localhost with the default port, run `connect`.\n\n\n\nOtherwise, run the following command with the IP address of your Milvus server. The following example uses `172.16.20.3` as the IP address and `19530` as the port number.\n\n```\nconnect -h 172.16.20.3\n```\n\n\n\n### Create a collection\n\nThis section introduces how to create a collection.\n\nA collection consists of entities and is similar to a table in RDBMS. See [Glossary](https://milvus.io/docs/v2.0.x/glossary.md) for more information.\n\n\n\n#### Example\n\nThe following example creates a collection named `car`. The `car` collection has four fields which are `id`, `vector`, `color`, and `brand`. The primary key field is `id`. See [create collection](https://milvus.io/docs/v2.0.x/cli_commands.md#create-collection) for more information.\n\n```\ncreate collection -c car -f id:INT64:primary_field -f vector:FLOAT_VECTOR:128 -f color:INT64:color -f brand:INT64:brand -p id -a -d 'car_collection'\n```\n\n### List collections\n\nRun the following command to list all collections in this Milvus instance.\n\n```\nlist collections\n```\n\n\n\nRun the following command to check the details of the `car` collection.\n\n```\ndescribe collection -c car \n```\n\n\n\n### Calculate the distance between two vectors\n\nRun the following command to import data into the `car` collection.\n\n```\nimport -c car 'https://raw.githubusercontent.com/zilliztech/milvus_cli/main/examples/import_csv/vectors.csv'\n```\n\n\n\nRun `query` and enter `car` as the collection name and `id\u003e0` as the query expression when prompted. The IDs of the entities that meet the criteria are returned as shown in the following figure.\n\n\n\n\nRun `calc` and enter appropriate values when prompted to calculate the distances between vector arrays.\n\n### Delete a collection\n\nRun the following command to delete the `car` collection.\n\n```\ndelete collection -c car\n```\n\n\n\n## More\n\nMilvus_CLI is not limited to the preceding functions. Run `help` to view all commands that Milvus_CLI includes and the respective descriptions. Run `\u003ccommand\u003e --help` to view the details of a specified command.\n\n\n\n**See also:**\n\n[Milvus_CLI Command Reference](https://milvus.io/docs/v2.0.x/cli_commands.md) under Milvus Docs\n\nWe hope Milvus_CLI could help you easily use the Milvus vector database. We will keep optimizing Milvus_CLI and your contributions are welcome.\n\nIf you have any questions, feel free to [file an issue](https://github.com/zilliztech/milvus_cli/issues) on GitHub.\n","title":"Get started with Milvus_CLI","metaData":{}},{"id":"2021-12-21-milvus-2.0.md","author":"Jun Gu","desc":"The thinking process of how we designed the new Milvus database cluster architecture.","date":"2021-12-21T00:00:00.000Z","cover":"https://assets.zilliz.com/Evolution_dd677ce3be.png","tags":["Engineering"],"href":"/blog/2021-12-21-milvus-2.0.md","content":"\n\u003eIn this article, we will share the thinking process of how we designed the new Milvus database cluster architecture.\n\n## Objectives of Milvus vector database\n\nWhen the idea of the [Milvus vector database](https://github.com/milvus-io/milvus) first came to our minds, we wanted to build a data infrastructure that could help people accelerate AI adoptions in their organizations.\n\nWe have set two crucial objectives for the Milvus project to fulfill this mission.\n\n### Ease of use\n\nAI/ML is an emerging area where new technologies keep coming out. Most developers are not entirely familiar with the fast-growing technologies and tools of AI. Developers have already consumed most of their energies finding, training, and tuning the models. It's hard for them to spend additional efforts handling the large amounts of embedding vectors generated by the models. Not to mention the manipulation of a large volume of data is always a very challenging task.\n\nThus we give \"ease of use\" a very high priority since it could significantly reduce the development cost.\n\n### Low running costs\n\nOne of the primary hurdles of AI in production is to justify the return of investment. We would have more opportunities to put our AI applications into production with lower running costs. And it would be conducive to lifting the margin of potential benefits.\n\n### Design principles of Milvus 2.0\n\nWe made a start towards these goals in Milvus 1.0. But it's far from enough, especially in scalability and availability. Then we started the development of Milvus 2.0 to improve these points. The principles we have laid down for this new version include:\n\n* Aiming for high scalability and availability\n* Building upon mature cloud infrastructure and practice\n* Minimum performance compromise in the cloud\n\nIn other words, we want to make the Milvus database cluster cloud-native.\n\n## The evolution of database clusters\n\nThe vector database is a new species of database, as it handles new types of data (vectors). But it still shares the same challenges as other databases, with some of its own requirements. In the rest of this article, I will focus on what we have learned from the existing database cluster implementations and the thinking process of how we designed the new Milvus group architecture.\n\nIf you are interested in the implementation details of Milvus group components, please stay on top of the Milvus documentation. We will continuously publish technical articles in the Milvus GitHub repo, Milvus website, and Milvus Blog.\n\n### The ideal database cluster\n\n\u003e\"Aim small, miss small.\"\n\nLet's first list the critical capabilities an **ideal** database cluster should have.\n\n1. Concurrency and no single point of failure: users connected to different group members can simultaneously have read/write access to the same piece of data.\n2. Consistency: different group members should see the same data.\n3. Scalability: we can add or remove group members on the go.\n\nHonestly, all of these capabilities are hard to acquire together. In the modern implementations of database clusters, people have to compromise some of these capabilities. People don't expect a perfect database cluster as long as it can fit into the user scenarios. However, the shared-everything cluster was once very close to an ideal database cluster. If we want to learn something, we should start from here.\n\n### The key considerations of a database cluster\n\nThe shared-everything cluster has a more extended history compared to other modern implementations. Db2 data sharing group and Oracle RAC are typical of shared-everything clusters. Many people think shared-everything means sharing disks. It's far more than that.\n\nA shared-everything cluster only has one kind of database member in the group. Users could connect to any one of these symmetric members to access any data. What is \"everything\" that needs to be shared for making this work?\n\n#### The sequence of events in the group\n\nFirst, the group event sequence is crucial to resolve the potential conflicts caused by the concurrent access from different groups members. We usually use the database log record sequence number to represent the event sequence. At the same time, the log record sequence number is generally generated from the timestamp.\n\nThus the requirement of group event sequence is equal to the need of a global timer. If we could have an atomic clock for the group, that would be fabulous. Yet, Milvus is an open-source software project, which means we should rely on commonly available resources. To date, an atomic clock is still a premium option for large companies.\n\nWe have implemented the time synchronization component in Milvus 2.0 database cluster. You can find the link in the appendix.\n\n#### Global locking\n\nThe database has a locking mechanism to resolve concurrent access conflicts, whether optimistic or pessimistic locks. Similarly, we need global locking to resolve simultaneous access conflicts across different group members.\n\nGlobal locking means different group members have to talk with each other to negotiate the lock requests. Several vital factors would impact the efficiency of this global lock negotiation process:\n\n* The speed of inter-system connections\n* The number of group members who need to participate in the negotiation process\n* The frequency of group conflicts\n\nThe typical group size is no more than 100. For example, Db2 DSG is 32; Oracle RAC is 100. Those group members will be placed in one server room connected with optical fiber to minimize transfer latency. That's why it is sometimes called a centralized cluster. Due to the group size limitation, people will choose high-end servers (mainframes or minicomputers, which have much more capacity in CPU, memory, I/O channels, etc.) to consist of the shared-everything clusters.\n\nThis hardware presumption has dramatically changed in the modern cloud environment. Nowadays, cloud data centers comprise high-dense server rooms full of (thousands of) commodity X86 servers with TCP/IP connections. If we rely on these X86 servers to build the database cluster, the group size should increase to hundreds of (even thousands of) machines. And in some business scenarios, we will want these hundreds of X86 machines to spread in different regions. Thus implementing global locking might not be worth it anymore, as the global locking performance will not be good enough.\n\nIn Milvus 2.0, we are not going to implement the global locking facility. On the one hand, there is no update for vector data. (People should rather delete-then-insert instead of update.) So we don't need to worry about the multi-writer conflicts on the same piece of data in the Milvus group with sharding arrangement. Meantime, we could use MVCC (multi-version concurrency control, a lock-avoidance concurrency control method) to resolve the reader-writer conflicts.\n\nOn the other hand, vector data processing consumes a much higher memory footprint than structured data processing. People are looking for much higher scalability in vector databases.\n\n#### Shared in-memory data cache\n\nWe can briefly divide a database engine into two parts: the storage engine and the computing engine. The storage engine is responsible for two critical tasks:\n\n* Write data to permanent storage for durability purposes.\n* Load data from the permanent storage to the in-memory data cache (AKA buffer pool); this is the only place where the computing engine accesses data.\n\nIn the database cluster scenario, what if member A has updated the data cached in member B? How could member B know its in-memory data is expired? The classic shared-everything cluster has a buffer cross invalidation mechanism to resolve this issue. The buffer cross invalidation mechanism will work similarly to global locking if we maintain a strong consistency across the group members. As stated before, it is not practical in the modern cloud environment. **So we decided to lower the consistency level in the Milvus cloud-scalable group to an eventual consistency manner.** In this way, the buffer cross invalidation mechanism in Milvus 2.0 can be an asynchronous process.\n\n#### Shared storage\n\nShared storage is probably the first thing people would think about when discussing a database cluster.\n\nStorage options have also significantly changed in recent years of cloud storage evolution. The storage attached network (SAN) was (and still is) the storage foundation of the shared-everything group. But in the cloud environment, there is no SAN. The database has to use the local disk attached to the cloud virtual machines. Using local disk introduces the challenge of data consistency across the group members. And we also have to worry about the high availability of the group members.\n\nThen Snowflake made a great role model for cloud databases using cloud shared storage (S3 storage). It inspires Milvus 2.0 too. As stated before, we intend to rely on mature cloud infrastructure. But before we could utilize cloud shared storage, we have to think about a couple of things.\n\nFirst, S3 storage is cheap and reliable, but it is not designed for instant R/W access like database scenarios. We need to create the data components (which we call data nodes in Milvus 2.0) to bridge the local memory/disk and S3 storage. There are some examples (like Alluxio, JuiceFS, etc.) we could learn. The reason we can not integrate these projects directly is we focus on different data granularity. Alluxio and JuiceFS are designed for datasets or POSIX files, while we focus on the data record (vector) level.\n\nWhen the vector data is settled on S3 storage, the answer for metadata is easy: store them in ETCD. How about the log data, then? In the classic implementations, the log store is also based on SAN. The log files of one database group member are shared within the database cluster for failure recovery purposes. So this was not a problem until we got into the cloud environment.\n\nIn the Spanner paper, Google illustrated how they implemented the globally-distributed database (group) with Paxos consensus algorithm. You need to program the database cluster as a state machine replication group. The redo log is usually the \"state\" that will be replicated across the group. \n\nRedo-log replication by consensus algorithms is a powerful tool, and it has substantial advantages in some business scenarios. But for the Milvus vector database, we don't find enough incentives for creating a state machine replication group as a whole. We decided to use the cloud messaging queue/platform (Apache Pulsar, Apache Kafka, etc.) as an alternative cloud shared storage for the log store. By delegating the log store to the messaging platform, we acquire the benefits below.\n\n* The group is more event-driven, which means many processes can be asynchronous. It improves scalability.\n* The components are more loosely coupled, making it much easier to perform online rolling upgrades. It improves availability and operability.\n\nWe will revisit this topic in the later section.\n\nSo far, we have wrapped up the crucial considerations of the database cluster. Before we can jump to the discussion on the Milvus 2.0 architecture, let me first explain how we manage vectors in Milvus.\n\n### Data management and performance predictability\n\nMilvus stores vectors in collections. The \"collection\" is a logical concept, equivalent to a \"table\" in SQL databases. A \"collection\" could have multiple physical files to keep vectors. A physical file is a \"segment\". The \"segment\" is a physical concept like a tablespace file in SQL databases. When the data volume is small, we can save everything in a single segment/physical file. But nowadays, we are constantly facing big data. When there are multiple segments/physical files, how should we spread the data in different data partitions?\n\nAlthough data comes first rather than indexes, we have to store data in the way that the index algorithm prefers to make the data access efficiently in most cases. A frequently used strategy in SQL databases is partition by the range of partitioning key values. People usually create a clustered index to enforce the partitioning key. Overall, this is a decent approach for SQL databases. Data is stored in good shape, optimized for I/O (prefetch). But there are still defects.\n\n* Data skew. Some of the partitions might have much more data than others. The distribution of real-world data is not as simple as the numeric range.\n* Access hotspots. More workload might go to some of the data partitions.\n\nImagine more workload goes to partitions with more data. We need to rebalance the data across the partitions when these situations occur. (This is a DBA's tedious daily life.)\n\n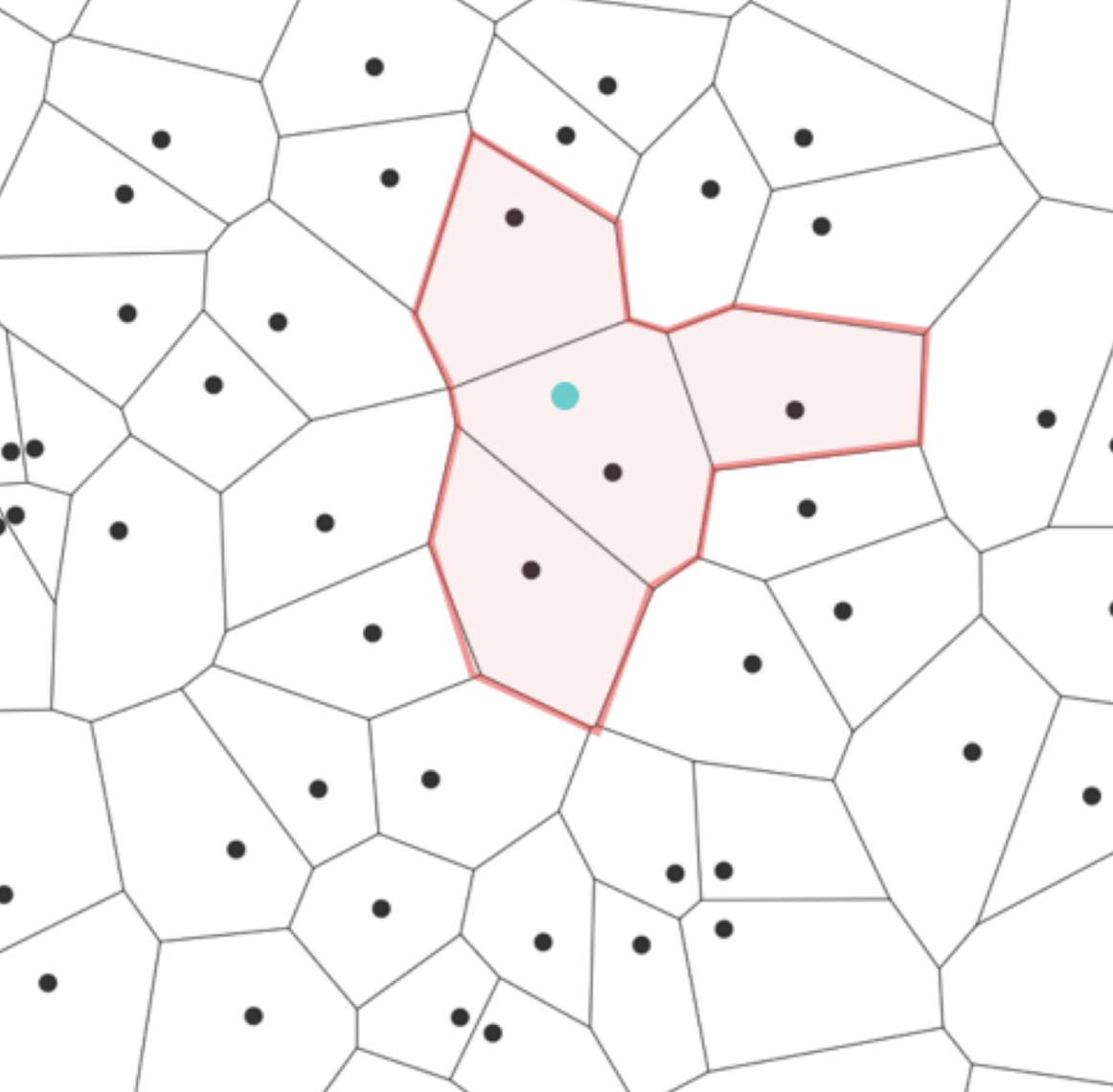\n\n\nWe can also create a clustered index for vectors (an inverted list index). But that is not the same case as SQL databases. Once the index is built in SQL databases, it's very efficient to access the data through the index, with less computation and less I/O operations. But for vector data, there will be far more computation and I/O operations even with an index. So the defects mentioned before will have a more severe impact on vector database clusters. Moreover, the cost of rebalancing vectors across different segments is very high due to the data volume and computing complexity.\n\nIn Milvus, we use the strategy of partition by growth. When we inject data into a vector collection, Milvus will append the new vectors to the latest segment in the collection. Milvus will close the segment once its size is large enough (the threshold is configurable) and build the index for the closed segment. In the meantime, a new segment will be created to store the upcoming data. This simple strategy is more balanced for vector processing.\n\nThe vector query is a process to search for the most similar candidates in the vector collection. It is a typical MapReduce procedure. For example, we want to search the top 20 similar results from a vector collection with ten segments. We can search the top 20 on each one of the segments and then merge the 20 * 10 results into the final 20 results. Since each segment has the same amount of vectors and a similar index, the processing time on each segment is almost identical. It gives us the advantage of performance predictability, which is essential when planning the scale of the database clusters.\n\n### New paradigms in Milvus 2.0\n\nIn Milvus 1.0, we implemented a read/write splitting sharding group like most SQL databases. It was a good attempt at scaling the Milvus database cluster. But the problems are quite obvious too.\n\n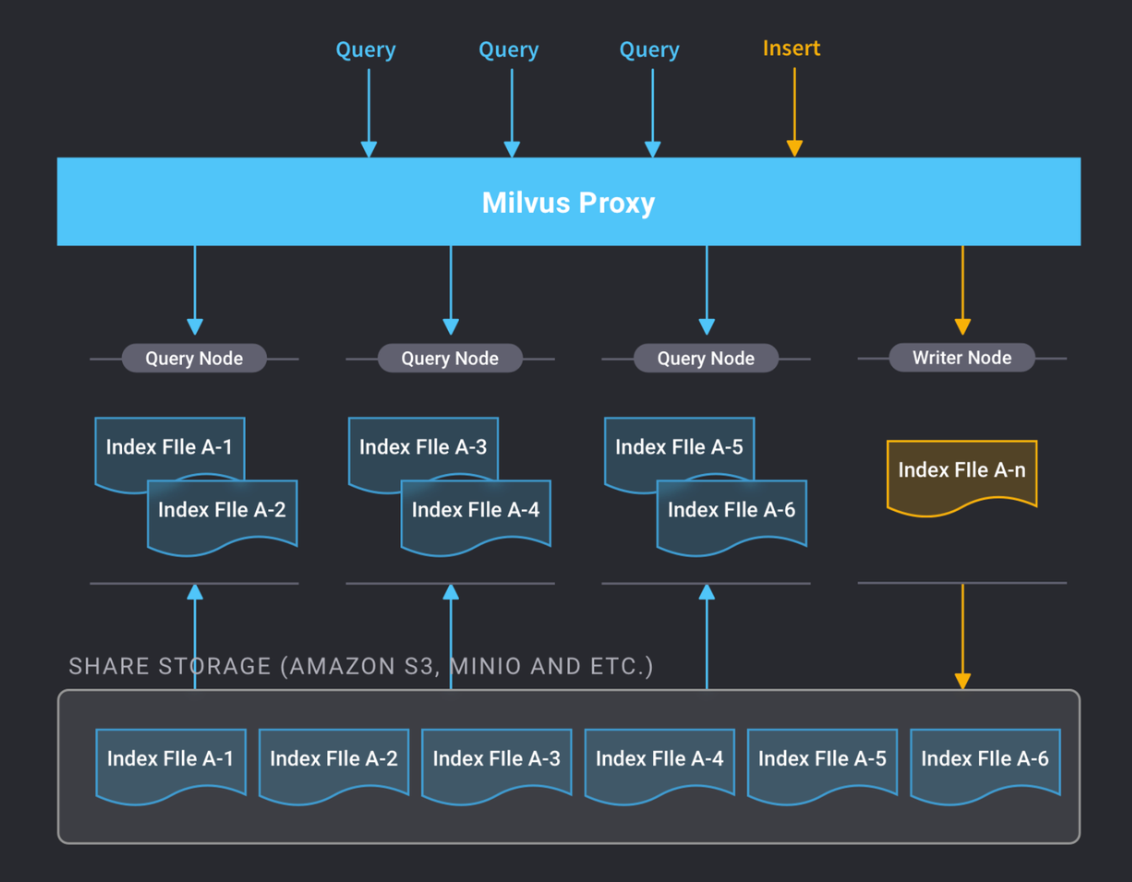\n\nIn Milvus 1.0, the R/W node has to take total care of the latest segment, including vector appending, searching in this unindexed segment, building index, etc. Since each collection only has one writer, the writer is very busy if the data is continuously streamed into the system. The performance of data sharing between the R/W node and the reader nodes is also a problem. Besides, we must either rely on NFS (not stable) or premium cloud storage (too expensive) for shared data storage.\n\nThese existing problems are hard to tackle in the Milvus 1.0 architecture. Thus, we have introduced new paradigms into the Milvus 2.0 design to resolve these issues.\n\n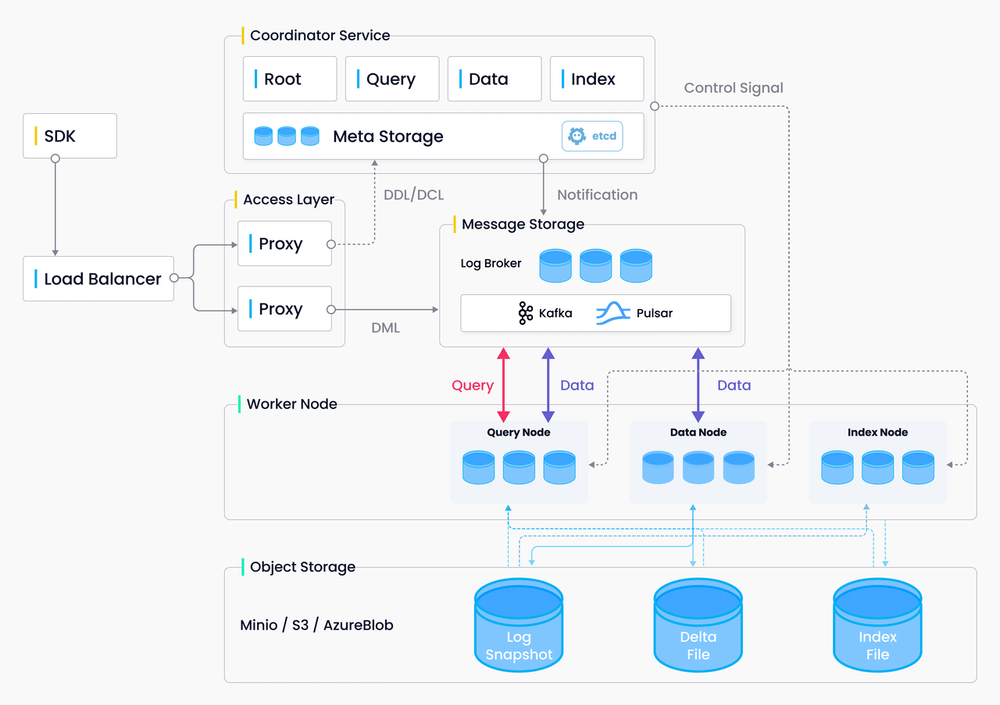\n\n#### Actor model\n\nThere are two models to program concurrent computation systems.\n\n* Shared memory that means concurrency control (locking) and synchronous processing\n* The actor model (AKA message passing) means message-driven and asynchronous processing\n\nWe can also apply these two models in distributed database clusters.\n\nAs stated before, most high-profile distributed databases use the same method: redo-log replication by consensus algorithms. This is synchronous processing using consensus algorithms to build a distributed shared memory for redo-log records. Different companies and venture capitals have invested billions of bucks in this technology. I didn't want to comment on this until we started to work on Milvus 2.0. Many people regard this technology as the only way to realize distributed database systems. This is annoying. If I don't say something, people might misunderstand that we were reckless in distributed database design.\n\nIn recent years, Redo-log replication by consensus algorithms has been the most overestimated database technology. There are two key issues.\n\n* The presumption that redo-log replication is better is fragile.\n* Vendors mislead people's expectations on the capability of consensus algorithms.\n\nLet's say we have two database nodes, the source node, and the target node. In the ever beginning, they have the exact copy of the data. We have some change operations (I/U/D SQL statements) on the source node, and we want to keep the target node updated. What should we do? The simplest way is to replay the operations on the target node. But this is not the most efficient way.\n\nThinking about the running cost of an I/U/D statement, we can divide it into the execution preparation and the physical work parts. The execution preparation part includes the work of SQL parser, SQL optimizer, etc. No matter how many data records will be affected, it is a fixed cost. The cost of the physical work part depends on how many data records will be affected; it is a floating cost. The idea behind redo-log replication is to save the fixed cost on the target node; we only replay the redo-log (the physical work) on the target node.\n\nThe cost-saving percentage is the reciprocal of the number of redo-log records. If one operation only affects one record, I should see significant savings from redo-log replication. What if it's 10,000 records? Then we should worry about the network reliability. Which one is more reliable, send the one operation or the 10,000 redo-log records? How about one million records? Redo-log replication is super in scenarios like payment systems, metadata systems, etc. In these scenarios, each database I/U/D operation only affects a small number of records (1 or 2). But it's hard to work with I/O intensive workloads like batch jobs.\n\nVendors always claim consensus algorithms could provide strong consistency to the database clusters. But people only use consensus algorithms to replicate the redo-log records. The redo-log records are consistent on different nodes, but that doesn't mean the data views on other nodes are consistent either. We have to merge the redo-log records into the actual table records. So even with this synchronous processing, we can still only get eventual consistency on the data views.\n\nWe should use redo-log replication by consensus algorithms in the appropriate places. The metadata system (ETCD) and messaging platform (e.g., Apache Pulsar) used in Milvus 2.0 have implemented consensus algorithms. But as I said before, \"for the Milvus vector database, we don't find enough incentives for being a state machine replication group as a whole.\"\n\nIn Milvus 2.0, we use the actor model to organize the worker nodes. The worker nodes are lonely. They only talk to the messaging platform, getting commands and sending results. It sounds boring.\n\n\u003e “What is our motto?\"\n\u003e “Boring is always best.”\n\u003e -- The Hitman's Bodyguard (2017)\n\nThe actor model is asynchronous. It is suitable for scalability and availability. Since the worker nodes don't know each other, there is no impact on other worker nodes if some of the worker nodes join or are removed.\n\n#### Separation of availability and durability\n\nIn Milvus 2.0, we do operation replay rather than log replay, because in the vector database, there is not much difference between operation replay and log replay. We don't have the Update function nor the Insert with Select function. And it's also much easier to do operation replay with the actor model.\n\nSo multiple worker nodes might execute the same operation from the messaging platform according to their responsibility. I mentioned before we decided to use the S3 cloud storage as the shared storage layer of the Milvus database cluster. The S3 storage is very reliable. Then is it necessary for different worker nodes to write out the same data to the shared storage?\n\nThus we designed three roles for the worker nodes.\n\n* The query node maintains an in-memory data view according to the assignment. The work of the query node includes doing vector search and keeping the in-memory data updated. But it doesn't need to write anything to the S3 storage. It is the most memory-sensitive node in the group.\n* The data node is responsible for writing the new data to the S3 storage. The data node doesn't need to maintain the in-memory data view, so the hardware configuration of the data node is quite different from the query node.\n* The index node builds indexes for the segments closed by the data node when the size of the segments reaches the threshold. This is the most CPU-intensive work in the group.\n\nThese three types of nodes represent different kinds of workload. They can scale independently. We call it separation of availability and durability learned from the Microsoft Socrates cloud database.\n\n## The end, also the beginning\n\nThis article has reviewed several design decisions of Milvus vector database 2.0. Let's quickly wrap up those points here.\n\n* We have chosen the eventual consistency for Milvus cluster 2.0.\n* We have integrated the mature cloud components into Milvus 2.0 as much as we can. We have controlled the new components introduced by Milvus 2.0 into users' production environments.\n* By following the actor model and separation of availability and durability, Milvus 2.0 is easy to scale in the cloud environment.\n\nSo far, we have formed the backbone of the Milvus 2.0 cloud-scalable database, but our backlog contains many requirements from the Milvus community that need to be satisfied. If you have the same mission (\"Build more open-source infrastructure software to accelerate the AI transformation\"), welcome to join the Milvus community.\n\nMilvus is a graduation project of the LF AI \u0026 Data foundation. You do NOT need to sign any CLA for Milvus!\n\n## Appendix\n\n### Milvus design doc\n\n[https://github.com/milvus-io/milvus/tree/master/docs/design_docs](https://github.com/milvus-io/milvus/tree/master/docs/design_docs)\n\n* [Milvus Time Synchronization](https://github.com/milvus-io/milvus/blob/master/docs/design_docs/20211215-milvus_timesync.md)\n\n### Raft implementation in C++\n\nIf you are still interested in the consensus algorithm, I suggest you check [eBay's open-source project Gringofts](https://github.com/eBay/Gringofts). It's a C++ implementation of the Raft consensus algorithm (a variant of the Paxos family). My friend Jacky and Elvis (my ex-colleagues at Morgan Stanley) built it for the eBay online payment system, which is precisely one of the most suitable scenarios for this technology.\n","title":"Evolution of Milvus Cloud-scalable Vector Database","metaData":{}},{"id":"2021-12-10-image-based-trademark-similarity-search-system-a-smarter-solution-to-ip-protection.md","author":"Zilliz","desc":"Learn how to apply vector similarity search in the industry of IP protection.","date":"2021-12-10T00:00:00.000Z","cover":"https://assets.zilliz.com/IP_protection_0a33547579.png","tags":["Scenarios"],"href":"/blog/2021-12-10-image-based-trademark-similarity-search-system-a-smarter-solution-to-ip-protection.md","content":"\nIn recent years, the issue of IP protection has come under the limelight as people's awareness of IP infringement is ever-increasing. Most notably, the multi-national technology giant Apple Inc. has been actively [filing lawsuits against various companies for IP infringement](https://en.wikipedia.org/wiki/Apple_Inc._litigation), including trademark, patent, and design infringement. Apart from those most notorious cases, Apple Inc. also [disputed a trademark application by Woolworths Limited](https://www.smh.com.au/business/apple-bites-over-woolworths-logo-20091005-ghzr.html), an Australian supermarket chain, on the grounds of trademark infringement in 2009. Apple. Inc argued that the logo of the Australian brand, a stylized \"w\", resembles their own logo of an apple. Therefore, Apple Inc. took objection to the range of products, including electronic devices, that Woolworths applied to sell with the logo. The story ends with Woolworths amending its logo and Apple withdrawing its opposition.\n\n\n\n\n\n\n\n\nWith the ever-increasing awareness of brand culture, companies are keeping a closer eye on any threats that will harm their intellectual properties (IP) rights. IP infringement includes:\n\n- Copyright infringement\n- Patent infringement\n- Trademark infringement\n- Design infringement\n- Cybersquatting\n\nThe aforementioned dispute between Apple and Woolworths is mainly over trademark infringement, precisely the similarity between the trademark images of the two entities. To refrain from becoming another Woolworths, an exhaustive trademark similarity search is a crucial step for applicants both prior to the filing as well as during the review of trademark applications. The most common resort is through a search on the [United States Patent and Trademark Office (USPTO) database](https://tmsearch.uspto.gov/search/search-information) that contains all of the active and inactive trademark registrations and applications. Despite the not so charming UI, this search process is also deeply flawed by its text-based nature as it relies on words and Trademark Design codes (which are hand annotated labels of design features) to search for images.\n\n.\")\n\n\nThis article thereby intends to showcase how to build an efficient image-based trademark similarity search system using [Milvus](https://milvus.io), an open-source vector database.\n\n## A vector similarity search system for trademarks\n\nTo build a vector similarity search system for trademarks, you need to go through the following steps:\n\n1. Prepare a massive dataset of logos. Likely, the system can use a dataset like [this](https://developer.uspto.gov/product/trademark-24-hour-box-and-supplemental),).\n2. Train an image feature extraction model using the dataset and data-driven models or AI algorithms.\n3. Convert logos into vectors using the trained model or algorithm in Step 2.\n4. Store the vectors and conduct vector similarity searches in Milvus, the open-source vector database.\n\n\n\n\nIn the following sections, let's take a closer look at the two major steps in building a vector similarity search system for trademarks: using AI models for image feature extraction, and using Milvus for vector similarity search. In our case, we used VGG16, a convolutional neural network (CNN), to extract image features and convert them into embedding vectors.\n\n### Using VGG16 for image feature extraction\n\n[VGG16](https://medium.com/@mygreatlearning/what-is-vgg16-introduction-to-vgg16-f2d63849f615) is a CNN designed for large-scale image recognition. The model is quick and accurate in image recognition and can be applied to images of all sizes. The following are two illustrations of the VGG16 architecture.\n\n\n\n\n\nThe VGG16 model, as its name suggests, is a CNN with 16 layers. All VGG models, including VGG16 and VGG19, contain 5 VGG blocks, with one or more convolutional layers in each VGG block. And at the end of each block, a max pooling layer is connected to reduce the size of the input image. The number of kernels is equivalent within each convolutional layer but doubles in each VGG block. Therefore, the number of kernels in the model grows from 64 in the first block, to 512 in the fourth and fifth blocks. All the convolutional kernels are 3*3-sized while the pooling kernels are all 2*2-sized. This is conducive to preserving more information about the input image.\n\nTherefore, VGG16 is a suitable model for image recognition of massive datasets in this case. You can use Python, Tensorflow, and Keras to train an image feature extraction model on the basis of VGG16.\n\n### Using Milvus for vector similarity search\n\nAfter using the VGG16 model to extract image features and convert logo images into embedding vectors, you need to search for similar vectors from a massive dataset. \n\nMilvus is a cloud-native database featuring high scalability and elasticity. Also, as a database, it can ensure data consistency. For a trademark similarity search system like this, new data like the latest trademark registrations are uploaded to the system in real time. And these newly uploaded data need to be available for search immediately. Therefore, this article adopts Milvus, the open-source vector database, to conduct vector similarity search.\n\nWhen inserting the logo vectors, you can create collections in Milvus for different types of logo vectors according to the [International (Nice) Classification of Goods and Services](https://en.wikipedia.org/wiki/International_(Nice)_Classification_of_Goods_and_Services), a system of classifying goods and services for registering trademarks. For example, you can insert a group of vectors of clothing brand logos into a collection named \"clothing\" in Milvus and insert another group of vectors of technological brand logos into a different collection named \"technology\". By doing so, you can greatly increase the efficiency and speed of your vector similarity search.\n\nMilvus not only supports multiple indexes for vector similarity search, but also provides rich APIs and tools to facilitate DevOps. The following diagram is an illustration of the [Milvus architecture](https://milvus.io/docs/v2.0.x/architecture_overview.md). You can learn more about Milvus by reading its [introduction](https://milvus.io/docs/v2.0.x/overview.md).\n\n\n\n\n## Looking for more resources?\n\n- Build more vector similarity search systems for other application scenarios with Milvus:\n - [DNA Sequence Classification based on Milvus](https://milvus.io/blog/dna-sequence-classification-based-on-milvus.md)\n - [Audio Retrieval Based on Milvus](https://milvus.io/blog/audio-retrieval-based-on-milvus.md)\n - [4 Steps to Building a Video Search System](https://milvus.io/blog/building-video-search-system-with-milvus.md)\n - [Building an Intelligent QA System with NLP and Milvus](https://milvus.io/blog/building-intelligent-chatbot-with-nlp-and-milvus.md)\n - [Accelerating New Drug Discovery](https://milvus.io/blog/molecular-structure-similarity-with-milvus.md)\n\n- Engage with our open-source community:\n - Find or contribute to Milvus on [GitHub](https://bit.ly/307b7jC).\n - Interact with the community via [Forum](https://bit.ly/3qiyTEk).\n - Connect with us on [Twitter](https://bit.ly/3ob7kd8).\n","title":"Milvus in IP Protection:Building a Trademark Similarity Search System with Milvus","metaData":{}},{"id":"2021-12-03-why-to-choose-fastapi-over-flask.md","author":"Yunmei","desc":"choose the appropriate framework according to your application scenario","isPublish":false,"date":"2021-12-03T00:00:00.000Z","cover":"https://assets.zilliz.com/1_d5de035def.png","tags":["Engineering"],"href":"/blog/2021-12-03-why-to-choose-fastapi-over-flask.md","content":"\nTo help you quickly get started with Milvus, the open-source vector database, we released another affiliated open-source project, [Milvus Bootcamp](https://github.com/milvus-io/bootcamp) on GitHub. The Milvus Bootcamp not only provides scripts and data for benchmark tests, but also includes projects that use Milvus to build some MVPs (minimum viable products), such as a reverse image search system, a video analysis system, a QA chatbot, or a recommender system. You can learn how to apply vector similarity search in a world full of unstructured data and get some hands-on experience in Milvus Bootcamp.\n\n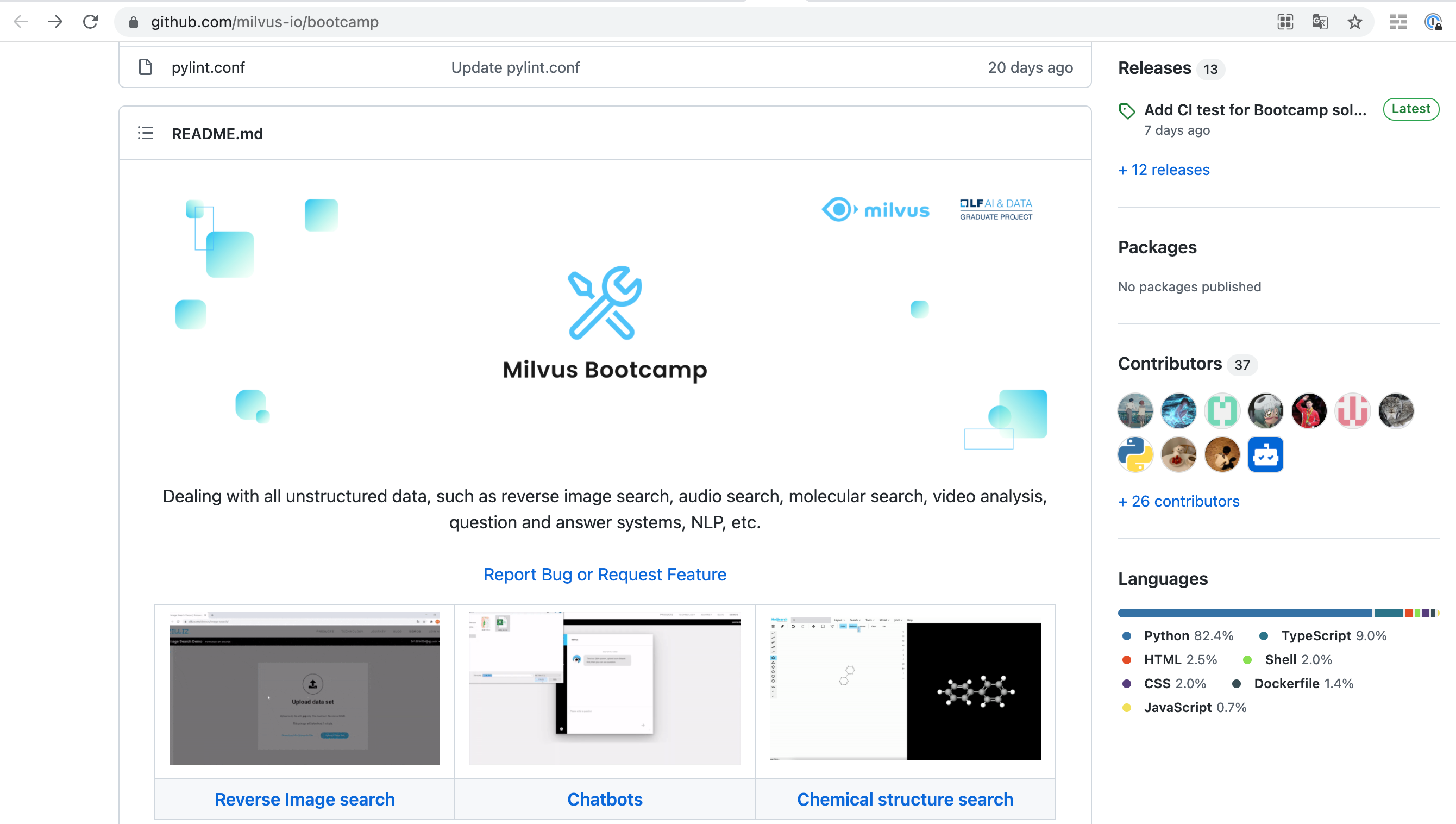\n\nWe provide both front-end and back-end services for the projects in Milvus Bootcamp. However, we have recently made the decision to change the adopted web framework from Flask to FastAPI.\n\nThis article aims to explain our motivation behind such a change in the adopted web framework for Milvus Bootcamp by clarifying why we chose FastAPI over Flask.\n\n## Web frameworks for Python\n\nA web framework refers to a collection of packages or modules. It is a set of software architecture for web development that allows you to write web applications or services and saves you the trouble of handling low-level details such as protocols, sockets, or process/thread management. Using web framework can significantly reduce the workload of developing web applications as you can simply \"plug in\" your code into the framework, with no extra attention needed when dealing with data caching, database access, and data security verification. For more information about what a web framework for Python is, see [Web Frameworks](https://wiki.python.org/moin/WebFrameworks). \n\nThere are various types of Python web frameworks. The mainstream ones include Django, Flask, Tornado, and FastAPI.\n\n### Flask\n\n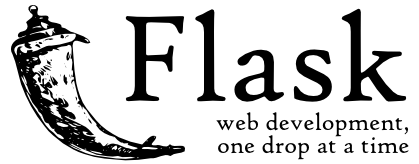\n\n[Flask](https://flask.palletsprojects.com/en/2.0.x/) is a lightweight microframework designed for Python, with a simple and easy-to-use core that allows you to develop your own web applications. In addition, the Flask core is also extensible. Therefore, Flask supports on-demand extension of different functions to meet your personalized needs during web application development. This is to say, with a library of various plug-ins in Flask, you can develop powerful websites.\n\nFlask has the following characteristics:\n\n1. Flask is a microframework that does not rely on other specific tools or components of third-party libraries to provide shared functionalities. Flask does not have a database abstraction layer, and does not require form validation. However, Flask is highly extensible and supports adding application functionality in a way similar to implementations within Flask itself. Relevant extensions include object-relational mappers, form validation, upload processing, open authentication technologies, and some common tools designed for web frameworks.\n2. Flask is a web application framework based on [WSGI](https://wsgi.readthedocs.io/) (Web Server Gateway Interface). WSGI is a simple interface connecting a web server with a web application or framework defined for the Python language.\n3. Flask includes two core function libraries, [Werkzeug](https://www.palletsprojects.com/p/werkzeug) and [Jinja2](https://www.palletsprojects.com/p/jinja). Werkzeug is a WSGI toolkit that implements request, response objects and practical functions, which allows you to build web frameworks on top of it. Jinja2 is a popular full-featured templating engine for Python. It has full support for Unicode, with an optional but widely-adopted integrated sandbox execution environment.\n\n### FastAPI\n\n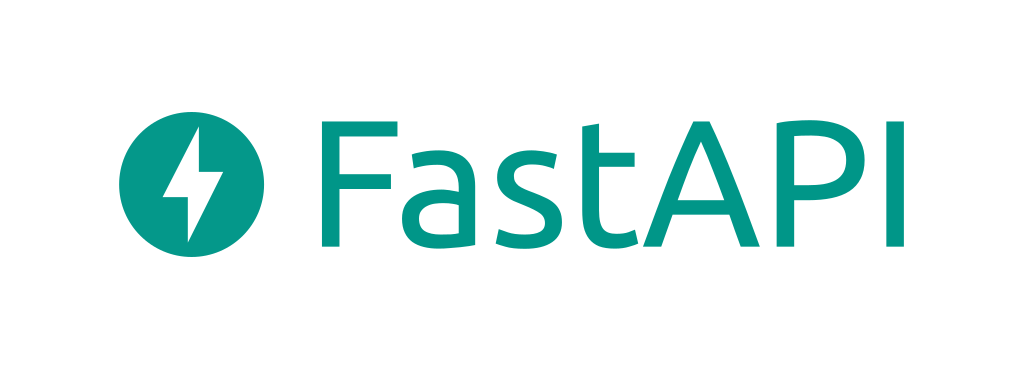\n\n[FastAPI](https://fastapi.tiangolo.com/) is a modern Python web application framework that has the same level of high performance as Go and NodeJS. The core of FastAPI is based on [Starlette](https://www.starlette.io/) and [Pydantic](https://pydantic-docs.helpmanual.io/). Starlette is a lightweight [ASGI](https://asgi.readthedocs.io/)(Asynchronous Server Gateway Interface) framework toolkit for building high-performance [Asyncio](https://docs.python.org/3/library/asyncio.html) services. Pydantic is a library that defines data validation, serialization, and documentation based on Python type hints.\n\nFastAPI has the following characteristics:\n\n1. FastAPI is a web application framework based on ASGI, an asynchronous gateway protocol interface connecting network protocol services and Python applications. FastAPI can handle a variety of common protocol types, including HTTP, HTTP2, and WebSocket.\n2. FastAPI is based on Pydantic, which provides the function of checking the interface data type. You do not need to additionally verify your interface parameter, or write extra code to verify whether the parameters are empty or whether the data type is correct. Using FastAPI can effectively avoid human errors in code and improve development efficiency.\n3. FastAPI supports document in two formats - [OpenAPI](https://swagger.io/specification/) (formerly Swagger) and [Redoc](https://www.redoc.com/). Therefore, as a user you do not need to spend extra time writing additional interface documents. The OpenAPI document provided by FastAPI is shown in the screenshot below.\n\n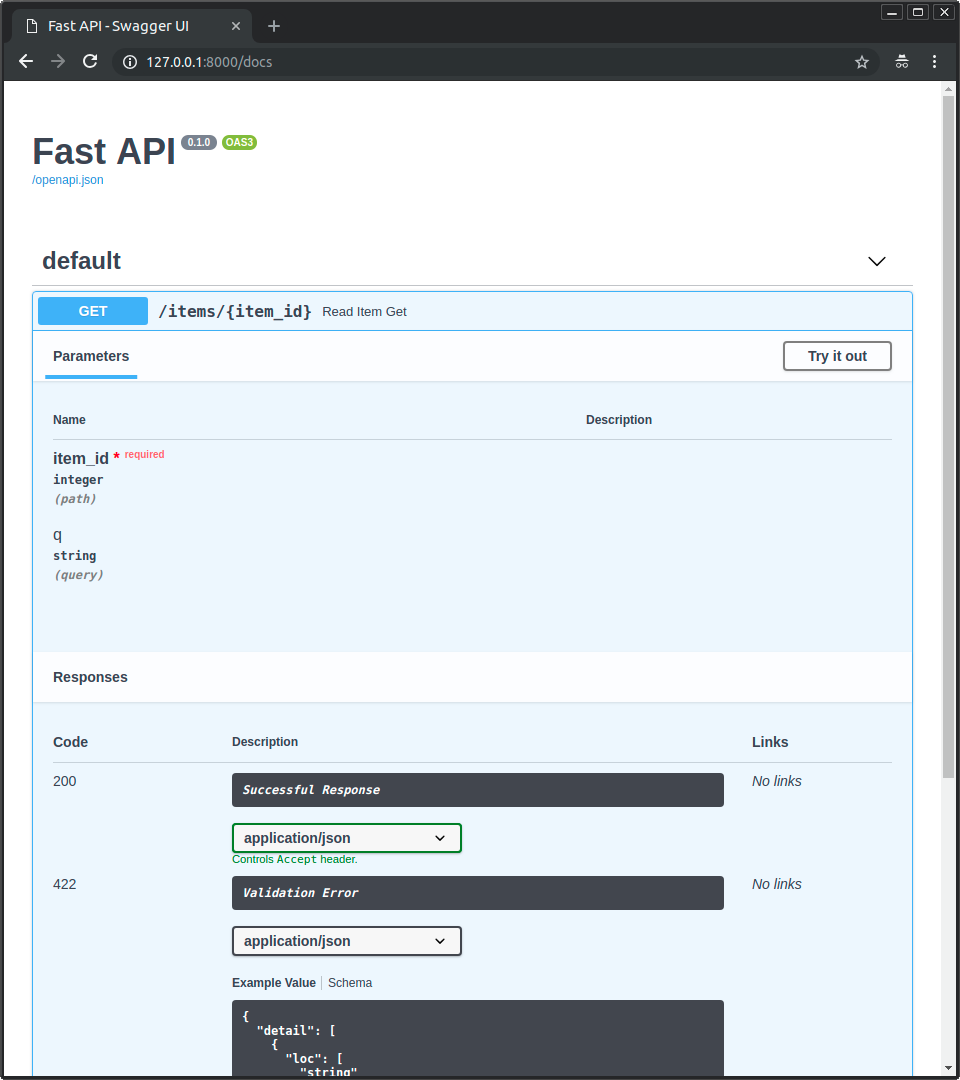\n\n### Flask Vs. FastAPI\n\nThe table below demonstrates the differences between Flask and FastAPI in several aspects.\n\n| | **FastAPI** | **Flask** |\n| -------------------------- | -------------- | ------------- |\n| **Interface gateway** | ASGI | WSGI |\n| **Asynchronous framework** | ✅ | ❌ |\n| **Performance** | Faster | Slower |\n| **Interactive doc** | OpenAPI, Redoc | None |\n| **Data verification** | ✅ | ❌ |\n| **Development costs** | Lower | Higher |\n| **Ease of use** | Lower | Higher |\n| **Flexibility** | Less flexible | More flexible |\n| **Community** | Smaller | More active |\n\n## Why FastAPI?\n\nBefore deciding which Python web application framework to choose for the projects in Milvus Bootcamp, we researched into several mainstream frameworks including Django, Flask, FastAPI, Tornado, and more. Since the projects in Milvus Bootcamp serve as references for you, our priority is to adopt an external framework of utmost lightweightness and dexterity. According to this rule, we narrowed down our choices to Flask and FastAPI.\n\nYou can see the comparison between the two web frameworks in the previous section. The following is a detailed explanation of our motivation to choose FastAPI over Flask for the projects in Milvus Bootcamp. There are several reasons:\n\n### 1. Performance\n\nMost of the projects in Milvus Bootcamp are built around reverse image search systems, QA chatbots, text search engines, which all have high demands for real-time data processing. Accordingly, we need a framework with outstanding performance, which is exactly a highlight of FastAPI. Therefore, from the perspective of system performance, we decided to choose FastAPI.\n\n### 2. Efficiency\n\nWhen using Flask, you need to write code for data type verification in each of the interfaces so that the system can determine whether the input data is empty or not. However, by supporting automatic data type verification, FastAPI helps avoid human errors in coding during system development and can greatly boost development efficiency. Bootcamp is positioned as a type of training resource. This means that the code and components we use must be intuitive and highly efficient. In this regard, we chose FastAPI to improve system efficiency and enhance user experience.\n\n### 3. Asynchronous framework\n\nFastAPI is inherently an asynchronous framework. Originally, we released four [demos](https://zilliz.com/milvus-demos?isZilliz=true), reverse image search, video analysis, QA chatbot, and molecular similarity search. In these demos, you can upload datasets and will be immediately prompted \"request received\". And when the data is uploaded to the demo system, you will receive another prompt \"data upload successful\". This is an asynchronous process which requires a framework that supports this feature. FastAPI is itself an asynchronous framework. To align all Milvus resources, we decided to adopt a single set of development tools and software for both Milvus Bootcamp and Milvus demos. As a result, we changed the framework from Flask to FastAPI.\n\n### 4. Automatic interactive documents\n\nIn a traditional way, when you finish writing the code for the server-side, you need to write an extra document to create an interface, and then use tools like [Postman](https://www.postman.com/) for API testing and debugging. So what if you only want to quickly get started with the web server-side development part of the projects in Milvus Bootcamp without writing additional code to create an interface? FastAPI is the fix. By providing an OpenAPI document, FastAPI can save you the trouble of testing or debugging APIs and collaborating with front-end teams to develop a user interface. With FastAPI, you can still quickly try the built application with an automatic but intuitive interface without extra efforts for coding.\n\n### 5. User-friendliness\n\nFastAPI is easier to use and develop, therefore enabling you to pay more attention to the specific implementation of the project itself. Without spending too much time on developing web frameworks, you can focus more on understanding the projects in Milvus Bootcamp.\n\n## Recap\n\nFlask and FlastAPI have their own pros and cons. As an emerging web application framework, FlastAPI, at its core, is built on mature toolkits and library, Starlette and Pydantic. FastAPI is an asynchronous framework with high performance. Its dexterity, extensibility, and support for automatic data type verification, together with many other powerful features, prompted us to adopt FastAPI as the framework for Milvus Bootcamp projects.\n\nPlease note that you should choose the appropriate framework according to your application scenario if you want to build a vector similarity search system in production.\n\n## About the author\n\nYunmei Li, Zilliz Data Engineer, graduated from Huazhong University of Science and Technology with a degree in computer science. Since joining Zilliz, she has been working on exploring solutions for the open source project Milvus and helping users to apply Milvus in real-world scenarios. Her main focus is on NLP and recommendation systems, and she would like to further deepen her focus in these two areas. She likes to spend time alone and read.\n\n## Looking for more resources?\n\n- Start to build AI system with Milvus and get more hands-on experience by reading our tutorials!\n - [What Is It? Who Is She? Milvus Helps Analyze Videos Intelligently](https://milvus.io/blog/2021-10-10-milvus-helps-analys-vedios.md)\n - [Combine AI Models for Image Search using ONNX and Milvus](https://milvus.io/blog/2021-09-26-onnx.md)\n - [DNA Sequence Classification based on Milvus](https://milvus.io/blog/dna-sequence-classification-based-on-milvus.md)\n - [Audio Retrieval Based on Milvus](https://milvus.io/blog/audio-retrieval-based-on-milvus.md)\n - [4 Steps to Building a Video Search System](https://milvus.io/blog/building-video-search-system-with-milvus.md)\n - [Building an Intelligent QA System with NLP and Milvus](https://milvus.io/blog/building-intelligent-chatbot-with-nlp-and-milvus.md)\n - [Accelerating New Drug Discovery](https://milvus.io/blog/molecular-structure-similarity-with-milvus.md)\n\n- Engage with our open-source community:\n - Find or contribute to Milvus on [GitHub](https://bit.ly/307b7jC).\n - Interact with the community via [Forum](https://bit.ly/3qiyTEk).\n - Connect with us on [Twitter](https://bit.ly/3ob7kd8).\n","title":"Why to Choose FastAPI over Flask?","metaData":{}},{"id":"2021-11-26-accelerating-candidate-generation-in-recommender-systems-using-milvus-paired-with-paddlepaddle.md","author":"Yunmei","desc":"the minimal workflow of a recommender system","canonicalUrl":"https://zilliz.com/blog/accelerating-candidate-generation-in-recommender-systems-using-milvus-paired-with-paddlepaddle","date":"2021-11-26T00:00:00.000Z","cover":"https://assets.zilliz.com/Candidate_generation_9baf7beb86.png","tags":["Scenarios"],"href":"/blog/2021-11-26-accelerating-candidate-generation-in-recommender-systems-using-milvus-paired-with-paddlepaddle.md","content":"\nIf you have experience developing a recommender system, you are likely to have fallen victim to at least one of the following: \n\n- The system is extremely slow when returning results due to the tremendous amount of datasets.\n- Newly inserted data cannot be processed in real time for search or query.\n- Deployment of the recommender system is daunting. \n\n\nThis article aims to address the issues mentioned above and provide some insights for you by introducing a product recommender system project that uses Milvus, an open-source vector database, paired with PaddlePaddle, a deep learning platform. \n\n\nThis article sets out to briefly describe the minimal workflow of a recommender system. Then it moves on to introduce the main components and the implementation details of this project.\n\n\n## The basic workflow of a recommender system\n\nBefore going deep into the project itself, let's first take a look at the basic workflow of a recommender system. A recommender system can return personalized results according to unique user interest and needs. To make such personalized recommendations, the system goes through two stages, candidate generation and ranking. \n\n\n\n\nThe first stage is candidate generation, which returns the most relevant or similar data, such as a product or a video that matches the user profile. During candidate generation, the system compares the user trait with the data stored in its database, and retrieves those similar ones. Then during ranking, the system scores and reorders the retrieved data. Finally, those results on the top of the list are shown to users. \n\n\n\nIn our case of a product recommender system, it first compares the user profile with the characteristics of the products in inventory to filter out a list of products catering to the user's needs. Then the system scores the products based on their similarity to user profile, ranks them, and finally returns the top 10 products to the user.\n\n\n\n\n\n## System architecture\n\nThe product recommender system in this project uses three components: MIND, PaddleRec, and Milvus.\n\n\n\n### MIND\n\n[MIND](https://arxiv.org/pdf/1904.08030), short for \"Multi-Interest Network with Dynamic Routing for Recommendation at Tmall\", is an algorithm developed by Alibaba Group. Before MIND was proposed, most of the prevalent AI models for recommendation used a single vector to represent a user's varied interests. However, a single vector is far from sufficient to represent the exact interests of a user. Therefore, MIND algorithm was proposed to turn a user's multiple interests into several vectors.\n\n\n\nSpecifically, MIND adopts a [multi-interest network](https://arxiv.org/pdf/2005.09347) with dynamic routing for processing multiple interests of one user during the candidate generation stage. The multi-interest network is a layer of multi-interest extractor built on capsule routing mechanism. It can be used to combine a user's past behaviors with his or her multiple interests, to provide an accurate user profile.\n\n\n\nThe following diagram illustrates the network structure of MIND. \n\n\n\n\n\n\nTo represent the trait of users, MIND takes user behaviors and user interests as inputs, and then feeds them into the embedding layer to generate user vectors, including user interest vectors and user behavior vectors. Then user behavior vectors are fed into the multi-interest extractor layer to generate users interest capsules. After concatenating the user interest capsules with user behavior embeddings and using several ReLU layers to transform them, MIND outputs several user representation vectors. This project has defined that MIND will ultimately output four user representation vectors.\n\n\n\nOn the other hand, product traits go through the embedding layer and are converted into sparse item vectors. Then each item vector goes through a pooling layer to become a dense vector.\n\n\n\nWhen all data are converted into vectors, an extra label-aware attention layer is introduced to guide the training process.\n\n\n\n### PaddleRec\n\n[PaddleRec](https://github.com/PaddlePaddle/PaddleRec/blob/release/2.2.0/README_EN.md) is a large-scale search model library for recommendation. It is part of the Baidu [PaddlePaddle](https://github.com/PaddlePaddle/Paddle) ecosystem. PaddleRec aims to provide developers with an integrated solution to build a recommendation system in an easy and rapid way.\n\n\n\n\nAs mentioned in the opening paragraph, engineers developing recommender systems often have to face the challenges of poor usability and complicated deployment of the system. However, PaddleRec can help developers in the following aspects:\n\n- Ease of use: PaddleRec is an open-source library that encapsulates various popular models in the industry, including models for candidate generation, ranking, reranking, multitasking, and more. With PaddleRec, you can instantly test the effectiveness of the model and improve its efficiency through iteration. PaddleRec offers you an easy way to train models for distributed systems with excellent performance. It is optimized for large-scale data processing of sparse vectors. You can easily scale PaddleRec horizontally and accelerate its computing speed. Therefore, you can quickly build training environments on Kubernetes using PaddleRec. \n\n- Support for deployment: PaddleRec provides online deployment solutions for its models. The models are immediately ready for use after training, featuring flexibility and high availability.\n\n\n\n### Milvus\n\n[Milvus](https://milvus.io/docs/v2.0.x/overview.md) is a vector database featuring a cloud-native architecture. It is open sourced on [GitHub](https://github.com/milvus-io) and can be used to store, index, and manage massive embedding vectors generated by deep neural networks and other machine learning (ML) models. Milvus encapsulates several first-class approximate nearest neighbor (ANN) search libraries including Faiss, NMSLIB, and Annoy. You can also scale out Milvus according to your need. The Milvus service is highly available and supports unified batch and stream processing. Milvus is committed to simplifying the process of managing unstructured data and providing a consistent user experience in different deployment environments. It has the following features:\n\n- High performance when conducting vector search on massive datasets.\n\n- A developer-first community that offers multi-language support and toolchain.\n\n- Cloud scalability and high reliability even in the event of a disruption.\n\n- Hybrid search achieved by pairing scalar filtering with vector similarity search.\n\n\n\nMilvus is used for vector similarity search and vector management in this project because it can solve the problem of frequent data updates while maintaining system stability.\n\n\n\n## System implementation\n\nTo build the product recommender system in this project, you need to go through the following steps:\n\n1. Data processing\n2. Model training\n3. Model testing\n4. Generating product item candidates\n 1. Data storage: item vectors are obtained through the trained model and are stored in Milvus.\n 2. Data search: four user vectors generated by MIND are fed into Milvus for vector similarity search.\n 3. Data ranking: each of the four vectors has its own `top_k` similar item vectors, and four sets of `top_k` vectors are ranked to return a final list of `top_k` most similar vectors.\n\n\n\nThe source code of this project is hosted on the [Baidu AI Studio](https://aistudio.baidu.com/aistudio/projectdetail/2250360?contributionType=1\u0026shared=1) platform. The following section is a detailed explanation of the source code for this project. \n\n### Step 1. Data processing\n\nThe original dataset comes from the Amazon book dataset provided by [ComiRec](https://github.com/THUDM/ComiRec). However, this project uses the data that is downloaded from and processed by PaddleRec. Refer to the [AmazonBook dataset](https://github.com/PaddlePaddle/PaddleRec/tree/release/2.1.0/datasets/AmazonBook) in the PaddleRec project for more information.\n\nThe dataset for training is expected to appear in the following format, with each column representing:\n\n- `Uid`: User ID.\n- `item_id`: ID of the product item that has been clicked by the user.\n- `Time`: The timestamp or order of click.\n\n\n\nThe dataset for testing is expected to appear in the following format, with each column representing:\n\n- `Uid`: User ID.\n\n- `hist_item`: ID of the product item in historical user click behavior. When there are multiple `hist_item`, they are sorted according to the timestamp.\n\n- `eval_item`: The actual sequence in which the user clicks the products.\n\n\n\n\n### Step 2. Model training\n\nModel training uses the processed data in the previous step and adopts the candidate generation model, MIND, built on PaddleRec.\n\n#### 1. **Model** **input**\n\nIn `dygraph_model.py`, run the following code to process the data and turn them into model input. This process sorts the items clicked by the same user in the original data according to the timestamp, and combines them to form a sequence. Then, randomly select an `item``_``id` from the sequence as the `target_item`, and extract the 10 items before `target_item` as the `hist_item` for model input. If the sequence is not long enough, it can be set as 0. `seq_len` should be the actual length of the `hist_item` sequence.\n\n```Python\ndef create_feeds_train(self, batch_data):\n hist_item = paddle.to_tensor(batch_data[0], dtype=\"int64\")\n target_item = paddle.to_tensor(batch_data[1], dtype=\"int64\")\n seq_len = paddle.to_tensor(batch_data[2], dtype=\"int64\")\n return [hist_item, target_item, seq_len]\n```\n\nRefer to the script `/home/aistudio/recommend/model/mind/mind_reader.py` for the code of reading the original dataset. \n\n#### 2. **Model networking**\n\nThe following code is an extract of `net.py`. `class Mind_Capsual_Layer` defines the multi-interest extractor layer built on the interest capsule routing mechanism. The function `label_aware_attention()` implements the label-aware attention technique in the MIND algorithm. The `forward()` function in the `class MindLayer` models the user characteristics and generates corresponding weight vectors.\n\n```Python\nclass Mind_Capsual_Layer(nn.Layer):\n def __init__(self):\n super(Mind_Capsual_Layer, self).__init__()\n self.iters = iters\n self.input_units = input_units\n self.output_units = output_units\n self.maxlen = maxlen\n self.init_std = init_std\n self.k_max = k_max\n self.batch_size = batch_size\n # B2I routing\n self.routing_logits = self.create_parameter(\n shape=[1, self.k_max, self.maxlen],\n attr=paddle.ParamAttr(\n name=\"routing_logits\", trainable=False),\n default_initializer=nn.initializer.Normal(\n mean=0.0, std=self.init_std))\n # bilinear mapping\n self.bilinear_mapping_matrix = self.create_parameter(\n shape=[self.input_units, self.output_units],\n attr=paddle.ParamAttr(\n name=\"bilinear_mapping_matrix\", trainable=True),\n default_initializer=nn.initializer.Normal(\n mean=0.0, std=self.init_std))\n \nclass MindLayer(nn.Layer):\n\n def label_aware_attention(self, keys, query):\n weight = paddle.sum(keys * query, axis=-1, keepdim=True)\n weight = paddle.pow(weight, self.pow_p) # [x,k_max,1]\n weight = F.softmax(weight, axis=1)\n output = paddle.sum(keys * weight, axis=1)\n return output, weight\n\n def forward(self, hist_item, seqlen, labels=None):\n hit_item_emb = self.item_emb(hist_item) # [B, seqlen, embed_dim]\n user_cap, cap_weights, cap_mask = self.capsual_layer(hit_item_emb, seqlen)\n if not self.training:\n return user_cap, cap_weights\n target_emb = self.item_emb(labels)\n user_emb, W = self.label_aware_attention(user_cap, target_emb)\n\n return self.sampled_softmax(\n user_emb, labels, self.item_emb.weight,\n self.embedding_bias), W, user_cap, cap_weights, cap_mask\n```\n\nRefer to the script `/home/aistudio/recommend/model/mind/net.py` for the specific network structure of MIND. \n\n#### 3. **Model optimization**\n\nThis project uses [Adam algorithm](https://arxiv.org/pdf/1412.6980) as the model optimizer. \n\n```Python\ndef create_optimizer(self, dy_model, config):\n lr = config.get(\"hyper_parameters.optimizer.learning_rate\", 0.001)\n optimizer = paddle.optimizer.Adam(\n learning_rate=lr, parameters=dy_model.parameters())\n return optimizer\n```\n\nIn addition, PaddleRec writes hyperparameters in `config.yaml`, so you just need to modify this file to see a clear comparison between the effectiveness of the two models to improve model efficiency. When training the model, the poor model effect can result from model underfitting or overfitting. You can therefore improve it by modifying the number of rounds of training. In this project, you only need to change the parameter epochs in `config.yaml` to find the perfect number of rounds of training. In addition, you can also change the model optimizer, `optimizer.class`,or `learning_rate` for debugging. The following shows part of the parameters in `config.yaml`.\n\n```YAML\nrunner:\n use_gpu: True\n use_auc: False\n train_batch_size: 128\n epochs: 20\n print_interval: 10\n model_save_path: \"output_model_mind\"\n\n# hyper parameters of user-defined network\nhyper_parameters:\n # optimizer config\n optimizer:\n class: Adam\n learning_rate: 0.005\n```\n\nRefer to the script `/home/aistudio/recommend/model/mind/dygraph_model.py` for detailed implementation. \n\n#### 4. **Model training**\n\nRun the following command to start model training.\n\n```Bash\npython -u trainer.py -m mind/config.yaml\n```\n\nRefer to `/home/aistudio/recommend/model/trainer.py` for the model training project. \n\n### Step 3. Model testing\n\nThis step uses test dataset to verify the performance, such as the recall rate of the trained model.\n\nDuring model testing, all item vectors are loaded from the model, and then imported into Milvus, the open-source vector database. Read the test dataset through the script `/home/aistudio/recommend/model/mind/mind_infer_reader.py`. Load the model in the previous step, and feed the test dataset into the model to obtain four interest vectors of the user. Search for the most similar 50 item vectors to the four interest vectors in Milvus. You can recommend the returned results to users.\n\nRun the following command to test the model.\n\n```Bash\npython -u infer.py -m mind/config.yaml -top_n 50\n```\n\nDuring model testing, the system provides several indicators for evaluating model effectiveness, such as Recall@50, NDCG@50, and HitRate@50. This article only introduces modifying one parameter. However, in your own application scenario, you need to train more epochs for better model effect. You can also improve model effectiveness by using different optimizers, setting different learning rates, and increasing the number of rounds of testing. It is recommended that you save several models with different effects, and then choose the one with the best performance and best fits your application. \n\n### Step 4. Generating product item candidates\n\nTo build the product candidate generation service, this project uses the trained model in the previous steps, paired with Milvus. During candidate generation, FASTAPI is used to provide the interface. When the service starts, you can directly run commands in the terminal via `curl`.\n\nRun the following command to generate preliminary candidates.\n\n```Bash\nuvicorn main:app\n```\n\nThe service provides four types of interfaces:\n\n- **Insert** : Run the following command to read the item vectors from your model and insert them into a collection in Milvus. \n\n```Nginx\ncurl -X 'POST' \\\n 'http://127.0.0.1:8000/rec/insert_data' \\\n -H 'accept: application/json' \\\n -d ''\n```\n\n- **Generate preliminary candidates**: Input the sequence in which products are clicked by the user, and find out the next product that the user may click. You can also generate product item candidates in batches for several users at one go. `hist_item` in the following command is a two-dimensional vector, and each row represents a sequence of the products that the user has clicked in the past. You can define the length of the sequence. The returned results are also sets of two-dimensional vectors, each row representing the returned `item id`s for users.\n\n```Ada\ncurl -X 'POST' \\\n 'http://127.0.0.1:8000/rec/recall' \\\n -H 'accept: application/json' \\\n -H 'Content-Type: application/json' \\\n -d '{\n \"top_k\": 50,\n \"hist_item\": [[43,23,65,675,3456,8654,123454,54367,234561],[675,3456,8654,123454,76543,1234,9769,5670,65443,123098,34219,234098]]\n}'\n```\n\n- **Query the total number of** **product items**: Run the following command to return the total number of item vectors stored in the Milvus database.\n\n```Nginx\ncurl -X 'POST' \\\n 'http://127.0.0.1:8000/rec/count' \\\n -H 'accept: application/json' \\\n -d ''\n```\n\n- **Delete**: Run the following command to delete all data stored in the Milvus database .\n\n```Nginx\ncurl -X 'POST' \\\n 'http://127.0.0.1:8000/qa/drop' \\\n -H 'accept: application/json' \\\n -d ''\n```\n\nIf you run the candidate generation service on your local server, you can also access the above interfaces at `127.0.0.1:8000/docs`. You can play around by clicking on the four interfaces and entering the value for the parameters. Then click \"Try it out\" to get the recommendation result. \n\n\n\n\n\n\n## Recap\n\nThis article mainly focuses on the first stage of candidate generation in building a recommender system. It also provides a solution to accelerating this process by combining Milvus with the MIND algorithm and PaddleRec and therefore has addressed the issue proposed in the opening paragraph. \n\nWhat if the system is extremely slow when returning results due to the tremendous amount of datasets? Milvus, the open-source vector database, is designed for blazing-fast similarity search on dense vector datasets containing millions, billions, or even trillions of vectors.\n\nWhat if newly inserted data cannot be processed in real time for search or query? You can use Milvus as it supports unified batch and stream processing and enables you to search and query newly inserted data in real time. Also, the MIND model is capable of converting new user behavior in real-time and inserting the user vectors into Milvus instantaneously. \n\nWhat if the complicated deployment is too intimidating? PaddleRec, a powerful library that belongs to the PaddlePaddle ecosystem, can provide you with an integrated solution to deploy your recommendation system or other applications in an easy and rapid way.\n\n\n## About the author\n\nYunmei Li, Zilliz Data Engineer, graduated from Huazhong University of Science and Technology with a degree in computer science. Since joining Zilliz, she has been working on exploring solutions for the open source project Milvus and helping users to apply Milvus in real-world scenarios. Her main focus is on NLP and recommendation systems, and she would like to further deepen her focus in these two areas. She likes to spend time alone and read.\n\n\n## Looking for more resources?\n\n- More user cases of building a recommender system:\n - [Building a Personalized Product Recommender System with Vipshop with Milvus](https://milvus.io/blog/building-a-personalized-product-recommender-system-with-vipshop-and-milvus.md)\n - [Building a Wardrobe and Outfit Planning App with Milvus](https://milvus.io/blog/building-a-wardrobe-and-outfit-planning-app-with-milvus.md)\n - [Building an Intelligent News Recommendation System Inside Sohu News App](https://milvus.io/blog/building-an-intelligent-news-recommendation-system-inside-sohu-news-app.md)\n - [Item-based Collaborative Filtering for Music Recommender System](https://milvus.io/blog/music-recommender-system-item-based-collaborative-filtering-milvus.md)\n - [Making with Milvus: AI-Powered News Recommendation Inside Xiaomi's Mobile Browser](https://milvus.io/blog/Making-with-Milvus-AI-Powered-News-Recommendation-Inside-Xiaomi-Mobile-Browser.md)\n- More Milvus projects in collaboration with other communities:\n - [Combine AI Models for Image Search Using ONNX and Milvus](https://milvus.io/blog/2021-09-26-onnx.md)\n - [Building a Graph-based recommendation system with Milvus, PinSage, DGL, and Movielens Datasets](https://milvus.io/blog/graph-based-recommendation-system-with-milvus.md)\n - [Building a Milvus Cluster Based on JuiceFS](https://milvus.io/blog/building-a-milvus-cluster-based-on-juicefs.md)\n- Engage with our open-source community:\n - Find or contribute to Milvus on [GitHub](https://bit.ly/307b7jC)\n - Interact with the community via [Forum](https://bit.ly/3qiyTEk)\n - Connect with us on [Twitter](https://bit.ly/3ob7kd8)\n","title":"Accelerating Candidate Generation in Recommender Systems Using Milvus paired with PaddlePaddle","metaData":{}},{"id":"2021-11-19-run-milvus-2.0-on-windows.md","author":"JiBin","desc":"This tutorial introduces how to run Milvus 2.0 on Windows.","date":"2021-11-19T00:00:00.000Z","cover":"https://assets.zilliz.com/Run_Milvus_2_0_4b49f077d9.png","tags":["Engineering"],"href":"/blog/2021-11-19-run-milvus-2.0-on-windows.md","content":"\nThis tutorial introduces how to run Milvus 2.0 on Windows. If you want to get started with Milvus 2.0 using Windows, or simply have to run Milvus on Windows due to environment limits, this tutorial can be a good reference. This article is originally written by [@matrixji](https://github.com/matrixji).\n\nThere are two ways to run Milvus 2.0 on Windows:\n\n- [Compile from source code](#Compile-from-source-code)\n- [Install from the binary package](#Install-from-the-binary-package)\n\n## Compile from source code\n\n### Prerequisites\n\nYou need to install MinGW64/MSYS toolchain before compiling Milvus 2.0 on Windows. See [MSYS2](https://www.msys2.org/) for the installation guide. The compilation in this tutorial is completed in the shell of MSYS2 MinGW 64-bit. You can run `$ pacman -Su` to update the toolchain.\n\nWe also recommend that you run `$ pacman -S git` to install git.\n\n### Step 1. Clone code\n\nRun the following command to clone the code from the **windows-dev** branch in the **matrixji/milvus** repository. Currently, all commits can be traced in **windows-dev**, which rebases commits from the upstream main branch [**milvus-io/milvus**](https://github.com/milvus-io/milvus) on a weekly basis.\n\n```python\n$ git clone git@github.com:matrixji/milvus.git -b windows-dev\n```\n\n### Step 2. Compile Milvus\n\n#### 1. Install dependencies and toolchain\n\nRun the following command to install the required dependencies and toolchain. After the installation, you need to restart the MinGW/MYSY shell to ensure the configurations of some tools are properly applied.\n\n```python\n$ cd milvus\n$ scripts/install_deps_msys.sh\n```\n\n#### 2. Compile Milvus\n\nRun the following command to compile and package Milvus.\n\n```python\n$ make\n$ sh scripts/package_windows.sh\n```\n\nIf successful, you will find a zip file in the subdirectory of `windows_package`. The zip file contains all the files for running `milvus.exe`, including `minio.exe` and `etcd.exe`.\n\n\u003cdiv class=\"alert note\"\u003e\nMilvus 2.0 is developed in Go. You might need to configure \u003ca href='https://goproxy.cn/'\u003eGoproxy\u003c/a\u003e to install third-party modules.\n\u003c/div\u003e\n\n## Install from the binary package\n\nYou can also download the binary package that has already been compiled from [Releases - matrixji/milvus](https://github.com/matrixji/milvus/releases). Each release has **windows-test-** as the prefix, for instance, **windows-test-v8**. Find the release you want and download the corresponding zip package.\n\n### Step 1. Start Milvus\n\n#### 1. Unzip the package\n\nUnzip the package, and you will find a `milvus` directory, which contains all the files required.\n\n#### 2. Start a MinIO service\n\nDouble-click the `run_minio.bat` file to start a MinIO service with default configurations. Data will be stored in the subdirectory `s3data`.\n\n#### 3. Start an etcd service\n\nDouble-click the `run_etcd.bat` file to start an etcd service with default configurations.\n\n#### 4. Start Milvus service\n\nDouble-click the `run_milvus.bat` file to start the Milvus service.\n\n### Step 2. Run `hello_milvus.py`\n\nAfter starting the Milvus service, you can test by running `hello_milvus.py`. See [Hello Milvus](https://milvus.io/docs/v2.0.x/example_code.md) for more information.\n\n\n## Engage with our open-source community:\n- Find or contribute to Milvus on [GitHub](https://bit.ly/3khejQB).\n- Interact with the community via [Forum](https://bit.ly/307HVsY).\n- Connect with us on [Twitter](https://bit.ly/3wn5aek).\n","title":"Run Milvus 2.0 on Windows","metaData":{}},{"id":"2021-11-10-milvus-hacktoberfest-2021.md","author":"Zilliz","desc":"Thank you to everyone who participated in Milvus Hacktoberfest 2021!","date":"2021-11-10T00:00:00.000Z","cover":"https://assets.zilliz.com/It_s_a_wrap_9c0b9f0b38.png","tags":["Events"],"href":"/blog/2021-11-10-milvus-hacktoberfest-2021.md","content":"\n# It's a Wrap - Milvus Hacktoberfest 2021\n\n\n\nHacktoberfest has come to an end but there is no end to contributing to open-source projects!\n\n\nThroughout October, we had a total of **44 pull requests** (PRs) across our repos from **36 contributors** (excluding our core team). Though it is the first year for the Milvus community to join Hacktoberfest, the number of participants we saw was beyond our expectation, an indication of the growing awareness of open-source spirit.\n\nWe hope everyone who participated in this event gained some hands-on experience or knowledge of open source, community, and helpful technical skills in the process.️️️\n\nIn this post, we’d like to invite you to celebrate our achievements together and how to continue to contribute to Milvus after Hacktoberfest.\n\n## **📣 Shout out to our contributors:**\n\nDuring Hacktoberfest this year, the Milvus project repositories saw **44 pull requests merged**! This is a huge accomplishment on all sides. Good job, everyone! 🎉\n\n[parthiv11](https://github.com/parthiv11), [joremysh](https://github.com/joremysh), [noviicee](https://github.com/noviicee), [Biki-das](https://github.com/Biki-das), [Nadyamilona](https://github.com/Nadyamilona), [ashish4arora](https://github.com/ashish4arora), [Dhruvacube](https://github.com/Dhruvacube), [iamartyaa](https://github.com/iamartyaa), [RafaelDSS](https://github.com/RafaelDSS), [kartikcho](https://github.com/kartikcho), [GuyKh](https://github.com/GuyKh), [Deep1Shikha](https://github.com/Deep1Shikha), [shreemaan-abhishek](https://github.com/shreemaan-abhishek), [daniel-shuy](https://github.com/daniel-shuy), [Hard-Coder05](https://github.com/Hard-Coder05), [sapora1](https://github.com/sapora1), [Rutam21](https://github.com/Rutam21), [idivyanshbansal](https://github.com/idivyanshbansal), [Mihir501](https://github.com/Mihir501), [YushChaudhary](https://github.com/Ayushchaudhary-Github), [sreyan-ghosh](https://github.com/sreyan-ghosh), [chiaramistro](https://github.com/chiaramistro), [appledora](https://github.com/appledora), [luisAzcuaga](https://github.com/luisAzcuaga), [matteomessmer](https://github.com/matteomessmer), [Nadyamilona](https://github.com/Nadyamilona), [Tititesouris](https://github.com/Tititesouris), [amusfq](https://github.com/amusfq), [matrixji](https://github.com/matrixji) \u0026 [generalZman](https://github.com/zamanmub)\n\n\n\n\n\n\n\n### Here are some extraordinary Milvus Hacktoberfest 2021 contributions:\n\n\n\n**⚙️ New features**\n\n[Compile \u0026 Run Milvus across multiple platforms](https://github.com/milvus-io/milvus/issues/7706) by [matrixji](https://github.com/matrixji) \n\n**(🏆 Top Contributor 🏆 )**\n\n\n\n**📝 Documentation**\n\n- [Translate Hello Milvus (example_code.md) to any language](https://github.com/milvus-io/bootcamp/issues/720) by [chiaramistro](https://github.com/chiaramistro), [appledora](https://github.com/appledora), [luisAzcuaga](https://github.com/luisAzcuaga), [matteomessmer](https://github.com/matteomessmer), [Nadyamilona](https://github.com/Nadyamilona), [Tititesouris](https://github.com/Tititesouris) \u0026 [amusfq](https://github.com/amusfq)\n- [Add NodeJS example to example_code.md](https://github.com/milvus-io/bootcamp/issues/720) by [GuyKh](https://github.com/GuyKh) \n- [translated upgrade.md](https://github.com/milvus-io/milvus-docs/pull/921/files) \u0026 [add and translate parameters in User Guide to CN](https://github.com/milvus-io/milvus-docs/pull/892) by [joremysh](https://github.com/joremysh)\n- [Translate tutorials/dna_sequence_classification.md into CN](https://github.com/milvus-io/milvus-docs/pull/753) \u0026 [Translate reference/schema/collection_schema.md into CN](https://github.com/milvus-io/milvus-docs/pull/752) by [daniel-shuy](https://github.com/daniel-shuy)\n\n\n\n**🚀 Bootcamp**\n\n- [Enhancement Jupyter Notebook tutorial for image hash search ](https://github.com/milvus-io/bootcamp/pull/858)by [generalZman](https://github.com/zamanmub)\n- [fix the TOPK bug of reverse_image_search](https://github.com/milvus-io/bootcamp/pull/792) by [RafaelDSS](https://github.com/RafaelDSS)\n\n\n\n**🐍 PyMilvus**\n\n- [Update GitHub issue forms ](https://github.com/milvus-io/pymilvus/issues/741)(multiple repos) by [Hard-Coder05](https://github.com/Hard-Coder05) \n\n\n\n### Share your feedback with us! \n\n\n\nYou are more than welcome to share your experience of Milvus Hacktoberfest 2021 with us! Whether it's a blog post, a tweet (@milvusio) or just a post in our [forum](https://discuss.milvus.io/c/hacktoberfest/9), anything will be greatly appreciated!\n\n\n\n## What's Next?\n\n\n\n### **👩💻** **Code \u0026 Documentation**\n\nIf you have limited knowledge of Milvus, you can get familiar with how the community works first by contributing to [pymilvus](https://github.com/milvus-io/pymilvus) or [docs](https://github.com/milvus-io/milvus-docs) repos. You can also look for tags such as **#goodfirstissue** or **#helpwanted**. \n\n\n\n\n\nIf you have any questions about contributing, you can always ask the community under the Forum's **Contributor** category: https://discuss.milvus.io/t/things-you-need-to-know-before-you-get-started/64. \n\n\n\n### 🏆 Be a Milvus Advocate\n\n\n\n\n\nShare your experience and things you've learned with the community;propose ideas on how we can improve; answer questions and help out others on Milvus Forum, etc. There are many ways for you to participate in the community, we've listed a few examples below but we welcome any forms of contribution: \n\n- **Demos and solutions:** Show Milvus users how to leverage the platform in specific scenarios (e.g. music recommendation system). Examples are available in the [Milvus Bootcamp](https://github.com/milvus-io/bootcamp).\n\n- **Blog articles, user stories, or whitepapers:** Write high-quality content that clearly and accurately explains technical details about Milvus.\n\n- **Tech talks/live broadcasts:** Give talks or host live broadcasts that help raise awareness around Milvus.\n\n- **Others:** Any content that plays a positive role in the development of Milvus and its open-source community will be considered eligible.\n\n\n\nFor more details, please read: https://milvus.io/community/milvus_advocate.md\n\n\n\nLastly, thank you again for joining this year's Hacktoberfest with us and letting us be your mentors and students. Thank you [Digital Ocean](https://hacktoberfest.digitalocean.com/) for hosting yet another year of this amazing event. Until next time!\n\n\n\nHappy Coding!\n\nMilvus Community Team\n","title":"It's a Wrap! Milvus Hacktoberfest 2021","metaData":{}},{"id":"2021-11-07-how-to-modify-milvus-advanced-configurations.md","author":"Zilliz","desc":"How to modify the configuration of Milvus deployed on Kubernetes","date":"2021-11-08T00:00:00.000Z","cover":"https://assets.zilliz.com/modify_4d93b9da3a.png","tags":["Engineering"],"href":"/blog/2021-11-07-how-to-modify-milvus-advanced-configurations.md","content":"\n*Yufen Zong, a Zilliz Test Development Engineer, graduated from Huazhong University of Science and Technology with a master's degree in computer technology. She is currently engaged in the quality assurance of Milvus vector database, including but not limited to interface integration testing, SDK testing, Benchmark testing, etc. Yufen is an enthusiastic problem-shooter in the test and development of Milvus, and a huge fan of chaos engineering theory and fault drill practice.*\n\n## Background\n\n\nWhile using Milvus vector database, you will need to modify the default configuration to satisfy the requirements of different scenarios. Previously, a Milvus user shared on [How to Modify the Configuration of Milvus Deployed Using Docker Compose](2021-10-22-apply-configuration-changes-on-milvus-2.md). And in this article, I would like to share with you on how to modify the configuration of Milvus deployed on Kubernetes.\n\n## Modify configuration of Milvus on Kubernetes\n\nYou may choose different modification plans according to the configuration parameters you wish to modify. All Milvus configuration files are stored under **milvus/configs**. While installing Milvus on Kubernetes, a Milvus Helm Chart repository will be added locally. By running `helm show values milvus/milvus`, you can check the parameters that can be modified directly with Chart. For the modifiable parameters with Chart, you can pass the parameter using `--values` or `--set`. For more information, see [Milvus Helm Chart](https://artifacthub.io/packages/helm/milvus/milvus) and [Helm](https://helm.sh/docs/).\n\nIf the parameters you expect to modify are not on the list, you can follow the instruction below.\n\nIn the following steps, the parameter `rootcoord.dmlChannelNum` in **/milvus/configs/advanced/root_coord.yaml** is to be modified for demonstration purposes. Configuration file management of Milvus on Kubernetes is implemented through ConfigMap resource object. To change the parameter, you should first update the ConfigMap object of corresponding Chart release, and then modify the deployment resource files of corresponding pods. \n\nBeware that this method only applies to parameter modification on deployed Milvus application. To modify the parameters in **/milvus/configs/advanced/\\*.yaml** before deployment, you will need to re-develop the Milvus Helm Chart.\n\n### Modify ConfigMap YAML\n\nAs shown below, your Milvus release running on Kubernetes corresponds to a ConfigMap object with the same name of the release. The `data` section of the ConfigMap object only includes configurations in **milvus.yaml**. To change the `rootcoord.dmlChannelNum` in **root_coord.yaml**, you must add the parameters in **root_coord.yaml** to the `data` section in the ConfigMap YAML and change the specific parameter. \n\n```\nkind: ConfigMap\napiVersion: v1\nmetadata:\n name: milvus-chaos\n ...\ndata:\n milvus.yaml: \u003e\n ......\n root_coord.yaml: |\n rootcoord:\n dmlChannelNum: 128\n maxPartitionNum: 4096\n minSegmentSizeToEnableIndex: 1024\n timeout: 3600 # time out, 5 seconds\n timeTickInterval: 200 # ms\n```\n\n### Modify Deployment YAML\n\nThe data stored in a ConfigMap can be referenced in a volume of type configMap and then consumed by containerized applications running in a pod. To direct the pods to the new configuration files, you must modify the pod templates that need to load the configurations in **root_coord.yaml**. Specifically, you need to add a mount declaration under the `spec.template.spec.containers.volumeMounts` section in deployment YAML.\n\nTaking the deployment YAML of rootcoord pod as an example, a `configMap` type volume named **milvus-config** is specified in `.spec.volumes` section. And, in `spec.template.spec.containers.volumeMounts` section, the volume is declared to mount **milvus.yaml** of your Milvus release on **/milvus/configs/milvus.yaml**. Similarly, you only need to add a mount declaration specifically for rootcoord container to mount the **root_coord.yaml** on **/milvus/configs/advanced/root_coord.yaml**, and thus the container can access the new configuration file.\n\n```yaml\nspec:\n replicas: 1\n selector:\n ......\n template:\n metadata:\n ...\n spec:\n volumes:\n - name: milvus-config\n configMap:\n name: milvus-chaos\n defaultMode: 420\n containers:\n - name: rootcoord\n image: 'milvusdb/milvus-dev:master-20210906-86afde4'\n args:\n ...\n ports:\n ...\n resources: {}\n volumeMounts:\n - name: milvus-config\n readOnly: true\n mountPath: /milvus/configs/milvus.yaml\n subPath: milvus.yaml\n - name: milvus-config\n readOnly: true\n mountPath: /milvus/configs/advanced/`root_coord.yaml\n subPath: root_coord.yaml\n terminationMessagePath: /dev/termination-log\n terminationMessagePolicy: File\n imagePullPolicy: IfNotPresent\n restartPolicy: Always\n terminationGracePeriodSeconds: 30\n dnsPolicy: ClusterFirst\n securityContext: {}\n schedulerName: default-scheduler\n```\n\n### Verify the result\n\nThe kubelet checks whether the mounted ConfigMap is fresh on every periodic sync. When the ConfigMap consumed in the volume is updated, projected keys are automatically updated as well. When the new pod is running again, you can verify if the modification is successful in the pod. Commands to check the parameter `rootcoord.dmlChannelNum` are shared below.\n\n```bash\n$ kctl exec -ti milvus-chaos-rootcoord-6f56794f5b-xp2zs -- sh\n# cd configs/advanced\n# pwd\n/milvus/configs/advanced\n# ls\nchannel.yaml common.yaml data_coord.yaml data_node.yaml etcd.yaml proxy.yaml query_node.yaml root_coord.yaml\n# cat root_coord.yaml\nrootcoord:\n dmlChannelNum: 128\n maxPartitionNum: 4096\n minSegmentSizeToEnableIndex: 1024\n timeout: 3600 # time out, 5 seconds\n timeTickInterval: 200 # ms\n# exit\n```\n\n\n\nAbove is the method to modify the advanced configurations in Milvus deployed on Kubernetes. Future release of Milvus will integrate all configurations in one file, and will support updating configuration via helm chart. But before that, I hope this article can help you as a temporary solution.\n\n\n\n## Engage with our open-source community:\n\n- Find or contribute to Milvus on [GitHub](https://bit.ly/307b7jC).\n\n- Interact with the community via [Forum](https://bit.ly/3qiyTEk).\n\n- Connect with us on [Twitter](https://bit.ly/3ob7kd8).\n\n \n","title":"How to Modify Milvus Advanced Configurations","metaData":{}},{"id":"2021-11-08-frustrated-with-new-data-our-vector-database-can-help.md","author":"Zilliz","desc":"Design and Practice of AI-oriented General-purpose Vector Database Systems","date":"2021-11-08T00:00:00.000Z","cover":"https://assets.zilliz.com/Frustrated_with_new_data_5051d3ad15.png","tags":["Engineering"],"href":"/blog/2021-11-08-frustrated-with-new-data-our-vector-database-can-help.md","content":"\n# Frustrated with New Data? Our Vector Database can Help\n\nIn the era of Big Data, what database technologies and applications will come into the limelight? What will be the next game-changer?\n\nWith unstructured data representing roughly 80-90% of all stored data; what are we supposed to do with these growing data lakes? One might think of using traditional analyitical methods, but these fail to pull out useful information, if any info at all. To answer this question, the \"Three Musketeers\" of Zilliz's Research and Developement team, Dr. Rentong Guo, Mr. Xiaofan Luan, and Dr. Xiaomeng Yi, have co-authored an article to discuss the design and challenges faced when building a general-purpose vector database system.\n\nThis article has been included in Programmer, a journal produced by CSDN, the biggest software developer community in China. This issue of Programmer also includes articles by Jeffrey Ullman, recipient of the 2020 Turing Award, Yann LeCun, recipient of the 2018 Turing Award, Mark Porter, CTO of MongoDB, Zhenkun Yang, founder of OceanBase, Dongxu Huang, founder of PingCAP, etc.\n\nBelow we share the full-length article with you: \n\n# Design and Practice of AI-oriented General-purpose Vector Database Systems\n\n## Introduction\n\nModern-day data applications can easily deal with structured data, which accounts for roughly 20% of today's data. In its toolbox are systems like relational databases, NoSQL databases, etc; in contrast, unstructured data, which accounts for roughly 80% of all data, does not have any reliable systems in place. To solve this problem, this article will discuss the pain points that traditional data analytics has with unstructured data and further discuss the architecture and challenges that we faced building up our own general- purpose vector database system.\n\n## Data Revolution in the AI era\n\nWith the rapid development of 5G and IoT technologies, industries are seeking to multiply their channels of data collection and further project the real world into the digital space. Although it has brought on some tremendous challenges, it has also brought with it tremendous benefits to the growing industry. One of these tough challenges is how to gain deeper insights into this new incoming data. \n\nAccording to IDC statistics, more than 40,000 exabytes of new data was generated worldwide in 2020 alone. Of the total, only 20% is structured data - data that is highly ordered and easy to organize and analyze via numerical calculations and relational algebra. In contrast, unstructured data (taking up the remaining 80%) is extremely rich in data type variations, making it difficult to uncover the deep semantics through traditional data analytic methods.\n\nFortunately, we are experiencing a concurrent, rapid evolution in unstructured data and AI, with AI allowing us to better understand the data through various types of neural networks, as shown in Figure 1.\n\n\n\n\n\n\n\nEmbedding technology has quickly gained popularity after the debut of Word2vec, with the idea of \"embed everything\" reaching all sectors of machine learning. This leads to the emergence of two major data layers: the raw data layer and the vector data layer. The raw data layer is comprised of unstructured data and certain types of structured data; the vector layer is the collection of easily analyzable embeddings that originates from the raw layer passing through machine learning models. \n\nWhen compared with raw data, vectorized data features the following advantages:\n\n- Embedding vectors are an abstract type of data, meaning we can build a unified algebra system dedicated to reducing the complexity of unstructured data.\n- Embedding vectors are expressed through dense floating-point vectors, allowing applications to take advantage of SIMD. With SIMD being supported by GPUs and nearly all modern CPUs, computations across vectors can achieve high performance at a relatively low cost.\n- Vector data encoded via machine learning models takes up less storage space than the original unstructured data, allowing for higher throughput.\n- Arithmetic can also be performed across embedding vectors. Figure 2 shows an example of cross-modal semantic approximate matching - the pictures shown in the figure are the result of matching words embeddings with image embeddings. \n\n\n\n\n\n\n\nAs shown in Figure 3, combining image and word semantics can be done with simple vector addition and subtraction across their corresponding embeddings.\n\n\n\n\nApart from the above features, these operators support more complicated query statements in practical scenarios. Content recommendation is a well-known example. Generally, the system embeds both the content and the users' viewing preferences. Next, the system matches the embedded user's preferences with the most similar embedded content via semantic similarity analysis, resulting in new content that is similar to users' preferences. This vector data layer isn't just limited to recommender systems, use cases include e-commerce, malware analysis, data analysis, biometric verification, chemical formula analysis, finance, insurance, etc.\n\n## Unstructured data requires a complete basic software stack\n\nSystem software sits at the foundation of all data-oriented applications, but the data system software built up over the past several decades, e.g. databases, data analysis engines, etc., are meant to deal with structured data. Modern data applications rely almost exclusively on unstructured data and do not benefit from traditional database management systems. \n\nTo tackle this issue, we have developed and open-sourced an AI-oriented general-purpose vector database system named *Milvus* (Reference No. 1~2). When compared with traditional database systems, Milvus works on a different layer of data. Traditional databases, such as relational databases, KV databases, text databases, images/video databases, etc... work on the raw data layer, while Milvus works on the vector data layer. \n\nIn the following chapters, we will discuss the novel features, architectural design, and technical challenges we faced when building Milvus.\n\n## Major attributes of vector database\n\nVector databases store, retrieve, analyze vectors, and, just as with any other database, also provide a standard interface for CRUD operations. In addition to these \"standard\" features, the attributes listed below are also important qualities for a vector database:\n\n- **Support for high-efficiency vector operators**\n\nSupport for vector operators in an analysis engine focuses on two levels. First, the vector database should support different types of operators, for example, semantic similarity matching and semantic arithmetic mentioned above. In addition to this, it should support a variety of similarity metrics for the underlying similarity calculations. Such similarity is usually quantified as spatial distance between vectors, with common metrics being Euclidean distance, cosine distance, and inner product distance.\n\n- **Support for vector indexing**\n\nCompared to B-tree or LSM-tree based indexes in traditional databases, high-dimensional vector indexes usually consume much more computing resources. We recommend using clustering and graph index algorithms, and giving priority to matrix and vector operations, hence taking full advantage of the hardware vector calculation acceleration abilities previously mentioned. \n\n- **Consistent user experience across different deployment environments**\n\nVector databases are usually dveloped and deployed in different environments. At the preliminary stage, data scientists and algorithm engineers work mostly on their laptops and workstations, as they pay more attention to verification efficiency and iteration speed. When verification is completed, they may deploy the full-size database on a private cluster or the cloud. Therefore, a qualified vector database system should deliver consistent performance and user experience across different deployment environments.\n\n- **Support for hybrid search**\n\nNew applications are emerging as vector databases become ubiquitous. Among all these demands, the most frequently mentioned is hybrid search on vectors and other types of data. A few examples of this is approximate nearest neighbor search (ANNS) after scalar filtering, multi-channel recall from full-text search and vector search, and hybrid search of spatio-temporal data and vector data. Such challenges demand elastic scalability and query optimization to effectively fuse vector search engines with KV, text, and other search engines. \n\n- **Cloud-native architecture**\n\nThe volume of vector data mushrooms with the exponential growth of data collection. Trillion-scale, high-dimensional vector data corresponds to thousands of TB of storage, which is far beyond the limit of a single node. As a result, horizontal extendability is a key ability for a vector database, and should satisfy the users' demands for elasticity and deployment agility. Furthermore, it should also lower the system operation and maintenance complexity while improving observability with the assistance of cloud infrastructure. Some of these needs come in the form of multi-tenant isolation, data snapshot and backup, data encryption, and data visualization, which are common in traditional databases.\n\n## Vector database system architecture\n\nMilvus 2.0 follows the design principles of \"log as data\", \"unified batch and stream processing\", \"stateless\", and \"micro-services\". Figure 4 depicts the overall architecture of Milvus 2.0.\n\n\n\n\n\n**Log as data**: Milvus 2.0 does not maintain any physical tables. Instead, it ensures data reliability via log persistence and log snapshots. The log broker (the system's backbone) stores logs and decouples components and services through the log publication-subscription (pub-sub) mechanism. As shown in Figure 5, the log broker is comprised of \"log sequence\" and \"log subscriber\". The log sequence records all operations that change the state of a collection (equivalent to a table in a relational database ); log subscriber subscribes to the log sequence to update its local data and provide services in the form of read-only copies. The pub-sub mechanism also makes room for system extendability in terms of change data capture (CDC) and globally-distributed deployment.\n\n\n\n\n**Unified batch and stream processing**: Log streaming allows Milvus to update data in real time, thereby ensuring real-time deliverability. Furthermore, by transforming data batches into log snapshots and building index on snapshots, Milvus is able to achieve higher query efficiency. During a query, Milvus merges the query results from both incremental data and historical data to guarantee the integrality of the data returned. Such design better balances real-time performance and efficiency, easing the maintenance burden of both online and offline systems compared to that of the traditional Lambda architecture.\n\n**Stateless**: Cloud infrastructure and open-source storage components free Milvus from persisting data within its own components. Milvus 2.0 persists data with three types of storage: metadata storage, log storage, and object storage. Metadata storage not only stores the metadata, but also handles services discovery and node management. Log storage executes incremental data persistence and data publication-subscription. Object storage stores log snapshots, indexes, and some intermediate calculation results.\n\n**Microservices**: Milvus follows the principles of data plane and control plane disaggregation, read/write separation, and online/offline task separation. It is compromised of four layers of service: the access layer, coordinator layer, worker layer, and storage layer. These layers are mutually independent when it comes to scaling and disaster recovery. As the front-facing layer and user endpoint, the access layer handles client connections, validates client requests, and combines query results. As the system's \"brain\", the coordinator layer takes on the tasks of cluster topology management, load balancing, data declaration, and data management. The worker layer contains the \"limbs\" of the system, executing data updates, queries, and index building operations. Finally, the storage layer is in charge of data persistence and replication. Overall, this microservice-based design ensures a controllable system complexity, with each component responsible to its own corresponding function. Milvus clarifies the service boundaries through well-defined interfaces, and decouples the services based on finer granularity, which further optimizes the elastic scalability and resource distribution.\n\n## Technical challenges faced by vector databases\n\nEarly research on vector databases was mainly concentrated on the design of high-efficiency index structures and query methods - this resulted in a variety of vector search algorithm libraries (Reference No. 3~5). Over the past few years, an increasing number of academic and engineering teams have taken a fresh look at vector search issues from system design perspective, and proposed some systematic solutions. Summarizing existing studies and user demand, we categorize the main technical challenges for vector databases as follows:\n\n- **Optimization of cost-to-performance ratio relative to load**\n\nCompared to that of traditional data types, analysis of vector data takes much more storage and computing resources because of its high dimensionality. Moreover, users have shown diverse preferences for load characteristics and cost-performance optimization on vector search solutions. For instance, users, who work with extremely large datasets (tens or hundreds of billions of vectors), would prefer solutions with lower data storage costs and variance in search latency, while others may demand higher search performance and a non-varying average latency. To satisfy such diverse preferences, the core index component of the vector database must be able to support index structures and search algorithms with different types of storage and computing hardware.\n\nFor example, storing vector data and the corresponding index data in cheaper storage mediums (such as NVM and SSD) should be taken into consideration when lowering storage costs. However, most existing vector search algorithms work on data read directly from memory. To avoid performance loss brought by the usage of disk drives, the vector database should be able to exploit the locality of data access combined with search algorithms in addition to being able to adjust to storage solutions for vector data and index structure (Reference No. 6~8). For the sake of performance improvements, contemporary research has been focused on hardware acceleration technologies involving GPU, NPU, FPGA, etc. (Reference No. 9). However, acceleration-specific hardware and chips vary in architecture design, and the problem of most efficient execution across different hardware accelerators is not yet solved.\n\n- **Automated system configuration and tuning**\n\nMost existing studies on vector search algorithms seek a flexible balance between storage costs, computational performance, and search accuracy. Generally, both algorithm parameters and data features influence the actual performance of an algorithm. As user demands differ in costs and performance, selecting a vector query method that suits their needs and data features poses a significant challenge.\n\nNevertheless, manual methods of analyzing the effects of data distribution on search algorithms aren't effective due to the high dimensionality of the vector data. To address this issue, academia and industry are seeking algorithm recommendation solutions based on machine learning (Reference No. 10). \n\nThe design of an ML-powered intelligent vector search algorithm is also a research hotspot. Generally speaking, existing vector search algorithms are developed universally for vector data with various dimensionality and distribution patterns. As a result, they do not support specific index structures according to the data features, and thus have little space for optimization. Future studies should also explore effective machine learning technologies that can tailor index structures for different data features (Reference No. 11-12). \n\n- **Support for advanced query semantics**\n\nModern applications often rely on more advanced queries across vectors - traditional nearest neighbour search semantics are no longer applicable to vector data search. Moreover, demand for combined search across multiple vector databases or on vector and non-vector data is emerging (Reference No. 13). \n\nSpecifically, variations in distance metrics for vector similarity grow fast. Traditional similarity scores, such as Euclidean distance, inner product distance, and cosine distance cannot satisfy all application demands. With the popularization of artificial intelligence technology, many industries are developing their own field-specific vector similarity metrics, such as Tanimoto distance, Mahalanobis distance, Superstructure, and Substructure. Integrating these evaluation metrics into existing search algorithms and designing novel algorithms utilizing said metrics are both challenging research problems.\n\nAs the complexity of user services increase, applications will need to search across both vector data and non-vector data. For example, a content recommender analyzes users' preferences, social relations, and matches them with current hot topics to pull proper content to users. Such searches normally involve queries on multiple data types or across multiple data processing systems. To support such hybrid searches efficiently and flexibly is another system design challenge.\n\n## Authors\n\nDr. Rentong Guo (Ph.D. of Computer Software and Theory, Huazhong University of Science and Technology), partner and R\u0026D Director of Zilliz. He is a member of China Computer Federation Technical Committee on Distributed Computing and Processing (CCF TCDCP). His research focuses on database, distributed system, caching system, and heterogeneous computing. His research works have been published on several top-tier conferences and journals, including Usenix ATC, ICS, DATE, TPDS. As the architect of Milvus, Dr. Guo is seeking solutions to develop highly scalable and cost-efficient AI-based data analytic systems.\n\nXiaofan Luan, partner and Engineering Director of Zilliz, and Technical Advisory Committee member of LF AI \u0026 Data Foundation. He worked successively in the Oracle US headquarters and Hedvig, a software defined storage startup. He joined Alibaba Cloud Database team and was in charge of the development of NoSQL database HBase and Lindorm. Luan obtained his master's degree in Electronic Computer Engineering from Cornell University.\n\nDr. Xiaomeng Yi (Ph.D. of Computer Architecture, Huazhong University of Science and Technology), Senior Researcher and Research team leader of Zilliz. His research concentrates on high-dimension data management, large-scale information retrieval, and resource allocation in distributed systems. Dr. Yi's research works have been published on leading journals and international conferences including IEEE Network Magazine, IEEE/ACM TON, ACM SIGMOD, IEEE ICDCS, and ACM TOMPECS.\n\nFilip Haltmayer, a Zilliz Data Engineer, graduated from University of California, Santa Cruz with a BS in Computer Science. After joining Zilliz, Filip spends most of his time working on cloud deployments, client interactions, techincal talks, and AI application development.\n\n## References\n\n1. Milvus Project: https://github.com/milvus-io/milvus\n2. Milvus: A Purpose-Built Vector Data Management System, SIGMOD'21\n3. Faiss Project: https://github.com/facebookresearch/faiss\n4. Annoy Project: https://github.com/spotify/annoy\n5. SPTAG Project: https://github.com/microsoft/SPTAG\n6. GRIP: Multi-Store Capacity-Optimized High-Performance Nearest Neighbor Search for Vector Search Engine, CIKM'19\n7. DiskANN: Fast Accurate Billion-point Nearest Neighbor Search on a Single Node, NIPS'19\n8. HM-ANN: Efficient Billion-Point Nearest Neighbor Search on Heterogeneous Memory, NIPS'20\n9. SONG: Approximate Nearest Neighbor Search on GPU, ICDE'20\n10. A demonstration of the ottertune automatic database management system tuning service, VLDB'18\n11. The Case for Learned Index Structures, SIGMOD'18\n12. Improving Approximate Nearest Neighbor Search through Learned Adaptive Early Termination, SIGMOD'20\n13. AnalyticDB-V: A Hybrid Analytical Engine Towards Query Fusion for Structured and Unstructured Data, VLDB'20\n\n\n\n## Engage with our open-source community:\n- Find or contribute to Milvus on [GitHub](https://bit.ly/3khejQB).\n- Interact with the community via [Forum](https://bit.ly/307HVsY).\n- Connect with us on [Twitter](https://bit.ly/3wn5aek).\n\n \n","title":"Frustrated with New Data? Our Vector Database can Help","metaData":{}},{"id":"2021-10-22-apply-configuration-changes-on-milvus-2.md","author":"Jingjing","desc":"Learn how apply configuration changes on Milvus 2.0","date":"2021-10-22T00:00:00.000Z","cover":"https://assets.zilliz.com/Modify_configurations_f9162c5670.png","tags":["Engineering"],"href":"/blog/2021-10-22-apply-configuration-changes-on-milvus-2.md","content":"\n# Technical Sharing: Apply Configuration Changes on Milvus 2.0 using Docker Compose\n\n\n*Jingjing Jia, Zilliz Data Engineer, graduated from Xi’an Jiaotong University with a degree in Computer Science. After joining Zilliz, she mainly works on data pre-processing, AI model deployment, Milvus related technology research, and helping community users to implement application scenarios. SHe is very patient, likes to communicate with community partners, and enjoys listening to music and watching anime.*\n\n\nAs a frequent user of Milvus, I was very excited about the newly released Milvus 2.0 RC. According to the introduction on the official website, Milvus 2.0 seems to outmatch its predecessors by a large margin. I was so eager to try it out myself.\n\n\n\nAnd I did. However, when I truly got my hands on Milvus 2.0, I realized that I wasn't able to modify the configuration file in Milvus 2.0 as easily as I did with Milvus 1.1.1. I couldn't change the configuration file inside the docker container of Milvus 2.0 started with Docker Compose, and even force change wouldn't take effect. Later, I learned that Milvus 2.0 RC was unable to detect changes to the configuration file after installation. And future stable release will fix this issue.\n\n\n\nHaving tried different approaches, I've found a reliable way to apply changes to configuration files for Milvus 2.0 standalone \u0026 cluster, and here is how.\n\n\n\nNote that all changes to configuration must be made before restarting Milvus using Docker Compose.\n\n## Modify configuration file in Milvus standalone\n\nFirst, you will need to [download](https://github.com/milvus-io/milvus/blob/master/configs/milvus.yaml) a copy of **milvus.yaml** file to your local device.\n\n\n\nThen you can change the configurations in the file. For instance, you can change the log format as `.json`. \n\n\n\n\n\nOnce **milvus.yaml** file is modified, you will also need to [download](https://github.com/milvus-io/milvus/blob/master/deployments/docker/standalone/docker-compose.yml) and modify in **docker-compose.yaml** file for standalone by mapping the local path to milvus.yaml onto the corresponding docker container path to configuration file `/milvus/configs/milvus.yaml` under the `volumes` section.\n\n\n\nLastly, start Milvus standalone using `docker-compose up -d` and check if the modifications are successful. For instance, run `docker logs` to check the log format.\n\n\n\n\n\n## Modify configuration file in Milvus cluster\n\nFirst, [download](https://github.com/milvus-io/milvus/blob/master/configs/milvus.yaml) and modify the **milvus.yaml** file to suit your needs.\n\n\n\n\nThen you will need to [download](https://github.com/milvus-io/milvus/blob/master/deployments/docker/cluster/docker-compose.yml) and modify the cluster **docker-compose.yml** file by mapping the local path to **milvus.yaml** onto the corresponding path to configuration files in all components, i.e. root coord, data coord, data node, query coord, query node, index coord, index node, and proxy.\n\n\n\n\n\n\n\n\nFinally, you can start Milvus cluster using `docker-compose up -d` and check if the modifications are successful.\n\n## Change log file path in configuration file\n\nFirst, [download](https://github.com/milvus-io/milvus/blob/master/configs/milvus.yaml) the **milvus.yaml** file, and change the `rootPath` section as the directory where you expect to store the log files in Docker container. \n\n\n\n\n\n\n\nAfter that, download the corresponding **docker-compose.yml** file for Milvus [standalone](https://github.com/milvus-io/milvus/blob/master/deployments/docker/standalone/docker-compose.yml) or [cluster](https://github.com/milvus-io/milvus/blob/master/deployments/docker/cluster/docker-compose.yml). \n\nFor standalone, you need to map the local path to **milvus.yaml** onto the corresponding docker container path to configuration file `/milvus/configs/milvus.yaml`, and map the local log file directory onto the Docker container directory you created previously. \n\nFor cluster, you will need to map both paths in every component.\n\n\n\nLastly, start Milvus standalone or cluster using `docker-compose up -d` and check the log files to see if the modification is successful.\n","title":"Technical Sharing:Apply Configuration Changes on Milvus 2.0 using Docker Compose","metaData":{}},{"id":"2021-10-10-milvus-helps-analyze-videos.md","author":"Shiyu Chen","desc":"Learn how Milvus powers the AI analysis of video contents.","canonicalUrl":"https://zilliz.com/blog/milvus-helps-analyze-videos-intelligently","date":"2021-10-11T00:00:00.000Z","cover":"https://assets.zilliz.com/Who_is_it_e9d4510ace.png","tags":["Scenarios"],"href":"/blog/2021-10-10-milvus-helps-analyze-videos.md","content":"\n# Building a Video Analysis System with Milvus Vector Database\n\n*Shiyu Chen, a data engineer at Zilliz, graduated from Xidian University with a degree in Computer Science. Since joining Zilliz, she has been exploring solutions for Milvus in various fields, such as audio and video analysis, molecule formula retrieval, etc., which has greatly enriched the application scenarios of the community. She is currently exploring more interesting solutions. In her spare time, she loves sports and reading.*\n\nWhen I was watching *Free Guy* last weekend, I felt that I'd seen the actor who plays Buddy, the security guard, somewhere before, yet couldn't recall any of his works. My head was stuffed with \"who's this guy?\" I was sure about having seen that face and was trying so hard to remember his name. A similar case is that once I saw the leading actor in a video having a drink I used to like a lot, but I ended up failing to recall the brand name.\n\nThe answer was on the tip of my tongue, but my brain felt completely stuck.\n\nThe tip of the tongue (TOT) phenomenon drives me crazy when watching movies. If only there was a reverse image search engine for videos that enables me to find videos and analyze video content. Before, I built a [reverse image search engine using Milvus](https://github.com/milvus-io/bootcamp/tree/master/solutions/reverse_image_search/quick_deploy). Considering that video content analysis somehow resembles image analysis, I decided to build a video content analysis engine based on Milvus.\n\n## Object detection\n\n### Overview\n\nBefore being analyzed, objects in a video should be detected first. Detecting objects in a video effectively and accurately is the main challenge of the task. It is also an important task for applications such as autopilot, wearable devices, and IoT.\n\nDeveloped from traditional image processing algorithms to deep neural networks (DNN), today's mainstream models for object detection include R-CNN, FRCNN, SSD, and YOLO. The Milvus-based deep learning video analysis system introduced in this topic can detect objects intelligently and quickly.\n\n### Implementation\n\nTo detect and recognize objects in a video, the system should first extract frames from a video and detect objects in the frame images using object detection, secondly, extract feature vectors from detected objects, and lastly, analyze the object based on the feature vectors.\n\n- Frame extraction\n\nVideo analysis is converted to image analysis using frame extraction. Currently, frame extraction technology is very mature. Programs such as FFmpeg and OpenCV support extracting frames at specified intervals. This article introduces how to extract frames from a video every second using OpenCV.\n\n- Object detection\n\nObject detection is about finding objects in extracted frames and extracting screenshots of the objects according to their positions. As shown in the following figures, a bike, a dog, and a car were detected. This topic introduces how to detect objects using YOLOv3, which is commonly used for object detection.\n\n\n- Feature extraction\n\nFeature extraction refers to converting unstructured data, which is difficult for machines to recognize, to feature vectors. For example, images can be converted to multi-dimensional feature vectors using deep learning models. Currently, the most popular image recognition AI models include VGG, GNN, and ResNet. This topic introduces how to extract features from detected objects using ResNet-50.\n\n- Vector analysis\n\nExtracted feature vectors are compared with library vectors, and the corresponding information to the most similar vectors is returned. For large-scale feature vector datasets, calculation is a huge challenge. This topic introduces how to analyze feature vectors using Milvus.\n\n## Key technologies\n\n### OpenCV \n\nOpen Source Computer Vision Library (OpenCV) is a cross-platform computer vision library, which provides many universal algorithms for image processing and computer vision. OpenCV is commonly used in the computer vision field. \n\nThe following example shows how to capture video frames at specified intervals and save them as images using OpenCV with Python.\n\n```python\nimport cv2 \ncap = cv2.VideoCapture(file_path) \nframerate = cap.get(cv2.CAP_PROP_FPS) \nallframes = int(cv2.VideoCapture.get(cap, int(cv2.CAP_PROP_FRAME_COUNT))) \nsuccess, image = cap.read() \ncv2.imwrite(file_name, image)\n```\n\n### YOLOv3\n\nYou Only Look Once, Version 3 (YOLOv3 [5]) is a one-stage object detection algorithm proposed in recent years. Compared to the traditional object detection algorithms with the same accuracy, YOLOv3 is twice as fast. YOLOv3 mentioned in this topic is the enhanced version from PaddlePaddle [6]. It uses multiple optimization methods with a higher inference speed.\n\n### ResNet-50\n\nResNet [7] is the winner of ILSVRC 2015 in image classification because of its simplicity and practicality. As the basis of many image analysis methods, ResNet proves to be a popular model specialized in image detection, segmentation, and recognition.\n\n### Milvus \n\n[Milvus](https://milvus.io/) is a cloud-native, open-source vector database built to manage embedding vectors generated by machine learning models and neural networks. It is widely used in scenarios such as computer vision, natural language processing, computational chemistry, personalized recommender systems, and more.\n\nThe following procedures describe how Milvus works.\n\n1. Unstructured data is converted to feature vectors by using deep learning models and is imported to Milvus.\n2. Milvus stores and indexes the feature vectors.\n3. Milvus returns the most similar vectors to the vector queried by users.\n\n\n## Deployment\n\nNow you have some understanding of Milvus-based video analysis systems. The system mainly consists of two parts, as shown in the following figure.\n\n- The red arrows indicate the data import process. Use ResNet-50 to extract feature vectors from the image dataset and import the feature vectors to Milvus.\n\n- The black arrows indicate the video analysis process. First, extract frames from a video and save the frames as images. Second, detect and extract objects in the images using YOLOv3. Then, use ResNet-50 to extract feature vectors from the images. Lastly, Milvus searches and returns the information of the objects with the corresponding feature vectors.\n\nFor more information, see [Milvus Bootcamp: Video Object Detection System](https://github.com/milvus-io/bootcamp/tree/master/solutions/video_similarity_search/object_detection). \n\n**Data import**\n\nThe data import process is simple. Convert the data into 2,048-dimensional vectors and import the vectors into Milvus. \n\n```python\nvector = image_encoder.execute(filename)\nentities = [vector]\ncollection.insert(data=entities)\n```\n\n**Video analysis**\n\nAs introduced above, the video analysis process includes capturing video frames, detecting objects in each frame, extracting vectors from the objects, calculating vector similarity with Euclidean distance (L2) metrics, and searching for results using Milvus.\n\n```python\nimages = extract_frame(filename, 1, prefix) \ndetector = Detector() \nrun(detector, DATA_PATH) \nvectors = get_object_vector(image_encoder, DATA_PATH)\nsearch_params = {\"metric_type\": \"L2\", \"params\": {\"nprobe\": 10}}\nresults = collection.search(vectors, param=search_params, limit=10)\n```\n\n## Conclusion\n\nCurrently, more than 80% of the data is unstructured. With the rapid development of AI, an increasing number of deep learning models have been developed for analyzing unstructured data. Technologies such as object detection and image processing have achieved great breakthroughs in both academia and industry. Empowered by these technologies, more and more AI platforms have fulfilled practical requirements.\n\nThe video analysis system discussed in this topic is built with Milvus, which can quickly analyze video content.\n\nAs an open-source vector database, Milvus supports feature vectors extracted using various deep learning models. Integrated with libraries such as Faiss, NMSLIB, and Annoy, Milvus provides a set of intuitive APIs, supporting switching index types according to scenarios. Additionally, Milvus supports scalar filtering, which increases recall rate and search flexibility. Milvus has been applied to many fields such as image processing, computer vision, natural language processing, speech recognition, recommender system, and new drug discovery.\n\n## References\n\n[1] A. D. Bagdanov, L. Ballan, M. Bertini, A. Del Bimbo. “Trademark matching and retrieval in sports video databases.” Proceedings of the international workshop on Workshop on multimedia information retrieval, ACM, 2007. https://www.researchgate.net/publication/210113141_Trademark_matching_and_retrieval_in_sports_video_databases \n\n[2] J. Kleban, X. Xie, W.-Y. Ma. “Spatial pyramid mining for logo detection in natural scenes.” IEEE International Conference, 2008. https://ieeexplore.ieee.org/document/4607625 \n\n[3] R. Boia, C. Florea, L. Florea, R. Dogaru. “Logo localization and recognition in natural images using homographic class graphs.” Machine Vision and Applications 27 (2), 2016. https://link.springer.com/article/10.1007/s00138-015-0741-7 \n\n[4] R. Boia, C. Florea, L. Florea. “Elliptical asift agglomeration in class prototype for logo detection.” BMVC, 2015. http://citeseerx.ist.psu.edu/viewdoc/download;jsessionid=5C87F52DE38AB0C90F8340DFEBB841F7?doi=10.1.1.707.9371\u0026rep=rep1\u0026type=pdf \n\n[5] https://arxiv.org/abs/1804.02767 \n\n[6] https://paddlepaddle.org.cn/modelbasedetail/yolov3 \n\n[7] https://arxiv.org/abs/1512.03385 \n","title":"Building a Video Analysis System with Milvus Vector Database","metaData":{}},{"id":"2021-09-28-hactoberfeat-warmup.md","author":"Zilliz","desc":"Everyone is welcome!","date":"2021-09-28T00:00:00.000Z","cover":"https://assets.zilliz.com/Hacktoberfest_89c929cace.png","tags":["Events"],"href":"/blog/2021-09-28-hactoberfeat-warmup.md","content":"\n# Milvus To Participate In Hacktoberfest 2021\n\n\n\n**Milvus, the vector database empowering AI applications, announced today its participation in the 8th annual Hacktoberfest event sponsored by DigitalOcean!**\n\nIn 2014, DigitalOcean initiated [Hacktoberfest](https://hacktoberfest.digitalocean.com/) with the idea of celebrating open source software projects. Through the years, the event has steadily grown from tens to thousands of participants. This, years the month-long event looks to grow to over 200,000 active participants! \n\nStarting October 1st, everyone from seasoned developers to students, code newbies and tech writers to UX designers, can contribute to open source communities and develop their skills, with the perks of winning limited edition swag and more.\n\n## Everyone is welcome\n\nThere are a lot of ways to contribute to the Milvus project - you don't have to be a code superstar to contribute! In fact, you don't even need an extensive background or long list of skills in order to help out. Are you a great writer or editor, awesome! Do you like to create graphics or illustrations - perfect! Here are a few ways you can make a contribution during the event, along with a list of GitHub \"issues\" for new contributors to tackle!\n\nCheck out the details [here](https://discuss.milvus.io/t/join-hacktoberfest-2021-with-us/72#how-to-participate-1).\n\n## Contribute, get noticed \u0026 earn swag\n\n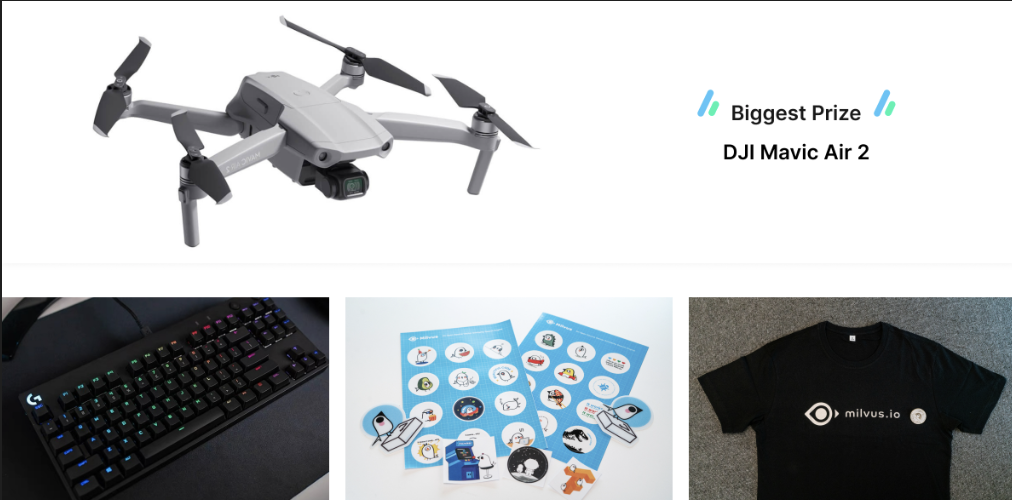\n\nCertainly contributing to open source, or the concept of open source, is in esence an embodiment of altruism, but some cool swag doesn't hurt. We have up for grabs exclusive stickers, t-shirts, keyboards and a drone too! Check out the details on our [forum page](https://discuss.milvus.io/t/join-hacktoberfest-2021-with-us/72#prizes-8).\n\nDigitalOcean also provides swag for participants, check out the rules from the official Hacktoberfest website.\n\n## What's next\n\n* We recently hosted a virtual Q\u0026A call about Hacktoberfest. You can view the recording [here](https://www.youtube.com/watch?v=cHjSTEHoiF8).\n\n* To participate in the event, we recommend starting on our milvus.io Hacktoberfest page and checking out the [\"Quick Start\" Guide]( https://hacktoberfest.com/)\n* Check out our [GitHub repo]( https://github.com/milvus-io) (and give us a star)!\n* View our list of curated [\"issues\"](https://github.com/milvus-io/milvus/issues?q=is%3Aopen+is%3Aissue+label%3AHacktoberfest) for participants that can help you become a rockstar!\n\n## Helpful links\n\n* [**Milvus x Hactoberfest event page**](https://hacktoberfest.com/)\n\n* [**Milvus Discussion Forum**](https://discuss.milvus.io/c/hacktoberfest/9) - Join our Milvus forum to stay up-to-date on Milvus releases, interact with other users, and ask questions during the event.\n\n* **Follow us on [Twitter](https://twitter.com/milvusio)** and if your contribution is highlighted during the event you could earn special swag!\n","title":"Milvus To Participate In Hacktoberfest 2021","metaData":{}},{"id":"2021-09-26-onnx.md","desc":"how to use multiple models for image search based on ONNX and Milvus","canonicalUrl":"https://zilliz.com/blog/combine-ai-models-for-image-search-using-onnx-and-milvus","date":"2021-09-26T00:00:00.000Z","cover":"https://assets.zilliz.com/medium_1_cfb009269a.png","tags":["Engineering"],"href":"/blog/2021-09-26-onnx.md","content":"\n# Combine AI Models for Image Search using ONNX and Milvus\n\nOpen Neural Network Exchange (ONNX) is an open format built to represent machine learning models. Since it was open-sourced in 2017, ONNX has developed into a standard for AI, providing building blocks for machine learning and deep learning models. ONNX defines a common file format to enable AI developers to use models with various frameworks, tools, runtimes, and compilers, and helps increase the speed of innovation in the artificial intelligence community.\n\nMilvus is an open-source vector database that is highly flexible, reliable and blazing fast. It supports adding, deleting, updating and near real-time search of vector. Milvus has a comprehensive set of intuitive APIs, and support for multiple widely adopted index libraries (e.g. Faiss, NMSLIB, and Annoy), simplifying the index selection for a given scenario. Milvus is simple to use, and it has been used in hundreds of organizations and institutions worldwide, including image, audio and video search, recommendation, chatbot, new drug search, etc.\n\nThis article will introduce you how to use multiple models for image search based on ONNX and Milvus. It takes VGG16 and ResNet50 models as examples, uses ONNX to run different AI models to generate feature vectors, and finally performs feature vector retrieval in Milvus to return similar images. \n\n## Process Models with ONNX\n\nThe ONNX format can be easily exchanged between AI models. For example, the TensorFlow model can be converted to ONNX format and run in the Caffe environment. In this example, we convert the pre-trained ResNet50 model under the Keras framework to the ONNX format, and then call the VGG16 model in ONNX format to analyze different models.\n\n```python\nfrom keras.applications.resnet50 import ResNet50\nimport tensorflow as tf\n\n# load keras-resnet50 model and save as a floder\nmodel_resnet50 = ResNet50(include_top=False, pooling='max', weights='imagenet')\ntf.saved_model.save(model_resnet50, \"keras_resnet50_model\")\n\n# convert resnet50 model to onnx\n! python -m tf2onnx.convert --saved-model \"keras_resnet50_model\" --output \"onnx_resnet50.onnx\"\n```\n\nNote: When we used the interface `keras2onnx.convert_keras(model, model.name)` to convert the model, it will return the error `AttributeError:'KerasTensor' object has no attribute'graph'`. Then we can use Python's Bash command to convert according to the solution on Stack Overflow.\n\n## Extract Feature Vectors using Models\n\nAfter converting ResNet50 model into ONNX format, you can extract the feature vector of the picture directly through the inference. Note: Feature vectors need to be normalized after extraction.\n\n```python\n# get the image vectors with onnx model\ndef get_onnx_vectors(onnx_model, img_path):\n img = image.load_img(img_path, target_size=(224, 224))\n x = image.img_to_array(img)\n x = np.expand_dims(x, axis=0)\n x = preprocess_input(x)\n \n sess = onnxruntime.InferenceSession(onnx_model)\n x = x if isinstance(x, list) else [x]\n feed = dict([(input.name, x[n]) for n, input in enumerate(sess.get_inputs())])\n feat = sess.run(None, feed)[0]\n \n norm_feat = feat[0] / LA.norm(feat[0])\n norm_feat = [i.item() for i in norm_feat]\n return norm_feat\n```\n\nUse ONNX-formatted VGG16 model to process image data:\n\n```python\n# generate vectors with ResNet50 and VGG16 ONNX model\n2vec_resnet = get_onnx_vectors(\"onnx_resnet50.onnx\", \"./pic/example.jpg\")\n3vec_vgg = get_onnx_vectors(\"onnx_vgg16.onnx\", \"./pic/example.jpg\")\n```\n\n## Store Vector Data\n\nUnstructured data such as pictures cannot be directly processed by computer, but it can be converted into vectors through AI model and then analyzed by a computer. Milvus vector database is designed power massive unstructured data analysis. It can store vector data and perform near real-time analysis. First, create a collection of the corresponding model in Milvus, and then insert the image vectors.\n\n```python\nfrom milvus import *\n\n# create collections in Milvus\nmilvus.create_collection(resnet_collection_param)\nmilvus.create_collection(vgg_collection_param)\n\n# insert data to Milvus and return ids\nstatus, resnet_ids = milvus.insert(resnet_collection_name, resnet_vectors)\nstatus, vgg_ids = milvus.insert(vgg_collection_name, vgg_vectors)\n```\n\nAfter inserting the data successfully, Milvus will return the ID corresponding to the vector, and then we can find the picture by ID. Since Milvus 1.1 used in this case does not support scalar filtering (which Milvus 2.0 now supports), Redis is used to store the vector ID and the key-value of the image path.\n\n```python\nimport redis\ndef img_ids_to_redis(img_directory, res_ids):\n for img, ids in zip(images, res_ids):\n redis.set(ids, img)\n```\n\n## Search for Similar Images\n\nAfter storing the data, we can retrieve the vector. Milvus supports multiple distance calculation methods, including Euclidean, inner product and Hamming distance. The image similarity search in this article adopts the Euclidean distance calculation between the vectors in Milvus, returns the similar vector ID, and then finds the image corresponding to the ID in Redis.\n\n```python\n# search in Milvus and return the similarly results with ids\ndef search_in_milvus(collection_name, search_vector):\n status, results = milvus.search(collection_name, TOP_K, [search_vector])\n print(status)\n re_ids = [x.id for x in results[0]]\n re_distance = [x.distance for x in results[0]]\n return re_ids, re_distance\n \n# get the images according the result ids\ndef get_sim_imgs(collection_name, search_vector):\n ids, distance = search_in_milvus(collection_name, search_vector)\n img = [red.get(i).decode(\"utf-8\") for i in ids]\n return ids, distance, img\n```\n\nTaking the VGG16 and ResNet50 models as examples, this article shows processing multiple models through ONNX and combining multiple models with Milvus for similar vector retrieval to get similar images. The above two models are based on the Keras framework, which can quickly extract feature vectors. It can be seen from the Notebook that although the results of Milvus's search for pictures on the COCO dataset based on these two models are similar, their Euclidean distances are not the same. You can also try to compare the search results of the two models using other datasets.\n\nMilvus is a high-performance, highly available vector database that can be used to process feature vectors generated from massive unstructured data. For more solutions, you can refer to [Milvus bootcamp](https://github.com/milvus-io/bootcamp).\n\n## References\n\n1. https://github.com/onnx/onnx\n2. https://onnx.ai/\n3. https://milvus.io/cn/\n4. https://github.com/milvus-io/bootcamp\n\n\n\n### About author\n\nShiyu Chen, a data engineer at Zilliz, graduated from Xidian University with a degree in Computer Science. Since joining Zilliz, she has been exploring solutions for Milvus in various fields, such as audio and video analysis, molecule formula retrieval, etc., which has greatly enriched the application scenarios of the community. She is currently exploring more interesting solutions. In her spare time, she loves sports and reading.\n","title":"Combine AI Models for Image Search using ONNX and Milvus","metaData":{}},{"id":"2021-09-24-diskann.md","author":"Zilliz","desc":"Paper reading with Zilliz engineers to learn more about how DiskANN performs on billion-scale dataset.","date":"2021-09-24T00:00:00.000Z","cover":"https://assets.zilliz.com/medium_1_10cebc1e50.png","tags":["Engineering"],"href":"/blog/2021-09-24-diskann.md","content":"\n# DiskANN: A Disk-based ANNS Solution with High Recall and High QPS on Billion-scale Dataset\n\n\u003e Chengming Li, R \u0026 D Engineer of Zilliz, graduated from SouthEast University with a Master degree in Computer Science. His current focus is on ANNS problems on high-dimensional data, including graph-based and quantization-based solutions.\n\n\n\n“DiskANN: Fast Accurate Billion-point Nearest Neighbor Search on a Single Node” is a paper published on NeurIPS in 2019. The paper introduces a state-of-the-art method to perform index building and search on the billion-scale dataset using a single machine with only 64GB of RAM and a large enough SSD. Moreover, it satisfies the three requirements of ANNS (Approximate Nearest Neighbor Search) on the large-scale dataset: high recall, low latency, and high density (number of nodes in a single machine). This method builds a graph-based index on a billion-scale dataset SIFT-1B using a single machine with 64GB of RAM and a 16-core CPU, reaching 5000 QPS (queries per second) at over 95 % recall@1, and the average latency lower than 3ms.\n\n## Authors\n\n**Suhas Jayaram Subramanya**: Former employee of Microsoft India Research Institute, doctoral student of CMU. The main research interests are high-performance computing and machine learning algorithms for large-scale data.\n\n**Devvrit**: Graduate Research Assistant at The University of Texas at Austin. His research interests are theoretical computer science, machine learning, and deep learning.\n\n**Rohan Kadekodi**: A doctoral student at the University of Texas. His research direction is system and storage, mainly including persistent storage, file system, and kV storage.\n\n**Ravishankar Krishaswamy**: Microsoft Indian research institute principal researcher. Doctor of CMU. The research direction is the approximation algorithm based on graph and clustering.\n\n**Harsha Vardhan Simhadri**: Microsoft Indian research institute principal researcher. Doctor of CMU. In the past, he studied parallel algorithms and runtime systems. Now his main work is to develop new algorithms and write programming models.\n\n## Motivations\n\nMost of the mainstream ANNS algorithms make some trade-offs among index building performance, search performance, and recall. Graph-based algorithms such as HNSW and NSG are state-of-art methods in terms of search performance and recall at present. Since the memory-resident graph-based indexing method occupies too much memory, it is relatively difficult to index and search a large-scale dataset using a single machine with limited memory resources.\n\nMany applications require quick responses of Euclidean distance-based ANNS on the billion-scale dataset. Below are two major solutions:\n\n1. Inverted index + quantization: to cluster the dataset into M partitions and compress the dataset using quantization schemes such PQ (Product Quantization). This solution produces low recall because of a loss of precision caused by data compression. Increasing the topk helps improve the recall while the QPS would drop correspondingly.\n2. Divide and index: to divide the dataset into several disjoint shards and build an in-memory index for each shard. When query requests come, the search will be performed on indexes of each shard and the results will be returned after merging. This solution causes the over-expansion of the dataset scale, and thus more machines are needed because of the restriction of memory resources in a single machine, leading to low QPS.\n\nBoth solutions mentioned above are limited by the memory restriction of a single machine. This paper proposes the design of an SSD-resident indexing mechanism to solve this problem. The challenge of SSD-resident indexing is to reduce the number of random disk access and the number of requests for disk access.\n\n## Contributions\n\nThis paper presents an SSD-resident ANNS scheme called DiskANN, which can effectively support search on large-scale datasets. This scheme is based on a graph-based algorithm presented in this paper: Vamana. Contributions of this paper include:\n\n1. DiskANN can index and search a billion-scale dataset of over 100 dimensions on a single machine with 64GB RAM, providing over 95% recall@1 with latencies under 5 milliseconds.\n2. A new graph-based algorithm called Vamana with a smaller search radius than those of NSG and HNSW was proposed to minimize the number of disk access.\n3. Vamana can work in memory and its performance is not slower than NSG and HNSW.\n4. Smaller Vamana indexes built on overlapping partitions of the large dataset can be merged into one graph without losing connectivity.\n5. Vamana can be combined with quantization schemes such as PQ. The graph structure and the original data are stored on the disk while compressed data is kept in memory.\n\n## Vamana\n\nThis algorithm is similar to the idea of NSG[2][4] (for those who don't understand NSG, please refer to Reference [2], and if you do not want to read papers, you can refer to Reference [4]). Their main difference lies in the trimming strategy. To be precise, a switch alpha has been added to the NSG's trimming strategy. The main idea of the NSG trimming strategy is that the choice of neighbors of the target point is as diverse as possible. If the new neighbor is closer to a neighbor of the target point than the target point, we do not need to add this point to the neighbor point set. In other words, for each neighbor of the target point, there can be no other neighbor points within the surrounding radius dist (target point, neighbor point). This trimming strategy effectively controls the out-degree of graph, and is relatively radical. It reduces the memory footprint of the index, improves the search speed, but also reduces the search accuracy. Vamana's trimming strategy is to freely control the scale of trimming through the parameter alpha. The working principle is to multiply the dist (a neighbor point, candidate point) in the trimming condition with a parameter alpha (not less than 1). Only when the dist (target point, a certain candidate point) is greater than the enlarged reference distance is the trimming strategy adopted, increasing the tolerance of mutual exclusion between neighbors of the target point.\n\nVamana's indexing process is relatively simple:\n\n1. Initialize a random graph;\n2. Calculate the starting point, which is similar to the navigation point of NSG. First, find the global centroid, and then find the point closest to the global centroid as the navigation point. The difference between Vamana and NSG is that the input of NSG is already a nearest neighbor graph, so users can simply do an approximate nearest neighbor search on the centroid point directly on the initial neighbor graph. However, Vamana initializes a random nearest neighbor graph, thus users cannot conduct approximate search directly on the random graph. They need to do a global comparison to get a navigation point as the starting point of subsequent iterations. The purpose of this point is to minimize the average search radius;\n3. Perform Approximate Nearest Neighbor Search on each point based on the initialized random neighbor graph and the search starting point determined in step 2, make all points on the search path the candidate neighbor sets, and execute the edge trimming strategy using alpha = 1. Similar to that of NSG, selecting the point set on the search path starting from the navigation point as the candidate neighbor set will increase some long edges and effectively reduce the search radius.\n4. Adjust alpha \u003e 1 (the paper recommends 1.2) and repeat step 3. Whereas step 3 is based on a random nearest neighbor graph, the graph is low-quality after the first iteration. Therefore, another iteration is needed to improve the graph quality, which is very important to the recall rate.\n\nThis paper compares the three graph indexes, i.e. Vamana, NSG, and HNSW. In terms of indexing and query performance, Vamana and NSG are relatively close, and both outmatch HNSW slightly. Refer to the Experiment section below for the data.\n\n\n\nTo visualize the building process of Vamana index, the paper provides a graph, in which 200 two-dimensional points are used to simulate two rounds of iteration. The first row uses alpha = 1 to trim the edges. It can be seen that the trimming strategy is relatively radical, and a large number of edges are trimmed. After increasing the value alpha and loosening the trimming conditions, a lot of edges are obviously added back. In the final graph, quite some long edges are added. It can effectively reduce the search radius.\n\n## DiskANN\n\nA personal computer with only 64GB of memory would not even hold a billion pieces of raw data, let alone the index built on them. There are two challenges ahead: 1. How to index such a large-scale data set with limited memory resources? 2. How to calculate the distance when searching if the original data cannot be loaded in memory?\n\nThe paper proposed the following solutions:\n\n1. For the first challenge: first, divide the data into k clusters using k-means, and then allocate each point into nearest i clusters. Generally, 2 is enough for the number i. Build a memory-based Vamana index for each cluster, and finally merge k Vamana indexes into one.\n2. For the second challenge: build index on the original vectors and query compressed vectors. Building indexes on the original vector ensures the quality of the graph, while the compressed vector can be loaded in the memory for coarse-grained search. Although searching with the compressed vectors may cause a loss of accuracy, the general direction will be correct as long as the quality of the graph is high enough. The final distance result will be calculated using the original vector.\n\nThe index layout of DiskANN is similar to those of the general graph indexes. The neighbor set of each point and the original vector data are stored together. This makes better use of the locality of the data.\n\nAs mentioned earlier, if the index data is stored on the SSD, the number of disk accesses and the disk read and write requests must be reduced as much as possible to ensure low search delay. Therefore DiskANN proposes two optimization strategies:\n\n1. Cache hotspot: cache all points within C jumps from the starting point in memory. The value of C is better set within 3 to 4.\n2. Beam search: Simply put, it is to preload the neighbor information. When searching for point p, the neighbor point of p needs to be loaded from the disk if it is not in memory. Since a small amount of SSD random access operation takes about the same time as an SSD single-sector access operation, the neighbor information of W non-accessed points can be loaded at a time. W cannot be set too large or small. A large W will waste computing resources and SSD bandwidth, while a small one will increase the search delay.\n\n## Experiment\n\nThe experiment consists of three groups:\n\n#### Comparison among memory-based indexes: Vamana VS. NSG VS. HNSW\n\nData sets: SIFT1M (128 dimensions), GIST1M (960 dimensions), DEEP1M (96 dimensions) and a 1M data set randomly sampled from DEEP1B.\n\nIndex parameters (all data sets use the same set of parameters):\n\nHNSW:M = 128, efc = 512.\n\nVamana: R = 70, L = 75, alpha = 1.2.\n\nNSG: R = 60, L = 70, C= 500.\n\nThe search parameters are not provided in the paper, which may be consistent with the indexing parameters. For the parameter selection, the parameters of NSG mentioned in the article are based on the parameters listed in the GitHub repository of NSG to select the group with better performance. Vamana and NSG are relatively close, so the parameters are also set close. However, the reason of HNSW parameters selection is not given. We believe that the parameter M of HNSW is set relatively large. It might lead to a less convincing comparison between graph-based indexes if their out-degrees are not set at the same level.\n\nUnder the above indexing parameters, the indexing time of Vamana, HNSW, and NSG are 129s, 219s, and 480s respectively. The NSG indexing time includes the time to construct the initial neighbor graph with EFANN [3].\n\nRecall-QPS curve:\n\n\n\nIt can be seen from Figure 3 that Vamana has an excellent performance on the three data sets, similar to NSG and slightly better than HNSW.\n\nComparison of search radius:\n\nFrom Figure 2.c, we can see that Vamana has the shortest average search path under the same recall rate compared to those of NSG and HNSW.\n\n#### Comparison between a one-time built index and a large merged index\n\nData set: SIFT1B\n\nThe one-time built index parameters: L = 50, R = 128, alpha = 1.2. After running for 2 days on a 1800G DDR3 machine, the peak memory is about 1100 G, and the average out-degree is 113.9.\n\nIndexing procedure based on the merging:\n\n1. Train 40 clusters on the dataset using kmeans;\n2. Each point is distributed into the nearest 2 clusters;\n3. Build a Vamana index with L = 50, R = 64, and alpha = 1.2 for each cluster;\n4. Merge the indexes of each cluster.\n\nThis index generated a 384GB index with an average out-of-degree of 92.1. This index ran for 5 days on a 64GB DDR4 machine.\n\nThe comparison results are as follows (Figure 2a): \n\nIn conclusion:\n\n1. The one-time built index is significantly better than the merging-based index;\n2. The merging-based index is also excellent;\n3. The merging-based indexing scheme is also applicable to the DEEP1B data set (Figure 2b).\n\n#### Disk-based index: DiskANN VS. FAISS VS. IVF-OADC+G+P\n\nIVFOADC+G+P is an algorithm proposed in Reference [5].\n\nThis paper only compares DiskANN with IVFOADC+G+P, since the reference [5] has proved that IVFOADC+G+P is better than FAISS. In addition, FAISS requires GPU resources, which are not supported by all platforms.\n\nIVF-OADC+G+P seems to be a combination of HNSW and IVF-PQ. It determines clusters using HNSW, and performs search by adding some pruning strategies to the target cluster.\n\nThe result is in Figure 2a. The 16 and 32 in the figure are the codebook size. The dataset is SIFT1B, quantified by OPQ.\n\n#### Code implementation details\n\nThe source code of DiskANN is open-sourced on https://github.com/microsoft/DiskANN\n\nIn January 2021, the source code of the disk solution was open-sourced.\n\nThe following mainly introduces the indexing process and the search process.\n\n**Index building**\n\nThere are 8 parameters for building index:\n\ndata_type: options include float/int8/uint8.\n\ndata_file.bin: The original data binary file. The first two integers in the file respectively represent the total number n of the dataset vector and the vector dimension dim. The last n *dim* sizeof(data_type) bytes are continuous vector data.\n\nindex_prefix_path: The path prefix of the output file. After the index is built, several index-related files will be generated. This parameter is the common prefix of the directory where they are stored.\n\nR: The maximum out-degree of the global index.\n\nL: The parameter L of Vamana index, the upper bound of the candidate set size.\n\nB: The memory threshold when querying. It controls the PQ codebook size, in GB.\n\nM: The memory threshold when building an index. It determines the size of the fragment, in GB.\n\nT: The number of threads.\n\nIndexing process (entry function: aux_utils.cpp::build_disk_index):\n\n1. Generate various output file names according to index_prefix_path.\n2. Parameter check.\n3. Read the meta of data_file.bin to get n and dim. Determine the codebook subspace number m of PQ according to B and n.\n4. generate_pq_pivots: Sample the center point of the PQ training set using the sampling rate of p = 1500000/n uniformly to train PQ globally.\n5. generate_pq_data_from_pivots: Generate global PQ codebook, and save the center point and codebook separately.\n6. build_merged_vamana_index: slice the original data set, build Vamana indexes in segments, and finally merge the indexes in one.\n\n- partition_with_ram_budget: Determine the number of fragments k according to the parameter M. Sample the data set using kmeans, disributing each point to two nearest clusters. Fragment the dataset, and each fragment produces two files: a data file and an ID file. The ID file and the data file correspond to each other, and each ID in the ID file corresponds to a vector in the data file. The ID are obtained by numbering each vector of the original data from 0 to n-1. The ID is relatively important and is related to the merge.\n - Globally uniformly sample the training set with a sampling rate of 1500000 / n;\n - Initialize num_parts = 3. Iterate from 3:\n - Do num_parts-means++ on the training set in step i;\n - Use a sampling rate of 0.01 to sample a test set uniformly globally, and divide the test set into the nearest 2 clusters;\n - Count the number of points in each cluster and divide it by the sampling rate to estimate the number of points in each cluster;\n - Estimate the memory required by the largest cluster in step 3 according to the Vamana index size, if it does not exceed the parameter M, go on to step iii, otherwise num_parts ++ go back to step 2;\n - Divide the original data set into num_parts group files, each group of files includes fragmented data files and ID files corresponding to the fragmented data.\n- Create Vamana indexes separately for all the slices in step a and save them to disk;\n- merge_shards: merge num_parts shard Vamana into a global index:\n - Read the ID file of num_parts fragments into idmap. This idmap is equivalent to establishing a forward mapping of fragment-\u003eid;\n - Establish a reverse mapping of id-\u003e fragments according to idmap, and know which two fragments each vector is in;\n - Use a reader with 1GB cache to open num_parts slice Vamana indexes, and use a writer with 1GB cache to open the output file, ready to merge;\n - Place num_parts navigation points of Vamana index into the center point file, which will be used when searching;\n - Start merging according to ID from small to large, read the neighbor point set of each original vector in each fragment in turn according to the reverse mapping, deduplicate, shuffle, truncate, and write to the output file. Because the slicing was originally globally ordered, and now the merging is also in order, so the ID in the final flushed index and the ID of the original data are one-to-one correspondence.\n - Delete temporary files, including fragment files, fragment indexes, and fragment ID files.\n\n7. create_disk_layout: The global index generated in step 6 only has only a compact adjacency table. This step is to align the index. The adjacency table and the original data are stored together. When searching, load the adjacency table and read the original vector together for accurate distance calculation. There is also the concept of SECTOR, with the default size is 4096. Each SECTOR only contains 4096 / node_size pieces of vector information. node_size = single vector size + single node adjacency table size.\n\n8. Finally, do a global uniform sampling of 150000 / n, save it, and use it for warmup when searching.\n\n**Search**\n\nThere are 10 search parameters:\n\n- index_type: Options include Float/int8/uint8, similar to the first parameter data_type when building an index.\n- index_prefix_path: Refer to index parameter index_prefix_path.\n- num_nodes_to_cache: Number of cache hotspots.\n- num_threads: Number of search threads.\n- beamwidth: Upper limit of the number of preload points. The system determines if it is set 0.\n- query_file.bin: Query set file.\n- truthset.bin: Result set file, \"null\" means that the result set is not provided, the program calculates it by itself;\n- K: topk;\n- result_output_prefix: Path to save search results;\n- L*: Search parameter list. Multiple values can be added. For each L, statistical information will be given while searching with different L.\n\nSearch process:\n\n1. Load related data: load query set, PQ center point data, codebook data, search starting point and other data, and read index meta.\n2. Use the data set sampled during indexing to do cached_beam_search, count the access times of each point, and load num_nodes_to_cache points with the highest access frequency to the cache.\n3. There is a WARMUP operation by default. Like step 2, this sample data set is also used to do a cached_beam_search.\n4. According to the number of parameters L given, each L will be performed with cached_beam_search again with the query set, and statistics such as recall rate and QPS will be output. The process of warmup and statistics hotspot data is not counted in the query time.\n\nAbout cached_beam_search:\n\n1. Find the closest candidate to the query point from the candidate starting point. The PQ distance is used here, and the starting point is added to the search queue.\n2. Start searching:\n\n- From the search queue, there are no more than beam_width + 2 unvisited points. If these points are in the cache, add them to the cache hit queue. If they are not hit, add them to the miss queue. Make sure that the size of the miss queue does not exceed beam_width.\n- Send asynchronous disk access requests to points in the miss queue.\n- For the points hit by the cache, use the original data and the query data to calculate the exact distance, add to the result queue, and then use PQ to calculate the distance to the neighbor points that have not been visited before adding to the search queue. The length of the search queue is limited by parameters.\n- Process the cached miss points in step a, similar to step c.\n- When the search queue is empty, the search ends, and the result queue topk is returned.\n\n#### Summarize\n\nAlthough this is a relatively lengthy piece of work, it is overall excellent. The paper and code ideas are clear: divide a number of overlapping buckets through k-means, and then divide the buckets to build a map index, and finally merge the indexes, which is a relatively new idea. As for the memory-based graph index Vamana, it is essentially a randomly initialized version of NSG that can control the trimming granularity. When querying, it makes full use of cache + pipeline, covers up part of the io time, and improves QPS. However, according to the paper, even if the machine condition is not extraordinary, the training time takes up to 5 days, and the usability is relatively low. Optimizations to the training are definitely necessary in the future. From the code perspective, the quality is relatively high and can be directly used in the production environment.\n\n\n\n#### References\n\n1. [Suhas Jayaram Subramanya, Fnu Devvrit, Harsha Vardhan Simhadri, Ravishankar Krishnawamy, Rohan Kadekodi. DiskANN: Fast Accurate Billion-point Nearest Neighbor Search on a Single Node. NeurIPS 2019.](https://www.microsoft.com/en-us/research/publication/diskann-fast-accurate-billion-point-nearest-neighbor-search-on-a-single-node/)\n2. [Cong Fu, Chao Xiang, Changxu Wang, and Deng Cai. Fast approximate nearest neighbor search with the navigating spreading-out graphs. PVLDB, 12(5):461 – 474, 2019. doi: 10.14778/3303753.3303754.] (http://www.vldb.org/pvldb/vol12/p461-fu.pdf)\n3. Cong Fu and Deng Cai. [GitHub - ZJULearning/efanna: fast library for ANN search and KNN graph construction.](https://github.com/ZJULearning/efanna)\n4. [Search Engine For AI:高维数据检索工业级解决方案](https://zhuanlan.zhihu.com/p/50143204)\n\n5.[ Dmitry Baranchuk, Artem Babenko, and Yury Malkov. Revisiting the inverted indices for billion-scale approximate nearest neighbors.](https://arxiv.org/abs/1802.02422)","title":"DiskANN, A Disk-based ANNS Solution with High Recall and High QPS on Billion-scale Dataset","metaData":{}},{"id":"2021-09-15-Implement-Milvus-CLI-by-Python-Click.md","author":"Zhen Chen","desc":"Introduce how to implement a CLI based on Python Click.","isPublish":true,"date":"2021-09-15T00:00:00.000Z","cover":"https://zilliz-cms.s3.us-west-2.amazonaws.com/pc_blog_8ed7696269.jpg","tags":["Engineering"],"href":"/blog/2021-09-15-Implement-Milvus-CLI-by-Python-Click.md","content":"\n# Implement Milvus CLI by Python Click\n\n- [Implement Milvus CLI by Python Click](#Implement-Milvus-CLI-by-Python-Click)\n - [Overview](#Overview)\n - [Group commands](#Group-commands)\n - [Custom a command](#Custom-a-command)\n - [Implement prompt CLI for user to input](#Implement-prompt-cli-for-user-to-input)\n - [Manually implement autocomplete](#Manually-implement-autocomplete)\n - [Add one-time option](#Add-one-time-option)\n - [Build and release](#Build-and-release)\n - [Learn more about Milvus](#Learn-more-about-Milvus)\n\n## Overview\n\nProject URL: https://github.com/milvus-io/milvus_cli\n\nPreparation: `Python3.8`,[ `Click 8.0.x`](https://click.palletsprojects.com/en/8.0.x/api/)\n\n## Group commands\n\n### Create a command\n\n```python\nimport click\nfrom utils import PyOrm\n\n@click.group(no_args_is_help=False, add_help_option=False, invoke_without_command=True)\n@click.pass_context\ndef cli(ctx):\n \"\"\"Milvus CLI\"\"\"\n ctx.obj = PyOrm() # PyOrm is a util class which wraps the milvus python SDK. You can pass any class instance here. Any command function passed by @click.obj can call it.\n\nif __name__ == '__main__':\n cli()\n```\n\nAs the code above, we use `@click.group()` to create a command group `cli` as entry point. To implement a prompt CLI we need to disable help messages for the entry, so we add `no_args_is_help=False`, `add_help_option=False` and `invoke_without_command=True`. And nothing will be printed if we input `cli` in terminal only.\n\nBesides we use `@click.pass_context` to pass a context to this group for further usage.\n\n### Create a sub command of command group\n\nThen we add the first sub command `help` under `cli`:\n\n```python\n# Print the help message of specified command.\ndef print_help_msg(command):\n with click.Context(command) as ctx:\n click.echo(command.get_help(ctx))\n\n\n# Use @cli.command() to create a sub command of cli.\n@cli.command()\ndef help():\n \"\"\"Show help messages.\"\"\"\n # Print help message of cli.\n click.echo(print_help_msg(cli))\n```\n\nNow we can use `cli help` in terminal:\n\n```shell\n$ python milvus_cli/scripts/milvus_cli.py help\n```\n\n### Create a sub group of a command group\n\nNot only we want to have a sub command like `cli help`, but also we need a sub group commands such as `cli list collection` , `cli list partition` and `cli list indexes`.\n\nFirst we create a sub group command `list`, here we can pass the first parameter to `@cli.group` as the command name instead of use defult function name, so that we can reduce duplicated function names.\n\nAttention here, we use `@cli.group()` instead of `@click.group` so that we create a sub group of origin group.\n\nThe we use `@click.pass_obj` to pass the `context.obj` to sub commands of this sub group.\n\n```python\n@cli.group('list', no_args_is_help=False)\n@click.pass_obj\ndef listDetails(obj):\n \"\"\"List collections, partitions and indexes.\"\"\"\n pass\n```\n\nThen we add some sub commands into this sub group by `@listDetails.command()` (not `@cli.command()`). Here's just an example, you can ignore the implement and we will discuss it later.\n\n```python\n@listDetails.command()\n@click.option('--timeout', 'timeout', help=\"[Optional] - An optional duration of time in seconds to allow for the RPC. When timeout is set to None, client waits until server response or error occur.\", default=None)\n@click.option('--show-loaded', 'showLoaded', help=\"[Optional] - Only show loaded collections.\", default=False)\n@click.pass_obj\ndef collections(obj, timeout, showLoaded):\n \"\"\"List all collections.\"\"\"\n try:\n obj.checkConnection()\n click.echo(obj.listCollections(timeout, showLoaded))\n except Exception as e:\n click.echo(message=e, err=True)\n\n\n@listDetails.command()\n@click.option('-c', '--collection', 'collection', help='The name of collection.', default='')\n@click.pass_obj\ndef partitions(obj, collection):\n \"\"\"List all partitions of the specified collection.\"\"\"\n try:\n obj.checkConnection()\n validateParamsByCustomFunc(\n obj.getTargetCollection, 'Collection Name Error!', collection)\n click.echo(obj.listPartitions(collection))\n except Exception as e:\n click.echo(message=e, err=True)\n```\n\nAfter all these complete, we have a miltigroup commands that look like:\n\n\n\n## Custom a command\n\n### Add options\n\nYou can add some options to a command which will be used like `cli --test-option value`.\n\nHere's an example, we add three options `alias`, `host` and `port` to specified an address to connect to Milvus.\n\nFirst two parameters define the short and full option name, the third parameter defines the variable name, the `help` parameter specifies the short help message, the `default` parameter specifies the default value and the `type` specifies the value type.\n\nAnd all options' values will be passed into the function in order of definition.\n\n```python\n@cli.command(no_args_is_help=False)\n@click.option('-a', '--alias', 'alias', help=\"Milvus link alias name, default is `default`.\", default='default', type=str)\n@click.option('-h', '--host', 'host', help=\"Host name, default is `127.0.0.1`.\", default='127.0.0.1', type=str)\n@click.option('-p', '--port', 'port', help=\"Port, default is `19530`.\", default=19530, type=int)\n@click.pass_obj\ndef connect(obj, alias, host, port):\n pass\n```\n\n### Add flag options\n\nWe use options above to pass a value, but some times we just need a flag as a boolean value.\n\nAs the example below, option `autoId` is a flag option and don't pass any data to function, so we can use it like `cli create collection -c c_name -p p_name -a`.\n\n```python\n@createDetails.command('collection')\n@click.option('-c', '--collection-name', 'collectionName', help='Collection name to be created.', default='')\n@click.option('-p', '--schema-primary-field', 'primaryField', help='Primary field name.', default='')\n@click.option('-a', '--schema-auto-id', 'autoId', help='Enable auto id.', default=False, is_flag=True)\n@click.pass_obj\ndef createCollection(obj, collectionName, primaryField, autoId, description, fields):\n pass\n```\n\n### Add arguments\n\nIn this project we replace all arguments usage with options usage. But we still introduce argument usage here. Different from options, argements are used like `cli COMMAND [OPTIONS] ARGUEMENTS`. If we convert the example above into arguements usage, it'll be like this:\n\n```python\n@createDetails.command('collection')\n@click.argument('collectionName')\n@click.option('-p', '--schema-primary-field', 'primaryField', help='Primary field name.', default='')\n@click.option('-a', '--schema-auto-id', 'autoId', help='Enable auto id.', default=False, is_flag=True)\n@click.pass_obj\ndef createCollection(obj, collectionName, primaryField, autoId, description, fields):\n pass\n```\n\nThen the usage should be `cli create collection c_name -p p_name -a`.\n\n### Add full help message\n\nAs we define the short help message above, we can define the full help message in function:\n\n```python\n@cli.command(no_args_is_help=False)\n@click.option('-a', '--alias', 'alias', help=\"Milvus link alias name, default is `default`.\", default='default', type=str)\n@click.option('-h', '--host', 'host', help=\"Host name, default is `127.0.0.1`.\", default='127.0.0.1', type=str)\n@click.option('-p', '--port', 'port', help=\"Port, default is `19530`.\", default=19530, type=int)\n@click.pass_obj\ndef connect(obj, alias, host, port):\n \"\"\"\n Connect to Milvus.\n\n Example:\n\n milvus_cli \u003e connect -h 127.0.0.1 -p 19530 -a default\n \"\"\"\n try:\n obj.connect(alias, host, port)\n except Exception as e:\n click.echo(message=e, err=True)\n else:\n click.echo(\"Connect Milvus successfully!\")\n click.echo(obj.showConnection(alias))\n```\n\nThe first block inside of the function is the help message which will be printed after we input `cli connect --help`.\n\n```shell\nmilvus_cli \u003e connect --help\nUsage: milvus_cli.py connect [OPTIONS]\n\n Connect to Milvus.\n\n Example:\n\n milvus_cli \u003e connect -h 127.0.0.1 -p 19530 -a default\n\nOptions:\n -a, --alias TEXT Milvus link alias name, default is `default`.\n -h, --host TEXT Host name, default is `127.0.0.1`.\n -p, --port INTEGER Port, default is `19530`.\n --help Show this message and exit.\n```\n\n### Add confirm\n\nSometimes we need user to confirm some action especially delete something. We can add `click.confirm` to pause and ask user to confirm:\n\n```python\n@deleteSth.command('collection')\n@click.option('-c', '--collection', 'collectionName', help='The name of collection to be deleted.', default='')\n@click.option('-t', '--timeout', 'timeout', help='An optional duration of time in seconds to allow for the RPC. If timeout is set to None, the client keeps waiting until the server responds or an error occurs.', default=None, type=int)\n@click.pass_obj\ndef deleteCollection(obj, collectionName, timeout):\n \"\"\"\n Drops the collection together with its index files.\n\n Example:\n\n milvus_cli \u003e delete collection -c car\n \"\"\"\n click.echo(\n \"Warning!\\nYou are trying to delete the collection with data. This action cannot be undone!\\n\")\n if not click.confirm('Do you want to continue?'):\n return\n pass\n```\n\nAs the example above, a confirm conversation will show like `Aborted!ant to continue? [y/N]:`.\n\n### Add prompts\n\nTo implement prompts we juest need to add `click.prompt`.\n\n```python\n@cli.command()\n@click.pass_obj\ndef query(obj):\n \"\"\"\n Query with a set of criteria, and results in a list of records that match the query exactly.\n \"\"\"\n collectionName = click.prompt(\n 'Collection name', type=click.Choice(obj._list_collection_names()))\n expr = click.prompt('The query expression(field_name in [x,y])')\n partitionNames = click.prompt(\n f'The names of partitions to search(split by \",\" if multiple) {obj._list_partition_names(collectionName)}', default='')\n outputFields = click.prompt(\n f'Fields to return(split by \",\" if multiple) {obj._list_field_names(collectionName)}', default='')\n timeout = click.prompt('timeout', default='')\n pass\n```\n\nThe prompt will show when each `click.prompt`. We use a few prompts in series so that it'll look like a continuously conversation. This ensure user will input the data in order we want. In this case we need user to choose a collection first, and we need to get all partitions under this collections, then show them to user to choose.\n\n### Add choices\n\nSometimes you want user just input the limited range/type of value, you can add `type=click.Choice([\u003cany\u003e])` to `click.prompt` , `click.options` and etc..\n\nSuch as,\n\n```python\ncollectionName = click.prompt(\n 'Collection name', type=click.Choice(['collection_1', 'collection_2']))\n```\n\nThen user can only input `collection_1` or `collection_2` , error will be raised if any other inputs.\n\n### Add clear screen\n\nYou can use `click.clear()` to implement it.\n\n```python\n@cli.command()\ndef clear():\n \"\"\"Clear screen.\"\"\"\n click.clear()\n```\n\n### Additional tips\n\n- Default value is `None`, so it's meaningless if you specified the default value as `None`. And default `None` will cause `click.prompt` continously show if you want to leave a value empty to jump over it.\n\n## Implement prompt CLI for user to input\n\n### Why prompt CLI\n\nFor database operation, we need a continuously connection to a instance. If we use origin command line mode, the connection will be dropped after each command performed. We also want to store some data when using CLI, and clean them after exit.\n\n### Implement\n\n1. Use `while True` for continuously listening user's input.\n\n```python\ndef runCliPrompt():\n while True:\n astr = input('milvus_cli \u003e ')\n try:\n cli(astr.split())\n except SystemExit:\n # trap argparse error message\n # print('error', SystemExit)\n continue\n\n\nif __name__ == '__main__':\n runCliPrompt()\n```\n\n2. Use `input` only will cause `up`, `down`, `left`, `right` arrow keys, `tab` key and some other keys converted to Acsii string automatically. Besides history commands can not be read from session. So we add `readline` into `runCliPrompt` function.\n\n```python\ndef runCliPrompt():\n while True:\n \t\timport readline\n readline.set_completer_delims(' \\t\\n;')\n astr = input('milvus_cli \u003e ')\n try:\n cli(astr.split())\n except SystemExit:\n # trap argparse error message\n # print('error', SystemExit)\n continue\n```\n\n3. Add `quit` CLI.\n\n```python\n@cli.command('exit')\ndef quitapp():\n \"\"\"Exit the CLI.\"\"\"\n global quitapp\n quitapp = True\n\n\nquitapp = False # global flag\n\n\ndef runCliPrompt():\n while not quitapp:\n \t\timport readline\n readline.set_completer_delims(' \\t\\n;')\n astr = input('milvus_cli \u003e ')\n try:\n cli(astr.split())\n except SystemExit:\n # trap argparse error message\n # print('error', SystemExit)\n continue\n```\n\n4. Catch `KeyboardInterrupt` error when use `ctrl C` to exit.\n\n```python\ndef runCliPrompt():\n try:\n while not quitapp:\n import readline\n readline.set_completer_delims(' \\t\\n;')\n astr = input('milvus_cli \u003e ')\n try:\n cli(astr.split())\n except SystemExit:\n # trap argparse error message\n # print('error', SystemExit)\n continue\n except KeyboardInterrupt:\n sys.exit(0)\n```\n\n5. After all settled, the CLI now looks like:\n\n```shell\nmilvus_cli \u003e\nmilvus_cli \u003e connect\n+-------+-----------+\n| Host | 127.0.0.1 |\n| Port | 19530 |\n| Alias | default |\n+-------+-----------+\n\nmilvus_cli \u003e help\nUsage: [OPTIONS] COMMAND [ARGS]...\n\n Milvus CLI\n\nCommands:\n clear Clear screen.\n connect Connect to Milvus.\n create Create collection, partition and index.\n delete Delete specified collection, partition and index.\n describe Describe collection or partition.\n exit Exit the CLI.\n help Show help messages.\n import Import data from csv file with headers and insert into target...\n list List collections, partitions and indexes.\n load Load specified collection.\n query Query with a set of criteria, and results in a list of...\n release Release specified collection.\n search Conducts a vector similarity search with an optional boolean...\n show Show connection, loading_progress and index_progress.\n version Get Milvus CLI version.\n\nmilvus_cli \u003e exit\n```\n\n## Manually implement autocomplete\n\nDifferent from click's shell autocomplete, our project wrap the command line and use a loop to get user's input to implement a prompt command line. So we need to bind a completer to `readline`.\n\n```python\nclass Completer(object):\n RE_SPACE = re.compile('.*\\s+$', re.M)\n CMDS_DICT = {\n 'clear': [],\n 'connect': [],\n 'create': ['collection', 'partition', 'index'],\n 'delete': ['collection', 'partition', 'index'],\n 'describe': ['collection', 'partition'],\n 'exit': [],\n 'help': [],\n 'import': [],\n 'list': ['collections', 'partitions', 'indexes'],\n 'load': [],\n 'query': [],\n 'release': [],\n 'search': [],\n 'show': ['connection', 'index_progress', 'loading_progress'],\n 'version': [],\n }\n\n def __init__(self) -\u003e None:\n super().__init__()\n self.COMMANDS = list(self.CMDS_DICT.keys())\n self.createCompleteFuncs(self.CMDS_DICT)\n\n def createCompleteFuncs(self, cmdDict):\n for cmd in cmdDict:\n sub_cmds = cmdDict[cmd]\n complete_example = self.makeComplete(cmd, sub_cmds)\n setattr(self, 'complete_%s' % cmd, complete_example)\n\n def makeComplete(self, cmd, sub_cmds):\n def f_complete(args):\n f\"Completions for the {cmd} command.\"\n if not args:\n return self._complete_path('.')\n if len(args) \u003c= 1 and not cmd == 'import':\n return self._complete_2nd_level(sub_cmds, args[-1])\n return self._complete_path(args[-1])\n return f_complete\n\n def _listdir(self, root):\n \"List directory 'root' appending the path separator to subdirs.\"\n res = []\n for name in os.listdir(root):\n path = os.path.join(root, name)\n if os.path.isdir(path):\n name += os.sep\n res.append(name)\n return res\n\n def _complete_path(self, path=None):\n \"Perform completion of filesystem path.\"\n if not path:\n return self._listdir('.')\n dirname, rest = os.path.split(path)\n tmp = dirname if dirname else '.'\n res = [os.path.join(dirname, p)\n for p in self._listdir(tmp) if p.startswith(rest)]\n # more than one match, or single match which does not exist (typo)\n if len(res) \u003e 1 or not os.path.exists(path):\n return res\n # resolved to a single directory, so return list of files below it\n if os.path.isdir(path):\n return [os.path.join(path, p) for p in self._listdir(path)]\n # exact file match terminates this completion\n return [path + ' ']\n\n def _complete_2nd_level(self, SUB_COMMANDS=[], cmd=None):\n if not cmd:\n return [c + ' ' for c in SUB_COMMANDS]\n res = [c for c in SUB_COMMANDS if c.startswith(cmd)]\n if len(res) \u003e 1 or not (cmd in SUB_COMMANDS):\n return res\n return [cmd + ' ']\n\n def complete(self, text, state):\n \"Generic readline completion entry point.\"\n buffer = readline.get_line_buffer()\n line = readline.get_line_buffer().split()\n # show all commands\n if not line:\n return [c + ' ' for c in self.COMMANDS][state]\n # account for last argument ending in a space\n if self.RE_SPACE.match(buffer):\n line.append('')\n # resolve command to the implementation function\n cmd = line[0].strip()\n if cmd in self.COMMANDS:\n impl = getattr(self, 'complete_%s' % cmd)\n args = line[1:]\n if args:\n return (impl(args) + [None])[state]\n return [cmd + ' '][state]\n results = [\n c + ' ' for c in self.COMMANDS if c.startswith(cmd)] + [None]\n return results[state]\n```\n\nAfter define `Completer` we can bind it with readline:\n\n```python\ncomp = Completer()\n\n\ndef runCliPrompt():\n try:\n while not quitapp:\n import readline\n readline.set_completer_delims(' \\t\\n;')\n readline.parse_and_bind(\"tab: complete\")\n readline.set_completer(comp.complete)\n astr = input('milvus_cli \u003e ')\n try:\n cli(astr.split())\n except SystemExit:\n # trap argparse error message\n # print('error', SystemExit)\n continue\n except KeyboardInterrupt:\n sys.exit(0)\n```\n\n## Add one-time option\n\nFor prompt command line, sometimes we don't want to fully run into the scripts to get some informations such as version. A good example is `Python`, when you type `python` in terminal the promtp command line will show, but it only returns a version message and will not entry the prompt scripts if you type `python -V`. So we can use `sys.args` in our code to implement.\n\n```python\ndef runCliPrompt():\n args = sys.argv\n if args and (args[-1] == '--version'):\n print(f\"Milvus Cli v{getPackageVersion()}\")\n return\n try:\n while not quitapp:\n import readline\n readline.set_completer_delims(' \\t\\n;')\n readline.parse_and_bind(\"tab: complete\")\n readline.set_completer(comp.complete)\n astr = input('milvus_cli \u003e ')\n try:\n cli(astr.split())\n except SystemExit:\n # trap argparse error message\n # print('error', SystemExit)\n continue\n except KeyboardInterrupt:\n sys.exit(0)\n\n\nif __name__ == '__main__':\n runCliPrompt()\n```\n\nWe get `sys.args` before the loop when first run into CLI scripts. If the last arguments is `--version` , the code will return the package version without running into loop.\n\nIt will be helpful after we build the codes as a package. User can type `milvus_cli` to jump into a prompt CLI, or type `milvus_cli --version` to only get the version.\n\n## Build and release\n\nFinally we want to build a package and release by PYPI. So that user can simply use `pip install \u003cpackage name\u003e` to install.\n\n### Install locally for test\n\nBefore you publish the package to PYPI, you may want to install it locally for some tests.\n\nIn this case, you can simply `cd` into the package directory and run `pip install -e .` (Don't forget the `.`).\n\n### Create package files\n\nRefer to: https://packaging.python.org/tutorials/packaging-projects/\n\nA package's structure should look like:\n\n```shell\npackage_example/\n├── LICENSE\n├── README.md\n├── setup.py\n├── src/\n│ ├── __init__.py\n│ ├── main.py\n│ └── scripts/\n│ ├── __init__.py\n│ └── example.py\n└── tests/\n```\n\n#### Create the package directory\n\nCreate `Milvus_cli` directory with the structure below:\n\n```shell\nMilvus_cli/\n├── LICENSE\n├── README.md\n├── setup.py\n├── milvus_cli/\n│ ├── __init__.py\n│ ├── main.py\n│ ├── utils.py\n│ └── scripts/\n│ ├── __init__.py\n│ └── milvus_cli.py\n└── dist/\n```\n\n#### Write the entry code\n\nThe script's entry should be in `Milvus_cli/milvus_cli/scripts`, and the `Milvus_cli/milvus_cli/scripts/milvus_cli.py` should be like:\n\n```python\nimport sys\nimport os\nimport click\nfrom utils import PyOrm, Completer\n\n\npass_context = click.make_pass_decorator(PyOrm, ensure=True)\n\n\n@click.group(no_args_is_help=False, add_help_option=False, invoke_without_command=True)\n@click.pass_context\ndef cli(ctx):\n \"\"\"Milvus CLI\"\"\"\n ctx.obj = PyOrm()\n\n\"\"\"\n...\nHere your code.\n...\n\"\"\"\n\n@cli.command('exit')\ndef quitapp():\n \"\"\"Exit the CLI.\"\"\"\n global quitapp\n quitapp = True\n\n\nquitapp = False # global flag\ncomp = Completer()\n\n\ndef runCliPrompt():\n args = sys.argv\n if args and (args[-1] == '--version'):\n print(f\"Milvus Cli v{getPackageVersion()}\")\n return\n try:\n while not quitapp:\n import readline\n readline.set_completer_delims(' \\t\\n;')\n readline.parse_and_bind(\"tab: complete\")\n readline.set_completer(comp.complete)\n astr = input('milvus_cli \u003e ')\n try:\n cli(astr.split())\n except SystemExit:\n # trap argparse error message\n # print('error', SystemExit)\n continue\n except Exception as e:\n click.echo(\n message=f\"Error occurred!\\n{str(e)}\", err=True)\n except KeyboardInterrupt:\n sys.exit(0)\n\n\nif __name__ == '__main__':\n runCliPrompt()\n```\n\n#### Edit the `setup.py`\n\n```python\nfrom setuptools import setup, find_packages\n\nwith open(\"README.md\", \"r\", encoding=\"utf-8\") as fh:\n long_description = fh.read()\n\nsetup(\n name='milvus_cli',\n version='0.1.6',\n author='Milvus Team',\n author_email='milvus-team@zilliz.com',\n url='https://github.com/milvus-io/milvus_cli',\n description='CLI for Milvus',\n long_description=long_description,\n long_description_content_type='text/markdown',\n license='Apache-2.0',\n packages=find_packages(),\n include_package_data=True,\n install_requires=[\n 'Click==8.0.1',\n 'pymilvus==2.0.0rc5',\n 'tabulate==0.8.9'\n ],\n entry_points={\n 'console_scripts': [\n 'milvus_cli = milvus_cli.scripts.milvus_cli:runCliPrompt',\n ],\n },\n python_requires='\u003e=3.8'\n)\n```\n\nSome tips here:\n\n1. We use `README.md` content as the package's long description.\n2. Add all dependencies to `install_requires`.\n3. Specify the `entry_points`. In this case, we set `milvus_cli` as a child of `console_scripts`, so that we can type `milvus_cli` as a command directly after we install this package. And the `milvus_cli`'s entry point is `runCliPrompt` function in `milvus_cli/scripts/milvus_cli.py`.\n\n#### Build\n\n1. Upgrade the `build` package: `python3 -m pip install --upgrade build`\n\n2. Run build: `python -m build --sdist --wheel --outdir dist/ .`\n3. Two files will be generated under the `dist/` directory:\n\n```shell\ndist/\n example_package_YOUR_USERNAME_HERE-0.0.1-py3-none-any.whl\n example_package_YOUR_USERNAME_HERE-0.0.1.tar.gz\n```\n\n### Publish release\n\nRefer to: https://packaging.python.org/tutorials/packaging-projects/#uploading-the-distribution-archives\n\n1. Upgrade `twine` package: `python3 -m pip install --upgrade twine`\n2. Upload to `PYPI` test env: `python3 -m twine upload --repository testpypi dist/*`\n3. Upload to `PYPI` : `python3 -m twine upload dist/*`\n\n### CI/CD by Github workflows\n\nRefer to: https://packaging.python.org/guides/publishing-package-distribution-releases-using-github-actions-ci-cd-workflows/\n\nWe want a way to upload assets automatically, it can build the packages and upload them to github releases and PYPI.\n\n(For some reason we just want the workflow only publish the release to test PYPI.)\n\n```yaml\n# This is a basic workflow to help you get started with Actions\n\nname: Update the release's assets after it published\n\n# Controls when the workflow will run\non:\n release:\n # The workflow will run after release published\n types: [published]\n\n# A workflow run is made up of one or more jobs that can run sequentially or in parallel\njobs:\n # This workflow contains a single job called \"build\"\n build:\n # The type of runner that the job will run on\n runs-on: ubuntu-latest\n\n # Steps represent a sequence of tasks that will be executed as part of the job\n steps:\n # Checks-out your repository under $GITHUB_WORKSPACE, so your job can access it\n - uses: actions/checkout@v2\n - uses: actions/setup-python@v2\n with:\n python-version: '3.8'\n architecture: 'x64'\n - name: Install pypa/build\n run: \u003e-\n python -m\n pip install\n build\n --user\n - name: Clean dist/\n run: |\n sudo rm -fr dist/*\n - name: Build a binary wheel and a source tarball\n run: \u003e-\n python -m\n build\n --sdist\n --wheel\n --outdir dist/\n .\n # Update target github release's assets\n - name: Update assets\n uses: softprops/action-gh-release@v1\n if: startsWith(github.ref, 'refs/tags/')\n with:\n files: ./dist/*\n - name: Publish distribution 📦 to Test PyPI\n if: contains(github.ref, 'beta') \u0026\u0026 startsWith(github.ref, 'refs/tags')\n uses: pypa/gh-action-pypi-publish@release/v1\n with:\n user: __token__\n password: ${{ secrets.TEST_PYPI_API_TOKEN }}\n repository_url: https://test.pypi.org/legacy/\n packages_dir: dist/\n verify_metadata: false\n```\n\n## Learn more about Milvus\nMilvus is a powerful tool capable of powering a vast array of artificial intelligence and vector similarity search applications. To learn more about the project, check out the following resources:\n- Read our [blog](https://milvus.io/blog).\n- Interact with our open-source community on [Slack](https://milvusio.slack.com/join/shared_invite/zt-e0u4qu3k-bI2GDNys3ZqX1YCJ9OM~GQ#/shared-invite/email).\n- Use or contribute to the world’s most popular vector database on [GitHub](https://github.com/milvus-io/milvus/).\n- Quickly test and deploy AI applications with our new [bootcamp](https://github.com/milvus-io/bootcamp).\n","title":"Implement Milvus CLI by Python Click","metaData":{}},{"id":"2021-09-06-dna-sequence-classification-based-on-milvus.md","author":"Jael Gu","desc":"Use Milvus, an open-source vector database, to recognize gene families of DNA sequences. Less space but higher accuracy.","canonicalUrl":"https://zilliz.com/blog/dna-sequence-classification-based-on-milvus","date":"2021-09-06T06:02:27.431Z","cover":"https://assets.zilliz.com/11111_5d089adf08.png","tags":["Scenarios"],"href":"/blog/2021-09-06-dna-sequence-classification-based-on-milvus.md","content":" \n# DNA Sequence Classification based on Milvus\n\u003e Author:\nMengjia Gu, a data engineer at Zilliz, graduated from McGill University with a Master degree in Information Studies. Her interests include AI applications and similarity search with vector databases. As a community member of open-source project Milvus, she has provided and improved various solutions, like recommendation system and DNA sequence classification model. She enjoys challenges and never gives up!\n\n# Introduction\n\nDNA sequence is a popular concept in both academic research and practical applications, such as gene traceability, species identification, and disease diagnosis. Whereas all industries starve for a more intelligent and efficient research method, artificial intelligence has attracted much attention especially from biological and medical domain. More and more scientists and researchers are contributing to machine learning and deep learning in bioinformatics. To make experimental results more convincing, one common option is increasing sample size. The collaboration with big data in genomics as well brings more possibilities of use case in reality. However, the traditional sequence alignment has limitations, which make it [unsuitable for large data](https://www.frontiersin.org/articles/10.3389/fbioe.2020.01032/full#h5). In order to make less trade-off in reality, vectorization is a good choice for a large dataset of DNA sequences.\n\nThe open source vector database [Milvus](https://milvus.io/docs/v2.0.x/overview.md) is friendly for massive data. It is able to store vectors of nucleic acid sequences and perform high-efficiency retrieval. It can also help reduce the cost of production or research. The DNA sequence classification system based on Milvus only takes milliseconds to do gene classification. Moreover, it shows a higher accuracy than other common classifiers in machine learning.\n\n# Data Processing\n\nA gene that encodes genetic information is made up of a small section of DNA sequences, which consists of 4 nucleotide bases [A, C, G, T]. There are about 30,000 genes in human genome, nearly 3 billion DNA base pairs, and each base pair has 2 corresponding bases. To support diverse uses, DNA sequences can be classified into various categories. In order to reduce the cost and make easier use of data of long DNA sequnces, [k-mer ](https://en.wikipedia.org/wiki/K-mer#:~:text=Usually%2C%20the%20term%20k%2Dmer,total%20possible%20k%2Dmers%2C%20where)is introduced to data preprocessing. Meanwhile, it makes DNA sequence data more similar to plain text. Furthermore, vectorized data can speed up calculation in data analysis or machine learning.\n\n\n\n**k-mer**\n\nThe k-mer method is commonly used in DNA sequence preprocessing. It extracts a small section of length k starting from each base of the original sequence, thereby converting a long sequence of length s to (s-k+1) short sequences of length k. Adjusting the value of k will improve the model performance. Lists of short sequences are easier for data reading, feature extraction, and vectorization.\n\n**Vectorization**\n\nDNA sequences are vectorized in the form of text. A sequence transformed by k-mer becomes a list of short sequences, which looks like a list of individual words in a sentence. Therefore, most natural language processing models should work for DNA sequence data as well. Similar methodologies can be applied to model training, feature extraction, and encoding. Since each model has its own advantages and drawbacks, the selection of models depends on the feature of data and the purpose of research. For example, CountVectorizer, a bag-of-words model, implements feature extraction through straightforward tokenization. It sets no limit on data length, but the result returned is less obvious in terms of similarity comparison.\n\n# Milvus Demo\n\nMilvus can easily manage unstructured data and recall most similar results among trillions of vectors within an average delay of milliseconds. Its similarity search is based on Approximate Nearest Neighbor (ANN) search algorithm. These highlights make Milvus a great option to manage vectors of DNA sequences, hence promote the development and applications of bioinformatics.\n\nHere is a demo showing how to build a DNA sequence classification system with Milvus. The [experimental dataset ](https://www.kaggle.com/nageshsingh/dna-sequence-dataset)includes 3 organisms and 7 gene families. All data are converted to lists of short sequences by k-mers. With a pre-trained CountVectorizer model, the system then encodes sequence data into vectors. The flow chart below depicts the system structure and the processes of inserting and searching.\n\n\n\nTry out this demo at [Milvus bootcamp](https://github.com/milvus-io/bootcamp/tree/master/solutions/dna_sequence_classification).\n\nIn Milvus, the system creates collection and inserts corresponding vectors of DNA sequences into the collection (or partition if enabled). When receiving a query request, Milvus will return distances between the vector of input DNA sequence and most similar results in database. The class of input sequence and similarity between DNA sequences can be determined by vector distances in results.\n\n```\n# Insert vectors to Milvus collection (partition \"human\")\nDNA_human = collection.insert([human_ids, human_vectors], partition_name='human')\n# Search topK results (in partition \"human\") for test vectors\nres = collection.search(test_vectors, \"vector_field\", search_params, limit=topK, partition_names=['human'])\nfor results in res:\n res_ids = results.ids # primary keys of topK results\n res_distances = results.distances # distances between topK results \u0026 search input\n```\n\n**DNA Sequence Classification**\nSearching for most similar DNA sequences in Milvus could imply the gene family of an unknown sample, thus learn about its possible functionality.[ If a sequence is classified as GPCRs, then it probably has influence in body functions. ](https://www.nature.com/scitable/topicpage/gpcr-14047471/)In this demo, Milvus has successfully enabled the system to identify the gene families of the human DNA sequences searched with.\n\n\n\n\n**Genetic Similarity**\n\nAverage DNA sequence similarity between organisms illustrates how close between their genomes. The demo searches in human data for most similar DNA sequences as that of chimpanzee and dog respectively. Then it calculates and compares average inner product distances (0.97 for chimpanzee and 0.70 for dog), which proves that chimpanzee shares more similar genes with human than dog shares. With more complex data and system design, Milvus is able to support genetic research even on a higher level.\n\n```\nsearch_params = {\"metric_type\": \"IP\", \"params\": {\"nprobe\": 20}}\n```\n\n**Performance**\n\nThe demo trains the classification model with 80% human sample data (3629 in total) and uses the remaining as test data. It compares performance of the DNA sequence classification model which uses Milvus with the one powered by Mysql and 5 popular machine learning classifiers. The model based on Milvus outperforms its counterparts in accuracy.\n\n```\nfrom sklearn.model_selection import train_test_split\nX, y = human_sequence_kmers, human_labels\nX_train, X_test, y_train, y_test = train_test_split(X, y, test_size=0.2, random_state=42)\n```\n\n\n\n\n# Further Exploration\n\nWith the development of big data technology, vectorization of DNA sequence will play a more important role in genetic research and practice. Combined with professional knowledge in bioinformatics, related studies can further benefit from the involvement of DNA sequence vectorization. Therefore, Milvus can present better results in practice. According to different scenarios and user needs, Milvus-powered similarity search and distance calculation show great potential and many possibilities.\n\n- **Study unknown sequences**: [According to some researchers, vectorization can compress DNA sequence data.](https://iopscience.iop.org/article/10.1088/1742-6596/1453/1/012071/pdf) At the same time, it requires less effort to study structure, function, and evolution of unknown DNA sequences. Milvus can store and retrieve a huge number of DNA sequence vectors without losing accuracy.\n- **Adapt devices**: Limited by traditional algorithms of sequence alignment, similarity search can barely benefit from device ([CPU](https://www.ncbi.nlm.nih.gov/pmc/articles/PMC7884812/)/[GPU](https://mjeer.journals.ekb.eg/article_146090.html)) improvement. Milvus, which supports both regular CPU computation and GPU acceleration, resolves this problem with approximate nearest neighbor algorithm.\n- **Detect virus \u0026 trace origins**: [Scientists have compared genome sequences and reported that COVID19 virus of probable bat origin belongs to SARS-COV](https://www.nature.com/articles/s41586-020-2012-7?fbclid=IwAR2hxnXb9nLWgA8xexEoNrCNH8WHqvHhhbN38aSm48AaH6fTzGMB1BLljf4). Based on this conclusion, researchers can expand sample size for more evidence and patterns.\n- **Diagnose diseases**: Clinically, doctors could compare DNA sequences between patients and healthy group to identify variant genes that cause diseases. It is possible to extract features and encode these data using proper algorithms. Milvus is able to return distances between vectors, which can be related to disease data. In addition to assisting diagnosis of disease, this application can also help to inspire the study of [targeted therapy](https://www.frontiersin.org/articles/10.3389/fgene.2021.680117/full).\n\n\n# Learn more about Milvus\nMilvus is a powerful tool capable of powering a vast array of artificial intelligence and vector similarity search applications. To learn more about the project, check out the following resources:\n- Read our [blog](https://milvus.io/blog).\n- Interact with our open-source community on [Slack](https://milvusio.slack.com/join/shared_invite/zt-e0u4qu3k-bI2GDNys3ZqX1YCJ9OM~GQ#/shared-invite/email).\n- Use or contribute to the world’s most popular vector database on [GitHub](https://github.com/milvus-io/milvus/).\n- Quickly test and deploy AI applications with our new [bootcamp](https://github.com/milvus-io/bootcamp).\n \n","title":"DNA Sequence Classification based on Milvus","metaData":{}},{"id":"2021-08-26-paper-reading-hm-ann-when-anns-meets-heterogeneous-memory.md","author":"Jigao Luo","desc":"HM-ANN Efficient Billion-Point Nearest Neighbor Search on Heterogeneous Memory","canonicalUrl":"https://zilliz.com/blog/paper-reading-hm-ann-when-anns-meets-heterogeneous-memory","date":"2021-08-26T07:18:47.925Z","cover":"https://assets.zilliz.com/blog_cover_4a9807b9e0.png","tags":["Engineering"],"href":"/blog/2021-08-26-paper-reading-hm-ann-when-anns-meets-heterogeneous-memory.md","content":"\n# Paper Reading | HM-ANN: When ANNS Meets Heterogeneous Memory\n\n[HM-ANN: Efficient Billion-Point Nearest Neighbor Search on Heterogenous Memory](https://proceedings.neurips.cc/paper/2020/file/788d986905533aba051261497ecffcbb-Paper.pdf) is a research paper that was accepted at the 2020 Conference on Neural Information Processing Systems ([NeurIPS 2020](https://nips.cc/Conferences/2020)). In this paper, a novel algorithm for graph-based similarity search, called HM-ANN, is proposed. This algorithm considers both memory heterogeneity and data heterogeneity in a modern hardware setting. HM-ANN enables billion-scale similarity search on a single machine without compression technologies. Heterogeneous memory (HM) represents the combination of fast but small dynamic random-access memory (DRAM) and slow but large persistent memory (PMem). HM-ANN achieves low search latency and high search accuracy, especially when the dataset cannot fit into DRAM. The algorithm has a distinct advantage over the state-of-art approximate nearest neighbor (ANN) search solutions.\n\n# Motivation\n\nSince their inception, ANN search algorithms have posed a fundamental tradeoff between query accuracy and query latency due to the limited DRAM capacity. To store indexes in DRAM for fast query access, it is necessary to limit the number of data points or store compressed vectors, both of which hurt search accuracy. Graph-based indexes (e.g. Hierarchical Navigable Small World, HNSW) have superior query runtime performance and query accuracy. However, these indexes can also consume 1-TiB-level DRAM when operating on billion-scale datasets.\n\nThere are other workarounds to avoid letting DRAM store billion-scale datasets in raw format. When a dataset is too large to fit into memory on a single machine, compressed approaches such as product quantization of the dataset’s points are used. But the recall of those indexes with the compressed dataset is normally low because of the loss of precision during quantization. Subramanya et al. [1] explore leveraging solid-state drive (SSD) to achieve billion-scale ANN search using a single machine with an approach called Disk-ANN, where the raw dataset is stored on SSD and the compressed representation on DRAM.\n\n# Introduction to Heterogeneous Memory\n\n\")\n\n\nHeterogeneous memory (HM) represents the combination of fast but small DRAM and slow but large PMem. DRAM is normal hardware that can be found in every modern server, and its access is relatively fast. New PMem technologies, such as Intel® Optane™ DC Persistent Memory Modules, bridge the gap between NAND-based flash (SSD) and DRAM, eliminating the I/O bottleneck. PMem is durable like SSD, and directly addressable by the CPU, like memory. Renen et al. [2] discover that the PMem read bandwidth is 2.6× lower, and the write bandwidth 7.5× lower, than DRAM in the configured experiment environment.\n\n# HM-ANN Design\n\nHM-ANN is an accurate and fast billion-scale ANN search algorithm that runs on a single machine without compression. The design of HM-ANN generalizes the idea of HNSW, whose hierarchical structure naturally fits into HM. HNSW consists of multiple layers—only layer 0 contains the whole dataset, and each remaining layer contains a subset of elements from the layer directly underneath it.\n\n\" )\n\n\n- Elements in the upper layers, which include only subsets of the dataset, consume a small portion of the whole storage. This observation makes them decent candidates to be placed in DRAM. In this way, the majority of searches on HM-ANN are expected to happen in the upper layers, which maximizes the utilization of the fast access characteristic of DRAM. However, in the cases of HNSW, most searches happen in the bottom layer.\n- The bottom-most layer carries the whole dataset, which makes it suitable to be placed in PMem. Since accessing layer 0 is slower, it is preferable to have only a small portion accessed by each query and the access frequency reduced.\n\n## Graph Construction Algorithm\n\n\")\n\n\n\nThe key idea of HM-ANN’s construction is to create high-quality upper layers, in order to provide better navigation for search at layer 0. Thus most memory access happens in DRAM, and access in PMem is reduced. To make this possible, the construction algorithm of HM-ANN has a top-down insertion phase and a bottom-up promotion phase.\n\nThe top-down insertion phase builds a navigable small-world graph as the bottom-most layer is placed on the PMem.\n\nThe bottom-up promotion phase promotes pivot points from the bottom layer to form upper layers that are placed on DRAM without losing much accuracy. If a high-quality projection of elements from layer 0 is created in layer 1, search in layer 0 finds the accurate nearest neighbors of the query with only a few hops.\n\n- Instead of using HNSW’s random selection for promotion, HM-ANN uses a high-degree promotion strategy to promote elements with the highest degree in layer 0 into layer 1. For higher layers, HM-ANN promotes high-degree nodes to the upper layer based on a promotion rate.\n- HM-ANN promotes more nodes from layer 0 to layer 1 and sets a larger maximum number of neighbors for each element in layer 1. The number of nodes in the upper layers is decided by the available DRAM space. Since layer 0 is not stored in DRAM, making each layer stored in DRAM denser increases the search quality.\n\n## Graph Seach Algorithm\n\n\")\n\n\nThe search algorithm consists of two phases: fast memory search and parallel layer-0 search with prefetching.\n\n### Fast memory search\n\nAs the same as in HNSW, the search in DRAM begins at the entry point in the very top layer and then performs 1-greedy search from top to layer 2. To narrow down the search space in layer 0, HM-ANN performs the search in layer 1 with a search budget with `efSearchL1`, which limits the size of the candidate list in layer 1. Those candidates of the list are used as multiple entry points for search in layer 0, to enhance search quality in layer 0. While HNSW using only one entry point, the gap between layer 0 and layer 1 is more specially handled in HM-ANN than gaps between any other two layers.\n\n### Parallel layer-0 search with prefetching\n\nIn the bottom layer, HM-ANN evenly partitions the aforementioned candidates from searching layer 1 and sees them as entry points to perform a parallel multi-start 1-greedy search with threads. The top candidates from each search are collected to find the best candidates. As known, going down from layer 1 to layer 0 is exactly going to PMem. Parallel search hides the latency of PMem and makes the best use of memory bandwidth, to improve search quality without increasing search time.\n\nHM-ANN implements a software-managed buffer in DRAM to prefetch data from PMem before the memory access happens. When searching layer 1, HM-ANN asynchronously copies neighbor elements of those candidates in `efSearchL1` and the neighbor elements’ connections in layer 1 from PMem to the buffer. When the search in layer 0 happens, a portion of to-be-accessed data is already prefetched in DRAM, which hides the latency to access PMem and leads to shorter query time. It matches the design goal of HM-ANN, where most memory accesses happen in DRAM and memory accesses in PMem are reduced.\n\n# Evaluation\n\nIn this paper, an extensive evaluation is conducted. All experiments are done on a machine with Intel Xeon Gold 6252 CPU@2.3GHz. It uses DDR4 (96GB) as fast memory and Optane DC PMM (1.5TB) as slow memory. Five datasets are evaluated: BIGANN, DEEP1B, SIFT1M, DEEP1M, and GIST1M. For billion-scale tests, the following schemes are included: billion-scale quantization-based methods (IMI+OPQ and L\u0026C), the non-compression-based methods (HNSW and NSG).\n\n## Billion-scale algorithm comparison\n\n\n\nIn table 1, the build time and storage of different graph-based indexes are compared. HNSW takes the shortest build time and HM-ANN needs 8% additional time than HNSW. In terms of whole storage usage, HM-ANN indexes are 5–13% larger than HSNW, because it promotes more nodes from layer 0 to layer 1.\n\n\n\nIn Figure 1, the query performance of different indexes is analyzed. Figure 1 (a) and (b) show that HM-ANN achieves the top-1 recall of \u003e 95% within 1ms. Figures 1 (c) and (d) show that HM-ANN obtains top-100 recall of \u003e 90% within 4 ms. HM-ANN provides the best latency-vs-recall performance than all other approaches.\n\n## Million-scale algorithm comparison\n\n\n\nIn Figure 2, the query performance of different indexes is analyzed in a pure DRAM setting. HNSW, NSG, and HM-ANN are evaluated with the three million-scale datasets fitting in DRAM. HM-ANN still achieves better query performance than HNSW. The reason is that the total number of distance computations from HM-ANN is lower (on average 850/query) than that of HNSW (on average 900/query) to achieve 99% recall target.\n\n\n\n## Effectiveness of high-degree promotion\n\nIn Figure 3, the random promotion and high-degree promotion strategies are compared in the same configuration. The high-degree promotion outperforms the baseline. The high-degree promotion performs 1.8x, 4.3x, and 3.9x faster than the random promotion to reach 95%, 99%, and 99.5% recall targets, respectively.\n\n\n\n## Performance benefit of memory management techniques\n\nFigure 5 contains a series of steps between HNSW and HM-ANN to show how each optimization of HM-ANN contributes to its improvements. BP stands for the Bottom-up Promotion while building index. PL0 represents for Parallel layer-0 search, while DP for data prefetching from PMem to DRAM. Step by step, HM-ANN’s search performance is pushed further.\n\n# Conclusion\n\nA new graph-based indexing and search algorithm, called HM-ANN, maps the hierarchical design of the graph-based ANNs with memory heterogeneity in HM. Evaluations show that HM-ANN belongs to the new state-of-the-art indexes in billion point datasets.\n\nWe notice a trend in academia as well as in industry, where building indexes on persistent storage devices is focused on. To offload the pressure of DRAM, Disk-ANN [1] is an index built on SSD, whose throughput is significantly lower than PMem. However, the building of HM-ANN still takes few days, where no huge differences compared with Disk-ANN is established. We believe it is possible to optimize the build time of HM-ANN, when we utilize PMem’s characteristics more carefully, e.g. to be aware of PMem's granularity (256 Bytes) and to use streaming instruction to bypass cachelines. We also believe there would be more approaches with durable storage devices are proposed in the future.\n\n# Reference\n\n[1]: Suhas Jayaram Subramanya and Devvrit and Rohan Kadekodi and Ravishankar Krishaswamy and Ravishankar Krishaswamy: DiskANN: Fast Accurate Billion-point Nearest Neighbor Search on a Single Node, NIPS, 2019\n\n[DiskANN: Fast Accurate Billion-point Nearest Neighbor Search on a Single Node - Microsoft Research](https://www.microsoft.com/en-us/research/publication/diskann-fast-accurate-billion-point-nearest-neighbor-search-on-a-single-node/)\n\n[DiskANN: Fast Accurate Billion-point Nearest Neighbor Search on a Single Node](https://papers.nips.cc/paper/2019/hash/09853c7fb1d3f8ee67a61b6bf4a7f8e6-Abstract.html)\n\n[2]: Alexander van Renen and Lukas Vogel and Viktor Leis and Thomas Neumann and Alfons Kemper: Persistent Memory I/O Primitives, CoRR \u0026 DaMoN, 2019\n\n[https://dl.acm.org/doi/abs/10.1145/3329785.3329930](https://dl.acm.org/doi/abs/10.1145/3329785.3329930)\n\n[Persistent Memory I/O Primitives](https://arxiv.org/abs/1904.01614)\n","title":"Paper Reading|HM-ANN When ANNS Meets Heterogeneous Memory","metaData":{}},{"id":"2021-08-01-milvus2.0-redefining-vector-database.md","author":"Xiaofan Luan","desc":"Milvus 2.0 is available now. This refactored, cloud-native version powers image/video search, chatbots, and many more AI applications.","origin":null,"date":"2021-08-01T00:00:00.000Z","cover":"https://assets.zilliz.com/2_0_cover_bbc582b347.jpg","tags":["Engineering"],"href":"/blog/2021-08-01-milvus2.0-redefining-vector-database.md","content":"\n# Milvus 2.0: Redefining Vector Database\n\nIt was only like yesterday when we put down the first line of code for Milvus in October 2018. In March 2021, after 19 iterations tested by 1,000+ users around the world, we launched Milvus 1.0, our first offical release with long-term support. As the world's most popular open-source vector database, Milvus 1.0 managed to solve some fundamental issues in vector management, such as CRUD operations and data persistence. However, as new scenarios and requirements emerge, we began to realize that there are so many more issues yet to resolve. This article offers a recap on the observations we made in the past three years, the challenges that Milvus 2.0 is expected to address, and why Milvus 2.0 is deemed a better solution to such challenges.\nTo learn more about what Milvus 2.0 has to offer, check out the [Milvus 2.0 Release Notes](https://milvus.io/docs/v2.0.x/release_notes.md).\n\n## Challenges Milvus 1.x is faced with\n\n**Data silo:** Milvus 1.0 is only capable of handling vector embeddings generated from unstructured data, and gives little support for scalar query. The disaggregation of data storage in its design results in duplicate data and adds to the complexity of application development, and hybrid search between vector and scalar data is unsatisfactory due to the lack of a unified optimizer.\n\n**Dilemma between timeliness and efficiency:** Milvus 1.0 is a near real-time system, which relies on regular or force flush to ensure data visibility. This approach adds to the complexity and uncertainty in stream data processing at a number of levels. Besides, although this batch insertion approach is said to improve processing efficiency, it still consumes plenty of resources. So bulkload approach is needed.\n\n**Lacking scalability \u0026 elasticity:** Milvus 1.0 relies on Mishards, a sharding middleware solution, to achieve scability, and network-attached storage (NAS) for data persistence. This classical architecture built upon shared storage does not contribute much to the overall scalability for the following reasons:\n\n1. Only one write node is supported in Mishards and cannot be scaled.\n2. The scaling of the read nodes in Mishards is implemented using consistent hash-based routing. Although consistent hashing is easy to implement and helps solve the issue of data distribution uniformity, it is not flexible enough in data scheduling and falls short of addressing the mismatch between data size and computational power.\n3. Milvus 1.0 relies on MySQL to manage metadata, but the queries and dataset size that a standalone MySQL server is capable of handling is fairly limited.\n\n**Lacking high availability:** One observation we've made is that most of Milvus' users tend to favor availability over consistency, whilst Milvus 1.x lacks capacities such as in-memory replicas and disaster recovery and is not quite up to par in terms of high availability. Therefore, we are exploring the possibility of sacrificing a certain degree of accuracy to achieve higher availability.\n\n**Prohibitively high costs:** Milvus 1.0 relies on NAS for data persistence, the cost of which is usually tenfold that of a local or object storage. Since vector search relies heavily on computing resources and memory, the high costs it incurs could well become a hurdle to further exploration in large-scale datasets or complex business scenarios.\n\n**Unintuitive user experience:**\n\n1. Complicated distributed deployment incurs high operational costs.\n2. A well-designed graphical user interface (GUI) is not available.\n3. Unintuitive APIs have become a drag on the development of applications.\n\nWhether to move on from patch or to start from scratch is a big question. Charles Xie, the father of Milvus, believes that, just as many traditional automakers could never progressively turn Tesla, Milvus has to become a game changer in the field of unstructured data processing and analytics in order to thrive. It is this conviction that spurred us to kick start Milvus 2.0, a refactored cloud-native vector database.\n\n## The Making of Milvus 2.0\n\n### Design principles\n\nAs our next-generation cloud-native vector database, Milvus 2.0 is built around the following three principles:\n\n**Cloud-native first:** We believe that only architectures supporting storage and computing separation can scale on demand and take the full advantage of cloud's elasticity. We'd also like to bring your attention to the microservice design of Milvus 2.0, which features read and write separation, incremental and historical data separation, and CPU-intensive, memory-intensive, and IO-intensive task separation. Microservices help optimize allocation of resources for the ever-changing heterogeneous workload.\n\n**Logs as data:** In Milvus 2.0, the log broker serves as the system' backbone: All data insert and update operations must go through the log broker, and worker nodes execute CRUD operations by subscribing to and consuming logs. This design reduces system complexity by moving core functions such as data persistence and flashback down to the storage layer, and log pub-sub make the system even more flexible and better positioned for future scaling.\n\n**Unified batch and stream processing:** Milvus 2.0 implements the unified Lambda architecture, which integrates the processing of the incremental and historical data. Compared with the Kappa architecture, Milvus 2.0 introduces log backfill, which stores log snapshots and indexes in the object storage to improve failure recovery efficiency and query performance. To break unbounded (stream) data down into bounded windows, Milvus embraces a new watermark mechanism, which slices the stream data into multiple message packs according to write time or event time, and maintains a timeline for users to query by time.\n\n\n\n\n\n### System architecture\n\nAs mentioned above, the design of Milvus 2.0 strictly follows the principles of storage and computing separation and control and data plane separation. The system breaks down into four layers: access layer, coordinator service, worker nodes, and storage.\n\n**Access layer:** The interface: The access layer is the front layer of the system and endpoint to users. It is in charge of forwarding requests and gathering results.\n\n**Coordinator service:** The coordinator service assigns tasks to the worker nodes and functions as the system's brain. There are four coordinator types: root coordinator (root coord), data coordinator (data coord), query coordinator (query coord), and index coordinator (index coord).\n\n**Worker nodes:** The arms and legs. Worker nodes are dumb executors that follow the instructions from the coordinator service and respond to the read/write requests from the access layer. There are three types of worker nodes: data nodes, query nodes, and index nodes.\n\n**Storage:** The bones. Storage has three types: meta storage, log broker, and object storage.\n\n- Implemented by etcd, meta storage is used to store metadata such as collection and checkpoint for the coordinator service.\n- Implemented by Pulsar, log broker is used mainly to store incremental logs and implement reliable asynchronous notifications.\n- Implemented by MinIO or S3, object storage is used mainly to store log snapshots and index files.\n\nThe following is the system architecture diagram of Milvus 2.0:\n\n\n\n\n### Key features\n\nThe costs of running a database involve not only runtime resource consumption, but also the potential learning costs and the operational and maintenance costs. Practically speaking, the more user-friendly a database is, the more likely it is going to save such potential costs. From Milvus' calendar day one, ease of use is always put on the top of our list, and the latest Milvus 2.0 has quite a few to offer in the way of reducing such costs.\n\n#### Always online\n\nData reliability and service sustainability are the basic requirements for a database, and our strategy is \"fail cheap, fail small, and fail often\".\n\n- \"Fail cheap\" refers to storage and computing separation, which makes the handling of node failure recovery straightforward and at a low cost.\n- \"Fail small\" refers to the \"divide and conquer\" strategy, which simplifies the design complexity by having each coordinator service handle only a small portion of read/write/incremental/historical data.\n- \"Fail often\" refers to the introduction of chaos testing, which uses fault injection in a testing environment to simulate situations such as hardware failures and dependency failures and accelerate bug discovery.\n\n#### Hybrid search between scalar and vector data\n\nTo leverage synergy between structured and unstructured data, Milvus 2.0 supports both scalar and vector data and enables hybrid search between them. Hybrid search helps users find the approximate nearest neighbors that match a filter criteria. Currently, Milvus supports relational operations such as EQUAL, GREATER THAN, and LESS THAN, and logical operations such as NOT, AND, OR, and IN.\n\n#### Tunable consistency\n\nAs a distributed database abiding by the PACELC theorem, Milvus 2.0 has to trade off between consistency and availability \u0026 latency. In most scenarios, overemphasizing data consistency in production can overkill because allowing a small portion of data to be invisible has little impact on the overall recall but can significantly improve the query performance. Still, we believe that consistency levels, such as _strong, bounded staleness, and session_, have their own unique application. Therefore, Milvus supports tunable consistency at the request level. Taking testing as an example, users may require _strong_ consistence to ensure test results are absolutely correct.\n\n#### Time travel\n\nData engineers often need to do data rollback to fix dirty data and code bugs. Traditional databases usually implement data rollback through snapshots or even data retrain. This could bring excessive overhead and maintenance costs. Milvus maintains a timeline for all data insert and delete operations, and users can specify a timestamp in a query to retrieve a data view at a specified point in time. With time travel, Milvus can also implement a lightweight data backup or data clone.\n\n#### ORM Python SDK\n\nObject relational mapping (ORM) allows users to focus more on the upper-level business model than on the underlying data model, making it easier for developers to manage relations between collections, fields, and programs. To close the gap between proof of concept (PoC) for AI algorithms and production deployment, we engineered PyMilvus ORM APIs, which can work with an embedded library, a standalone deployment, a distributed cluster , or even a cloud service. With a unified set of APIs, we provide users with a consistent user experience and reduce code migration or adaptation costs.\n\n.\")\n\n\n\n#### Supporting tools\n\n- Milvus Insight is Milvus's graphical user interface offering practical functionalities such as cluster state management, meta management, and data query. The source code of Milvus Insight will also be open sourced as an independent project. We are looking for more contributors to join this effort.\n- Out-of-box experience (OOBE), faster deployment: Milvus 2.0 can be deployed using helm or docker-compose.\n- Milvus 2.0 uses Prometheus, an open-source time-series database, to store performance and monitor data, and Grafana, an open observability platform, for metrics visualization.\n\n## Looking to the future\n\nIn retrospect, we believe the system architecture based on big data + AI application is overly complicated. The top priority of the Milvus community has always been to make Milvus easier to use. Moving forward, the Milvus project will focus on the following areas:\n\n**DB for AI:** Besides the basic CRUD functions, Milvus, as a database system, needs to have a smarter query optimizer, more powerful data query capabilities, and more comprehensive data management functions. Our work for the next stage will be focusing on the data manipulation language (DML) functions and data types not yet available in Milvus 2.0, including adding delete and update operations and supporting string data types.\n\n**AI for DB:** Knob tuning of parameters such as index types, system configurations, user workload, and hardware types complicates the use of Milvus and should be avoided as much as possible. We've set about analyzing system load and gathering access frequency of the data, and plan on introducing auto-tuning in the future to reduce learning costs.\n\n**Cost optimization:** The biggest challenge for vector retrieval is the need to process massive-scale datasets within a limited period of time. This is both CPU-intensive and memory-intensive. Introducing GPU and FPGA heterogeneous hardware acceleration at the physical layer can greatly reduce CPU overhead. We are also developing a hybrid on-disk and in-memory ANN indexing algorithms to realize high-performance queries on massive datasets with limited memory. What's more, we are evaluating the performance of existing open-source vector indexing algorithms such as ScaNN and NGT.\n\n**Ease of use:** Milvus keeps improving its usability by providing cluster management tools, SDKs in multiple languages, deployment tools, operational tools, and more.\n\nTo learn more about Milvus' release plans, check out the [Milvus Roadmap](https://milvus.io/docs/v2.0.x/roadmap.md).\n\nKudos to all Milvus community contributors, without whom Milvus 2.0 would not have been possible. Feel free to [submit an issue](https://github.com/milvus-io/milvus) or [contribute your code](https://github.com/milvus-io/milvus) to the Milvus community!\n\n\u003cbr/\u003e\n\n_About the author_\n\n_Xiaofan Luan is now working at Zilliz as Director of Engineering managing R\u0026D of the Milvus project. He has 7 years' work experience focusing on building database/storage systems. After graduating from Cornell University, he worked at Oracle, HEDVIG, and Alibaba Cloud consecutively._\n","title":"Milvus 2.0 Redefining Vector Database","metaData":{}},{"id":"2021-07-29-building-a-personalized-product-recommender-system-with-vipshop-and-milvus.md","author":"milvus","desc":"Milvus makes it easy to provide the personalized recommendation service to users.","canonicalUrl":"https://zilliz.com/blog/building-a-personalized-product-recommender-system-with-vipshop-and-milvus","date":"2021-07-29T08:46:39.920Z","cover":"https://assets.zilliz.com/blog_shopping_27fba2c990.jpg","tags":["Scenarios"],"href":"/blog/2021-07-29-building-a-personalized-product-recommender-system-with-vipshop-and-milvus.md","content":" \n# Building a Personalized Product Recommender System with Vipshop and Milvus\nWith the explosive growth of Internet data scale, the product quantity as well as category in the current mainstream e-commerce platform increase on the one hand, the difficulty for users to find the products they need surges on the other hand.\n\n[Vipshop](https://www.vip.com/) is a leading online discount retailer for brands in China. The Company offers high-quality and popular branded products to consumers throughout China at a significant discount from retail prices. To optimize the shopping experience for their customers, the company decided to build a personalized search recommendation system based on user query keywords and user portraits.\n\nThe core function of the e-commerce search recommendation system is to retrieve suitable products from a large number of products and display them to users according to their search intent and preference. In this process, the system needs to calculate the similarity between products and users' search intent \u0026 preference, and recommends the TopK products with the highest similarity to users.\n\nData such as product information, user search intent, and user preferences are all unstructured data. We tried to calculate the similarity of such data using CosineSimilarity(7.x) of the search engine Elasticsearch (ES), but this approach has the following drawbacks.\n\n- Long computational response time - the average latency to retrieve TopK results from millions of items is around 300 ms.\n\n- High maintenance cost of ES indexes - the same set of indexes is used for both commodity feature vectors and other related data, which hardly facilitates the index construction, but produces a massive amount of data.\n\nWe tried to develop our own locally sensitive hash plug-in to accelerate the CosineSimilarity calculation of ES. Although the performance and throughput were significantly improved after the acceleration, the latency of 100+ ms was still difficult to meet the actual online product retrieval requirements.\n\nAfter a thorough research, we decided to use Milvus, an open source vector database, which is advantaged with the support for distributed deployment, multi-language SDKs, read/write separation, etc. compared to the commonly used standalone Faiss.\n\nUsing various deep learning models, we convert massive unstructured data into feature vectors, and import the vectors into Milvus. With the excellent performance of Milvus, our e-commerce search recommendation system can efficiently query the TopK vectors that are similar to the target vectors.\n\n## Overall Architecture\n\nAs shown in the diagram, the system overall architecture consists of two main parts.\n\n- Write process: the item feature vectors (hereinafter referred to as item vectors) generated by the deep learning model are normalized and written into MySQL. MySQL then reads the processed item feature vectors using the data synchronization tool (ETL) and import them into the vector database Milvus.\n\n- Read process: The search service obtains user preference feature vectors (hereinafter referred to as user vectors) based on user query keywords and user portraits, queries similar vectors in Milvus and recalls TopK item vectors.\n\nMilvus supports both incremental data update and entire data update. Each incremental update has to delete the existing item vector and insert a new item vector, meaning that every newly updated collection will be re-indexed. It better suits the scenario with more reads and fewer writes. Therefore, we choose the entire data update method. Moreover, it takes only a few minutes to write the entire data in batches of multiple partitions, which is equivalent to near real-time updates.\n\nMilvus write nodes perform all write operations, including creating data collections, building indexes, inserting vectors, etc., and provide services to the public with write domain names. Milvus read nodes perform all read operations and provide services to the public with read-only domain names.\n\nWhereas the current version of Milvus does not support switching collection aliases, we introduce Redis to seamlessly switch aliases between multiple entire data collections.\n\nThe read node only needs to read existing metadata information and vector data or indexes from MySQL, Milvus, and GlusterFS distributed file system, so the read capability can be horizontally extended by deploying multiple instances.\n\n## Implementation Details\n\n### Data Update\n\nThe data update service includes not only writing vector data, but also data volume detection of vectors, index construction, index pre-loading, alias control, etc. The overall process is as follows.\n\n1. Assume that before building the entire data, CollectionA provides data service to the public, and the entire data being used is directed to CollectionA (`redis key1 = CollectionA`). The purpose of constructing entire data is to create a new collection CollectionB.\n\n2. Commodity data check - check the item number of commodity data in the MySQL table, compare the commodity data with the existing data in CollectionA. Alert can be set in accordance with quantity or percentage. If the set quantity (percentage) is not reached, the entire data will not be built, and it will be regarded as the failure of this building operation, triggering the alert; once the set quantity (percentage) is reached, the entire data building process starts.\n\n3. Start building the entire data - initialize the alias of the entire data being built, and update Redis. After updating, the alias of the entire data being built is directed to CollectionB (`redis key2 = CollectionB`).\n\n4. Create a new entire collection - determine if CollectionB exists. If it does, delete it before creating a new one.\n\n5. Data batch write-in - calculate the partition ID of each commodity data with its own ID using modulo operation, and write the data to multiple partitions to the newly created collection in batches.\n\n6. Build and pre-load index - Create index (`createIndex()`) for the new collection. The index file is stored in distributed storage server GlusterFS. The system automatically simulates query on the new collection and pre-load the index for query warm-up. \n\n7. Collection data check - check the item number of data in the new collection, compare the data with the existing collection, and set alarms based on the quantity and percentage. If the set number (percentage) is not reached, the collection will not be switched and the building process will be regarded as a failure, triggering the alert.\n\n8. Switching collection - Alias control. After updating Redis, the entire data alias being used is directed to CollectionB (`redis key1 = CollectionB`), the original Redis key2 is deleted, and the building process is completed.\n\n### Data Recall\n\nThe Milvus partition data is called several times to calculate the similarity between user vectors, obtained based on user query keywords and user portrait, and item vector, and the TopK item vectors are returned after merging. The overall workflow schematic is as follow:\n\nThe following table lists the main services involved in this process. It can be seen that the average latency for recalling TopK vectors is about 30 ms.\n\n| **Service** | **Role** | **Input Parameters** | **Output parameters** | **Response latency** |\n| ------------------------ | ------------------------------------------------------- | ------------------------------------------------------------ | --------------------- | -------------------- |\n| User vectors acquisition | Obtain user vector | user info + query | user vector | 10 ms |\n| Milvus Search | Calculate the vector similarity and return TopK results | user vector | item vector | 10 ms |\n| Scheduling Logic | Concurrent result recalling and merging | Multi-channel recalled item vectors and the similarity score | TopK items | 10 ms |\n\n**Implementation process:**\n\n1. Based on the user query keywords and user portrait, the user vector is calculated by the deep learning model.\n2. Obtain the collection alias of the entire data being used from Redis currentInUseKeyRef and get Milvus CollectionName. This process is data synchronization service, i.e. switching alias to Redis after entire data update.\n3. Milvus is called concurrently and asynchronously with the user vector to obtain data from different partitions of the same collection, and Milvus calculates the similarity between the user vector and the item vector, and returns the TopK similar item vectors in each partition.\n4. Merge the TopK item vectors returned from each partition, and rank the results in the reverse order of similarity distance, which are calculated using the IP inner product (the greater the distance between the vectors, the more similar they are). The final TopK item vectors are returned.\n\n## Looking Ahead\n\nAt present, Milvus-based vector search can be used steadily in the search of recommendation scenarios, and its high performance gives us more room to play in the dimensionality of the model and algorithm selection.\n\nMilvus will play a crucial role as the middleware for more scenarios, including recall of main site search and all-scenario recommendations.\n\nThe three most anticipated features of Milvus in the future are as follows.\n\n- Logic for collection alias switching - coordinate the switching across collections without external conponents.\n- Filtering mechanism - Milvus v0.11.0 only supports ES DSL filtering mechanism in standalone version. The newly released Milvus 2.0 supports scalar filtering, and read/write separation.\n- Storage support for Hadoop Distributed File System (HDFS) - The Milvus v0.10.6 we are using only supports POSIX file interface, and we have deployed GlusterFS with FUSE support as the storage backend. However, HDFS is a better choice in terms of performance and ease of scaling.\n\n## Lessons Learned and Best Practices\n\n1. For applications where read operations are the primary focus, a read-write separation deployment can significantly increase the processing power and improve performance.\n2. The Milvus Java client lacks a reconnection mechanism because the Milvus client used by the recall service is resident in memory. We have to build our own connection pool to ensure the availability of the connection between the Java client and the server through heartbeat test.\n3. Slow queries occur occasionally on Milvus. This is due to insufficient warm-up of the new collection. By simulating the query on the new collection, the index file is loaded into the memory to achieve the index warm-up.\n4. nlist is the index building parameter and nprobe is the query parameter. You need to get a reasonable threshold value according to your business scenario through pressure testing experiments to balance the retrieval performance and accuracy.\n5. For static data scenario, it is more efficient to import all data into the collection first and build indexes later. \n ","title":"Building a Personalized Product Recommender System with Vipshop and Milvus","metaData":{}},{"id":"2021-07-27-audio-retrieval-based-on-milvus.md","author":"Shiyu Chen","desc":"Audio retrieval with Milvus makes it possible to classify and analyze sound data in real time.","canonicalUrl":"https://zilliz.com/blog/audio-retrieval-based-on-milvus","date":"2021-07-27T03:05:57.524Z","cover":"https://assets.zilliz.com/blog_audio_search_56b990cee5.jpg","tags":["Scenarios"],"href":"/blog/2021-07-27-audio-retrieval-based-on-milvus.md","content":" \n# Audio Retrieval Based on Milvus\nSound is an information dense data type. Although it may feel antiquated in the era of video content, audio remains a primary information source for many people. Despite long-term decline in listeners, 83% of Americans ages 12 or older listened to terrestrial (AM/FM) radio in a given week in 2020 (down from 89% in 2019). Conversely, online audio has seen a steady rise in listeners over the past two decades, with 62% of Americans reportedly listening to some form of it on a weekly basis according to the same [Pew Research Center study](https://www.journalism.org/fact-sheet/audio-and-podcasting/).\n\nAs a wave, sound includes four properties: frequency, amplitude, wave form, and duration. In musical terminology, these are called pitch, dynamic, tone, and duration. Sounds also help humans and other animals perceive and understand our environment, providing context clues for the location and movement of objects in our surroundings.\n\nAs an information carrier, audio can be classified into three categories:\n\n1. **Speech:** A communication medium composed of words and grammar. With speech recognition algorithms, speech can be converted to text.\n2. **Music:** Vocal and/or instrumental sounds combined to produce a composition comprised of melody, harmony, rhythm, and timbre. Music can be represented by a score.\n3. **Waveform:** A digital audio signal obtained by digitizing analog sounds. Waveforms can represent speech, music, and natural or synthesized sounds.\n\nAudio retrieval can be used to search and monitor online media in real-time to crack down on infringement of intellectual property rights. It also assumes an important role in the classification and statistical analysis of audio data.\n\n## Processing Technologies\n\nSpeech, music, and other generic sounds each have unique characteristics and demand different processing methods. Typically, audio is separated into groups that contain speech and groups that do not:\n\n- Speech audio is processed by automatic speech recognition.\n- Non-speech audio, including musical audio, sound effects, and digitized speech signals, are processed using audio retrieval systems. \n\nThis article focuses on how to use an audio retrieval system to process non-speech audio data. Speech recognition is not covered in this article\n\n### Audio feature extraction\n\nFeature extraction is the most important technology in audio retrieval systems as it enables audio similarity search. Methods for extracting audio features are divided into two categories: \n\n- Traditional audio feature extraction models such as Gaussian mixture models (GMMs) and hidden Markov models (HMMs);\n- Deep learning-based audio feature extraction models such as recurrent neural networks (RNNs), long short-term memory (LSTM) networks, encoding-decoding frameworks, attention mechanisms, etc.\n\nDeep learning-based models have an error rate that is an order of magnitude lower than traditional models, and therefore are gaining momentum as core technology in the field of audio signal processing.\n\nAudio data is usually represented by the extracted audio features. The retrieval process searches and compares these features and attributes rather than the audio data itself. Therefore, the effectiveness of audio similarity retrieval largely depends on the feature extraction quality.\n\nIn this article, [large-scale pre-trained audio neural networks for audio pattern recognition (PANNs)](https://github.com/qiuqiangkong/audioset_tagging_cnn) are used to extract feature vectors for its mean average accuracy (mAP) of 0.439 (Hershey et al., 2017).\n\nAfter extracting the feature vectors of the audio data, we can implement high-performance feature vector analysis using Milvus.\n\n### Vector similarity search\n\n[Milvus](https://milvus.io/) is a cloud-native, open-source vector database built to manage embedding vectors generated by machine learning models and neural networks. It is widely used in scenarios such as computer vision, natural language processing, computational chemistry, personalized recommender systems, and more.\n\nThe following diagram depicts the general similarity search process using Milvus: \n \n\n\n1. Unstructured data are converted to feature vectors by deep learning models and inserted into Milvus.\n2. Milvus stores and indexes these feature vectors.\n3. Upon request, Milvus searches and returns vectors most similar to the query vector.\n\n## System overview\n\nThe audio retrieval system mainly consists of two parts: insert (black line) and search(red line).\n\n\n\n\nThe sample dataset used in this project contains open-source game sounds, and the code is detailed in the [Milvus bootcamp](https://github.com/milvus-io/bootcamp/tree/master/solutions/audio_similarity_search).\n\n### Step 1: Insert data\n\nBelow is the example code for generating audio embeddings with the pre-trained PANNs-inference model and inserting them into Milvus, which assigns a unique ID to each vector embedding.\n\n```\n1 wav_name, vectors_audio = get_audio_embedding(audio_path) \n2 if vectors_audio: \n3 embeddings.append(vectors_audio) \n4 wav_names.append(wav_name) \n5 ids_milvus = insert_vectors(milvus_client, table_name, embeddings) \n6 \n```\n\nThen the returned **ids_milvus** are stored along with other relevant information (e.g. **wav_name**) for the audio data held in a MySQL database for subsequent processing. \n\n```\n1 get_ids_correlation(ids_milvus, wav_name) \n2 load_data_to_mysql(conn, cursor, table_name) \n3 \n```\n\n### Step 2: Audio search\n\nMilvus calculates the inner product distance between the pre-stored feature vectors and the input feature vectors, extracted from the query audio data using the PANNs-inference model, and returns the **ids_milvus** of similar feature vectors, which correspond to the audio data searched.\n\n```\n1 _, vectors_audio = get_audio_embedding(audio_filename) \n2 results = search_vectors(milvus_client, table_name, [vectors_audio], METRIC_TYPE, TOP_K) \n3 ids_milvus = [x.id for x in results[0]] \n4 audio_name = search_by_milvus_ids(conn, cursor, ids_milvus, table_name) \n5\n````\n\n## API reference and demo\n\n### API\n\nThis audio retrieval system is built with open-source code. Its main features are audio data insertion and deletion. All APIs can be viewed by typing **127.0.0.1:\u003cport\u003e/docs** in the browser. \n\n\n\n\n\n### Demo\n\nWe host a [live demo](https://zilliz.com/solutions ) of the Milvus-based audio retrieval system online that you can try out with your own audio data. \n\n\n\n## Conclusion\n\nLiving in the era of big data, people find their lives abound with all sorts of information. To make better sense of it, traditional text retrieval no long cuts it. Today's information retrieval technology is in urgent need of the retrieval of various unstructured data types, such as videos, images, and audio.\n\nUnstructured data, which is difficult for computers to process, can be converted into feature vectors using deep learning models. This converted data can easily be processed by machines, enabling us to analyze unstructured data in ways our predecessors were never able to. Milvus, an open-source vector database, can efficiently process the feature vectors extracted by AI models and provides a variety of common vector similarity calculations.\n\n## References\nHershey, S., Chaudhuri, S., Ellis, D.P., Gemmeke, J.F., Jansen, A., Moore, R.C., Plakal, M., Platt, D., Saurous, R.A., Seybold, B. and Slaney, M., 2017, March. CNN architectures for large-scale audio classification. In 2017 IEEE International Conference on Acoustics, Speech and Signal Processing (ICASSP), pp. 131-135, 2017 \n\n## Don't be a stranger\n\n- Find or contribute to Milvus on [GitHub](https://github.com/milvus-io/milvus/).\n\n- Interact with the community via [Slack](https://join.slack.com/t/milvusio/shared_invite/zt-e0u4qu3k-bI2GDNys3ZqX1YCJ9OM~GQ).\n\n- Connect with us on [Twitter](https://twitter.com/milvusio).\n ","title":"Audio Retrieval Based on Milvus","metaData":{}},{"id":"2021-07-20-milmil-a-milvus-powered-faq-chatbot-that-answers-questions-about-milvus.md","author":"milvus","desc":"Using open-source vector search tools to build a question answering service.","canonicalUrl":"https://zilliz.com/blog/milmil-a-milvus-powered-faq-chatbot-that-answers-questions-about-milvus","date":"2021-07-20T07:21:43.897Z","cover":"https://assets.zilliz.com/milmil_4600f33f1c.jpg","tags":["Scenarios"],"href":"/blog/2021-07-20-milmil-a-milvus-powered-faq-chatbot-that-answers-questions-about-milvus.md","content":"\n# MilMil: A Milvus-powered FAQ Chatbot that Answers Questions About Milvus\n\nopen-source community recently created MilMil—a Milvus FAQ chatbot built by and for Milvus users. MilMil is available 24/7 at [Milvus.io](https://milvus.io/) to answer common questions about Milvus, the world's most advanced open-source vector database.\n\nThis question answering system not only helps solve common problems Milvus users encounter more quickly, but identifies new problems based on user submissions. MilMil's database includes questions users have asked since the project was first released under an open-source license in 2019. Questions are stored in two collections, one for Milvus 1.x and earlier and another for Milvus 2.0.\n\nMilMil is currently only available in English.\n\n## How does MilMil work?\n\nMilMil relies on the _sentence-transformers/paraphrase-mpnet-base-v2_ model to obtain vector representations of the FAQ database, then Milvus is used for vector similarity retrieval to return semantically similar questions.\n\nFirst, the FAQ data is converted into semantic vectors using BERT, a natural language processing (NLP) model. The embeddings are then inserted into Milvus and each one assigned a unique ID. Finally, the questions and answers are inserted into PostgreSQL, a relational database, together with their vector IDs.\n\nWhen users submit a question, the system converts it into a feature vector using BERT. Next it searches Milvus for five vectors that are most similar to the query vector and retrieves their IDs. Finally, the questions and answers that correspond with the retrieved vector IDs are returned to the user.\n\n\n\n\nSee the [question answering system](https://github.com/milvus-io/bootcamp/tree/master/solutions/question_answering_system) project in the Milvus bootcamp to explore the code used to build AI chatbots.\n\n## Ask MilMil about Milvus\n\nTo chat with MilMil, navigate to any page on [Milvus.io](https://milvus.io/) and click the bird icon in the lower-right corner. Type your question into the text input box and hit send. MilMil will get back to you in milliseconds! Additionally, the dropdown list in the upper-left corner can be used to switch between technical documentation for different versions of Milvus.\n\n\n\n\nAfter submitting a question, the bot immediately returns three questions that are semantically similar to the query question. You can click \"See answer\" to browse potential answers to your question, or click \"See more\" to view more questions related to your search. If a suitable answer is unavailable, click \"Put in your feedback here\" to ask your question along with an email address. Help from the Milvus community will arrive shortly!\n\n\n\n\nGive MilMil a try and let us know what you think. All questions, comments, or any form of feedback are welcome.\n\n## Don't be a stranger\n\n- Find or contribute to Milvus on [GitHub](https://github.com/milvus-io/milvus/).\n- Interact with the community via [Slack](https://join.slack.com/t/milvusio/shared_invite/zt-e0u4qu3k-bI2GDNys3ZqX1YCJ9OM~GQ).\n- Connect with us on [Twitter](https://twitter.com/milvusio).\n","title":"MilMil A Milvus-powered FAQ Chatbot that Answers Questions About Milvus","metaData":{}},{"id":"2021-07-15-test-and-deploy-vector-search-solutions-milvus-bootcamp.md","author":"milvus","desc":"Build, test, and customize vector similarity search solutions with Milvus, an open-source vector database.","canonicalUrl":"https://zilliz.com/blog/test-and-deploy-vector-search-solutions-milvus-bootcamp","date":"2021-07-15T03:05:45.742Z","cover":"https://assets.zilliz.com/cover_80db9ee49c.png","tags":["Engineering"],"href":"/blog/2021-07-15-test-and-deploy-vector-search-solutions-milvus-bootcamp.md","content":"\n# Quickly Test and Deploy Vector Search Solutions with the Milvus 2.0 Bootcamp\n\nWith the release of Milvus 2.0, the team has revamped the Milvus [bootcamp](https://github.com/milvus-io/bootcamp). The new and improved bootcamp offers updated guides and easier to follow code examples for a variety of use cases and deployments. Additionally, this new version is updated for [Milvus 2.0](https://milvus.io/blog/milvus2.0-redefining-vector-database.md), a reimagined version of the world's most advanced vector databse.\n\n### Stress test your system against 1M and 100M dataset benchmarks\n\nThe [benchmark directory](https://github.com/milvus-io/bootcamp/tree/master/benchmark_test) contains 1 million and 100 million vector benchmark tests that indicate how your system will react to differently sized datasets.\n\n\u003cbr/\u003e\n\n### Explore and build popular vector similarity search solutions\n\nThe [solution directory](https://github.com/milvus-io/bootcamp/tree/master/solutions) includes the most popular vector similarity search use cases. Each use case contains a notebook solution and a docker deployable solution. Use cases include:\n\n- [Image similarity search](https://github.com/milvus-io/bootcamp/tree/master/solutions/reverse_image_search)\n- [Video similarity search](https://github.com/milvus-io/bootcamp/tree/master/solutions/video_similarity_search)\n- [Audio similarity search](https://github.com/milvus-io/bootcamp/tree/master/solutions/audio_similarity_search)\n- [Recommendation system](https://github.com/milvus-io/bootcamp/tree/master/solutions/recommendation_system)\n- [Molecular search](https://github.com/milvus-io/bootcamp/tree/master/solutions/molecular_similarity_search)\n- [Question answering system](https://github.com/milvus-io/bootcamp/tree/master/solutions/question_answering_system)\n\n\u003cbr/\u003e\n\n### Quickly deploy a fully built application on any system\n\nThe quick deploy solutions are dockerized solutions that allow users to deploy fully built applications on any system. These solutions are ideal for brief demos, but require additional work to customize and understand compared to notebooks.\n\n\u003cbr/\u003e\n\n### Use scenario specific notebooks to easily deploy pre-configured applications\n\nThe notebooks contain a simple example of deploying Milvus to solve the problem in a given use case. Each of the examples are able to be run from start to finish without the need to manage files or configurations. Each notebook is also easy to follow and modifiable, making them ideal base files for other projects.\n\n\u003cbr/\u003e\n\n### Image similarity search notebook example\n\nImage similarity search is one of the core ideas behind many different technologies, including autonomous cars recognizing objects. This example explains how to easily build computer vision programs with Milvus.\n\nThis notebookrevolves around three things:\n\n- Milvus server\n- Redis server (for metadata storage)\n- Pretrained Resnet-18 model.\n\n#### Step 1: Download required packages\n\nBegin by downloading all the required packages for this project. This notebook includes a table listing the packages to use.\n\n```\npip install -r requirements.txt\n```\n\n#### Step 2: Server startup\n\nAfter the packages are installed, start the servers and ensure both are running properly. Be sure to follow the correct instructions for starting the [Milvus](https://milvus.io/docs/v2.0.x/install_standalone-docker.md) and [Redis](https://hub.docker.com/_/redis) servers.\n\n#### Step 3: Download project data\n\nBy default, this notebook pulls a snippet of the VOCImage data for use as an example, but any directory with images should work as long as it follows the file structure that can be seen at the top of the notebook.\n\n```\n! gdown \"https://drive.google.com/u/1/uc?id=1jdudBiUu41kL-U5lhH3ari_WBRXyedWo\u0026export=download\"\n! tar -xf 'VOCdevkit.zip'\n! rm 'VOCdevkit.zip'\n```\n\n#### Step 4: Connect to the servers\n\nIn this example, the servers are running on the default ports on the localhost.\n\n```\nconnections.connect(host=\"127.0.0.1\", port=19537)\nred = redis.Redis(host = '127.0.0.1', port=6379, db=0)\n```\n\n#### Step 5: Create a collection\n\nAfter starting the servers, create a collection in Milvus for storing all the vectors. In this example, the dimension size is set to 512, the size of the resnet-18 output, and the similarity metric is set to the Euclidean distance (L2). Milvus supports a variety of different [similarity metrics](https://milvus.io/docs/v2.0.x/metric.md).\n\n```\ncollection_name = \"image_similarity_search\"\ndim = 512\ndefault_fields = [\n schema.FieldSchema(name=\"id\", dtype=DataType.INT64, is_primary=True, auto_id=True),\n schema.FieldSchema(name=\"vector\", dtype=DataType.FLOAT_VECTOR, dim=dim)\n]\ndefault_schema = schema.CollectionSchema(fields=default_fields, description=\"Image test collection\")\ncollection = Collection(name=collection_name, schema=default_schema)\n```\n\n#### Step 6: Build an index for the collection\n\nOnce the collection is made, build an index for it. In this case, the IVF_SQ8 index is used. This index requires the 'nlist' parameter, which tells Milvus how many clusters to make within each datafile (segment). Different [indices](https://milvus.io/docs/v2.0.x/index.md) require different parameters.\n\n```\ndefault_index = {\"index_type\": \"IVF_SQ8\", \"params\": {\"nlist\": 2048}, \"metric_type\": \"L2\"}\ncollection.create_index(field_name=\"vector\", index_params=default_index)\ncollection.load()\n```\n\n#### Step 7: Set up model and data loader\n\nAfter the IVF_SQ8 index is built, set up the neural network and data loader. The pretrained pytorch resnet-18 used in this example is sans its last layer, which compresses vectors for classification and may lose valuable information.\n\n```\nmodel = torch.hub.load('pytorch/vision:v0.9.0', 'resnet18', pretrained=True)\nencoder = torch.nn.Sequential(*(list(model.children())[:-1]))\n```\n\nThe dataset and data loader needs to be modified so that they are able to preprocess and batch the images while also providing the file paths of the images. This can be done with a slightly modified torchvision dataloader. For preprocessing, the images need to be cropped and normalized due to the resnet-18 model being trained on a specific size and value range.\n\n```\ndataset = ImageFolderWithPaths(data_dir, transform=transforms.Compose([\n transforms.Resize(256),\n transforms.CenterCrop(224),\n transforms.ToTensor(),\n transforms.Normalize(mean=[0.485, 0.456, 0.406], std=[0.229, 0.224, 0.225])]))\n\ndataloader = torch.utils.data.DataLoader(dataset, num_workers=0, batch_si\n```\n\n#### Step 8: Insert vectors into the collection\n\nWith the collection setup, the images can be processed and loaded into the created collection. First the images are pulled by the dataloader and run through the resnet-18 model. The resulting vector embeddings are then inserted into Milvus, which returns a unique ID for each vector. The vector IDs and image file paths are then inserted as key-value pairs into the Redis server.\n\n```\nsteps = len(dataloader)\nstep = 0\nfor inputs, labels, paths in dataloader:\n with torch.no_grad():\n output = encoder(inputs).squeeze()\n output = output.numpy()\n\n mr = collection.insert([output.tolist()])\n ids = mr.primary_keys\n for x in range(len(ids)):\n red.set(str(ids[x]), paths[x])\n if step%5 == 0:\n print(\"Insert Step: \" + str(step) + \"/\" + str(steps))\n step += 1\n```\n\n#### Step 9: Conduct a vector similarity search\n\nOnce all of the data is inserted into Milvus and Redis, the actual vector similarity search can be performed. For this example, three randomly selected images are pulled out of the Redis server for a vector similarity search.\n\n```\nrandom_ids = [int(red.randomkey()) for x in range(3)]\nsearch_images = [x.decode(\"utf-8\") for x in red.mget(random_ids)]\n```\n\nThese images first go through the same preprocessing that is found in Step 7 and are then pushed through the resnet-18 model.\n\n```\ntransform_ops = transforms.Compose([\n transforms.Resize(256),\n transforms.CenterCrop(224),\n transforms.ToTensor(),\n transforms.Normalize(mean=[0.485, 0.456, 0.406], std=[0.229, 0.224, 0.225])])\n\nembeddings = [transform_ops(Image.open(x)) for x in search_images]\nembeddings = torch.stack(embeddings, dim=0)\n\nwith torch.no_grad():\n embeddings = encoder(embeddings).squeeze().numpy()\n```\n\nThen the resulting vector embeddings are used to perform a search. First, set the search parameters, including the name of the collection to search, nprobe (the number of the clusters to search), and top_k (the number of returned vectors). In this example, the search should be very quick.\n\n```\nsearch_params = {\"metric_type\": \"L2\", \"params\": {\"nprobe\": 32}}\nstart = time.time()\nresults = collection.search(embeddings, \"vector\", param=search_params, limit=3, expr=None)\nend = time.time() - start\n```\n\n#### Step 10: Image search results\n\nThe vector IDs returned from the queries are used to find the corresponding images. Matplotlib is then used to display the image search results.\n\u003cbr/\u003e\n\n\n\n\n\n\u003cbr/\u003e\n\n### Learn how to deploy Milvus in different enviroments\n\nThe [deployments section](https://github.com/milvus-io/bootcamp/tree/master/deployments) of the new bootcamp contains all the information for using Milvus in different environments and setups. It includes deploying Mishards, using Kubernetes with Milvus, load balancing, and more. Each environment has a detailed step by step guide explaining how to get Milvus working in it.\n\n\u003cbr/\u003e\n\n### Don't be a stranger\n\n- Read our our [blog](https://zilliz.com/blog).\n- Interact with our open-source community on [Slack](https://join.slack.com/t/milvusio/shared_invite/zt-e0u4qu3k-bI2GDNys3ZqX1YCJ9OM~GQ).\n- Use or contribute to Milvus, the world’s most popular vector database, on [Github](https://github.com/milvus-io/milvus).\n","title":"Quickly Test and Deploy Vector Search Solutions with the Milvus 2.0 Bootcamp","metaData":{}},{"id":"2021-07-09-building-a-wardrobe-and-outfit-planning-app-with-milvus.md","author":"Yu Fang","desc":"Discover how Milvus, an open-source vector database, is used by Mozat to power a fashion app that offers personalized style recommendations and an image search system.","canonicalUrl":"https://zilliz.com/blog/building-a-wardrobe-and-outfit-planning-app-with-milvus","date":"2021-07-09T06:30:06.439Z","cover":"https://assets.zilliz.com/mozat_blog_0ea9218c71.jpg","tags":["Scenarios"],"href":"/blog/2021-07-09-building-a-wardrobe-and-outfit-planning-app-with-milvus.md","content":" \n# Building a Wardrobe and Outfit Planning App with Milvus\n\n\nFounded in 2003, [Mozat](http://www.mozat.com/home) is a start-up headquartered in Singapore with offices in China and Saudi Arabia. The company specializes in building social media, communication, and lifestyle applications. [Stylepedia](https://stylepedia.com/) is a wardrobe app built by Mozat that helps users discover new styles and connect with other people that are passionate about fashion. Its key features include the ability to curate a digital closet, personalized style recommendations, social media functionality, and an image search tool for finding similar items to something seen online or in real life.\n\n[Milvus](https://milvus.io) is used to power the image search system within Stylepedia. The app deals with three image types: user images, product images, and fashion photographs. Each image can include one or more items, further complicating each query. To be useful, an image search system must be accurate, fast, and stable, features that lay a solid technical foundation for adding new functionality to the app such as outfit suggestions and fashion content recommendations.\n\n## System overview\n\n\n\nThe image search system is divided into offline and online components. \n\nOffline, images are vectorized and inserted into a vector database (Milvus). In the data workflow, relevant product images and fashion photographs are converted into 512-dimensional feature vectors using object detection and feature extraction models. The vector data is then indexed and added to the vector database.\n\nOnline, the image database is queried and similar images are returned to the user. Similar to the off-line component, a query image is processed by object detection and feature extraction models to obtain a feature vector. Using the feature vector, Milvus searches for TopK similar vectors and obtains their corresponding image IDs. Finally, after post-processing (filtering, sorting, etc.), a collection of images similar to the query image are returned.\n\n## Implementation\n\nThe implementation breaks down into four modules:\n1. Garment detection\n2. Feature extraction\n3. Vector similarity search\n4. Post-processing\n\n### Garment detection\n\nIn the garment detection module, [YOLOv5](https://pytorch.org/hub/ultralytics_yolov5/), a one-stage, anchor-based target detection framework, is used as the object detection model for its small size and real-time inference. It offers four model sizes (YOLOv5s/m/l/x), and each specific size has pros and cons. The larger models will perform better (higher precision) but require a lot more computing power and run slower. Because the objects in this case are relatively large items and easy to detect, the smallest model, YOLOv5s, suffices.\n\nClothing items in each image are recognized and cropped out to serve as the feature extraction model inputs used in subsequent processing. Simultaneously, the object detection model also predicts the garment classification according to predefined classes (tops, outerwear, trousers, skirts, dresses, and rompers).\n\n### Feature extraction\n\nThe key to similarity search is the feature extraction model. Cropped clothes images are embedded into 512-dimensional floating point vectors that represent their attributes in a machine readable numeric data format. The [deep metric learning (DML)](https://github.com/Joon-Park92/Survey_of_Deep_Metric_Learning) methodology is adopted with [EfficientNet](https://arxiv.org/abs/1905.11946) as the backbone model.\n\nMetric learning aims to train a CNN-based nonlinear feature extraction module (or an encoder) to reduce the distance between the feature vectors corresponding to the same class of samples, and increase the distance between the feature vectors corresponding to different classes of samples. In this scenario, the same class of samples refers to the same piece of clothing.\n\nEfficientNet takes into account both speed and precision when uniformly scaling network width, depth, and resolution. EfficientNet-B4 is used as the feature extraction network, and the output of the ultimate fully connected layer is the image features needed to conduct vector similarity search.\n\n### Vector similarity search\n\nMilvus is an open-source vector database that supports create, read, update, and delete (CRUD) operations as well as near real-time search on trillion-byte datasets. In Stylepedia, it is used for large-scale vector similarity search because it is highly elastic, stable, reliable, and lightening fast. Milvus extends the capabilities of widely used vector index libraries (Faiss, NMSLIB, Annoy, etc.), and provides a set of simple and intuitive APIs that allow users to select the ideal index type for a given scenario.\n\nGiven the scenario requirements and data scale, Stylepedia's developers used the CPU-only distribution of Milvus paired with the HNSW index. Two indexed collections, one for products and the other for fashion photographs, are built to power different application functionalities. Each collection is further divided into six partitions based on the detection and classification results to narrow the search scope. Milvus performs search on tens of millions of vectors in milliseconds, providing optimal performance while keeping development costs low and minimizing resource consumption.\n\n### Post-processing\n\nTo improve the similarity between the image retrieval results and the query image, we use color filtering and key label (sleeve length, clothes length, collar style, etc.) filtering to filter out ineligible images. In addition, an image quality assessment algorithm is used to make sure that higher quality images are presented to users first.\n\n## Application\n\n### User uploads and image search\n\nUsers can take pictures of their own clothes and upload them to their Stylepedia digital closet, then retrieve product images most similar to their uploads.\n\n\n\n### Outfit suggestions\n\nBy conducting similarity search on the Stylepedia database, users can find fashion photographs that contain a specific fashion item. These could be new garments someone is thinking about purchasing, or something from their own collection that could be worn or paired differently. Then, through the clustering of the items it is often paired with, outfit suggestions are generated. For example, a black biker jacket can go with a variety of items, such as a pair of black skinny jeans. Users can then browse relevant fashion photographs where this match occurs in the selected formula.\n\n\n\n\n\n### Fashion photograph recommendations\n\nBased on a user's browsing history, likes, and the contents of their digital closet, the system calculates similarity and provides customized fashion photograph recommendations that may be of interest.\n\n\n\n\n\n\nBy combining deep learning and computer vision methodologies, Mozat was able to build a fast, stable, and accurate image similarity search system using Milvus to power various features in the Stylepedia app.\n\n## Don't be a stranger\n\n- Find or contribute to Milvus on [GitHub](https://github.com/milvus-io/milvus/).\n- Interact with the community via [Slack](https://join.slack.com/t/milvusio/shared_invite/zt-e0u4qu3k-bI2GDNys3ZqX1YCJ9OM~GQ).\n- Connect with us on [Twitter](https://twitter.com/milvusio).\n\n \n","title":"Building a Wardrobe and Outfit Planning App with Milvus","metaData":{}},{"id":"2021-06-15-building-a-milvus-cluster-based-on-juicefs.md","author":"Changjian Gao and Jingjing Jia","desc":"Learn how to build a Milvus cluster based on JuiceFS, a shared file system designed for cloud-native environments.","canonicalUrl":"https://zilliz.com/blog/building-a-milvus-cluster-based-on-juicefs","date":"2021-06-15T07:21:07.938Z","cover":"https://assets.zilliz.com/Juice_FS_blog_cover_851cc9e726.jpg","tags":["Engineering"],"href":"/blog/2021-06-15-building-a-milvus-cluster-based-on-juicefs.md","content":"\n# Building a Milvus Cluster Based on JuiceFS\n\nCollaborations between open-source communities are a magical thing. Not only do passionate, intelligent, and creative volunteers keep open-source solutions innovative, they also work to bring different tools together in interesting and useful ways. [Milvus](https://milvus.io/), the world's most popular vector database, and [JuiceFS](https://github.com/juicedata/juicefs), a shared file system designed for cloud-native environments, were united in this spirit by their respective open-source communities. This article explains what JuiceFS is, how to build a Milvus cluster based on JuiceFS shared file storage, and the performance users can expect using this solution.\n\n## **What is JuiceFS?**\n\nJuiceFS is a high-performance, open-source distributed POSIX file system, which can be built on top of Redis and S3. It was designed for cloud-native environments and supports managing, analyzing, archiving, and backing up data of any type. JuiceFS is commonly used for solving big data challenges, building artificial intelligence (AI) applications, and log collection. The system also supports data sharing across multiple clients and can be used directly as shared storage in Milvus.\n\nAfter data, and its corresponding metadata, are persisted to object storage and [Redis](https://redis.io/) respectively, JuiceFS serves as a stateless middleware. Data sharing is realized by enabling different applications to dock with each other seamlessly through a standard file system interface. JuiceFS relies on Redis, an open-source in-memory data store, for metadata storage. Redis is used because it guarantees atomicity and provides high performance metadata operations. All data is stored in object storage through the JuiceFS client. The architecture diagram is as follows:\n\n\n\n\n## **Build a Milvus cluster based on JuiceFS**\n\nA Milvus cluster built with JuiceFS (see architecture diagram below) works by splitting upstream requests using Mishards, a cluster sharding middleware, to cascade the requests down to its sub-modules. When inserting data, Mishards allocates upstream requests to the Milvus write node, which stores newly inserted data in JuiceFS. When reading data, Mishards loads the data from JuiceFS through a Milvus read node to memory for processing, then collects and returns results from sub-services upstream.\n\n\n\n\n\n### **Step 1: Launch MySQL service**\n\nLaunch the MySQL service on **any** node in the cluster. For details, see [Manage Metadata with MySQL](https://milvus.io/docs/v1.1.0/data_manage.md).\n\n### **Step 2: Create a JuiceFS file system**\n\nFor demonstration purposes, the pre-compiled binary JuiceFS program is used. Download the correct [installation package](https://github.com/juicedata/juicefs/releases) for your system and follow the JuiceFS [Quick Start Guide](https://github.com/juicedata/juicefs-quickstart) for detailed install instructions. To create a JuiceFS file system, first set up a Redis database for metadata storage. It is recommended that for public cloud deployments you host the Redis service on the same cloud as the application. Additionally, set up object storage for JuiceFS. In this example, Azure Blob Storage is used; however, JuiceFS supports almost all object services. Select the object storage service that best suits the demands of your scenario.\n\nAfter configuring the Redis service and object storage, format a new file system and mount JuiceFS to the local directory:\n\n```\n1 $ export AZURE_STORAGE_CONNECTION_STRING=\"DefaultEndpointsProtocol=https;AccountName=XXX;AccountKey=XXX;EndpointSuffix=core.windows.net\"\n2 $ ./juicefs format \\\n3 --storage wasb \\\n4 --bucket https://\u003ccontainer\u003e \\\n5 ... \\\n6 localhost test #format\n7 $ ./juicefs mount -d localhost ~/jfs #mount\n8\n```\n\n\u003e If the Redis server is not running locally, replace the localhost with the following address: `redis://\u003cuser:password\u003e@host:6379/1`.\n\nWhen the installation succeeds, JuiceFS returns the shared storage page **/root/jfs**.\n\n\n\n### **Step 3: Start Milvus**\n\nAll the nodes in the cluster should have Milvus installed, and each Milvus node should be configured with read or write permission. Only one Milvus node can be configured as write node, and the rest must be read nodes. First, set the parameters of sections `cluster` and `general` in the Milvus system configuration file **server_config.yaml**:\n\n**Section** `cluster`\n\n| **Parameter** | **Description** | **Configuration** |\n| :------------ | :----------------------------- | :---------------- |\n| `enable` | Whether to enable cluster mode | `true` |\n| `role` | Milvus deployment role | `rw`/`ro` |\n\n**Section** `general`\n\n```\n# meta_uri is the URI for metadata storage, using MySQL (for Milvus Cluster). Format: mysql://\u003cusername:password\u003e@host:port/database\ngeneral:\n timezone: UTC+8\n meta_uri: mysql://root:milvusroot@host:3306/milvus\n```\n\nDuring installation, the configured JuiceFS shared storage path is set as **/root/jfs/milvus/db**.\n\n```\n1 sudo docker run -d --name milvus_gpu_1.0.0 --gpus all \\\n2 -p 19530:19530 \\\n3 -p 19121:19121 \\\n4 -v /root/jfs/milvus/db:/var/lib/milvus/db \\ #/root/jfs/milvus/db is the shared storage path\n5 -v /home/$USER/milvus/conf:/var/lib/milvus/conf \\\n6 -v /home/$USER/milvus/logs:/var/lib/milvus/logs \\\n7 -v /home/$USER/milvus/wal:/var/lib/milvus/wal \\\n8 milvusdb/milvus:1.0.0-gpu-d030521-1ea92e\n9\n```\n\nAfter the installation completes, start Milvus and confirm that it is launched properly.\nFinally, start the Mishards service on **any** of the nodes in the cluster. The image below shows a successful launch of Mishards. For more information, refer to the GitHub [tutorial](https://github.com/milvus-io/bootcamp/tree/new-bootcamp/deployments/juicefs).\n\n\n\n## **Performance benchmarks**\n\nShared storage solutions are usually implemented by network-attached storage (NAS) systems. Commonly used NAS systems types include Network File System (NFS) and Server Message Block (SMB). Public cloud platforms generally provide managed storage services compatible with these protocols, such as Amazon Elastic File System (EFS).\n\nUnlike traditional NAS systems, JuiceFS is implemented based on Filesystem in Userspace (FUSE), where all data reading and writing takes place directly on the application side, further reducing access latency. There are also features unique to JuiceFS that cannot be found in other NAS systems, such as data compression and caching.\n\nBenchmark testing reveals that JuiceFS offers major advantages over EFS. In the metadata benchmark (Figure 1), JuiceFS sees I/O operations per second (IOPS) up to ten times higher than EFS. Additionally, the I/O throughput benchmark (Figure 2) shows JuiceFS outperforms EFS in both single- and multi-job scenarios.\n\n\n\n\n\n\n\n\nAdditionally, benchmark testing shows first query retrieval time, or time to load newly inserted data from disk to memory, for the JuiceFS-based Milvus cluster is just 0.032 seconds on average, indicating that data is loaded from disk to memory almost instantaneously. For this test, first query retrieval time is measured using one million rows of 128-dimensional vector data inserted in batches of 100k at intervals of 1 to 8 seconds.\n\nJuiceFS is a stable and reliable shared file storage system, and the Milvus cluster built on JuiceFS offers both high performance and flexible storage capacity.\n\n## **Learn more about Milvus**\n\nMilvus is a powerful tool capable of powering a vast array of artificial intelligence and vector similarity search applications. To learn more about the project, check out the following resources:\n\n- Read our [blog](https://zilliz.com/blog).\n- Interact with our open-source community on [Slack](https://join.slack.com/t/milvusio/shared_invite/zt-e0u4qu3k-bI2GDNys3ZqX1YCJ9OM~GQ).\n- Use or contribute to the world’s most popular vector database on [GitHub](https://github.com/milvus-io/milvus/).\n- Quickly test and deploy AI applications with our new [bootcamp](https://github.com/milvus-io/bootcamp).\n\n\n\n","title":"Building a Milvus Cluster Based on JuiceFS","metaData":{}},{"id":"2021-06-08-building-an-intelligent-news-recommendation-system-inside-sohu-news-app.md","author":"milvus","desc":"Learn how Milvus was used to build an intelligent news recommendation system inside an app.","canonicalUrl":"https://zilliz.com/blog/building-an-intelligent-news-recommendation-system-inside-sohu-news-app","date":"2021-06-08T01:42:53.489Z","cover":"https://assets.zilliz.com/blog_Sohu_News_dec53d0814.jpg","tags":["Scenarios"],"href":"/blog/2021-06-08-building-an-intelligent-news-recommendation-system-inside-sohu-news-app.md","content":" \n# Building an Intelligent News Recommendation System Inside Sohu News App\nWith [71% of Americans](https://www.socialmediatoday.com/news/new-research-shows-that-71-of-americans-now-get-news-content-via-social-pl/593255/) getting their news recommendations from social platforms, personalized content has quickly become how new media is discovered. Whether people are searching for specific topics, or interacting with recommended content, everything users see is optimized by algorithms to improve click-through rates (CTR), engagement, and relevance. Sohu is a NASDAQ-listed Chinese online media, video, search and gaming group. It leveraged [Milvus](https://milvus.io/), an open-source vector database built by [Zilliz](https://zilliz.com/), to build a semantic vector search engine inside its news app. This article explains how the company used user profiles to fine-tune personalized content recommendations over time, improving user experience and engagement.\n\n## Recommending content using semantic vector search\n\nSohu News user profiles are built from browsing history and adjusted as users search for, and interact with, news content. Sohu’s recommender system uses semantic vector search to find relevant news articles. The system works by identifying a set of tags that are expected to be of interest to each user based on browsing history. It then quickly searches for relevant articles and sorts the results by popularity (measured by average CTR), before serving them to users. \n\nThe New York Times alone publishes [230 pieces of content](https://www.theatlantic.com/technology/archive/2016/05/how-many-stories-do-newspapers-publish-per-day/483845/) a day, which offers a glimpse at the magnitude of new content an effective recommendation system must be capable of processing. Ingesting large volumes of news demands millisecond similarity search and hourly matching of tags to new content. Sohu chose Milvus because it processes massive datasets efficiently and accurately, reduces memory usage during search, and supports high-performance deployments.\n\n## Understanding a news recommendation system workflow\n\nSohu’s semantic vector search-based content recommendation relies on the Deep Structured Semantic Model (DSSM), which uses two neural networks to represent user queries and news articles as vectors. The model calculates the cosine similarity of the two semantic vectors, then the most similar batch of news is sent to the recommendation candidates pool. Next, news articles are ranked based on their estimated CTR, and those with the highest predicted click-through rate are displayed to users.\n\n### Encoding news articles into semantic vectors with BERT-as-service\n\nTo encode news articles into semantic vectors, the system uses the [BERT-as-service](https://github.com/hanxiao/bert-as-service.git) tool. If the word count of any piece of content exceeds 512 while using this model, information loss occurs during the embedding process. To help overcome this, the system first extracts a summary and encodes it into a 768-dimensional semantic vector. Then the two most relevant topics from each news article are extracted, and the corresponding pre-trained topic vectors (200-dimensions) are identified based on topic ID. Next the topic vectors are spliced into the 768-dimensional semantic vector extracted from the article summary, forming a 968-dimensional semantic vector. \n\nNew content continuously comes in through Kafta, and is converted into semantic vectors before being inserted into the Milvus database.\n\n### Extracting semantically similar tags from user profiles with BERT-as-service\n\nThe other neural network of the model is user semantic vector. Semantically similar tags (e.g., coronavirus, covid, COVID-19, pandemic, novel strain, pneumonia) are extracted from user profiles based on interests, search queries, and browsing history. The list of acquired tags is sorted by weight, and the top 200 are divided into different semantic groups. Permutations of the tags within each semantic group are used to generate new tag phrases, which are then encoded into semantic vectors through BERT-as-service\n\nFor each user profile, sets of tag phrases have a [corresponding set of topics](https://github.com/baidu/Familia) that are marked by a weight indicating a user's interest level. The top two topics out of all relevant topics are selected and encoded by the machine learning (ML) model to be spliced into the corresponding tag semantic vector, forming a 968-dimensional user semantic vector. Even if the system generates the same tags for different users, different weights for tags and their corresponding topics, as well as explicit variance between each user's topic vectors, ensures recommendations are unique\n\nThe system is able to make personalized news recommendations by calculating the cosine similarity of the semantic vectors extracted from both user profiles and news articles.\n\n\n\n\n### Computing new semantic user profile vectors and inserting them to Milvus\n\nSemantic user profile vectors are computed daily, with data from the previous 24-hour period processed the following evening. Vectors are inserted into Milvus individually and run through the query process to serve relevant news results to users. News content is inherently topical, requiring computation to be run hourly to generate a current newsfeed that contains content that has a high predicted click-through rate and is relevant to users. News content is also sorted into partitions by date, and old news is purged daily.\n\n### Decreasing semantic vector extraction time from days to hours\n\nRetrieving content using semantic vectors requires converting tens of millions of tag phrases into semantic vectors every day. This is a time-consuming process that would require days to complete even when running on graphics processing units (GPU), which accelerate this type of computation. To overcome this technical issue, semantic vectors from the previous embedding must be optimized so that when similar tag phrases surface corresponding semantic vectors are directly retrieved.\n\nThe semantic vector of the existing set of tag phrases is stored, and a new set of tag phrases that is generated daily gets encoded into MinHash vectors. [Jaccard distance](https://milvus.io/docs/v1.1.1/metric.md) is used to compute similarity between the MinHash vector of the new tag phrase and the saved tag phrase vector. If the Jaccard distance exceeds a pre-defined threshold, the two sets are considered similar. If the similarity threshold is met, new phrases can leverage the semantic information from previous embeddings. Tests suggest a distance above 0.8 should guarantee enough accuracy for most situations.\n\nThrough this process, the daily conversion of the tens of millions of vectors mentioned above is reduced from days to around two hours. Although other methods of storing semantic vectors might be more appropriate depending on specific project requirements, computing similarity between two tag phrases using Jaccard distance in a Milvus database remains an efficient and accurate method in a wide variety of scenarios.\n\n\n\n\n## Overcoming “bad cases” of short text classification\n\nWhen classifying news text, short news articles have fewer features for extraction than longer ones. Because of this, classification algorithms fail when content of varying lengths is run through the same classifier. Milvus helps solve this issue by searching for multiple pieces of long text classification information with similar semantics and reliable scores, then using a voting mechanism to modify short text classification. \n\n### Identifying and resolving misclassified short text \n\nPrecise classification of each news article is crucial to providing useful content recommendations. Since short news articles have fewer features, applying the same classifier for news with different lengths results in a higher error rate for short text classification. Human labeling is too slow and inaccurate for this task, so BERT-as-service and Milvus are used to quickly identify misclassified short text in batches, correctly reclassify them, then use batches of data as a corpus for training against this problem.\n\nBERT-as-service is used to encode a total number of five million long news articles with a classifier score greater than 0.9 into semantic vectors. After inserting the long text articles into Milvus, short text news is encoded into semantic vectors. Each short news semantic vector is used to query the Milvus database and obtain the top 20 long news articles with the highest cosine similarity to the target short news. If 18 of the top 20 semantically similar long news appear to be in the same classification and it differs from that of the query short news, then the short news classification is considered incorrect and must be adjusted to align with the 18 long news articles.\n\nThis process quickly identifies and corrects inaccurate short text classifications. Random sampling statistics show that after short text classifications are corrected, the overall accuracy of text classification exceeds 95%. By leveraging the classification of high-confidence long text to correct the classification of short text, the majority of bad classification cases are corrected in a short amount of time. This also offers a good corpus for training a short text classifier.\n\n\n\n\n## Milvus can power real-time news content recommendation and more\n\nMilvus greatly improved the real-time performance of Sohu’s news recommendation system, and also bolstered the efficiency of identifying misclassified short text. If you’re interested in learning more about Milvus and its various applications:\n\n- Read our [blog](https://zilliz.com/blog).\n- Interact with our open-source community on [Slack](https://join.slack.com/t/milvusio/shared_invite/zt-e0u4qu3k-bI2GDNys3ZqX1YCJ9OM~GQ).\n- Use or contribute to the world’s most popular vector database on [GitHub](https://github.com/milvus-io/milvus/).\n- Quickly test and deploy AI applications with our new [bootcamp](https://github.com/milvus-io/bootcamp).\n \n","title":"Building an Intelligent News Recommendation System Inside Sohu News App","metaData":{}},{"id":"2021-05-28-accelerating-compilation-with-dependency-decoupling-and-testing-containerization.md","author":"Zhifeng Zhang","desc":"Discover how zilliz to reduce compile times 2.5x using dependency decoupling and containerization techniques for large-scale AI and MLOps projects.","canonicalUrl":"https://zilliz.com/blog/accelerating-compilation-with-dependency-decoupling-and-testing-containerization","date":"2021-05-28T00:00:00.000Z","cover":"https://assets.zilliz.com/cover_20e3cddb96.jpeg","tags":["Engineering"],"href":"/blog/2021-05-28-accelerating-compilation-with-dependency-decoupling-and-testing-containerization.md","content":" \n# Accelerating Compilation 2.5X with Dependency Decoupling \u0026 Testing Containerization\nCompile time can be compounded by complex internal and external dependencies that evolve throughout the development process, as well as changes in compilation environments such as the operating system or hardware architectures. Following are common issues one may encounter when working on large-scale AI or MLOps projects: \n\n**Prohibitively long compilation** - Code integration is done hundreds of times each day. With hundreds of thousands of lines of code in place, even a small change could result in a full compilation that typically takes one or more hours. \n\n**Complex compilation environment** - The project code needs to be compiled under different environments, which involve different operating systems, such as CentOS and Ubuntu, underlying dependencies, such as GCC, LLVM, and CUDA, and hardware architectures. And compilation under a specific environment normally may not work under a different environment. \n\n**Complex dependencies** - Project compilation involves more than 30 between-component and third-party dependencies. Project development often leads to changes in dependencies, inevitably causing dependency conflicts. The version control between dependencies is so complex that updating version of dependencies will easily affect other components. \n\n**Third-party dependency download is slow or fails** - Network delays or unstable third-party dependency libraries cause slow resource downloads or access failures, seriously affecting code integration. \n\nBy decoupling dependencies and implementing testing containerization, we managed to decrease average compile time by 60% while working on the open-source embeddings similarity search project [Milvus](https://milvus.io/). \n\n\u003cbr/\u003e\n\n### Decouple the dependencies of the project\n\nProject compilation usually involves a large number of internal and external component dependencies. The more dependencies a project has, the more complex it becomes to manage them. As software grows, it becomes more difficult and costly to change or remove dependencies, as well as identify the effects of doing so. Regular maintenance is required throughout the development process to ensure the dependencies functions properly.\nPoor maintenance, complex dependencies, or faulty dependencies can cause conflicts that slow or stall development. In practice, this can mean lagging resource downloads, access failures that negatively impact code integration, and more. Decoupling project dependencies can mitigate defects and reduce compile time, accelerating system testing and avoiding unnecessary drag on software development.\n\nTherefore, we recommend decouple dependencies your project:\n- Split up components with complex dependencies\n- Use different repositories for version management.\n- Use configuration files to manage version information, compilation options, dependencies, etc.\n- Add the configuration files to the component libraries so that they are updated as the project iterates.\n\n**Compile optimization between components** — Pull and compile the relevant component according to the dependencies and the compile options recorded in the configuration files. Tag and pack the binary compilation results and the corresponding manifest files, and then upload them to your private repository. If no change is made to a component or the components it depends on, playback its compilation results according to the manifest files. For issues such as network delays or unstable third-party dependency libraries, try setting up an internal repository or using mirrored repositories.\n\nTo optimize compilation between components:\n\n1.Create dependency relationship graph — Use the configuration files in the component libraries to create dependency relationship graph. Use the dependency relationship to retrieve the version information (Git Branch, Tag, and Git commit ID) and compilation options and more of both upstream and downstream dependent components.\n\n\n\n2.**Check for dependencies** — Generate alerts for circular dependencies, version conflicts, and other issues that arise between components.\n\n3.**Flatten dependencies** — Sort dependencies by Depth First Search (DFS) and front-merge components with duplicate dependencies to form a dependency graph.\n\n\n\n4.Use MerkleTree algorithm to generate a hash (Root Hash) containing dependencies of each component based on version information, compilation options, and more. Combined with information such as component name, the algorithm forms a unique tag for each component.\n\n\n\n5.Based on the component’s unique tag information, check if a corresponding compilation archive exists in the private repo. If a compilation archive is retrieved, unzip it to get the manifest file for playback; if not, compile the component, mark up the generated compilation object files and manifest file, and upload them to the private repo.\n\n\u003cbr/\u003e\n\n**Implement compilation optimizations within components** — Choose a language-specific compilation cache tool to cache the compiled object files, and upload and store them in your private repository. For C/C++ compilation, choose a compilation cache tool like CCache to cache the C/C++ compilation intermediate files, and then archive the local CCache cache after compilation. Such compile cache tools simply cache the changed code files one by one after compilation, and copy the compiled components of the unchanged code file so that they can be directly involved in the final compilation.\nOptimization of the compilation within components includes the following steps:\n\n1. Add the necessary compilation dependencies to Dockerfile. Use Hadolint to perform compliance checks on Dockerfile to ensure that the image conforms to Docker’s best practices.\n2. Mirror the compilation environment according to the project sprint version (version + build), operating system, and other information.\n3. Run the mirrored compilation environment container, and transfer the image ID to the container as an environment variable. Here’s an example command for getting image ID: “docker inspect ‘ — type=image’ — format ‘{{.ID}}’ repository/build-env:v0.1-centos7”.\n4. Choose the appropriate compile cache tool: Enter your containter to integrate and compile your codes and check in your private repository if an appropriate compile cache exists. If yes, download and extract it to the specified directory. After all components are compiled, the cache generated by the compile cache tool is packaged and uploaded to your private repository based on the project version and image ID.\n\n\u003cbr/\u003e\n\n### Further compilation optimization\n\nOur initially-built occupies too much disk space and network bandwidth, and takes a long time to deploy, we took the following measures:\n1. Choose the leanest base image to reduce the image size, e.g. alpine, busybox, etc.\n2. Reduce the number of image layers. Reuse dependencies as much as possible. Merge multiple commands with “\u0026\u0026”.\n3. Clean up the intermediate products during image building.\n4. Use image cache to build image as much as possible.\n\nAs our project continues to progress, disk usage and network resource began to soar as the compilation cache increases, while some of the compilation caches are underutilized. We then made the following adjustments:\n\n**Regularly clean up cache files** — Regularly check the private repository (using scripts for example), and clean up cache files that have not changed for a while or have not been downloaded much.\n\n**Selective compile caching** — Only cache resource-demanding compiles, and skip caching compiles that do not require much resource.\n\n\u003cbr/\u003e\n\n### Leveraging containerized testing to reduce errors, improve stability and reliability\n\nCodes have to be compiled in different environments, which involve variety of operating systems (e.g. CentOS and Ubuntu), underlying dependencies (e.g. GCC, LLVM, and CUDA), and specific hardware architectures. Code that successfully compiles under a specific environment fail in a different environment. By running tests inside containers, the testing process becomes faster and more accurate.\n\nContainerization ensures that the test environment is consistent, and that an application is working as expected. The containerized testing approach packages tests as image containers and builds a truly-isolated test environment. Our testers found that this approach pretty useful, which ended up reducing compile times by as much as 60%.\n\n**Ensure a consistent compile environment** — As the compiled products are sensitive to changes in the system environment, unknown errors may occur in different operating systems. We have to tag and archive the compiled product cache according to the changes in the compile environment, but they are difficult to categorize. So we introduced containerization technology to unify the compile environment to solve such issues.\n\n\u003cbr/\u003e\n\n### Conclusion\n\nBy analyzing project dependencies, this article introduces different methods for compilation optimization between and within components, providing ideas and best practices for building stable and efficient continuous code integration. These methods helped solve slow code integration caused by complex dependencies, unify operations inside the container to ensure the consistency of the environment, and improve compilation efficiency through the playback of the compilation results and the use of compilation cache tools to cache the intermediate compilation results.\n\nThis above-mentioned practices have reduced the compile time of the project by 60% on average, greatly improving the overall efficiency of code integration. Moving forward, we will continue parallelizing compilation between and within components to further reduce compilation times.\n\n\u003cbr/\u003e\n\n*The following sources were used for this article:*\n\n- “Decoupling Source Trees into Build-Level Components”\n- “[Factors to consider when adding third party dependencies to a project](https://dev.to/brpaz/factors-to-consider-when-adding-third-party-dependencies-to-a-project-46hf)”\n- “[Surviving Software Dependencies](https://queue.acm.org/detail.cfm?id=3344149)”\n- “[Understanding Dependencies: A Study of the Coordination Challenges in Software Development](https://www.cc.gatech.edu/~beki/t1.pdf)”\n\n\u003cbr/\u003e\n\n### About the author\n\nZhifeng Zhang is a senior DevOps engineer at Zilliz.com working on Milvus, an open-source vector database, and authorized instructor of the LF open-source software university in China. He received his bachelor’s degree in Internet of Things (IOT) from Software Engineering Institute of Guangzhou. He spends his career participating in and leading projects in the area of CI/CD, DevOps, IT infrastructure management, Cloud-Native toolkit, containerization, and compilation process optimization.\n\n\n\n\n\n\n\n\n ","title":"Accelerating Compilation 2.5X with Dependency Decoupling \u0026 Testing Containerization","metaData":{}},{"id":"2021-05-20-inside-milvus-1.1.0.md","author":"milvus","desc":"Milvus v1.1.0 has arrived! New features, improvements, and bug fixes are available now.","canonicalUrl":"https://zilliz.com/blog/inside-milvus-1.1.0","date":"2021-05-20T08:35:42.700Z","cover":"https://assets.zilliz.com/v1_1_cover_487e70971a.jpeg","tags":["News"],"href":"/blog/2021-05-20-inside-milvus-1.1.0.md","content":" \n# Inside Milvus 1.1.0\n[Milvus](https://github.com/milvus-io) is an ongoing open-source software (OSS) project focused on building the world's fastest and most reliable vector database. New features inside Milvus v1.1.0 are the first of many updates to come, thanks to long-term support from the open-source community and sponsorship from Zilliz. This blog article covers the new features, improvements, and bug fixes included with Milvus v1.1.0.\n\n**Jump to:**\n\n- [New features](#new-features)\n- [Improvements](#improvements)\n- [Bug fixes](#bug-fixes)\n\n\u003cbr/\u003e\n\n## New features\n\nLike any OSS project, Milvus is a perpetual work in progress. We strive to listen to our users and the open-source community to prioritize the features that matter most. The latest update, Milvus v1.1.0, offers the following new features:\n\n### Specify partitions with `get_entity_by_id()` method calls\n\nTo further accelerate vector similarity search, Milvus 1.1.0 now supports retrieving vectors from a specified partition. Generally, Milvus supports querying vectors through specified vector IDs. In Milvus 1.0, calling the method `get_entity_by_id()` searches the entire collection, which can be time consuming for large datasets. As we can see from the code below, `GetVectorsByIdHelper` uses a `FileHolder` structure to loop through and find a specific vector. \n\n```\nstd::vector\u003cmeta::CollectionSchema\u003e collection_array; \n auto status = meta_ptr_-\u003eShowPartitions(collection.collection_id_, collection_array); \n \n collection_array.push_back(collection); \n status = meta_ptr_-\u003eFilesByTypeEx(collection_array, file_types, files_holder); \n if (!status.ok()) { \n std::string err_msg = \"Failed to get files for GetVectorByID: \" + status.message(); \n LOG_ENGINE_ERROR_ \u003c\u003c err_msg; \n return status; \n } \n \n if (files_holder.HoldFiles().empty()) { \n LOG_ENGINE_DEBUG_ \u003c\u003c \"No files to get vector by id from\"; \n return Status(DB_NOT_FOUND, \"Collection is empty\"); \n } \n \n cache::CpuCacheMgr::GetInstance()-\u003ePrintInfo(); \n status = GetVectorsByIdHelper(id_array, vectors, files_holder); \nDBImpl::GetVectorsByIdHelper(const IDNumbers\u0026 id_array, std::vector\u003cengine::VectorsData\u003e\u0026 vectors, \n meta::FilesHolder\u0026 files_holder) { \n // attention: this is a copy, not a reference, since the files_holder.UnMarkFile will change the array internal \n milvus::engine::meta::SegmentsSchema files = files_holder.HoldFiles(); \n LOG_ENGINE_DEBUG_ \u003c\u003c \"Getting vector by id in \" \u003c\u003c files.size() \u003c\u003c \" files, id count = \" \u003c\u003c id_array.size(); \n \n // sometimes not all of id_array can be found, we need to return empty vector for id not found \n // for example: \n // id_array = [1, -1, 2, -1, 3] \n // vectors should return [valid_vector, empty_vector, valid_vector, empty_vector, valid_vector] \n // the ID2RAW is to ensure returned vector sequence is consist with id_array \n using ID2VECTOR = std::map\u003cint64_t, VectorsData\u003e; \n ID2VECTOR map_id2vector; \n \n vectors.clear(); \n \n IDNumbers temp_ids = id_array; \n for (auto\u0026 file : files) { \n```\n\nHowever, this structure is not filtered by any partitions in `FilesByTypeEx()`. In Milvus v1.1.0, it is possible for the system to pass partition names to the `GetVectorsIdHelper` loop so that the `FileHolder` only contains segments from specified partitions. Put differently, if you know exactly which partition the vector for a search belongs to, you can specify the partition name in a `get_entity_by_id()` method call to accelerate the search process.\n\nWe not only made modifications to code controlling system queries at the Milvus server level, but also updated all our SDKs(Python, Go, C++, Java, and RESTful) by adding a parameter for specifying partition names. For example, in pymilvus, the definition of `get_entity_by_id` `def get_entity_by_id(self, collection_name, ids, timeout=None)` is changed to `def get_entity_by_id(self, collection_name, partition_tags=None, ids, timeout=None)`.\n\n\u003cbr/\u003e\n\n### Specify partitions with `delete_entity_by_id()` method calls\n\nTo make vector management more efficient, Milvus v1.1.0 now supports specifying partition names when deleting a vector in a collection. In Milvus 1.0, vectors in a collection can only be deleted by ID. When calling the delete method, Milvus will scan all vectors in the collection. However, it is far more efficient to scan only relevant partitions when working with massive million, billion, or even trillion vector datasets. Similar to the new feature for specifying partitions with `get_entity_by_id()` method calls, modifications were made to the Milvus code using the same logic.\n\n\u003cbr/\u003e\n\n### New method `release_collection()`\n\nTo free up memory Milvus used to load collections at runtime, a new method `release_collection()` has been added in Milvus v1.1.0 to manually unload specific collections from cache.\n\n\u003cbr/\u003e\n\n## Improvements\n\nAlthough new features are usually all the rage, it's also important to improve what we already have. What follows are upgrades and other general improvements over Milvus v1.0.\n\n\u003cbr/\u003e\n\n### Improved performance of `get_entity_by_id()` method call\n\nThe chart below is a comparison of vector search performance between Milvus v1.0 and Milvus v1.1.0:\n\n\u003e CPU: Intel(R) Core(TM) i7-8550U CPU @ 1.80GHz * 8 \u003cbr/\u003e\n\u003e Segment file size = 1024 MB \u003cbr/\u003e\n\u003e Row count = 1,000,000 \u003cbr/\u003e\n\u003e Dim = 128 \n\n| Query ID Num | v 1.0.0 | v1.1.0 |\n| :-----------: | :-----------: | :-----------: |\n| 10 | 9 ms | 2 ms |\n| 100 | 149 ms | 19 ms |\n\n\u003cbr/\u003e\n\n### Hnswlib upgraded to v0.5.0\n\nMilvus adopts multiple widely used index libraries, including Faiss, NMSLIB, Hnswlib, and Annoy to simplify the process of choosing the right index type for a given scenario.\n\nHnswlib has been upgraded from v0.3.0 to v0.5.0 in Milvus 1.1.0 due to a bug detected in the earlier version. Additionally, upgrading Hnswlib improves `addPoint()` performance in index building.\n\nA Zilliz developer created a pull request (PR) to improve Hnswlib performance while building indexes in Milvus. See [PR #298](https://github.com/nmslib/hnswlib/pull/298) for details.\n\nThe chart below is a comparison of `addPoint()` performance between Hnswlib 0.5.0 and the proposed PR:\n\n\u003e CPU: Intel(R) Core(TM) i7-8550U CPU @ 1.80GHz * 8 \u003cbr/\u003e\n\u003e Dataset: sift_1M (row count = 1000000, dim = 128, space = L2)\n\n| | 0.5.0 | PR-298 |\n| :-----------: | :-----------: | :-----------: |\n| M = 16, ef_construction = 100 | 274406 ms | 265631 ms |\n| M = 16, ef_construction = 200 | 522411 ms | 499639 ms |\n\n\u003cbr/\u003e\n\n### Improved IVF index training performance\n\nCreating an index includes training, inserting and writing data to disk. Milvus 1.1.0 improves the training component of index building. The chart below is a comparison of IVF index training performance between Milvus 1.0 and Milvus 1.1.0:\n\n\u003e CPU: Intel(R) Core(TM) i7-8550U CPU @ 1.80GHz * 8 \u003cbr/\u003e\n\u003e Dataset: sift_1m (row_count = 1000000, dim = 128, metric_type = L2)\n\n| | v1.0.0 (ms) | v1.1.0 (ms) |\n| :-----------: | :-----------: | :-----------: |\n| ivf_flat (nlist = 2048) | 90079 | 81544 |\n| ivf_pq (nlist = 2048, m=16) | 103535 | 97115 |\n| ivf_pq (nlist = 2048, m=32) | 108638 | 104558 |\n| ivf_flat (nlist = 4096) | 340643 | 310685 |\n| ivf_pq (nlist = 4096, m=16) | 351982 | 323758 |\n| ivf_pq (nlist = 4096, m=32) | 357359 | 330887 |\n\n\n\u003cbr/\u003e\n\n## Bug fixes\n\nWe also fixed some bugs to make Milvus more stable and efficient when managing vector datasets. See [Fixed Issues](https://milvus.io/docs/v1.1.0/release_notes.md#Fixed-issues) for more details.\n\n ","title":"Inside Milvus 1.1.0","metaData":{}},{"id":"2021-05-19-ai-in-finance.md","author":"milvus","desc":"Milvus can be used to build AI applications for the finance industry including chatbots, recommender systems, and more.","canonicalUrl":"https://zilliz.com/blog/ai-in-finance","date":"2021-05-19T03:41:20.776Z","cover":"https://assets.zilliz.com/03_1_1e5aaf7dd1.jpg","tags":["Scenarios"],"href":"/blog/2021-05-19-ai-in-finance.md","content":" \n# Accelerating AI in Finance with Milvus, an Open-Source Vector Database\nBanks and other financial institutions have long been early adopters of open-source software for big data processing and analytics. In 2010, Morgan Stanley [began using](https://www.forbes.com/sites/tomgroenfeldt/2012/05/30/morgan-stanley-takes-on-big-data-with-hadoop/?sh=19f4f8cd16db) the open-source Apache Hadoop framework as part of a small experiment. The company was struggling to successfully scale traditional databases to the massive volumes of data its scientists wanted to leverage, so it decided to explore alternative solutions. Hadoop is now a staple at Morgan Stanley, helping with everything from managing CRM data to portfolio analysis. Other open-source relational database software such as MySQL, MongoDB, and PostgreSQL have been indispensable tools for making sense of big data in the finance industry.\n\nTechnology is what gives the financial services industry a competitive edge, and artificial intelligence (AI) is rapidly becoming the standard approach to extracting valuable insights from big data and analyzing activity in real-time across the banking, asset management, and insurance sectors. By using AI algorithms to convert unstructured data such as images, audio, or video to vectors, a machine-readable numeric data format, it is possible to run similarity searches on massive million, billion, or even trillion vector datasets. Vector data is stored in high-dimensional space, and similar vectors are found using similarity search, which requires a dedicated infrastructure called a vector database.\n\n\n\n\n[Milvus](https://github.com/milvus-io/milvus) is an open-source vector database built specifically for managing vector data, which means engineers and data scientists can focus on building AI applications or conducting analysis—instead of the underlying data infrastructure. The platform was built around AI application development workflows and is optimized to streamline machine learning operations (MLOps). For more information about Milvus and its underlying technology, check out our [blog](https://zilliz.com/blog/Vector-Similarity-Search-Hides-in-Plain-View).\n\nCommon applications of AI in the financial services industry include algorithmic trading, portfolio composition and optimization, model validation, backtesting, Robo-advising, virtual customer assistants, market impact analysis, regulatory compliance, and stress testing. This article covers three specific areas where vector data is leveraged as one of the most valuable assets for banking and financial companies:\n\n1. Enhancing customer experience with banking chatbots\n2. Boosting financial services sales and more with recommender systems\n3. Analyzing earnings reports and other unstructured financial data with semantic text mining\n\n\u003cbr/\u003e\n\n### Enhancing customer experience with banking chatbots\n\nBanking chatbots can improve customer experiences by helping consumers select investments, banking products, and insurance policies. Digital services are rising rapidly in popularity in part due to trends accelerated by the coronavirus pandemic. Chatbots work by using natural language processing (NLP) to convert user-submitted questions into semantic vectors to search for matching answers. Modern banking chatbots offer a personalized natural experience for users and speak in a conversational tone. Milvus provides a data fabric well suited for creating chatbots using real-time vector similarity search.\n\nLearn more in our demo that covers building [chatbots with Milvus](https://zilliz.com/blog/building-intelligent-chatbot-with-nlp-and-milvus).\n\n\n\n \u003cbr/\u003e\n\n#### Boosting financial services sales and more with recommender systems:\n\nThe private banking sector uses recommender systems to increase sales of financial products through personalized recommendations based on customer profiles. Recommender systems can also be leveraged in financial research, business news, stock selection, and trading support systems. Thanks to deep learning models, every user and item is described as an embedding vector. A vector database offers an embedding space where similarities between users and items can be calculated.\n\nLearn more from our [demo](https://zilliz.com/blog/graph-based-recommendation-system-with-milvus) covering graph-based recommendation systems with Milvus.\n\n\u003cbr/\u003e\n\n#### Analyzing earnings reports and other unstructured financial data with semantic text mining:\n\nText mining techniques had a substantial impact on the financial industry. As financial data grows exponentially, text mining has emerged as an important field of research in the domain of finance.\n\nDeep learning models are currently applied to represent financial reports through word vectors capable of capturing numerous semantic aspects. A vector database like Milvus is able to store massive semantic word vectors from millions of reports, then conduct similarity searches on them in milliseconds.\n\nLearn more about how to [use deepset's Haystack with Milvus](https://medium.com/deepset-ai/semantic-search-with-milvus-knowledge-graph-qa-web-crawlers-and-more-837451eae9fa).\n\n\u003cbr/\u003e\n\n### Don’t be a stranger\n\n- Find or contribute to Milvus on [GitHub](https://github.com/milvus-io/milvus/).\n- Interact with the community via [Slack](https://join.slack.com/t/milvusio/shared_invite/zt-e0u4qu3k-bI2GDNys3ZqX1YCJ9OM~GQ).\n- Connect with us on [Twitter](https://twitter.com/milvusio).\n\n\n ","title":"Accelerating AI in Finance with Milvus, an Open-Source Vector Database","metaData":{}},{"id":"2021-05-13-building-a-search-by-image-shopping-experience-with-vova-and-milvus.md","author":"milvus","desc":"Discover how Milvus, an open-source vector database, was used by e-commerce platform VOVA to power shopping by image.","canonicalUrl":"https://zilliz.com/blog/building-a-search-by-image-shopping-experience-with-vova-and-milvus","date":"2021-05-13T08:44:05.528Z","cover":"https://assets.zilliz.com/vova_thumbnail_db2d6c0c9c.jpg","tags":["Scenarios"],"href":"/blog/2021-05-13-building-a-search-by-image-shopping-experience-with-vova-and-milvus.md","content":" \n# Building a Search by Image Shopping Experience with VOVA and Milvus\nJump to:\n \n- [Building a Search by Image Shopping Experience with VOVA and Milvus](#building-a-search-by-image-shopping-experience-with-vova-and-milvus)\n - [How does image search work?](#how-does-image-search-work)\n - [*System process of VOVA's search by image functionality.*](#system-process-of-vovas-search-by-image-functionality)\n - [Target detection using the YOLO model](#target-detection-using-the-yolo-model)\n - [*YOLO network architecture.*](#yolo-network-architecture)\n - [Image feature vector extraction with ResNet](#image-feature-vector-extraction-with-resnet)\n - [*ResNet structure.*](#resnet-structure)\n - [Vector similarity search powered by Milvus](#vector-similarity-search-powered-by-milvus)\n - [*Mishards architecture in Milvus.*](#mishards-architecture-in-milvus)\n - [VOVA's shop by image tool](#vovas-shop-by-image-tool)\n - [*Screenshots of VOVA's search by image shopping tool.*](#screenshots-of-vovas-search-by-image-shopping-tool)\n - [Reference](#reference)\n\nOnline shopping surged in 2020, [up 44%](https://www.digitalcommerce360.com/2021/02/15/ecommerce-during-coronavirus-pandemic-in-charts/) in large part due to the coronavirus pandemic. As people sought to socially distance and avoid contact with strangers, no-contact delivery became an incredibly desirable option for many consumers. This popularity has also led to people buying a greater variety of goods online, including niche items that can be hard to describe using a traditional keyword search.\n\nTo help users overcome the limitations of keyword-based queries, companies can build image search engines that allow users to use images instead of words for search. Not only does this allow users to find items that are difficult to describe, but it also helps them shop for things they encounter in real life. This functionality helps build a unique user experience and offers general convenience that customers appreciate.\n\nVOVA is an emerging e-commerce platform that focuses on affordability and offering a positive shopping experience to its users, with listings covering millions of products and support for 20 languages and 35 major currencies. To enhance the shopping experience for its users, the company used Milvus to build image search functionality into its e-commerce platform. The article explores how VOVA successfully built an image search engine with Milvus. \n\n\u003cbr/\u003e\n\n### How does image search work?\n\nVOVA's shop by image system searches the company's inventory for product images that are similar to user uploads. The following chart shows the two stages of the system process, the data import stage (blue) and the query stage (orange):\n\n1. Use the YOLO model to detect targets from uploaded photos;\n2. Use ResNet to extract feature vectors from the detected targets; \n3. Use Milvus for vector similarity search.\n\n\n\n\u003cbr/\u003e\n\n### Target detection using the YOLO model\n\nVOVA's mobile apps on Android and iOS currently support image search. The company uses a state-of-the-art, real-time object detection system called YOLO (You only look once) to detect objects in user uploaded images. The YOLO model is currently in its fifth iteration. \n\nYOLO is a one-stage model, using only one convolutional neural network (CNN) to predict categories and positions of different targets. It is small, compact, and well suited for mobile use. \n\nYOLO uses convolutional layers to extract features and fully-connected layers to obtain predicted values. Drawing inspiration from the GooLeNet model, YOLO’s CNN includes 24 convolutional layers and two fully-connected layers.\n\nAs the following illustration shows, a 448 \u0026times; 448 input image is converted by a number of convolutional layers and pooling layers to a 7 \u0026times; 7 \u0026times; 1024-dimensional tensor (depicted in the third to last cube below), and then converted by two fully-connected layers to a 7 \u0026times; 7 \u0026times; 30-dimensional tensor output.\n\nThe predicted output of YOLO P is a two-dimensional tensor, whose shape is [batch,7 \u0026times;7 \u0026times;30]. Using slicing, P[:,0:7\u0026times;7\u0026times;20] is the category probability, P[:,7\u0026times;7\u0026times;20:7\u0026times;7\u0026times;(20+2)] is the confidence, and P[:,7\u0026times;7\u0026times;(20+2)]:] is the predicted result of the bounding box.\n\n\n\n\n\u003cbr/\u003e\n\n### Image feature vector extraction with ResNet\n\nVOVA adopted the residual neural network (ResNet) model to extract feature vectors from an extensive product image library and user uploaded photos. ResNet is limited because as the depth of a learning network increases, the accuracy of the network decreases. The image below depicts ResNet running the VGG19 model (a variant of the VGG model) modified to include a residual unit through the short circuit mechanism. VGG was proposed in 2014 and includes just 14 layers, while ResNet came out a year later and can have up to 152.\n\nThe ResNet structure is easy to modify and scale. By changing the number of channels in the block and the number of stacked blocks, the width and depth of the network can be easily adjusted to obtain networks with different expressive capabilities. This effectively solves the network degeneration effect, where accuracy declines as the depth of learning increases. With sufficient training data, a model with improving expressive performance can be obtained while gradually deepening the network. Through model training, features are extracted for each picture and converted to 256-dimensional floating point vectors.\n\n\n\n\n\u003cbr/\u003e\n\n### Vector similarity search powered by Milvus\n \nVOVA's product image database includes 30 million pictures and is growing rapidly. To quickly retrieve the most similar product images from this massive dataset, Milvus is used to conduct vector similarity search. Thanks to a number of optimizations, Milvus offers a fast and streamlined approach to managing vector data and building machine learning applications. Milvus offers integration with popular index libraries (e.g., Faiss, Annoy), supports multiple index types and distance metrics, has SDKs in multiple languages, and provides rich APIs for managing vector data.\n\nMilvus can conduct similarity search on trillion-vector datasets in milliseconds, with a query time under 1.5 seconds when nq=1 and an average batch query time under 0.08 seconds. To build its image search engine, VOVA referred to the design of Mishards, Milvus' sharding middleware solution (see the chart below for its system design), to implement a highly available server cluster. By leveraging the horizontal scalability of a Milvus cluster, the project requirement for high query performance on massive datasets was met.\n\n\n\n\n\n### VOVA's shop by image tool\n \nThe screenshots below show the VOVA search by image shopping tool on the company's Android app.\n\n\n\n \nAs more users search for products and upload photos, VOVA will continue to optimize the models that power the system. Additionally, the company will incorporate new Milvus functionality that can further enhance the online shopping experience of its users.\n\n### Reference\n\n**YOLO:**\n\nhttps://arxiv.org/pdf/1506.02640.pdf\n\nhttps://arxiv.org/pdf/1612.08242.pdf\n\n**ResNet:**\n\nhttps://arxiv.org/abs/1512.03385\n\n**Milvus:**\n\nhttps://milvus.io/docs\n\n\n\n\n\n \n \n","title":"Building a Search by Image Shopping Experience with VOVA and Milvus","metaData":{}},{"id":"2021-04-29-Whats-Inside-Milvus-1.0.md","author":"milvus","desc":"Milvus v1.0 is available now. Learn about the Milvus fundamentals as well as key features of Milvus v1.0.","canonicalUrl":"https://zilliz.com/blog/Whats-Inside-Milvus-1.0","date":"2021-04-29T08:46:04.019Z","cover":"https://assets.zilliz.com/Milvus_510cf50aee.jpeg","tags":["Engineering"],"href":"/blog/2021-04-29-Whats-Inside-Milvus-1.0.md","content":"\n# What's Inside Milvus 1.0?\n\n\n\nMilvus is an open-source vector database designed to manage massive million, billion, or even trillion vector datasets. Milvus has broad applications spanning new drug discovery, computer vision, autonomous driving, recommendation engines, chatbots, and much more.\n\nIn March, 2021 Zilliz, the company behind Milvus, released the platform's first long-term support version—Milvus v1.0. After months of extensive testing, a stable, production ready version of the world's most popular vector database is ready for prime time. This blog article covers some Milvus fundamentals as well as key features of v1.0.\n\n\u003cbr/\u003e\n\n### Milvus distributions\n\nMilvus is available in CPU-only and GPU-enabled distributions. The former relies exclusively on CPU for index building and search; the latter enables CPU and GPU hybrid search and index building that further accelerates Milvus. For example, using the hybrid distribution, CPU can be used for search and GPU for index building, further improving query efficiency.\n\nBoth Milvus distributions are available in Docker. You can either compile Milvus from Docker (if your operating system supports it) or compile Milvus from source code on Linux (other operating systems are not supported).\n\n\u003cbr/\u003e\n\n### Embedding vectors\n\nVectors are stored in Milvus as entities. Each entity has one vector ID field and one vector field. Milvus v1.0 supports integer vector IDs only. When creating a collection within Milvus, vector IDs can be automatically generated or manually defined. Milvus ensures auto-generated vector IDs are unique however, manually defined IDs can be duplicated within Milvus. If manually defining IDs, users are responsible for making sure all IDs are unique.\n\n\u003cbr/\u003e\n\n### Partitions\n\nMilvus supports creating partitions in a collection. In situations where data is inserted regularly and historical data isn't significant (e.g., streaming data), partitions can be used to accelerate vector similarity search. One collection can have up to 4,096 partitions. Specifying a vector search within a specific partition narrows the search and may significantly reduce query time, particularly for collections that contain more than a trillion vectors.\n\n\u003cbr/\u003e\n\n### Index algorithm optimizations\n\nMilvus is built on top of multiple widely-adopted index libraries, including Faiss, NMSLIB, and Annoy. Milvus is far more than a basic wrapper for these index libraries. Here are some of the major enhancements that have been made to the underlying libraries:\n\n- IVF index performance optimizations using the Elkan k-means algorithm.\n- FLAT search optimizations.\n- IVF_SQ8H hybrid index support, which can reduce index file sizes by up to 75% without sacrificing data accuracy. IVF_SQ8H is built upon IVF_SQ8, with identical recall but much faster query speed. It was designed specifically for Milvus to harnesses the parallel processing capacity of GPUs, and the potential for synergy between CPU/GPU co-processing.\n- Dynamic instruction set compatibility.\n\n\u003cbr/\u003e\n\n### Search, index building, and other Milvus optimizations\n\nThe following optimizations have been made to Milvus to improve search and index building performance.\n\n- Search performance is optimized in situations when the number of queries (nq) is less than the number of CPU threads.\n- Milvus combines search requests from a client that take the same topK and search parameters.\n- Index building is suspended when search requests come in.\n- Milvus automatically preloads collections to memory at start.\n- Multiple GPU devices can be assigned to accelerate vector similarity search.\n\n\u003cbr/\u003e\n\n### Distance metrics\n\nMilvus is a vector database built to power vector similarity search. The platform was built with MLOps and production level AI applications in mind. Milvus supports a wide range of distance metrics for calculating similarity, such as Euclidean distance (L2), inner product (IP), Jaccard distance, Tanimoto, Hamming distance, superstructure, and substructure. The last two metrics are commonly used in molecular search and AI-powered new drug discovery.\n\n\u003cbr/\u003e\n\n### Logging\n\nMilvus supports log rotation. In the system configuration file, milvus.yaml, you can set the size of a single log file, the number of log files, and log output to stdout.\n\n\u003cbr/\u003e\n\n### Distributed solution\n\nMishards, a Milvus sharding middleware, is the distributed solution for Milvus With one write node and an unlimited number of read nodes, Mishards unleashes the computational potential of server cluster. Its features include request forwarding, read/write splitting, dynamic/horizontal scaling, and more.\n\n\u003cbr/\u003e\n\n### Monitoring\n\nMilvus is compatible with Prometheus, an open-source system monitoring and alerts toolkit. Milvus adds support for Pushgateway in Prometheus, making it possible for Prometheus to acquire short-lived batch metrics. The monitoring and alerts system works as follows:\n\n- The Milvus server pushes customized metrics data to Pushgateway.\n- Pushgateway ensures ephemeral metric data is safely sent to Prometheus.\n- Prometheus continues pulling data from Pushgateway.\n- Alertmanager is used to set the alert threshold for different indicators and send alerts via email or message.\n\n\u003cbr/\u003e\n\n### Metadata management\n\nMilvus uses SQLite for metadata management by default. SQLite is implemented in Milvus and does not require configuration. In a production environment, it is recommended that you use MySQL for metadata management.\n\n\u003cbr/\u003e\n\n### Engage with our open-source community:\n\n- Find or contribute to Milvus on [GitHub](https://github.com/milvus-io/milvus/).\n- Interact with the community via [Slack](https://join.slack.com/t/milvusio/shared_invite/zt-e0u4qu3k-bI2GDNys3ZqX1YCJ9OM~GQ).\n- Connect with us on [Twitter](https://twitter.com/milvusio).\n","title":"What's Inside Milvus 1.0?","metaData":{}},{"id":"2021-04-23-Making-with-Milvus-Detecting-Android-Viruses-in-Real-Time-for-Trend-Micro.md","author":"milvus","desc":"Learn how Milvus is used to mitigate threats to critical data and strengthen cybersecurity with real-time virus detection.","canonicalUrl":"https://zilliz.com/blog/Making-with-Milvus-Detecting-Android-Viruses-in-Real-Time-for-Trend-Micro","date":"2021-04-23T06:46:13.732Z","cover":"https://assets.zilliz.com/blog_Trend_Micro_5c8ba3e2ce.jpg","tags":["Scenarios"],"href":"/blog/2021-04-23-Making-with-Milvus-Detecting-Android-Viruses-in-Real-Time-for-Trend-Micro.md","content":" \n# Making with Milvus: Detecting Android Viruses in Real Time for Trend Micro\nCybersecurity remains a persistent threat to both individuals and businesses, with data privacy concerns increasing for [86% of companies](https://www.getapp.com/resources/annual-data-security-report/) in 2020 and just [23% of consumers](https://merchants.fiserv.com/content/dam/firstdata/us/en/documents/pdf/digital-commerce-cybersecurity-ebook.pdf) believing their personal data is very secure. As malware becomes steadily more omnipresent and sophisticated, a proactive approach to threat detection has become essential. [Trend Micro](https://www.trendmicro.com/en_us/business.html) is a global leader in hybrid cloud security, network defense, small business security, and endpoint security. To protect Android devices from viruses, the company built Trend Micro Mobile Security—a mobile app that compares APKs (Android Application Package) from the Google Play Store to a database of known malware. The virus detection system works as follows:\n\n- External APKs (Android application package) from the Google Play Store are crawled.\n- Known malware is converted into vectors and stored in [Milvus](https://www.milvus.io/docs/v1.0.0/overview.md).\n- New APKs are also converted into vectors, then compared to the malware database using similarity search.\n- If an APK vector is similar to any of the malware vectors, the app provides users with detailed information about the virus and its threat level.\n\nTo work, the system has to perform highly efficient similarity search on massive vector datasets in real time. Initially, Trend Micro used [MySQL](https://www.mysql.com/). However, as its business expanded so did the number of APKs with nefarious code stored in its database. The company’s algorithm team began searching for alternative vector similarity search solutions after quickly outgrowing MySQL.\n\n\u003cbr/\u003e\n\n### Comparing vector similarity search solutions\n\nThere are a number of vector similarity search solutions available, many of which are open source. Although the circumstances vary from project to project, most users benefit from leveraging a vector database built for unstructured data processing and analytics rather than a simple library that requires extensive configuration. Below we compare some popular vector similarity search solutions and explain why Trend Micro chose Milvus.\n\n#### Faiss\n[Faiss](https://ai.facebook.com/tools/faiss/) is a library developed by Facebook AI Research that enables efficient similarity search and clustering of dense vectors. The algorithms it contains search vectors of any size in sets. Faiss is written in C++ with wrappers for Python/numpy, and supports a number of indexes including IndexFlatL2, IndexFlatIP, HNSW, and IVF.\n\nAlthough Faiss is an incredibly useful tool, it has limitations. It only works as a basic algorithm library, not a database for managing vector datasets. Additionally, it does not offer a distributed version, monitoring services, SDKs, or high availability, which are the key features of most cloud-based services. \n\n#### Plug-ins based on Faiss \u0026 other ANN search libraries\nThere are several plug-ins built on top of Faiss, NMSLIB, and other ANN search libraries that are designed to enhance the basic functionality of the underlying tool that powers them. Elasticsearch (ES) is a search engine based on the Lucene library with a number of such plugins. Below is an architecture diagram of an ES plug-in:\n\n\n\n\n\nBuilt in support for distributed systems is a major advantage of an ES solution. This saves developers time and companies money thanks to code that doesn’t have to be written. ES plug-ins are technically advanced and prevalent. Elasticsearch provides a QueryDSL (domain-specific language), which defines queries based on JSON and is easy to grasp. A full set of ES services makes it possible to conduct vector/text search and filter scalar data simultaneously.\n\nAmazon, Alibaba, and Netease are a few large tech companies that currently rely on Elasticsearch plug-ins for vector similarity search. The primary downsides with this solution are high memory consumption and no support for performance tuning. In contrast, [JD.com](http://jd.com/) has developed its own distributed solution based on Faiss called [Vearch](https://github.com/vearch/vearch). However, Vearch is still an incubation-stage project and its open-source community is relatively inactive.\n\n#### Milvus \n[Milvus](https://www.milvus.io/) is an open-source vector database created by [Zilliz](https://zilliz.com). It is highly flexible, reliable, and blazing fast. By encapsulating multiple widely adopted index libraries, such as Faiss, NMSLIB, and Annoy, Milvus provides a comprehensive set of intuitive APIs, allowing developers to choose the ideal index type for their scenario. It also provides distributed solutions and monitoring services. Milvus has a highly active open-source community and over 5.5K stars on [Github](https://github.com/milvus-io/milvus).\n\n#### Milvus bests the competition\nWe compiled a number of different test results from the various vector similarity search solutions mentioned above. As we can see in the following comparison table, Milvus was significantly faster than the competition despite being tested on a dataset of 1 billion 128-dimensional vectors.\n\n| **Engine** | **Performance (ms)** | **Dataset Size (million)** |\n| :---- | :---- | :---- |\n| ES | 600 | 1 |\n| ES + Alibaba Cloud | 900 | 20 |\n| Milvus | 27 | 1000+ |\n| SPTAG | Not good | |\n| ES + nmslib, faiss | 90 | 150 |\n\n###### *A comparison of vector similarity search solutions.*\n\nAfter weighing the pros and cons of each solution, Trend Micro settled on Milvus for its vector retrieval model. With exceptional performance on massive, billion-scale datasets, it's obvious why the company chose Milvus for a mobile security service that requires real-time vector similarity search.\n\n\u003cbr/\u003e\n\n### Designing a system for real-time virus detection\nTrend Micro has more than 10 million malicious APKs stored in its MySQL database, with 100k new APKs added each day. The system works by extracting and calculating Thash values of different components of an APK file, then uses the Sha256 algorithm to transform it into binary files and generate 256-bit Sha256 values that differentiate the APK from others. Since Sha256 values vary with APK files, one APK can have one combined Thash value and one unique Sha256 value.\n\nSha256 values are only used to differentiate APKs, and Thash values are used for vector similarity retrieval. Similar APKs may have the same Thash values but different Sha256 values.\n\nTo detect APKs with nefarious code, Trend Micro developed its own system for retrieving similar Thash values and corresponding Sha256 values. Trend Micro chose Milvus to conduct instantaneous vector similarity search on massive vector datasets converted from Thash values. After similarity search is run, the corresponding Sha256 values are queried in MySQL. A Redis caching layer is also added to the architecture to map Thash values to Sha256 values, significantly reducing query time.\n\nBelow is the architecture diagram of Trend Micro’s mobile security system.\n\n\n\n\n\u003cbr/\u003e\n\nChoosing an appropriate distance metric helps improve vector classification and clustering performance. The following table shows the [distance metrics](https://www.milvus.io/docs/v1.0.0/metric.md#binary) and the corresponding indexes that work with binary vectors. \n\n| **Distance Metrics** | **Index Types** |\n| ---- | ---- |\n| - Jaccard \u003cbr/\u003e - Tanimoto \u003cbr/\u003e - Hamming | - FLAT \u003cbr/\u003e - IVF_FLAT |\n| - Superstructure \u003cbr/\u003e - Substructure | FLAT |\n\n###### *Distance metrics and indexes for binary vectors.*\n\n\u003cbr/\u003e\n\nTrend Micro converts Thash values into binary vectors and stores them in Milvus. For this scenario, Trend Micro is using Hamming distance to compare vectors.\n\nMilvus will soon support string vector ID, and integer IDs won’t have to be mapped to the corresponding name in string format. This makes the Redis caching layer unnecessary and the system architecture less bulky.\n\nTrend Micro adopts a cloud-based solution and deploys many tasks on [Kubernetes](https://kubernetes.io/). To achieve high availability, Trend Micro uses [Mishards](https://www.milvus.io/docs/v1.0.0/mishards.md), a Milvus cluster sharding middleware developed in Python. \n\n\n\n\n\u003cbr/\u003e\n\nTrend Micro separates storage and distance calculation by storing all vectors in the [EFS](https://aws.amazon.com/efs/) (Elastic File System) provided by [AWS](https://aws.amazon.com/). This practice is a popular trend in the industry. Kubernetes is used to start multiple reading nodes, and develops LoadBalancer services on these reading nodes to ensure high availability.\n\nTo maintain data consistency Mishards supports just one writing node. However, a distributed version of Milvus with support for multiple writing nodes will be available in the coming months. \n\n\u003cbr/\u003e\n\n### Monitoring and Alert Functions\nMilvus is compatible with monitoring systems built on [Prometheus](https://prometheus.io/), and uses [Grafana](https://grafana.com/), an open-source platform for time-series analytics, to visualize various performance metrics.\n\nPrometheus monitors and stores the following metrics:\n\n- Milvus performance metrics including insertion speed, query speed, and Milvus uptime. \n- System performance metrics including CPU/GPU usage, network traffic, and disk access speed.\n- Hardware storage metrics including data size and total file number.\n\nThe monitoring and alert system works as follows: \n\n- A Milvus client pushes customized metrics data to Pushgateway.\n- The Pushgateway ensures short-lived, ephemeral metric data is safely sent to Prometheus.\n- Prometheus keeps pulling data from Pushgateway. \n- Alertmanager sets the alert threshold for different metrics and raises alarms through emails or messages.\n\n\u003cbr/\u003e\n\n### System Performance\nA couple months have passed since the ThashSearch service built on Milvus was first launched. The graph below shows that end-to-end query latency is less than 95 milliseconds.\n\n\n\n\n\u003cbr/\u003e\n\nInsertion is also fast. It takes around 10 seconds to insert 3 million 192-dimensional vectors. With help from Milvus, the system performance was able to meet the performance criteria set by Trend Micro. \n\n\u003cbr/\u003e\n\n### Don’t be a stranger\n- Find or contribute to Milvus on [GitHub](https://github.com/milvus-io/milvus/).\n- Interact with the community via [Slack](https://join.slack.com/t/milvusio/shared_invite/zt-e0u4qu3k-bI2GDNys3ZqX1YCJ9OM~GQ).\n- Connect with us on [Twitter](https://twitter.com/milvusio).\n\n\n\n\n\n\n\n\n \n","title":"Making with Milvus Detecting Android Viruses in Real Time for Trend Micro","metaData":{}},{"id":"2021-04-19-build-semantic-search-at-speed-milvus-lucidworks.md","author":"Elizabeth Edmiston","desc":"Learn more about using semantic machine learning methodologies to power more relevant search results across your organization.","canonicalUrl":"https://zilliz.com/blog/build-semantic-search-at-speed-milvus-lucidworks","date":"2021-04-19T07:32:50.416Z","cover":"https://assets.zilliz.com/lucidworks_4753c98727.png","tags":["Scenarios"],"href":"/blog/2021-04-19-build-semantic-search-at-speed-milvus-lucidworks.md","content":"\n# Build Semantic Search at Speed\n\n[Semantic search](https://lucidworks.com/post/what-is-semantic-search/) is a great tool to help your customers—or your employees—find the right products or information. It can even surface difficult-to-index information for better results. That said, if your semantic methodologies aren’t being deployed to work fast, they won’t do you any good. The customer or employee isn’t just going to sit around while the system takes its time responding to their query—and a thousand others are likely being ingested at the same time.\n\nHow can you make semantic search fast? Slow semantic search isn’t going to cut it.\n\nFortunately, this is the kind of problem Lucidworks loves to solve. We recently tested a modest-sized cluster—read on for more details—that resulted in 1500 RPS (requests per second) against a collection of over one million documents, with an average response time of roughly 40 milliseconds. Now that’s some serious speed.\n\n\u003cbr/\u003e\n\n### Implementing Semantic Search\n\nTo make lightning-fast, machine learning magic happen, Lucidworks has implemented semantic search using the semantic vector search approach. There are two critical parts.\n\n\u003cbr/\u003e\n\n#### Part One: The Machine Learning Model\n\nFirst, you need a way to encode text into a numerical vector. The text could be a product description, a user search query, a question, or even an answer to a question. A semantic search model is trained to encode text such that text that is semantically similar to other text is encoded into vectors that are numerically “close” to one another. This encoding step needs to be fast in order to support the thousand or more possible customer searches or user queries coming in every second.\n\n\u003cbr/\u003e\n\n#### Part Two: The Vector Search Engine\n\nSecond, you need a way to quickly find the best matches to the customer search or user query. The model will have encoded that text into a numerical vector. From there, you need to compare that to all the numerical vectors in your catalog or lists of questions and answers to find those best matches—the vectors that are “closest” to the query vector. For that, you will need a vector engine that can handle all of that information effectively and at lightning speed. The engine could contain millions of vectors and you really just want the best twenty or so matches to your query. And of course, it needs to handle a thousand or so such queries every second.\n\nTo tackle these challenges, we added the vector search engine [Milvus](https://doc.lucidworks.com/fusion/5.3/8821/milvus) in our [Fusion 5.3 release](https://lucidworks.com/post/enhance-personalization-efforts-with-new-features-in-fusion/). Milvus is open-source software and it is fast. Milvus uses FAISS ([Facebook AI Similarity Search](https://ai.facebook.com/tools/faiss/)), the same technology Facebook uses in production for its own machine learning initiatives. When needed, it can run even faster on [GPU](https://en.wikipedia.org/wiki/Graphics_processing_unit). When Fusion 5.3 (or higher) is installed with the machine learning component, Milvus is automatically installed as part of that component so you can turn on all of these capabilities with ease.\n\nThe size of the vectors in a given collection, specified when the collection is created, depends on the model that produces those vectors. For example, a given collection could store the vectors created from encoding (via a model) all of the product descriptions in a product catalog. Without a vector search engine like Milvus, similarity searches would not be feasible across the entire vector space. So, similarity searches would have to be limited to pre-selected candidates from the vector space (for example, 500) and would have both slower performance and lower quality results. Milvus can store hundreds of billions of vectors across multiple collections of vectors to ensure that search is fast and results are relevant.\n\n\u003cbr/\u003e\n\n### Using Semantic Search\n\nLet’s get back to the semantic search workflow, now that we’ve learned a little about why Milvus might be so important. Semantic search has three stages. During the first stage, the machine learning model is loaded and/or trained. Afterwards, data is indexed into Milvus and Solr. The final stage is the query stage, when the actual search occurs. We’ll focus on those last two stages below.\n\n\u003cbr/\u003e\n\n### Indexing into Milvus\n\n\n\n\nAs shown in the above diagram, the query stage begins similarly to the indexing stage, just with queries coming in instead of documents. For each query:\n\n1. The query is sent to the [Smart Answers](https://lucidworks.com/products/smart-answers/) index pipeline.\n2. The query is then sent to the ML model.\n3. The ML model returns a numeric vector (encrypted from the query). Again, the type of model determines the size of the vector.\n4. The vector is sent to Milvus, which then determines which vectors, in the specified Milvus collection, best match the provided vector.\n5. Milvus returns a list of unique IDs and distances corresponding to the vectors determined in step four.\n6. A query containing those IDs and distances is sent to Solr.\n7. Solr then returns an ordered list of the documents associated with those IDs.\n\n\u003cbr/\u003e\n\n### Scale Testing\n\nIn order to prove that our semantic search flows are running at the efficiency we require for our customers, we run scale tests using Gatling scripts on the Google Cloud Platform using a Fusion cluster with eight replicas of the ML model, eight replicas of the query service, and a single instance of Milvus. Tests were run using the Milvus FLAT and HNSW indexes. The FLAT index has 100% recall, but is less efficient – except when the datasets are small. The HNSW (Hierarchical Small World Graph) index still has high quality results and it has improved performance on larger datasets.\n\nLet’s jump into some numbers from a recent example we ran:\n\n\n\n\n\n\n\n\n\n\n\u003cbr/\u003e\n\n### Getting Started\n\nThe [Smart Answers](https://lucidworks.com/products/smart-answers/) pipelines are designed to be easy-to-use. Lucidworks has [pre-trained models that are easy-to-deploy](https://doc.lucidworks.com/how-to/734/set-up-a-pre-trained-cold-start-model-for-smart-answers) and generally have good results—though training your own models, in tandem with pre-trained models, will offer the best results. Contact us today to learn how you can implement these initiatives into your search tools to power more effective and delightful results.\n\n\u003e This blog is reposted from: https://lucidworks.com/post/how-to-build-fast-semantic-search/?utm_campaign=Oktopost-Blog+Posts\u0026utm_medium=organic_social\u0026utm_source=linkedin\n","title":"Build Semantic Search at Speed","metaData":{}},{"id":"2021-04-15-Milvus-V1.0-is-coming.md","author":"milvus","desc":"Today we proudly announce the release of Milvus V1.0.","origin":null,"date":"2021-04-15T00:00:00.000Z","cover":"https://assets.zilliz.com/Milvus_510cf50aee.jpeg","tags":["Engineering"],"href":"/blog/2021-04-15-Milvus-V1.0-is-coming.md","content":"\n# Milvus V1.0 is coming\n\nToday we proudly announce the release of Milvus v1.0. After 8 months of painstaking tests and trials by hundreds of Milvus community users, Milvus v0.10.x finally became stable, and it’s now time to release Milvus v1.0 based on Milvus v0.10.6.\n\nMilvus v1.0 brings with it the following features:\n\n- Support for mainstream similarity metrics, including Euclidean distance, inner product, Hamming distance, Jaccard coefficient, and more.\n- Integration with and improvements to SOTA ANNs algorithms, including Faiss, Hnswlib, Annoy, NSG, and more.\n- Scale-out capability through the Mishards sharding proxy.\n- Support for processors commonly used in AI scenarios, including X86, Nvidia GPU, Xilinx FPGA, and more.\n\nSee the [Release Notes](https://www.milvus.io/docs/v1.0.0/release_notes.md) for more of the v1.0 features.\n\nMilvus is an ongoing Open-Source Software (OSS) project. Still, we believe the first major release is of crucial importance to our community users for the following reasons:\n\n- Milvus v1.0 will be supported for the long term.\n- A stable version of Milvus can be readily integrated into the AI ecosystem.\n- Milvus is now well structured to move to the next stage.\n\n\u003cbr/\u003e\n\n### Long-term support\n\nMilvus v1.0 is our first Long-Term Support (LTS) version. The Milvus community will provide bug fix support for Milvus v1.0 till December 31st, 2024 (sponsored by Zilliz). New features will be available only in releases subsequent to v1.0.\n\nSee [The Milvus Release Guideline](https://www.milvus.io/docs/v1.0.0/milvus_release_guideline.md) for more information about the release strategy of Milvus.\n\n\u003cbr/\u003e\n\n### Toolchain and AI Ecosystem Integration\n\nWhile the development of the Milvus engine is rapidly iterating, we have not spent much time on the toolchain of Milvus. As of v1.0, we plan on developing necessary tooling and utilities for the Milvus users. Please find more details in [The Toolchain SIG](https://www.milvus.io/docs/v1.0.0/sig_tool.md).\n\nA stable version makes integration with the AI ecosystem a breeze. Now, we are looking for more collaboration between the Milvus community and other AI OSS communities. We also encourage support for new AI ASICs in Milvus.\n\n\u003cbr/\u003e\n\n### The Future of Milvus\n\nWe believe a bright future of Milvus lies with the following factors.\n\n- Active contribution from the developers in the Milvus community.\n- Ability to integrate with any cloud-native environment.\n\nTo continuously nurture and advance the Milvus community, we have drawn up our [community charters](https://www.milvus.io/docs/v1.0.0/milvus_community_charters.md), whereby several technical decisions have been made to attract more participants into the community.\n\n- We will switch to Golang for the development of the Milvus engine, while the ANNS algorithm component will still be developed in C++.\n- Moving forward, the distributed/cluster/cloud Milvus will use the existing cloud components as much as possible.\n\nLet’s work together to build the next-generation cloud data fabric made for AI!\n","title":"Milvus V1.0 is coming","metaData":{}},{"id":"2021-04-08-AI-applications-with-Milvus.md","author":"milvus","desc":"Milvus accelerates machine learning application development and machine learning operations (MLOps). With Milvus, you can rapidly develop a minimum viable product (MVP) while keeping costs at lower limits.","canonicalUrl":"https://zilliz.com/blog/AI-applications-with-Milvus","date":"2021-04-08T04:14:03.700Z","cover":"https://assets.zilliz.com/blog_cover_4a9807b9e0.png","tags":["Scenarios"],"href":"/blog/2021-04-08-AI-applications-with-Milvus.md","content":" \n# How to Make 4 Popular AI Applications with Milvus\n\n\n[Milvus](https://milvus.io/) is an open-source vector database. It supports adding, deleting, updating, and near real-time search of massive vector datasets created by extracting feature vectors from unstructured data using AI models. With a comprehensive set of intuitive APIs, and support for multiple widely adopted index libraries (e.g., Faiss, NMSLIB, and Annoy), Milvus accelerates machine learning application development and machine learning operations (MLOps). With Milvus, you can rapidly develop a minimum viable product (MVP) while keeping costs at lower limits.\n\n\"What resources are available for developing an AI application with Milvus?” is commonly asked in the Milvus community. Zilliz, the [company](https://zilliz.com/) behind Milvus, developed a number of demos that leverage Milvus to conduct lightening-fast similarity search that powers intelligent applications. Source code of Milvus solutions can be found at [zilliz-bootcamp](https://github.com/zilliz-bootcamp). The following interactive scenarios demonstrate natural language processing (NLP), reverse image search, audio search, and computer vision.\n\n\nFeel free to try out the solutions to gain some hands-on experience with specific scenarios! Share your own application scenarios via: \n- [Slack](https://join.slack.com/t/milvusio/shared_invite/zt-e0u4qu3k-bI2GDNys3ZqX1YCJ9OM~GQ)\n- [GitHub](https://github.com/milvus-io/milvus/discussions)\n\n\u003cbr/\u003e\n\n**Jump to:**\n\n- [Natural language processing (chatbots)](#natural-language-processing-chatbots)\n- [Reverse image search](#reverse-image-search-systems)\n- [Audio search](#audio-search-systems)\n- [Video object detection (computer vision)](#video-object-detection-computer-vision)\n\n\u003cbr/\u003e\n\n### Natural language processing (chatbots)\nMilvus can be used to build chatbots that use natural language processing to simulate a live operator, answer questions, route users to relevant information, and reduce labor costs. To demonstrate this application scenario, Zilliz built an AI-powered chatbot that understands semantic language by combining Milvus with [BERT](https://en.wikipedia.org/wiki/BERT_(language_model)), a machine learning (ML) model developed for NLP pre-training.\n\n👉Source code:[zilliz-bootcamp/intelligent_question_answering_v2](https://github.com/zilliz-bootcamp/intelligent_question_answering_v2)\n\n\n\n#### How to use\n\n1. Upload a dataset that includes question-answer pairs. Format questions and answers in two separate columns. Alternatively, a [sample dataset](https://zilliz.com/solutions/qa) is available for download.\n\n2. After typing in your question, a list of similar questions will be retrieved from the uploaded dataset.\n\n3. Reveal the answer by selecting the question most similar to your own.\n\n👉Video:[[Demo] QA System Powered by Milvus](https://www.youtube.com/watch?v=ANgoyvgAxgU)\n\n#### How it works\n\nQuestions are converted into feature vectors using Google’s BERT model, then Milvus is used to manage and query the dataset.\n\n**Data processing:**\n\n1. BERT is used to convert the uploaded question-answer pairs into 768-dimensional feature vectors. The vectors are then imported to Milvus and assigned individual IDs.\n2. Question, and corresponding answer, vector IDs are stored in PostgreSQL.\n\n**Searching for similar questions:**\n\n1. BERT is used to extract feature vectors from a user's input question.\n2. Milvus retrieves vector IDs for questions that are most similar to the input question.\n3. The system looks up the corresponding answers in PostgreSQL.\n\n\u003cbr/\u003e\n\n### Reverse image search systems\nReverse image search is transforming e-commerce through personalized product recommendations and similar product lookup tools that can boost sales. In this application scenario, Zilliz built a reverse image search system by combining Milvus with [VGG](https://towardsdatascience.com/how-to-use-a-pre-trained-model-vgg-for-image-classification-8dd7c4a4a517), an ML model that can extract image features.\n\n👉Source code:[zilliz-bootcamp/image_search](https://github.com/zilliz-bootcamp/image_search)\n\n\n\n\n#### How to use\n1. Upload a zipped image dataset comprised of .jpg images only (other image file types are not accepted). Alternatively, a [sample dataset](https://zilliz.com/solutions/image-search) is available for download.\n2. Upload an image to use as the search input for finding similar images.\n\n👉Video: [[Demo] Image Search Powered by Milvus](https://www.youtube.com/watch?v=mTO8YdQObKY)\n\n#### How it works\nImages are converted into 512-dimensional feature vectors using the VGG model, then Milvus is used to manage and query the dataset.\n\n**Data processing:**\n\n1. The VGG model is used to convert the uploaded image dataset to feature vectors. The vectors are then imported to Milvus and assigned individual IDs.\n2. Image feature vectors, and corresponding image file paths, are stored in CacheDB.\n\n**Searching for similar images:**\n\n1. VGG is used to convert a user’s uploaded image into feature vectors.\n2. Vector IDs of images most similar to the input image are retrieved from Milvus.\n3. The system looks up the corresponding image file paths in CacheDB.\n\n\u003cbr/\u003e\n\n### Audio search systems\nSpeech, music, sound effects, and other types of audio search makes it possible to quickly query massive volumes of audio data and surface similar sounds. Applications include identifying similar sound effects, minimizing IP infringement, and more. To demonstrate this application scenario, Zilliz built a highly efficient audio similarity search system by combining Milvus with [PANNs](https://arxiv.org/abs/1912.10211)—a large-scale pretrained audio neural networks built for audio pattern recognition.\n\n👉Source code:[zilliz-bootcamp/audio_search](https://github.com/zilliz-bootcamp/audio_search)\n\n\n#### How to use\n1. Upload a zipped audio dataset comprised of .wav files only (other audio file types are not accepted). Alternatively, a [sample dataset](https://zilliz.com/solutions/audio-search) is available for download.\n2. Upload a .wav file to use as the search input for finding similar audio.\n\n👉Video: [[Demo] Audio Search Powered by Milvus](https://www.youtube.com/watch?v=0eQHeqriCXw)\n#### How it works\nAudio is converted into feature vectors using PANNs, large-scale pre-trained audio neural networks built for audio pattern recognition. Then Milvus is used to manage and query the dataset.\n\n**Data processing:**\n\n1. PANNs converts audio from the uploaded dataset to feature vectors. The vectors are then imported to Milvus and assigned individual IDs.\n2. Audio feature vector IDs and their corresponding .wav file paths are stored in PostgreSQL.\n\n**Searching for similar audio:**\n\n1. PANNs is used to convert a user’s uploaded audio file into feature vectors.\n2. Vector IDs of audio most similar to the uploaded file are retrieved from Milvus by calculating inner product (IP) distance.\n3. The system looks up the corresponding audio file paths in MySQL.\n\n\u003cbr/\u003e\n\n### Video object detection (computer vision)\nVideo object detection has applications in computer vision, image retrieval, autonomous driving, and more. To demonstrate this application scenario, Zilliz built a video object detection system by combining Milvus with technologies and algorithms including [OpenCV](https://en.wikipedia.org/wiki/OpenCV), [YOLOv3](https://towardsdatascience.com/yolo-v3-object-detection-53fb7d3bfe6b), and [ResNet50](https://www.mathworks.com/help/deeplearning/ref/resnet50.html).\n\n👉Source code: [zilliz-bootcamp/video_analysis](https://github.com/zilliz-bootcamp/video_analysis)\n\n\n\n\n#### How to use\n1. Upload a zipped image dataset comprised of .jpg files only (other image file types are not accepted). Ensure that each image file is named by the object it depicts. Alternatively, a [sample dataset](https://zilliz.com/solutions/video-obj-analysis) is available for download. \n2. Upload a video to use for analysis.\n3. Click the play button to view the uploaded video with object detection results shown in real time.\n\n👉Video: [[Demo] Video Object Detection System Powered by Milvus](https://www.youtube.com/watch?v=m9rosLClByc)\n\n#### How it works\nObject images are converted into 2048-dimensional feature vectors using ResNet50. Then Milvus is used to manage and query the dataset.\n\n**Data processing:**\n\n1. ResNet50 converts object images to 2048-dimensional feature vectors. The vectors are then imported to Milvus and assigned individual IDs.\n2. Audio feature vector IDs and their corresponding image file paths are stored in MySQL.\n\n**Detecting objects in video:**\n\n1. OpenCV is used to trim the video.\n2. YOLOv3 is used to detect objects in the video.\n3. ResNet50 converts detected object images into 2048-dimensional feature vectors.\n\nMilvus searches for the most similar object images in the uploaded dataset. Corresponding object names and image file paths are retrieved from MySQL.\n\n\n\n ","title":"How to Make 4 Popular AI Applications with Milvus","metaData":{}},{"id":"2021-03-31-Operationalize-AI-at-Scale-with-Software-MLOps-and-Milvus.md","author":"milvus","desc":"MLOps is superseding DevOps as we make the transition to Software 2.0. Find what model operations is, and how the open-source vector database Milvus supports it.","canonicalUrl":"https://zilliz.com/blog/Operationalize-AI-at-Scale-with-Software-MLOps-and-Milvus","date":"2021-03-31T09:51:38.653Z","cover":"https://assets.zilliz.com/milvus_5b2cdec665.jpg","tags":["Engineering"],"href":"/blog/2021-03-31-Operationalize-AI-at-Scale-with-Software-MLOps-and-Milvus.md","content":"\n# Operationalize AI at Scale with Software 2.0, MLOps, and Milvus\n\nBuilding machine learning (ML) applications is a complex and iterative process. As more companies realize the untapped potential of unstructured data, the demand for [AI-powered data processing and analytics](https://milvus.io/blog/Thanks-to-Milvus-Anyone-Can-Build-a-Vector-Database-for-1-Billion-Images.md) will continue to rise. Without effective machine learning operations, or MLOps, most ML application investments will wither on the vine. Research has found that [as little as 5%](https://www.forbes.com/sites/cognitiveworld/2020/03/31/modelops-is-the-key-to-enterprise-ai/?sh=44c0f5066f5a) of the AI adoptions companies plan to deploy actually reach deployment. Many organizations incur \"model debt,\" where changes in market conditions, and failure to adapt to them, result in unrealized investments in models that linger unrefreshed (or worse, never get deployed at all).\n\nThis article explains MLOps, a systemic approach to AI model life cycle management, and how the open-source vector data management platform [Milvus](https://milvus.io/) can be used to operationalize AI at scale.\n\n\u003cbr/\u003e\n\n### What is MLOps?\n\nMachine learning operations (MLOps), also known as model operations (ModelOps) or AI model operationalization, is necessary to build, maintain, and deploy AI applications at scale. As companies seek to apply the AI models they develop to hundreds of different scenarios, it is mission critical that models in use, and those under development, are operationalized across the entire organization. MLOps involves monitoring a machine learning model throughout its lifecycle, and governing everything from underlying data to the effectiveness of a production system that relies on a particular model.\n\n\n\n\n\nGartner [defines](https://www.gartner.com/en/information-technology/glossary/modelops) ModelOps as the governance and life cycle management of a wide range of operationalized artificial intelligence and decision models. The core functionality of MLOps can be broken down as follows:\n\n- **Continuous integration/continuous delivery (CI/CD):** A set of best practices borrowed from developer operations (DevOps), CI/CD is a method for delivering code changes more frequently and more reliably. [Continuous integration](https://www.gartner.com/en/information-technology/glossary/continuous-integration-ci) promotes implementing code changes in small batches while monitoring them with strict version control. [Continuous delivery](https://www.gartner.com/smarterwithgartner/5-steps-to-master-continuous-delivery/) automates the delivery of applications to various environments (e.g., testing and development environments).\n\n- **Model development environments (MDE):** A complex process for building, reviewing, documenting, and examining models, MDE help ensure models are created iteratively, documented as they are developed, trusted, and reproducible. Effective MDE ensures models can be explored, researched, and experimented on in a controlled manner.\n\n- **Champion-challenger testing:** Similar to A/B testing methodology used by marketers, [champion-challenger testing](https://medium.com/decision-automation/what-is-champion-challenger-and-how-does-it-enable-choosing-the-right-decision-f57b8b653149) involves experimenting with different solutions to aid the decision-making process that proceeds committing to a single approach. This technique involves monitoring and measuring performance in real time to identify which deviation works best.\n\n- **Model versioning:** As with any complex system, machine learning models are developed in steps by many different people—resulting in data management questions around versions of data and ML models. Model versioning helps manage and govern the iterative process of ML development where data, models, and code may evolve at different rates.\n\n- **Model store and rollback:** When a model is deployed, its corresponding image file must be stored. Rollback and recovery abilities allow MLOps teams to revert to a previous model version if needed.\n\nUsing just one model in a production application presents a number of difficult challenges. MLOps is a structured, repeatable method that relies on tools, technology, and best practices to overcome technical or business problems that arise during a machine learning model’s life cycle. Successful MLOps maintains efficiency across teams working to build, deploy, monitor, retrain, and govern AI models and their use in production systems.\n\n\u003cbr/\u003e\n\n### Why is MLOps necessary?\n\nAs depicted in the ML model life cycle above, building a machine learning model is an iterative process that involves incorporating new data, retraining models, and dealing with general model decay over time. These are all issues that traditional developer operations, or DevOps, does not address or provide solutions for. MLOps has become necessary as a way to manage investment in AI models and ensure a productive model life cycle. Because machine learning models will be leveraged by a variety of different production systems, MLOps becomes integral to making sure requirements can be met across different environments and amid varying scenarios.\n\n\n\n\n\u003cbr/\u003e\n\nThe simple illustration above depicts a machine learning model being deployed in a cloud environment that feeds into an application. In this basic scenario, a number of problems could arise that MLOps helps overcome. Because the production application relies on a specific cloud environment, there are latency requirements that the data scientists who developed the ML model don’t have access to. Operationalizing the model life cycle would make it possible for data scientists or engineers with deep knowledge of the model to identify and troubleshoot problems that arise in specific production environments.\n\nNot only are machine learning models trained in different environments from the production applications they are used in, but they also often rely on historical datasets that differ from the data used in production applications. With MLOps the entire data science team, from those developing the model to people working at the application level, have a means of sharing and requesting information and assistance. The rate at which data and markets change make it imperative that there is as little friction as possible between all key stakeholders and contributors that will come to depend on a given machine learning model.\n\n### Supporting the transition to Software 2.0\n\n[Software 2.0](https://karpathy.medium.com/software-2-0-a64152b37c35) is the idea that software development will experience a paradigm shift as artificial intelligence increasingly plays a central role in writing AI models that power software applications. Under Software 1.0, development involves programmers writing explicit instructions using a specific programming language (e.g., Python, C++). Software 2.0 is far more abstract. Although people provide input data and set parameters, neural networks are difficult for humans to understand due to their sheer complexity— with typical networks containing millions of weights that impact outcomes (and sometimes billions or trillions).\n\nDevOps was built around Software 1.0’s reliance on specific instructions dictated by programmers using languages, but never considered the life cycle of a machine learning model that powers a variety of different applications. MLOps addresses the need for the process of managing software development to change alongside the software under development. As Software 2.0 becomes the new standard for computer-based problem solving, having the right tools and processes for managing model life cycles will make or break investments in new technology. Milvus is an open-source vector similarity search engine built to support the transition to Software 2.0 and manage model life cycles with MLOps.\n\n\n\n\n\u003cbr/\u003e\n\n### Operationalizing AI at scale with Milvus\n\nMilvus is a vector data management platform that was made specifically for storing, querying, updating, and maintaining massive, trillion-scale vector datasets. The platform powers vector similarity search and can integrate with widely adopted index libraries, including Faiss, NMSLIB, and Annoy. By pairing AI models that convert unstructured data to vectors with Milvus, applications spanning new drug development, biometric analysis, recommendation systems and much more can be created.\n\n[Vector similarity search](https://blog.milvus.io/vector-similarity-search-hides-in-plain-view-654f8152f8ab) is the go-to solution for unstructured data data processing and analytics, and vector data is quickly emerging as a core data type. A comprehensive data management system like Milvus facilitates operationalizing AI in many ways, including:\n\n- Providing an environment for model training that ensures more aspects of development are done in one place, facilitating cross team collaboration, model governance, and more.\n\n- Offering a comprehensive set of APIs that support popular frameworks such as Python, Java, and Go, making it easy to integrate a common set of ML models.\n\n- Compatibility with Google Colaboratory, a Jupyter notebook environment that runs in a browser, simplifies the process of compiling Milvus from source code and running basic Python operations.\n\n- Automated machine learning (AutoML) functionality makes it possible to automate the tasks associated with applying machine learning to real world problems. Not only does AutoML lead to efficiency improvements, but it makes it possible for non-experts to take advantage of machine learning models and techniques.\n\nRegardless of the machine learning applications you’re building today, or the plans you have for applications in the future, Milvus is a flexible data management platform created with Software 2.0 and MLOps in mind. To learn more about Milvus or make contributions, find the project on [Github](https://github.com/milvus-io). To get involved with the community or ask questions, join our [Slack](https://join.slack.com/t/milvusio/shared_invite/zt-e0u4qu3k-bI2GDNys3ZqX1YCJ9OM~GQ) channel. Hungry for more content? Check out the following resources:\n\n- [Milvus Is an Open-Source Scalable Vector Database](https://milvus.io/blog/Milvus-Is-an-Open-Source-Scalable-Vector-Database.md)\n- [Milvus Was Built for Massive-Scale (Think Trillion) Vector Similarity Search](https://milvus.io/blog/Milvus-Was-Built-for-Massive-Scale-Think-Trillion-Vector-Similarity-Search.md)\n- [Set Up Milvus in Google Colaboratory for Easy ML Application Building](https://milvus.io/blog/Set-Up-Milvus-in-Google-Colaboratory-for-Easy-ML-Application-Building.md)\n","title":"Operationalize AI at Scale with Software 2.0, MLOps, and Milvus","metaData":{}},{"id":"2021-03-18-Making-With-Milvus-AI-Infused-Proptech-for-Personalized-Real-Estate-Search.md","author":"milvus","desc":"AI is transforming the real estate industry, discover how intelligent proptech accelerates the home search and purchase process.","canonicalUrl":"https://zilliz.com/blog/Making-With-Milvus-AI-Infused-Proptech-for-Personalized-Real-Estate-Search","date":"2021-03-18T03:53:54.736Z","cover":"https://assets.zilliz.com/blog_realistate_search_da4e8ee01d.jpg","tags":["Scenarios"],"href":"/blog/2021-03-18-Making-With-Milvus-AI-Infused-Proptech-for-Personalized-Real-Estate-Search.md","content":" \n# Making With Milvus: AI-Infused Proptech for Personalized Real Estate Search\nArtificial intelligence (AI) has [powerful applications](https://medium.com/unstructured-data-service/the-easiest-way-to-search-among-1-billion-image-vectors-d6faf72e361f#d62b) in real estate that are transforming the home search process. Tech savvy real estate professionals have been taking advantage of AI for years, recognizing its ability to help clients find the right home faster and simplify the process of purchasing property. The coronavirus pandemic has [accelerated](https://www.pwc.com/ca/en/industries/real-estate/emerging-trends-in-real-estate-2021/shifting-landscape-proptech.html) interest, adoption, and investement in property technology (or proptech) worldwide, suggesting it will play an increasingly greater role in the real estate industry moving forward. \n\nThis article explores how [Beike](https://bj.ke.com/) used vector similarity search to build a house hunting platform that provides personalized results and recommends listings in near real-time.\n\n### What is vector similarity search?\n\n[Vector similarity search](https://medium.com/unstructured-data-service/vector-similarity-search-hides-in-plain-view-654f8152f8ab) has applications spanning a wide variety of artificial intelligence, deep learning, and traditional vector calculation scenarios. The proliferation of AI technology is in part attributed to vector search and its ability to make sense of unstructured data, which includes things like images, video, audio, behavior data, documents, and much more.\n\nUnstructured data makes up an estimated 80-90% of all data, and extracting insights from it is quickly becoming a requrement for businesses that want to remain competitive in an ever-changing world. Increasing demand for unstructured data analytics, rising compute power, and declining compute costs have made AI-enabled vector search more accessible than ever.\n\n\n\n\nTraditionally, unstructured data has been a challenge to process and analyze at scale because it doesn't follow a predefined model or organizational structure. Neural networks (e.g., CNN, RNN, and BERT) make it possible to convert unstructured data into feature vectors, a numerical data format that can be easily interpreted by computers. Algorithms are then used to calculate similarity between vectors using metrics like cosine similarity or Euclidean distance.\n\nUltimately, vector similarity search is a broad term that desribes techniques for identifying similar things in massive datasets. Beike uses this technology to power an intelligent home search engine that automatically recommends listings based on individual user preferences, search history, and property criteria—accelerating the real estate search and purchase process. Milvus is an open-source vector database that connects information with algorithms, enabling Beike to develop and manage its AI real estate platform.\n\n\u003cbr/\u003e\n\n### How does Milvus manage vector data?\n\nMilvus was built specifically for large-scale vector data management, and has applications spanning image and video search, chemical similarity analysis, personalized recommendation systems, conversational AI, and much more. Vector datasets stored in Milvus can be efficiently queried, with most implementations following this general process:\n\n\n\n\u003cbr/\u003e\n\n### How does Beike use Milvus to make house hunting smarter?\n\nCommonly described as China's answer to Zillow, Beike is an online platform that allows real estate agents to list properties for rent or sale. To help improve the home search experience for house hunters, and to help agents close deals faster, the company built an AI-powered search engine for its listing database. Beike’s real estate listing database was converted into feature vectors then fed into Milvus for indexing and storage. Milvus is then used to conduct similarity search based on an input listing, search criteria, user profile, or other criteria.\n\nFor example, when searching for more homes similar to a given listing, features such as floor plan, size, orientation, interior finishings, paint colors, and more are extracted. Since the original database of property listings listing data has been [indexed](https://medium.com/unstructured-data-service/how-to-choose-an-index-in-milvus-4f3d15259212), searches can be conducted in mere milliseconds. Beike’s final product had an average query time of 113 milliseconds on a dataset containing over 3 million vectors. However, Milvus is capable of maintaining efficient speeds on trillion-scale datasets—making light work of this relatively small real estate database. In general, the system follows the following process:\n\n1. Deep learning models (e.g., CNN, RNN, or BERT) convert unstructured data to feature vectors, which are then imported to Milvus.\n\n2. Milvus stores and indexes the feature vectors.\n\n3. Milvus returns similarity search results based on user queries. \n\n\n\n\n\u003cbr/\u003e\n\nBeike’s intelligent real estate search platform is powered by a recommendation algorithm that calculates vector similarity using cosine distance. The system finds similar homes based on favorite listings and search criteria. At a high level, it works as follows:\n\n1. Based on an input listing, characteristics such as floor plan, size, and orientation are used to extract 4 collections of feature vectors.\n\n2. The extracted feature collections are used to perform similarity search in Milvus. Results of the query for each collection of vectors are a measure of similarity between the input listing and other similar listings. \n\n3. The search results from each of the 4 vector collections are compared then used to recommend similar homes. \n\n\n\n\n\u003cbr/\u003e\n\nAs the figure above shows, the system implements an A/B table switching mechanism for updating data. Milvus stores the data for the first T days in table A, on day T+1 it starts to store data in table B, on day 2T+1, it starts to rewrite table A, and so forth. \n\n\u003cbr/\u003e\n\n### To learn more about making things with Milvus, check out the following resources:\n\n- [Building an AI-Powered Writing Assistant for WPS Office](https://zilliz.com/blog/Building-an-AI-Powered-Writing-Assistant-with-WPS-Office)\n\n- [Making with Milvus: AI-Powered News Recommendation Inside Xiaomi's Mobile Browser](https://zilliz.com/blog/Making-with-Milvus-AI-Powered-News-Recommendation-Inside-Xiaomi-Mobile-Browser)\n ","title":"Making With Milvus AI-Infused Proptech for Personalized Real Estate Search","metaData":{}},{"id":"2021-03-16-Extracting-Events-Highlights-Using-iYUNDONG-Sports-App.md","author":"milvus","desc":"Making with Milvus Intelligent image retrieval system for sports App iYUNDONG","canonicalUrl":"https://zilliz.com/blog/Extracting-Events-Highlights-Using-iYUNDONG-Sports-App","date":"2021-03-16T03:41:30.983Z","cover":"https://assets.zilliz.com/blog_iyundong_6db0f70ef4.jpg","tags":["Scenarios"],"href":"/blog/2021-03-16-Extracting-Events-Highlights-Using-iYUNDONG-Sports-App.md","content":" \n# Extracting Event Highlights Using iYUNDONG Sports App\niYUNDONG is an Internet company aiming to engage more sport lovers and participants of events such as marathon races. It builds [artificial intelligence (AI)](https://en.wikipedia.org/wiki/Artificial_intelligence) tools that can analyze media captured during sporting events to automatically generate highlights. For example, by uploading a selfie, a user of the iYUNDONG sports App who took part in a sport event can instaneously retrieve his or her own photos or video clips from a massive media dataset of the event.\n\n \n\nOne of the key features of iYUNDONG App is called “Find me in motion“. Photographers usually take massive volumes of photos or videos during a sporting event such as a marathon race, and would upload the photos and videos in real time to the iYUNDONG media database. Marathon runners who want to see their highlighted moments can retrieve pictures including themselves simply by uploading one of their selfies. This saves them a lot of time because an image retrieval system in the iYUNDONG App does all the image matching. [Milvus](https://milvus.io/) is adopted by iYUNDONG to power this system as Milvus can greatly accelerate the retrieval process and return highly accurate results.\n\n\u003cbr/\u003e\n\n**Jump to:**\n- [Extracting Event Highlights Using iYUNDONG Sports App](#extracting-event-highlights-using-iyundong-sports-app)\n - [Difficulties and solutions](#difficulties-and-solutions)\n - [What is Milvus](#what-is-milvus)\n - [*An overview of Milvus.*](#an-overview-of-milvus)\n - [Why Milvus](#why-milvus)\n - [System and Workflow](#system-and-workflow)\n - [iYUNDONG App Interface](#iyundong-app-interface)\n - [*iYUNDONG app interface.*](#iyundong-app-interface-1)\n - [Conclusion](#conclusion)\n\n\u003cbr/\u003e\n\n### Difficulties and solutions\n\niYUNDONG faced the following issues and successfully found corresponding solutions when building its image retrieval system. \n\n- Event photos must be immediately available for search.\n\niYUNDONG developed a function called InstantUpload to ensure that event photos are available for search immediately after they are uploaded.\n\n- Storage of massive datasets\n\nMassive data such as photos and videos are uploaded to the iYUNDONG backend every millisecond. So iYUNDONG decided to migrate onto cloud storage systems including [AWS](https://aws.amazon.com/), [S3](https://aws.amazon.com/s3/?nc1=h_ls), and [Alibaba Cloud Object Storage Service (OSS)](https://www.alibabacloud.com/product/oss) for handling gargantuan volumes of unstructured data in a secure, fast and reliable way.\n\n- Instant reading\n\nIn order to achieve instant reading, iYUNDONG developed its own sharding middleware to achieve horizontal scalability easily and mitigate the impact on the system from disk reading. In addition, [Redis](https://redis.io/) is used to serve as a caching layer to ensure consistent performance in situation of high concurrency.\n\n- Instant extraction of facial features\n\nIn order to accurately and efficiently extract facial features from user-uploaded photos, iYUNDONG developed a proprietary image conversion algorithm which converts images into 128-dimensional feature vectors. Another issue encountered was that, oftentimes, many users and photographers uploaded images or videos simultaneously. So system engineers needed to take dynamic scalability into consideration when deploying the system. More specifically, iYUNDONG fully leveraged its elastic compute service (ECS) on the cloud to achieve dynamic scaling.\n\n- Quick and large-scale vector search\n\niYUNDONG needed a vector database to store its large number of feature vectors extracted by AI models. According to its own unique business application scenario, iYUNDONG expected the vector database to be able to: \n1. Perform blazing fast vector retrieval on ultra-large datasets. \n2. Achieve mass storage at lower costs.\n\nInitially, an average of 1 million images were processed annually, so iYUNDONG stored all its data for search in RAM. However, in the past two years, its business boomed and saw an exponential growth of unstructured data – the number of images in iYUNDONG’s database exceeded 60 milllion in 2019, meaning that there were more than 1 billion feature vectors that needed to be stored. A tremendous amount of data inevitably made the iYUNDONG system heavily-built and resource-consuming. So it had to continuously invest in hardware facilities to ensure high performance. Specifically, iYUNDONG deployed more search servers, larger RAM, and a better-performing CPU to achieve greater efficiency and horizontal scalability. However, one of the defects of this solution was that it drove the operating costs prohibitively high. Therefore, iYUNDONG started to explore a better solution to this issue and pondered on leveraging vector index libraries like Faiss to save costs and better steer its business. Finally iYUNDONG chose open-source vector database Milvus.\n\n\u003cbr/\u003e\n\n### What is Milvus\n\nMilvus is an open-source vector database that is easy to use, highly flexible, reliable, and blazing fast. Combined with various deep learning models such as photo and voice recognition, video processing, natural language processing, Milvus can process and analyze unstructured data that are converted into vectors by using various AI algorithms. Below is the workflow of how Milvus processes all unstructured data:\n\n● Unstructured data are converted into embedding vectors by deep learning models or other AI algorithms.\n\n● Then embedding vectors are inserted into Milvus for storage. Milvus also builds indexes for those vectors.\n\n● Milvus performs similarity search and returns accurate search results based on various business needs.\n\n\n\n\n\u003cbr/\u003e\n\n### Why Milvus\nSince the end of 2019, iYUNDONG has carried out a series of testings on using Milvus to power its image retrieval system. The testing results turned out that Milvus outperforms other mainstream vector databases as it supports multiple indexes and can efficiently reduce RAM usage, significantly compressing the timeline for vector similarity search. \n\nMoreover, new versions of Milvus are released regularly. Over the testing period, Milvus has went through multiple version updates from v0.6.0 to v0.10.1.\n\nAdditionally, with its active open-source community and powerful out-of-the-box features, Milvus allows iYUNDONG to operate on a tight development budget.\n\n\u003cbr/\u003e\n\n### System and Workflow\niYUNDONG’s system extracts facial features by detecting faces in event photos uploaded by photographers first. Then those facial features are converted into 128-dimensional vectors and stored in the Milvus library. Milvus creates indexes for those vectors and can instantaneously return highly accurate results. \n\nOther additional information such as photo IDs and coordinates indicating the position of a face in a photo are stored in a third-party database.\n\nEach feature vector has its unique ID in the Milvus library. iYUNDONG adopted the [Leaf algorithm](https://github.com/Meituan-Dianping/Leaf), a distributed ID generation service developed by [Meituan](https://about.meituan.com/en) basic R\u0026D platform, to associate the vector ID in Milvus with its corresponing additional information stored in another database. By combining the feature vector and the additional information, the iYUNDONG system can return similar results upon user search.\n\n \u003cbr/\u003e\n\n### iYUNDONG App Interface\nA series of latest sports events are listed on the homepage. By tapping one of the events, users can see the full details.\n\nAfter tapping the button on the top of the photo gallery page, users can then upload a photo of their own to retrieve images of their highlights. \n\n\n\n\n\u003cbr/\u003e\n\n### Conclusion\nThis article introduces how iYUNDONG App builds an intelligent image retrieval system that can return accurate search results based on user uploaded photos varying in resolution, size, clarity, angle, and other ways that complicate similarity search. With the help of Milvus, iYUNDONG App can successfully run millisecond-level queries on a database of 60+ million images. And accuracy rate of photo retrieval is constantly above 92%. Milvus makes it easier for iYUNDONG to create a powerful, enterprise-grade image retrieval system in a short time with limited resources.\n\n\nRead other [user stories](https://zilliz.com/user-stories) to learn more about making things with Milvus.\n\n\n\n ","title":"Extracting Event Highlights Using iYUNDONG Sports App","metaData":{}},{"id":"2021-03-15-Milvus-Data-Migration-Tool.md","author":"Zilliz","desc":"Learn how to use Milvus data migration tool to greatly improve efficiency of data management and reduce DevOps costs.","canonicalUrl":"https://zilliz.com/blog/Milvus-Data-Migration-Tool","date":"2021-03-15T10:19:51.125Z","cover":"https://assets.zilliz.com/Generic_Tool_Announcement_97eb04a898.jpg","tags":["Engineering"],"href":"/blog/2021-03-15-Milvus-Data-Migration-Tool.md","content":"\n# Introducing Milvus Data Migration Tool\n\n\n***Important Note**: The Mivus Data Migration Tool has been deprecated. For data migration from other databases to Milvus, we recommend that you use the more advanced Milvus-migration Tool.*\n\nThe Milvus-migration tool currently supprots: \n\n- Elasticsearch to Milvus 2.x\n- Faiss to Milvus 2.x\n- Milvus 1.x to Milvus 2.x\n- Milvus 2.3.x to Milvus 2.3.x or above\n\nWe will support migration from more vector data sources such as Pinecone, Chroma, and Qdrant. Stay tuned. \n\n**For more information, see the [Milvus-migration documentation](https://milvus.io/docs/migrate_overview.md) or its [GitHub repository](https://github.com/zilliztech/milvus-migration).**\n\n\n\n--------------------------------- **Mivus Data Migration Tool has been deprecated** ----------------------\n\n\n\n\n\n### Overview\n\n[MilvusDM](https://github.com/milvus-io/milvus-tools) (Milvus Data Migration) is an open-source tool designed specifically for importing and exporting data files with Milvus. MilvusDM can greatly improve data mangement efficiency and reduce DevOps costs in the following ways:\n\n- [Faiss to Milvus](#faiss-to-milvus): Import unzipped data from Faiss to Milvus.\n\n- [HDF5 to Milvus](#hdf5-to-milvus): Import HDF5 files to Milvus.\n\n- [Milvus to Milvus](#milvus-to-milvus): Migrate data from a source Milvus to a different target Milvus.\n\n- [Milvus to HDF5](#milvus-to-hdf5): Save data in Milvus as HDF5 files.\n\n\n\n\nMilvusDM is hosted on [Github](https://github.com/milvus-io/milvus-tools) and can be easily installed by running the command line `pip3 install pymilvusdm`. MilvusDM allows you to migrate data in a specific collection or partition. In the following sections, we will explain how to use each data migration type.\n\n\u003cbr/\u003e\n\n### Faiss to Milvus\n\n#### Steps\n\n1.Download **F2M.yaml**:\n\n```\n$ wget https://raw.githubusercontent.com/milvus-io/milvus-tools/main/yamls/F2\n```\n\n2.Set the following parameters:\n\n- `data_path`: Data path (vectors and their corresponding IDs) in Faiss.\n\n- `dest_host`: Milvus server address.\n\n- `dest_port`: Milvus server port.\n\n- `mode`: Data can be imported to Milvus using the following modes:\n\n - Skip: Ignore data if the collection or partition already exists.\n\n - Append: Append data if the collection or partition already exists.\n\n - Overwrite: Delete data before insertion if the collection or partition already exists.\n\n- `dest_collection_name`: Name of receiving collection for data import.\n\n- `dest_partition_name`: Name of receiving partition for data import.\n\n- `collection_parameter`: Collection-specific information such as vector dimension, index file size, and distance metric.\n\n```\nF2M:\n milvus_version: 1.0.0\n data_path: '/home/data/faiss.index'\n dest_host: '127.0.0.1'\n dest_port: 19530\n mode: 'append' # 'skip/append/overwrite'\n dest_collection_name: 'test'\n dest_partition_name: ''\n collection_parameter:\n dimension: 256\n index_file_size: 1024\n metric_type: 'L2'\n```\n\n3.Run **F2M.yaml:**\n\n```\n$ milvusdm --yaml F2M.yaml\n```\n\n#### Sample Code\n\n1.Read Faiss files to retrieve vectors and their corresponding IDs.\n\n```\nids, vectors = faiss_data.read_faiss_data()\n```\n\n2.Insert the retrieved data into Milvus:\n\n```\ninsert_milvus.insert_data(vectors, self.dest_collection_name, self.collection_parameter, self.mode, ids, self.dest_partition_name)\n```\n\n\u003cbr/\u003e\n\n### HDF5 to Milvus\n\n#### Steps\n\n1.Download **H2M.yaml**.\n\n```\n$ wget https://raw.githubusercontent.com/milvus-io/milvus-tools/main/yamls/H2M.yaml\n```\n\n2.Set the following parameters:\n\n- `data_path`: Path to the HDF5 files.\n\n- `data_dir`: Directory holding the HDF5 files.\n\n- `dest_host`: Milvus server address.\n\n- `dest_port`: Milvus server port.\n\n- `mode`: Data can be imported to Milvus using the following modes:\n\n - Skip: Ignore data if the collection or partition already exists.\n\n - Append: Append data if the collection or partition already exists.\n\n - Overwrite: Delete data before insertion if the collection or partition already exists.\n\n- `dest_collection_name`: Name of receiving collection for data import.\n\n- `dest_partition_name`: Name of receiving partition for data import.\n\n- `collection_parameter`: Collection-specific information such as vector dimension, index file size, and distance metric.\n\n\u003e Set either `data_path` or `data_dir`. Do **not** set both. Use `data_path` to specify multiple file paths, or `data_dir` to specify the directory holding your data file.\n\n```\nH2M:\n milvus-version: 1.0.0\n data_path:\n - /Users/zilliz/float_1.h5\n - /Users/zilliz/float_2.h5\n data_dir:\n dest_host: '127.0.0.1'\n dest_port: 19530\n mode: 'overwrite' # 'skip/append/overwrite'\n dest_collection_name: 'test_float'\n dest_partition_name: 'partition_1'\n collection_parameter:\n dimension: 128\n index_file_size: 1024\n metric_type: 'L2'\n```\n\n3.Run **H2M.yaml:**\n\n```\n$ milvusdm --yaml H2M.yaml\n```\n\n#### Sample Code\n\n1.Read the HDF5 files to retrieve vectors and their corresponding IDs:\n\n```\nvectors, ids = self.file.read_hdf5_data()\n```\n\n2.Insert the retrieved data into Milvus:\n\n```\nids = insert_milvus.insert_data(vectors, self.c_name, self.c_param, self.mode, ids,self.p_name)\n```\n\n\u003cbr/\u003e\n\n### Milvus to Milvus\n\n#### Steps\n\n1.Download **M2M.yaml**.\n\n```\n$ wget https://raw.githubusercontent.com/milvus-io/milvus-tools/main/yamls/M2M.yaml\n```\n\n2.Set the following parameters:\n\n- `source_milvus_path`: Source Milvus work path.\n\n- `mysql_parameter`: Source Milvus MySQL settings. If MySQL is not used, set mysql_parameter as ''.\n\n- `source_collection`: Names of the collection and its partitions in the source Milvus.\n\n- `dest_host`: Milvus server address.\n\n- `dest_port`: Milvus server port.\n\n- `mode`: Data can be imported to Milvus using the following modes:\n\n - Skip: Ignore data if the collection or partition already exists.\n\n - Append: Append data if the collection or partition already exists.\n\n - Overwrite: If the collection or partition already exists, delete the data before inserting it.Delete data before insertion if the collection or partition already exists.\n\n```\nM2M:\n milvus_version: 1.0.0\n source_milvus_path: '/home/user/milvus'\n mysql_parameter:\n host: '127.0.0.1'\n user: 'root'\n port: 3306\n password: '123456'\n database: 'milvus'\n source_collection:\n test:\n - 'partition_1'\n - 'partition_2'\n dest_host: '127.0.0.1'\n dest_port: 19530\n mode: 'skip' # 'skip/append/overwrite'\n```\n\n3.Run **M2M.yaml.**\n\n```\n$ milvusdm --yaml M2M.yaml\n```\n\n#### Sample Code\n\n1.According to a specified collection or partition's metadata, read the files under **milvus/db** on your local drive to retrieve vectors and their corresponding IDs from the source Milvus.\n\n```\ncollection_parameter, _ = milvus_meta.get_collection_info(collection_name)\nr_vectors, r_ids, r_rows = milvusdb.read_milvus_file(self.milvus_meta, collection_name, partition_tag)\n```\n\n2.Insert the retrieved data into the target Milvus.\n\n```\nmilvus_insert.insert_data(r_vectors, collection_name, collection_parameter, self.mode, r_ids, partition_tag)\n```\n\n\u003cbr/\u003e\n\n### Milvus to HDF5\n\n#### Steps\n\n1.Download **M2H.yaml**:\n\n```\n$ wget https://raw.githubusercontent.com/milvus-io/milvus-tools/main/yamls/M2H.yaml\n```\n\n2.Set the following parameters:\n\n- `source_milvus_path`: Source Milvus work path.\n\n- `mysql_parameter`: Source Milvus MySQL settings. If MySQL is not used, set mysql_parameter as ''.\n\n- `source_collection`: Names of the collection and its partitions in the source Milvus.\n\n- `data_dir`: Directory for holding the saved HDF5 files.\n\n```\nM2H:\n milvus_version: 1.0.0\n source_milvus_path: '/home/user/milvus'\n mysql_parameter:\n host: '127.0.0.1'\n user: 'root'\n port: 3306\n password: '123456'\n database: 'milvus'\n source_collection: # specify the 'partition_1' and 'partition_2' partitions of the 'test' collection.\n test:\n - 'partition_1'\n - 'partition_2'\n data_dir: '/home/user/data'\n```\n\n3.Run **M2H.yaml**:\n\n```\n$ milvusdm --yaml M2H.yaml\n```\n\n#### Sample Code\n\n1.According to a specified collection or partition's metadata, read the files under **milvus/db** on your local drive to retrieve vectors and their corresponding IDs.\n\n```\ncollection_parameter, version = milvus_meta.get_collection_info(collection_name)\nr_vectors, r_ids, r_rows = milvusdb.read_milvus_file(self.milvus_meta, collection_name, partition_tag)\n```\n\n2.Save the retrieved data as HDF5 files.\n\n```\ndata_save.save_yaml(collection_name, partition_tag, collection_parameter, version, save_hdf5_name)\n```\n\n### MilvusDM File Structure\n\nThe flow chart below shows how MilvusDM performs different tasks according to the YAML file it receives:\n\n\n\n\nMilvusDM file structure:\n\n- pymilvusdm\n\n - core\n\n - **milvus_client.py**: Performs client operations in Milvus.\n\n - **read_data.py**: Reads the HDF5 data files on your local drive. (Add your code here to support reading data files in other formats.)\n\n - **read_faiss_data.py**: Reads the data files in Faiss.\n\n - **read_milvus_data.py**: Reads the data files in Milvus.\n\n - **read_milvus_meta.py**: Reads the metadata in Milvus.\n\n - **data_to_milvus.py**: Creates collections or partitions based on parameters in YAML files and imports the vectors and the corresponding vector IDs into Milvus.\n\n - **save_data.py**: Saves the data as HDF5 files.\n\n - **write_logs.py**: Writes logs during runtime.\n\n - **faiss_to_milvus.py**: Imports data from Faiss into Milvus.\n\n - **hdf5_to_milvus.py**: Imports data in HDF5 files into Milvus.\n\n - **milvus_to_milvus.py**: Migrates data from a source Milvus to the target Milvus.\n\n - **milvus_to_hdf5.p**y: Exports data in Milvus and saves them as HDF5 files.\n\n - **main.py**: Performs corresponding tasks according to the received YAML file.\n\n - **setting.py**: Configurations relating to running the MilvusDM code.\n\n- **setup.py**: Creates **pymilvusdm** file packages and uploads them to PyPI (Python Package Index).\n\n\u003cbr/\u003e\n\n### Recap\n\nMilvusDM primarily handles migrating data in and out of Milvus, which includes Faiss to Milvus, HDF5 to Milvus, Milvus to Milvus, and Milvus to HDF5.\n\nThe following features are planned for upcoming releases:\n\n- Import binary data from Faiss to Milvus.\n\n- Blocklist and allowlist for data migration between source Milvus and target Milvus.\n\n- Merge and import data from multiple colletions or partitions in source Milvus into a new collection in target Milvus.\n\n- Backup and recovery of the Milvus data.\n\nThe MilvusDM project is open sourced on [Github](https://github.com/milvus-io/milvus-tools). Any and all contributions to the project are welcome. Give it a star 🌟, and feel free to file an [issue](https://github.com/milvus-io/milvus-tools/issues) or submit your own code!\n","title":"Introducing Milvus Data Migration Tool","metaData":{}},{"id":"2021-03-10-milvus-1-0-the-worlds-most-popular-open-source-vector-database-just-got-better.md","author":"milvus","desc":"Milvus v1.0, a stable, long-term support version, is available now. Milvlus powers image/video search, chatbots, and many more AI applications.","canonicalUrl":"https://zilliz.com/blog/milvus-1-0-the-worlds-most-popular-open-source-vector-database-just-got-better","date":"2021-03-10T06:58:36.647Z","cover":"https://assets.zilliz.com/Milvus_510cf50aee.jpeg","tags":["Engineering"],"href":"/blog/2021-03-10-milvus-1-0-the-worlds-most-popular-open-source-vector-database-just-got-better.md","content":"\n# Milvus 1.0: The World's Most Popular Open-Source Vector Database Just Got Better\n\nZilliz is proud to announce the release of Milvus v1.0. After months of extensive testing Milvus v1.0, which is based on a stable version of Milvus v0.10.6, is available for use.\n\nMilvus v1.0 offers the following key features:\n\n- Support for mainstream similarity metrics, including Euclidean distance, inner product, Hamming distance, Jaccard coefficient, and more.\n- Integration with, and improvements to, SOTA ANNs algorithms, including Faiss, Hnswlib, Annoy, NSG, and more.\n- Scale-out capability through the Mishards sharding proxy.\n- Support for processors commonly used in AI scenarios, including X86, Nvidia GPU, Xilinx FPGA, and more.\n\nSee the [Release Notes](https://www.milvus.io/docs/v1.0.0/release_notes.md) for additional Milvus v1.0 features.\n\nMilvus is an ongoing open-source software (OSS) project. Its first major release has the following implications for users:\n\n- Milvus v1.0 will receive long-term support (3+ years).\n- The most stable Milvus release to date is well structured and ready for integration with existing AI ecosystems.\n\n### The first version of Milvus with long-term support\n\nThanks in part to sponsorship from Zilliz, the Milvus community will provide bug fix support for Milvus v1.0 until December 31st, 2024. New features will be available only in releases following v1.0.\n\nSee [The Milvus release guideline](https://milvus.io/docs/v1.0.0/milvus_release_guideline.md) for information about release cadences and more.\n\n### Toolchain enhancements and seamless AI ecosystem integration\n\nBeginning with v1.0, Milvus' toolchain will be a primary development focus. We plan to create the necessary tooling and utilities to meet the needs of the Milvus user community.\n\nStability makes integrating Milvus with AI ecosystems a breeze. We are seeking further collaboration between the Milvus community and other AI-focused OSS communities. We encourage contributions to the new AI ASICs (application-specific integrated circuits) in Milvus.\n\n### The future of Milvus\n\nWe believe Milvus has a bright future thanks to the following factors:\n\n- Regular contributions from developers in the Milvus community.\n- Support for integration with any cloud-native environment.\n\nWe have drafted [community charters](https://milvus.io/docs/v1.0.0/milvus_community_charters.md) to help guide, nurture, and advance the Milvus community as our technology and user base grows. The charters include several technical decisions made to attract more participants to the community.\n\n- Golang will now be used to develop the Milvus engine however, the ANNS algorithm component will still be developed in C++.\n- The forthcoming distributed version of Milvus will use existing cloud components as much as possible.\n\nWe are thrilled to partner with the open-source software community to build the next-generation cloud data fabric made for AI. Let's get to work!\n\n### Don’t be a stranger\n\n- Find or contribute to Milvus on [GitHub](https://github.com/milvus-io/milvus/)\n- Interact with the community via [Slack](https://join.slack.com/t/milvusio/shared_invite/zt-e0u4qu3k-bI2GDNys3ZqX1YCJ9OM~GQ).\n- Connect with us on [Twitter](https://twitter.com/milvusio).\n","title":"Milvus 1.0 The World's Most Popular Open-Source Vector Database Just Got Better","metaData":{}},{"id":"2021-02-24-building-personalized-recommender-systems-milvus-paddlepaddle.md","author":"Shiyu Chen","desc":"How to build a deep learning powered recommender system","canonicalUrl":"https://zilliz.com/blog/building-personalized-recommender-systems-milvus-paddlepaddle","date":"2021-02-24T23:12:34.209Z","cover":"https://assets.zilliz.com/header_e6c4a8aee6.jpg","tags":["Scenarios"],"href":"/blog/2021-02-24-building-personalized-recommender-systems-milvus-paddlepaddle.md","content":" \n# Building Personalized Recommender Systems with Milvus and PaddlePaddle\n## Background Introduction\n\nWith the continuous development of network technology and the ever-expanding scale of e-commerce, the number and variety of goods grow rapidly and users need to spend a lot of time to find the goods they want to buy. This is information overload. In order to solve this problem, recommendation system came into being.\n\nThe recommendation system is a subset of the Information Filtering System, which can be used in a range of areas such as movies, music, e-commerce, and Feed stream recommendations. The recommendation system discovers the user’s personalized needs and interests by analyzing and mining user behaviors, and recommends information or products that may be of interest to the user. Unlike search engines, recommendation system do not require users to accurately describe their needs, but model their historical behavior to proactively provide information that meets user interests and needs.\n\nIn this article we use PaddlePaddle, a deep learning platform from Baidu, to build a model and combine Milvus, a vector similarity search engine, to build a personalized recommendation system that can quickly and accurately provide users with interesting information.\n\n## Data Preparation\n\nWe take MovieLens Million Dataset (ml-1m) [1] as an example. The ml-1m dataset contains 1,000,000 reviews of 4,000 movies by 6,000 users, collected by the GroupLens Research lab. The original data includes feature data of the movie, user feature, and user rating of the movie, you can refer to ml-1m-README [2] .\n\nml-1m dataset includes 3 .dat articles: movies.dat、users.dat and ratings.dat.movies.dat includes movie’s features, see example below:\n\n MovieID::Title::Genres\n 1::ToyStory(1995)::Animation|Children's|Comedy\n\nThis means that the movie id is 1, and the title is 《Toy Story》, which is divided into three categories. These three categories are animation, children, and comedy.\n\nusers.dat includes user’s features, see example below:\n\n UserID::Gender::Age::Occupation::Zip-code\n 1::F::1::10::48067\n\nThis means that the user ID is 1, female, and younger than 18 years old. The occupation ID is 10.\n\nratings.dat includes the feature of movie rating, see example below:\n\nUserID::MovieID::Rating::Timestamp\n1::1193::5::978300760\n\nThat is, the user 1 evaluates the movie 1193 as 5 points.\n\n## Fusion Recommendation Model\n\nIn the film personalized recommendation system we used the Fusion Recommendation Model [3] which PaddlePaddle has implemented. This model is created from its industrial practice.\n\nFirst, take user features and movie features as input to the neural network, where:\n\n- The user features incorporate four attribute information: user ID, gender, occupation, and age.\n- The movie feature incorporate three attribute information: movie ID, movie type ID, and movie name.\n\nFor the user feature, map the user ID to a vector representation with a dimension size of 256, enter the fully connected layer, and do similar processing for the other three attributes. Then the feature representations of the four attributes are fully connected and added separately.\n\nFor movie features, the movie ID is processed in a manner similar to the user ID. The movie type ID is directly input into the fully connected layer in the form of a vector, and the movie name is represented by a fixed-length vector using a text convolutional neural network. The feature representations of the three attributes are then fully connected and added separately.\n\nAfter obtaining the vector representation of the user and the movie, calculate the cosine similarity of them as the score of the personalized recommendation system. Finally, the square of the difference between the similarity score and the user’s true score is used as the loss function of the regression model.\n\n\n\n## System Overview\n\nCombined with PaddlePaddle’s fusion recommendation model, the movie feature vector generated by the model is stored in the Milvus vector similarity search engine, and the user feature is used as the target vector to be searched. Similarity search is performed in Milvus to obtain the query result as the recommended movies for the user.\n\n\n\n\u003e The inner product (IP) method is provided in Milvus to calculate the vector distance. After normalizing the data, the inner product similarity is consistent with the cosine similarity result in the fusion recommendation model.\n\n## Application of Personal Recommender System\n\nThere are three steps in building a personalized recommendation system with Milvus, details on how to operate please refer to Mivus Bootcamp [4].\n\n### Step 1:Model Training\n\n # run train.py\n $ python train.py\n\nRunning this command will generate a model recommender_system.inference.model in the directory, which can convert movie data and user data into feature vectors, and generate application data for Milvus to store and retrieve.\n\n### Step 2:Data Preprocessing\n\n # Data preprocessing, -f followed by the parameter raw movie data file name\n $ python get_movies_data.py -f movies_origin.txt\n\nRunning this command will generate test data movies_data.txt in the directory to achieve pre-processing of movie data.\n\n### Step 3:Implementing Personal Recommender System with Milvus\n\n # Implementing personal recommender system based on user conditions\n $ python infer_milvus.py -a \u003cage\u003e-g \u003cgender\u003e-j \u003cjob\u003e[-i]\n\nRunning this command will implement personalized recommendations for specified users.\n\nThe main process is:\n\n- Through the load_inference_model, the movie data is processed by the model to generate a movie feature vector.\n- Load the movie feature vector into Milvus via milvus.insert.\n- According to the user’s age / gender / occupation specified by the parameters, it is converted into a user feature vector, milvus.search_vectors is used for similarity retrieval, and the result with the highest similarity between the user and the movie is returned.\n\nPrediction of the top five movies that the user is interested in:\n\n TopIdsTitleScore\n 03030Yojimbo2.9444923996925354\n 13871Shane2.8583481907844543\n 23467Hud2.849525213241577\n 31809Hana-bi2.826111316680908\n 43184Montana2.8119677305221558 \n\n## Summary\n\nBy inputing user information and movie information to the fusion recommendation model we can get matching scores, and then sort the scores of all movies based on the user to recommend movies that may be of interest to the user. **This article combines Milvus and PaddlePaddle to build a personalized recommendation system. Milvus, a vector search engine, is used to store all movie feature data, and then similarity retrieval is performed on user features in Milvus.** The search result is the movie ranking recommended by the system to the user.\n\nMilvus [5] vector similarity search engine is compatible with various deep learning platforms, searching billions of vectors with only millisecond response. You can explore more possibilities of AI applications with Milvus with ease!\n\n## Reference\n1. MovieLens Million Dataset (ml-1m): http://files.grouplens.org/datasets/movielens/ml-1m.zip\n2. ml-1m-README: http://files.grouplens.org/datasets/movielens/ml-1m-README.txt\n3. Fusion Recommendation Model by PaddlePaddle: https://www.paddlepaddle.org.cn/documentation/docs/zh/beginners_guide/basics/recommender_system/index.html#id7\n4. Bootcamp: https://github.com/milvus-io/bootcamp/tree/master/solutions/recommendation_system\n5. Milvus: https://milvus.io/\n\n\n \n","title":"Building Personalized Recommender Systems with Milvus and PaddlePaddle","metaData":{}},{"id":"2021-02-05-How-we-used-semantic-search-to-make-our-search-10-x-smarter.md","author":"Rahul Yadav","desc":"Tokopedia used Milvus to build a 10x smarter search system that has dramatically enhanced the user experience.","canonicalUrl":"https://zilliz.com/blog/How-we-used-semantic-search-to-make-our-search-10-x-smarter","date":"2021-02-05T06:27:15.076Z","cover":"https://assets.zilliz.com/Blog_How_we_used_semantic_search_to_make_our_search_10x_smarter_1_a7bac91379.jpeg","tags":["Scenarios"],"href":"/blog/2021-02-05-How-we-used-semantic-search-to-make-our-search-10-x-smarter.md","content":" \n# How we used semantic search to make our search 10x smarter\nAt Tokopedia, we understand that the value in our product corpus is only unlocked when our buyers can find products that are relevant to them, so we strive to improve the relevance of search results.\n\nTo further that effort, we are introducing **similarity search** on Tokopedia. If you go to the search result page on mobile devices, you will find a “…” button that exposes a menu that gives you the option to search for products similar to the product.\n\n## Keyword-based search\nTokopedia Search uses **Elasticsearch** for the search and ranking of products. For each search request, we first query Elasticsearch, which ranks products according to the search query. ElasticSearch stores each word as a sequence of numbers representing [ASCII](https://en.wikipedia.org/wiki/ASCII) (or UTF) codes for each letter. It builds an [inverted-index](https://en.wikipedia.org/wiki/Inverted_index) to quickly find out, which documents contain words from the user query, and then finds the best match among them using various scoring algorithms. These scoring algorithms pay little attention to what the words mean, but rather to how frequently they occur in the document, how close they are to each other, etc. ASCII representation obviously contains enough information to convey the semantics (after all we, humans, can understand it). Unfortunately, there’s no good algorithm for the computer to compare ASCII-encoded words by their meaning.\n\n## Vector representation\nOne solution to this would be to come up with an alternative representation, which tells us not only about the letters contained in the word but also something about its meaning. For example, we could encode *which other words our word is frequently used together with* (represent by the probable context). We’d then assume that similar contexts represent similar things, and try to compare them using mathematical methods. We could even find a way to encode whole sentences by their meaning.\n\n\n\n\n## Select an embedding similarity search engine\nNow that we have feature vectors, the remaining issue is how to retrieve from the large volume of vectors the ones that are similar to the target vector. When it comes to the embeddings search engine, we tried POC on several engines available on Github some of them are FAISS, Vearch, Milvus.\n\nWe prefer Milvus to other engines based on load test results. On the one hand, we have used FAISS before on other teams and hence would like to try something new. Compared to Milvus, FAISS is more of an underlying library, therefore not quite convenient to use. As we learned more about Milvus, we finally decided to adopt Milvus for its two main features:\n\n- Milvus is very easy to use. All you need to do is to pull its Docker image and update the parameters based on your own scenario.\n\n- It supports more indexes and has detailed supporting documentation.\n\nIn a nutshell, Milvus is very friendly to users and the documentation is quite detailed. If you come across any problem, you can usually find solutions in the documentation; otherwise, you can always get support from the Milvus community.\n\n## Milvus cluster service\nAfter deciding to use Milvus as the feature vector search engine, we decided to use Milvus for one of our Ads service use-case where we wanted to match [low fill rate](https://www.tradegecko.com/blog/wholesale-management/what-is-fill-rate-and-why-does-it-matter-for-wholesalers) keywords with high fill rate keywords. We configured a standalone node in a development (DEV) environment and started serving, it had been running well for a few days, and giving us improved CTR/CVR metrics. If a standalone node crashed in production, the entire service would become unavailable. Thus, we need to deploy a highly available search service.\n\nMilvus provides both Mishards, a cluster sharding middleware, and Milvus-Helm for configuration. In Tokopedia we use Ansible playbooks for infrastructure setup so we created a playbook for infra orchestration. The diagram below from Milvus’ documentation shows how Mishards works:\n\n\n\nMishards cascade a request from upstream down to its sub-modules splitting the upstream request, and then collects and returns the results of the sub-services to upstream. The overall architecture of the Mishards-based cluster solution is shown below:\n\n\nThe official documentation provides a clear introduction of Mishards. You can refer to [Mishards](https://milvus.io/cn/docs/v0.10.2/mishards.md) if you are interested.\n\nIn our keyword-to-keyword service, we deployed one writable node, two read-only nodes, and one Mishards middleware instance in GCP, using Milvus ansible. It has been stable so far. A huge component of what makes it possible to efficiently query the million-, billion-, or even trillion-vector datasets that similarity search engines rely on is [indexing](https://milvus.io/docs/v0.10.5/index.md), a process of organizing data that drastically accelerates big data search.\n\n## How does vector indexing accelerate similarity search?\nSimilarity search engines work by comparing input to a database to find objects that are most similar to the input. Indexing is the process of efficiently organizing data, and it plays a major role in making similarity search useful by dramatically accelerating time-consuming queries on large datasets. After a massive vector dataset is indexed, queries can be routed to clusters, or subsets of data, that are most likely to contain vectors similar to an input query. In practice, this means a certain degree of accuracy is sacrificed to speed up queries on really big vector data.\n\nAn analogy can be drawn to a dictionary, where words are sorted alphabetically. When looking up a word, it is possible to quickly navigate to a section that only contains words with the same initial — drastically accelerating the search for the input word’s definition.\n\n## What next, you ask?\n\n\nAs shown above, there is no solution that fits all, we always want to improve the model’s performance used for getting the embeddings.\n\nAlso, from a technical point of view, we want to run multiple learning models at the same time and compare the results from the various experiments. Watch this space for more information on our experiments like image search, video search.\n\n\u003cbr/\u003e\n\n## References:\n- Mishards Docs:https://milvus.io/docs/v0.10.2/mishards.md\n- Mishards: https://github.com/milvus-io/milvus/tree/master/shards\n- Milvus-Helm: https://github.com/milvus-io/milvus-helm/tree/master/charts/milvus\n\n\u003cbr/\u003e\n\n*This blog article is reposted from: https://medium.com/tokopedia-engineering/how-we-used-semantic-search-to-make-our-search-10x-smarter-bd9c7f601821*\n\nRead other [user stories](https://zilliz.com/user-stories) to learn more about making things with Milvus.\n ","title":"How we used semantic search to make our search 10x smarter","metaData":{}},{"id":"2021-01-13-Milvus-Was-Built-for-Massive-Scale-Think-Trillion-Vector-Similarity-Search.md","author":"milvus","desc":"Explore the power of open-source in your next AI or machine learning project. Manage massive-scale vector data and power similarity search with Milvus.","canonicalUrl":"https://zilliz.com/blog/Milvus-Was-Built-for-Massive-Scale-Think-Trillion-Vector-Similarity-Search","date":"2021-01-13T08:56:00.480Z","cover":"https://assets.zilliz.com/1_9a6be0b54f.jpg","tags":["Engineering"],"href":"/blog/2021-01-13-Milvus-Was-Built-for-Massive-Scale-Think-Trillion-Vector-Similarity-Search.md","content":" \n# Milvus Was Built for Massive-Scale (Think Trillion) Vector Similarity Search\nEvery day, an incalculable number of business-critical insights are squandered because companies can’t make sense of their own data. Unstructured data, such as text, image, video, and audio, is estimated to account for 80% of all data — but just 1% of it is ever analyzed. Fortunately, [artificial intelligence (AI)](https://medium.com/unstructured-data-service/the-easiest-way-to-search-among-1-billion-image-vectors-d6faf72e361f), open-source software, and Moore’s law are making machine-scale analytics more accessible than ever before. Using vector similarity search, it is possible to extract value from massive unstructured datasets. This technique involves converting unstructured data into feature vectors, a machine-friendly numerical data format that can be processed and analyzed in real time.\n\nVector similarity search has applications spanning e-commerce, security, new drug development, and more. These solutions rely on dynamic datasets containing millions, billions, or even trillions of vectors, and their usefulness often depends on returning near instantaneous results. [Milvus](https://milvus.io/) is an open-source vector data management solution built from the ground up for efficiently managing and searching large vector datasets. This article covers Milvus’ approach to vector data management, as well as how the platform has been optimized for vector similarity search.\n\n**Jump to:**\n- [Milvus Was Built for Massive-Scale (Think Trillion) Vector Similarity Search](#milvus-was-built-for-massive-scale-think-trillion-vector-similarity-search)\n - [LSM trees keep dynamic data management efficient at massive scales](#lsm-trees-keep-dynamic-data-management-efficient-at-massive-scales)\n - [*A segment of 10-dimensional vectors in Milvus.*](#a-segment-of-10-dimensional-vectors-in-milvus)\n - [Data management is optimized for rapid access and limited fragmentation](#data-management-is-optimized-for-rapid-access-and-limited-fragmentation)\n - [*An illustration of inserting vectors in Milvus.*](#an-illustration-of-inserting-vectors-in-milvus)\n - [*Queried data files before the merge.*](#queried-data-files-before-the-merge)\n - [*Queried data files after the merge.*](#queried-data-files-after-the-merge)\n - [Similarity searched is accelerated by indexing vector data](#similarity-searched-is-accelerated-by-indexing-vector-data)\n - [Learn more about Milvus](#learn-more-about-milvus)\n\n### LSM trees keep dynamic data management efficient at massive scales\n\nTo provide efficient dynamic data management, Milvus uses a log-structured merge-tree (LSM tree) data structure. LSM trees are well suited for accessing data that has a high number of inserts and deletes. For detailed information on specific attributes of LSM trees that help ensure high-performance dynamic data management, see the [original research](http://paperhub.s3.amazonaws.com/18e91eb4db2114a06ea614f0384f2784.pdf) published by its inventors. LSM trees are the underlying data structure used by many popular databases, including [BigTable](https://cloud.google.com/bigtable), [Cassandra](https://cassandra.apache.org/), and [RocksDB](https://rocksdb.org/).\n\nVectors exist as entities in Milvus and are stored in segments. Each segment contains anywhere from one up to ~8 million entities. Each entity has fields for a unique ID and vector inputs, with the latter representing anywhere from 1 to 32768 dimensions.\n\n\n\n\n### Data management is optimized for rapid access and limited fragmentation\n\nWhen receiving an insert request, Milvus writes new data to the [write ahead log (WAL)](https://milvus.io/docs/v0.11.0/write_ahead_log.md). After the request is successfully recorded to the log file, the data is written to a mutable buffer. Finally, one of three triggers results in the buffer becoming immutable and flushing to disk:\n\n1. **Timed intervals:** Data is regularly flushed to disk at defined intervals (1 second by default).\n2. **Buffer size:** Accumulated data reaches the upper limit for the mutable buffer (128 MB).\n3. **Manual trigger:** Data is manually flushed to disk when the client calls the flush function.\n\n\n\n\nUsers can add tens or millions of vectors at a time, generating data files of different sizes as new vectors are inserted. This results in fragmentation that can complicate data management and slow down vector similarity search. To prevent excessive data fragmentation, Milvus constantly merges data segments until the combined file size reaches a user configurable limit (e.g., 1 GB). For example, given an upper limit of 1 GB, inserting 100 million 512-dimensional vectors will result in just ~200 data files.\n\nIn incremental computation scenarios where vectors are inserted and searched concurrently, Milvus makes newly inserted vector data immediately available for search before merging it with other data. After data merges, the original data files will be removed and the newly created merged file will be used for search instead.\n\n\n\n\n\n\n\n### Similarity searched is accelerated by indexing vector data\n\nBy default, Milvus relies on brute-force search when querying vector data. Also known as exhaustive search, this approach checks all vector data each time a query is run. With datasets containing millions or billions of multi-dimensional vectors, this process is too slow to be useful in most similarity search scenarios. To help expedite query time, algorithms are used to build a vector index. The indexed data is clustered such that similar vectors are closer together, allowing the similarity search engine to query just a portion of the total data, drastically reducing query times while sacrificing accuracy.\n\nMost of the vector index types supported by Milvus use approximate nearest neighbor (ANN) search algorithms. There are numerous ANN indexes, and each one comes with tradeoffs between performance, accuracy, and storage requirements. Milvus supports quantization-, graph, and tree-based indexes, all of which serve different application scenarios. See Milvus’ [technical documentation](https://milvus.io/docs/v0.11.0/index.md#CPU) for more information about building indexes and the specific types of vector indexes it supports.\n\nIndex building generates a lot of metadata. For example, indexing 100 million 512-dimensional vectors saved in 200 data files will result in an additional 200 index files. In order to efficiently check file statuses and delete or insert new files, an efficient metadata management system is required. Milvus uses online transactional processing (OLTP), a data processing technique that is well-suited for updating and/or deleting small amounts of data in a database. Milvus uses SQLite or MySQL to manage metadata.\n\n### Learn more about Milvus\n\nMilvus is an open-source vector data management platform currently in incubation at [LF AI \u0026 Data](https://lfaidata.foundation/), an umbrella organization of the Linux Foundation. Milvus was made open source in 2019 by [Zilliz](https://zilliz.com), the data science software company that initiated the project. More information about Milvus can be found on its [website](https://milvus.io/). If you’re interested in vector similarity search, or using AI to unlock the potential of unstructured data, please join our [open-source community](https://github.com/milvus-io) on GitHub.\n\n\n\n\n\n ","title":"Milvus Was Built for Massive-Scale (Think Trillion) Vector Similarity Search","metaData":{}},{"id":"2021-01-13-Milvus-Is-an-Open-Source-Scalable-Vector-Database.md","author":"milvus","desc":"Build powerful machine learning applications and manage massive-scale vector data with Milvus.","canonicalUrl":"https://zilliz.com/blog/Milvus-Is-an-Open-Source-Scalable-Vector-Database","date":"2021-01-13T07:46:40.506Z","cover":"https://assets.zilliz.com/milvus_5b2cdec665.jpg","tags":["Scenarios"],"href":"/blog/2021-01-13-Milvus-Is-an-Open-Source-Scalable-Vector-Database.md","content":"\n# Milvus Is an Open-Source Scalable Vector Database\n\nSearching data using easily defined criteria, for example querying a movie database by actor, director, genre, or release date, is simple. A relational database is well equipped for these types of basic searches using a query language such as SQL. But when searches involve complex objects and more abstract queries, such as searching a video streaming library using natural language or a video clip, simple similarity metrics like matching words in a title or description are no longer sufficient.\n\n[Artificial intelligence (AI)](https://medium.com/unstructured-data-service/the-easiest-way-to-search-among-1-billion-image-vectors-d6faf72e361f#d62b) has made computers significantly more capable of understanding the semantics of language, as well as helping people make sense of massive, difficult to analyze unstructured datasets (e.g., audio, video, documents, and social media data). AI makes it possible for Netflix to create sophisticated content recommendation engines, Google users to search the web by image, and pharmaceutical companies to discover new drugs.\n\n### The challenge of searching large unstructured datasets\n\nThese feats of technology are accomplished by using AI algorithms to convert dense unstructured data into vectors, a numerical data format that is easily read by machines. Next, additional algorithms are used to calculate the similarity between vectors for a given search. The large size of unstructured datasets makes searching them in their entirety far too time consuming for most machine learning applications. To overcome this, approximate nearest neighbor (ANN) algorithms are used to cluster similar vectors together, then only search the portion of the dataset most likely to contain similar vectors to the target search vector.\n\nThis results in [drastically faster](https://medium.com/unstructured-data-service/how-to-choose-an-index-in-milvus-4f3d15259212#7a9a) (though slightly less accurate) similarity search, and is key to building useful AI tools. Thanks to vast public resources, it has never been easier or cheaper to build machine learning applications. However, AI-powered vector similarity search often requires interlacing different tools that vary in number and complexity depending on specific project requirements. Milvus is an open-source AI search engine that aims to simplify the process of building machine learning applications by providing robust functionality under a unified platform.\n\n### What is Milvus?\n\n[Milvus](https://milvus.io/) is an open-source data management platform built specifically for massive-scale vector data and streamlining machine learning operations (MLOps). Powered by Facebook AI Similarity Search (Faiss), Non-Metric Space Library (NMSLIB), and Annoy, Milvus brings a variety of powerful tools together in one place while extending their standalone functionality. The system was purpose built for storing, processing, and analyzing large vector datasets, and can be used to build AI applications spanning computer vision, recommendation engines, and much more.\n\n\n\n\n### Milvus was made to power vector similarity search\n\nMilvus was designed for flexibility, allowing developers to optimize the platform for their specific use case. Support for CPU/GPU-only and heterogeneous computing makes it possible to accelerate data processing and optimize resource requirements for any scenario. Data is stored in Milvus on a distributed architecture, making it trivial to scale data volumes. With support for various AI models, programming languages (e.g., C++, Java, and Python), and processor types (e.g., x86, ARM, GPU, TPU, and FPGA), Milvus offers high compatibility with a wide variety of hardware and software.\n\nFor more information about Milvus, check out the following resources:\n\n- Explore Milvus' [technical documentation](https://milvus.io/) and learn more about the platform’s inner workings.\n- Learn how to launch Milvus, build applications, and more using [Milvus tutorials](https://tutorials.milvus.io/).\n- Make contributions to the project and engage with Milvus' open-source community on [GitHub](https://github.com/milvus-io).\n","title":"Milvus Is an Open-Source Scalable Vector Database","metaData":{}},{"id":"2021-01-05-Vector-Similarity-Search-Hides-in-Plain-View.md","author":"milvus","desc":"Discover what vector similarity search is, its various applications, and the public resources making artificial intelligence more accessible than ever.","canonicalUrl":"https://zilliz.com/blog/Vector-Similarity-Search-Hides-in-Plain-View","date":"2021-01-05T03:40:20.821Z","cover":"https://assets.zilliz.com/plainview_703d8497ca.jpg","tags":["Engineering"],"href":"/blog/2021-01-05-Vector-Similarity-Search-Hides-in-Plain-View.md","content":"\n# Vector Similarity Search Hides in Plain View\n\n[Artificial intelligence (AI)](https://medium.com/unstructured-data-service/the-easiest-way-to-search-among-1-billion-image-vectors-d6faf72e361f#a291) has the potential to change the way even the most obscure things are done. For example, every year (before COVID, anyway) over 73,000 people congregate to compete in the Hong Kong Marathon. In order to properly sense and record finishing times for all race participants, organizers distribute 73,000 RFID chip timers to attach to each runner. Chip timing is a complex undertaking with obvious downsides. Materials (chips and electronic reading devices) must be purchased or rented from timing companies, and a registration area must be staffed for runners to collect chips on race day. Additionally, if sensors are installed only at the start and finish lines, it’s possible for unscrupulous runners to cut the course.\n\n\n\n\nNow imagine a [video AI](https://cloud.google.com/video-intelligence) application capable of automatically identifying individual runners from footage captured at the finish line using a single photo. Rather than attach timing chips to each participant, runners simply upload a photo of themselves via an app after they cross the finish line. Instantly, a personalized highlight reel, race stats, and other relevant information is provided. Cameras installed at various points throughout the race can capture additional footage of participants and ensure each runner traverses the entire course. Which solution seems easier and more cost-effective to implement?\n\nAlthough the Hong Kong Marathon doesn’t leverage machine learning to replace timing chips (yet), this example illustrates the potential AI has to drastically alter everything around us. For race timing, it reduces tens of thousands of chips to a few cameras paired with machine learning algorithms. But video AI is just one of many applications for vector similarity search, a process that uses artificial intelligence to analyze massive, trillion-scale unstructured datasets. This article provides an overview of vector search technology including what it is, how it can be used, as well as the open-source software and resources making it more accessible than ever before.\n\n**Jump to:**\n\n- [What is vector similarity search?](#what-is-vector-similarity-search)\n\n- [What are some applications of vector similarity search?](#what-are-some-applications-of-vector-similarity-search)\n\n- [Open-source vector similarity search software and resources.](#open-source-vector-similarity-search-software-and-resources)\n\n### What is vector similarity search?\n\nVideo data is incredibly detailed and increasingly common, so logically it seems like it would be a great unsupervised learning signal for building video AI. In reality, this is not the case. Processing and analyzing video data, especially in large volumes, remains a [challenge for artificial intelligence](https://arxiv.org/pdf/1905.11954.pdf). Recent progress in this field, like much of the progress made in unstructured data analytics, is owed in large part to vector similarity search.\n\nThe problem with video, like all unstructured data, is that it doesn’t follow a predefined model or organizational structure, making it difficult to process and analyze at scale. Unstructured data includes things like images, audio, social media behavior, and documents, collectively accounting for an estimated 80-90%+ of all data. Companies are increasingly aware of the business-critical insights buried in massive, enigmatic unstructured datasets, driving demand for AI applications that can tap into this unrealized potential.\n\nUsing [neural networks](https://en.wikipedia.org/wiki/Neural_network) such as CNN, RNN, and BERT, unstructured data can be converted into feature vectors (aka embeddings), a machine-readable numerical data format. Algorithms are then used to calculate the similarity between vectors using measures like cosine similarity or Euclidean distance. Vector embedding and similarity search make it possible to analyze and build machine learning applications using previously indiscernible datasets.\n\nVector similarity is calculated using established algorithms however, unstructured datasets are typically massive. This means efficient and accurate search requires vast storage and compute power. To [accelerate similarity search](https://medium.com/unstructured-data-service/how-to-choose-an-index-in-milvus-4f3d15259212#7a9a) and reduce resource requirements, approximate nearest neighbor (ANN) search algorithms are used. By clustering similar vectors together, ANN algorithms make it possible to send queries to the clusters of vectors most likely to contain similar vectors, rather than searching the entire dataset. Although this approach is faster, it sacrifices some degree of accuracy. Leveraging ANN algorithms allows vector search to comb through billions of deep learning model insights in millisecond.\n\n### What are some applications of vector similarity search?\n\nVector similarity search has applications spanning a wide variety of artificial intelligence, deep learning, and traditional vector calculation scenarios. The following provides a high-level overview of various vector similarity search applications:\n\n**E-commerce:** Vector similarity search has broad applicability in e-commerce, including reverse image search engines that allow shoppers to search for products using an image captured on their smartphone or found online. Additionally, personalized recommendations based on user behavior, interests, purchase history, and more can be served by specialized recommender systems that rely on vector search.\n\n**Physical \u0026 Cyber Security:** Video AI is just one of many applications for vector similarity search in the security field. Other scenarios include facial recognition, behavior tracing, identity authentication, intelligent access control, and more. Additionally, vector similarity search plays an important role in thwarting increasingly common and sophisticated cyberattacks. For example, [code similarity search](https://medium.com/gsi-technology/application-of-ai-to-cybersecurity-part-3-19659bdb3422) can be used to identify security risks by comparing a piece of software to a database of known vulnerabilities or malware.\n\n**Recommendation Engines:** Recommendation engines are systems that use machine learning and data analysis to suggest products, services, content, and information to users. User behavior, the behavior of similar users, and other data is processed using deep learning methods to generate recommendations. With enough data, algorithms can be trained to understand relationships between entities and invent ways to represent them autonomously. Recommendation systems have broad applicability and are something people already interact with every day, including content recommendations on Netflix, shopping recommendations on Amazon, and news feeds on Facebook.\n\n**Chatbots:** Traditionally, chatbots are built using a regular knowledge graph that requires a large training dataset. However, chatbots built using deep learning models don’t need to preprocess data—instead, a map between frequent questions and answers is created. Using a pre-trained natural language processing (NLP) model, feature vectors can be extracted from the questions and then stored and queried using a [vector data management platform](https://medium.com/unstructured-data-service/the-easiest-way-to-search-among-1-billion-image-vectors-d6faf72e361f#92e0).\n\n**Image or Video Search:** Deep learning networks have been used to recognize visual patterns since the late 1970s, and modern technology trends have made image and video search more powerful and accessible than ever before.\n\n**Chemical Similarity Search:** Chemical similarity is key to predicting the properties of chemical compounds and finding chemicals with specific attributes, making it indispensable to the development of new drugs. Fingerprints represented by feature vectors are created for each molecule, and then the distances between vectors are used to measure similarity. Using AI for new drug discovery is gaining momentum in the tech industry, with ByteDance (TikTok’s Chinese parent company) starting to [hire talent in the field](https://techcrunch.com/2020/12/23/bytedance-ai-drug/).\n\n### Open-source vector similarity search software and resources.\n\nMoore’s law, cloud computing, and declining resource costs are macro trends that have made artificial intelligence more accessible than ever. Thanks to open-source software and other publicly available resources, building AI/ML applications isn’t just for big tech companies. Below we provide a brief overview of Milvus, an open-source vector data management platform, and also highlight some publicly available datasets that help put AI within everyone’s reach.\n\n#### Milvus, an open-source vector data management platform\n\n[Milvus](https://milvus.io/) is an open-source vector data management platform built specifically for massive-scale vector data. Powered by Facebook AI Similarity Search (Faiss), Non-Metric Space Library (NMSLIB), and Annoy, Milvus brings a variety of powerful tools together under a single platform while extending their standalone functionality. The system was purpose built for storing, processing, and analyzing large vector datasets, and can be used to build all the AI applications (and more) mentioned above.\n\nMore information about Milvus can be found on its [website](https://milvus.io/). Tutorials, instructions for setting up Milvus, benchmark testing, and information on building a variety of different applications is available in the [Milvus bootcamp](https://github.com/milvus-io/bootcamp). Developers interested in making contributions to the project can join Milvus' open-source community on [GitHub](https://github.com/milvus-io).\n\n#### Public datasets for artificial intelligence and machine learning\n\nIt is no secret that technology giants like Google and Facebook have a data advantage over the little guys, with some pundits even advocating for a “[progressive data-sharing mandate](https://www.technologyreview.com/2019/06/06/135067/making-big-tech-companies-share-data-could-do-more-good-than-breaking-them-up/)” that would force companies that exceed a certain size to share some anonymized data with smaller rivals. Fortunately, there are thousands of publicly available datasets that can be used for AL/ML projects:\n\n- **The People’s Speech Dataset:** This [dataset from ML Commons](https://mlcommons.org/en/peoples-speech/) offers the largest speech dataset in the world, with over 87,000 hours of transcribed speech in 59 different languages.\n\n- **UC Irvine Machine Learning Repository:** The University of California at Irvine maintains [hundreds of public datasets](https://archive.ics.uci.edu/ml/index.php) in an effort to help the machine learning community.\n\n- **Data.gov:** The U.S. government offers [hundreds of thousands of open datasets](https://www.data.gov/) that span education, climate, COVID-19, and more.\n\n- **Eurostat:** The European Union’s statistical office provides [open datasets](https://ec.europa.eu/eurostat/data/database) spanning a variety of industries from economy and finance to population and social conditions.\n\n- **Harvard Dataverse:** [The Harvard Dataverse Repository](https://dataverse.harvard.edu/) is a free data repository open to researchers across disciplines. Many datasets are public, while others come with more restricted terms of use.\n\nAlthough this list is by no means exhaustive, it is a good starting point for discovering the surprisingly wide variety of open datasets. For more information on public datasets as well as choosing the right data for your next ML or data science project, check out this [Medium post](https://altexsoft.medium.com/best-public-datasets-for-machine-learning-and-data-science-sources-and-advice-on-the-choice-636a0e754052).\n\n## To learn more about vector similarity search, check out the following resources:\n\n- [Thanks to Milvus, Anyone Can Build a Search Engine for 1+ Billion Images](https://milvus.io/blog/Thanks-to-Milvus-Anyone-Can-Build-a-Vector-Database-for-1-Billion-Images.md)\n- [Milvus Was Built for Massive-Scale (Think Trillion) Vector Similarity Search](https://milvus.io/blog/Milvus-Was-Built-for-Massive-Scale-Think-Trillion-Vector-Similarity-Search.md)\n- [Accelerating Similarity Search on Really Big Data with Vector Indexing](https://zilliz.com/blog/Accelerating-Similarity-Search-on-Really-Big-Data-with-Vector-Indexing)\n- [Accelerating Similarity Search on Really Big Data with Vector Indexing (Part II)](https://zilliz.com/learn/index-overview-part-2)\n","title":"Vector Similarity Search Hides in Plain View","metaData":{}},{"id":"2020-12-23-Set-Up-Milvus-in-Google-Colaboratory-for-Easy-ML-Application-Building.md","author":"milvus","desc":"Google Colab makes developing and testing machine learning applications a breeze. Learn how to setup Milvus in Colab for better massive-scale vector data management.","canonicalUrl":"https://zilliz.com/blog/Set-Up-Milvus-in-Google-Colaboratory-for-Easy-ML-Application-Building","date":"2020-12-23T10:30:58.020Z","cover":"https://assets.zilliz.com/3_cbea41e9a6.jpg","tags":["Engineering"],"href":"/blog/2020-12-23-Set-Up-Milvus-in-Google-Colaboratory-for-Easy-ML-Application-Building.md","content":"\n# Set Up Milvus in Google Colaboratory for Easy ML Application Building\n\nTechnological progress is perpetually making artificial intelligence (AI) and machine-scale analytics more accessible and easier to use. The [proliferation](https://techcrunch.com/2019/01/12/how-open-source-software-took-over-the-world/?guccounter=1\u0026guce_referrer=aHR0cHM6Ly93d3cuZ29vZ2xlLmNvbS8\u0026guce_referrer_sig=AQAAAL_qokucWT-HjbiOznq2RlO5TG78V6UYf332FKnWl3_knuMo6t5HZvZkIHFL3fhYX0nLzM6V1lVVAK4G3BXZHENX3zCXXgggGt39L9HKde3BufW1-iM2oKm0NIav2fcqgxvfpvx_7EPGstI7c_n99stI9oJf9sdsRPTQ6Wnu7DYX) of open-source software, public datasets, and other free tools are primary forces driving this trend. By pairing two free resources, [Milvus](https://milvus.io/) and [Google Colaboratory](https://colab.research.google.com/notebooks/intro.ipynb#scrollTo=5fCEDCU_qrC0) (“Colab” for short), anyone can create powerful, flexible AI and data analytics solutions. This article provides instructions for setting up Milvus in Colab, as well as performing basic operations using the Python software development kit (SDK).\n\n**Jump to:**\n\n- [What is Milvus?](#what-is-milvus)\n- [What is Google Colaboratory?](#what-is-google-colaboratory)\n- [Getting started with Milvus in Google Colaboratory](#getting-started-with-milvus-in-google-colaboratory)\n- [Run basic Milvus operations in Google Colab with Python](#run-basic-milvus-operations-in-google-colab-with-python)\n- [Milvus and Google Colaboratory work beautifully together](#milvus-and-google-colaboratory-work-beautifully-together)\n\n### What is Milvus?\n\n[Milvus](https://milvus.io/) is an open-source vector similarity search engine that can integrate with widely adopted index libraries, including Faiss, NMSLIB, and Annoy. The platform also includes a comprehensive set of intuitive APIs. By pairing Milvus with artificial intelligence (AI) models, a wide variety of applications can be built including:\n\n- Image, video, audio, and semantic text search engines.\n- Recommendation systems and chatbots.\n- New drug development, genetic screening, and other biomedical applications.\n\n### What is Google Colaboratory?\n\n[Google Colaboratory](https://colab.research.google.com/notebooks/intro.ipynb#recent=true) is a product from the Google Research team that allows anyone to write and run python code from a web browser. Colab was built with machine learning and data analysis applications in mind, offers a free Jupyter notebook environment, syncs with Google Drive, and gives users access to powerful cloud computing resources (including GPUs). The platform supports many popular machine learning libraries and can be integrated with PyTorch, TensorFlow, Keras, and OpenCV.\n\n### Getting started with Milvus in Google Colaboratory\n\nAlthough Milvus recommends [using Docker](https://milvus.io/docs/v0.10.4/milvus_docker-cpu.md) to install and start the service, the current Google Colab cloud environment does not support Docker installation. Additionally, this tutorial aims to be as accessible as possible — and not everyone uses Docker. Install and start the system by [compiling Milvus’ source code](https://github.com/milvus-io/milvus/blob/master/DEVELOPMENT.md) to avoid using Docker.\n\n### Download Milvus’ source code and create a new notebook in Colab\n\nGoogle Colab comes with all supporting software for Milvus preinstalled, including required compilation tools GCC, CMake, and Git and drivers CUDA and NVIDIA, simplifying the installation and setup process for Milvus. To begin, download Milvus’ source code and create a new notebook in Google Colab:\n\n1. Download Milvus’ source code: Milvus_tutorial.ipynb.\n\n`Wget https://raw.githubusercontent.com/milvus-io/bootcamp/0.10.0/getting_started/basics/milvus_tutorial/Milvus_tutorial.ipynb`\n\n2. Upload Milvus’ source code to [Google Colab](https://colab.research.google.com/notebooks/intro.ipynb#recent=true) and create a new notebook.\n\n\n\n### Compile Milvus from source code\n\n#### Download Milvus source code\n\n`git clone -b 0.10.3 https://github.com/milvus-io/milvus.git`\n\n#### Install dependencies\n\n`% cd /content/milvus/core ./ubuntu_build_deps.sh./ubuntu_build_deps.sh`\n\n#### Build Milvus source code\n\n```\n% cd /content/milvus/core\n!ls\n!./build.sh -t Release\n# To build GPU version, add -g option, and switch the notebook settings with GPU\n#((Edit -\u003e Notebook settings -\u003e select GPU))\n# !./build.sh -t Release -g\n```\n\n\u003e Note: If the GPU version is correctly compiled, a “GPU resources ENABLED!” notice appears.\n\n### Launch Milvus server\n\n#### Add lib/ directory to LD_LIBRARY_PATH:\n\n```\n% cd /content/milvus/core/milvus\n! echo $LD_LIBRARY_PATH\nimport os\nos.environ['LD_LIBRARY_PATH'] +=\":/content/milvus/core/milvus/lib\"\n! echo $LD_LIBRARY_PATH\n```\n\n#### Start and run Milvus server in the background:\n\n```\n% cd scripts\n! ls\n! nohup ./start_server.sh \u0026\n```\n\n#### Show Milvus server status:\n\n```\n! ls\n! cat nohup.out\n```\n\n\u003e Note: If the Milvus server is launched successfully, the following prompt appears:\n\n\n\n### Run basic Milvus operations in Google Colab with Python\n\nAfter successfully launching in Google Colab, Milvus can provide a variety of API interfaces for Python, Java, Go, Restful, and C++. Below are instructions for using the Python interface to perform basic Milvus operations in Colab.\n\n#### Install pymilvus:\n\n`! pip install pymilvus==0.2.14`\n\n#### Connect to the server:\n\n```\n# Connect to Milvus Server\nmilvus = Milvus(_HOST, _PORT)\n\n\n# Return the status of the Milvus server.\nserver_status = milvus.server_status(timeout=10)\n```\n\n#### Create a collection/partition/index:\n\n```\n# Information needed to create a collection\nparam={'collection_name':collection_name, 'dimension': _DIM, 'index_file_size': _INDEX_FILE_SIZE, 'metric_type': MetricType.L2}\n\n# Create a collection.\nmilvus.create_collection(param, timeout=10)\n\n# Create a partition for a collection.\nmilvus.create_partition(collection_name=collection_name, partition_tag=partition_tag, timeout=10)\nivf_param = {'nlist': 16384}\n\n# Create index for a collection.\nmilvus.create_index(collection_name=collection_name, index_type=IndexType.IVF_FLAT, params=ivf_param)\n```\n\n#### Insert and flush:\n\n```\n# Insert vectors to a collection.\nmilvus.insert(collection_name=collection_name, records=vectors, ids=ids)\n\n# Flush vector data in one collection or multiple collections to disk.\nmilvus.flush(collection_name_array=[collection_name], timeout=None)\n```\n\n#### Load and search:\n\n```\n# Load a collection for caching.\nmilvus.load_collection(collection_name=collection_name, timeout=None)\n\n# Search vectors in a collection.\nsearch_param = { \"nprobe\": 16 }\nmilvus.search(collection_name=collection_name,query_records=[vectors[0]],partition_tags=None,top_k=10,params=search_param)\n```\n\n#### Get collection/index information:\n\n```\n# Return information of a collection. milvus.get_collection_info(collection_name=collection_name, timeout=10)\n\n# Show index information of a collection. milvus.get_index_info(collection_name=collection_name, timeout=10)\n```\n\n#### Get vectors by ID:\n\n```\n# List the ids in segment\n# you can get the segment_name list by get_collection_stats() function.\nmilvus.list_id_in_segment(collection_name =collection_name, segment_name='1600328539015368000', timeout=None)\n\n# Return raw vectors according to ids, and you can get the ids list by list_id_in_segment() function.\nmilvus.get_entity_by_id(collection_name=collection_name, ids=[0], timeout=None)\n```\n\n#### Get/set parameters:\n\n```\n# Get Milvus configurations. milvus.get_config(parent_key='cache', child_key='cache_size')\n\n# Set Milvus configurations. milvus.set_config(parent_key='cache', child_key='cache_size', value='5G')\n```\n\n#### Delete index/vectors/partition/collection:\n\n```\n# Remove an index. milvus.drop_index(collection_name=collection_name, timeout=None)\n\n# Delete vectors in a collection by vector ID.\n# id_array (list[int]) -- list of vector id milvus.delete_entity_by_id(collection_name=collection_name, id_array=[0], timeout=None)\n\n# Delete a partition in a collection. milvus.drop_partition(collection_name=collection_name, partition_tag=partition_tag, timeout=None)\n\n# Delete a collection by name. milvus.drop_collection(collection_name=collection_name, timeout=10)\n```\n\n\u003cbr/\u003e\n\n### Milvus and Google Colaboratory work beautifully together\n\nGoogle Colaboratory is a free and intuitive cloud service that greatly simplifies compiling Milvus from source code and running basic Python operations. Both resources are available for anyone to use, making AI and machine learning technology more accessible to everyone. For more information about Milvus, check out the following resources:\n\n- For additional tutorials covering a wide variety of applications, visit [Milvus Bootcamp](https://github.com/milvus-io/bootcamp).\n- For developers interested in making contributions or leveraging the system, find [Milvus on GitHub](https://github.com/milvus-io/milvus).\n- For more information about the company that launched Milvus, visit [Zilliz.com](https://zilliz.com/).\n","title":"Set Up Milvus in Google Colaboratory for Easy ML Application Building","metaData":{}},{"id":"2020-12-01-graph-based-recommendation-system-with-milvus.md","author":"Shiyu Chen","desc":"Recommender systems can generate revenue, reduce costs, and offer a competitive advantage. Learn how to build one for free with open-source tools.","canonicalUrl":"https://zilliz.com/blog/graph-based-recommendation-system-with-milvus","date":"2020-12-01T21:41:08.582Z","cover":"https://assets.zilliz.com/thisisengineering_raeng_z3c_Mj_I6k_P_I_unsplash_2228b9411c.jpg","tags":["Scenarios"],"href":"/blog/2020-12-01-graph-based-recommendation-system-with-milvus.md","content":" \n# Building a Graph-based Recommendation System with Milvus, PinSage, DGL, and MovieLens Datasets\nRecommendation systems are powered by algorithms that have [humble beginnings](https://www.npr.org/2021/06/03/1002772749/the-rise-of-recommendation-systems-how-machines-figure-out-the-things-we-want) helping humans sift through unwanted email. In 1990, the inventor Doug Terry used a collaborative filtering algorithm to sort desirable email from junk mail. By simply \"liking\" or \"hating\" an email, in collaboration with others doing the same thing to similar mail content, users could quickly train computers to determine what to push through to a user's inbox—and what to sequester to the junk mail folder. \n\nIn a general sense, recommendation systems are algorithms that make relevant suggestions to users. Suggestions can be movies to watch, books to read, products to buy, or anything else depending on the scenario or industry. These algorithms are all around us, influencing the content we consume and the products we purchase from major tech companies such as Youtube, Amazon, Netflix and many more.\n\nWell designed recommendation systems can be essential revenue generators, cost reducers, and competitive differentiators. Thanks to open-source technology and declining compute costs, customized recommendation systems have never been more accessible. This article explains how to use Milvus, an open-source vector database; PinSage, a graph convolutional neural network (GCN); deep graph library (DGL), a scalable python package for deep learning on graphs; and MovieLens datasets to build a graph-based recommendation system.\n\n**Jump to:**\n- [How do recommendation systems work?](#how-do-recommendation-systems-work)\n- [Tools for building a recommender system](#tools-for-building-a-recommender-system)\n- [Building a graph-based recommender system with Milvus](#building-a-graph-based-recommender-system-with-milvus)\n\n## How do recommendation systems work?\n\nThere are two common approaches to building recommendation systems: collaborative filtering and content-based filtering. Most developers make use of either or both methods and, though recommendation systems can vary in complexity and construction, they typically include three core elements:\n\n1. **User model:** Recommender systems require modeling user characteristics, preferences, and needs. Many recommendation systems base their suggestion on implicit or explicit item-level input from users.\n2. **Object model:** Recommender systems also model items in order to make item recommendations based on user portraits.\n3. **Recommendation algorithm:** The core component of any recommendation system is the algorithm that powers its recommendations. Commonly used algorithms include collaborative filtering, implicit semantic modeling, graph-based modeling, combined recommendation, and more. \n\nAt a high level, recommender systems that rely on collaborative filtering build a model from past user behavior (including behavior inputs from similar users) to predict what a user might be interested in. Systems that rely on content-based filtering use discrete, predefined tags based on item characteristics to recommend similar items.\n\nAn example of collaborative filtering would be a personalized radio station on Spotify that is based on a user's listening history, interests, music library and more. The station plays music that the user hasn't saved or otherwise expressed interest in, but that other users with similar taste often have. A content-based filtering example would be a radio station based on a specific song or artist that uses attributes of the input to recommend similar music.\n\n## Tools for building a recommender system\n\nIn this example, building a graph-based recommendation system from scratch depends on the following tools:\n\n### Pinsage: A graph convolutional network\n\n[PinSage](https://medium.com/pinterest-engineering/pinsage-a-new-graph-convolutional-neural-network-for-web-scale-recommender-systems-88795a107f48) is a random-walk graph convolutional network capable of learning embeddings for nodes in web-scale graphs containing billions of objects. The network was developed by [Pinterest](https://www.pinterest.com/), an online pinboard company, to offer thematic visual recommendations to its users. \n\nPinterest users can \"pin\" content that interests them to \"boards,\" which are collections of pinned content. With over [478 million](https://business.pinterest.com/audience/) monthly active users (MAU) and over [240 billion](https://newsroom.pinterest.com/en/company) objects saved, the company has an immense amount of user data that it must build new technology to keep up with. \n\n\n\n\nPinSage uses pins-boards bipartite graphs to generate high-quality embeddings from pins that are used to recommend visually similar content to users. Unlike traditional GCN algorithms, which perform convolutions on the feature matrices and the full graph, PinSage samples the nearby nodes/Pins and performs more efficient local convolutions through dynamic construction of computational graphs.\n\nPerforming convolutions on the entire neighborhood of a node will result in a massive computational graph. To reduce resource requirements, traditional GCN algorithms update a node's representation by aggregating information from its k-hop neighborhood. PinSage simulates random-walk to set frequently visited content as the key neighborhood and then constructs a convolution based on it. \n\nBecause there is often overlap in k-hop neighborhoods, local convolution on nodes results in repeated computation. To avoid this, in each aggregate step PinSage maps all nodes without repeated calculation, then links them to the corresponding upper-level nodes, and finally retrieves the embeddings of the upper-level nodes.\n\n### Deep Graph Library: A scalable python package for deep learning on graphs\n\n\n\n[Deep Graph Library (DGL)](https://www.dgl.ai/) is a Python package designed for building graph-based neural network models on top of existing deep learning frameworks (e.g., PyTorch, MXNet, Gluon, and more). DGL includes a user friendly backend interface, making it easy to implant in frameworks based on tensors and that support automatic generation. The PinSage algorithm mentioned above is optimized for use with DGL and PyTorch.\n\n### Milvus: An open-source vector database built for AI and similarity search\n\n\n\nMilvus is an open-source vector database built to power vector similarity search and artificial intelligence (AI) applications. At a high level, Using Milvus for similarity search works as follows:\n1. Deep learning models are used to convert unstructured data to feature vectors, which are imported into Milvus.\n2. Milvus stores and indexes the feature vectors.\n3. Upon request, Milvus searches and returns vectors most similar to an input vector.\n\n## Building a graph-based recommendation system with Milvus\n\n\n\n\n\nBuilding a graph-based recommendation system with Milvus involves the following steps: \n\n### Step 1: Preprocess data\n\nData preprocessing involves turning raw data into a more easily understandable format. This example uses the open data sets MovieLens[5] (m1–1m), which contain 1,000,000 ratings of 4,000 movies contributed by 6,000 users. This data was collected by GroupLens and includes movie descriptions, movie ratings, and user characteristics.\n\nNote that the MovieLens datasets used in this example requires minimal data cleaning or organization. However, if you are using different datasets your mileage may vary.\n\nTo begin building a recommendation system, build a user-movie bipartite graph for classification purposes using historical user-movie data from the MovieLens dataset.\n\n graph_builder = PandasGraphBuilder()\n graph_builder.add_entities(users, 'user_id', 'user')\n graph_builder.add_entities(movies_categorical, 'movie_id', 'movie')\n graph_builder.add_binary_relations(ratings, 'user_id', 'movie_id', 'watched')\n graph_builder.add_binary_relations(ratings, 'movie_id', 'user_id', 'watched-by')\n g = graph_builder.build()\n\n### Step 2: Train model with PinSage\n\nEmbedding vectors of pins generated using the PinSage model are feature vectors of the acquired movie information. Create a PinSage model based on bipartite graph g and the customized movie feature vector dimensions (256-d by default). Then, train the model with PyTorch to obtain the h_item embeddings of 4,000 movies.\n\n # Define the model\n model = PinSAGEModel(g, item_ntype, textset, args.hidden_dims, args.num_layers).to(device)\n opt = torch.optim.Adam(model.parameters(), lr=args.lr)\n # Get the item embeddings\n for blocks in dataloader_test:\n for i in range(len(blocks)):\n blocks[i] = blocks[i].to(device)\n h_item_batches.append(model.get_repr(blocks))\n h_item = torch.cat(h_item_batches, 0)\n\n### Step 3: Load data\n\nLoad the movie embeddings h_item generated by the PinSage model into Milvus, which will return the corresponding IDs. Import the IDs and the corresponding movie information into MySQL.\n\n # Load data to Milvus and MySQL\n status, ids = milvus.insert(milvus_table, h_item)\n load_movies_to_mysql(milvus_table, ids_info)\n\n### Step 4: Conduct vector similarity search\nGet the corresponding embeddings in Milvus based on the movie IDs, then use Milvus to carry run similarity search with these embeddings. Next, identify the corresponding movie information in a MySQL database.\n\n # Get embeddings that users like\n _, user_like_vectors = milvus.get_entity_by_id(milvus_table, ids)\n # Get the information with similar movies\n _, ids = milvus.search(param = {milvus_table, user_like_vectors, top_k})\n sql = \"select * from \" + movies_table + \" where milvus_id=\" + ids + \";\"\n results = cursor.execute(sql).fetchall()\n\n### Step 5: Get recommendations\n\nThe system will now recommend movies most similar to user search queries. This is the general workflow for building a recommendation system. To quickly test and deploy recommender systems and other AI applications, try the Milvus [bootcamp](https://github.com/milvus-io/bootcamp). \n\n## Milvus can power more than recommender systems\n\nMilvus is a powerful tool capable of powering a vast array of artificial intelligence and vector similarity search applications. To learn more about the project, check out the following resources:\n\n- Read our [blog](https://zilliz.com/blog).\n- Interact with our open-source community on [Slack](https://join.slack.com/t/milvusio/shared_invite/zt-e0u4qu3k-bI2GDNys3ZqX1YCJ9OM~GQ).\n- Use or contribute to the world’s most popular vector database on [GitHub](https://github.com/milvus-io/milvus/).\n\n \n","title":"Building a Graph-based Recommendation System with Milvus, PinSage, DGL, and MovieLens Datasets","metaData":{}},{"id":"2020-11-11-Thanks-to-Milvus-Anyone-Can-Build-a-Vector-Database-for-1-Billion-Images.md","author":"milvus","desc":"AI and open-source software make it possible to build a reverse image search engine with just one server and 10 lines of code. Search 1+ billion images in real time and more with Milvus, an open-source vector data management platform.","canonicalUrl":"https://zilliz.com/blog/Thanks-to-Milvus-Anyone-Can-Build-a-Vector-Database-for-1-Billion-Images","date":"2020-11-11T07:13:02.135Z","cover":"https://assets.zilliz.com/build_search_9299109ca7.jpg","tags":["Scenarios"],"href":"/blog/2020-11-11-Thanks-to-Milvus-Anyone-Can-Build-a-Vector-Database-for-1-Billion-Images.md","content":"\n# Thanks to Milvus, Anyone Can Build a Vector Database for 1+ Billion Images\n\nRising compute power and declining compute costs have made machine-scale analytics and artificial intelligence (AI) more accessible than ever before. In practice, this means with just one server and 10 lines of code, it is possible to build a reverse image search engine capable of querying 1+ billion images in real time. This article explains how [Milvus](https://milvus.io/), an open-source vector data management platform, can be used to create powerful systems for unstructured data processing and analysis, as well as the underlying technology that makes this all possible.\n\n**Jump to:**\n\n- [Thanks to Milvus, Anyone Can Build a Vector Database for 1+ Billion Images](#thanks-to-milvus-anyone-can-build-a-vector-database-for-1-billion-images)\n - [How does AI enable unstructured data analytics?](#how-does-ai-enable-unstructured-data-analytics)\n - [Neural networks convert unstructured data into computer-friendly feature vectors](#neural-networks-convert-unstructured-data-into-computer-friendly-feature-vectors) - [_AI algorithms convert unstructured data to vectors_](#ai-algorithms-convert-unstructured-data-to-vectors)\n - [What are vector data management platforms?](#what-are-vector-data-management-platforms)\n - [What are limitations of existing approaches to vector data management?](#what-are-limitations-of-existing-approaches-to-vector-data-management) - [_An overview of Milvus’ architecture._](#an-overview-of-milvus-architecture)\n - [What are applications for vector data management platforms and vector similarity search?](#what-are-applications-for-vector-data-management-platforms-and-vector-similarity-search)\n - [Reverse image search](#reverse-image-search) - [_Google’s “search by image” feature._](#googles-search-by-image-feature)\n - [Video recommendation systems](#video-recommendation-systems)\n - [Natural language processing (NLP)](#natural-language-processing-nlp)\n - [Learn more about Milvus](#learn-more-about-milvus)\n\n### How does AI enable unstructured data analytics?\n\nAn oft-cited statistic is that 80% of the world’s data is unstructured, but just 1% ever gets analyzed. Unstructured data, including images, video, audio, and natural language, doesn’t follow a predefined model or manner of organization. This makes processing and analyzing large unstructured datasets difficult. As the proliferation of smartphones and other connected devices pushes unstructured data production to new heights, businesses are increasingly aware of how important insights derived from this nebulous information can be.\n\nFor decades, computer scientists have developed indexing algorithms tailored for organizing, searching, and analyzing specific data types. For structured data, there is bitmap, hash tables, and B-tree, which are commonly used in relational databases developed by tech giants such as Oracle and IBM. For semi-structured data, inverted indexing algorithms are standard, and can be found in popular search engines like [Solr](http://www.solrtutorial.com/basic-solr-concepts.html) and [ElasticSearch](https://www.elastic.co/blog/found-elasticsearch-from-the-bottom-up#inverted-indexes-and-index-terms). However, unstructured data indexing algorithms rely on compute-intensive artificial intelligence that has only become widely accessible in the past decade.\n\n### Neural networks convert unstructured data into computer-friendly feature vectors\n\nUsing neural networks (e.g. [CNN](https://en.wikipedia.org/wiki/Convolutional_neural_network), [RNN](https://en.wikipedia.org/wiki/Recurrent_neural_network), and [BERT](https://towardsdatascience.com/bert-explained-state-of-the-art-language-model-for-nlp-f8b21a9b6270)) unstructured data can be converted into feature vectors (a.k.a., embeddings), which are a string of integers or floats. This numerical data format is far more readily processed and analyzed by machines. Applications spanning reverse image search, video search, natural language processing (NLP) and more can be built by embedding unstructured data into feature vectors, then calculating similarity between vectors using measures like Euclidean distance or cosine similarity.\n\n\n\n\nComputing vector similarity is a relatively simple process that relies on established algorithms. However, unstructured datasets, even after being converted into feature vectors, are typically several orders of magnitude larger than traditional structured and semi-structured datasets. Vector similarity search is complicated by the sheer storage space and compute power required to efficiently and accurately query massive-scale unstructured data.\nHowever, if some degree of accuracy can be sacrificed, there are various approximate nearest neighbor (ANN) search algorithms that can drastically improve query efficiency for massive datasets with high dimensionality. These ANN algorithms decrease storage requirements and computation load by clustering similar vectors together, resulting in faster vector search. Commonly used algorithms include tree-based, graph-based, and combined ANNs.\n\n### What are vector data management platforms?\n\nVector data management platforms are purpose-built applications for storing, processing, and analyzing massive vector datasets. These tools are designed to easily interface with large amounts of data, and include functionality that streamlines vector data management. Unfortunately, few systems exist that are both flexible and powerful enough to solve modern big data challenges. Milvus, a vector data management platform initiated by [Zilliz](https://zilliz.com/) and released under an open-source license in 2019, attempts to fill this void.\n\n### What are limitations of existing approaches to vector data management?\n\nA common way to build an unstructured data analytics system is to pair algorithms like ANN with open-source implementation libraries such as Facebook AI Similarity Search (Faiss). Due to several limitations, these algorithm-library combinations are not equivalent to a full-fledged vector data management system like Milvus. Existing technology used for managing vector data faces the following problems:\n\n1. **Flexibility:** By default, existing systems typically store all data in main memory, meaning they cannot be run across multiple machines and are poorly suited for handling massive datasets.\n2. **Dynamic data handling:** Data is often assumed to be static once fed into existing systems, complicating processing for dynamic data and making near real-time search impossible.\n3. **Advanced query processing:** Most tools do not support advanced query processing (e.g., attribute filtering and multi-vector queries), which is essential for building useful similarity search engines.\n4. **Heterogeneous computing optimizations:** Few platforms offer optimizations for heterogenous system architectures on both CPUs and GPUs (excluding Faiss), leading to efficiency losses.\n\nMilvus attempts to overcome all of these limitations. The system enhances flexibility by offering support for a variety of application interfaces (including SDKs in Python, Java, Go, C++ and RESTful APIs), multiple vector index types (e.g., quantization-based indexes and graph-based indexes), and advanced query processing. Milvus handles dynamic vector data using a log-structured merge-tree (LSM tree), keeping data insertions and deletions efficient and searches humming along in real time. Milvus also provides optimizations for heterogeneous computing architectures on modern CPUs and GPUs, allowing developers to adjust systems for specific scenarios, datasets, and application environments.\n\n\n\n\nUsing various ANN indexing techniques, Milvus is able to achieve a 99% top-5 recall rate. The system is also capable of loading 1+ million data entries per minute. This results in query time of less than one second when running a reverse image search on 1 billion images. As a cloud native application that can operate as a distributed system deployed across multiple nodes, Milvus can easily and reliably achieve similar performance on datasets that contain 10, or even 100, billion images. Additionally, the system is not limited to image data, with applications spanning computer vision, conversational AI, recommendation systems, new drug discovery, and more.\n\n### What are applications for vector data management platforms and vector similarity search?\n\nAs outlined above, a capable vector data management platform like Milvus paired with approximate nearest neighbor algorithms enables similarity search on gigantic volumes of unstructured data. This technology can be used to develop applications that span a diverse array of fields. Below we briefly explain several common use cases for vector data management tools and vector similarity search.\n\n### Reverse image search\n\nMajor search engines like Google already give users the option to search by image. Additionally, e-commerce platforms have realized the benefits this functionality offers online shoppers, with Amazon incorporating image search into its smartphone applications.\n\n\n\n\nOpen-source software like Milvus makes it possible for any business to create their own reverse image search system, lowering the barriers to entry for this increasingly in-demand feature. Developers can use pre-trained AI models to convert their own image datasets into vectors, and then leverage Milvus to enable searching for similar products by image.\n\n#### Video recommendation systems\n\nMajor online video platforms like YouTube, which receives [500 hours of user generated content each minute](https://www.tubefilter.com/2019/05/07/number-hours-video-uploaded-to-youtube-per-minute/), present unique demands when it comes to content recommendation. In order to make relevant, real-time recommendations that take into consideration new uploads, video recommendation systems must offer lightning-fast query time and efficient dynamic data handling. By converting key frames into vectors and then feeding the results into Milvus, billions of videos can be searched and recommended in near real time.\n\n#### Natural language processing (NLP)\n\nNatural language processing is a branch of artificial intelligence that aims to build systems that can interpret human languages. After converting text data into vectors, Milvus can be used to quickly identify and remove duplicate text, power semantic search, or even [build an intelligent writing assistant](https://medium.com/unstructured-data-service/how-artificial-intelligence-empowered-professional-writing-f433c7e5b561%22%20/). An effective vector data management platform helps maximize the utility of any NLP system.\n\n### Learn more about Milvus\n\nIf you would like to learn more about Milvus visit our [website](https://milvus.io/). Additionally, our [bootcamp](https://github.com/milvus-io/bootcamp) offers several tutorials, with instructions for setting up Milvus, benchmark testing, and building a variety of different applications. If you’re interested in vector data management, artificial intelligence, and big data challenges, please join our open-source community on [GitHub](https://github.com/milvus-io) and chat with us on [Slack](https://join.slack.com/t/milvusio/shared_invite/zt-e0u4qu3k-bI2GDNys3ZqX1YCJ9OM~GQ).\n\nWant more information about building an image search system? Check out this case study:\n\n- [The Journey to Optimizing Billion-scale Image Search (1/2)](https://medium.com/vector-database/the-journey-to-optimize-billion-scale-image-search-part-1-a270c519246d)\n- [The Journey to Optimizing Billion-scale Image Search (2/2)](https://medium.com/unstructured-data-service/the-journey-to-optimizing-billion-scale-image-search-2-2-572a36d5d0d)\n","title":"Thanks to Milvus, Anyone Can Build a Vector Database for 1+ Billion Images","metaData":{}},{"id":"2020-11-10-milvus-performance-AVX-512-vs-AVX2.md","author":"milvus","desc":"Discover how Milvus performs on AVX-512 vs. AVX2 using a variety of different vector indexes.","canonicalUrl":"https://zilliz.com/blog/milvus-performance-AVX-512-vs-AVX2","date":"2020-11-10T22:15:39.156Z","cover":"https://assets.zilliz.com/header_milvus_performance_avx_512_vs_avx2_2c9f14ef96.png","tags":["Engineering"],"href":"/blog/2020-11-10-milvus-performance-AVX-512-vs-AVX2.md","content":" \n# Milvus performance on AVX-512 vs. AVX2\nConscious intelligent machines that want to take over the world are a steady fixture in science fiction, but in reality modern computers are very obedient. Without being told, they seldom know what to do with themselves. Computers perform tasks based on instructions, or orders, sent from a program to a processor. At their lowest level, each instruction is a sequence of ones and zeroes that describes an operation for a computer to execute. \nTypically, in computer assembly languages each machine language statement corresponds to a processor instruction. The central processing unit (CPU) relies on instructions to perform calculations and control systems. Additionally, CPU performance is often measured in terms of instruction execution capability (e.g., execution time).\n\n## What are Advanced Vector Extensions?\nAdvanced Vector Extensions (AVX) are an instruction set for microprocessors that rely on the x86 family of instruction set architectures. First proposed by Intel in March 2008, AVX saw broad support three years later with the launch of Sandy Bridge—a microarchitecture used in the second generation of Intel Core processors (e.g, Core i7, i5, i3)— and AMD's competing microarchitecture also released in 2011, Bulldozer.\n\nAVX introduced a new coding scheme, new features, and new instructions. AVX2 expands most integer operations to 256 bits and introduces fused multiply-accumulate (FMA) operations. AVX-512 expands AVX to 512-bit operations using a new enhanced vector extension (EVEX) prefix encoding.\n\n[Milvus](https://milvus.io/docs) is an open-source vector database designed for similarity search and artificial intelligence (AI) applications. The platform supports the AVX-512 instruction set, meaning it can be used with all CPUs that include the AVX-512 instructions. Milvus has broad applications spanning recommender systems, computer vision, natural language processing (NLP) and more. This article presents performance results and analysis of a Milvus vector database on AVX-512 and AVX2.\n\n## Milvus performance on AVX-512 vs. AVX2\n### System configuration\n- CPU: Intel(R) Platinum 8163 CPU @ 2.50GHz24 cores 48 threads\n- Number of CPU: 2\n- Graphics card, GeForce RTX 2080Ti 11GB 4 cards\n- Mem: 768GB\n- Disk: 2TB SSD\n\n### Milvus parameters\n- cahce.cahe_size: 25, The size of CPU memory used for caching data for faster query.\n- nlist: 4096\n- nprobe: 128\n\nNote: `nlist` is the indexing parameter to create from the client; `nprobe` the searching parameter. Both IVF_FLAT and IVF_SQ8 use a clustering algorithm to partition a large number of vectors into buckets, `nlist` being the total number of buckets to partition during clustering. The first step in a query is to find the number of buckets that are closest to the target vector, and the second step is to find the top-k vectors in these buckets by comparing the distance of the vectors. `nprobe` refers to the number of buckets in the first step.\n\n### Dataset: SIFT10M dataset\nThese tests use the [SIFT10M dataset](https://archive.ics.uci.edu/ml/datasets/SIFT10M), which contains one million 128-dimensional vectors and is often used for analyzing the performance of corresponding nearest-neighbor search methods. The top-1 search time for nq = [1, 10, 100, 500, 1000] will be compared between the two instruction sets.\n\n### Results by vector index type\n[Vector indexes](https://zilliz.com/blog/Accelerating-Similarity-Search-on-Really-Big-Data-with-Vector-Indexing) are time- and space-efficient data structures built on the vector field of a collection using various mathematical models. Vector indexing allows large datasets to be searched efficiently when trying to identify similar vectors to an input vector. Due to the time consuming nature of accurate retrieval, most of the index types [supported by Milvus](https://milvus.io/docs/v2.0.x/index.md#CPU) use approximate nearest neighbor (ANN) search.\n\nFor these tests, three indexes were used with AVX-512 and AVX2: IVF_FLAT, IVF_SQ8, and HNSW.\n\n### IVF_FLAT\nInverted file (IVF_FLAT) is an index type based on quantization. It is the most basic IVF index, and the encoded data stored in each unit is consistent with the original data. \nThe index divides vector data into a number of cluster units (nlist), and then compares distances between the target input vector and the center of each cluster. Depending on the number of clusters the system is set to query (nprobe), similarity search results are returned based on comparisons between the target input and the vectors in the most similar cluster(s) only — drastically reducing query time. By adjusting nprobe, an ideal balance between accuracy and speed can be found for a given scenario. \n\n**Performance results**\n\n\n### IVF_SQ8\n\nIVF_FLAT does not perform any compression, so the index files it produces are roughly the same size as the original, raw non-indexed vector data. When disk, CPU, or GPU memory resources are limited, IVF_SQ8 is a better option than IVF_FLAT. \nThis index type can convert each dimension of the original vector from a four-byte floating-point number to a one-byte unsigned integer by performing scalar quantization. This reduces disk, CPU, and GPU memory consumption by 70–75%.\n\n**Performance results**\n\n\n### HNSW\nHierarchical Small World Graph (HNSW) is a graph-based indexing algorithm. Queries begin in the uppermost layer by finding the node closest to the target, it then goes down to the next layer for another round of search. After multiple iterations, it can quickly approach the target position.\n\n**Performance results**\n\n\n## Comparing vector indexes\nVector retrieval is consistently faster on the AVX-512 instruction set than on AVX2. This is because AVX-512 supports 512-bit computation, compared to just 256-bit computation on AVX2. Theoretically, AVX-512 should be twice as fast as the AVX2 however, Milvus conducts other time-consuming tasks in addition to vector similarity calculations. The overall retrieval time of AVX-512 is unlikely to be twice as short as AVX2 in real-world scenarios.\n\n\nRetrieval is significantly faster on the HNSW index than the other two indexes, while IVF_SQ8 retrieval is slightly faster than IVF_FLAT on both instruction sets. This is likely because IVF_SQ8 requires just 25% of the memory need by IVF_FLAT. IVF_SQ8 loads 1 byte for each vector dimension, while IVF_FLAT loads 4 bytes per vector dimension. The time required for the calculation is most likely constrained by memory bandwidth. As a result, IVF_SQ8 not only takes up less space, but also requires less time to retrieve vectors.\n\n## Milvus is a versatile, high-performance vector database \n\nThe tests presented in this article demonstrate that Milvus offers excellent performance on both the AVX-512 and AVX2 instruction sets using different indexes. Regardless of the index type, Milvus performs better on AVX-512.\n\nMilvus is compatible with a variety of deep learning platforms and is used in miscellaneous AI applications. [Milvus 2.0](https://zilliz.com/news/lfaidata-launches-milvus-2.0-an-advanced-cloud-native-vector-database-built-for-ai), a reimagined version of the world's most popular vector database, was released under an open-source license in July 2021. For more information about the project, check out the following resources:\n- Find or contribute to Milvus on [GitHub](https://github.com/milvus-io/milvus/).\n- Interact with the community via [Slack](https://join.slack.com/t/milvusio/shared_invite/zt-e0u4qu3k-bI2GDNys3ZqX1YCJ9OM~GQ).\n- Connect with us on [Twitter](https://twitter.com/milvusio).\n\n \n","title":"Milvus performance on AVX-512 vs. AVX2","metaData":{}},{"id":"2020-09-11-ArtLens-AI-Share-Your-View.md","author":"Anna Faxon and Haley Kedziora","desc":"Artificial Intelligence Offers a New Way to Find Your View in the CMA Collection","canonicalUrl":"https://zilliz.com/blog/ArtLens-AI-Share-Your-View","date":"2020-09-11T06:55:00.939Z","cover":"https://assets.zilliz.com/Blog_Art_Lens_AI_1_a7b1054511.jpeg","tags":["Scenarios"],"href":"/blog/2020-09-11-ArtLens-AI-Share-Your-View.md","content":" \n# ArtLens AI: Share Your View\n\n\u003cbr/\u003e\n\nArt is one way people reflect and shape the world they see, and the Cleveland Museum of Art has a new way to match your view to views created by some of history’s greatest artists.\n\nThe CMA’s Digital Innovation team has launched [ArtLens AI: Share Your View](https://www.clevelandart.org/art/collection/share-your-view). This interactive tool on the museum website and on [Twitter](https://twitter.com/ArtlensAI) matches what you see in your world to art from the CMA’s remarkable collection.\n\nThis easy point of entry into the CMA answers the fatigue of people learning new technologies to stay connected to friends, family, and co-workers. We also wanted to give the community a resource in which— without previous knowledge of history or art theory — someone can make visual connections between their world and a work of art at the click of a button.\n\nThe reverse image search tool uses [Artificial Intelligence (AI)](https://en.wikipedia.org/wiki/Artificial_intelligence) to recognize the shapes, patterns, and objects in your own photos to find surprising and delightful matches from the CMA’s encyclopedic collection. The tool can even recognize famous architecture in your photo.\n\n, 1910. Mortimer Menpes (British, 1860–1938). Etching; sheet: 44.7 x 28 cm. The Cleveland Museum of Art, Gift of Carole W. and Charles B. Rosenblatt, 1996.221. Image courtesy of the Cleveland Museum of Art.\")\n\n\n\u003cbr/\u003e\n\nWhen making a match on the website, you can shuffle through other close matches, download a composite image to share with your friends, or add the image to our visitor gallery. Links to the CMA’s [Collection Online](https://www.clevelandart.org/art/collection/search) offer you more than 36 fields of metadata about a work of art, such as an artist biography, citations, and curator videos. On [Twitter](https://twitter.com/ArtlensAI), attach a photo or an image to your tweet and mention @ArtLensAI to share your view. The Twitter bot will automatically reply with a matching artwork.\n\n\n\n\u003cbr/\u003e\n\n. Francis Seymour Haden (British, 1818–1910). Etching; The Cleveland Museum of Art, Gift of Mrs. T. Wingate Todd from the Collection of Dr. T. Wingate Todd, 1961.300. Image courtesy of the Cleveland Museum of Art.\")\n\n\n\u003cbr/\u003e\n\nUsing Machine Learning and the open source vector similarity engine [Milvus](https://milvus.io/), the CMA’s Digital Innovation development team created the AI tool with [Design IO](https://www.design-io.com/), who had previously collaborated on the museum’s [ArtLens Studio](https://www.clevelandart.org/artlens-gallery/artlens-studio) in 2016, and Jeff Schuler, a partner who leads the CMA team on website development. The new initiative builds on AI used in the museum’s on-site ArtLens Exhibition to read emotion and match a visitor’s face to portraits in the CMA’s collection.\n\nWe hope this will be another source of inspiration and fun in these uncertain times. We know that people need new, creative, and easy ways to engage with art. The CMA has grown as a digital museum during the COVID-19 pandemic, with many new gifts to the community. But CMA staffers feel the “Zoom fatigue” of remote work and video conferencing as much as anyone else after six months of self-isolation. Share Your View is one answer to the cautious steps we all are taking into a hybrid experience of life online and life in safe physical spaces.\n\nArtLens AI: Share Your View can help students, teachers, and parents feeling drained by the continuation of virtual learning. Schools in hybrid mode still rely on video calls and online platforms for classroom engagement. College and high school students are learning from their homes and dorms, without access to on-campus resources. The CMA’s education team has already begun to share this tool as a way to spark conversations about composition, palette, and pattern. Placing an artwork side-by-side with a contemporary view creates new comparisons and opportunities for critical thinking.\n\n, 1885. Odilon Redon (French, 1840–1916), printed by Lemercier \u0026 Cie.. Lithograph on China paper laid on wove paper; image: 29.1 x 23.9 cm. The Cleveland Museum of Art, Gift of The Print Club of Cleveland, 1927.344.1. Image courtesy of the Cleveland Museum of Art.\")\n\n\u003cbr/\u003e\n\nSince the launch of [ARTLENS Gallery](https://www.clevelandart.org/artlens-gallery) in 2012, the CMA has been a leader in creating digital connections to art. This year we have added many [new curator videos](https://www.clevelandart.org/home-where-art-video-series) for online viewing and created the [ArtLens for Slack app](https://www.clevelandart.org/artlens-for-slack) so workplaces can share a daily moment of discussion and interaction centered around artwork in the museum’s [Open Access](https://www.clevelandart.org/open-access) collection. We hope the new AI feature will help you find yourself in some surprising and inspiring places inside some of the most beautiful art in the world.\n\n, 1882. William James Stillman (American, 1828–1901). Albumen print from gelatin dry plate negative; image: 38.2 x 36.7 cm. The Cleveland Museum of Art, John L. Severance Fund, 1993.34. Image courtesy of the Cleveland Museum of Art.\")\n\n\u003cbr/\u003e\n\n*This blog article is reposted from https://medium.com/cma-thinker/artlens-ai-share-your-view-5a326c15943f*\n\nRead other [user stories](https://zilliz.com/user-stories) to learn more about making things with Milvus.\n\n\n\n ","title":"ArtLens AI Share Your View","metaData":{}},{"id":"2020-09-08-music-recommender-system-item-based-collaborative-filtering-milvus.md","author":"milvus","desc":"A case study with WANYIN APP","canonicalUrl":"https://zilliz.com/blog/music-recommender-system-item-based-collaborative-filtering-milvus","date":"2020-09-08T00:01:59.064Z","cover":"https://assets.zilliz.com/header_f8cea596d2.png","tags":["Scenarios"],"href":"/blog/2020-09-08-music-recommender-system-item-based-collaborative-filtering-milvus.md","content":" \n# Item-based Collaborative Filtering for Music Recommender System\nWanyin App is an AI-based music sharing community with an intention to encourage music sharing and make music composition easier for music enthusiasts.\n\nWanyin’s library contains a massive amount of music uploaded by users. The primary task is to sort out the music of interest based on users’ previous behavior. We evaluated two classic models: user-based collaborative filtering (User-based CF) and item-based collaborative filtering (Item-based CF), as the potential recommender system models.\n\n- User-based CF uses similarity statistics to obtain neighboring users with similar preferences or interests. With the retrieved set of nearest neighbors, the system can predict the interest of the target user and generate recommendations.\n- Introduced by Amazon, item-based CF, or item-to-item (I2I) CF, is a well-known collaborative filtering model for recommender systems. It calculates similarities between items instead of users, based on the assumption that items of interest must be similar to the items of high scores.\n\nUser-based CF may lead to prohibitively longer time for calculation when the user number passes a certain point. Taking the characteristics of our product into consideration, we decided to go with I2I CF to implement the music recommender system. Given that we do not possess much metadata about the songs, we have to deal with the songs per se, extracting feature vectors (embeddings) from them. Our approach is to convert these songs into mel-frequency cepstrum (MFC), design a convolutional neural network (CNN) to extract the songs’ feature embeddings, and then make music recommendations through embedding similarity search.\n\n## 🔎 Select an embedding similarity search engine\n\nNow that we have feature vectors, the remaining issue is how to retrieve from the large volume of vectors the ones that are similar to the target vector. When it comes to embeddings search engine, we were weighing between Faiss and Milvus. I noticed Milvus when I was going through GitHub’s trending repositories in November, 2019. I took a look at the project and it appealed to me with its abstract APIs. (It was on v0.5.x by then and v0.10.2 by now.)\n\nWe prefer Milvus to Faiss. On the one hand, we have used Faiss before, and hence would like to try something new. One the other hand, compared to Milvus, Faiss is more of an underlying library, therefore not quite convenient to use. As we learned more about Milvus, we finally decided to adopt Milvus for its two main features:\n\n- Milvus is very easy to use. All you need to do is to pull its Docker image and update the parameters based on your own scenario.\n- It supports more indexes and has detailed supporting documentation.\n\nIn a nutshell, Milvus is very friendly to users and the documentation is quite detailed. If you come across any problem, you can usually find solutions in the documentation; otherwise, you can always get support from the Milvus community.\n\n## Milvus cluster service ☸️ ⏩\n\nAfter deciding to use Milvus as the feature vector search engine, we configured a standalone node in a development (DEV) environment. It had been running well for a few days, so we planned to run tests in a factory acceptance test (FAT) environment. If a standalone node crashed in production, the entire service would become unavailable. Thus, we need to deploy a highly available search service.\n\nMilvus provides both Mishards, a cluster sharding middleware, and Milvus-Helm for configuration. The process of deploying a Milvus cluster service is simple. We only need to update some parameters and pack them for deployment in Kubernetes. The diagram below from Milvus’ documentation shows how Mishards works:\n\n\n\nMishards cascades a request from upstream down to its sub-modules splitting the upstream request, and then collects and returns the results of the sub-services to upstream. The overall architecture of the Mishards-based cluster solution is shown below:\n\n\n\nThe official documentation provides a clear introduction of Mishards. You can refer to [Mishards](https://milvus.io/cn/docs/v0.10.2/mishards.md) if you are interested.\n\nIn our music recommender system, we deployed one writable node, two read-only nodes, and one Mishards middleware instance in Kubernetes, using Milvus-Helm. After the service had been running stably in a FAT environment for a while, we deployed it in production. It has been stable so far.\n\n## 🎧 I2I music recommendation 🎶\n\nAs mentioned above, we built Wanyin’s I2I music recommender system using the extracted embeddings of the existing songs. First, we separated the vocal and the BGM (track separation) of a new song uploaded by the user and extracted the BGM embeddings as the feature representation of the song. This also helps sort out cover versions of original songs. Next, we stored these embeddings in Milvus, searched for similar songs based on the songs that the user listened to, and then sorted and rearranged the retrieved songs to generate music recommendations. The implementation process is shown below:\n\n\n\n## 🚫 Duplicate song filter\n\nAnother scenario in which we use Milvus is duplicate song filtering. Some users upload the same song or clip several times, and these duplicate songs may appear in their recommendation list. This means that it would affect user experience to generate recommendations without pre-processing. Therefore, we need to find out the duplicate songs and ensure that they do not appear on the same list through pre-processing.\n\nAnother scenario in which we use Milvus is duplicate song filtering. Some users upload the same song or clip several times, and these duplicate songs may appear in their recommendation list. This means that it would affect user experience to generate recommendations without pre-processing. Therefore, we need to find out the duplicate songs and ensure that they do not appear on the same list through pre-processing.\n\nSame with the previous scenario, we implemented duplicate song filtering by means of searching for similar feature vectors. First, we separated the vocal and the BGM and retrieved a number of similar songs using Milvus. In order to filter duplicate songs accurately, we extracted the audio fingerprints of the target song and the similar songs (with technologies such as Echoprint, Chromaprint, etc.), calculated the similarity between the audio fingerprint of the target song with each of the similar songs’ fingerprints. If the similarity goes beyond the threshold, we define a song as a duplicate of the target song. The process of audio fingerprint matching makes the filtering of duplicate songs more accurate, but it is also time-consuming. Therefore, when it comes to filtering songs in a massive music library, we use Milvus to filter our candidate duplicate songs as a preliminary step.\n\n\n\nTo implement the I2I recommender system for Wanyin’s massive music library, our approach is to extract the embeddings of songs as their feature, recall similar embeddings to the embedding of the target song, and then sort and rearrange the results to generate recommendation lists for the user. To achieve real-time recommendation, we choose Milvus over Faiss as our feature vector similarity search engine, since Milvus proves to be more user-friendly and sophisticated. By the same token, we have also applied Milvus to our duplicate song filter, which improves user experience and efficiency.\n\nYou can download [Wanyin App](https://enjoymusic.ai/wanyin) 🎶 and try it out. (Note: might not be available on all app stores.)\n\n### 📝 Authors:\n\nJason, Algorithm Engineer at Stepbeats\nShiyu Chen, Data Engineer at Zilliz\n\n### 📚 References:\n\nMishards Docs: https://milvus.io/docs/v0.10.2/mishards.md\nMishards: https://github.com/milvus-io/milvus/tree/master/shards\nMilvus-Helm: https://github.com/milvus-io/milvus-helm/tree/master/charts/milvus\n\n**🤗 Don’t be a stranger, follow us on [Twitter](https://twitter.com/milvusio/) or join us on [Slack](https://milvusio.slack.com/join/shared_invite/zt-e0u4qu3k-bI2GDNys3ZqX1YCJ9OM~GQ#/)!👇🏻**\n ","title":"Item-based Collaborative Filtering for Music Recommender System","metaData":{}},{"id":"2020-08-29-building-video-search-system-with-milvus.md","author":"milvus","desc":"Searching for videos by image with Milvus","canonicalUrl":"https://zilliz.com/blog/building-video-search-system-with-milvus","date":"2020-08-29T00:18:19.703Z","cover":"https://assets.zilliz.com/header_3a822736b3.gif","tags":["Scenarios"],"href":"/blog/2020-08-29-building-video-search-system-with-milvus.md","content":" \n# 4 Steps to Building a Video Search System\nAs its name suggests, searching for videos by image is the process of retrieving from the repository videos containing similar frames to the input image. One of the key steps is to turn videos into embeddings, which is to say, extract the key frames and convert their features to vectors. Now, some curious readers might wonder what the difference is between searching for video by image and searching for an image by image? In fact, searching for the key frames in videos is equivalent to searching for an image by image.\n\nYou can refer to our previous article [Milvus x VGG: Building a Content-based Image Retrieval System](https://medium.com/unstructured-data-service/milvus-application-1-building-a-reverse-image-search-system-based-on-milvus-and-vgg-aed4788dd1ea) if interested.\n\n## System overview\n\nThe following diagram illustrates the typical workflow of such a video search system.\n\n\n\nWhen importing videos, we use the OpenCV library to cut each video into frames, extract vectors of the key frames using image feature extraction model VGG, and then insert the extracted vectors (embeddings) into Milvus. We use Minio for storing the original videos and Redis for storing correlations between videos and vectors.\n\nWhen searching for videos, we use the same VGG model to convert the input image into a feature vector and insert it into Milvus to find vectors with the most similarity. Then, the system retrieves the corresponding videos from Minio on its interface according to the correlations in Redis.\n\n## Data preparation\n\nIn this article, we use about 100,000 GIF files from Tumblr as a sample dataset in building an end-to-end solution for searching for video. You can use your own video repositories.\n\n## Deployment\n\nThe code for building the video retrieval system in this article is on GitHub.\n\n### Step 1: Build Docker images.\n\nThe video retrieval system requires Milvus v0.7.1 docker, Redis docker, Minio docker, the front-end interface docker, and the back-end API docker. You need to build the front-end interface docker and the back-end API docker by yourself, while you can pull the other three dockers directly from Docker Hub.\n\n # Get the video search code\n $ git clone -b 0.10.0 https://github.com/JackLCL/search-video-demo.git\n\n # Build front-end interface docker and api docker images\n $ cd search-video-demo \u0026 make all\n\n## Step 2: Configure the environment.\n\nHere we use docker-compose.yml to manage the above-mentioned five containers. See the following table for the configuration of docker-compose.yml:\n\n\n\nThe IP address 192.168.1.38 in the table above is the server address especially for building the video retrieval system in this article. You need to update it to your server address.\n\nYou need to manually create storage directories for Milvus, Redis, and Minio, and then add the corresponding paths in docker-compose.yml. In this example, we created the following directories:\n\n /mnt/redis/data /mnt/minio/data /mnt/milvus/db\n\nYou can configure Milvus, Redis, and Minio in docker-compose.yml as follows:\n\n\n\n## Step 3: Start the system.\n\nUse the modified docker-compose.yml to start up the five docker containers to be used in the video retrieval system:\n\n $ docker-compose up -d\n\nThen, you can run docker-compose ps to check whether the five docker containers have started up properly. The following screenshot shows a typical interface after a successful startup.\n\n\n\nNow, you have successfully built a video search system, though the database has no videos.\n\n## Step 4: Import videos.\n\nIn the deploy directory of the system repository, lies import_data.py, script for importing videos. You only need to update the path to the video files and the importing interval to run the script.\n\n\n\ndata_path: The path to the videos to import.\n\ntime.sleep(0.5): The interval at which the system imports videos. The server that we use to build the video search system has 96 CPU cores. Therefore, it is recommended to set the interval to 0.5 second. Set the interval to a greater value if your server has fewer CPU cores. Otherwise, the importing process will put a burden on the CPU, and create zombie processes.\n\nRun import_data.py to import videos.\n\n $ cd deploy\n $ python3 import_data.py\n\nOnce the videos are imported, you are all set with your own video search system!\n\n## Interface display\n\nOpen your browser and enter 192.168.1.38:8001 to see the interface of the video search system as shown below.\n\n\n\nToggle the gear switch in the top right to view all videos in the repository.\n\n\n\nClick on the upload box on the top left to input a target image. As shown below, the system returns videos containing the most similar frames.\n\n\n\nNext, have fun with our video search system!\n\n## Build your own\n\nIn this article, we used Milvus to build a system for searching for videos by images. This exemplifies the application of Milvus in unstructured data processing.\n\nMilvus is compatible with multiple deep learning frameworks, and it makes possible searches in milliseconds for vectors at the scale of billions. Feel free to take Milvus with you to more AI scenarios: https://github.com/milvus-io/milvus.\n\nDon’t be a stranger, follow us on [Twitter](https://twitter.com/milvusio/) or join us on [Slack](https://milvusio.slack.com/join/shared_invite/zt-e0u4qu3k-bI2GDNys3ZqX1YCJ9OM~GQ#/)!👇🏻\n\n\n\n\n\n ","title":"4 Steps to Building a Video Search System","metaData":{}},{"id":"2020-08-22-deleting-data-in-milvus.md","author":"milvus","desc":"In Milvus v0.7.0 we came up with a brand new design to make deletion more efficient and support more index types.","canonicalUrl":"https://zilliz.com/blog/deleting-data-in-milvus","date":"2020-08-22T20:27:23.266Z","cover":"https://assets.zilliz.com/header_c9b45e546c.png","tags":["Engineering"],"href":"/blog/2020-08-22-deleting-data-in-milvus.md","content":" \n# How Milvus Realizes the Delete Function\nThis article deals with how Milvus implements the delete function. As a much-anticipated feature for many users, the delete function was introduced to Milvus v0.7.0. We did not call remove_ids in FAISS directly, instead, we came up with a brand new design to make deletion more efficient and support more index types.\n\nIn [How Milvus Realizes Dynamic Data Update and Query](https://medium.com/unstructured-data-service/how-milvus-implements-dynamic-data-update-and-query-d15e04a85e7d?source=friends_link\u0026sk=cc38bee61bc194f30324ed17e86886f3), we introduced the entire process from inserting data to flushing data. Let’s recap on some of the basics. MemManager manages all insert buffers, with each MemTable corresponding to a collection (we renamed “table” to “collection” in Milvus v0.7.0). Milvus automatically divides the data inserted to the memory into multiple MemTableFiles. When data is flushed to the disk, each MemTableFile is serialized into a raw file. We kept this architecture when designing the delete function.\n\nWe define the function of the delete method as deleting all data corresponding to the specified entity IDs in a specific collection. When developing this function, we designed two scenarios. The first is to delete the data that is still in the insert buffer, and the second is to delete the data that has been flushed to the disk. The first scenario is more intuitive. We can find the MemTableFile corresponding to the specified ID, and delete the data in the memory directly (Figure 1). Because deletion and insertion of data cannot be performed at the same time, and because of the mechanism that changes the MemTableFile from mutable to immutable when flushing data, deletion is only performed in the mutable buffer. In this way, the deletion operation does not clash with data flushing, hence ensuring the consistency of data.\n\n\n\n\nThe second scenario is more complex but more commonplace, as in most cases the data stays in the insert buffer briefly before being flushed to the disk. Given that it is so inefficient to load flushed data to the memory for a hard deletion, we decided to go for soft deletion, a more efficient approach. Instead of actually deleting the flushed data, soft deletion saves deleted IDs in a separate file. In this way, we can filter out those deleted IDs during read operations, such as search.\n\nWhen it comes to implementation, we have several issues to consider. In Milvus, data is visible or, in other words, recoverable, only when it is flushed to the disk. Therefore, flushed data is not deleted during the delete method call, but in the next flush operation. The reason is that the data files that have been flushed to the disk will no longer include new data, thus soft deletion does not impact the data that has been flushed. When calling delete, you can directly delete the data that is still in the insert buffer, while for the flushed data, you need to record the ID of the deleted data in the memory. When flushing data to the disk, Milvus writes the deleted ID to the DEL file to record which entity in the corresponding segment is deleted. These updates will be visible only after the data flushing completes. This process is illustrated in Figure 2. Before v0.7.0, we only had an auto-flush mechanism in place; that is, Milvus serializes the data in the insert buffer every second. In our new design, we added a flush method allowing developers to call after the delete method, ensuring that the newly inserted data is visible and the deleted data is no longer recoverable.\n\n\n\nThe second issue is that the raw data file and the index file are two separate files in Milvus, and two independent records in the metadata. When deleting a specified ID, we need to find the raw file and index file corresponding to the ID and record them together. Accordingly, we introduced the concept of segment. A segment contains the raw file (which includes the raw vector files and ID files), the index file, and the DEL file. Segment is the most basic unit for reading, writing, and searching vectors in Milvus. A collection (Figure 3) is composed of multiple segments. Thus, there are multiple segment folders under a collection folder in the disk. Since our metadata is based on relational databases (SQLite or MySQL), it is very simple to record the relationship within a segment, and the delete operation no longer requires separate processing of the raw file and the index file.\n\n\n\nThe third issue is how to filter out deleted data during a search. In practice, the ID recorded by DEL is the offset of the corresponding data stored in the segment. Since the flushed segment does not include new data, the offset will not change. The data structure of DEL is a bitmap in the memory, where an active bit represents a deleted offset. We also updated FAISS accordingly: when you search in FAISS, the vector corresponding to the active bit will no longer be included in the distance calculation (Figure 4). The changes to FAISS will not be addressed in detail here.\n\n\n\nThe last issue is about performance improvement. When deleting flushed data, you first need to find out which segment of the collection the deleted ID is in and then record its offset. The most straightforward approach is to search all IDs in each segment. The optimization we are thinking about is adding a bloom filter to each segment. Bloom filter is a random data structure used to check whether an element is a member of a set. Therefore, we can load only the bloom filter of each segment. Only when the bloom filter determines that the deleted ID is in the current segment can we find the corresponding offset in the segment; otherwise, we can ignore this segment (Figure 5). We choose bloom filter because it uses less space and is more efficient in searching than many of its peers, such as hash tables. Although the bloom filter has a certain rate of false positive, we can reduce the segments that need to be searched to the ideal number to adjust the probability. Meanwhile, the bloom filter also needs to support deletion. Otherwise, the deleted entity ID can still be found in the bloom filter, resulting in an increased false-positive rate. For this reason, we use the counting bloom filter as it supports deletion. In this article, we will not elaborate on how the bloom filter works. You may refer to Wikipedia if you are interested.\n\n\n\n## Wrapping up\n\nSo far, we have given you a brief introduction about how Milvus deletes vectors by ID. As you know, we use soft deletion to delete the flushed data. As the deleted data increases, we need to compact the segments in the collection to release the space occupied by the deleted data. Besides, if a segment has already been indexed, compact also deletes the previous index file and creates new indexes. For now, developers need to call the compact method to compact data. Going forward, we hope to introduce an inspection mechanism. For example, when the amount of deleted data reaches a certain threshold or the data distribution has changed after a deletion, Milvus automatically compacts the segment.\n\nNow we have introduced the design philosophy behind the delete function and its implementation. There is definitely room for improvement, and any of your comments or suggestions are welcome.\n\nGet to know about Milvus: https://github.com/milvus-io/milvus. You can also join our community [Slack](https://milvusio.slack.com/join/shared_invite/zt-e0u4qu3k-bI2GDNys3ZqX1YCJ9OM~GQ#/) for technical discussions!\n\n\n\n\n ","title":"How Milvus Realizes the Delete Function","metaData":{}},{"id":"2020-08-11-optimizing-billion-scale-image-search-milvus-part-2.md","author":"Rife Wang","desc":"A user case of leveraging Milvus to build an image similarity search system for real-world business.","canonicalUrl":"https://zilliz.com/blog/optimizing-billion-scale-image-search-milvus-part-2","date":"2020-08-11T22:20:27.855Z","cover":"https://assets.zilliz.com/header_c73631b1e7.png","tags":["Scenarios"],"href":"/blog/2020-08-11-optimizing-billion-scale-image-search-milvus-part-2.md","content":"\n# The Journey to Optimizing Billion-scale Image Search (2/2)\n\nThis article is the second part of **The Journey to Optimizing Billion-scale Image Search by UPYUN**. If you miss the first one, click [here](https://zilliz.com/blog/optimizing-billion-scale-image-search-milvus-part-1).\n\n## The second-generation search-by-image system\n\nThe second-generation search-by-image system technically chooses the CNN + Milvus solution. The system is based on feature vectors and provides better technical support.\n\n## Feature extraction\n\nIn the field of computer vision, the use of artificial intelligence has become the mainstream. Similarly, the feature extraction of the second-generation search-by-image system uses convolutional neural network (CNN) as the underlying technology\n\nThe term CNN is difficult to understand. Here we focus on answering two questions:\n\n- What can CNN do?\n- Why can I use CNN for an image search?\n\n\n\nThere are many competitions in the AI field and image classification is one of the most important. The job of image classification is to determine whether the content of the picture is about a cat, a dog, an apple, a pear, or other types of objects.\n\nWhat can CNN do? It can extract features and recognize objects. It extracts features from multiple dimensions and measures how close the features of an image are to the features of cats or dogs. We can choose the closest ones as our identification result which indicates whether the content of a specific image is about a cat, a dog, or something else.\n\nWhat is the connection between the object identification function of CNN and search by image? What we want is not the final identification result, but the feature vector extracted from multiple dimensions. The feature vectors of two images with similar content must be close.\n\n### Which CNN model should I use?\n\nThe answer is VGG16. Why choose it? First, VGG16 has good generalization capability, that is, it is very versatile. Second, the feature vectors extracted by VGG16 have 512 dimensions. If there are very few dimensions, the accuracy may be affected. If there are too many dimensions, the cost of storing and calculating these feature vectors is relatively high.\n\nUsing CNN to extract image features is a mainstream solution. We can use VGG16 as the model and Keras + TensorFlow for technical implementation. Here is the official example of Keras:\n\n from keras.applications.vgg16 import VGG16\n from keras.preprocessing import image\n from keras.applications.vgg16 import preprocess_input\n import numpy as np\n model = VGG16(weights=’imagenet’, include_top=False)\n img_path = ‘elephant.jpg’\n img = image.load_img(img_path, target_size=(224, 224))\n x = image.img_to_array(img)\n x = np.expand_dims(x, axis=0)\n x = preprocess_input(x)\n features = model.predict(x)\n\nThe features extracted here are feature vectors.\n\n### 1. Normalization\n\nTo facilitate subsequent operations, we often normalize feature:\n\nWhat is used subsequently is also the normalized \u003ccode\u003enorm_feat\u003c/code\u003e.\n\n### 2. Image description\n\nThe image is loaded using the \u003ccode\u003eimage.load_img\u003c/code\u003e method of \u003ccode\u003ekeras.preprocessing\u003c/code\u003e:\n\n from keras.preprocessing import image\n img_path = 'elephant.jpg'\n img = image.load_img(img_path, target_size=(224, 224))\n\nIn fact, it is the TensorFlow method called by Keras. For details, see the TensorFlow documentation. The final image object is actually a PIL Image instance (the PIL used by TensorFlow).\n\n### 3. Bytes conversion\n\nIn practical terms, image content is often transmitted through the network. Therefore, instead of loading images from path, we prefer converting bytes data directly into image objects, that is, PIL Images:\n\n import io\n from PIL import Image\n\n # img_bytes: 图片内容 bytes\n img = Image.open(io.BytesIO(img_bytes))\n img = img.convert('RGB')\n\n img = img.resize((224, 224), Image.NEAREST)\n\nThe above img is the same as the result obtained by the image.load_img method. There are two things to pay attention to:\n\n- You must do RGB conversion.\n- You must resize (resize is the second parameter of the \u003ccode\u003eload_img method\u003c/code\u003e).\n\n### 4. Black border processing\n\nImages, such as screenshots, may occasionally have quite a few black borders. These black borders have no practical value and cause much interference. For this reason, removing black borders is also a common practice.\n\nA black border is essentially a row or column of pixels where all pixels are (0, 0, 0) (RGB image). To remove the black border is to find these rows or columns and delete them. This is actually a 3-D matrix multiplication in NumPy.\n\nAn example of removing horizontal black borders:\n\n # -*- coding: utf-8 -*-\n import numpy as np\n from keras.preprocessing import image\n def RemoveBlackEdge(img):\n Args:\n img: PIL image instance\n Returns:\n PIL image instance\n \"\"\"\n width = img.width\n img = image.img_to_array(img)\n img_without_black = img[~np.all(img == np.zeros((1, width, 3), np.uint8), axis=(1, 2))]\n img = image.array_to_img(img_without_black)\n return img\n\nThis is pretty much what I want to talk about using CNN to extract image features and implement other image processing. Now let’s take a look at vector search engines.\n\n## Vector search engine\n\nThe problem of extracting feature vectors from images has been solved. Then the remaining problems are:\n\n- How to store feature vectors?\n- How to calculate the similarity of feature vectors, that is, how to search?\n The open-source vector search engine Milvus can solve these two problems. So far, it has been running well in our production environment.\n\n\n\n## Milvus, the vector search engine\n\nExtracting feature vectors from an image is far from enough. We also need to dynamically manage these feature vectors (addition, deletion, and update), calculate the similarity of the vectors, and return the vector data in the nearest neighbor range. The open-source vector search engine Milvus performs these tasks quite well.\n\nThe rest of this article will describe specific practices and points to be noted.\n\n### 1. Requirements for CPU\n\nTo use Milvus, your CPU must support the avx2 instruction set. For Linux systems, use the following command to check which instruction sets your CPU supports:\n\n\u003ccode\u003ecat /proc/cpuinfo | grep flags\u003c/code\u003e\n\nThen you get something like:\n\n flags : fpu vme de pse tsc msr pae mce cx8 apic sep mtrr pge mca cmov pat pse36 clflush dts acpi mmx fxsr sse sse2 ss ht tm pbe syscall nx pdpe1gb rdtscp lm constant_tsc arch_perfmon pebs bts rep_good nopl xtopology nonstop_tsc cpuid aperfmperf pni pclmulqdq dtes64 monitor ds_cpl vmx smx est tm2 ssse3 sdbg fma cx16 xtpr pdcm pcid dca sse4_1 sse4_2 x2apic movbe popcnt aes xsave avx f16c rdrand lahf_lm abm cpuid_fault epb invpcid_single pti intel_ppin tpr_shadow vnmi flexpriority ept vpid ept_ad fsgsbase tsc_adjust bmi1 avx2 smep bmi2 erms invpcid cqm xsaveopt cqm_llc cqm_occup_llc dtherm ida arat pln pts\n\nWhat follows flags is the instruction sets your CPU supports. Of course, these are much more than I need. I just want to see if a specific instruction set, such as avx2, is supported. Just add a grep to filter it:\n\n cat /proc/cpuinfo | grep flags | grep avx2\n\nIf no result is returned, it means that this specific instruction set is not supported. You need to change your machine then.\n\n### 2. Capacity planning\n\nCapacity planning is our first consideration when we design a system. How much data do we need to store? How much memory and disk space does the data require?\n\nLet’s do some quick maths. Each dimension of a vector is float32. A float32 type takes up 4 Bytes. Then a vector of 512 dimensions requires 2 KB of storage. By the same token:\n\n- One thousand 512-dimensional vectors require 2 MB of storage.\n- One million 512-dimensional vectors require 2 GB of storage.\n- 10 million 512-dimensional vectors require 20 GB of storage.\n- 100 million 512-dimensional vectors require 200 GB of storage.\n- One billion 512-dimensional vectors require 2 TB of storage.\n\nIf we want to store all the data in the memory, then the system needs at least the corresponding memory capacity.\n\nIt is recommended that you use the official size calculation tool: Milvus sizing tool.\n\nActually our memory may not be that big. (It doesn’t really matter if you don’t have enough memory. Milvus automatically flushes data onto the disk.) In addition to the original vector data, we also need to consider the storage of other data such as logs.\n\n### 3. System configuration\n\nFor more information about the system configuration, see the Milvus documentation:\n\n- Milvus server configuration: https://milvus.io/docs/v0.10.1/milvus_config.md\n\n### 4. Database design\n\n**Collection \u0026 Partition**\n\n- Collection is also known as table.\n- Partition refers to the partitions inside a collection.\n\nThe underlying implementation of partition is actually the same with that of collection, except that a partition is under a collection. But with partitions, the organization of data becomes more flexible. We can also query a specific partition in a collection to achieve better query results.\n\nHow many collections and partitions can we have? The basic information on collection and partition is in Metadata. Milvus uses either SQLite (Milvus internal integration) or MySQL (requires external connection) for internal metadata management. If you use SQLite by default to manage Metadata, you will suffer severe performance loss when the numbers of collections and partitions are too large. Therefore, the total number of collections and partitions should not exceed 50,000 (Milvus 0.8.0 will limit this number to 4,096). If you need to set a larger number, it is recommended that you use MySQL via an external connection.\n\nThe data structure supported by Milvus’ collection and partition is very simple, that is, \u003ccode\u003eID + vector\u003c/code\u003e. In other words, there are only two columns in the table: ID and vector data.\n\n**Note:**\n\n- ID should be integers.\n- We need to ensure that the ID is unique within a collection instead of within a partition.\n\n**Conditional filtering**\n\nWhen we use traditional databases, we can specify field values as filtering conditions. Though Milvus does not filter exactly the same way, we can implement simple conditional filtering using collections and partitions. For example, we have a large amount of image data and the data belongs to specific users. Then we can divide the data into partitions by user. Therefore, using the user as the filter condition is actually specifying the partition.\n\n**Structured data and vector mapping**\n\nMilvus only supports the ID + vector data structure. But in business scenarios, what we need is structured data-bearing business meaning. In other words, we need to find structured data through vectors. Accordingly, we need to maintain the mapping relations between structured data and vectors through ID.\n\n structured data ID \u003c--\u003e mapping table \u003c--\u003e Milvus ID\n\n**Selecting index**\n\nYou can refer to the following articles:\n\n- Types of index: https://www.milvus.io/docs/v0.10.1/index.md\n- How to select index: https://medium.com/@milvusio/how-to-choose-an-index-in-milvus-4f3d15259212\n\n### 5. Processing search results\n\nThe search results of Milvus are a collection of ID + distance:\n\n- ID: the ID in a collection.\n- Distance: a distance value of 0 ~ 1 indicates similarity level; the smaller the value, the more similar the two vectors.\n\n**Filtering data whose ID is -1**\n\nWhen the number of collections is too small, the search results may contain data whose ID is -1. We need to filter it out by ourselves.\n\n**Pagination**\n\nThe search for vectors is quite different. The query results are sorted in descending order of similarity, and the most similar (topK) of results are selected (topK is specified by the user at the time of query).\n\nMilvus does not support pagination. We need to implement the pagination function by ourselves if we need it for business. For example, if we have ten results on each page and only want to display the third page, we need to specify that topK = 30 and only return the last ten results.\n\n**Similarity threshold for business**\n\nThe distance between the vectors of two images is between 0 and 1. If we want to decide whether two images are similar in a specific business scenario, we need to specify a threshold within this range. The two images are similar if the distance is smaller than the threshold, or they are quite different from each other if the distance is larger than the threshold. You need to adjust the threshold to meet your own business needs.\n\n\u003e This article is written by rifewang, Milvus user and software engineer of UPYUN. If you like this article, welcome to come say hi @ https://github.com/rifewang.\n","title":"The Journey to Optimizing Billion-scale Image Search (2/2)","metaData":{}},{"id":"2020-08-04-optimizing-billion-scale-image-search-milvus-part-1.md","author":"Rife Wang","desc":"A case study with UPYUN. Learn about how Milvus stands out from traditional database solutions and helps to build an image similarity search system.","canonicalUrl":"https://zilliz.com/blog/optimizing-billion-scale-image-search-milvus-part-1","date":"2020-08-04T20:39:09.882Z","cover":"https://assets.zilliz.com/header_23bbd76c8b.jpg","tags":["Scenarios"],"href":"/blog/2020-08-04-optimizing-billion-scale-image-search-milvus-part-1.md","content":"\n# The Journey to Optimizing Billion-scale Image Search (1/2)\n\nYupoo Picture Manager serves tens of millions of users and manages tens of billions of pictures. As its user gallery is growing larger, Yupoo has an urgent business need for a solution that can quickly locate the image. In other words, when a user inputs an image, the system should find its original image and similar images in the gallery. The development of the search by image service provides an effective approach to this problem.\n\nThe search by image service has undergone two evolutions:\n\n1. Began the first technical investigation in early 2019 and launched the first-generation system in March and April 2019;\n2. Began the investigation of the upgrade plan in early 2020 and started the overall upgrade to the second-generation system in April 2020.\n\nThis article describes the technology selection and basic principles behind the two generations of search by image system based on my own experience on this project.\n\n## Overview\n\n### What is an image?\n\nWe must know what is an image before dealing with images.\n\nThe answer is that an image is a collection of pixels.\n\nFor example, the part in the red box on this image is virtually a series of pixels.\n\n\n\nSuppose the part in the red box is an image, then each independent small square in the image is a pixel, the basic information unit. Then, the size of the image is 11 x 11 px.\n\n\n\n### Mathematical representation of images\n\nEach image can be represented by a matrix. Each pixel in the image corresponds to an element in the matrix.\n\n### Binary images\n\nThe pixels of a binary image is either black or white, so each pixel can be represented by 0 or 1.\nFor example, the matrix representation of a 4 \\* 4 binary image is:\n\n 0 1 0 1\n 1 0 0 0\n 1 1 1 0\n 0 0 1 0\n\n### RGB images\n\nThe three primary colors (red, green, and blue) can be mixed to produce any color. For RGB images, each pixel has the basic information of three RGB channels. Similarly, if each channel uses an 8-bit number (in 256 levels) to represent its gray scale, then the mathematical representation of a pixel is:\n\n ([0 .. 255], [0 .. 255], [0 .. 255])\n\nTaking a 4 \\* 4 RGB image as an example:\n\n\n\nThe essence of image processing is to process these pixel matrices.\n\n## The technical problem of search by image\n\nIf you are looking for the original image, that is, an image with exactly the same pixels, then you can directly compare their MD5 values. However, images uploaded to the Internet are often compressed or watermarked. Even a small change in an image can create a different MD5 result. As long as there is inconsistency in pixels, it is impossible to find the original image.\n\nFor a search-by-image system, we want to search for images with similar content. Then, we need to solve two basic problems:\n\n- Represent or abstract an image as a data format that can be processed by a computer.\n- The data must be comparable for calculation.\n\nMore specifically, we need the following features:\n\n- Image feature extraction.\n- Feature calculation (similarity calculation).\n\n## The first-generation search-by-image system\n\n### Feature extraction — image abstraction\n\nThe first-generation search-by-image system uses Perceptual hash or pHash algorithm for feature extraction. What are the basics of this algorithm?\n\n\n\nAs shown in the figure above, the pHash algorithm performs a series of transformations on the image to get the hash value. During the transformation process, the algorithm continuously abstract images, thereby pushing the results of similar images closer to each other.\n\n### Feature calculation — similarity calculation\n\nHow to calculate the similarity between the pHash values of two images? The answer is to use the Hamming distance. The smaller the Hamming distance, the more similar the images’ content.\n\nWhat is Hamming distance? It is the number of different bits.\n\nFor example,\n\n Value 1: 0 1 0 1 0\n Value 2: 0 0 0 1 1\n\nThere are two different bits in the above two values, so the Hamming distance between them is 2.\n\nNow we know the principle of similarity calculation. The next question is, how to calculate the Hamming distances of 100-million-scale data from 100-million-scale pictures? In short, how to search for similar images?\n\nIn the early stage of the project, I did not find a satisfactory tool (or a computing engine) that can quickly calculate the Hamming distance. So I changed my plan.\n\nMy idea is that if the Hamming distance of two pHash values is small, then I can cut the pHash values and the corresponding small parts are likely to be equal.\n\nFor example:\n\n Value 1: 8 a 0 3 0 3 f 6\n Value 2: 8 a 0 3 0 3 d 8\n\nWe divide the above two values into eight segments and the values of six segments are exactly the same. It can be inferred that their Hamming distance is close and thus these two images are similar.\n\nAfter the transformation, you can find that the problem of calculating Hamming distance has become a problem of matching equivalence. If I divide each pHash value into eight segments, as long as there are more than five segments that have exactly the same values, then the two pHash values are similar.\n\nThus it is very simple to solve equivalence matching. We can use the classical filtering of a traditional database system.\n\nOf course, I use the multi-term matching and specify the degree of matching using minimum_should_match in ElasticSearch (this article does not introduce the principle of ES, you can learn it by yourself).\n\nWhy do we choose ElasticSearch? First, it provides the above-mentioned search function. Second, the image manager project in itself is using ES to provide a full-text search function and it is very economical to use the existing resources.\n\n## Summary of the first-generation system\n\nThe first-generation search-by-image system chooses the pHash + ElasticSearch solution, which has the following features:\n\n- The pHash algorithm is simple to use and can resist a certain degree of compression, watermark, and noise.\n- ElasticSearch uses the existing resources of the project without adding additional costs to the search.\n- But the limitation of this system is obvious: The pHash algorithm is an abstract representation of the entire image. Once we destroy the integrity of the image, such as adding a black border to the original image, it is almost impossible to judge the similarity between the original and the others.\n\nTo break through such limitations, the second-generation image search system with a completely different underlying technology emerged.\n\nThis article is written by rifewang, Milvus user and software engineer of UPYUN. If you like this article, welcome to come say hi! https://github.com/rifewang\n","title":"The Journey to Optimizing Billion-scale Image Search (1/2)","metaData":{}},{"id":"2020-07-28-Building-an-AI-Powered-Writing-Assistant-with-WPS-Office.md","author":"milvus","desc":"Learn how Kingsoft leveraged Milvus, an open-source similarity search engine, to build a recommendation engine for WPS Office’s AI-powered writing assistant.","canonicalUrl":"https://zilliz.com/blog/Building-an-AI-Powered-Writing-Assistant-with-WPS-Office","date":"2020-07-28T03:35:40.105Z","cover":"https://assets.zilliz.com/wps_thumbnail_6cb7876963.jpg","tags":["Scenarios"],"href":"/blog/2020-07-28-Building-an-AI-Powered-Writing-Assistant-with-WPS-Office.md","content":" \n# Building an AI-Powered Writing Assistant for WPS Office\nWPS Office is a productivity tool developed by Kingsoft with over 150M users worldwide. The company’s artificial intelligence (AI) department built a smart writing assistant from scratch using semantic matching algorithms such as intent recognition and text clustering. The tool exists both as a web application and [WeChat mini program](https://walkthechat.com/wechat-mini-programs-simple-introduction/) that helps users quickly create outlines, individual paragraphs, and entire documents by simply inputting a title and selecting up to five keywords.\n\nThe writing assistant’s recommendation engine uses Milvus, an open-source similarity search engine, to power its core vector processing module. Below we’ll explore the process for building WPS Offices’ smart writing assistant, including how features are extracted from unstructured data as well as the role Milvus plays in storing data and powering the tool’s recommendation engine.\n\nJump to:\n- [Building an AI-Powered Writing Assistant for WPS Office](#building-an-ai-powered-writing-assistant-for-wps-office)\n - [Making sense of unstructured textual data](#making-sense-of-unstructured-textual-data)\n - [Using the TFIDF model to maximize feature extraction](#using-the-tfidf-model-to-maximize-feature-extraction)\n - [Extracting features with the bi-directional LSTM-CNNs-CRF deep learning model](#extracting-features-with-the-bi-directional-lstm-cnns-crf-deep-learning-model)\n - [Creating sentence embeddings using Infersent](#creating-sentence-embeddings-using-infersent)\n - [Storing and querying vectors with Milvus](#storing-and-querying-vectors-with-milvus)\n - [AI isn’t replacing writers, it’s helping them write](#ai-isnt-replacing-writers-its-helping-them-write)\n\n\n### Making sense of unstructured textual data\nMuch like any modern problem worth solving, building the WPS writing assistant begins with messy data. Tens of millions of dense text documents from which meaningful features must be extracted, to be a bit more precise. To understand the complexity of this problem consider how two journalists from different news outlets might report on the same topic.\n\nWhile both will adhere to the rules, principles, and processes that govern sentence structure, they will make different word choices, create sentences of varying length, and use their own article structures to tell similar (or perhaps dissimilar) stories. Unlike structured datasets with a fixed number of dimensions, bodies of text inherently lack structure because the syntax that governs them is so malleable. In order to find meaning, machine readable features must be extracted from an unstructured corpus of documents. But first, the data must be cleaned.\n\nThere are a variety of ways to clean textual data, none of which this article will cover in depth. Nonetheless, this is an important step that preempts processing the data, and can include removing tags, removing accented characters, expanding contractions, removing special characters, removing stopwords, and more. A detailed explanation of methods for pre-processing and cleaning text data can be found [here](https://towardsdatascience.com/understanding-feature-engineering-part-3-traditional-methods-for-text-data-f6f7d70acd41).\n\n\n### Using the TFIDF model to maximize feature extraction\n\nTo begin making sense of unstructured textual data, the term frequency–inverse document frequency (TFIDF) model was applied to the corpus the WPS writing assistant pulls from. This model uses a combination of two metrics, term frequency and inverse document frequency, to give each word within a document a TFIDF value. Term frequency (TF) represents the raw count of a term in a document divided by the total number of terms in the document, while inverse document frequency (IDF) is the number of documents in a corpus divided by the number of documents in which a term appears.\n\nThe product of TF and IDF provides a measure of how frequent a term appears in a document multiplied by how unique the word is in the corpus. Ultimately, TFIDF values are a measure of how relevant a word is to a document within a collection of documents. Terms are sorted by TFIDF values, and those with low values (i.e. common words) can be given less weight when using deep learning to extract features from the corpus.\n\n\n### Extracting features with the bi-directional LSTM-CNNs-CRF deep learning model\n\nUsing a combination of bi-directional long short-term memory (BLSTM), convolutional neural networks (CNN), and conditional random fields (CRF) both word- and character-level representations can be extracted from the corpus. The [BLSTM-CNNs-CRF model](https://arxiv.org/pdf/1603.01354.pdf) used to build the WPS Office writing assistant works as follows:\n\n1. **CNN:** Character embeddings are used as inputs to the CNN, then semantically relevant word structures (i.e. the prefix or suffix) are extracted and encoded into character-level representation vectors.\n2. **BLSTM:** Character-level vectors are concatenated with word embedding vectors then fed into the BLSTM network. Each sequence is presented forwards and backwards to two separate hidden states to capture past and future information.\n3. **CRF:** The output vectors from the BLSTM are fed to the CRF layer to jointly decode the best label sequence.\n\nThe neural network is now capable of extracting and classifying named entities from unstructured text. This process is called [named entity recognition (NER)](https://en.wikipedia.org/wiki/Named-entity_recognition) and involves locating and classifying categories such as person names, institutions, geographic locations, and more. These entities play an important role in sorting and recalling data. From here key sentences, paragraphs, and summaries can be extracted from the corpus.\n\n\n### Creating sentence embeddings using Infersent\n\n[Infersent](https://github.com/facebookresearch/InferSent), a supervised sentence embeddings method designed by Facebook that embeds full sentences into vector space, is used to create vectors that will be fed into the Milvus database. Infersent was trained using the Stanford Natural Language Inference (SNLI) corpus, which contains 570k pairs of sentences that were written and labelled by humans. Additional information about how Infersent works can be found [here](https://medium.com/analytics-vidhya/sentence-embeddings-facebooks-infersent-6ac4a9fc2001).\n\n\n### Storing and querying vectors with Milvus\n\n[Milvus](https://www.milvus.io/) is an open source similarity search engine that supports adding, deleting, updating, and near-real-time search of embeddings on a trillion bytes scale. To improve query performance, Milvus allows an index type to be specified for each vector field. The WPS Office smart assistant uses the IVF_FLAT index, the most basic Inverted File (IVF) index type where “flat” means vectors are stored without compression or quantization. Clustering is based on IndexFlat2, which uses exact search for L2 distance.\n\nAlthough IVF_FLAT has a 100% query recall rate, its lack of compression results in comparatively slow query speeds. Milvus’ [partitioning function](https://milvus.io/docs/manage-partitions.md) is used to divide data into multiple parts of physical storage based on predefined rules, making queries faster and more accurate. When vectors are added to Milvus, tags specify which partition the data should be added to. Queries of the vector data use tags to specify which partition the query should be executed on. Data can be further broken down into segments within each partition to further improve speed.\n\nThe intelligent writing assistant also uses Kubernetes clusters, allowing application containers to run across multiple machines and environments, as well as MySQL for metadata management.\n\n### AI isn’t replacing writers, it’s helping them write\n\nKingsoft’s writing assistant for WPS Office relies on Milvus to manage and query a database of more than 2 million documents. The system is highly flexible, capable of running near real-time search on trillion-scale datasets. Queries complete in 0.2 seconds on average, meaning entire documents can be generated almost instantaneously using just a title or a few keywords. Although AI isn’t replacing professional writers, technology that exists today is capable of augmenting the writing process in novel and interesting ways. The future is unknown, but at the very least writers can look forward to more productive, and for some less difficult, methods of “putting pen to paper.”\n\n\nThe following sources were used for this article:\n\n- “[End-to-end Sequence Labeling via Bi-directional LSTM-CNNs-CRF](https://arxiv.org/pdf/1603.01354.pdf),” Xuezhe Ma and Eduard Hovy.\n- “[Traditional Methods for Text Data](https://towardsdatascience.com/understanding-feature-engineering-part-3-traditional-methods-for-text-data-f6f7d70acd41),” Dipanjan (DJ) Sarkar.\n- “[Text Features Extraction based on TF-IDF Associating Semantic](https://ieeexplore.ieee.org/document/8780663),” Qing Liu, Jing Wang, Dehai Zhang, Yun Yang, NaiYao Wang.\n- “[Understanding Sentence Embeddings using Facebook’s Infersent](https://medium.com/analytics-vidhya/sentence-embeddings-facebooks-infersent-6ac4a9fc2001),” Rehan Ahmad\n- “[Supervised Learning of Universal Sentence Representations from Natural Language Inference Data](https://arxiv.org/pdf/1705.02364.pdf),” Alexis Conneau, Douwe Kiela, Holger Schwenk, LoÏc Barrault, Antoine Bordes.V1\n\nRead other [user stories](https://zilliz.com/user-stories) to learn more about making things with Milvus.\n\n\n ","title":"Building an AI-Powered Writing Assistant for WPS Office","metaData":{}},{"id":"2020-06-04-Making-with-Milvus-AI-Powered-News-Recommendation-Inside-Xiaomi-Mobile-Browser.md","author":"milvus","desc":"Discover how Xiaomi leveraged AI and Milvus to build an intelligent news recommendation system capable of finding the most relevant content for users of its mobile web browser.","canonicalUrl":"https://zilliz.com/blog/Making-with-Milvus-AI-Powered-News-Recommendation-Inside-Xiaomi-Mobile-Browser","date":"2020-06-04T02:30:34.750Z","cover":"https://assets.zilliz.com/blog_Sohu_News_dec53d0814.jpg","tags":["Scenarios"],"href":"/blog/2020-06-04-Making-with-Milvus-AI-Powered-News-Recommendation-Inside-Xiaomi-Mobile-Browser.md","content":" \n# Making with Milvus: AI-Powered News Recommendation Inside Xiaomi's Mobile Browser\nFrom social media feeds to playlist recommendations on Spotify, [artificial intelligence](https://zilliz.com/blog/Vector-Similarity-Search-Hides-in-Plain-View) already plays a major role in the content we see and interact with each day. In an effort to differentiate their mobile web browser, multinational electronics manufacturer Xiaomi built an AI-powered news recommendation engine. [Milvus](https://milvus.io/), an open-source vector database built specifically for similarity search and artificial intelligence, was used as the application’s core data management platform. This article explains how Xiaomi built its AI-powered news recommendation engine, and how Milvus and other AI algorithms were used. \n\n\u003cbr/\u003e\n\n\n### Using AI to suggest personalized content and cut through news noise\n\nWith the New York Times alone publishing over [230 pieces](https://www.theatlantic.com/technology/archive/2016/05/how-many-stories-do-newspapers-publish-per-day/483845/) of content each day, the sheer volume of articles produced makes it impossible for individuals to get a comprehensive view of all the news. To help sift through large volumes of content, and recommend the most relevant or interesting pieces, we increasingly turn to AI. Although recommendations remain far from perfect, machine learning is increasingly necessary to cut through the constant stream of new information pouring out of our increasingly complex and interconnected world.\n\nXiaomi makes and invests in smartphones, mobile apps, laptops, home appliances, and many more products. In an effort to differentiate a mobile browser that comes preinstalled on many of 40+ million smartphones the company sells each quarter, Xiaomi built a news recommendation system into it. When users launch Xiaomi’s mobile browser, artificial intelligence is used to recommend similar content based on user search history, interests, and more. Milvus is an open-source vector similarity search database used to accelerate retrieval of related articles.\n\n\u003cbr/\u003e\n\n### How does AI-powered content recommendation work?\n\nAt its core, news recommendation (or any other type of content recommendation system) involves comparing input data to a massive database to find similar information. Successful content recommendation involves balancing relevance with timeliness, and efficiently incorporating huge volumes of new data—often in real time. \n\nTo accommodate massive datasets, recommendation systems are typically divided into two stages:\n\n1. **Retrieval**: During retrieval, content is narrowed down from the broader library based on user interests and behavior. In Xiaomi’s mobile browser, thousands of pieces of content are selected from a massive dataset that contains millions of news articles.\n2. **Sorting**: Next, content selected during retrieval is sorted according to certain indicators before being pushed to the user. As users engage with recommended content, the system adapts in real time to provide more relevant suggestions. \n\nNews content recommendations need to be made in real-time based on user behavior and recently published content. Additionally, suggested content must match user interests and search intent as much as possible.\n\n\u003cbr/\u003e\n\n### Milvus + BERT = intelligent content suggestions\n\nMilvus is an open-source vector similarity search database that can be integrated with deep learning models to power applications spanning natural language processing, identity verification, and much more. Milvus indexes large vector datasets to make search more efficient, and supports a variety of popular AI frameworks to simplify the process of developing machine learning applications. These characteristics make the platform the ideal for storing and querying vector data, a critical component of many machine learning applications. \n\nXiaomi selected Milvus to manage vector data for its intelligent news recommendation system because it is fast, reliable, and requires minimal configuration and maintenance. However, Milvus must be paired with an AI algorithm to build deployable applications. Xiaomi selected BERT, short for Bidirectional Encoder Representation Transformers, as the language representation model in its recommendation engine. BERT can be used as a general NLU (natural language understanding) model that can drive a number of different NLP (natural language processing) tasks. Its key features include:\n\n- BERT’s transformer is used as the main framework of the algorithm and is capable of capturing explicit and implicit relationships within and between sentences.\n- Multi-task learning goals, masked language modeling (MLM), and next sentence prediction (NSP).\n- BERT performs better with greater amounts of data, and can enhance other natural language processing techniques such as Word2Vec by acting as a conversion matrix.\n\n\n\n\u003cbr/\u003e\n\nBERT’s network architecture uses a multi-layer transformer structure that abandons the traditional RNN and CNN neural networks. It works by converting the distance between two words at any position into one through its attention mechanism, and solves the dependency issue that has persisted in NLP for some time.\n\n\n\n\u003cbr/\u003e\n\n\n\n\n\u003cbr/\u003e\n\nBERT provides a simple and a complex model. The corresponding hyperparameters are as follows: BERT BASE: L = 12, H = 768, A = 12, total parameter 110M; BERT LARGE: L = 24, H = 1024, A = 16, the total number of parameters is 340M.\n\nIn the above hyperparameters, L represents the number of layers in the network (i.e. the number of Transformer blocks), A represents the number of self-Attention in Multi-Head Attention, and the filter size is 4H.\n\n\u003cbr/\u003e\n\n\n### Xiaomi’s content recommendation system \n\nXiaomi browser-based news recommender system relies on three key components: vectorization, ID mapping, and approximate nearest neighbor (ANN) service. \n\nVectorization is process where article titles are converted into general sentence vectors. The SimBert model, based on BERT, is used in Xiaomi’s recommendation system. SimBert is a 12-layer model with a hidden size of 768. Simbert uses the training model Chinese L-12_H-768_A-12 for continuous training (training task being “metric learning +UniLM”, and has trained 1.17 million steps on a signle TITAN RTX with the Adam optimizer (learning rate 2e-6, batch size 128). Simply put, this is an optimized BERT model.\n\nANN algorithms compare vectorized article titles to the entire news library stored in Milvus, then return similar content for users. ID mapping is used to obtain relevant information such as page views and clicks for corresponding articles.\n\n\n\n\n\u003cbr/\u003e\n\nThe data stored in Milvus that powers Xiaomi’s news recommendation engine is constantly being updated, including additional articles and activity information. As the system incorporates new data, old data must be purged. In this system, full data updates are done for the first T-1 days and incremental updates are done in the subsequent T days.\n\nAt defined intervals, old data is deleted and processed data of the T-1 days is inserted into the collection. Here newly generated data is incorporated in real time. Once new data is inserted, similarity search is conducted in Milvus. Retrieved articles are again sorted by click rate and other factors, and the top content is shown to users. In a scenario like this where data is frequently updated and results must be delivered in real time, Milvus' ability to rapidly incorporate and search new data makes it possible to drastically accelerate news content recommendation in Xiaomi’s mobile browser. \n\n\u003cbr/\u003e\n\n### Milvus makes vector similarity search better\n\nVectorizing data and then calculating similarity between vectors is the most commonly used retrieval technology. The rise of ANN-based vector similarity search engines has greatly improved the efficiency of vector similarity calculations. Compared with similar solutions, Milvus offers optimized data storage, abundant SDKs, and a distributed version that greatly reduces the workload of building a retrieval layer. Additionally, Milvus' active open-source community is a powerful resource that can help answer questions and troubleshoot problems as they arise.\n\nIf you would like to learn more about vector similarity search and Milvus, check out the following resources:\n\n- Check out [Milvus](https://github.com/milvus-io/milvus) on Github. \n- [Vector Similarity Search Hides in Plain View](https://zilliz.com/blog/Vector-Similarity-Search-Hides-in-Plain-View)\n- [Accelerating Similarity Search on Really Big Data with Vector Indexing](https://zilliz.com/blog/Accelerating-Similarity-Search-on-Really-Big-Data-with-Vector-Indexing)\n\nRead other [user stories](https://zilliz.com/user-stories) to learn more about making things with Milvus.\n\n ","title":"Making with Milvus AI-Powered News Recommendation Inside Xiaomi's Mobile Browser","metaData":{}},{"id":"2020-05-12-building-intelligent-chatbot-with-nlp-and-milvus.md","author":"milvus","desc":"The Next-Gen QA Bot is here","canonicalUrl":"https://zilliz.com/blog/building-intelligent-chatbot-with-nlp-and-milvus","date":"2020-05-12T22:33:34.726Z","cover":"https://assets.zilliz.com/header_ce3a0e103d.png","tags":["Scenarios"],"href":"/blog/2020-05-12-building-intelligent-chatbot-with-nlp-and-milvus.md","content":" \n# Building an Intelligent QA System with NLP and Milvus\nMilvus Project:github.com/milvus-io/milvus\n\nThe question answering system is commonly used in the field of natural language processing. It is used to answer questions in the form of natural language and has a wide range of applications. Typical applications include: intelligent voice interaction, online customer service, knowledge acquisition, personalized emotional chatting, and more. Most question answering systems can be classified as: generative and retrieval question answering systems, single-round question answering and multi-round question answering systems, open question answering systems, and specific question answering systems.\n\nThis article mainly deals with a QA system designed for a specific field, which is usually called an intelligent customer service robot. In the past, building a customer service robot usually required conversion of the domain knowledge into a series of rules and knowledge graphs. The construction process relies heavily on “human” intelligence. Once the scenes were changed, a lot of repetitive work would be required.\nWith the application of deep learning in natural language processing (NLP), machine reading can automatically find answers to matching questions directly from documents. The deep learning language model converts the questions and documents to semantic vectors to find the matching answer.\n\nThis article uses Google’s open source BERT model and Milvus, an open source vector search engine, to quickly build a Q\u0026A bot based on semantic understanding.\n\n## Overall Architecture\n\nThis article implements a question answering system through semantic similarity matching. The general construction process is as follows:\n\n1. Obtain a large number of questions with answers in a specific field ( a standard question set).\n2. Use the BERT model to convert these questions into feature vectors and store them in Milvus. And Milvus will assign a vector ID to each feature vector at the same time.\n3. Store these representative question IDs and their corresponding answers in PostgreSQL.\n\nWhen a user asks a question:\n\n1. The BERT model converts it to a feature vector.\n2. Milvus performs a similarity search and retrieves the ID most similar to the question.\n3. PostgreSQL returns the corresponding answer.\n\nThe system architecture diagram is as follows (the blue lines represent the import process and the yellow lines represent the query process):\n\n\n\nNext, we will show you how to build an online Q\u0026A system step by step.\n\n## Steps to Build the Q\u0026A System\n\nBefore you start, you need to install Milvus and PostgreSQL. For the specific installation steps, see the Milvus official website.\n\n### 1. Data preparation\n\nThe experimental data in this article comes from: https://github.com/chatopera/insuranceqa-corpus-zh\n\nThe data set contains question and answer data pairs related to the insurance industry. In this article we extracts 20,000 question and answer pairs from it. Through this set of question and answer data sets, you can quickly build a customer service robot for the insurance industry.\n\n### 2. Generate feature vectors\n\nThis system uses a model that BERT has pre-trained. Download it from the link below before starting a service: https://storage.googleapis.com/bert_models/2018_10_18/cased_L-24_H-1024_A-16.zip\n\nUse this model to convert the question database to feature vectors for future similarity search. For more information about the BERT service, see https://github.com/hanxiao/bert-as-service.\n\n\n\n### 3. Import to Milvus and PostgreSQL\n\nNormalize and import the generated feature vectors import to Milvus, and then import the IDs returned by Milvus and the corresponding answers to PostgreSQL. The following shows the table structure in PostgreSQL:\n\n\n\n\n\n### 4. Retrieve Answers\n\nThe user inputs a question, and after generating the feature vector through BERT, they can find the most similar question in the Milvus library. This article uses the cosine distance to represent the similarity between two sentences. Because all vectors are normalized, the closer the cosine distance of the two feature vectors to 1, the higher the similarity.\n\nIn practice, your system may not have perfectly matched questions in the library. Then, you can set a threshold of 0.9. If the greatest similarity distance retrieved is less than this threshold, the system will prompt that it does not include related questions.\n\n\n\n## System Demonstration\n\nThe following shows an example interface of the system:\n\n\n\nEnter your question in the dialog box and you will receive a corresponding answer:\n\n\n\n## Summary\n\nAfter reading this article, we hope you find it easy to build your own Q\u0026A System.\n\nWith the BERT model, you no longer need to sort and organize the text corpora beforehand. At the same time, thanks to the high performance and high scalability of the open source vector search engine Milvus, your QA system can support a corpus of up to hundreds of millions of texts.\n\nMilvus has officially joined the Linux AI (LF AI) Foundation for incubation. You are welcome to join the Milvus community and work with us to accelerate the application of AI technologies!\n\n=\u003e Try our online demo here: https://www.milvus.io/scenarios\n\n\n\n\n\n\n\n\n ","title":"Building an Intelligent QA System with NLP and Milvus","metaData":{}},{"id":"2020-04-13-dynamic-data-update-and-query-milvus.md","author":"milvus","desc":"Vector search is now more intuitive and convenient","canonicalUrl":"https://zilliz.com/blog/dynamic-data-update-and-query-milvus","date":"2020-04-13T21:02:08.632Z","cover":"https://assets.zilliz.com/header_62d7b8c823.png","tags":["Engineering"],"href":"/blog/2020-04-13-dynamic-data-update-and-query-milvus.md","content":" \n# How Milvus Implements Dynamic Data Update And Query\nIn this article, we will mainly describe how vector data are recorded in the memory of Milvus, and how these records are maintained.\n\nBelow are our main design goals:\n\n1. Efficiency of data import should be high.\n2. Data can be seen as soon as possible after data import.\n3. Avoid fragmentation of data files.\n\nTherefore, we have established a memory buffer (insert buffer) to insert data to reduce the number of context switches of random IO on the disk and operating system to improve the performance of data insertion. The memory storage architecture based on MemTable and MemTableFile enables us to manage and serialize data more conveniently. The state of the buffer is divided into Mutable and Immutable, which allows the data to be persisted to disk while keeping external services available.\n\n## Preparation\n\nWhen the user is ready to insert a vector into Milvus, he first needs to create a Collection (* Milvus renames Table to Collection in 0.7.0 version). Collection is the most basic unit for recording and searching vectors in Milvus.\n\nEach Collection has a unique name and some properties that can be set, and vectors are inserted or searched based on the Collection name. When creating a new Collection, Milvus will record the information of this Collection in the metadata.\n\n## Data Insertion\n\nWhen the user sends a request to insert data, the data are serialized and deserialized to reach the Milvus server. Data are now written into memory. Memory writing is roughly divided into the following steps:\n\n\n\n1. In MemManager, find or create a new MemTable corresponding to the name of the Collection. Each MemTable corresponds to a Collection buffer in memory.\n2. A MemTable will contain one or more MemTableFile. Whenever we create a new MemTableFile, we will record this information in the Meta at the same time. We divide MemTableFile into two states: Mutable and Immutable. When the size of MemTableFile reaches the threshold, it will become Immutable. Each MemTable can only have one Mutable MemTableFile to be written at any time.\n3. The data of each MemTableFile will be finally recorded in the memory in the format of the set index type. MemTableFile is the most basic unit for managing data in memory.\n4. At any time, the memory usage of the inserted data will not exceed the preset value (insert_buffer_size). This is because every request to insert data comes in, MemManager can easily calculate the memory occupied by the MemTableFile contained in each MemTable, and then coordinate the insertion request according to the current memory.\n\nThrough MemManager, MemTable and MemTableFile multi-level architecture, data insertion can be better managed and maintained. Of course, they can do much more than that.\n\n## Near Real-time Query\n\nIn Milvus, you only need to wait for one second at the longest for the inserted data to move from memory to disk. This entire process can be roughly summarized by the following picture:\n\n\n\nFirst, the inserted data will enter an insert buffer in memory. The buffer will periodically change from the initial Mutable state to the Immutable state in preparation for serialization. Then, these Immutable buffers will be serialized to disk periodically by the background serialization thread. After the data are placed, the order information will be recorded in the metadata. At this point, the data can be searched!\n\nNow, we will describe the steps in the picture in detail.\n\nWe already know the process of inserting data into the mutable buffer. The next step is to switch from the mutable buffer to the immutable buffer:\n\n\n\nImmutable queue will provide the background serialization thread with the immutable state and the MemTableFile that is ready to be serialized. Each MemTable manages its own immutable queue, and when the size of the MemTable’s only mutable MemTableFile reaches the threshold, it will enter the immutable queue. A background thread responsible for ToImmutable will periodically pull all the MemTableFiles in the immutable queue managed by MemTable and send them to the total Immutable queue. It should be noted that the two operations of writing data into the memory and changing the data in the memory into a state that cannot be written cannot occur at the same time, and a common lock is required. However, the operation of ToImmutable is very simple and almost does not cause any delay, so the performance impact on inserted data is minimal.\n\nThe next step is to serialize the MemTableFile in the serialization queue to disk. This is mainly divided into three steps:\n\n\n\nFirst, the background serialization thread will periodically pull MemTableFile from the immutable queue. Then, they are serialized into fixed-size raw files (Raw TableFiles). Finally, we will record this information in the metadata. When we conduct a vector search, we will query the corresponding TableFile in the metadata. From here, these data can be searched!\n\nIn addition, according to the set index_file_size, after the serialization thread completes a serialization cycle, it will merge some fixed-size TableFiles into a TableFile, and also record these information in the metadata. At this time, the TableFile can be indexed. Index building is also asynchronous. Another background thread responsible for index building will periodically read the TableFile in the ToIndex state of the metadata to perform the corresponding index building.\n\n## Vector search\n\nIn fact, you will find that with the help of TableFile and metadata, vector search becomes more intuitive and convenient. In general, we need to get the TableFiles corresponding to the queried Collection from the metadata, search in each TableFile, and finally merge. In this article, we do not delve into the specific implementation of search.\n\nIf you want to know more, welcome to read our source code, or read our other technical articles about Milvus!\n\n ","title":"How Milvus Implements Dynamic Data Update And Query","metaData":{}},{"id":"2020-03-17-mishards-distributed-vector-search-milvus.md","author":"milvus","desc":"How to scale out","canonicalUrl":"https://zilliz.com/blog/mishards-distributed-vector-search-milvus","date":"2020-03-17T21:36:16.974Z","cover":"https://assets.zilliz.com/tim_j_ots0_EO_Yu_Gt_U_unsplash_14f939b344.jpg","tags":["Engineering"],"href":"/blog/2020-03-17-mishards-distributed-vector-search-milvus.md","content":" \n# Mishards — Distributed Vector Search in Milvus\nMilvus aims to achieve efficient similarity search and analytics for massive-scale vectors. A standalone Milvus instance can easily handle vector search for billion-scale vectors. However, for 10 billion, 100 billion or even larger datasets, a Milvus cluster is needed. The cluster can be used as a standalone instance for upper-level applications and can meet the business needs of low latency, high concurrency for massive-scale data. A Milvus cluster can resend requests, separate reading from writing, scale horizontally, and expand dynamically, thus providing a Milvus instance that can expand without limit. Mishards is a distributed solution for Milvus.\n\nThis article will briefly introduce components of the Mishards architecture. More detailed information will be introduced in the upcoming articles.\n\n\n\n## Distributed architecture overview\n\n\n\n## Service tracing\n\n\n\n## Primary service components\n\n- Service discovery framework, such as ZooKeeper, etcd, and Consul.\n- Load balancer, such as Nginx, HAProxy, Ingress Controller.\n- Mishards node: stateless, scalable.\n- Write-only Milvus node: single node and not scalable. You need to use high availability solutions for this node to avoid single-point-of-failure.\n- Read-only Milvus node: Stateful node and scalable.\n- Shared storage service: All Milvus nodes use shared storage service to share data, such as NAS or NFS.\n- Metadata service: All Milvus nodes use this service to share metadata. Currently, only MySQL is supported. This service requires MySQL high availability solution.\n\n## Scalable components\n- Mishards\n- Read-only Milvus nodes\n\n## Components introduction\n**Mishards nodes**\n\nMishards is responsible for breaking up upstream requests and routing sub-requests to sub-services. The results are summarized to return to upstream.\n\n\n\nAs is indicated in the chart above, after accepting a TopK search request, Mishards first breaks up the request into sub-requests and send the sub-requests to the downstream service. When all sub-responses are collected, the sub-responses are merged and returned to upstream.\n\nBecause Mishards is a stateless service, it does not save data or participate in complex computation. Thus, nodes do not have high configuration requirements and the computing power is mainly used in merging sub-results. So, it is possible to increase the number of Mishards nodes for high concurrency.\n\n## Milvus nodes\n\nMilvus nodes are responsible for CRUD related core operations, so they have relatively high configuration requirements. Firstly, the memory size should be large enough to avoid too many disk IO operations. Secondly, CPU configurations can also affect performance. As the cluster size increases, more Milvus nodes are required to increase system throughput.\n\n### Read-only nodes and writable nodes\n\n- Core operations of Milvus are vector insertion and search. Search has extremely high requirements on CPU and GPU configurations, while insertion or other operations have relatively low requirements. Separating the node that runs search from the node that runs other operations leads to more economical deployment.\n- In terms of service quality, when a node is performing search operations, the related hardware is running in full load and cannot ensure the service quality of other operations. Therefore, two node types are used. Search requests are processed by read-only nodes and other requests are processed by writable nodes.\n\n### Only one writable node is allowed\n\n- Currently, Milvus does not support sharing data for multiple writable instances.\n\n- During deployment, a single-point-of-failure of writable nodes needs to be considered. High availability solutions need to be prepared for writable nodes.\n\n### Read-only node scalability\n\nWhen the data size is extremely large, or the latency requirement is extremely high, you can horizontally scale read-only nodes as stateful nodes. Assume there are 4 hosts and each has the following configuration: CPU Cores: 16, GPU: 1, Memory: 64 GB. The following chart shows the cluster when horizontally scaling stateful nodes. Both computing power and memory scale linearly. The data is split into 8 shards with each node processing requests from 2 shards.\n\n\n\nWhen the number of requests is large for some shards, stateless read-only nodes can be deployed for these shards to increase throughput. Take the hosts above as an example. when the hosts are combined into a serverless cluster, the computing power increases linearly. Because the data to process does not increase, the processing power for the same data shard also increases linearly.\n\n\n\n### Metadata service\n\nKeywords: MySQL\n\nFor more information about Milvus metadata, refer to How to view metadata. In a distributed system, Milvus writable nodes are the only producer of metadata. Mishards nodes, Milvus writable nodes, and Milvus read-only nodes are all consumers of metadata. Currently, Milvus only supports MySQL and SQLite as the storage backend of metadata. In a distributed system, the service can only be deployed as highly-available MySQL.\n\n### Service discovery\n\nKeywords: Apache Zookeeper, etcd, Consul, Kubernetes\n\n\n\nService discovery provides information about all Milvus nodes. Milvus nodes register their information when going online and log out when going offline. Milvus nodes can also detect abnormal nodes by periodically checking the health status of services.\n\nService discovery contains a lot of frameworks, including etcd, Consul, ZooKeeper, etc. Mishards defines the service discovery interfaces and provides possibilities for scaling by plugins. Currently, Mishards provides two kinds of plugins, which correspond to Kubernetes cluster and static configurations. You can customize your own service discovery by following the implementation of these plugins. The interfaces are temporary and need a redesign. More information about writing your own plugin will be elaborated in the upcoming articles.\n\n### Load balancing and service sharding\n\nKeywords: Nginx, HAProxy, Kubernetes\n\n\n\nService discovery and load balancing are used together. Load balancing can be configured as polling, hashing, or consistent hashing.\n\nThe load balancer is responsible for resending user requests to the Mishards node.\n\nEach Mishards node acquires the information of all downstream Milvus nodes via the service discovery center. All related metadata can be acquired by metadata service. Mishards implements sharding by consuming these resources. Mishards defines the interfaces related to routing strategies and provides extensions via plugins. Currently, Mishards provides a consistent hashing strategy based on the lowest segment level. As is shown in the chart, there are 10 segments, s1 to s10. Per the segment-based consistent hashing strategy, Mishards routes requests concerning s1, 24, s6, and s9 to the Milvus 1 node, s2, s3, s5 to the Milvus 2 node, and s7, s8, s10 to the Milvus 3 node.\n\nBased on your business needs, you can customize routing by following the default consistent hashing routing plugin.\n\n### Tracing\n\nKeywords: OpenTracing, Jaeger, Zipkin\n\nGiven the complexity of a distributed system, requests are sent to multiple internal service invocations. To help pinpoint problems, we need to trace the internal service invocation chain. As the complexity increases, the benefits of an available tracing system are self-explanatory. We choose the CNCF OpenTracing standard. OpenTracing provides platform-independent, vendor-independent APIs for developers to conveniently implement a tracing system.\n\n\n\nThe previous chart is an example of tracing during search invocation. Search invokes \u003ccode\u003eget_routing\u003c/code\u003e, \u003ccode\u003edo_search\u003c/code\u003e, and \u003ccode\u003edo_merge\u003c/code\u003e consecutively. \u003ccode\u003edo_search\u003c/code\u003e also invokes \u003ccode\u003esearch_127.0.0.1\u003c/code\u003e.\n\nThe whole tracing record forms the following tree:\n\n\n\nThe following chart shows examples of request/response info and tags of each node:\n\n\n\nOpenTracing has been integrated to Milvus. More information will be covered in the upcoming articles.\n\n### Monitoring and alerting\n\nKeywords: Prometheus, Grafana\n\n\n\n## Summary\n\nAs the service middleware, Mishards integrates service discovery, routing request, result merging, and tracing. Plugin-based expansion is also provided. Currently, distributed solutions based on Mishards still have the following setbacks:\n\n- Mishards uses proxy as the middle layer and has latency costs.\n- Milvus writable nodes are single-point services.\n- Dependent on highly-available MySQL service.\n-Deployment is complicated when there are multiple shards and a single shard has multiple copies.\n- Lacks a cache layer, such as access to metadata.\n\nWe will fix these know issues in the upcoming versions so that Mishards can be applied to the production environment more conveniently.\n\n\n\n\n\n\n\n\n\n\n ","title":"Mishards — Distributed Vector Search in Milvus","metaData":{}},{"id":"2020-03-03-scheduling-query-tasks-milvus.md","author":"milvus","desc":"The work behind the scene","canonicalUrl":"https://zilliz.com/blog/scheduling-query-tasks-milvus","date":"2020-03-03T22:38:17.829Z","cover":"https://assets.zilliz.com/eric_rothermel_Fo_KO_4_Dp_Xam_Q_unsplash_469fe12aeb.jpg","tags":["Engineering"],"href":"/blog/2020-03-03-scheduling-query-tasks-milvus.md","content":" \n# How Does Milvus Schedule Query Tasks\nn this article, we will discuss how Milvus schedules query tasks. We will also talk about problems, solutions, and future orientations for implementing Milvus scheduling.\n\n## Background\n\nWe know from Managing Data in Massive-Scale Vector Search Engine that vector similarity search is implemented by the distance between two vectors in high-dimensional space. The goal of vector search is to find K vectors that are closest to the target vector.\n\nThere are many ways to measure vector distance, such as Euclidean distance:\n\n\n\nwhere x and y are two vectors. n is the dimension of the vectors.\n\nIn order to find K nearest vectors in a dataset, Euclidean distance needs to be computed between the target vector and all vectors in the dataset to be searched. Then, vectors are sorted by distance to acquire K nearest vectors. The computational work is in direct proportion to the size of the dataset. The larger the dataset, the more computational work a query requires. A GPU, specialized for graph processing, happens to have a lot of cores to provide the required computing power. Thus, multi-GPU support is also taken into consideration during Milvus implementation.\n\n## Basic concepts\n\n### Data block(TableFile)\n\nTo improve support for massive-scale data search, we optimized the data storage of Milvus. Milvus splits the data in a table by size into multiple data blocks. During vector search, Milvus searches vectors in each data block and merges the results. One vector search operation consists of N independent vector search operations (N is the number of data blocks) and N-1 result merge operations.\n\n### Task queue(TaskTable)\n\nEach Resource has a task array, which records tasks belonging to the Resource. Each task has different states, including Start, Loading, Loaded, Executing, and Executed. The Loader and Executor in a computing device share the same task queue.\n\n### Query scheduling\n\n\n\n1. When the Milvus server starts, Milvus launches the corresponding GpuResource via the \u003ccode\u003egpu_resource_config\u003c/code\u003e parameters in the \u003ccode\u003eserver_config.yaml\u003c/code\u003e configuration file. DiskResource and CpuResource still cannot be edited in \u003ccode\u003eserver_config.yaml\u003c/code\u003e. GpuResource is the combination of \u003ccode\u003esearch_resources\u003c/code\u003e and \u003ccode\u003ebuild_index_resources\u003c/code\u003e and referred to as \u003ccode\u003e{gpu0, gpu1}\u003c/code\u003e in the following example:\n\n\n\n\n\n2. Milvus receives a request. Table metadata is stored in an external database, which is SQLite or MySQl for single-host and MySQL for distributed. After receiving a search request, Milvus validates whether the table exists and the dimension is consistent. Then, Milvus reads the TableFile list of the table.\n\n\n\n3. Milvus creates a SearchTask. Because the computation of each TableFile is performed independently, Milvus creates a SearchTask for each TableFile. As the basic unit of task scheduling, a SearchTask contains the target vectors, search parameters, and the filenames of TableFile.\n\n\n\n4. Milvus chooses a computing device. The device that a SearchTask performs computation depends on the **estimated completion** time for each device. The **estimated completion** time specifies the estimated interval between the current time and the estimated time when the computation completes.\n\nFor example, when a data block of a SearchTask is loaded to CPU memory, the next SearchTask is waiting in the CPU computation task queue and the GPU computation task queue is idle. The **estimated completion time** for CPU is equal to the sum of the estimated time cost of the previous SearchTask and the current SearchTask. The **estimated completion time** for a GPU is equal to the sum of the time for data blocks to be loaded to the GPU and the estimated time cost of the current SearchTask. The **estimated completion time** for a SearchTask in a Resource is equal to the average execution time of all SearchTasks in the Resource. Milvus then chooses a device with the least **estimated completion time** and assign SearchTask to the device.\n\nHere we assume that the **estimated completion time** for GPU1 is shorter.\n\n\n\n5. Milvus adds SearchTask to the task queue of DiskResource.\n\n6. Milvus moves SearchTask to the task queue of CpuResource. The loading thread in CpuResource loads each task from the task queue sequentially. CpuResource reads the corresponding data blocks to CPU memory.\n\n7. Milvus moves SearchTask to GpuResource. The loading thread in GpuResource copies data from CPU memory to GPU memory. GpuResource reads the corresponding data blocks to GPU memory.\n\n8. Milvus executes SearchTask in GpuResource. Because the result of a SearchTask is relatively small, the result is directly returned to CPU memory.\n\n \n\n9. Milvus merges the result of SearchTask to the whole search result.\n\n\n\nAfter all SearchTasks are complete, Milvus returns the whole search result to the client.\n\n## Index building\n\nIndex building is basically the same as the search process without the merging process. We will not talk about this in detail.\n\n## Performance optimization\n\n### Cache\n\nAs mentioned before, data blocks need to be loaded to corresponding storage devices such as CPU memory or GPU memory before computation. To avoid repetitive data loading, Milvus introduces LRU (Least Recently Used) cache. When the cache is full, new data blocks push away old data blocks. You can customize the cache size by the configuration file based on the current memory size. A large cache to store search data is recommended to effectively save data loading time and improve search performance.\n\n### Data loading and computation overlap\n\nThe cache cannot satisfy our needs for better search performance. Data needs to be reloaded when memory is insufficient or the size of the dataset is too large. We need to decrease the effect of data loading on search performance. Data loading, whether it be from disk to CPU memory or from CPU memory to GPU memory, belongs to IO operations and barely needs any computational work from processors. So, we consider performing data loading and computation in parallel for better resource usage.\n\nWe split the computation on a data block into 3 stages (loading from disk to CPU memory, CPU computation, result merging) or 4 stages (loading from disk to CPU memory, loading from CPU memory to GPU memory, GPU computation and result retrieval, and result merging). Take 3-stage computation as an example, we can launch 3 threads responsible for the 3 stages to function as instruction pipelining. Because the results sets are mostly small, result merging does not take much time. In some cases, the overlap of data loading and computation can reduce the search time by 1/2.\n\n\n\n## Problems and solutions\n\n### Different transmission speeds\n\nPreviously, Milvus uses the Round Robin strategy for multi-GPU task scheduling. This strategy worked perfectly in our 4-GPU server and the search performance was 4 times better. However, for our 2-GPU hosts, the performance was not 2 times better. We did some experiments and discovered that the data copy speed for a GPU was 11 GB/s. However, for another GPU, it was 3 GB/s. After referring to the mainboard documentation, we confirmed that the mainboard was connected to one GPU via PCIe x16 and another GPU via PCIe x4. That is to say, these GPUs have different copy speeds. Later, we added copy time to measure the optimal device for each SearchTask.\n\n## Future work\n\n### Hardware environment with increased complexity\n\nIn real conditions, the hardware environment may be more complicated. For hardware environments with multiple CPUs, memory with NUMA architecture, NVLink, and NVSwitch, communication across CPUs/GPUs brings a lot of opportunities for optimization.\n\nQuery optimization\n\nDuring experimentation, we discovered some opportunities for performance improvement. For example, when the server receives multiple queries for the same table, the queries can be merged under some conditions. By using data locality, we can improve the performance. These optimizations will be implemented in our future development.\nNow we already know how queries are scheduled and performed for the single-host, multi-GPU scenario. We will continue to introduce more inner mechanisms for Milvus in the upcoming articles.\n\n \n\n\n\n \n\n\n\n\n\n\n\n\n\n\n\n\n\n ","title":"How Does Milvus Schedule Query Tasks","metaData":{}},{"id":"2020-02-26-select-index-parameters-ivf-index.md","author":"milvus","desc":"Best practices for IVF index","canonicalUrl":"https://zilliz.com/blog/select-index-parameters-ivf-index","date":"2020-02-26T22:57:02.071Z","cover":"https://assets.zilliz.com/header_4d3fc44879.jpg","tags":["Engineering"],"href":"/blog/2020-02-26-select-index-parameters-ivf-index.md","content":" \n# How to Select Index Parameters for IVF Index\nIn [Best Practices for Milvus Configuration](https://medium.com/@milvusio/best-practices-for-milvus-configuration-f38f1e922418), some best practices for Milvus 0.6.0 configuration were introduced. In this article, we will also introduce some best practices for setting key parameters in Milvus clients for operations including creating a table, creating indexes, and searching. These parameters can affect search performance.\n\n## 1. \u003ccode\u003eindex_file_size\u003c/code\u003e\n\nWhen creating a table, the index_file_size parameter is used to specify the size, in MB, of a single file for data storage. The default is 1024. When vector data is being imported, Milvus incrementally combines data into files. When the file size reaches index_file_size, this file does not accept new data and Milvus saves new data to another file. These are all raw data files. When an index is created, Milvus generates an index file for each raw data file. For the IVFLAT index type, the index file size approximately equals to the size of the corresponding raw data file. For the SQ8 index, the size of an index file is approximately 30 percent of the corresponding raw data file.\n\nDuring a search, Milvus searches each index file one by one. Per our experience, when index_file_size changes from 1024 to 2048, the search performance improves by 30 percent to 50 percent. However, if the value is too large, large files may fail to be loaded to GPU memory (or even CPU memory). For example, if GPU memory is 2 GB and index_file_size is 3 GB, the index file cannot be loaded to GPU memory. Usually, we set index_file_size to 1024 MB or 2048 MB.\n\nThe following table shows a test using sift50m for index_file_size. The index type is SQ8.\n\n\n\nWe can see that in CPU mode and GPU mode, when index_file_size is 2048 MB instead of 1024 MB, the search performance significantly improves.\n\n## 2. \u003ccode\u003enlist\u003c/code\u003e **and** \u003ccode\u003enprobe\u003c/code\u003e\n\nThe \u003ccode\u003enlist\u003c/code\u003e parameter is used for index creating and the \u003ccode\u003enprobe\u003c/code\u003e parameter is used for searching. IVFLAT and SQ8 both use clustering algorithms to split a large number of vectors into clusters, or buckets. \u003ccode\u003enlist\u003c/code\u003e is the number of buckets during clustering.\n\nWhen searching using indexes, the first step is to find a certain number of buckets closest to the target vector and the second step is to find the most similar k vectors from the buckets by vector distance. \u003ccode\u003enprobe\u003c/code\u003e is the number of buckets in step one.\n\nGenerally, increasing \u003ccode\u003enlist\u003c/code\u003e leads to more buckets and fewer vectors in a bucket during clustering. As a result, the computation load decreases and search performance improves. However, with fewer vectors for similarity comparison, the correct result might be missed.\n\nIncreasing \u003ccode\u003enprobe\u003c/code\u003e leads to more buckets to search. As a result, the computation load increases and search performance deteriorates, but search precision improves. The situation may differ per datasets with different distributions. You should also consider the size of the dataset when setting \u003ccode\u003enlist\u003c/code\u003e and \u003ccode\u003enprobe\u003c/code\u003e. Generally, it is recommended that `nlist` can be \u003ccode\u003e4 * sqrt(n)\u003c/code\u003e, where n is the total number of vectors. As for \u003ccode\u003enprobe\u003c/code\u003e, you must make a trade-off between precision and efficiency and the best way is to determine the value through trial and error.\n\nThe following table shows a test using sift50m for \u003ccode\u003enlist\u003c/code\u003e and \u003ccode\u003enprobe\u003c/code\u003e. The index type is SQ8.\n\n\n\nThe table compares search performance and precision using different values of \u003ccode\u003enlist\u003c/code\u003e/\u003ccode\u003enprobe\u003c/code\u003e. Only GPU results are displayed because CPU and GPU tests have similar results. In this test, as the values of \u003ccode\u003enlist\u003c/code\u003e/\u003ccode\u003enprobe\u003c/code\u003e increase by the same percentage, search precision also increase. When \u003ccode\u003enlist\u003c/code\u003e = 4096 and \u003ccode\u003enprobe\u003c/code\u003e is 128, Milvus has the best search performance. In conclusion, when determining the values for \u003ccode\u003enlist\u003c/code\u003e and \u003ccode\u003enprobe\u003c/code\u003e, you must make a trade-off between performance and precision with consideration to different datasets and requirements.\n\n## Summary\n\n\u003ccode\u003eindex_file_size\u003c/code\u003e: When the data size is greater than \u003ccode\u003eindex_file_size\u003c/code\u003e, the greater the value of \u003ccode\u003eindex_file_size\u003c/code\u003e, the better the search performance.\n\u003ccode\u003enlist\u003c/code\u003e and \u003ccode\u003enprobe\u003c/code\u003e:You must make a trade-off between performance and precision.\n\n \n","title":"How to Select Index Parameters for IVF Index","metaData":{}},{"id":"2020-02-06-molecular-structure-similarity-with-milvus.md","author":"Shiyu Chen","desc":"How to run molecular structure similarity analysis in Milvus","canonicalUrl":"https://zilliz.com/blog/molecular-structure-similarity-with-milvus","date":"2020-02-06T19:08:18.815Z","cover":"https://assets.zilliz.com/header_44d6b6aacd.jpg","tags":["Scenarios"],"href":"/blog/2020-02-06-molecular-structure-similarity-with-milvus.md","content":" \n# Accelerating New Drug Discovery\n## Introduction\n\nDrug discovery, as the source of medicine innovation, is an important part of new medicine research and development. Drug discovery is implemented by target selection and confirmation. When fragments or lead compounds are discovered, similar compounds are usually searched in internal or commercial compound libraries in order to discover structure-activity relationship (SAR), compound availability, thus evaluating the potential of the lead compounds to be optimized to candidate compounds.\n\nIn order to discover available compounds in the fragment space from billion-scale compound libraries, chemical fingerprint is usually retrieved for substructure search and similarity search. However, the traditional solution is time-consuming and error-prone when it comes to billion-scale high-dimensional chemical fingerprints. Some potential compounds may also be lost in the process. This article discusses using Milvus, a similarity search engine for massive-scale vectors, with RDKit to build a system for high-performance chemical structure similarity search.\n\nCompared with traditional methods, Milvus has faster search speed and broader coverage. By processing chemical fingerprints, Milvus can perform substructure search, similarity search, and exact search in chemical structure libraries in order to discover potentially available medicine.\n\n## System overview\n\nThe system uses RDKit to generate chemical fingerprints, and Milvus to perform chemical structure similarity search. Refer to https://github.com/milvus-io/bootcamp/tree/master/solutions/molecular_similarity_search to learn more about the system.\n\n\n\n## 1. Generating chemical fingerprints\n\nChemical fingerprints are usually used for substructure search and similarity search. The following image shows a sequential list represented by bits. Each digit represents an element, atom pair, or functional groups. The chemical structure is \u003ccode\u003eC1C(=O)NCO1\u003c/code\u003e.\n\n\n\nWe can use RDKit to generate Morgan fingerprints, which defines a radius from a specific atom and calculates the number of chemical structures within the range of the radius to generate a chemical fingerprint. Specify different values for the radius and bits to acquire the chemical fingerprints of different chemical structures. The chemical structures are represented in SMILES format.\n\n from rdkit import Chem\n mols = Chem.MolFromSmiles(smiles)\n mbfp = AllChem.GetMorganFingerprintAsBitVect(mols, radius=2, bits=512)\n mvec = DataStructs.BitVectToFPSText(mbfp)\n\n## 2. Searching chemical structures\n\nWe can then import the Morgan fingerprints into Milvus to build a chemical structure database. With different chemical fingerprints, Milvus can perform substructure search, similarity search, and exact search.\n\n from milvus import Milvus\n Milvus.add_vectors(table_name=MILVUS_TABLE, records=mvecs)\n Milvus.search_vectors(table_name=MILVUS_TABLE, query_records=query_mvec, top_k=topk)\n\n### Substructure search\nChecks whether a chemical structure contains another chemical structure.\n\n### Similarity search\nSearches similar chemical structures. Tanimoto distance is used as the metric by default.\n\n### Exact search\nChecks whether a specified chemical structure exists. This kind of search requires exact match.\n\n## Computing chemical fingerprints\nTanimoto distance is often used as a metric for chemical fingerprints. In Milvus, Jaccard distance corresponds with Tanimoto distance.\n\n\n\nBased on the previous parameters, chemical fingerprint computation can be described as:\n\n\n\nWe can see that \u003ccode\u003e1- Jaccard = Tanimoto\u003c/code\u003e. Here we use Jaccard in Milvus to compute the chemical fingerprint, which is actually consistent with Tanimoto distance.\n\n## System demo\n\nTo better demonstrate how the system works, we have built a demo that uses Milvus to search more than 90 million chemical fingerprints. The data used comes from ftp://ftp.ncbi.nlm.nih.gov/pubchem/Compound/CURRENT-Full/SDF. The initial interface looks as follows:\n\n\n\nWe can search specified chemical structures in the system and returns similar chemical structures:\n\n\n\n## Conclusion\n\nSimilarity search is indispensable in a number of fields, such as images and videos. For drug discovery, similarity search can be applied to chemical structure databases to discover potentially available compounds, which are then converted to seeds for practical synthesis and point-of-care testing. Milvus, as an open-source similarity search engine for massive-scale feature vectors, is built with heterogeneous computing architecture for the best cost efficiency. Searches over billion-scale vectors take only milliseconds with minimum computing resources. Thus, Milvus can help implement accurate, fast chemical structure search in fields such as biology and chemistry.\n\nYou can access the demo by visiting http://40.117.75.127:8002/, and don’t forget to also pay a visit to our GitHub https://github.com/milvus-io/milvus to learn more!\n\n\n\n\n\n\n\n \n","title":"Accelerating New Drug Discovery","metaData":{}},{"id":"2019-12-31-managing-metadata-in-milvus-2.md","author":"milvus","desc":"Fields in the Metadata Table","canonicalUrl":"https://zilliz.com/blog/managing-metadata-in-milvus-2","date":"2019-12-31T20:41:13.864Z","cover":"https://assets.zilliz.com/header_c65a2a523c.png","tags":["Engineering"],"href":"/blog/2019-12-31-managing-metadata-in-milvus-2.md","content":" \n# Milvus Metadata Management (2)\nIn the last blog, we mentioned how to view your metadata using MySQL or SQLite. This article mainly intends to introduce in detail the fields in the metadata tables.\n\n## Fields in the \u003ccode\u003eTables\u003c/code\u003e table\n\nTake SQLite as an example. The following result comes from 0.5.0. Some fields are added to 0.6.0, which will be introduced later. There is a row in \u003ccode\u003eTables\u003c/code\u003e specifying a 512-dimensional vector table with the name \u003ccodetable_1\u003c/code\u003e. When the table is created, \u003ccode\u003eindex_file_size\u003c/code\u003e is 1024 MB, \u003ccode\u003eengine_type\u003c/code\u003e is 1 (FLAT), \u003ccode\u003enlist\u003c/code\u003e is 16384, \u003ccode\u003emetric_type\u003c/code\u003e is 1 (Euclidean distance L2). id is the unique identifier of the table. \u003ccode\u003estate\u003c/code\u003e is the state of the table with 0 indicating a normal state. \u003ccode\u003ecreated_on\u003c/code\u003e is the creation time. \u003ccode\u003eflag\u003c/code\u003e is the flag reserved for internal use.\n\n\n\nThe following table shows field types and descriptions of the fields in \u003ccode\u003eTables\u003c/code\u003e.\n\n\n\nTable partitioning is enabled in 0.6.0 with a few new fields, including \u003ccode\u003eowner_table\u003c/code\u003e,\u003ccode\u003epartition_tag\u003c/code\u003e and \u003ccode\u003eversion\u003c/code\u003e. A vector table, \u003ccode\u003etable_1\u003c/code\u003e, has a partition called \u003ccode\u003etable_1_p1\u003c/code\u003e, which is also a vector table. \u003ccode\u003epartition_name\u003c/code\u003e corresponds to \u003ccode\u003etable_id\u003c/code\u003e. Fields in a partition table are inherited from the \u003ccode\u003eowner table\u003c/code\u003e, with the owner table field specifying the name of the owner table and the \u003ccode\u003epartition_tag\u003c/code\u003e field specifying the tag of the partition.\n\n\n\nThe following table shows new fields in 0.6.0:\n\n\n\n## Fields in the TableFiles table\n\nThe following example contains two files, which both belong to the \u003ccode\u003etable_1\u003c/code\u003e vector table. The index type (\u003ccode\u003eengine_type\u003c/code\u003e) of the first file is 1 (FLAT); file status (\u003ccode\u003efile_type\u003c/code\u003e) is 7 (backup of the original file); \u003ccode\u003efile_size\u003c/code\u003e is 411200113 bytes; number of vector rows is 200,000. The index type of the second file is 2 (IVFLAT); file status is 3 (index file). The second file is actually the index of the first file. We will introduce more information in upcoming articles.\n\n\n\nThe following table shows fields and descriptions of \u003ccode\u003eTableFiles\u003c/code\u003e:\n\n\n\n\n## What’s coming next\n\nThe upcoming article will show you how to use SQLite to manage metadata in Milvus. Stay tuned!\n\nAny questions, welcome to join our [Slack channel](https://join.slack.com/t/milvusio/shared_invite/enQtNzY1OTQ0NDI3NjMzLWNmYmM1NmNjOTQ5MGI5NDhhYmRhMGU5M2NhNzhhMDMzY2MzNDdlYjM5ODQ5MmE3ODFlYzU3YjJkNmVlNDQ2ZTk)or file an issue in the repo.\n\nGitHub repo: https://github.com/milvus-io/milvus\n\n\n ","title":"Milvus Metadata Management (2)","metaData":{}},{"id":"2019-12-27-meta-table.md","author":"Yihua Mo","desc":"Learn about the detail of the fields in metadata tables in Milvus.","date":"2019-12-27T00:00:00.000Z","cover":"https://zilliz-cms.s3.us-west-2.amazonaws.com/pc_blog_8ed7696269.jpg","tags":["Engineering"],"href":"/blog/2019-12-27-meta-table.md","content":"\n# Milvus Metadata Management (2)\n\n## Fields in the Metadata Table\n\n\u003e Author: Yihua Mo\n\u003e\n\u003e Date: 2019-12-27\n\nIn the last blog, we mentioned how to view your metadata using MySQL or SQLite. This article mainly intends to introduce in detail the fields in the metadata tables.\n\n### Fields in the \"`Tables`” table\n\nTake SQLite as an example. The following result comes from 0.5.0. Some fields are added to 0.6.0, which will be introduced later. There is a row in `Tables` specifying a 512-dimensional vector table with the name `table_1`. When the table is created, `index_file_size` is 1024 MB, `engine_type` is 1 (FLAT), `nlist` is 16384, `metric_type` is 1 (Euclidean distance L2). `id` is the unique identifier of the table. `state` is the state of the table with 0 indicating a normal state. `created_on` is the creation time. `flag` is the flag reserved for internal use.\n\n\n\nThe following table shows field types and descriptions of the fields in `Tables`.\n\n| Field Name | Data Type | Description |\n| :---------------- | :-------- | :------------------------------------------------------------------------------------------------------------------------------------------------------------------------------------------ |\n| `id` | int64 | Unique identifier of the vector table. `id` automatically increments. |\n| `table_id` | string | Name of the vector table. `table_id` must be user-defined and follow Linux filename guidelines. |\n| `state` | int32 | State of the vector table. 0 stands for normal and 1 stands for deleted (soft delete). |\n| `dimension` | int16 | Vector dimension of the vector table. Must be user-defined. |\n| `created_on` | int64 | Number of milliseconds from Jan 1, 1970 to the time when the table is created. |\n| `flag` | int64 | Flag for internal use, such as whether the vector id is user-defined. The default is 0. |\n| `index_file_size` | int64 | If the size of a data file reaches `index_file_size`, the file is not combined and is used to build indexes. The default is 1024 (MB). |\n| `engine_type` | int32 | Type of index to build for a vector table. The default is 0, which specifies invalid index. 1 specifies FLAT. 2 specifies IVFLAT. 3 specifies IVFSQ8. 4 specifies NSG. 5 specifies IVFSQ8H. |\n| `nlist` | int32 | Number of clusters the vectors in each data file are divided into when the index is being built. The default is 16384. |\n| `metric_type` | int32 | Method to compute vector distance. 1 specifies Euclidean distance (L1) and 2 specifies inner product. |\n\nTable partitioning is enabled in 0.6.0 with a few new fields, including `owner_table`,`partition_tag` and `version`. A vector table, `table_1`, has a partition called `table_1_p1`, which is also a vector table. `partition_name` corresponds to `table_id`. Fields in a partition table are inherited from the owner table, with the `owner table` field specifying the name of the owner table and the `partition_tag` field specifying the tag of the partition.\n\n\n\nThe following table shows new fields in 0.6.0:\n\n| Field Name | Data Type | Description |\n| :-------------- | :-------- | :------------------------------------------------- |\n| `owner_table` | string | Parent table of the partition. |\n| `partition_tag` | string | Tag of the partition. Must not be an empty string. |\n| `version` | string | Milvus version. |\n\n### Fields in the “`TableFiles\"` table\n\nThe following example contains two files, which both belong to the `table_1` vector table. The index type (`engine_type`) of the first file is 1 (FLAT); file status (`file_type`) is 7 (backup of the original file); `file_size` is 411200113 bytes; number of vector rows is 200,000. The index type of the second file is 2 (IVFLAT); file status is 3 (index file). The second file is actually the index of the first file. We will introduce more information in upcoming articles.\n\n\n\nThe following table shows fields and descriptions of `TableFiles`:\n\n| Field Name | Data Type | Description |\n| :------------- | :-------- | :-------------------------------------------------------------------------------------------------------------------------------------------------------------------------------------------------------------------------------------------------------------------------------------------------------------------------------------------------------------------------------------------------------------------------------------------------------------------------------- |\n| `id` | int64 | Unique identifier of a vector table. `id` automatically increments. |\n| `table_id` | string | Name of the vector table. |\n| `engine_type` | int32 | Type of index to build for a vector table. The default is 0, which specifies invalid index. 1 specifies FLAT. 2 specifies IVFLAT. 3 specifies IVFSQ8. 4 specifies NSG. 5 specifies IVFSQ8H. |\n| `file_id` | string | Filename generated from file creation time. Equals 1000 multiplied by the number of milliseconds from Jan 1, 1970 to the time when the table is created. |\n| `file_type` | int32 | File status. 0 specifies a newly generated raw vector data file. 1 specifies raw vector data file. 2 specifies that index will be built for the file. 3 specifies that the file is an index file. 4 specifies that the file will be deleted (soft delete). 5 specifies that the file is newly-generated and used to store combination data. 6 specifies that the file is newly-generated and used to store index data. 7 specifies the backup status of the raw vector data file. |\n| `file_size` | int64 | File size in bytes. |\n| `row_count` | int64 | Number of vectors in a file. |\n| `updated_time` | int64 | Timestamp for the latest update time, which specifies the number of milliseconds from Jan 1, 1970 to the time when the table is created. |\n| `created_on` | int64 | Number of milliseconds from Jan 1, 1970 to the time when the table is created. |\n| `date` | int32 | Date when the table is created. It is still here for historical reasons and will be removed in future versions. |\n\n## Related blogs\n\n- [Managing Data in Massive Scale Vector Search Engine](https://medium.com/@milvusio/managing-data-in-massive-scale-vector-search-engine-db2e8941ce2f)\n- [Milvus Metadata Management (1): How to View Metadata](https://medium.com/@milvusio/milvus-metadata-management-1-6b9e05c06fb0)\n","title":"Milvus Metadata Management (2) Fields in the Metadata Table","metaData":{}},{"id":"2019-12-25-managing-metadata-in-milvus-1.md","author":"milvus","desc":"Learnn about how to view metadata in the Milvus vector database.","canonicalUrl":"https://zilliz.com/blog/managing-metadata-in-milvus-1","date":"2019-12-25T19:21:42.469Z","cover":"https://assets.zilliz.com/header_c2eb459468.jpg","tags":["Engineering"],"href":"/blog/2019-12-25-managing-metadata-in-milvus-1.md","content":" \n# Milvus Metadata Management (1)\nWe introduced some information about metadata in [Managing Data in Massive-Scale Vector Search Engine](https://medium.com/@milvusio/managing-data-in-massive-scale-vector-search-engine-db2e8941ce2f). This article mainly shows how to view the metadata of Milvus.\n\nMilvus supports metadata storage in SQLite or MySQL. There’s a parameter \u003ccode\u003ebackend_url\u003c/code\u003e (in the configuration file \u003ccode\u003eserver_config.yaml\u003c/code\u003e) by which you can specify if to use SQLite or MySQL to manage your metadata.\n\n## SQLite\n\nIf SQLite is used, a \u003ccode\u003emeta.sqlite\u003c/code\u003e file will be generated in the data directory (defined in the \u003ccode\u003eprimary_path\u003c/code\u003e of the configuration file \u003ccode\u003eserver_config.yaml\u003c/code\u003e) after Milvus is started. To view the file, you only need to install a SQLite client.\n\nInstall SQLite3 from the command line:\n\n sudo apt-get install sqlite3\n\nThen enter the Milvus data directory, and open the meta file using SQLite3:\n\n sqlite3 meta.sqlite\n\nNow, you’ve already entered the SQLite client command line. Just use a few commands to see what is in the metadata.\n\nTo make the printed results typeset easier for humans to read:\n\n . mode column\n . header on\n\nTo query Tables and TableFiles using SQL statements (case-insensitive):\n\n SELECT * FROM Tables\n SELECT * FROM TableFiles\n\n\n\n## MySQL\n\nIf you are using MySQL, you need to specify the address of the MySQL service in the \u003ccode\u003ebackend_url\u003c/code\u003e of the configuration file \u003ccode\u003eserver_config.yaml\u003c/code\u003e.\n\nFor example, the following settings indicate that the MySQL service is deployed locally, with port ‘3306’, user name ‘root’, password ‘123456’, and database name ‘milvus’:\n\n db_config:\n backend_url: mysql://root:123456@127.0.0.1:3306/milvus\n\nFirst of all, install MySQL client:\n\nsudo apt-get install default-mysql-client\n\nAfter Milvus is started, two tables (Tables and TableFiles) will be created in the MySQL service specified by \u003ccode\u003ebackend_url\u003c/code\u003e.\n\nUse the following command to connect to MySQL service:\n\n mysql -h127.0.0.1 -uroot -p123456 -Dmilvus\n\nNow, you can use SQL statements to query metadata information:\n\n\n\n## What’s coming next\n\nNext articles will introduce in details the schema of metadata tables. Stay tuned!\n\nAny questions, welcome to join our [Slack channel](https://join.slack.com/t/milvusio/shared_invite/enQtNzY1OTQ0NDI3NjMzLWNmYmM1NmNjOTQ5MGI5NDhhYmRhMGU5M2NhNzhhMDMzY2MzNDdlYjM5ODQ5MmE3ODFlYzU3YjJkNmVlNDQ2ZTk) or file an issue in the repo.\n\nGitHub repo: https://github.com/milvus-io/milvus\n\nIf you like this article or find it useful, don’t forget to clap!\n\n\n\n \n","title":"Milvus Metadata Management (1)","metaData":{}},{"id":"2019-12-24-view-metadata.md","author":"Yihua Mo","desc":"Milvus supports metadata storage in SQLite or MySQL. This post introduces how to view metadata With SQLite and MySQL.","isPublish":false,"date":"2019-12-24T00:00:00.000Z","cover":"https://zilliz-cms.s3.us-west-2.amazonaws.com/pc_blog_2_9e3f35962c.jpg","tags":["Engineering"],"href":"/blog/2019-12-24-view-metadata.md","content":"\n# Milvus Metadata Management (1)\n\n## How to View Metadata\n\n\u003e Author: Yihua Mo\n\u003e\n\u003e Date: 2019-12-24\n\nWe introduced some information about metadata in [Managing Data in Massive-Scale Vector Search Engine](https://medium.com/@milvusio/managing-data-in-massive-scale-vector-search-engine-db2e8941ce2f). This article mainly shows how to view the metadata of Milvus.\n\nMilvus supports metadata storage in SQLite or MySQL. There’s a parameter `backend_url` (in the configuration file `server_config.yaml`) by which you can specify if to use SQLite or MySQL to manage your metadata.\n\n### SQLite\n\nIf SQLite is used, a `meta.sqlite` file will be generated in the data directory (defined in the `primary_path` of the configuration file `server_config.yaml`) after Milvus is started. To view the file, you only need to install a SQLite client.\n\nInstall SQLite3 from the command line:\n\n```shell\nsudo apt-get install sqlite3\n```\n\nThen enter the Milvus data directory, and open the meta file using SQLite3:\n\n```shell\nsqlite3 meta.sqlite\n```\n\nNow, you’ve already entered the SQLite client command line. Just use a few commands to see what is in the metadata.\n\nTo make the printed results typeset easier for humans to read:\n\n```sql\n.mode column\n.header on\n```\n\nTo query Tables and TableFiles using SQL statements (case-insensitive):\n\n```sql\nSELECT \\* FROM Tables\n```\n\n```sql\nSELECT \\* FROM TableFiles\n```\n\n\n\n### MySQL\n\nIf you are using MySQL, you need to specify the address of the MySQL service in the `backend_url` of the configuration file `server_config.yaml`.\n\nFor example, the following settings indicate that the MySQL service is deployed locally, with port ‘3306’, user name ‘root’, password ‘123456’, and database name ‘milvus’:\n\n```\ndb_config:\n\n backend_url: mysql://root:123456@127.0.0.1:3306/milvus\n```\n\nFirst of all, install MySQL client:\n\n```shell\nsudo apt-get install default-mysql-client\n```\n\nAfter Milvus is started, two tables (Tables and TableFiles) will be created in the MySQL service specified by `backend_url`.\n\nUse the following command to connect to MySQL service:\n\n```shell\nmysql -h127.0.0.1 -uroot -p123456 -Dmilvus\n```\n\nNow, you can use SQL statements to query metadata information:\n\n\n\n## 相关博客\n\n- [Managing Data in Massive Scale Vector Search Engine](https://medium.com/@milvusio/managing-data-in-massive-scale-vector-search-engine-db2e8941ce2f)\n- [Milvus Metadata Management (2): Fields in the Metadata Table](https://medium.com/@milvusio/milvus-metadata-management-2-fields-in-the-metadata-table-3bf0d296ca6d)\n","title":"Milvus Metadata Management (1) How to View Metadata","metaData":{}},{"id":"2019-12-18-datafile-cleanup.md","author":"Yihua Mo","desc":"We improved the file delete strategy to fix the query operation related issues.","date":"2019-12-18T00:00:00.000Z","cover":"https://zilliz-cms.s3.us-west-2.amazonaws.com/pc_blog_8ed7696269.jpg","tags":["Engineering"],"href":"/blog/2019-12-18-datafile-cleanup.md","content":"\n# Improvements of the Data File Cleanup Mechanism\n\n\u003e author: Yihua Mo\n\u003e\n\u003e Date: 2019-12-18\n\n## Previous delete strategy and related problems\n\nIn [Managing Data in Massive-Scale Vector Search Engine](2019-11-08-data-management.md), we mentioned the delete mechanism of data files. Delete includes soft-delete and hard-delete. After performing a delete operation on a table, the table is marked with soft-delete. Search or update operations afterwards are no longer allowed. However, the query operation that starts before delete can still run. The table is really deleted together with metadata and other files only when the query operation is complete.\n\nSo, when the files marked with soft-delete are really deleted? Before 0.6.0, the strategy is that a file is really deleted after soft-deleted for 5 minutes. The following figure displays the strategy:\n\n \n\nThis strategy is based on the premise that queries normally do not last more than 5 minutes and is not reliable. If a query lasts more than 5 minutes, the query will fail. The reason is that when a query starts, Milvus collects information about files that can be searched and creates query tasks. Then, the query scheduler loads files to memory one by one and searches for files one by one. If a file no longer exists when loading a file, the query will fail.\n\nExtending the time may help reduce the risk of query failures, but also causes another problem: disk usage is too large. The reason is that when large quantities of vectors are being inserted, Milvus continually combines data files and the combined files are not immediately removed from the disk, even though no query happens. If data insertion is too fast and/or the amount of inserted data is too large, extra disk usage can amount to tens of GBs. Refer to the following figure as an example:\n\n\n\nAs shown in the previous figure, the first batch of inserted data (insert_1) is flushed to disk and becomes file_1, then insert_2 becomes file_2. The thread responsible for file combination combines the files to file_3. Then, file_1 and file_2 are marked as soft-delete. The third batch of insert data becomes file_4. The thread combines file_3 and file_4 to file_5 and marks file_3 and file_4 as soft-delete.\n\nLikewise, insert_6 and insert_5 are combined. In t3, file_5 and file_6 are marked as soft-delete. Between t3 and t4, although many files are marked as soft-delete, they are still in the disk. Files are really deleted after t4. Thus, between t3 and t4, the disk usage is 64 + 64 + 128 + 64 + 196 + 64 + 256 = 836 MB. The inserted data is 64 + 64 + 64 + 64 = 256 MB. The disk usage is 3 times the size of inserted data. The faster the write speed of the disk, the higher the disk usage during a specific time period.\n\n## Improvements of the delete strategy in 0.6.0\n\nThus, we changed the strategy to delete files in v0.6.0. Hard-delete no longer uses time as triggers. Instead, the trigger is when the file is not in use by any task.\n\n\n\nAssume two batches of vectors are inserted. In t1 a query request is given, Milvus acquires two files to be queried (file_1 and file_2, because file_3 still does not exist.) Then, the backend thread starts combining the two files with the query running at the same time. When file_3 is generated, file_1 and file_2 are marked as soft-delete. After the query, no other tasks will use file_1 and file_2, so they will be hard-deleted in t4. The interval between t2 and t4 is very small and depends on the interval of the query. In this way, unused files will be removed in time.\n\nAs for internal implementation, reference counting, which is familiar to software engineers, is used to determine whether a file can be hard-deleted. To explain using comparison, when a player has lives in a game, he can still play. When the number of lives becomes 0, the game is over. Milvus monitors the status of each file. When a file is used by a task, a life will be added to the file. When the file is no longer used, a life will be removed from the file. When a file is marked with soft-delete and the number of lives is 0, the file is ready for hard-delete.\n\n## Related blogs\n\n- [Managing Data in Massive-Scale Vector Search Engine](2019-11-08-data-management.md)\n- [Milvus Metadata Management (1): How to View Metadata](https://milvus.io/blog/managing-metadata-in-milvus-1.md)\n- [Milvus Metadata Management (2): Fields in the Metadata Table](2019-12-27-meta-table.md)\n","title":"Improvements of the Data File Cleanup Mechanism","metaData":{}},{"id":"2019-12-05-Accelerating-Similarity-Search-on-Really-Big-Data-with-Vector-Indexing.md","author":"milvus","desc":"Without vector indexing, many modern applications of AI would be impossibly slow. Learn how to select the right index for your next machine learning application.","canonicalUrl":"https://zilliz.com/blog/Accelerating-Similarity-Search-on-Really-Big-Data-with-Vector-Indexing","date":"2019-12-05T08:33:04.230Z","cover":"https://assets.zilliz.com/4_1143e443aa.jpg","tags":["Engineering"],"href":"/blog/2019-12-05-Accelerating-Similarity-Search-on-Really-Big-Data-with-Vector-Indexing.md","content":" \n# Accelerating Similarity Search on Really Big Data with Vector Indexing\nFrom computer vision to new drug discovery, vector similarity search engines power many popular artificial intelligence (AI) applications. A huge component of what makes it possible to efficiently query the million-, billion-, or even trillion-vector datasets that similarity search engines rely on is indexing, a process of organizing data that drastically accelerates big data search. This article covers the role indexing plays in making vector similarity search efficient, different vector inverted file (IVF) index types, and advice on which index to use in different scenarios.\n\n**Jump to:**\n\n- [Accelerating Similarity Search on Really Big Data with Vector Indexing](#accelerating-similarity-search-on-really-big-data-with-vector-indexing)\n - [How does vector indexing accelerate similarity search and machine learning?](#how-does-vector-indexing-accelerate-similarity-search-and-machine-learning)\n - [What are different types of IVF indexes and which scenarios are they best suited for?](#what-are-different-types-of-ivf-indexes-and-which-scenarios-are-they-best-suited-for)\n - [FLAT: Good for searching relatively small (million-scale) datasets when 100% recall is required.](#flat-good-for-searching-relatively-small-million-scale-datasets-when-100-recall-is-required)\n - [FLAT performance test results:](#flat-performance-test-results)\n - [*Query time test results for the FLAT index in Milvus.*](#query-time-test-results-for-the-flat-index-in-milvus)\n - [Key takeaways:](#key-takeaways)\n - [IVF_FLAT: Improves speed at the expense of accuracy (and vice versa).](#ivf_flat-improves-speed-at-the-expense-of-accuracy-and-vice-versa)\n - [IVF_FLAT performance test results:](#ivf_flat-performance-test-results)\n - [*Query time test results for IVF_FLAT index in Milvus.*](#query-time-test-results-for-ivf_flat-index-in-milvus)\n - [Key takeaways:](#key-takeaways-1)\n - [*Recall rate test results for the IVF_FLAT index in Milvus.*](#recall-rate-test-results-for-the-ivf_flat-index-in-milvus)\n - [Key takeaways:](#key-takeaways-2)\n - [IVF_SQ8: Faster and less resource hungry than IVF_FLAT, but also less accurate.](#ivf_sq8-faster-and-less-resource-hungry-than-ivf_flat-but-also-less-accurate)\n - [IVF_SQ8 performance test results:](#ivf_sq8-performance-test-results)\n - [*Query time test results for IVF_SQ8 index in Milvus.*](#query-time-test-results-for-ivf_sq8-index-in-milvus)\n - [Key takeaways:](#key-takeaways-3)\n - [*Recall rate test results for IVF_SQ8 index in Milvus.*](#recall-rate-test-results-for-ivf_sq8-index-in-milvus)\n - [Key takeaways:](#key-takeaways-4)\n - [IVF_SQ8H: New hybrid GPU/CPU approach that is even faster than IVF_SQ8.](#ivf_sq8h-new-hybrid-gpucpu-approach-that-is-even-faster-than-ivf_sq8)\n - [IVF_SQ8H performance test results:](#ivf_sq8h-performance-test-results)\n - [*Query time test results for IVF_SQ8H index in Milvus.*](#query-time-test-results-for-ivf_sq8h-index-in-milvus)\n - [Key takeaways:](#key-takeaways-5)\n - [Learn more about Milvus, a massive-scale vector data management platform.](#learn-more-about-milvus-a-massive-scale-vector-data-management-platform)\n - [Methodology](#methodology)\n - [Performance testing environment](#performance-testing-environment)\n - [Relevant technical concepts](#relevant-technical-concepts)\n - [Resources](#resources)\n\n\n### How does vector indexing accelerate similarity search and machine learning?\n\nSimilarity search engines work by comparing an input to a database to find objects that are most similar to the input. Indexing is the process of efficiently organizing data, and it plays a major role in making similarity search useful by dramatically accelerating time-consuming queries on large datasets. After a massive vector dataset is indexed, queries can be routed to clusters, or subsets of data, that are most likely to contain vectors similar to an input query. In practice, this means a certain degree of accuracy is sacrificed to speed up queries on really big vector data.\n\nAn analogy can be drawn to a dictionary, where words are sorted alphabetically. When looking up a word, it is possible to quickly navigate to a section that only contains words with the same initial — drastically accelerating the search for the input word’s definition.\n\n### What are different types of IVF indexes and which scenarios are they best suited for?\n\nThere are numerous indexes designed for high-dimensional vector similarity search, and each one comes with tradeoffs in performance, accuracy, and storage requirements. This article covers several common IVF index types, their strengths and weaknesses, as well as performance test results for each index type. Performance testing quantifies query time and recall rates for each index type in [Milvus](https://milvus.io/), an open-source vector data management platform. For additional information on the testing environment, see the methodology section at the bottom of this article.\n\n### FLAT: Good for searching relatively small (million-scale) datasets when 100% recall is required.\n\nFor vector similarity search applications that require perfect accuracy and depend on relatively small (million-scale) datasets, the FLAT index is a good choice. FLAT does not compress vectors, and is the only index that can guarantee exact search results. Results from FLAT can also be used as a point of comparison for results produced by other indexes that have less than 100% recall.\n\nFLAT is accurate because it takes an exhaustive approach to search, which means for each query the target input is compared to every vector in a dataset. This makes FLAT the slowest index on our list, and poorly suited for querying massive vector data. There are no parameters for the FLAT index in Milvus, and using it does not require data training or additional storage.\n\n#### FLAT performance test results:\n\nFLAT query time performance testing was conducted in Milvus using a dataset comprised of 2 million 128-dimensional vectors.\n\n\n\n#### Key takeaways:\n\n- As nq (the number of target vectors for a query) increases, query time increases.\n- Using the FLAT index in Milvus, we can see that query time rises sharply once nq exceeds 200.\n- In general, the FLAT index is faster and more consistent when running Milvus on GPU vs. CPU. However, FLAT queries on CPU are faster when nq is below 20.\n\n### IVF_FLAT: Improves speed at the expense of accuracy (and vice versa).\n\nA common way to accelerate the similarity search process at the expense of accuracy is to conduct an approximate nearest neighbor (ANN) search. ANN algorithms decrease storage requirements and computation load by clustering similar vectors together, resulting in faster vector search. IVF_FLAT is the most basic inverted file index type and relies on a form of ANN search.\n\nIVF_FLAT divides vector data into a number of cluster units (nlist), and then compares distances between the target input vector and the center of each cluster. Depending on the number of clusters the system is set to query (nprobe), similarity search results are returned based on comparisons between the target input and the vectors in the most similar cluster(s) only — drastically reducing query time.\n\nBy adjusting nprobe, an ideal balance between accuracy and speed can be found for a given scenario. Results from our IVF_FLAT performance test demonstrate that query time increases sharply as both the number of target input vectors (nq), and the number of clusters to search (nprobe), increase. IVF_FLAT does not compress vector data however, index files include metadata that marginally increases storage requirements compared to the raw non-indexed vector dataset.\n\n#### IVF_FLAT performance test results:\n\nIVF_FLAT query time performance testing was conducted in Milvus using the public 1B SIFT dataset, which contains 1 billion 128-dimensional vectors.\n\n\n\n#### Key takeaways:\n- When running on CPU, query time for the IVF_FLAT index in Milvus increases with both nprobe and nq. This means the more input vectors a query contains, or the more clusters a query searches, the longer query time will be.\n- On GPU, the index shows less time variance against changes in nq and nprobe. This is because the index data is large, and copying data from CPU memory to GPU memory accounts for the majority of total query time.\n- In all scenarios, except when nq = 1,000 and nprobe = 32, the IVF_FLAT index is more efficient when running on CPU.\n\nIVF_FLAT recall performance testing was conducted in Milvus using both the public 1M SIFT dataset, which contains 1 million 128-dimensional vectors, and the glove-200-angular dataset, which contains 1+ million 200-dimensional vectors, for index building (nlist = 16,384).\n\n\n\n#### Key takeaways:\n\n- The IVF_FLAT index can be optimized for accuracy, achieving a recall rate above 0.99 on the 1M SIFT dataset when nprobe = 256.\n\n\n### IVF_SQ8: Faster and less resource hungry than IVF_FLAT, but also less accurate.\n\nIVF_FLAT does not perform any compression, so the index files it produces are roughly the same size as the original, raw non-indexed vector data. For example, if the original 1B SIFT dataset is 476 GB, its IVF_FLAT index files will be slightly larger (~470 GB). Loading all the index files into memory will consume 470 GB of storage.\n\nWhen disk, CPU, or GPU memory resources are limited, IVF_SQ8 is a better option than IVF_FLAT. This index type can convert each FLOAT (4 bytes) to UINT8 (1 byte) by performing scalar quantization. This reduces disk, CPU, and GPU memory consumption by 70–75%. For the 1B SIFT dataset, the IVF_SQ8 index files require just 140 GB of storage.\n\n#### IVF_SQ8 performance test results:\n\nIVF_SQ8 query time testing was conducted in Milvus using the public 1B SIFT dataset, which contains 1 billion 128-dimensional vectors, for index building.\n\n\n\n\n#### Key takeaways:\n\n- By reducing index file size, IVF_SQ8 offers marked performance improvements over IVF_FLAT. IVF_SQ8 follows a similar performance curve to IVF_FLAT, with query time increasing with nq and nprobe.\n- Similar to IVF_FLAT, IVF_SQ8 sees faster performance when running on CPU and when nq and nprobe are smaller.\n\nIVF_SQ8 recall performance testing was conducted in Milvus using both the public 1M SIFT dataset, which contains 1 million 128-dimensional vectors, and the glove-200-angular dataset, which contains 1+ million 200-dimensional vectors, for index building (nlist = 16,384).\n\n\n\n#### Key takeaways:\n\n- Despite compressing the original data, IVF_SQ8 does not see a significant decrease in query accuracy. Across various nprobe settings, IVF_SQ8 has at most a 1% lower recall rate than IVF_FLAT.\n\n### IVF_SQ8H: New hybrid GPU/CPU approach that is even faster than IVF_SQ8.\n\nIVF_SQ8H is a new index type that improves query performance compared to IVF_SQ8. When an IVF_SQ8 index running on CPU is queried, most of the total query time is spent finding nprobe clusters that are nearest to the target input vector. To reduce query time, IVF_SQ8 copies the data for coarse quantizer operations, which is smaller than the index files, to GPU memory — greatly accelerating coarse quantizer operations. Then gpu_search_threshold determines which device runs the query. When nq \u003e= gpu_search_threshold, GPU runs the query; otherwise, CPU runs the query.\n\nIVF_SQ8H is a hybrid index type that requires the CPU and GPU to work together. It can only be used with GPU-enabled Milvus.\n\n#### IVF_SQ8H performance test results:\n\nIVF_SQ8H query time performance testing was conducted in Milvus using the public 1B SIFT dataset, which contains 1 billion 128-dimensional vectors, for index building.\n\n\n\n#### Key takeaways:\n\n- When nq is less than or equal to 1,000, IVF_SQ8H sees query times nearly twice as fast as IVFSQ8.\n- When nq = 2000, query times for IVFSQ8H and IVF_SQ8 are the same. However, if the gpu_search_threshold parameter is lower than 2000, IVF_SQ8H will outperform IVF_SQ8.\n- IVF_SQ8H’s query recall rate is identical to IVF_SQ8’s, meaning less query time is achieved with no loss in search accuracy.\n\n### Learn more about Milvus, a massive-scale vector data management platform.\n\nMilvus is a vector data management platform that can power similarity search applications in fields spanning artificial intelligence, deep learning, traditional vector calculations, and more. For additional information about Milvus, check out the following resources:\n\n- Milvus is available under an open-source license on [GitHub](https://github.com/milvus-io/milvus).\n- Additional index types, including graph- and tree-based indexes, are supported in Milvus. For a comprehensive list of supported index types, see [documentation for vector indexes](https://milvus.io/docs/v0.11.0/index.md) in Milvus.\n- To learn more about the company that launched Milvus, visit [Zilliz.com](https://zilliz.com/).\n- Chat with the Milvus community or get help with a problem on [Slack](https://join.slack.com/t/milvusio/shared_invite/zt-e0u4qu3k-bI2GDNys3ZqX1YCJ9OM~GQ).\n\n### Methodology\n\n#### Performance testing environment\n\nThe server configuration used across performance tests referenced in this article is as follows:\n\n- Intel (R) Xeon (R) Platinum 8163 @ 2.50GHz, 24 cores\n- GeForce GTX 2080Ti x 4\n- 768 GB memory\n\n#### Relevant technical concepts\n\nAlthough not required for understanding this article, here are a few technical concepts that are helpful for interpreting the results from our index performance tests:\n\n\n\n#### Resources\n\nThe following sources were used for this article:\n\n- “[Encyclopedia of database systems](https://books.google.com/books/about/Encyclopedia_of_Database_Systems.html?id=YdT3wQEACAAJ),” Ling Liu and M. Tamer Özsu.\n\n\n\n\n\n\n ","title":"Accelerating Similarity Search on Really Big Data with Vector Indexing","metaData":{}},{"id":"2019-11-08-data-management.md","author":"Yihua Mo","desc":"This post introduces the data management strategy in Milvus.","origin":null,"date":"2019-11-08T00:00:00.000Z","cover":"https://zilliz-cms.s3.us-west-2.amazonaws.com/pc_blog_8ed7696269.jpg","tags":["Engineering"],"href":"/blog/2019-11-08-data-management.md","content":"\n# Managing Data in Massive-Scale Vector Search Engine\n\n\u003e Author: Yihua Mo\n\u003e\n\u003e Date: 2019-11-08\n\n## How data management is done in Milvus\n\nFirst of all, some basic concepts of Milvus:\n\n- Table: Table is a data set of vectors, with each vector having a unique ID. Each vector and its ID represent a row of the table. All vectors in a table must have the same dimensions. Below is an example of a table with 10-dimensional vectors:\n\n\n\n- Index: Building index is the process of vector clustering by certain algorithm, which requires additional disk space. Some index types require less space since they simplify and compress vectors, while some other types require more space than raw vectors.\n\nIn Milvus, users can perform tasks such as creating a table, inserting vectors, building indexes, searching vectors, retrieving table information, dropping tables, removing partial data in a table and removing indexes, etc.\n\nAssume we have 100 million 512-dimensional vectors, and need to insert and manage them in Milvus for efficient vector search.\n\n**(1) Vector Insert**\n\nLet’s take a look at how vectors are inserted into Milvus.\n\nAs each vector takes 2 KB space, the minimum storage space for 100 million vectors is about 200 GB, which makes one-time insertion of all these vectors unrealistic. There need to be multiple data files instead of one. Insertion performance is one of the key performance indicators. Milvus supports one-time insertion of hundreds or even tens of thousands of vectors. For example, one-time insertion of 30 thousand 512-dimensional vectors generally takes only 1 second.\n\n\n\nNot every vector insertion is loaded into disk. Milvus reserves a mutable buffer in the CPU memory for every table that is created, where inserted data can be quickly written to. And as the data in the mutable buffer reaches a certain size, this space will be labeled as immutable. In the mean time, a new mutable buffer will be reserved. Data in immutable buffer are written to disk regularly and corresponding CPU memory is freed up. The regular writing to disk mechanism is similar to the one used in Elasticsearch, which writes buffered data to disk every 1 second. In addition, users that are familiar with LevelDB/RocksDB can see some resemblance to MemTable here.\n\nThe goals of the Data Insert mechanism are:\n\n- Data insertion must be efficient.\n- Inserted data can be used instantly.\n- Data files should not be too fragmented.\n\n**(2) Raw Data File**\n\nWhen vectors are written to disk, they are saved in Raw Data File containing the raw vectors. As mentioned before, massive-scale vectors need to be saved and managed in multiple data files. Inserted data size varies as users can insert 10 vectors, or 1 million vectors at one time. However, the operation of writing to disk is executed once every 1 second. Thus data files of different sizes are generated.\n\nFragmented data files are neither convenient to manage nor easy to access for vector search. Milvus constantly merges these small data files until the merged file size reaches a particular size, for example, 1GB. This particular size can be configured in the API parameter `index_file_size` in table creation. Therefore, 100 million 512-dimensional vectors will be distributed and saved in about 200 data files.\n\nIn consideration to incremental computation scenarios, where vectors are inserted and searched concurrently, we need to make sure that once vectors are written to disk, they are available for search. Thus, before the small data files are merged, they can be accessed and searched. Once the merge is completed, the small data files will be removed, and newly merged files will be used for search instead.\n\nThis is how queried files look before the merge:\n\n\n\nQueried files after the merge:\n\n\n\n**(3) Index File**\n\nThe search based on Raw Data File is brute-force search which compares the distances between query vectors and origin vectors, and computes the nearest k vectors. Brute-force search is inefficient. Search efficiency can be greatly increased if the search is based on Index File where vectors are indexed. Building index requires additional disk space and is usually time-consuming.\n\nSo what are the differences between Raw Data Files and Index Files? To put it simple, Raw Data File records every single vector together with their unique ID while Index File records vector clustering results such as index type, cluster centroids, and vectors in each cluster.\n\n\n\nGenerally speaking, Index File contains more information than Raw Data File, yet the file sizes are much smaller as vectors are simplified and quantized during the index building process (for certain index types).\n\nNewly created tables are by default searched by brute-computation. Once the index is created in the system, Milvus will automatically build index for merged files that reach the size of 1 GB in a standalone thread. When the index building is completed, a new Index File is generated. The raw data files will be archived for index building based on other index types.\n\nMilvus automatically build index for files that reach 1 GB:\n\n\n\nIndex building completed:\n\n\n\nIndex will not be automatically built for raw data files that do not reach 1 GB, which may slow down the search speed. To avoid this situation, you need to manually force build index for this table.\n\n\n\nAfter index is force built for the file, the search performance is greatly enhanced.\n\n\n\n**(4) Meta Data**\n\nAs mentioned earlier, 100 million 512-dimensional vectors are saved in 200 disk files. When index is built for these vectors, there would be 200 additional index files, which makes the total number of files to 400 (including both disk files and index files). An efficient mechanism is required to manage the meta data (file statuses and other information) of these files in order to check the file statuses, remove or create files.\n\nUsing OLTP databases to manage these information is a good choice. Standalone Milvus uses SQLite to manage meta data while in distributed deployment, Milvus uses MySQL. When Milvus server starts, 2 tables (namely ‘Tables’ and ‘TableFiles’) are created in SQLite/MySQL respectively. ‘Tables’ records table information and ‘TableFiles’ records information of data files and index files.\n\nAs demonstrated in below flowchart, ‘Tables’ contains meta data information such as table name (table_id), vector dimension (dimension), table creation date (created_on), table status (state), index type (engine_type), and number of vector clusters (nlist) and distance computation method (metric_type).\n\nAnd ‘TableFiles’ contains name of the table the file belongs to (table_id), index type of the file (engine_type), file name (file_id), file type (file_type), file size (file_size), number of rows (row_count) and file creation date (created_on).\n\n\n\nWith these meta data, various operations can be executed. The following are some examples:\n\n- To create a table, Meta Manager only needs to execute a SQL statement: `INSERT INTO TABLES VALUES(1, 'table_2, 512, xxx, xxx, ...)`.\n- To execute vector search on table_2, Meta Manager will execute a query in SQLite/MySQL, which is a de facto SQL statement: `SELECT * FROM TableFiles WHERE table_id='table_2'` to retrieve the file information of table_2. Then these files will be loaded into memory by Query Scheduler for search computation.\n- It is not allowed to instantly delete a table as there might be queries being executed on it. That’s why there are soft-delete and hard-delete for a table. When you delete a table, it will be labeled as ‘soft-delete’, and no further querying or changes are allowed to be made to it. However, the queries that were running before the deletion is still going on. Only when all these pre-deletion queries are completed, the table, together with its meta data and related files, will be hard-deleted for good.\n\n**(5) Query Scheduler**\n\nBelow chart demonstrates the vector search process in both CPU and GPU by querying files (raw data files and index files) which are copied and saved in disk, CPU memory and GPU memory for the topk most similar vectors.\n\n\n\nQuery scheduling algorithm significantly improves system performance. The basic design philosophy is to achieve the best search performance through maximum utilization of hardware resources. Below is just a brief description of query scheduler and there will be a dedicated article about this topic in the future.\n\nWe call the first query against a given table the ‘cold’ query, and subsequent queries the ‘warm’ query. When the first query is made against a given table, Milvus does a lot of work to load data into CPU memory, and some data into GPU memory, which is time-consuming. In further queries, the search is much faster as partial or all the data are already in CPU memory which saves the time to read from the disk.\n\nTo shorten the search time of the first query, Milvus provides Preload Table (`preload_table`) configuration which enables automatic pre-loading of tables into CPU memory upon server start-up. For a table containing 100 million 512-dimensional vectors, which is 200 GB, the search speed is the fastest if there's enough CPU memory to store all these data. However, if the table contains billion-scale vectors, it is sometimes inevitable to free up CPU/GPU memory to add new data that are not queried. Currently, we use LRU (Latest Recently Used) as the data replacement strategy.\n\nAs shown in below chart, assume there is a table that has 6 index files stored on the disk. The CPU memory can only store 3 index files, and GPU memory only 1 index file.\n\nWhen the search starts, 3 index files are loaded into CPU memory for query. The first file will be released from CPU memory immediately after it is queried. Meanwhile, the 4th file is loaded into CPU memory. In the same way, when a file is queried in GPU memory, it will be instantly released and replaced with a new file.\n\nQuery scheduler mainly handles 2 sets of task queues, one queue is about data loading and another is about search execution.\n\n\n\n**(6) Result Reducer**\n\nThere are 2 key parameters related to vector search: one is ’n’ which means n number of target vectors; another is ‘k’ meaning the top k most similar vectors. The search results are actually n sets of KVP (key-value pairs), each having k pairs of key-value. As queries need to be executed against each individual file, no matter it is raw data file or index file, n sets of top-k result sets will be retrieved for each file. All these result sets are merged to get the top-k result sets of the table.\n\nBelow example shows how result sets are merged and reduced for the vector search against a table with 4 index files (n=2, k=3). Note that each result set has 2 columns. The left column represents the vector id and the right column represents the Euclidean distance.\n\n\n\n**(7) Future Optimization**\n\nThe following are some thoughts on possible optimizations of data management.\n\n- What if the data in immutable buffer or even mutable buffer can also be instantly queried? Currently, the data in immutable buffer cannot be queried, not until they are written to disk. Some users are more interested in instantaneous access of data after insertion.\n- Provide table partitioning functionality that allows user to divide very large tables into smaller partitions, and execute vector search against a given partition.\n- Add to vectors some attributes which can be filtered. For example, some users only want to search among the vectors with certain attributes. It is required to retrieve vector attributes and even raw vectors. One possible approach is to use a KV database such as RocksDB.\n- Provide data migration functionality that enables automatic migration of outdated data to other storage space. For some scenarios where data flows in all the time, data might be aging. As some users only care about and execute searches against the data of the most recent month, the older data become less useful yet they consume much disk space. A data migration mechanism helps free up disk space for new data.\n\n## Summary\n\nThis article mainly introduces the data management strategy in Milvus. More articles about Milvus distributed deployment, selection of vector indexing methods and query scheduler will be coming soon. Stay tuned!\n\n## Related blogs\n\n- [Milvus Metadata Management (1): How to View Metadata](https://medium.com/@milvusio/milvus-metadata-management-1-6b9e05c06fb0)\n- [Milvus Metadata Management (2): Fields in the Metadata Table](https://medium.com/@milvusio/milvus-metadata-management-2-fields-in-the-metadata-table-3bf0d296ca6d)\n","title":"Managing Data in Massive-Scale Vector Search Engine","metaData":{}}]},"__N_SSG":true},"page":"/blog","query":{},"buildId":"SWuVyeBuFzTkSMeqLwUio","isFallback":false,"gsp":true,"scriptLoader":[]}</script></body></html>